- 1Institut National de la Recherche Scientifique, Centre Eau Terre Environnement, Quebec City, QC, Canada
- 2Department of Soil and Agricultural Engineering, Laval University, Quebec City, QC, Canada
- 3Institut National de la Recherche Scientifique, Institut Armand-Frappier Research Centre, Laval, QC, Canada
In agriculture, water consumption for crop production represents 69% of all water use. Precision irrigation systems based on available soil water have been developed to improve crop production, reducing water use. Besides the improvements in irrigation management, a better resolution of the optimal water level is required, and revealing the impact of soil matric potential on the soil microbial community may help. Here, the effect of four soil matric potential treatments (−15 kPa, −25 kPa, −30 kPa, and −45 kPa) was evaluated on the soil microbial community across three potato cultivars and two soil types (silt and sand). The results confirmed the soil matric potential with −25 kPa as the optimal irrigation level, which promoted high potato production using the minimal water use. The irrigation levels affected the composition, predicted functionality, and the ecological network of soil bacterial community. Water excess (−15 kPa) and deficit (−30 and −45 kPa) promoted an increase in microbial interaction and alpha-diversity. The results suggested higher Positive/Negative ratio for phyla Proteobacteria, Actinobacteria, and Acidobacteria in optimal irrigation level (−25 kPa) than other irrigation levels. Also, correlation analyses revealed an interesting association between the irrigation levels, potato production, and bacterial functionality, especially in the carbon cycle (fixation and emission). Therefore, this study proposed important associations between the soil bacterial community and water management, focusing on high potato production and minimal water use. The advancement of this knowledge may lead to a more comprehensive assessment of the optimal irrigation levels of others crop production systems. Furthermore, the inclusion of biological mechanisms, especially microbial interactions, in agriculture studies has the potential to contribute to the development of water management practices conducive to both increasing crop yield and maintaining a sustainable soil environment.
Introduction
Reducing water consumption is one of the most critical challenges facing the twenty-first century (Cosgrove and Loucks, 2015). Based on the parameters of population growth, economic growth, consumption patterns (diet, bio-energy, and demand), global production and trade pattern, and technological development, it has been estimated that in 2050, the population in the world will be around 8–10 billion, increasing by 180% agricultural water use in the worst case scenario (Ercin and Hoekstra, 2014). Given that agriculture is responsible for 69% of the global water use, this will have a significant impact on the world's water resources (Frenken and Gillet, 2012). Thus, there are remarkable ongoing efforts to improve irrigation strategies while maintaining food production and reducing adverse environmental impact (BajŽelj et al., 2014). Accordingly, accurate methods have been developed to monitor water in the soil system. The adsorbed soil water content can be characterized by the total potential (i.e., energy per unit volume [Pa]), or ΨT (Groenevelt et al., 2004) which corresponds to the sum of four potentials: matric potential (Ψm); osmotic, or solute potential (ΨS); the gravitational potential (ΨZ); and pressure potential (ΨA), where only the matric and solute potentials affect plant absorbance (Kirkham, 2005). Thus, the optimal irrigation level for different crops is mostly assumed and based on the water matric potential (Caron et al., 2017).
Although current irrigation strategies have considerably improved crop production, methods to improve their efficiency are strongly desirable. One possible solution is to integrate soil water management with other environmental components, such as the soil microbial community. The soil has been classified as one of the most diverse ecosystems on Earth (Thompson et al., 2017). It has been verified that the microbial community plays an essential role in the soil environment system; that is acting on the biogeochemical cycles, such as those of nitrogen (Prosser and Nicol, 2008), sulfur (Zecchin et al., 2017), and carbon (Meyer et al., 2017). The soil microbial community also plays significant roles in plant nutrition. Castrillo et al. (2017) demonstrated that there is a direct correlation between the rhizosphere microbial community, the phosphorus uptake by plants, and the plant immunity to nutritional-stress events. Moreover, it has been suggested there is a correlation between the quality of irrigation water and the soil microbial functionality and stability (Frenk et al., 2018); however, the effect of water management on the soil microbial community remains unclear.
The available soil water may drive the structural, functionality and interaction of the soil microbial community, and this correlation may improve the use of water by plants. In this study, the effect of four targeted matric potential levels (−15, −25, −30, and −45 kPa) on the soil microbial community (composition, predicted functionality, and microbial interaction) was explored using three potato cultivars and two soil types (silt and sand). Potatoes, which are sensitive to dry soil conditions (Dalla Costa et al., 1997) and saturated soil conditions (Jackson and Colmer, 2005), require timely irrigation and thus, there is a requirement to assess a potential correlation between soil matric potential, soil microbial community, and crop production. In particular, the hypothesis tested was that, despite soil type and potato cultivars, (i) the water management affects soil bacterial structure, functionality, and co-occurrence patterns; (ii) the soil bacterial co-occurrence patterns and functionality have a potential association with potato yields; and (iii) the irrigation level which promotes the best potato yields is associated with the highest soil microbial diversity. This research represents a stepping stone toward the integration of microbial community dynamics into the development of comprehensive irrigation strategies.
Materials and Methods
Soil Preparation and Characterization
The soils were obtained from potato fields located at Peribonka city (silt soil; 48°45′37.07″N and 72°1′24.84″W), and Dolbeau-Mistassini city (sandy soil; 48°51′31.04″N and 72°11'50.49″W). Both soils were collected at 20 cm topsoil, homogenized and placed into the pots (not sieved). The soils were classified as Sandy soil (0% clay, 8.16% silt, 91.84% sand, 3.13% Corg, and pH 5.01) and Silt loam soil (0.08% clay, 58.16% silt, 41.76% sand, 2.73% Corg, and pH 5.35), considering the USDA Soil Taxonomy (Soil Survey Staff, 1999). The retention curve of each soil is available at Figure S1. The retention curve correlates the soil matric potential values and the water content of each soil, indicating their, respectively, Field Capacity (0.0938 m3/m3 for sand soil, and 0.2735 m3/m3 for silt soil) and Wilting point (0.053 m3/m3 for sandy soil, and 0.065 m3/m3 for silt soil). The water retention curve was obtained by the van Genuchten equation (van Genuchten, 1980),
Where, θr is the saturated water content (m3 m−3), θs is the residual water content (m3 m−3), α is related to the inverse of air entry suction (m−1), ψ is the soil matric potential in module, and n is the pore-size distribution.
Irrigation Experiment
The experimental work was conducted in a greenhouse located at the Laval University campus (Québec, QC, Canada). Potatoes were cultivated in 72 pots (60 × 40 × 60 cm), using three replicates of four soil matric potential, two types of soils and three potato cultivars. The water management treatment was classified into different levels of soil water matric potential mean, namely and ~ −15, −25, −30, and −45 kPa. The levels of soil water matric potential were choose based on the previous study focused on irrigation levels and potato production (see Wang et al., 2007). For sand soil the matric potential levels corresponded to 0.0846 (−15 kPa), 0.0757 (−25 kPa), 0.0731 (−30 kPa), and 0.0685 (−45 kPa) m3/m3 of water content. For silt soil the matric potential levels corresponded to 0.2549 (−15 kPa), 0.2328 (−25 kPa), 0.2256 (−30 kPa), and 0.2104 (−45 kPa) m3/m3 of water content. Thus, the irrigation level of −25 kPa showed 11% (sand soil) and 9% (silt soil) less water content than the irrigation level of −15 kPa.
Eight irrigation supply lines allowed to control irrigation at the subplot level independently. The soil matric potential at a depth of 15 cm was measured at 2-min intervals with a datalogger, and the sub-plot level mean values (3 reps × 3 varieties) were calculated. Irrigation was manually initiated when the predefined thresholds were reached, and irrigation durations were adjusted throughout the growing season to avoid leaching following the procedure described in Létourneau et al. (2015). The three potato cultivars used were Goldrush (most cultivated mid-season variety in Quebec), Envol (popular early variety), and Red Maria (mid-late to late variety), which are expected to show similar physiological response across irrigation variance (FPPTQ, 2016). After the development of potato plants (30 days), the water experiment went on for 90 days, until harvest. During the experiment, it was used rainfall water (pH ≈ 7 and EC ≈ 180 μS cm−1) for irrigation, and the mean temperature in greenhouse was 24°C. No fertilizer was coupled into the water supply. Potato tuber number and fresh biomass were measured for each pot. The soil samples were taken from each pot (0–10 cm depth) at the end of the experimental period for downstream elemental concentrations analyses by X-ray fluorescence spectrometer, available nutrients analyses by Mehlich III and DNA extraction.
DNA Extraction and 16S Sequencing
For total DNA extraction and 16S sequencing, we followed the protocol available from the Earth Microbiome Project (EMP; www.earthmicrobiome.org). The total DNA was obtained from 0.25 g of soil using the PowerSoil DNA Isolation kit (MoBio, Carlsbad, USA) according to the manufacturer's instructions. Quality of extracted DNA was visualized by electrophoresis at 0.8 % agarose gel. The sequence of prokaryotes (bacteria and archaea) were obtained by the primers 515F-806R, targeting the V3–V4 region of the 16S SSU rRNA (Caporaso et al., 2011). The DNA library was performed using the Nextera XT Library Prep kit® with sequences of 300 base pairs. The products were quantified using the Quant-iT PicoGreen dsDNA Assay Kit® (ThermoFisher/Invitrogen cat. no. P11496; follow manufacturer's instructions) for equimolar pool tuning and sequenced using the MiSeq PE Cluster v3 cBot kit® (2 × 300 bp) on the Illumina MiSeq2500® platform (IRDA, Quebec, Canada). A total of 5.2 million sequences were obtained. The bioinformatics analyses were performed in Qiime (Caporaso et al., 2010), following the protocols for Illumina 16S analyses available at http://qiime.org. The reads were joined into paired-end sequences, and filtered by the quality and chimeric sequences. The sequences filtered were binned into operational taxonomic units (OTU) using Sumaclust method at 97% identity (Kopylova et al., 2016). The taxonomic classification was based on SILVAS's ribosomal database-123 (Quast et al., 2013). We removed the singleton and Archaea sequences. The OTU table was rarefied at 54,535 sequences/sample depth.
Statistical Analyses
The statistical analyses were performed in R.3.4.3 (Sasaki et al., 2005). Firstly, we confirmed the differences in irrigation levels (−15, −25, −30, and −45 kPa) by ANOVA-test followed by Tukey test (confidential level of 95%) using package 'laercio'. We evaluated the elemental (X-ray) and chemical (Mehlich III) composition of soil types (silt and sand) by principal component analysis (PCA) after the irrigation experiment. We standardized the soil types composition data by Hellinger, and the PCA was performed using the packages “FactoMineR” and “factoextra.” The results of potato experiment, such as potato production (Kg/pot), potato tuber number (unit/pot), and relative mass per potato tuber (Kg/unit), were compared by ANOVA-test and ranked by Tukey test, considering p < 0.05). The coefficient of variation (CV) was lower than 32% indicating no transformation necessity (Box and Cox, 1964).
We performed the bacterial functionality prediction using the method and package ‘Tax4Fun’ (Aßhauer et al., 2015). The Tax4fun produced the KO table (KO codes per samples), which we standardized by percentage of row. Using the package “PathView” and KO table, we evaluated the relative abundance of each gene predicted across nitrogen metabolism (KEGG map 00910), sulfur metabolism (KEGG map 00920), methane metabolism (KEGG map 00680), and carbon fixation pathways in prokaryotes (KEEG map 00720). The microbial association network using the package “SpiecEasi” (Kurtz et al., 2015). Microbial co-occurrence patterns were inferred using the covariance selection method and Stability Approach to Regularization Selection (StARS) as bootstrap. The microbial association network was based on the minimum occurrence of 40% of treatment samples, as indicated by Faust and Raes (2012). The SPIEC-EASI package produced the nodes and edges list, which were used in Gephi 0.9.2 (Bastian et al., 2009) to build the network plots. The topological properties of each network analysis, such as the number of interactions, the minimum distance between nodes (Newman, 2003), modularity (Newman, 2006), and Positive/Negative ratio (Ma, 2018), were performed using the package “igraph” (Csardi and Nepusz, 2006). Positive/Negative ratio (P/N) is obtained by the Equation (2), where P/N higher than 1 indicates low competition, and lower than 1 indicates high competition of microbial community (Ma, 2018).
The first step of correlation analyses between irrigation levels, soil type and potato variety on the total structure of the soil bacterial community (OTU table) were performed using ‘veganș package. Here, we used permutation MANOVA (PERMANOVA; Anderson, 2017) with the three-way correlation formula,
These correlations were performed using “Bray-Curtis” as a microbial distance (dist), 9999 permutations, and no constrain permutation groups. The second step of PERMANOVA correlations was performed using the formula using two-way factorial design,
Here, we used 'Bray-Curtis' as the microbial distance (dist), 9999 permutations. These correlations aimed to verified one-way correlations such as microbial tables related with potato experiment results (production and tuber number), and also two-ways correlations such as microbial tables related with irrigation levels × potato production. At this second step, the objective of the analysis was more focused on the microbial community. Thus, soil types were used as a constraint permutation group. The microbial tables used were OTU table (complete, and filtered by phyla and class), KO table (obtained from Tax4fun method), and co-occurrence nodes (OTU table filtered by nodes with interaction in network analysis). The alpha diversity was evaluated by Shannon index (H; equation 5), which were compared by ANOVA-test and ranked by Tukey test (confidence level of 95%)
The Shapiro-Wilk normality test was performed using package ‘veganș (Oksanen et al., 2013). Normal distribution of alpha diversity values was obtained after interquartile range transformation.
Results
The Irrigation Affected the Total Potato Production
The values of soil matric potentials were confirmed around −15, −25, −30, and −45 kPa for the irrigation levels A, B, C, and D, respectively (Figure 1A), showing a significant difference (ANOVA, p-value < 0.05). The elemental composition and available nutrients of each soil type are indicated in Figure 2 and Table 1, respectively. In general, the two soil types showed significant variances in the elemental composition, and it was not observed differences in the soil matric potentials between soil types, as expected (Figure 1A). In Figure 1B, we observed that the total potato production (Kg/pot) was affected by the irrigation levels. While the soil matric potentials of −15 (44.27 t/ha) and −25 kPa (44.95 t/ha) presented the highest potato mean yield, treatments with −30 (30.72 t/ha) and −45 kPa (12.21 t/ha) showed a significant reduction in the potato mean yield (ANOVA, p < 0.05). Furthermore, it was not observed a significant difference in potato production (ANOVA, p-value > 0.05) for soil types and potato cultivars (Figures S2A,C).
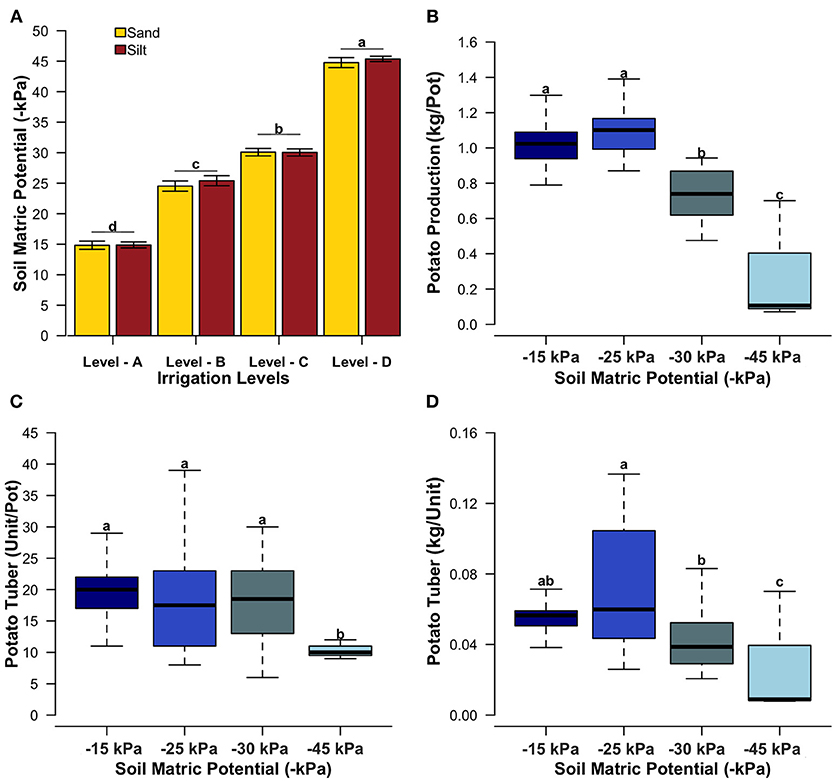
Figure 1. Bar plot of soil matric potential for each irrigation level (−15, −25, −30, and −45 kPa) across two types of soil (A). Boxplot of Potato Production (Kg/pot) (B), Potato tuber number (Unit/pot) (C) and relative mass of Potato tuber (Kg/unit) (D) for each soil matric potential. The averages were compared by Tukey test (considering F-test with p-value < 0.05) and they are indicated by the small letters.
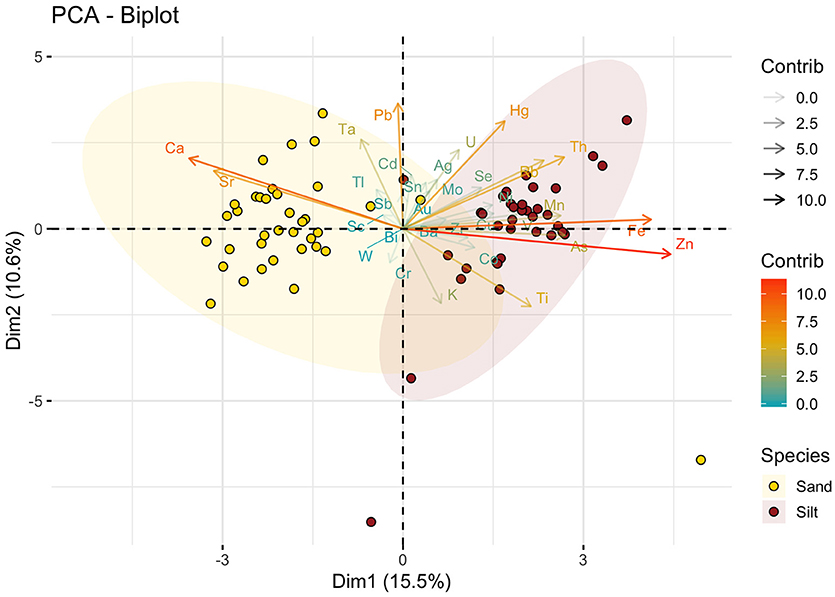
Figure 2. Principal component analyses of soil physics composition from X-ray system. The sand soils are indicated by yellow points and silt are indicated by brown points.
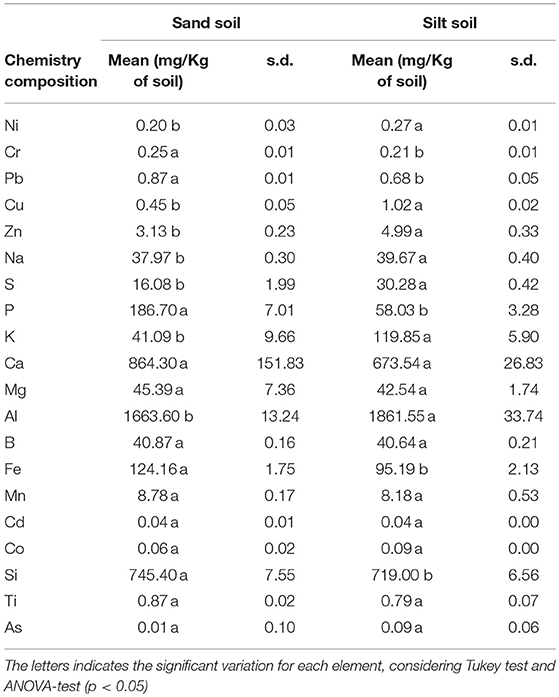
Table 1. Averages and standard deviation (s.d.) of the soil chemical composition for silt and sand soils.
The number of potato tuber per pot was similar across the soil matric potentials −15, −25, and −30 kPa, showing significant reduction only for −45 kPa (Figure 1C). As indicated in Figures S3B,D, the number of potato tuber was significantly different across the soil type (sand > silt), and potato cultivars (Goldrush > Red Maria > Envol). We also verified the relative mass per potato tuber (Kg/unit) which showed a significant variance across the soil matric potential (Figure 1D), with highest values for −25 kPa. The irrigation level with −15 kPa showed an intermediate average of relative mass per potato tuber between the irrigation levels −25 and −30 kPa.
Taxonomic Composition, Predicted Functionality, and Microbial Interactions Across Irrigation Levels
In general, the irrigation levels affected the taxonomic composition of the soil bacterial community. In Figure 3, there is a clear trend of decreasing the relative abundance of phyla Actinobacteria and Proteobacteria from −15 to −45 kPa. Also in general, it was observed a trend of increasing of phyla Acidobacteria, Firmicutes, and Chloroflexi from −15 to −45 kPa. Retrieved OTU were classified into 704 bacterial genera across the soil samples (Table S1). We observed that Bacillus (average of 4.9%), Arthrobacter (average of 4.5%), Streptomyces (average of 4.3%), Bradyrhizobium (average of 3.3%), and Variibacter (average of 3.2%) were the five most abundant genera classified. In general, we observed an effect of the irrigation levels on the genera composition, such as Bacillus (increasing pattern) and Arthrobacter (decrease pattern) from −15 to −45 kPa. Also, we verified the soil bacterial alpha diversity (by Shannon index). As the values of the alpha diversity from −45 kPa did not follow a normal distribution (Shapiro–Wilk test, p < 0.01) we transformed the data by interquartile range. The alpha diversity indicated unexpected results for each irrigation level (Figure 4). The irrigation levels with higher potato production (−15 and −25 kPa) showed the lowest alpha diversity. On the other hand, irrigation levels of −30 and −45 kPa showed high alpha diversity.
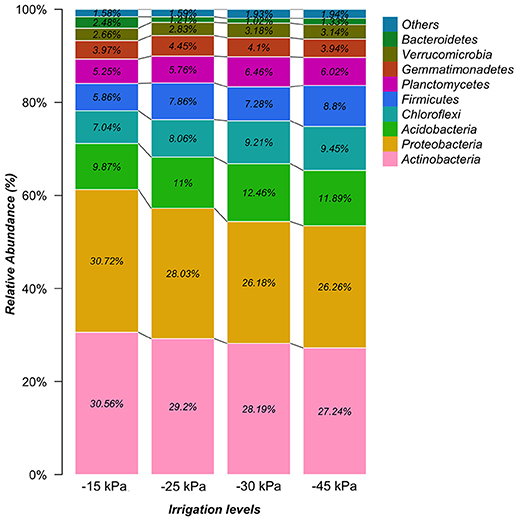
Figure 3. Relative abundance of bacterial phyla for each irrigation level (−15, −25, −30, and −45 kPa).
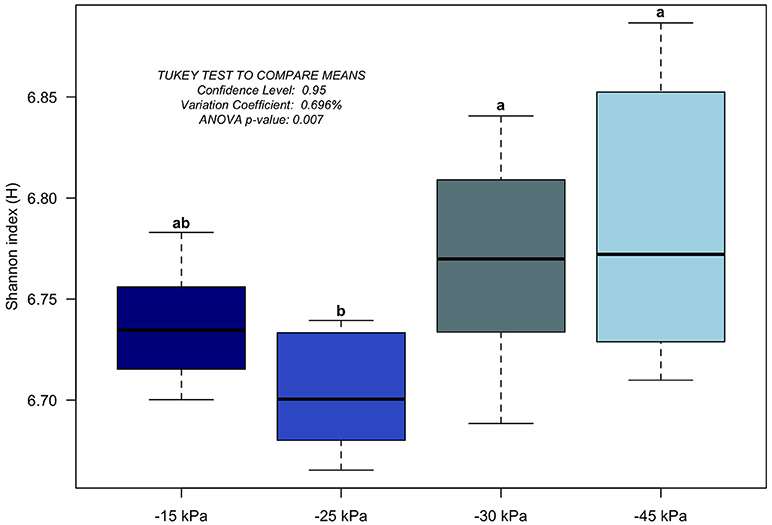
Figure 4. Boxplot of alpha-diversity by Shannon index for each irrigation level (−15, −25, −30, and −45 kPa). The averages were compared by ANOVA and Tukey test (considering p-value < 0.05) and they are indicated by the small letters.
We tested four metabolic paths, those of nitrogen, sulfur, methane, and carbon fixation for prokaryotes, aiming to verify the impacts of irrigation levels on the potential functionality of soil bacterial community. The results obtained for each metabolic paths are indicated in the Supplemental Material (Figures S3–6). Overall, the soil matric potential affected the occurrence of predicted genes in soil. As indicated in Figure 5, more than 50% of the gene ccsB (K15233)—belonging to carbon fixation in prokaryotes—was predicted for the irrigation level −25 kPa, while no prediction was found in −45 kPa. For nitrogen metabolism, the genes amoABC (K10944, K10945, and K10946) were predicted to be less abundant in irrigation level with −45 kPa (21%) compared with the other treatments. Also for the irrigation level −45 kPa, it was observed higher prediction for genes related to the reduction process, such as 27% of the gene cysNCD (K00955 and K00957) in sulfur metabolism, and 78% of genes mtaA (K14080) and mtbB (K16178) encoding methyltransferases involved in methanogenesis.
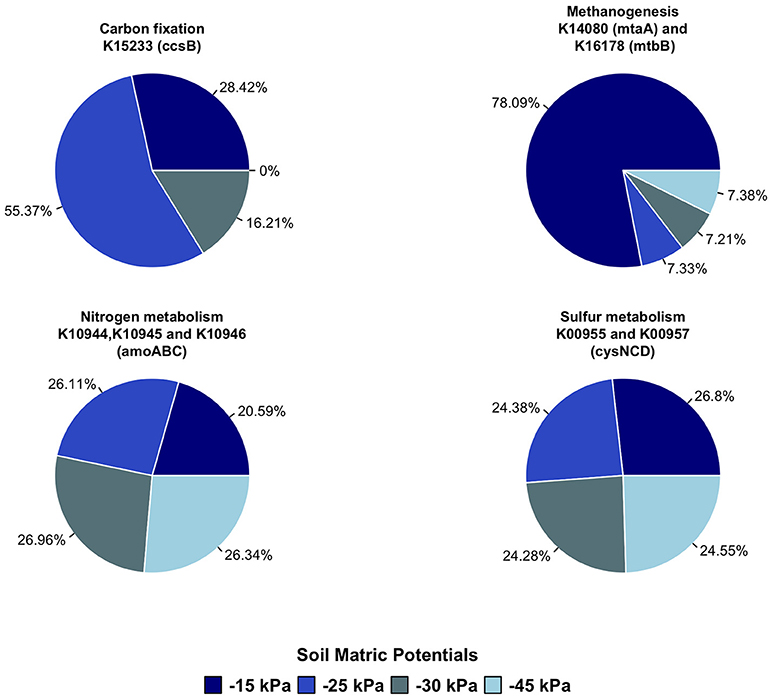
Figure 5. Relative abundance of predicted genes related with carbon fixation (K15233—ccsB), methanogenesis (K14080—mtaA; K16178—mtbB), nitrogen metabolism (K10944, K10945, and K10946—amoABC), and sulfur metabolism (K00955, and K00957—cysNCD) of each irrigation level (−15, −25, −30, and −45 kPa).
The soil microbial co-occurrence patterns also showed a significant variation across soil matric potential. The network graphs are presented in Figure 6, and their analyses are indicated in Table 2. Overall, we observed that the irrigation level with −25 kPa promoted the lowest quantity of edges specifying pairwise covariation of OTU (10,193), with lower average connections per node (average degree of 9.97). We also observed that this irrigation level showed the highest Positive/Negative ratio, with the highest minimum distance between the nodes (minimum spanning tree of 3.10), and highest modularity (0.42). On the other hand, the irrigation level with −45 kPa presented the highest number of edges (13,044). We also observed that the irrigation level with −45 kPa presented a bacterial network with the highest node connectivity (Average degree of 12.13), and lowest Positive/Negative ratio (1.62). The irrigation levels of −15 and −30 kPa showed similar ecological network topology.
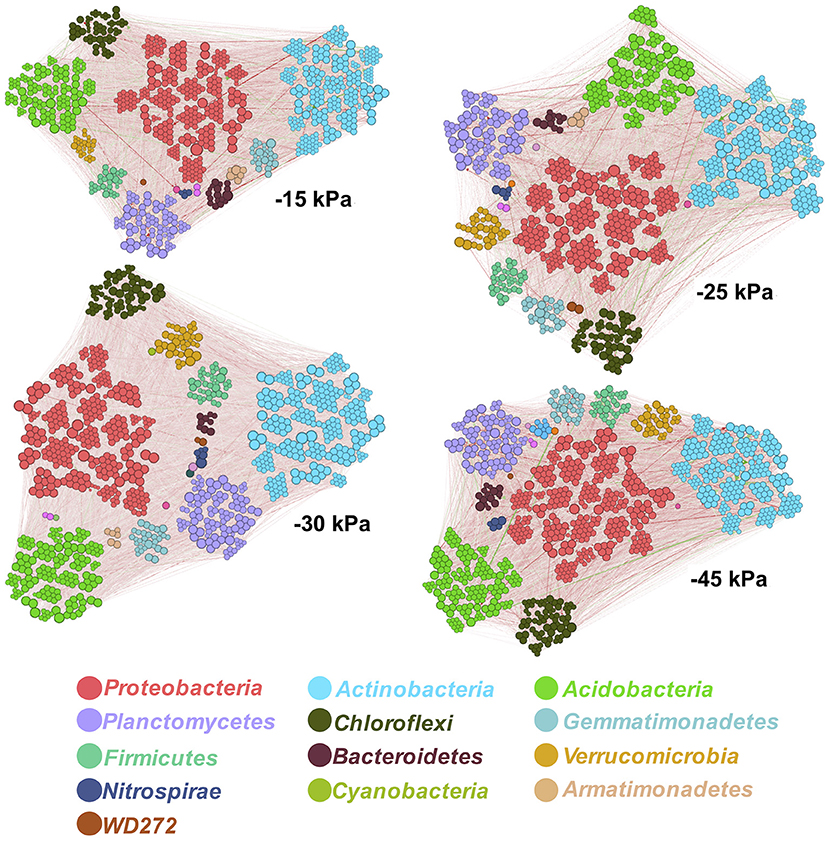
Figure 6. Network analyses based on Stability Approach to Regularization Selection (StARS) method. The color of nodes indicates the taxonomic classification at phyla level. The negative (red lines) and positive (green lines) interactions were filtered by p-value < 0.05. The size of points indicates the degree level (number of interaction per node).
We verified the composition of phyla displaying connectivity in ecological networks and also evaluated their Positive/Negative ratio across the irrigation levels (Table 3). The three phyla Proteobacteria, Actinobacteria, and Acidobacteria displaying the highest levels of connectivity showed the highest Positive/Negative ratio at irrigation level at −25 kPa. This irrigation level also showed the highest Positive/Negative ratio for Bacteroidetes (2.08) and Verrucomicrobia (2.44); and lowest Positive/Negative ratio for Armatimonadetes (1.03) and Nitrospirae (1.83). We also observed others patterns in phyla interactions, such as Firmicutes Positive/Negative ratio reduced from −15 kPa (4.59) to −45 kPa (2.22).
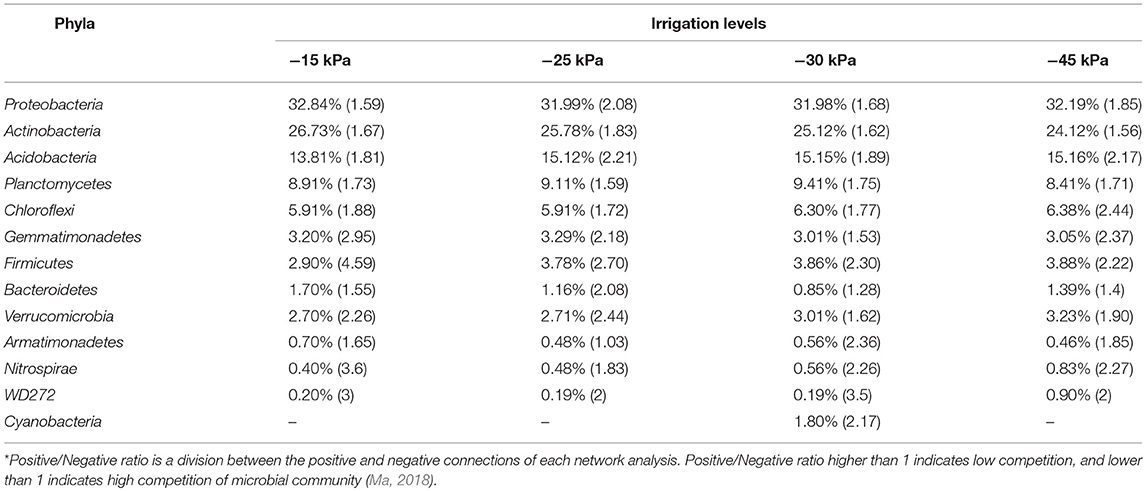
Table 3. Relative abundance (percentage) and Positive/Negative ratio* at phylum level for the nodes of each network analysis.
Correlation Between Soil Bacterial Community, Irrigation Levels, and Potato Production
The first step of correlation analyses aimed to verify the effect of irrigation levels, soil type and potato variety on the total structure of soil bacterial community. The PERMANOVA analysis indicated that soil types (sand and silt), water management treatment and potato variety showed a significant correlation with soil bacterial community. In Figure 7 we observed that the soil type explained 44.95% of the soil microbial community variation, while irrigation levels and potato cultivar explained 4.69 and 2.31%, respectively. We also observed two-way correlations, where irrigation level × soil type (3.1%), and irrigation level × potato cultivar (2.31%) were also explained by the variation in soil microbial community. The second step of analyses aimed to correlate the potato tuber number (unit/pot) and potato production (Kg/pot) with taxonomic composition (OTU table), predicted functionality (KO table), and also microbial interactions nodes (OTU table filtered by network nodes). In overall in Table 4, we observed that the potato tuber number showed a correlation with all microbial tables (taxonomic, functionality, and interaction). Besides, the correlation between potato tuber number and each microbial tables were similar, such as 15.2% for taxonomic composition, 15.6% for co-occurrence table, and 14.1% for predicted functionality table. The PERMANOVA analyses indicated that the phyla Firmicutes (20.75%), Gemmatimonadetes (25.80%), and Chloroflexi (20.75%) were the phyla with the highest correlation with potato tuber number. At the class level, Thermoleophilia (19.46%) and Bacilli (19.79%) were more correlated with potato tuber number. For the correlation between predicted functionality and potato tuber number, we verified the highest and lowest correlation in the Sulfur cycle (15.16%) than Carbon fixation (12.23%), respectively.
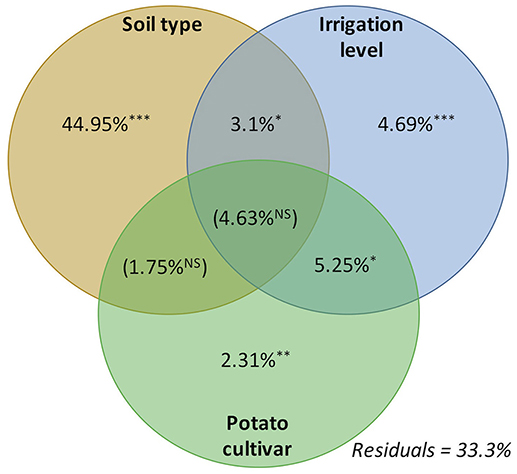
Figure 7. Permutation MONOVA (PERMANOVA) analysis of the main factors soil types, irrigation level, and potato cultivars. The significance was verified at four levels, where p > 0.001 “***,” >0.01 “**,” >0.05 “*,” and “NS” for no significant correlations.
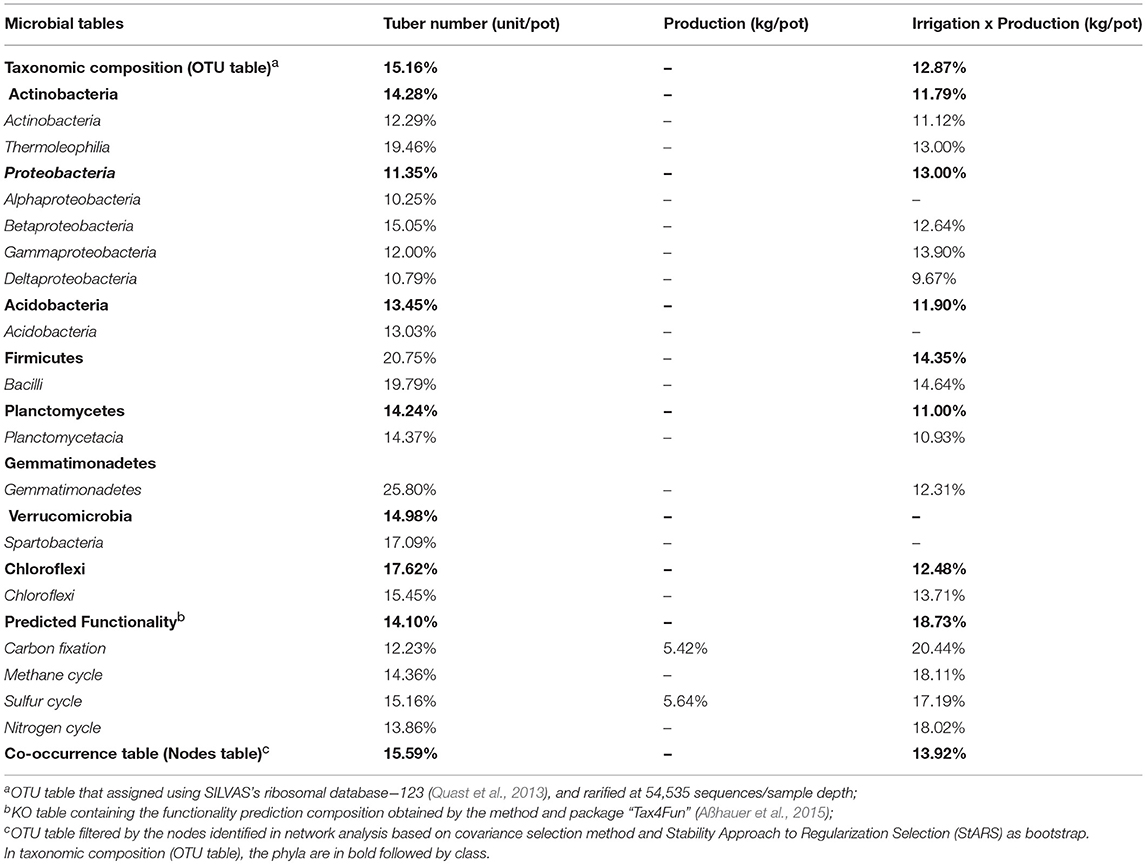
Table 4. PERMANOVA analysis between potato tuber number, potato production, and irrigation: production with the microbial tables taxonomic composition (OTU table), predicted functionality (KO table), and microbial interaction (OTU table filtered by nodes).
Interestingly, it was not observed a significant one-way correlation between microbial tables × potato production, with an exception for carbon fixation (5.42%) and sulfur cycle (5.64%). However, we observed a significant correlation in a two-way correlation using the factorial design of microbial tables ~ (irrigation × potato production). This two-way correlation indicated a stronger correlation (18.73%) between predicted functionality and irrigation × potato production. Also, the carbon fixation explained 20.44% of the variation on the correlation irrigation × potato production. The co-occurrence table (nodes selected in network analysis) showed a higher correlation with irrigation × potato production (13.92%) than taxonomic composition table (12.87%), which present all OTU's. We also observed Firmicutes and Proteobacteria was the phyla with the highest correlation with irrigation: potato production. Gammaproteobacteria (13.9%), Chloroflexi (13.71%), and Thermoleophilia (13%) were the three classes with the highest correlation with irrigation: potato production. Three-way correlations with the factorial design irrigation levels × potato variety × potato tuber number showed no significant correlation (p > 0.05) with microbial tables.
Discussion
Reducing water use is among the most challenging issue in irrigated agriculture, and one way to contribute to this challenge is to integrate soil microbial ecology knowledge to fine-tune irrigation strategies. This study evaluated the effect of four irrigation levels (−15, −25, −30, and −45 kPa) on potato productivity and soil microbial community across three potato cultivars and two soil types (silt and sand). The irrigation levels affected the potato production (Kg/pot), suggesting the soil matric potential −25 kPa as the optimal irrigation level for potato plants. Comparing the irrigation levels from −15 to −25 kPA, we estimate an increase of 680.74 Kg of potato per ha, and reduction of ~10% in the soil water content. Thus, irrigation levels higher than −25 kPa, such as −15 kPa, have excessive irrigation, and lower than −25 kPa, such as −30 and −45 kPa, deficient irrigation. The absence of difference in potato production (Kg/pot) of −25 and −15 kPa demonstrated that high production could be reached with the minimal use of water. Our experiment also indicated that the soil matric potential −25 kPa resulted in best values for the relative mass of potato tuber (Kg/unit of potato accordingly). Thus, we compared the soil microbial community at −25 kPa with other soil matric potentials aiming to reveal soil microbial indicators associated with potato production, and to improve their irrigation management efficiently.
The first set of questions was addressed to the effect of irrigation levels on soil bacterial composition, functionality, and interaction. Five most abundant bacterial phyla (such as Proteobacteria, Actinobacteria, Acidobaceria, Firmicutes, and Chloroflexi) shift in response to soil water content. As reviewed by Naylor and Coleman-Derr (2018), the bacterial community has been presented evolutionary survival strategies under drought stress. These strategies are related to three aspects in general: morphological resistance (e.g., sporulation activity), life strategy (e.g., oligotroph or copiotroph), and interaction with plants (e.g., plants recruitment). Thus, the increases of Firmicutes phyla, as well as genus Bacillus (Firmicutes phylum), in samples with low quantity of water (−45 kPa) can be explained by their sporulation capacity (Vos et al., 2009). Copiotroph is microorganisms that grown fast and requires a rich environment, especially related to carbon (Ho et al., 2017). Irrigation selects copiotroph microorganisms (Hartmann et al., 2017). The Proteobacteria is classified as copiotroph group (Fierer et al., 2007), explaining their reduction under drought condition (−45 kPa). In opposite point, the Acidobacteria has been classified as oligotrophic (i.e., microorganisms with slow growth and poor environment adapted) (Ho et al., 2017), explaining their increase in treatments with low quantity of water (−45 kPa). Plants under drought stress increase the exudation of organic carbon (Henry et al., 2007), recruiting specifics groups of soil microorganisms (Naylor and Coleman-Derr, 2018). The high occurrence of the genus Bradyrhizobium (Proteobacteria phyla) at the −30 kPa treatment could be related to microbial recruitment of potato under drought stress. By similar strategy, different microbial groups may be selected by plants under different irrigation levels, such as the phyla Actinobacteria, as well as the genus Arthrobacter (Actinobacteria phyla) showing higher abundance at −15 kPa.
The carbon cycle has been associated to plant response to drought systems, especially with the plant exudation, and microbial recruitment (Naylor and Coleman-Derr, 2018). The predicted functionality indicated interesting variation at predicted genes related to carbon fixation and emission. The gene ccsB, predicted in 55% of the samples with the irrigation level of −25 kPa, is related to the production of citryl-CoA synthetase enzyme (Aoshima et al., 2004). The gene ccsB acts on the reduction of the citrate cycle (Arnon-Buchanan cycle) where the CO2 is fixated into the microbial cells. These findings suggest that the microbial community in −25 kPa (i.e., optimal water level) is more related to carbon fixation than in the other irrigation levels. For the other genes predicted, the genes mtaA [coenzyme M methyltransferase—EC:2.1.1.246] (Sauer and Thauer, 1999), and mtbB [protein Co-methyltransferase—EC:2.1.1.249] (Soares et al., 2005) are both related with methanogenesis. The prediction of genes was strongly related to −15 kPa (i.e., excessive irrigation), suggesting a possible reduction in the oxygen and emission of CH4. It is important to note that this study not aimed to verify gas emission, but suggests that an optimal irrigation level may be associated with carbon cycle (such as high carbon fixation and low carbon emission). Besides the slight variation across the treatments, the prediction of genes related to the sulfur and nitrogen metabolic paths indicates a variation between the irrigation level with excess available water (−15 kPa) and the other levels. The genes cysNCD [bifunctional enzyme CysN/CysC—EC:2.7.7.4 2.7.1.25 and sulfate adenylyltransferase subunit 2—EC:2.7.7.4] (Leyh et al., 1992) are related to sulfate reduction producing hydrogen sulfide (H2S). This gene has been found in soils with low oxygen levels, such as wetlands (Pester et al., 2012), reinforcing the results associated with excessive irrigation (−15 kPa). Also, the genes amoABC [ammonia monooxygenase—EC:1.14.18.3 1.14.99.39] (Norton et al., 2002) related to oxidation of ammonium () producing nitrite () were predicted to be slightly less prevalent in irrigation level with −15 kPa than in the other treatments. The ammonium oxidation may occur under either aerobic or anaerobic conditions (i.e., anammox) (Van de Graaf et al., 1995); however, the gene relates to ammonia monooxygenase under aerobic conditions (Norton et al., 2002), agreeing with the lower prediction in the treatment with excessive water available (−15 kPa).
The potential microbial interaction, based on co-occurrence or covariation, has been suggested as a promising indicator of system stability and quality. Karimi et al. (2017) observed a variation from 10 to 50% in the connections linked with system perturbation induced by changes in environmental conditions, affecting the local community. Overall, perturbed systems show lower connections (edges) than no-perturbed systems. For example, urban air (polluted) showed 40% fewer connections than rural air (no polluted) (Karimi et al., 2016). This pattern has also been observed in soil systems by different land uses, where crop soils have shown lower connections than natural forest soils (Lupatini et al., 2014). Thus, it was expected that irrigation levels which promote excess or deficit of available soil water (considered as perturbed systems) would be related with system disturbance, showing lower connections than a system with an optimal level of available soil water (considered as a stable system). However, the results suggested the opposite, where the potato system with optimal irrigation level (−25 kPa) showed the lowest number of connections. Other topological properties observed for the irrigation level of −25 kPa were highest positive: negative ratio, the highest distance between groups of nodes (average path length), and densely connection inside of each group of nodes (modularity). The positive: negative ratio >1 indicates more quantities of promotion between the nodes (Xiao et al., 2017). Besides, the positive: negative ratio was higher than 1 for all of the irrigation levels, the irrigation level with less quantity of available water (−45 kPa) showed the lower positive: negative ratio. This result was also observed at phyla level. Then, we suggest that an optimal irrigation level (such as −25 kPa) it is not merely limited to the irrigation level supporting highest crop production but also a level where the microbial community shows higher promotion (positive interaction) between the nodes with densely intern interaction. On the other hand, the excess or water deficit (such as −15, −30, and −45 kPa) may promote more competition between the microbial community, increasing the total connections (edges). The high number of connections may be related with a response of the microbial community to tolerate the water excess or deficit, such as an increase in the production of secondary metabolites (Braga et al., 2016). Thus, we suggest for irrigation levels in crop systems that the increase in the total connections may be related to water disturbance. These variations on the taxonomic composition, predicted functionality, and potential microbial interactions associated with irrigation levels confirms our first hypothesis.
The second set of questions were addressed to evaluate the correlation between irrigation levels, soil type, and potato cultivar with soil bacterial community. The results confirmed that the difference in soil composition was strongly correlated with the structure of the soil bacterial community, suggesting that each soil type was a different source of soil bacterial community. Interestingly, the irrigation level was the only variable showing a significant one-way correlation (bacterial community ~ irrigation level), and also two-way interactions with soil type (bacterial community ~ irrigation level × soil type) and potato cultivar (bacterial community ~ irrigation level × potato cultivar). This result suggested that the soil bacterial community is not only affected by the available quantity of water but also by an effect that water may promote on the soil and potato cultivar. It has been observed that variation on the water supply affects the soil microbial community in natural (Taketani et al., 2017) and crop (Bastida et al., 2017) systems. Our first set of correlations confirmed an effect of soil matric potentials on soil bacterial community, and also reveal an effect of irrigation levels inside of other factors (soil type and potato cultivars). Nevertheless, there are still many unanswered questions about the association between crop plants and soil microbial communities across different environmental conditions, including systems with water excess or deficit.
The second group of correlations analyses was addressed to potato yield, where we evaluated the correlation between the production (i.e., potato production and potato tuber number) with microbial composition, predicted functionality, and co-occurrence nodes. Overall, the results suggested that soil microbial community (composition, predicted functionality, and co-occurrence nodes) are one-way correlated with the potato tuber number, but two-way correlated with potato production and irrigation levels (i.e., irrigation level × potato production). The two-way correlation suggested that only when the variation in potato production is associated with irrigation level information by the factorial design, they present a significant correlation with bacterial community variances. The results also suggested that the potato tuber number was more correlated with taxonomic table (OTU table) and co-occurrence nodes, while the potato production was more two-way correlated with predicted biogeochemistry cycles, mainly the carbon cycle. The phylum Gemmatimonadetes showed the highest correlation with potato tuber number. This phylum has been already identified in soil cultivated with potato (Liu et al., 2014), and their distribution has been related to soil moisture (DeBruyn et al., 2011). Besides the small variation of Gemmatimonadetes relative abundance across the irrigation levels, the −45 kPa showed the lowest Gemmatimonadetes relative abundance and also the lowest potato tuber number (unit/pot). These findings suggest that the Gemmatimonadetes occurrence may be associated with potato tuber formation. For potato production, we suggest a different and more complex interaction where the bacterial community correlated may be better classified by functionality, such as carbon cycle (fixation and emission), than them taxonomic affiliation. Further, the irrigation level would promote or reduce the bacterial functionality, which may regulate the potato production in this system. It is important to note that these general suggestions are one of the other explanations that could justify the link between microbial predicted functionality, irrigation levels, and potato production. Besides some questions remain about this link, we confirmed our second hypothesis suggesting that the soil bacterial interaction and functionality have a potential association with potato yields.
The third hypothesis tested was whether the irrigation level with the highest production is the same as the highest bacterial diversity. The microbial diversity has been considered as one of the most important indexes of soil quality (Brussaard et al., 2007). The high microbial diversity has benefits for the cropping system, e.g., the crop rotation improving the soil microbial diversity and promoting disease suppressive capacity in soil (Peralta et al., 2018). Thus, we expected that the irrigation level with higher production would also show a higher soil bacterial diversity. However, the irrigation levels −25 and −15 kPa, which showed the highest potato production also showed the lowest alpha-diversity. It is crucial to note that this result did not disqualify the microbial soil diversity as an excellent soil quality indicator. This result suggested that, in crop systems, the alpha-diversity of the bacterial community may also indicate water stress (excess or deficit), also affecting the potato production. For this fact, we propose a similar explanation used for the potential microbial interactions, where the excess or deficit of water may increase the connections of soil bacterial community, producing secondary metabolites and also opening different niches in the system. Thus, the opening of new niches would be a collateral effect of the microbial community interactions aiming to tolerate the water stress. This unexpected absence of linkage between alpha diversity and productivity might be more important in the field (e.g., the impact on long-term productivity), which also could include other variables such as microbial distribution (beta diversity). Irrigation level with high potato production showed lower alpha-diversity, refuting our third hypothesis.
The present study was designed to determine the effect of irrigation levels on soil bacterial community, focusing on a high potato yield with minimal water use. These experiments confirmed that the water matric potential of −25 kPa as an optimal irrigation level for potato production, and also revealed unexpected patterns of soil bacterial community. The most apparent finding to emerge from this study is that the water excess or deficit promotes an increase in total microbial interaction, and also in bacterial alpha-diversity, which may be used in further studies to identify irrigation levels with optimal water use. It is important to emphasize that as the water was the only factor that varied in the experiment for two soil types and three potato cultivars, any possible variation among other soil parameters are a consequence of the water matric potential. The correlations revealed associations between the irrigation levels, potato production, and bacterial functionality, especially in carbon fixation. These associations raised questions more specific for soil microbial community in crop systems. The advancement of this knowledge may lead to a more comprehensive assessment of the optimal irrigation levels of various crop production systems. Furthermore, the inclusion of ecological studies in field agriculture has the potential to contribute to the development of water management practices conducive to both increasing crop yield and maintaining a sustainable soil environment.
Data accessibility
Fastq files, OTU table, and raw environmental data will be available for download from https://doi.org/10.6084/m9.figshare.6840533. The fastq files containing the genomic sequence (DNA) will also be available at European Nucleotide Archive (ENA; https://www.ebi.ac.uk/ena), study number PRJEB27037.
Author Contributions
TG, SG, and AR conceived the presented idea. SG conceived the original idea of irrigations levels on potato cultivars. J-PM and GL carried out the irrigation experiment. TG and PC performed the bioinformatic and statistical analysis. AR encouraged TG to investigate network analysis and supervised the findings of this work. All authors discussed the results and contributed to the final manuscript.
Conflict of Interest Statement
The authors declare that the research was conducted in the absence of any commercial or financial relationships that could be construed as a potential conflict of interest.
Acknowledgments
This work was supported by NSERC (Natural Sciences and Engineering Research Council of Canada). We are grateful to Silvio's team (Laval University/Department of Soils and Agri-Food Engineering) for providing the soil samples and the metadata. We thank Charles Goulet and Marie-Claire Goulet for all the support with molecular analyses. We also acknowledge the comments and discussion provided by Annelise Mendes Nascimento and Ademir Durrer.
Supplementary Material
The Supplementary Material for this article can be found online at: https://www.frontiersin.org/articles/10.3389/fenvs.2018.00161/full#supplementary-material
References
Anderson, M. J. (2017). “Permutational multivariate analysis of variance (PERMANOVA),” in Wiley StatsRef: Statistics Reference Online (Chichester, UK: John Wiley and Sons Ltd.), 1–15. doi: 10.1002/9781118445112.stat07841
Aoshima, M., Ishii, M., and Igarashi, Y. (2004). A novel enzyme, citryl-CoA lyase, catalysing the second step of the citrate cleavage reaction in Hydrogenobacter thermophilus TK-6. Mol. Microbiol. 52, 763–770. doi: 10.1111/j.1365-2958.2004.04010.x
Aßhauer, K. P., Wemheuer, B., Daniel, R., and Meinicke, P. (2015). Tax4Fun: Predicting functional profiles from metagenomic 16S rRNA data. Bioinformatics 31, 2882–2884. doi: 10.1093/bioinformatics/btv287
BajŽelj, B., Richards, K. S., Allwood, J. M., Smith, P., Dennis, J. S., Curmi, E., et al. (2014). Importance of food-demand management for climate mitigation. Nat. Clim. Chang. 4, 924–929. doi: 10.1038/nclimate2353
Bastian, M., Heymann, S., and Jacomy, M. (2009). Gephi: an open source software for exploring and manipulating networks. Third Int. AAAI Conf. Weblogs Soc. Media 361–362. doi: 10.1136/qshc.2004.010033
Bastida, F., Torres, I. F., Romero-Trigueros, C., Baldrian, P., Větrovský, T., Bayona, J. M., et al. (2017). Combined effects of reduced irrigation and water quality on the soil microbial community of a citrus orchard under semi-arid conditions. Soil Biol. Biochem. 104, 226–237. doi: 10.1016/j.soilbio.2016.10.024
Box, G. E. P., and Cox, D. R. (1964). An analysis of transformations. J. R. Stat. Soc. Ser. B 26, 211–252.
Braga, R. M., Dourado, M. N., and Araújo, W. L. (2016). Microbial interactions: ecology in a molecular perspective. Brazil. J. Microbiol. 47, 86–98. doi: 10.1016/j.bjm.2016.10.005
Brussaard, L., de Ruiter, P. C., and Brown, G. G. (2007). Soil biodiversity for agricultural sustainability. Agric. Ecosyst. Environ. 121, 233–244. doi: 10.1016/j.agee.2006.12.013
Caporaso, J. G., Kuczynski, J., Stombaugh, J., Bittinger, K., Bushman, F. D., Costello, E. K., et al. (2010). QIIME allows analysis of high-throughput community sequencing data. Nat. Methods 7, 335–336. doi: 10.1038/nmeth.f.303
Caporaso, J. G., Lauber, C. L., Walters, W. A., Berg-Lyons, D., Lozupone, C. A., Turnbaugh, P. J., et al. (2011). Global patterns of 16S rRNA diversity at a depth of millions of sequences per sample. Proc. Natl. Acad. Sci. U.S.A. 108, 4516–4522. doi: 10.1073/pnas.1000080107
Caron, J., Pelletier, V., Kennedy, C. D., Gallichand, J., Gumiere, S., Bonin, S., et al. (2017). Irrigation and drainage management strategies to enhance cranberry production and optimize water use in North America. Can. J. Soil Sci. 91, CJSS-2016-0086. doi: 10.1139/CJSS-2016-0086
Castrillo, G., Teixeira, P. J. P. L., Paredes, S. H., Law, T. F., De Lorenzo, L., Feltcher, M. E., et al. (2017). Root microbiota drive direct integration of phosphate stress and immunity. Nature 543, 513–518. doi: 10.1038/nature21417
Cosgrove, W. J., and Loucks, D. P. (2015). Water management: current and future challenges and research directions. Water Resour. Res. 51, 4823–4839. doi: 10.1002/2014WR016869
Csardi, G., and Nepusz, T. (2006). The igraph software package for complex network research. InterJ. Complex Sy, 1695, 1–9. doi: 10.1109/ICCSN.2010.34
Dalla Costa, L., Delle Vedove, G., Gianquinto, G., Giovanardi, R., and Peressotti, A. (1997). Yield, water use efficiency and nitrogen uptake in potato: influence of drought stress. Potato Res. 40, 19–34. doi: 10.1007/BF02407559
DeBruyn, J. M., Nixon, L. T., Fawaz, M. N., Johnson, A. M., and Radosevich, M. (2011). Global biogeography and quantitative seasonal dynamics of gemmatimonadetes in soil. Appl. Environ. Microbiol. 77, 6295–6300. doi: 10.1128/AEM.05005-11
Ercin, A. E., and Hoekstra, A. Y. (2014). Water footprint scenarios for 2050: a global analysis. Environ. Int. 64, 71–82. doi: 10.1016/j.envint.2013.11.019
Faust, K., and Raes, J. (2012). Microbial interactions: from networks to models. Nat. Rev. Microbiol. 10, 538–550. doi: 10.1038/nrmicro2832
Fierer, N., Bradford, M. A., and Jackson, R. B. (2007). Toward an ecological classification of soil bacteria. Ecology 88, 1354–1364. doi: 10.1890/05-1839
FPPTQ (2016). Répertoire de la Production de Semences Certifiées de Pommes de Terre 2016-2017. Available online at: http://www.pptq.ca/documents/Repertoire2016-2017.pdf
Frenk, S., Hadar, Y., and Minz, D. (2018). Quality of irrigation water affects soil functionality and bacterial community stability in response to heat disturbance. Appl. Environ. Microbiol. 84:AEM.02087-17. doi: 10.1128/AEM.02087-17
Frenken, K., and Gillet, V. (2012). Irrigation Water Requirement and Water Withdrawal by Country. Available online at: http://www.fao.org/nr/water/aquastat/water_use_agr/IrrigationWaterUse.pdf
Groenevelt, P. H., Grant, C. D., and Murray, R. S. (2004). On water availability in saline soils. Aust. J. Soil Res. 42, 833–840. doi: 10.1071/SR03054
Hartmann, M., Brunner, I., Hagedorn, F., Bardgett, R. D., Stierli, B., Herzog, C., et al. (2017). A decade of irrigation transforms the soil microbiome of a semi-arid pine forest. Mol. Ecol. 26, 1190–1206. doi: 10.1111/mec.13995
Henry, A., Doucette, W., Norton, J., and Bugbee, B. (2007). Changes in crested wheatgrass root exudation caused by flood, drought, and nutrient stress. J. Environ. Qual. 36:904. doi: 10.2134/jeq2006.0425sc
Ho, A., Di Lonardo, D. P., and Bodelier, P. L. E. (2017). Revisiting life strategy concepts in environmental microbial ecology. FEMS Microbiol. Ecol. 93, 1–14. doi: 10.1093/femsec/fix006
Jackson, M. B., and Colmer, T. D. (2005). Response and adaptation by plants to flooding stress. Ann. Bot. 96, 501–505. doi: 10.1093/aob/mci205
Karimi, B., Maron, P. A., Chemidlin-Prevost Boure, N., Bernard, N., Gilbert, D., and Ranjard, L. (2017). Microbial diversity and ecological networks as indicators of environmental quality. Environ. Chem. Lett. 15, 265–281. doi: 10.1007/s10311-017-0614-6
Karimi, B., Meyer, C., Gilbert, D., and Bernard, N. (2016). Air pollution below WHO levels decreases by 40 % the links of terrestrial microbial networks. Environ. Chem. Lett. 14, 467–475. doi: 10.1007/s10311-016-0589-8
Kirkham, M. B. (2005). Principles of Soil and Plant Water Relations. London: Elsevier. doi: 10.1016/B978-0-12-409751-3.X5000-2
Kopylova, E., Navas-Molina, J. A., Mercier, C., Xu, Z. Z., Mahe, F., He, Y., et al. (2016). Open-source sequence clustering methods improve the state of the art. mSystems 1:e00003. doi: 10.1128/mSystems.00003-15.Editor
Kurtz, Z. D., Müller, C. L., Miraldi, E. R., Littman, D. R., Blaser, M. J., and Bonneau, R. A. (2015). Sparse and compositionally robust inference of microbial ecological networks. PLoS Comput. Biol. 11, 1–25. doi: 10.1371/journal.pcbi.1004226
Létourneau, G., Caron, J., Anderson, L., and Cormier, J. (2015). Matric potential-based irrigation management of field-grown strawberry: effects on yield and water use efficiency. Agri. Water Manag. 161, 102–113. doi: 10.1016/j.agwat.2015.07.005
Leyh, T. S., Vogt, T. F., and Suo, Y. (1992). The DNA sequence of the sulfate activation locus from Escherichia coli K-12. J. Biol. Chem. 267, 10405–10410.
Liu, X., Zhang, J., Gu, T., Zhang, W., Shen, Q., Yin, S., et al. (2014). Microbial community diversities and taxa abundances in soils along a seven-year gradient of potato monoculture using high throughput pyrosequencing approach. PLoS ONE 9:086610. doi: 10.1371/journal.pone.0086610
Lupatini, M., Suleiman, A. K. A., Jacques, R. J. S., Antoniolli, Z. I., de Siqueira Ferreira, A., Kuramae, E. E., et al. (2014). Network topology reveals high connectance levels and few key microbial genera within soils. Front. Environ. Sci. 2, 1–11. doi: 10.3389/fenvs.2014.00010
Ma, Z. (2018). The P/N (Positive-to-Negative Links) ratio in complex networks—a promising in silico biomarker for detecting changes occurring in the human microbiome. Microb. Ecol. 75, 1063–1073. doi: 10.1007/s00248-017-1079-7
Meyer, K. M., Klein, A. M., Rodrigues, J. L. M., Nüsslein, K., Tringe, S. G., Mirza, B. S., et al. (2017). Conversion of Amazon rainforest to agriculture alters community traits of methane-cycling organisms. Mol. Ecol. 26, 1547–1556. doi: 10.1111/mec.14011
Naylor, D., and Coleman-Derr, D. (2018). Drought stress and root-associated bacterial communities. Front. Plant Sci. 8, 1–16. doi: 10.3389/fpls.2017.02223
Newman, M. E. J. (2003). The structure and function of complex networks. SIAM Rev. 45, 167–256. doi: 10.1137/S003614450342480
Newman, M. E. J. (2006). Modularity and community structure in networks. Proc. Natl. Acad. Sci. U.S.A. 103, 8577–8582. doi: 10.1073/pnas.0601602103
Norton, J. M., Alzerreca, J. J., Suwa, Y., and Klotz, M. G. (2002). Diversity of ammonia monooxygenase operon in autotrophic ammonia-oxidizing bacteria. Arch. Microbiol. 177, 139–149. doi: 10.1007/s00203-001-0369-z
Oksanen, J., Blanchet, G. F., Friendly, M., Kindt, R., Legendre, P., McGlinn, D., et al. (2013). Community Ecology Package. 2. doi: 10.4135/9781412971874.n145
Peralta, A. L., Sun, Y., McDaniel, M. D., and Lennon, J. T. (2018). Crop diversity increases disease suppressive capacity of soil microbiomes. Ecosphere 5, 1–39. doi: 10.1002/ecs2.2235
Pester, M., Knorr, K. H., Friedrich, M. W., Wagner, M., and Loy, A. (2012). Sulfate-reducing microorganisms in wetlands - fameless actors in carbon cycling and climate change. Front. Microbiol. 3, 1–19. doi: 10.3389/fmicb.2012.00072
Prosser, J. I., and Nicol, G. W. (2008). Relative contributions of archaea and bacteria to aerobic ammonia oxidation in the environment. Environ. Microbiol. 10, 2931–2941. doi: 10.1111/j.1462-2920.2008.01775.x
Quast, C., Pruesse, E., Yilmaz, P., Gerken, J., Schweer, T., Yarza, P., et al. (2013). The SILVA ribosomal RNA gene database project: Improved data processing and web-based tools. Nucl. Acids Res. 41, 590–596. doi: 10.1093/nar/gks1219
Sasaki, T., Massaki, N., and Kubo, T. (2005). Wolbachia variant that induces two distinct reproductive phenotypes in different hosts. Heredity 95, 389–393. doi: 10.1038/sj.hdy.6800737
Sauer, K., and Thauer, R. K. (1999). Methanol:coenzyme M methyltransferase from Methanosarcina barkeri - Substitution of the corrinoid harbouring subunit MtaC by free cob(I)alamin. Eur. J. Biochem. 261, 674–681. doi: 10.1046/j.1432-1327.1999.00355.x
Soares, J. A., Zhang, L., Pitsch, R. L., Kleinholz, N. M., Jones, R. B., Wolff, J. J., et al. (2005). The residue mass of L-pyrrolysine in three distinct methylamine methyltransferases. J. Biol. Chem. 280, 36962–36969. doi: 10.1074/jbc.M506402200
Soil Survey Staff (1999). Soil Taxonomy. A Basic System of Soil Classification for Making and Interpreting Soil Surveys, 2nd Edn. Washington, DC: U.S. Government Printing Office. doi: 10.1007/BF01574372
Taketani, R. G., Lançoni, M. D., Kavamura, V. N., Durrer, A., Andreote, F. D., and Melo, I. S. (2017). dry season constrains bacterial phylogenetic diversity in a semi-arid rhizosphere system. Microb. Ecol. 73, 153–161. doi: 10.1007/s00248-016-0835-4
Thompson, L. R., Sanders, J. G., McDonald, D., Amir, A., Ladau, J., Locey, K. J., et al. (2017). A communal catalogue reveals Earth's multiscale microbial diversity. Nature 551, 457–463. doi: 10.1038/nature24621
Van de Graaf, A. A., Mulder, A., De Bruijn, P., Jetten, M. S. M., Robertson, L. A., and Kuenen, J. G. (1995). Anaerobic oxidation of ammonium is a biologically mediated process. Appl. Environ. Microbiol. 61, 1246–1251.
van Genuchten, M. T. (1980). A closed-form equation for predicting the hydraulic conductivity of unsaturated soils. Soil Sci. Soc. Am. J. 44, 892. doi: 10.2136/sssaj1980.03615995004400050002x
Vos, P., Garrity, G., Jones, D., Krieg, N. R., Ludwig, W., Rainey, F. A., et al. (2009). Bergey's Manual of Systematic Bacteriology, Vol. 3. New York, NY: The Firmicutes. doi: 10.1007/b92997
Wang, F. X., Kang, Y., Liu, S. P., and Hou, X. Y. (2007). Effects of soil matric potential on potato growth under drip irrigation in the North China Plain. Agric. Water Manag. 88, 34–42. doi: 10.1016/j.agwat.2006.08.006
Xiao, Y., Angulo, M. T., Friedman, J., Waldor, M. K., Weiss, S. T., and Liu, Y.-Y. (2017). Mapping the ecological networks of microbial communities. Nat. Commun. 8:2042. doi: 10.1038/s41467-017-02090-2
Keywords: precision irrigation, microbial interaction, soil matric potential, crop production, soil health, water use
Citation: Gumiere T, Gumiere SJ, Matteau J-P, Constant P, Létourneau G and Rousseau AN (2019) Soil Bacterial Community Associated With High Potato Production and Minimal Water Use. Front. Environ. Sci. 6:161. doi: 10.3389/fenvs.2018.00161
Received: 22 July 2018; Accepted: 19 December 2018;
Published: 10 January 2019.
Edited by:
Christophe Darnault, Clemson University, United StatesReviewed by:
Ademir Araujo, Federal University of Piauí, BrazilAchouak Arfaoui, Ministry of Agriculture (Tunisia), Tunisia
Copyright © 2019 Gumiere, Gumiere, Matteau, Constant, Létourneau and Rousseau. This is an open-access article distributed under the terms of the Creative Commons Attribution License (CC BY). The use, distribution or reproduction in other forums is permitted, provided the original author(s) and the copyright owner(s) are credited and that the original publication in this journal is cited, in accordance with accepted academic practice. No use, distribution or reproduction is permitted which does not comply with these terms.
*Correspondence: Thiago Gumiere, dGhpYWdvLmd1bWllcmVAZXRlLmlucnMuY2E=