- 1Earth and Life Institute-Environmental Sciences, Université catholique de Louvain, Louvain-la-Neuve, Belgium
- 2Hydraulics and Environmental Modeling Laboratory (HydroModE-Lab), Department of Natural Resources Management, Université de Parakou, Parakou, Benin
- 3Laboratory of Hydraulics and Water Management (LHME), Department of Natural Resources Management, Université d'Abomey-Calavi, Cotonou, Benin
Fertilizer microdosing is being widely promoted across sub-Saharan Africa, yet all recommendations regarding this technology are derived from short-term studies. Such studies are insufficient to properly assess the production risk caused by climatic variability. To address this issue while avoiding costly long-term experiments, a common and well accepted strategy is to combine results from short-term experiments with validated dynamic crop models. However, there have been few documented attempts so far to model fertilizer microdosing under sub-humid tropical conditions. The objective was therefore to evaluate the potential of the DSSAT model for simulating maize response to fertilizer microdosing, and to use the validated model to assess the effects of inter-annual rainfall variability on maize productivity and economic risk. The model was calibrated and validated against data from a 2-year on-station experiment (2014 and 2015) with 2 levels of hill-placed manure and five mineral fertilization options including broadcast and fertilizer microdosing. Model simulations were in good agreement with the observed grain and biomass yields for conventional broadcast fertilization, with relative RMSE and d-values of 12% and 0.96 for grain and 8% and 0.97 for biomass, respectively. For fertilizer microdosing, the N stress coefficient needed to be adjusted to avoid occurrence of large N stresses during simulation. After optimization, the model adequately reproduced grain yields for fertilizer microdosing, with relative RMSE of 10%. Considering the long-term scenario analysis, the use of the validated model showed that the application of 2 g of NPK15−15−15 fertilizer + 1 g urea per hill (equivalent to 23.8 kg N ha−1, 4.1 kg P ha−1 and 7.8 kg K ha−1) improved both the minimum guaranteed yield and the long-term average without increasing inter-annual variability and the economic risk compared to unfertilized plots. Even though combining microdosing with manure (1–3 t ha−1) was economically slightly riskier than microdosing alone, this risk remained low since a value-cost ratio of 2 could be achieved in almost 100% of the years. Furthermore, combined application consistently reduced the inter-annual yield variability. Considering this as well as the other benefits of manure for soil health, combining microdosing with small quantities of manure would be recommended to increase the sustainability of the system.
Introduction
In Sub-Saharan Africa (SSA), low crop yields are a persistent concern because of their impact on food security, chronic poverty, and hunger (Morris et al., 2007; Vanlauwe et al., 2015). A major cause for the low yields is the low inherent soil fertility as well as nutrient and organic matter depletion in smallholder farming systems (Henao and Baanante, 2006; Bationo and Waswa, 2011; Tittonell and Giller, 2013). Much effort has therefore been invested over the past decades to provide farmers with suitable nutrient management practices in view of raising crop yields and income.
Fertilizer microdosing (or microdose fertilization) is a strategic adaptation of conventional fertilizer management and has recently been advocated as a means to increase crop productivity, profitability, and resource use efficiency for smallholder farmers in SSA (Muehlig-Versen et al., 2003). Indeed, over the last two decades, a number of experiments (both on-station and on-farm) have demonstrated a generally strong positive impact of this low-input technology on crop yields and farmer income (Aune et al., 2007; Camara et al., 2013; Sime and Aune, 2014; Ibrahim et al., 2015; Okebalama et al., 2016; Tovihoudji et al., 2017). However, all presently published data regarding microdose fertilization are derived from short-term studies. Such studies are insufficient to properly assess the agronomic and economic risk of agricultural technologies. One critical risk component is related to the as yet unpredictable temporal distribution of rainfall over the course of a season. This uncertainty regarding the temporal rainfall distribution drives much of the behavior of smallholder farmers (Akponikpè et al., 2011; Marteau et al., 2011; Comoé and Siegrist, 2015; Guan et al., 2017), since partial or total crop failure due to drought stress can strongly affect the profitability of crop intensification technologies. Thus, it is crucial to consider this long-term variability when evaluating new technologies. This is especially true for fertilizer management practices given that rainfed crop response to fertilizer inputs is strongly dependent on the amount and distribution of rainfall (Akponikpè et al., 2010; MacCarthy et al., 2010; Folberth et al., 2013). Moreover, farmers' willingness to adopt the microdosing technology will depend not only on increased yields and profitability but also on yield stability. Indeed, microdosing is generally considered as a stopgap option for subsistence farmers for whom achieving some minimal yield every year to cover household food requirements is more important than maximizing yields in favorable years.
To address the issue of long-term climatic variability while avoiding costly long-term experiments, a common and well-accepted strategy is to combine results from short-term experiments with robust and validated dynamic crop models (Jones et al., 2003; Rezzoug et al., 2008; Holzworth et al., 2014). Such simulation models can be used to explore the impact of long-term climate variability on crop productivity and profitability for a range of soil and water management strategies. Among these models APSIM (Agricultural Production Systems Simulator; Keating et al., 2003) and DSSAT (Decision Support System for Agrotechnology Transfer; Jones et al., 2003) are the two most frequently and widely used in SSA.
Unlike for conventional fertilization practices, only a few studies have so far attempted to model crop response to fertilizer microdosing. Modeling fertilizer microdosing using soil-plant-atmosphere models such as DSSAT and APSIM represents a specific challenge since such 1-D models are not well suited to deal with localized fertilizer placement. Previous attempts were all based on the use of APSIM in the context of southern and eastern Africa (Cooper et al., 2008; Twomlow et al., 2008; Turner and Rao, 2013). However, none of these studies actually demonstrate that APSIM was capable of properly reproducing crop response to microdose fertilization since simulation results are not compared to measured data. In the present study, we seek to model the response of maize to microdose fertilization under the sub-humid tropical conditions of northern Benin using DSSAT. DSSAT was selected because the suitability of the CERES-Maize module implemented in DSSAT for simulating maize growth and yield has been successfully demonstrated across a broad range of soil, management and climatic conditions in smallholder farming systems in SSA (MacCarthy et al., 2012, 2017; Ngwira et al., 2014; Corbeels et al., 2016; Adnan et al., 2017a,b), including the sub-humid region of Benin (Igué et al., 2013; Saïdou et al., 2017; Amouzou et al., 2018). More specifically, the objectives of this study were to (1) assess the performance of DSSAT (calibration and validation) in simulating the effect of conventional nutrient management practices and fertilizer microdosing for maize in Northern Benin; (2) determine model sensitivity to key input, and (3) use the validated model to assess the effects of seasonal climate variability on maize productivity and economic risk under fertilizer microdosing with or without manure through a long-term (32 years) scenario analysis.
Materials and Methods
Experimental Data
Site Description
The experiments were conducted at the Agricultural Research Station of Northern Benin (CRA- Nord) located at Ina village (Ina district, municipality of Bembèrèkè) (9°57′N and 2°42′E, and altitude 365 m), 70 km north-east of Parakou. This village belongs to the agro-ecological region III in northern Benin, the main production zone of food and cash crops where annual rainfall ranges between 900 and 1,200 mm. The average annual rainfall at Ina is 1148 ± 184 mm (mean ± SD) and the average daily temperature is 27.5°C (CRA-Nord Climate Database, 1982–2015). The climate is characterized by a single rainy season between May and October. A marked characteristic of the climate in northern Benin is the intra-annual doubled with inter-annual variability of rainfall (coefficient of variation of 16%), with a general decreasing trend during the past decades (Gnanglè et al., 2011). The soil is classified as a ferruginous tropical soil in the French soil classification system with low inherent fertility, which corresponds to Lixisols according to the World Reference Base (Youssouf and Lawani, 2002).
Maize (Zea mays L.) is the major staple crop in Benin. The total annual national production has increased from 219,593 tons in 1961 to about 1,376,683 tons in 2016 (FAOSTAT., 2016). In northern Benin, it is mainly produced under rainfed conditions on ~82–84% of the total area devoted to cereal crops.
Experimental Design and Crop Management
The experiment was conducted during two rainy seasons (2014–2015). Details of this experiment have been published elsewhere (Tovihoudji et al., 2017) but are briefly described here. The experimental layout was a randomized complete block design with three replications within each manure stratum. Two levels of manure were considered: (i) no manure (NM) and (ii) hill-placement of farmyard manure applied each year at a rate of 3 t ha−1 (3 M) at 10 days after sowing (DAS). The five mineral fertilizer levels tested within each manure stratum were: (i) a control (no fertilizer, NF); fertilizer microdosing at a rate of (ii) 2 g of composite NPK15−15−15 fertilizer per hill at 10 DAS + 1 g urea (46% N) per hill at 45 DAS (MD1), (iii) 4 g of NPK15−15−15 fertilizer per hill at 10 DAS + 1 g urea per hill at 45 DAS (MD2); (iv) 50% (50F), and (v) 100% (100F) of the broadcasted recommended rate by the National Agricultural Research System (200 kg NPK15−15−15 ha−1 at 10 DAS + 100 kg urea ha−1 at 45 DAS). These rates are equivalent to 23.8 kg N ha−1, 4.1 kg P ha−1, and 7.8 kg K ha−1 for MD1, 33.1 kg N ha−1, 8.2 kg P ha−1, and 15.6 kg K ha−1 for MD2, 38 kg N ha−1, 6.5 kg P ha−1, and 12.5 kg K ha−1 for 50F and 76 kg N ha−1, 13.1 kg P ha−1, and 24.9 kg K ha−1 for 100F. Manure samples were taken in both years to determine chemical composition (Tovihoudji et al., 2017). Different plots were used in both seasons, i.e., there was no cumulative effect of the treatments.
At the onset of the experiment, land preparation was done uniformly across all plots by tractor disk-plowing (depth of 0.2 m). The improved and early maturing (90-days maturity) maize variety DMR-ESR-W (Downy Mildew Resistant, Early-Streak Resistant, White) was planted at a density of 31,250 hills ha−1. Maize seedlings were thinned to 2 plants hill−1 2 weeks after planting. Plots were weeded twice (15 and 30 DAS) and ridged with a hand hoe 45 DAS immediately after urea application.
Data Collection
Different data were collected during the experiment including crop phenology (emergence day, date to anthesis, and physiological maturity), leaf area and biomass time-series, grain and aboveground biomass yield, and N uptake at harvest. Pre-planting soil samples were analyzed for macronutrients (N, P, and K), texture, pH, organic carbon, and bulk density at various depths between 0 and 1 m.
Periodic soil water content measurements were taken using an in situ calibrated portable soil moisture meter (TRIME-PICO IPH/T3, IMKO Micromodultechnik GmbH). Measurements were taken every 0.1 m from 0 to 0.6 m depth (maximum root concentration zone) in all the plots. For the sake of clarity, water content data were aggregated over 0.2 m layers. Biomass was measured by destructively sampling whole plants from two planting holes every fortnight from 20 DAS until final harvest. Leaf area was recorded in each experimental plot by randomly tagging five plants from five different planting holes in the three middle rows reserved for final biomass measurement. The green leaf length and width were measured every fortnight with a ruler and the leaf area (LA) was calculated as : Leaf area (LA) = Leaf length * maximum width * k, where k is a shape factor with the value of 0.75 (Yi et al., 2006). The leaf area index (LAI) was calculated as the ratio of LA to the horizontal soil surface area occupied by each planting hill. Final harvest was done by hand, and aboveground biomass and grain yields were recorded as described in Tovihoudji et al. (2017).
CERES-Maize Model
Model Description
In this study, we used DSSAT version 4.6, with CERES-Maize as the crop model (Hoogenboom et al., 2015). In this study, we used the “daily canopy photosynthesis method” for maize photosynthesis (Jones and Kiniry, 1986), CENTURY to simulate soil carbon and nitrogen dynamics (Gijsman et al., 2002), the Priestly and Taylor method for evapotranspiration, and the Soil Conservation Service method (USDA-Soil Conservation Service, 1972) for soil water infiltration. Further detailed descriptions of the processes are available in Jones et al. (2003).
Model Parameterization and Calibration
Crop cultivar coefficients and management inputs
In the present study, the genetic coefficients for the maize cultivar used were calibrated based on the growth and development data recorded during the 2014 season (when the highest yields were observed) for the highest, broadcast mineral N treatment (NM-100F). To simulate the baseline soil water and N dynamics as well as the response to manure application, two additional treatments (the absolute control NM-NF and the manured treatment 3M-NF) were included in the calibration process.
Values used for species-specific parameters were the default values for maize in the CERES-Maize model. Since the maize cultivar used in the experiment had not been previously modeled with DSSAT, the genetic coefficients were calibrated by choosing a default, medium-maturing cultivar from Ghana (Obatampa; identification code = GH0010 and ecotype IB0001) as a starting point and manually adjusting these parameters to minimize the root mean square error (RMSE) between simulated and measured data. First, the coefficients controlling phenology (P1, P2, P5, and PHINT; Table 1) were modified to match anthesis and maturity dates, and leaf number. Later, the G2 and G3 parameters were adjusted so as to minimize the RMSE between measured and modeled biomass and yield (Table 1).
Soil input data
The soil fertility factor (SLPF) was adjusted after adjusting species-specific parameters. It was modified manually and the value was set to 0.90 by minimizing the error between the observed and simulated total biomass. The root distribution weighing factor (SRGF) is an input for each soil layer and reflects physical or chemical constraints on root growth in certain soil layers (Ritchie, 1998). Its value ranges from 1 (indicating that the soil layer is most hospitable to root growth) to 0 (indicating that the soil is inhospitable for root growth). SRGF was estimated using a function in the DSSAT soil creation utility program based on soil texture, bulk density and soil organic carbon (Supplementary Table S1). Measured soil properties including soil organic carbon, total nitrogen, soil bulk density (BD), pH, and soil texture (percent silt, clay, and sand) were used as input (Supplementary Table S1). Soil hydrological properties such as soil water content at field capacity (drained upper limit = DUL), at permanent wilting point (lower limit = LL), and at saturation (SAT) were taken from Tovihoudji (2018). Soil hydraulic conductivity was estimated using pedotransfer functions available in DSSAT. Initial mineral nitrogen content (NH4-N and NO3-N) was taken from unpublished data from the same experimental site. The runoff curve number (RCN) and drainage coefficient (SWCON) were set to 61 (default value) and 0.4 (adjusted), respectively, to simulate negligible runoff (accounting for the flat topography and good structure of the soil) and moderately well-draining soils which are characteristic of the soil at the experimental site. Measured field soil water content profiles were used as a basis for the calibration of the latter two parameters.
Weather input data
Weather files for 2014 and 2015 were created using daily minimum and maximum air temperatures, rainfall, and solar radiation recorded at the experimental site and plotted using the WeatherMan utility program in DSSAT (Pickering et al., 1994). In addition, for the long-term analysis, a weather file was created for 32 years (1984–2015) of observed daily minimum and maximum temperature, solar radiation (collected at the nearest Meteo Benin synoptic weather station in Parakou, 70 km from the research site), and rainfall (collected from the experimental site in the Agricultural Research center).
Sensitivity Analysis of N Demand Under Fertilizer Microdosing
When testing different placement methods (e.g., “bottom-of-the-hole”) and depths of N-fertilizer application available in DSSAT and representative of fertilizer microdosing, the initially calibrated model had a tendency to underpredict growth and yield compared to observed values in the microdosing treatments (see Supplementary Figure S2). In particular, for the low N rates used in fertilizer microdosing, the model simulated high nitrogen stress, thereby constraining the simulated crop growth to levels not consistent with observed growth data. As a result, and based on a sensitivity analysis, the default value of the N stress coefficient CTCNP2 was selected for optimization. Higher CTCNP2 values lead to lower Ncritical values, thereby broadening the range of N concentrations which allow unrestricted growth. Based on a realistic range (0.14 to 0.22), the value of 0.20 was retained for CTCNP2.
Model Evaluation
The calibrated model was evaluated against the phenology, LAI, and above-ground biomass time series, final grain and biomass yield data from the remaining treatments in 2014 and all treatments in 2015. The accuracy of model simulations was assessed based on the predicted deviation (PD, difference between the predicted and observed values in %), root mean-square error (RMSE) (Willmott et al., 1985), relative RMSE (RRMSE), index of agreement (d), and coefficient of efficiency (E1) (Liu et al., 2014). For time series data, the performance indicators were calculated across all measurement dates. A model is judged to simulate satisfactorily when PD, RMSE, RRMSE are close to zero, and “d” and “E1” are close to 1.
Model Application: Long-Term Simulation Experiment
The effect of yearly climate variability on maize productivity and sustainability was simulated over a period of 32 years (1984–2015) using the calibrated model for microdosing (“Seasonal analysis” option in DSSAT; Hoogenboom et al., 2015). A factorial combination (4 × 4) of farmyard manure at 4 levels [0 (NM), 1 (1M), 2 (2M), and 3 (3M) t ha−1] and the 4 levels of fertilizer tested in the experiment (NF, MD1, MD2, and 100F) was implemented. Manure and fertilizer microdosing were applied as in the experiment. During each simulation year, sowing was allowed when a total rainfall in excess of 20 mm occurred over three consecutive days between June 15 and July 15 which corresponds to the normal window for maize cultivation in the study area. Each year's simulation was independent of the previous years as soil initial conditions were reinitialized 30 days prior to each planting. This was done in order to evaluate the sensitivity of the treatments to annual rainfall variability independently of any residual effects the treatments may have. Indeed, fertilizer microdosing is not meant to be a long term fertilization strategy but rather a step toward further crop intensification (Aune and Bationo, 2008). Besides, no data is currently available that would allow to properly evaluate the extent of the residual effects of point-placed fertilizers.
The seasonal analysis was evaluated by plotting the frequency distributions of maize yields and assessing the stability of the model response using an agro-climatic risk indicator (INST, inter-annual standard deviation, Akponikpè et al., 2011):
where Ysim is grain yield per year, avYsim is average grain yield over the number of cropping years and n the number of cropping years. The lower the value of INST, the more stable grain yield, hence the lower the probability of attaining extremely low (but also high) yields over the period (Akponikpè et al., 2010; MacCarthy and Vlek, 2012).
Since greater temporal instability is not necessarily indicative of a higher risk provided that economic profitability is achieved, an economic profitability, and risk assessment was performed. Economic risk was assessed and expressed in terms of probability of achieving a certain value-cost ratio (VCR) for a given treatment using the outputs of the simulation. VCR was computed as the difference in grain yield between the fertilized and the control plot multiplied by the unit market price of grain, divided by the variable costs (amendments + labor costs) using the 10 year average (2006–2015) market price of maize grain and fertilizer and labor cost estimated by direct observation in on-farm experiments (Tovihoudji et al., 2018). In the computation of VCR, the costs of the other inputs and management operations such as tillage, seed, planting, plant protection, and harvesting were assumed to be constant for all treatments. The variability of VCRs (median, min, max, and CV) and the probability of achieving a VCR ≥ 2 or 4 (considered as a lower and upper limit justifying investment in risky environments) for a given management option were used as a measure of the long-term economic sustainability (CIMMYT, 1988; Kihara et al., 2015).
Results
Climatic Conditions During the Experimental Years
Annual rainfall amounted to 1,142 mm in 2014 and 1,085 mm in 2015. From planting to harvest, the rainfall amounts of the two seasons were similar, but their distribution was slightly contrasted (Supplementary Figure S1). The rainfall amount during the growing period was 694 mm in 2014 (43 rainfall events) and 797 mm in 2015 (46 rainfall events). Maximum daily temperature during the 2014 growing season ranged from 25 to 37°C with an average value of 31°C, while minimum daily temperature ranged between 20 and 24°C with an average value of 22°C (Supplementary Figure S1). Average daily solar radiation was 18 MJ m−2 with a minimum value of 7 MJ m−2 and maximum value of 25 MJ m−2. In the 2015 season, maximum daily temperature ranged from 27 to 38°C with an average value of 33°C, while minimum daily temperature ranged from 21 to 24°C with an average of 22°C. Daily solar radiation ranged from 6 to 24 MJ m−2 with a daily average of 17 MJ m−2 (Supplementary Figure S1).
Model Response to Manure and Conventional Fertilization: Calibration and Validation
Calibration
The six genetic parameters adjusted in the present study are presented in Table 1. The range of these parameters were all close to the DSSAT “default” values for early to medium maturing maize varieties. After calibration, the model predicted the anthesis date and date of physiological maturity well (Table 2). Similarly, the predicted deviation for LAImax was low and ranged from −2 to 10% depending on the treatment (Table 2). The model accurately predicted the observed time course of LAI and aboveground biomass for the three treatments selected for calibration (Figure 1). Low RMSE values (0.07 m2 m−2 and 335 kg ha−1, respectively, for LAI and aboveground biomass across treatments and measurement dates) and high d-index values (0.99 and 0.98 for these two variables, respectively) were found (Figures 1A,B). Likewise, the simulation of the soil water content (SWC) in the top 0.4 m of the soil profile was good as shown by low RMSEs (0.01–0.02 m3 m−3) and high d-values (0.79–0.84) (Figure 1). In addition, there was generally good agreement between predicted and observed maize grain and above ground biomass yields at final harvest, with prediction deviations ranging between −1 to 8% and 5 to 16% for grain and aboveground biomass yields, respectively (Table 2).
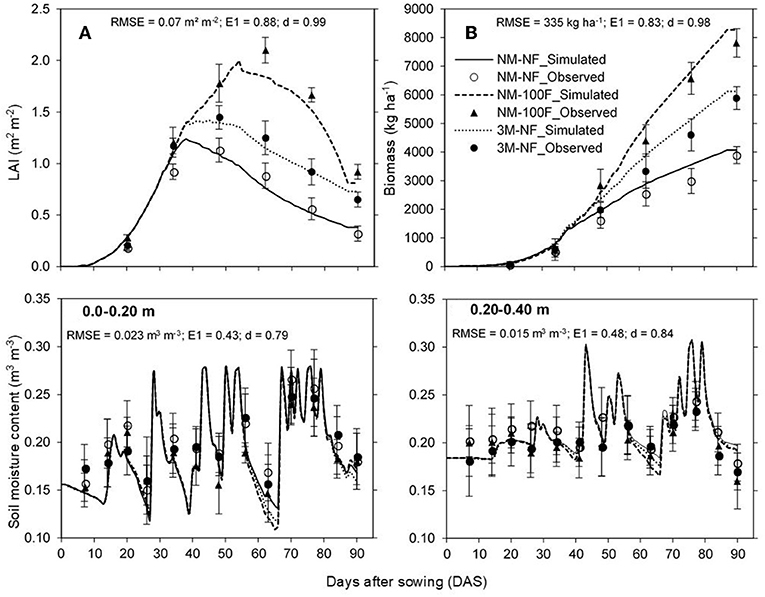
Figure 1. Comparison between observed and simulated time-series of maize LAI (Upper panel, A), above ground biomass (Upper panel, B) and soil water content in the 0.0–0.20 m and 0.20–0.40 m layers (Lower panel) during model calibration in 2014. NM, no manure; NF, no fertilizer; 100 F, 100% of the recommended fertilizer rate; 3M, farmyard manure at 3 t ha−1. Error bars, standard deviation (n = 3); RMSE, root mean-square error; E1, coefficient of efficiency; d, index of agreement.
Model Validation
After calibration, the model performance was checked with the remaining manure and broadcast fertilizer treatments in 2014 and all the manure and broadcast fertilizer treatments in 2015. The predicted days to anthesis were close to observations (55 and 56 days for simulated data against 56 and 54 days for observations during 2014 and 2015, respectively) for all treatments. Similarly for days to maturity, the deviation was 0 and −2 days between simulated and observed data during 2014 and 2015, respectively.
The simulated leaf area index (LAI) values were very close to observed values (Figure 2A), with RMSE values varying between 0.04 m2 m−2 at 20 DAS and 0.9 m2 m−2 at 62 DAS across treatments and years. On average, the RMSE was 0.12 and 0.31 m2 m−2 across treatments and measurement dates in 2014 and 2015, respectively. The corresponding d and E1 indices were 0.99 and 0.88, respectively, across treatments and measurement dates (Figure 2A). The model performance regarding above ground biomass was also good, with an absolute predicted deviation of 7–14%. RMSE values for above ground biomass increased from 25 kg ha−1 at 20 DAS to 750 kg ha−1 at 90 DAS across treatments and years. The average RMSE was 304 and 378 kg ha−1 across treatments and measurement dates in 2014 and 2015, respectively, with average relative RMSE (RRMSE), d and E1 values of 9%, 0.99, and 0.99, respectively, across treatments, measurement dates and years (Figure 2B).
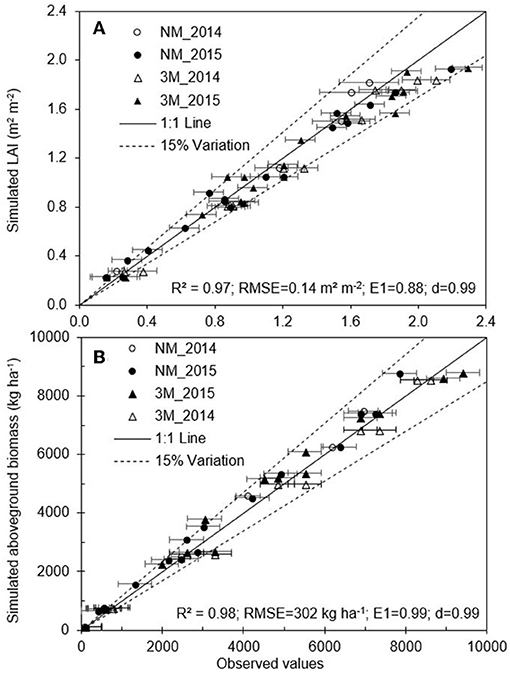
Figure 2. Model validation: comparison between observed and simulated maize LAI (A) and aboveground biomass (B) for all treatments not used during calibration (6 dates of measurement per treatment from 20 to 90 days after sowing) as affected by combined application of 0 (NM) or 3 (3M) t ha−1 of manure and 0 (NF), 50 (50 F), or 100% (100 F) of the recommended mineral fertilization rate (broadcast fertilizer) over 2 years. Error bars = standard deviation (n = 3); RMSE, root mean-square error; E1, coefficient of efficiency; d, index of agreement.
Regarding final grain and biomass yields at harvest, the model performed well in simulating the response to combined application of manure and fertilizer across the 2 years (Figure 3). For grain yield, the RMSE and RRMSE were 327 kg ha−1 and 12%, respectively (Figure 3A). The d index of agreement and model efficiency E1 were 0.96 and 0.70, respectively (Figure 3A). The model performed also well in terms of biomass yield as indicated by the low RMSE and RRMSE (569 kg ha−1 and 8%, respectively) and high d and E1 values (0.97 and 0.69, respectively; Figure 3B).
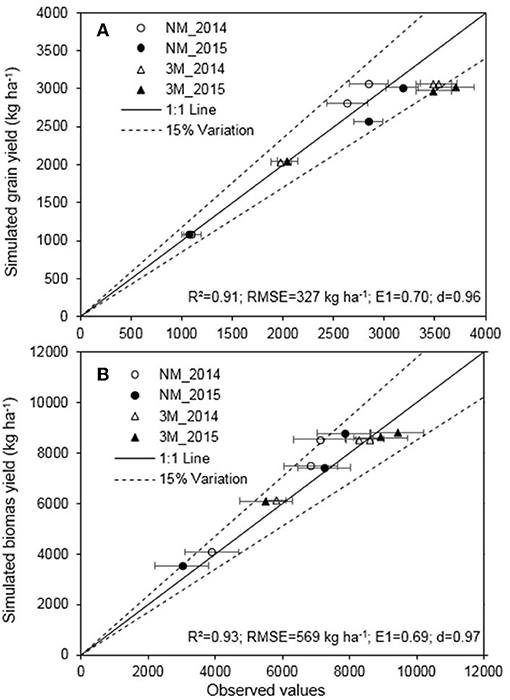
Figure 3. Model validation: comparison between observed and simulated maize grain (A) and aboveground biomass (B) yield at harvest as affected by combined application of 0 (NM) or 3 (3M) t ha−1 of manure and 0 (NF), 50 (50 F), or 100% (100 F) of the recommended mineral fertilization rate (broadcast fertilizer) over 2 years. Error bars, standard deviation (n = 3); RMSE, root mean-square error; E1, coefficient of efficiency; d, index of agreement.
Simulations showed that no treatment suffered from water deficit in both years despite some dry spells recorded in the two growing seasons, whereas excess water stress was simulated around 40–55 and 80 DAS in 2014 and 25–35 and 80 DAS in 2015 due to heavy rainfall events ≥40 mm per day recorded during those periods. The latter resulted in substantial simulated drainage and N leaching, especially in the high fertilized treatment (combined manure and fertilizer) in 2015 (data not shown). A short and long nitrogen stress period was simulated around 40 and 90 DAS, respectively, for the unfertilized treatments (NM-NF and 3M-NF) in both years (data not shown). The stress was more prominent in 2015 compared to 2014, especially at low levels of fertilization (NM-NF). The treatments with the highest fertilization rates (50 F and 100 F) were not affected by nitrogen stress but moderate N stress periods were simulated around 15 and 20 DAS in both years for the other treatments.
Sensitivity Analysis of N Demand Under Fertilizer Microdosing
After selecting the bottom-of-the-hole fertilizer-placement method at 0.10 m depth as proxy to fertilizer microdosing, the sensitivity analysis showed that the model was quite sensitive to changes in the function controlling crop N demand for both years (Figure 4). Lowering the N stress coefficient CTCNP2 by 0.02 from the default value of 0.16 caused a higher N uptake (0 to +8% depending on N rate and year) but mostly a much stronger N stress and consequent reduction in growth and yield (Figure 4). On the contrary, increasing the CTCNP2 value from 0.16 to 0.22 resulted in decreased N uptake (−19 to 0%; Figures 4A,B), and an increase in grain (0 to +33%) and biomass yields (0 to +21%; Figures 4C–F). Generally, these increases in yield led to predictions that approached those observed experimentally, with lower RMSEs (Supplementary Figure S3). Overall, aboveground biomass and grain yield predictions were found to be sensitive to the CTCNP2 coefficient only for N rates below 55 and 70 kg ha−1 in 2014 and 2015, respectively.
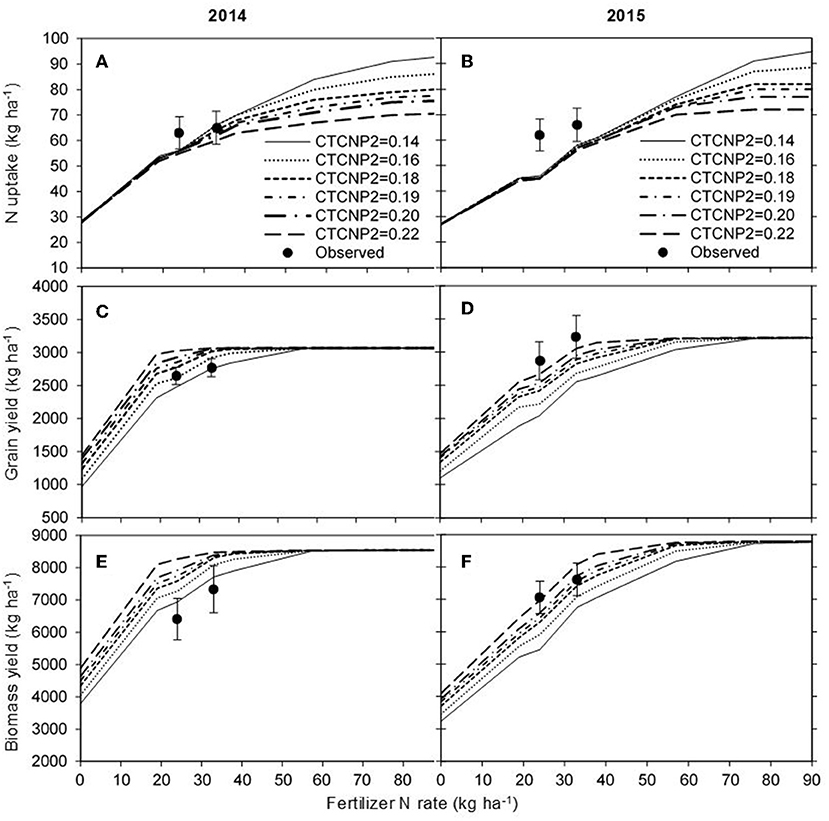
Figure 4. Sensitivity of maize aboveground N uptake (A,B), grain yield (C,D) and aboveground biomass (E,F) at harvest to incremental changes in the N stress coefficient from 0 to 90 kg ha−1 of N fertilizer rates using the hole-placement method at 0.10 m depth in 2014 (A,C,E) and 2015 (B,D,F). Error bars denote standard deviation (n = 3) for the two microdosing rates without manure (NM-MD1 and NM-MD2).
Model Testing for Fertilizer Microdosing
Following the sensitivity analysis, the CTCNP2 value was adjusted to 0.20 given the low RMSE values for the N uptake, grain and biomass yields over the 2 years (Supplementary Figure S3). This led to a reduction of the N stress and to a satisfactory agreement and model efficiency between simulated and measured values (Figure 5; Table 3). From Figures 5C,D, it can be seen that the simulated grain yield showed globally good agreement with measured data in both years [d = 0.68 and RMSE = 323 kg ha−1 (10%) across years]. The optimized model was also accurate for the aboveground biomass and N uptake as indicated by the performances indicators [RMSE and d values of 620 kg ha−1 (8%) and 0.79, and 7.1 kg ha−1 (10%) and 0.86 for aboveground biomass and N uptake, respectively, across years; Table 3]. However, the optimized model generally simulated biomass yield better than the baseline model in 2015 compared to 2014 where the opposite was observed (not shown). The latter will not affect the model use for scenario analyses since it is the grain yield data that will be used for this purpose.
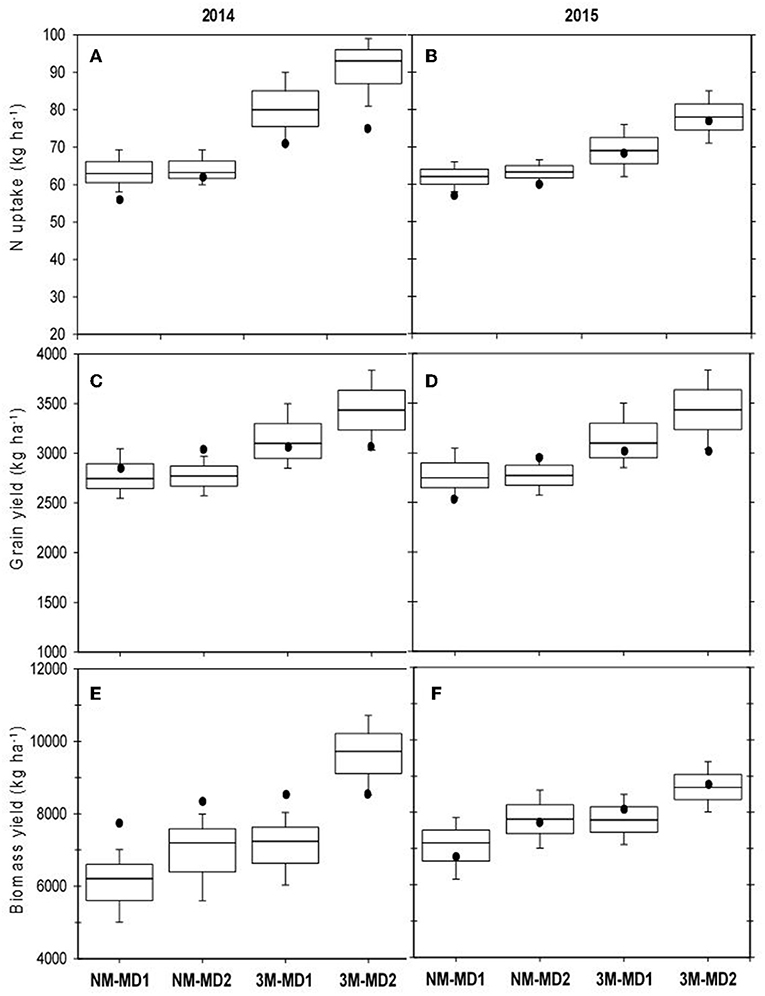
Figure 5. Comparison between observed (box-and-whisker plots) and simulated (black points) N uptake (A,B), maize grain (C,D), and aboveground biomass (E,F) yield at harvest as affected by combined application of 0 (NM) or 3 (3M) t ha−1 of manure and two microdosing rates (MD1–MD2) in 2014 and 2015 using CTCNP2 = 0.20. MD1 = microdosing option 1; MD2 = microdosing option 2.

Table 3. Statistical indicators showing the relationship between simulated and measured maize grain and biomass yield as affected by the combined application of 0 (NM) or 3 (3M) t ha−1 of manure and two microdosing rates (MD1-MD2) over the 2 years (2014–2015).
Long-Term Scenario Analysis Regarding Microdosing
The annual rainfall over the simulation period ranged between 843 and 1,472 mm (average of 1,182 ± 171 mm) while the cumulative rainfall from sowing to harvest maturity ranged between 490 and 1,030 mm (735 ± 121 mm). The highest annual rainfall amounts were observed in 2002, 2009, and 2012, while the lowest were observed in 1986 and 1987. For the cumulative rainfall from June 15 to harvest maturity, the highest amounts were observed in 1988 and 2000, and the lowest in 1984, 1986, and 1987.
Based on the long-term simulations using the optimized model, aboveground biomass, and grain yields responded similarly to fertilizer and organic amendment inputs. Hence, only grain yield data was used to perform all analyses. The cumulative probability distribution of the simulated grain yields showed that yields were consistently higher with microdosing and further enhanced when combined with manure, compared to the no input treatment (Figure 6). For instance, average grain yield increased by 1,272 kg ha−1 for MD1 without manure and by 1,458–1,647 kg ha−1 for MD1 with manure (1–3 t ha−1), respectively, compared to the no input treatment (1,398 kg ha−1). On average, the yield difference between the NF and MD or 100 F treatments tended to decrease with increasing rates of manure. In addition, the yield difference between MD1 and MD2 tended to decrease with increasing manure additions, becoming negligible for 3M (Figure 7). The average grain yield was not affected by the rate of manure application (0–3 t ha−1) for the 100 F treatment. Finally, the average yield difference between MD and 100 F tended to decrease with increasing manure additions, becoming nearly nil for 2M and 3M (Figure 7).
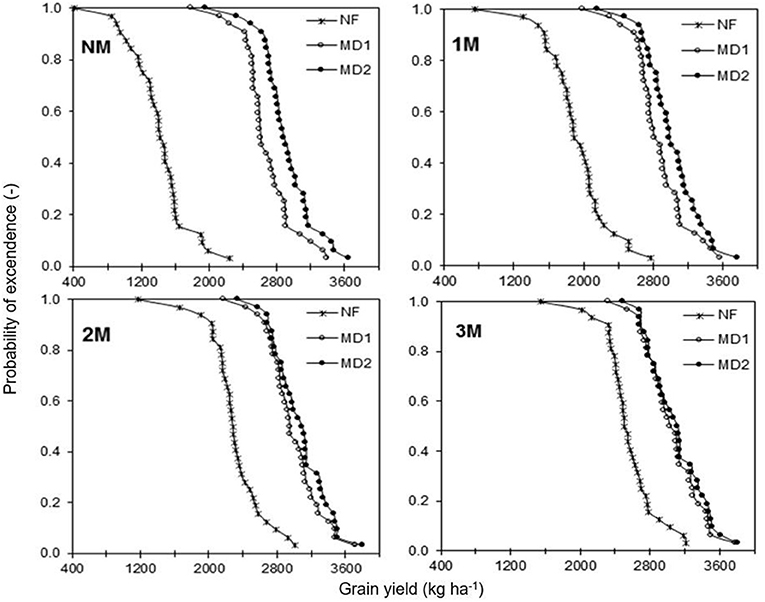
Figure 6. Frequency distributions of maize grain yields as simulated by DSSAT over a 32 year period (1984 to 2015) in response to combined application of manure and fertilizer microdosing. NF, no fertilizer; NM, no manure; MD1, microdosing option 1; MD2, microdosing option 2. 1M, 2M, and 3M correspond to the application of 1, 2, and 3 t ha−1 of manure.
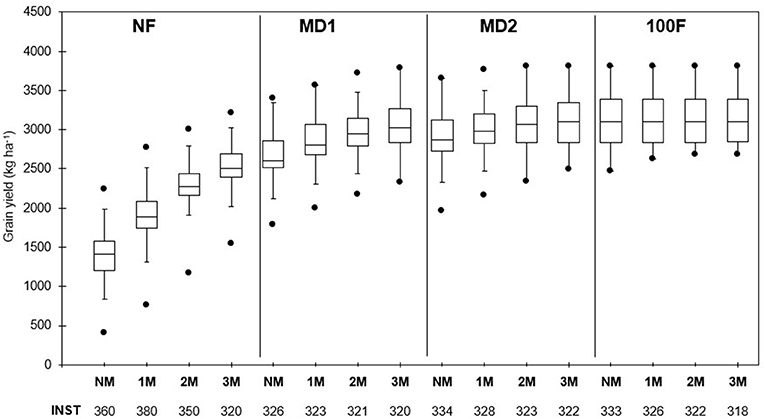
Figure 7. Maize yield variability as simulated by DSSAT over the study period (32 years-−1984 to 2015) in response to combined application of manure and fertilizer microdosing. NF, no fertilizer; NM, no manure; MD1, microdosing option 1; MD2, microdosing option 2; 100 F, 100% of the broadcasted recommended rate. 1M, 2M, and 3M correspond to the application of 1, 2, and 3 t ha−1 of manure. INST, inter-annual standard deviation.
Like the long-term average grain yields, the minimum yields also increased steadily with an increase in N inputs from manure and fertilizer. The minimum grain yields increased from 411 to 1,541 kg ha−1 in the no fertilizer treatment to 1,800–2,328 kg ha−1 in MD1 and 2,473–2,686 kg ha−1 in 100 F across manure rates (Figure 7). Thus, applying MD1 alone guarantees at least 1,800 kg ha−1 every year. At the high microdosing rate (MD2), the minimum guaranteed yield was 1,963 kg ha−1 while at the high recommended rate (100 F), the minimum guaranteed yield was 2,473 kg ha−1.
Considering the inter-annual standard deviation (INST), the variability of grain yields following microdose fertilization alone was lower or similar to the no fertilizer treatment NF (Figure 7). Applying the 100 F treatment resulted in slightly lower or similar yield variability compared to microdose fertilization. Yield variability was little affected by manure application (1–3 t ha−1).
Based on average input and output prices, microdose fertilization (alone or combined with manure) appears to be economically profitable each year when considering a VCR threshold of 2 (Table 4). Under no manure application, the VCRs ranged from 2.3 to 4.9 irrespective of microdosing rates (Table 4). Combining hill-placed manure (1–3 t ha−1) with microdosing decreased the median VCRs by 0.8 (MD1) to 1.0 (MD2) point, compared to the sole microdosing application as a result of increased labor costs, but the probability to achieve VCR ≥ 2 remained high (88–97%). Based on a VCR threshold of 4, sole microdose fertilization was noticeably less risky than microdose + manure (Table 4).
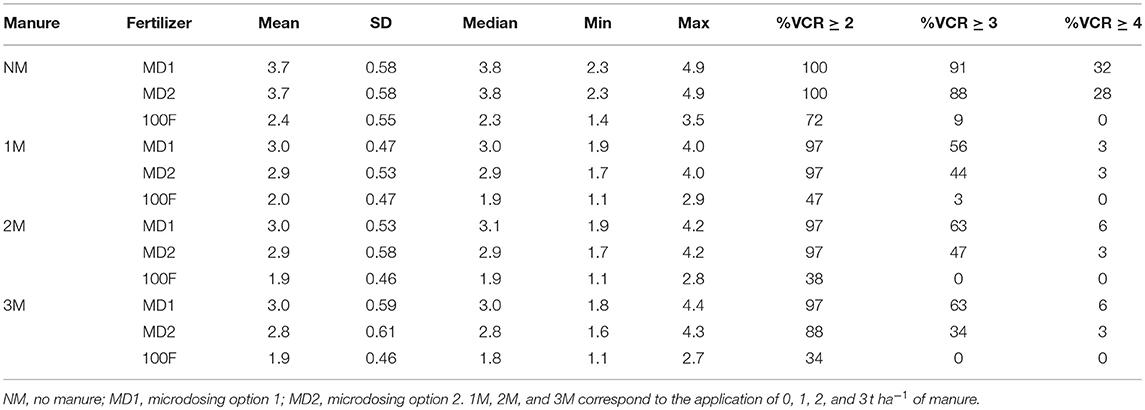
Table 4. Summary of simulated impact of seasonal climate variations (32 years−1984 to 2015) on value cost ratio (VCR) in response to the combined application of manure and fertilizer microdosing.
Discussion
Model Response to Manure and Conventional Fertilization
The model calibration resulted in good predictions of phenological stages like anthesis and maturity as indicated by the different indicators (Table 2). DSSAT also accurately simulated the changes in soil water content in the various layers of the soil profile (Figure 1). Finally, model performance regarding biomass and grain yields at harvest was good across treatments and years (Figure 3). Such good performance was achieved in spite of the fact that maize response to P and K was not considered in the simulations yet P is often a strongly limiting factor in Sub-Saharan Africa (e.g., Nziguheba et al., 2016). This good model performance may be explained by the fact that all fertilizer and manure treatments considered here included proportional amounts of P and K such that N remained the most limiting element in all treatments. Alternatively, one may consider that plant response to N in the calibrated model in fact reflects plant response to the combined additions of N, P, and K. The latter does not invalidate the model use for scenario analyses as long as treatments similar to those used for calibration are being investigated, which is the case in the present study.
DSSAT Response to Fertilizer Microdosing Practice
Using the default value of the N stress coefficient and the 0.10 m depth hole-placement setting, DSSAT tended to under-predict maize growth and yields in response to microdosing, especially for grain yield and in 2015. Fertilizer microdosing is known to increase fertilizer use efficiency compared to broadcast fertilization (Ibrahim et al., 2015). This positive effect has been attributed to faster early crop development (Hafner et al., 1993; Tabo et al., 2007; Aune and Bationo, 2008; Ibrahim et al., 2014, 2015). In addition, localized application of nutrients may promote rapid fine root and root hair proliferation (Hodge, 2004; Ibrahim et al., 2014, 2015). Ibrahim et al. (2014, 2015) have reported an increase in root dry weight, total root length, and root length density, which may result in higher plant water and nutrient uptake and lower nutrient losses by leaching. In addition, an increase in lateral roots within the upper soil layers at early growth stages could stimulate the uptake of native nutrients. Without further adjustments, DSSAT did not seem able to correctly simulate crop response to fertilizer microdosing. On the contrary, strong N stresses were simulated given the very low quantities of N supplied during microdosing.
DSSAT and the CERES-Maize models have been extensively tested in the low-input cropping systems of West Africa under broadcast fertilization conditions (e.g., Soler et al., 2011; Fosu-Mensah et al., 2012; Adnan et al., 2017a; Saïdou et al., 2017; Amouzou et al., 2018), but not under fertilizer microdosing conditions. A few studies have previously used APSIM to simulate fertilizer microdosing (Cooper et al., 2008; Twomlow et al., 2008; Turner and Rao, 2013), but none of these studies report on how microdosing was implemented in the model nor do they provide information regarding APSIM's performance. Hence the present study appears to be the first to report on the performance of a conventional soil-plant-atmosphere model in the context of fertilizer microdosing.
Following the sensitivity analysis, and given that N stress seemed to play a major role in the underestimation of maize yields in microdose treatments, the CTCNP2 value was adjusted in order to scale simulations of grain and biomass yields and N uptake to the observed values in both years (Figure 4). After optimization, model prediction at harvest matched satisfactorily with experimental observations, with a strong improvement in grain yield estimation (Figure 5; Table 3). Although adjusting the CTCNP2 factor does not capture the full complexity of the effects of fertilizer point-placement, other studies have previously had to adjust the N-stress factor in order to better model crop growth and N uptake under specific conditions (Liu et al., 2012; Yakoub et al., 2017). Adjusting the CTCNP2 factor offers a simple yet effective means of modeling the effect of microdose fertilization. Nevertheless, this approach should be confirmed in the future by testing the model against data from across a wider range of environmental and management conditions. Furthermore, it may be worthwhile in the future to investigate in more detail the physiological response mechanisms of maize to fertilizer microdosing so as to better represent them in 1-D soil-plant-atmosphere models such as DSSAT.
Long-Term Scenario Analysis and Recommendations Regarding Fertilizer Microdosing
Before widely promoting fertilizer microdosing in smallholder maize farming systems, and given that smallholder farmers are usually risk-averse, it must be demonstrated that the microdose technology does not substantially increase inter-annual variability in yield and income caused by variable rainfall conditions. The use of calibrated and validated decision support systems like DSSAT provides an efficient means to assess the long-term variability in maize yields following fertilizer microdosing. Overall, the predicted grain yields from the long-term simulations are within the ranges reported in on-farm experiments under the same microdosing treatments in northern Benin (Tovihoudji et al., 2018). This suggest that the results can be confidently used to make recommendations regarding fertilizer microdosing.
Considering the inter-annual standard deviation in grain yield (INST), applying microdose fertilization alone (MD1 or MD2) resulted in a lower variability compared to the no fertilizer input treatment (NF) but slightly higher or equal variability compared to 100 F (Figure 7). Interestingly, yield variability was little affected by manure application (1–3 t ha−1). Hence, it may be concluded that intensification strategies combining manure and microdosing appear to be less unstable (“less risky”) for smallholder farmers compared to the sole application of manure or microdosing. Equally interesting, applying microdosing alone guarantees at least 1,800 kg ha−1 every year without inducing additional inter-annual variability.
However, in smallholder subsistence farming, the economic risk associated with the adoption of a new technology are more important than temporal yield stability. The results show that combining microdosing with manure increases the economic risk. Indeed, while SD values are similar, the mean VCR is lower for manure + microdose compared to microdose alone (Table 4). Nevertheless, a VCR of 2 is reached in almost 100% of the years, indicating that the level of risk associated with manure + microdose remains acceptable. Only if farmers have a very strong aversion for risk (e.g., require a VCR of 3 or 4; CIMMYT, 1988), does one see a notable short-term advantage for microdosing compared to the combined application of fertilizer and manure. This is because of the added labor costs related to hill-placed manure application. For instance, a VCR ≥ 3 is reached 88–91% of the time under sole microdose fertilization, compared to 34–63% when combined with manure (Table 4). Even though the application of 100 F treatment resulted in a slightly lower yield variability than microdose fertilizer, the economic risk is much higher since a VCR of 2 could be achieved in only 34–72% of the years and a VCR of 3 in only 0–9% of the years depending on the rate of manure.
Model simulations do not take into account the long-term cumulative impacts of the technologies on the soil. Further experimentation is needed to assess the extent of these residuals effects in the special case of point-placed fertilizers and manure. Nevertheless, complementing microdose fertilization with manure would be recommended for soil quality considerations and to increase the long-term sustainability of the system even though it may compromise the short-term benefits. Although combining fertilizer microdosing and manure increases the total amount of N applied and hence the risk of N leaching, this risk remains limited since N balances generally remain negative for the rates tested here as was shown by Tovihoudji et al. (2017). Finally, it may be preferable for farmers to apply the lower microdosing rate (MD1) irrespective of the manure application rate because the VCR values can depend greatly on fluctuations in input and output market prices within and between seasons (Tovihoudji et al., 2018).
Conclusion
In this study, we examined the ability of the DSSAT CERES-Maize model to accurately simulate maize response to fertilizer microdosing, and whether the validated model can be used to assess the effects of seasonal climate variability on maize productivity and economic risk. Using independent datasets for the calibration and validation, DSSAT exhibited good performance when simulating phenological stages, LAI, total biomass, grain yield and total N uptake, capturing the whole range of these variables, for conventional fertility management practices (broadcast fertilizer). For fertilizer microdosing, the N stress coefficient (CTCNP2) needed to be adjusted to avoid the occurrence of large N stresses during simulation. After optimization, the model could adequately reproduce grain yields for fertilizer microdosing, indicating that it could be used as decision support tools through long-term scenario analysis. The 32 year, long-term simulation with the validated model showed that the application of 2 g of NPK15−15−15 fertilizer + 1 g urea per hill (equivalent to 23.8 kg N ha−1, 4.1 kg P ha−1, and 7.8 kg K ha−1) improved both the long-term average and the minimum guaranteed yield without increasing inter-annual variability and the economic risk compared to unfertilized plots. Combining the application of fertilizer microdosing with hill-placed manure (1–3 t ha−1) consistently reduced the inter-annual yield variability. Even though combining the application of fertilizer microdosing with hill-placed manure was economically slightly riskier than microdose fertilizer alone, this risk remained low since a VCR of 2 could be achieved in almost 100% of the years. Considering this as well as the other benefits of manure for soil health, combining fertilizer microdosing with small quantities of manure would be recommended to increase the sustainability of the system. Besides additional validation on the basis of broader datasets, more in-depth investigations of the physiological response mechanisms of crops to fertilizer microdosing should be carried out so as to better represent them in 1-D soil-plant-atmosphere models such as DSSAT.
Author Contributions
PT conducted most of the field experiments as well as simulations in this publication as part of his Master and Doctorate research. He was responsible for most of the manuscript preparation and is the lead author. PA is PT's co-supervisor and he helped in providing major insight into both experimental and simulated outputs. He helped in manuscript preparation and provided major input into soil and weather information. EA is also a member of the supervisory team. He gave major inputs into understanding the outcomes of experimental and modeled output. He also helped in manuscript preparation and gave deeper understanding of sensitivity analysis output. CB is PT's major supervisor and helped in providing major insight into both experimental and simulated outputs, advise throughout manuscript preparation.
Funding
This study was made possible through a doctoral grant from the West Africa Agricultural Productivity Program (WAAPP-Benin) and a fellowship from the Université catholique de Louvain (UCL).
Conflict of Interest Statement
The authors declare that the research was conducted in the absence of any commercial or financial relationships that could be construed as a potential conflict of interest.
Acknowledgments
We are also grateful to the staff of Agricultural Research Centre of Northern Benin (CRA-Nord) for their technical help.
Supplementary Material
The Supplementary Material for this article can be found online at: https://www.frontiersin.org/articles/10.3389/fenvs.2019.00013/full#supplementary-material
References
Adnan, A. A., Jibrin, J. M., Abdulrahman, B. L., Shaibu, A. S., and Garba, I. I. (2017b). CERES–maize model for determining the optimum planting dates of early maturing maize varieties in Northern Nigeria. Front. Plant Sci. 8:1118. doi: 10.3389/fpls.2017.01118
Adnan, A. A., Jibrin, J. M., Kamara, A. Y., Abdulrahman, B. L., and Shaibu, A. S. (2017a). Using CERES-Maize model to determine the nitrogen fertilization requirements of early maturing maize in the Sudan Savanna of Nigeria. J. Plant Nutr. 40, 1066–1082. doi: 10.1080/01904167.2016.1263330
Akponikpè, P. B. I., Gerard, B., Michels, K., and Bielders, C. L. (2010). Use of the APSIM model in long-term simulation to support decision making regarding nitrogen management for pearl millet in the Sahel. Eur. J. Agronomy 32, 144–154. doi: 10.1016/j.eja.2009.09.005
Akponikpè, P. B. I., Minet, J., Gerard, B., Defourny, P., and Bielders, C. L. (2011). Spatial fields' dispersion as a farmer strategy to reduce agro-climatic risk at the household level in pearl millet-based systems in the Sahel: a modeling perspective. Agric. Forest Meteorol. 151, 215–227. doi: 10.1016/j.agrformet.2010.10.007
Amouzou, K. A., Naab, J. B., Lamers, J. P., and Becker, M. (2018). CERES-Maize and CERES-Sorghum for modeling growth, nitrogen and phosphorus uptake, and soil moisture dynamics in the dry savanna of West Africa. Field Crops Res. 217, 134–149. doi: 10.1016/j.fcr.2017.12.017
Aune, J. B., and Bationo, A. (2008). Agricultural intensification in the Sahel–the ladder approach. Agric. Syst. 98, 119–125. doi: 10.1016/j.agsy.2008.05.002
Aune, J. B., Doumbia, M., and Berthe, A. (2007). Microfertilizing sorghum and pearl millet in Mali – agronomic, economic and social feasibility. Outlook Agric. 36, 199–203. doi: 10.5367/000000007781891504
Bationo, A., and Waswa, B. (2011). “New challenges and opportunities for integrated soil fertility management in Africa,” in Innovations as Key to the Green Revolution in Africa, eds A. Bationo B. S. Waswa, J. M. Okeyo, J. M. Kihara, and F. Maina (Dordrecht: Springer), 3–17.
Camara, B. S., Camara, F., Berthe, A., and Oswald, A. (2013). Micro-dosing of fertilizer - a technology for farmers' needs and resources. Int. J. AgriSci. 3, 387–399.
CIMMYT. (1988). From Agronomic Data to Farmer Recommendations: An Economics Training Manual. Mexico, DF: CIMMYT.
Comoé, H., and Siegrist, M. (2015). Relevant drivers of farmers' decision behavior regarding their adaptation to climate change: a case study of two regions in Côte d'Ivoire. Mitigat. Adapt. Strategies Global Change 20, 179–199. doi: 10.1007/s11027-013-9486-7
Cooper, P. J. M., Dimes, J., Rao, K. P. C., Shapiro, B., Shiferaw, B., and Twomlow, S. (2008). Coping better with current climatic variability in the rain-fed farming systems of sub-Saharan Africa: an essential first step in adapting to future climate change? Agric. Ecosyst. Environ. 126, 24–35. doi: 10.1016/j.agee.2008.01.007
Corbeels, M., Chirat, G., Messad, S., and Thierfelder, C. (2016). Performance and sensitivity of the DSSAT crop growth model in simulating maize yield under conservation agriculture. Eur. J. Agronomy 76, 41–53. doi: 10.1016/j.eja.2016.02.001
FAOSTAT. (2016). FAO Statistical Database: Production and Trade. Available online at: http://www.fao.org/faostat/en/#data/QC
Folberth, C., Yang, H., Gaiser, T., Abbaspour, K. C., and Schulin, R. (2013). Modeling maize yield responses to improvement in nutrient, water and cultivar inputs in sub-Saharan Africa. Agric. Syst. 119, 22–34. doi: 10.1016/j.agsy.2013.04.002
Fosu-Mensah, B. Y., MacCarthy, D. S., Vlek, P. L. G., and Safo, E. Y. (2012). Simulating impact of seasonal climatic variation on the response of maize (Zea mays L.) to inorganic fertilizer in sub-humid Ghana. Nutr. Cycling Agroecosyst. 94, 255–271. doi: 10.1007/s10705-012-9539-4
Gijsman, A. J., Hoogenboom, G., Parton, W. J., and Kerridge, P. C. (2002). Modifying DSSAT crop models for low-input agricultural systems using a soil organic matter–residue module from CENTURY. Agronomy J. 94, 462–474. doi: 10.2134/agronj2002.4620
Gnanglè, C. P., Glèlè Kakaï, R., Assogbadjo, A. E., Vodounnon, S., Yabi, J. A., and Sokpon, N. (2011). Tendances climatiques passées, modélisation, perceptions et adaptations locales au Bénin. Climatologie 8, 27–40. doi: 10.4267/climatologie.259
Guan, K., Sultan, B., Biasutti, M., Baron, C., and Lobell, D. B. (2017). Assessing climate adaptation options and uncertainties for cereal systems in West Africa. Agric. Forest Meteorol. 232, 291–305. doi: 10.1016/j.agrformet.2016.07.021
Hafner, H., George, E., Bationo, A., and Marschner, H. (1993). Effect of crop residues on root growth and phosphorus acquisition of pearl millet in an acid sandy soil in Niger. Plant Soil 150, 117–127. doi: 10.1007/BF00779182
Henao, J., and Baanante, C. (2006). Agricultural Production and Soil Nutrient Mining in Africa: Implications for Resource Conservation and Policy Development. International Fertilizer Development Center (IFDC), Muscle Shoals, AL.
Hodge, A. (2004). The plastic plant: root responses to heterogeneous supplies of nutrients. New Phytol. 162, 9–34. doi: 10.1111/j.1469-8137.2004.01015.x
Holzworth, D. P., Huth, N. I., deVoil, P. G., Zurcher, E. J., Herrmann, N. I., McLean, G., et al. (2014). APSIM–evolution towards a new generation of agricultural systems simulation. Environ. Model. Softw. 6, 327–350. doi: 10.1016/j.envsoft.2014.07.009
Hoogenboom, G., Jones, J. W., Wilkens, P. W., Porter, C. H., Boote, K. J., and Hunt, L. A. (2015). Decision Support System For Agrotechnology Transfer (DSSAT). Version 4.6. DSSAT Foundation, Prosser, WA.
Ibrahim, A., Abaidoo, R. C., Fatondji, D., and Opoku, A. (2015). Hill placement of manure and fertilizer micro-dosing improves yield and water use efficiency in the Sahelian low input millet-based cropping system. Field Crops Res. 180, 29–36. doi: 10.1016/j.fcr.2015.04.022
Ibrahim, A., Pasternak, D., and Fatondji, D. (2014). Impact of depth of placement of mineral fertilizer micro-dosing on growth, yield and partial nutrient balance in pearl millet cropping system in the Sahel. J. Agric. Sci. 153, 1412–1421. doi: 10.1017/S0021859614001075
Igué, A. M., Adjanohoun, A., Saïdou, A., Ezui, G., Attiogbe, P., Kpagbin, et al. (2013). Application et adaptation de l'approche intégrée DSSAT-SIG à la formulation des doses d'engrais pour la culture du maïs au Sud et au centre du Benin. Bull. Recherche Agron. Bénin 2013, 24–33.
Jones, C. A., and Kiniry, J. R. (1986). CERES-Maize. A Simulation Model of Maize Growth and Development. Texas, TX: A&M University Press.
Jones, J. W., Hoogenboom, G., Porter, C. H., Boote, K. J., Batchelor, W. D., Hunt, L. A., et al. (2003). The DSSAT cropping system model. Eur. J. Agron. 18, 235–265. doi: 10.1016/S1161-0301(02)00107-7
Keating, B. A., Carberry, P. S., Hammer, G. L., Probert, M. E., Robertson, M. J., Holzworth, D., et al. (2003). An overview of APSIM, a model designed for farming systems simulation. Eur. J. Agron. 18, 267–288. doi: 10.1016/S1161-0301(02)00108-9
Kihara, J., Huising, J., Nziguheba, G., Waswa, B. S., Njoroge, S., Kabambe, V., et al. (2015). Maize response to macronutrients and potential for profitability in sub-Saharan Africa. Nutr. Cycling Agroecosyst. 105, 171–181. doi: 10.1007/s10705-015-9717-2
Liu, H. L., Yang, J., He, P., Bai, Y., Jin, J., Drury, C. F., et al. (2012). Optimizing parameters of CSM-CERES-maize model to improve simulation performance of maize growth and nitrogen uptake in Northeast China. J. Integr. Agric. 11, 1898–1913. doi: 10.1016/S2095-3119(12)60196-8
Liu, S., Yang, J. Y., Drury, C. F., Liu, H. L., and Reynolds, W. D. (2014). Simulating maize (Zea mays L.) growth and yield, soil nitrogen concentration, and soil water content for a long-term cropping experiment in Ontario, Canada. Can. J. Soil Sci. 94, 435–452. doi: 10.4141/cjss2013-096
MacCarthy, D. S., Adiku, S. G. K., Freduah, B. S., Gbefo, F., and Kamara, A. Y. (2017). Using CERESMaize and ENSO as decision support tools to evaluate climate-sensitive farm management practices for maize production in the northern regions of Ghana. Front. Plant Sci. 8:31. doi: 10.3389/fpls.2017.00031
MacCarthy, D. S., and Vlek, P. L. G. (2012). Impact of climate change on sorghum production under different nutrient and crop residue management in semi-arid region of Ghana: a modeling perspective. Afr. Crop Sci. J. 20, 275–291. Available online at: https://www.ajol.info/index.php/acsj/article/view/81717
MacCarthy, D. S., Vlek, P. L. G., Bationo, A., Tabo, R., and Fosu, M. (2010). Modeling nutrient and water productivity of sorghum in smallholder farming systems in a semi-arid region of Ghana. Field Crops Res. 118, 251–258. doi: 10.1016/j.fcr.2010.06.005
MacCarthy, D. S., Vlek, P. L. G., and Fosu–Mensah, B. Y. (2012). “The response of maize to N fertilization in a sub–humid region of ghana; understanding the processes using a crop simulation model,” in Improving Soil Fertility Recommendations in Africa Using the Decision Support Systems for Agrotechnology Transfer (DSSAT), eds J. Kihara, D. Fatondji, J. W. Jones, G. Hoogenboom, R. Tabo, and A. Bationo (Dordrecht: Springer Science + Business Media, B. V), 61–75. doi: 10.1007/978-94-007-2960-5_5
Marteau, R., Sultan, B., Moron, V., Alhassane, A., Baron, C., and Traoré, S. B. (2011). The onset of the rainy season and farmers' sowing strategy for pearl millet cultivation in Southwest Niger. Agric. Forest Meteorol. 151, 1356–1369. doi: 10.1016/j.agrformet.2011.05.018
Morris, M., Kelley, V. A., Kopicki, R. J., and Byerlee, D. (2007). Fertilizer Use in African Agriculture: Lessons Learned and Good Practice Guidelines. Working Paper No. 39037. Washington, DC: World Bank. p. 162. doi: 10.1596/978-0-8213-6880-0
Muehlig-Versen, B., Buerkert, A., Bationo, A., and Roemheld, V. (2003). Phosphorus placement on acid arenosols of the west African Sahel. Exp. Agric. 39, 307–325. doi: 10.1017/S0014479703001261
Ngwira, A. R., Aune, J. B., and Thierfelder, C. (2014). DSSAT modelling of conservation agriculture maize response to climate change in Malawi. Soil Tillage Res. 143, 85–94. doi: 10.1016/j.still.2014.05.003
Nziguheba, G., Zingore, S., Kihara, J., Merckx, R., Njoroge, S., Otinga, A., et al. (2016). Phosphorus in smallholder farming systems of Sub-Saharan Africa: implications for agricultural intensification. Nutr. Cycling Agroecosyst. 104, 321–340. doi: 10.1007/s10705-015-9729-y
Okebalama, C. B., Safo, E. Y., Yeboah, E., Abaidoo, R. C., and Logah, V. (2016). Fertilizer microdosing in the humid forest zone of ghana: an efficient strategy for increasing maize yield and income in smallholder farming. Soil Sci. Soc. Am. J. 80, 1254–1261. doi: 10.2136/sssaj2016.03.0065
Pickering, N. B., Hansen, J. W., Jones, J. W., Wells, C. M., Chan, V. K., and Godwin, D. C. (1994). WeatherMan: a utility for managing and generating daily weather data. Agronomy J. 86, 332–337. doi: 10.2134/agronj1994.00021962008600020023x
Rezzoug, W., Gabrielle, B., Suleiman, A., and Benabdeli, K. (2008). Application and evaluation of the DSSAT-wheat in the Tiaret region of Algeria. Afr. J. Agric. Res. 3, 284–296.
Ritchie, J. T. (1998). “Soil water balance and plant water stress,” in Understanding Options for Agricultural Production, eds G. Y. Tsuji, G. Hoogenboom, and P. K. Thornton (Dordrecht: Kluwer Academic Publishers), 41–54.
Saïdou, A., Balogoun, I., Ahoton, E. L., Igué, A. M., Youl, S., Ezui, G., et al. (2017). Fertilizer recommendations for maize production in the South Sudan and Sudano-Guinean zones of Benin. Nutr. Cycling Agroecosyst. 110, 361–373. doi: 10.1007/s10705-017-9902-6
Sime, G., and Aune, J. B. (2014). Maize response to fertilizer dosing at three sites in the Central Rift Valley of Ethiopia. Agronomy 4, 436–451. doi: 10.3390/agronomy4030436
Soler, C. T., Bado, V. B., Traore, K., Bostick, W. M., Jones, J. W., and Hoogenboom, G. (2011). Soil organic carbon dynamics and crop yield for different crop rotations in a degraded ferruginous tropical soil in a semi-arid region: a simulation approach. J. Agric. Sci. 149, 579–593. doi: 10.1017/S0021859611000050
Tabo, R., Bationo, A., Gerard, B., Ndjeunga, J., Marchal, D., Amadou, B., et al. (2007). “Improving cereal productivity and farmers' income using a strategic application of fertilizers in West Africa,” in Advances in Integrated Soil Fertility Management in Sub-Saharan Africa: Challenges and Opportunities, eds A. Bationo, B. Waswa, J. Kihara, and J. Kimetu (Netherlands: Springer), 201–208.
Tittonell, P., and Giller, K. E. (2013). When yield gaps are poverty traps: The paradigm of ecological intensification in African smallholder agriculture. Field Crops Res. 143, 76–90. doi: 10.1016/j.fcr.2012.10.007
Tovihoudji, P. G. (2018). Improving Maize Productivity in Northern Benin Through Localized Placement of Amendments and Fertilizers. PhD dissertation thesis, Université catholique de Louvain, Belgium.
Tovihoudji, P. G., Akponikpè, P. B. I., Agbossou, E. K., Bertin, P., and Bielders, C. L. (2017). Fertilizer microdosing enhances maize yields but may exacerbate nutrient mining in maize cropping systems in northern Benin. Field Crops Res. 213, 130–142. doi: 10.1016/j.fcr.2017.08.003
Tovihoudji, P. G., Akponikpè, P. B. I., Agbossou, E. K., and Bielders, C. L. (2018). Variability in Maize Yield and Profitability Following Hill-Placement of Mineral Fertilizer and Manure Under Smallholder Farm Conditions in Northern Benin. Field Crops Research.
Turner, N. C., and Rao, K. P. C. (2013). Simulation analysis of factors affecting sorghum yield at selected sites in eastern and southern Africa, with emphasis on increasing temperatures. Agric. Syst. 121, 53–62. doi: 10.1016/j.agsy.2013.06.002
Twomlow, S., Mugabe, F. T., Mwale, M., Delve, R., Nanja, D., Carberry, P., et al. (2008). Building adaptive capacity to cope with increasing vulnerability due to climatic change in Africa–A new approach. Phys. Chem. Earth Parts A/B/C 33, 780–787. doi: 10.1016/j.pce.2008.06.048
USDA-Soil Conservation Service (1972). “Soil series of the United States, Puerto Rico and the Virgin Islands: their taxonomic classification,” in Supplement to Agricultural Handbook No. 436 (Washington, DC).
Vanlauwe, B., Six, J., Sanginga, N., and Adesina, A. A. (2015). Soil fertility decline at the base of rural poverty in sub-Saharan Africa. Nat. Plants 1:15101. doi: 10.1038/nplants.2015.101
Willmott, C. J., Ackleson, G. S., Davis, R. E., Feddema, J. J., Klink, K. M., Legates, D. R., et al. (1985). Statistics for the evaluation and comparison of models. J. Geophys. Res. 90, 8995–9005. doi: 10.1029/JC090iC05p08995
Yakoub, A., Lloveras, J., Biau, A., Lindquist, J. L., and Lizaso, J. I. (2017). Testing and improving the maize models in DSSAT: development, growth, yield, and N uptake. Field Crops Res. 212, 95–106. doi: 10.1016/j.fcr.2017.07.002
Yi, Z. X., Wang, P., Zhang, H. F., Shen, L. X., Liu, M., and Dai, M. H. (2006). Effects of type and application rate of nitrogen fertilizer on source-sink relationship in summer maize in North China Plain. Plant Nutr. Fertil. Sci. 12, 294–300.
Youssouf, I., and Lawani, M. (2002). “Les sols béninois : classification dans la base de référence mondiale,” in Quatorzième réunion du sous-comité ouest et centre africain de corrélation des sols pour la mise en valeur des terres, 9-13 octobre 2000, Abomey, Bénin. Rapport sur les Ressources en Sols du Monde n° 98 (Rome: FAO), 29–50.
Keywords: CERES-Maize, model, decision support, fertilizer microdosing, manure, inter-annual yield variability, economic risk
Citation: Tovihoudji PG, Akponikpè PBI, Agbossou EK and Bielders CL (2019) Using the DSSAT Model to Support Decision Making Regarding Fertilizer Microdosing for Maize Production in the Sub-humid Region of Benin. Front. Environ. Sci. 7:13. doi: 10.3389/fenvs.2019.00013
Received: 23 May 2018; Accepted: 18 January 2019;
Published: 06 February 2019.
Edited by:
Eike Luedeling, Universität Bonn, GermanyReviewed by:
Inacio de Barros, Empresa Brasileira de Pesquisa Agropecuária (EMBRAPA), BrazilManoj Shrivastava, Indian Agricultural Research Institute (ICAR), India
Copyright © 2019 Tovihoudji, Akponikpè, Agbossou and Bielders. This is an open-access article distributed under the terms of the Creative Commons Attribution License (CC BY). The use, distribution or reproduction in other forums is permitted, provided the original author(s) and the copyright owner(s) are credited and that the original publication in this journal is cited, in accordance with accepted academic practice. No use, distribution or reproduction is permitted which does not comply with these terms.
*Correspondence: Pierre G. Tovihoudji, cGllcnJldG92aWhvdWRqaUB5YWhvby5mcg==