Distribution of Landscape Units Within Catchments Influences Nutrient Export Dynamics
- 1INRA, UMR1069 SAS, Agrocampus Ouest, Rennes, France
- 2Department of Plant and Wildlife Sciences, Brigham Young University, Provo, UT, United States
- 3E.A. 6293 GéoHydrosystèmes Continentaux, University François Rabelais, Tours, France
Excess nutrients from agriculture have caused persistent eutrophication in aquatic ecosystems worldwide. Here, we present a conceptual framework for landscape management to achieve one or several water quality targets along the river continuum from headwaters to estuaries. Based on monitoring of representative headwaters and downstream reaches, we divide catchments into elementary landscape units defined by ecosystem properties and anthropogenic land use. We use a theoretical simulation to evaluate our hypothesis that the water-quality responses of redistributing these elementary units within the catchment will vary depending on the water quality targets (e.g., reduction in concentration or load). This landscape unit distribution (LUD) framework can efficiently assess the current ecohydrological functioning of a catchment and provide simple but robust predictions of its response to landscape management changes. Using simulated data, we show that different scenarios of landscape redistribution can allow attainment of one or several, but often not all desired water quality targets. Therefore, we recommend that water quality targets must be clearly defined and prioritized prior to designing landscape management strategies.
Introduction
Nutrient pollution of freshwater and estuarine water bodies is one of the most urgent global environmental crises, second only to loss of biodiversity by some estimations (Steffen et al., 2015). Nutrient loading from agricultural and urban activity is projected to increase through the middle of the century (Seitzinger et al., 2010; Sinha et al., 2017), further straining aquatic ecosystems that provide critical habitat and essential ecosystem services for human water consumption, recreation, and health (Le Moal et al., 2019). While substantial resources have been invested to address eutrophication at local to international levels, results have been underwhelming in many areas (Bouraoui and Grizzetti, 2011). Some of this unsatisfactory progress may simply be due to time lags between implementation of mitigation measures and improvements in water quality at the catchment scale (Vero et al., 2017; Dupas et al., 2018; Van Meter et al., 2018). However, there is growing evidence that environmental and agricultural goals are sometimes mutually exclusive (Withers et al., 2014; Doody et al., 2016).
To implement well-informed nutrient mitigation plans at the catchment scale requires conceptual representations of landscape systems that are understandable for policy makers without disregarding the systems' complexity (Bol et al., 2018). Previous conceptual frameworks have described catchments as temporally dynamic mosaics of nutrient sources and sinks (Heathwaite et al., 2000; Mcclain et al., 2003; Abbott et al., 2016, 2018). Because the spatial configuration of these source and sink patches can affect lateral transport, some authors have advocated a spatially explicit representation of landscape elements in models (e.g., Mineau et al., 2015; Vinatier et al., 2016; Musolff et al., 2017), while others have suggested semi-distributed models as a more viable approach especially in contexts with scarce data (e.g., Hrachowitz et al., 2016; Helton et al., 2018; Wollheim et al., 2018).
In headwater catchments, where a large part of a catchment's annual load is typically generated (Alexander et al., 2007; Bishop et al., 2008; Bol et al., 2018; Wollheim et al., 2018), nutrient export results from complex interactions among agricultural activities (e.g., farming systems and management) and ecosystem characteristics (e.g., climate, soil, geology, topography). As these headwater nutrient signals propagate through surface-water networks, mixing and transformation processes can strongly influence timing and magnitude of nutrient loading (Creed et al., 2015; Minaudo et al., 2015). Just as nutrient export regimes can be monitored and described in representative headwater catchments, their propagation downstream can be predicted with seasonal mass balance models and the effect of different spatial arrangement of landscape units can be assessed (Dupas et al., 2017; Helton et al., 2018).
In this context, we present a semi-distributed framework that evaluates nutrient signals in headwater catchments and their propagation through sensitive surface water networks for different landscape unit distributions (LUD). This LUD framework integrates previous conceptual frameworks describing terrestrial and aquatic ecosystems (Mineau et al., 2015; Hrachowitz et al., 2016; Helton et al., 2018; Wollheim et al., 2018). It considers the spatial distribution of landscape units in relation to the river network to assess the current ecohydrological functioning of the catchment and to predict its response to changes in landscape management. We present the four main steps of the LUD framework (section Presentation of the landscape unit distribution (LUD) framework) with simple simulations from a virtual catchment (section Virtual catchment) and explore how this approach could inform monitoring, modeling, and management. Specifically, we explore how landscape composition and landscape spatial distribution, described in a semi-distributed model, can influence nutrient loads and intra-annual dynamics.
Virtual Catchment
The LUD framework assumes that a limited number of combinations of dominant land uses, management types, and ecosystem properties can be identified and monitored in representative headwaters. LUD then summarizes the temporal dynamics of these archetypical systems into metrics of nutrient export regime. For simplicity, we illustrate the LUD framework with a virtual catchment comprised of four discrete landscape units, which are present in equal proportions in scenario S0 (Figure 1). These units are defined by the combination of:
• Two main ecosystem types, defined by soil, climate, topography, etc. These ecosystem characteristics determine the intrinsic vulnerability of a catchment to nutrient losses, equivalent to the “buffering capacity” concept of Doody et al. (2016). In the illustrative example, ecosystem characteristics (represented by the subsurface color in Figure 1) are organized spatially, resulting in higher discharge variability and higher nutrient buffering capacity in the upper part of the catchment. The buffering capacity of a subcatchment may be nutrient-specific.
• Two main types of land use and management (represented by the surface color in Figure 1). Here, they are discretized according to the intensity of nutrient loading, named as “high” in yellow or “low” in green, which determines the mean nutrient concentration. High and low nutrient loadings can refer to contrasting land use types (e.g., agricultural land vs. forest) or contrasting farming systems (e.g., arable vs. dairy farming systems) and management (e.g., fertilizer application, management of landscape buffer zones) in agricultural areas.
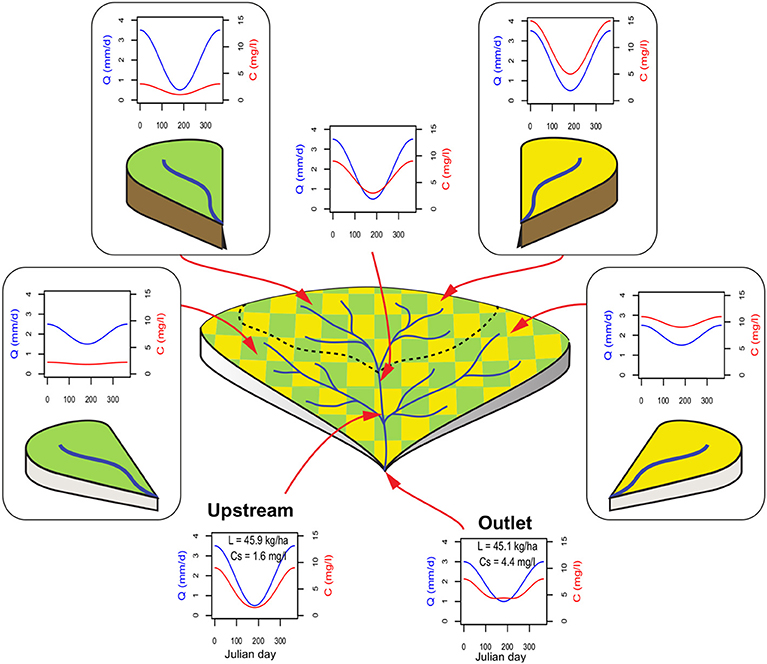
Figure 1. Conceptual illustration of the landscape unit distribution (LUD) framework for reference scenario S0. Synthetic discharge (Q) and concentration (C) time series are shown for four different landscape units and the overall catchment, which is a product of signal propagation through a virtual river network. Landscape units are defined by their ecosystem properties, represented by the subsurface color and by the intensity of nutrient loading, represented by the surface color.
While a real catchment would certainly contain more than four types of landscape units, we argue that landscape units may not be overly numerous because, (i) some combinations of ecosystem context and land use do not exist in the real world (e.g., soil types that are unsuitable for a specific land use, Thomas et al., 2016); and (ii) different factors of the ecosystem context often covary (Wollschläger et al., 2017) such as precipitation and topography.
In our simulation, the four landscape units are drained by a river network divided into an upper portion and a lower portion. Only nutrient loads from the upper portion are subjected to seasonal in-stream retention because of their long transit distance in the main river while nutrient loads in the lower section are delivered close to the catchment outlet (Wollheim et al., 2008; Helton et al., 2018).
Production of synthetic concentration and discharge time series for a virtual catchment
In the virtual catchment, which serves as an illustrative example, we considered a situation where seasonal variability of concentration and discharge are higher than long-term trends, interannual variations, runoff events, or diel cycles. Accordingly, we generated the concentration (C) and discharge (Q) time series in headwaters via a cosine function with three parameters: the mean C or Q, the amplitude of the seasonal variation, and a phase coefficient. We considered that C and Q are in phase with annual minima during summer (Julian day 182–183), which is a simplification as C can be opposite to Q in some situations (e.g., Dupas et al., 2017; Moatar et al., 2017) or seasonal hysteresis between C and Q can occur (e.g., Aubert et al., 2013). Discharge time series are considered to depend only on the ecosystem characteristics of the landscape type (same mean discharge but larger seasonal amplitude in headwaters of the upper part of the catchment compared to the lower part). In-stream retention is also described with a cosine function, with annual maxima during summer (50% for Julian day 182–183) and annual minima during winter (0% for Julian day 1 and 365) (Rode et al., 2016). Supplementary Table 1 summarizes the different coefficients used for all four landscape units. The parameter values chosen are typical of specific Q (in mm/d) and nitrate concentration (in mg N/l) for catchments of the temperate zone in agricultural areas (e.g., Dupas et al., 2017). The high nutrient loading landscape units have a mean nutrient concentration five times higher than the low nutrient loading landscape units. The subcatchments with a high buffering capacity have a seasonal amplitude five times higher than those with a low buffering capacity (reflecting temperature-dependent buffering processes). The C and Q dynamics in downstream reaches are simulated by mixing daily C and Q dynamics in headwaters and applying in-stream retention as described above.
Presentation of the Landscape Unit Distribution (LUD) Framework
Identify Sensitive Water Bodies and Define Water Quality Targets
The first steps of a nutrient management strategy are the identification of target water bodies and the determination of water-quality targets. A large catchment may include a variety of different types of surface water bodies (permanent and intermittent streams, low and high order rivers, standing and flowing surface water, etc.) and its outlet may discharge to an estuary. Some researchers hold that eutrophication can be effectively managed by focusing on a single limiting nutrient, typically nitrogen or phosphorus (e.g., Schindler et al., 2008), while other researchers consider that multi-nutrient decreases are required for significant reduction in eutrophication (e.g., Elser et al., 2007; Lewis and Wurtsbaugh, 2008; Paerl et al., 2016). Once both priority water bodies and priority nutrients have been identified, one needs to define a water quality target (e.g., load or concentration) and investigate whether there is a critical time period when eutrophication is most likely, due to environmental factors such as temperature and light. For example, Stamm et al. (2014) suggested that consideration of residence time in different types of water bodies is crucial, with annual nutrient loads being more important in standing waters (lakes and reservoirs) and base-flow concentrations during the growing season being more important in flowing waters (streams and rivers). We point out that the goal of the LUD framework is not to provide specific recommendations for what water bodies or targets are most relevant, but to provide insight into possible catchment response to land use changes once the local research or management authorities have decided on their priorities.
In the illustrative example, we considered both the upper portion of the main river network and the catchment outlet as sensitive water bodies. For simplicity, we only considered one generic nutrient but we simulated several situations regarding water bodies: with or without a reservoir in the upper portion of the river network and with the river terminating in a reservoir or an estuary. On these hydrological templates, we examined the capacity of seven landscape distribution scenarios to attain two water quality targets for both sensitive water bodies: annual loads for the reservoirs and summer concentration for the non-reservoir and estuary configurations (Figure 2). We note again that other target metrics are possible, such as frequency or duration of exceedance of a given water quality threshold.
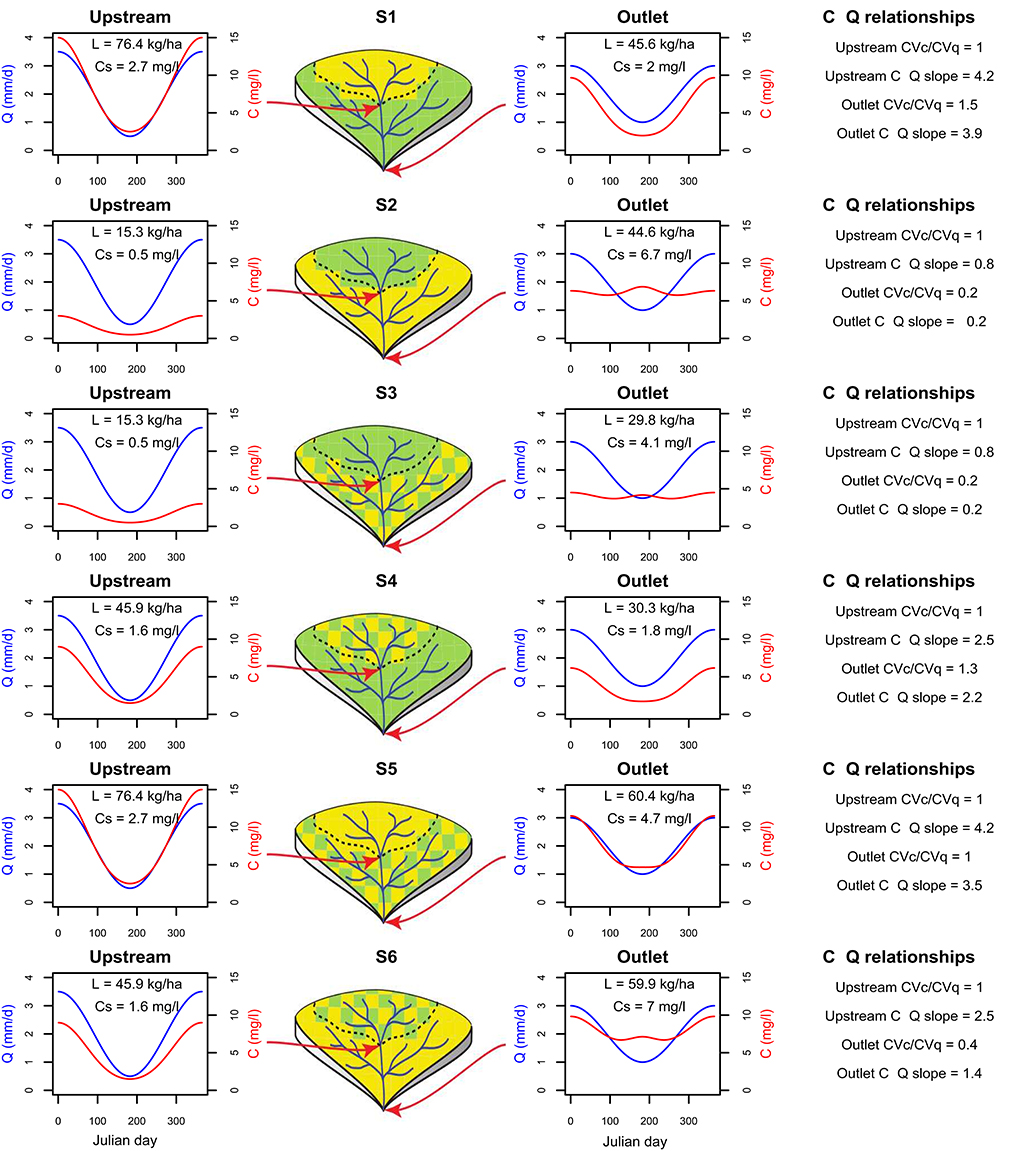
Figure 2. Nutrient concentration dynamics resulting from six landscape unit distribution (LUD) scenarios. Each scenario is evaluated for its capacity to reach two water quality targets: annual load (L) and summer concentration (Cs) in upstream reaches and at the outlet. The water quality metrics CVc/CVq (ratio of the coefficient of variation of concentration and discharge) and C-Q slope (slope of the concentration-discharge relationship) are also estimated at the upstream reaches and the outlet.
Assess Nutrient Export Regimes in Headwater Catchments and Downstream Reaches
Monitoring at the right place and at the right frequency is essential to the LUD framework. When selecting optimized monitoring stations in an existing or new monitoring network, careful consideration should be given to:
- The location of the monitoring stations. Both headwater catchments representative of elementary landscape units and downstream reaches should be monitored. Headwater outlets must be selected according to the size of landscape units in the catchment (Abbott et al., 2018), the rate of in-stream transformation (higher rates obscure upstream signals faster), and according to practical consideration (accessibility, power supply, etc.). Monitoring stations on the main river reach can be located either at the inlet of sensitive water bodies or at the confluence of the major river reaches (Figure 1).
- The temporal design of the monitoring strategy, which encompasses the duration, frequency, and temporal adaptability (e.g., frequency increased during specific events or periods). Here both the nutrient dynamics (interannual and seasonal variability, response to runoff events, etc.) and the priority water quality metrics have an influence, as well as the degree of acceptable uncertainty (Moatar et al., 2013; Skeffington et al., 2015). When summer base-flow concentration is the metric of interest, a low frequency sampling strategy is acceptable, whereas when load is the metric of interest, a higher frequency that is possibly supplemented by adaptable sampling during selected storm events might be necessary (Minaudo et al., 2017; Zarnetske et al., 2018).
In the illustrative example, monitoring takes place at the outlet of four elementary headwaters representative of the four dominant landscape units in the catchment, complemented by two stations on the main river reach to monitor the upper portion and the catchment outlet (Figure 1). Synthetic time series of discharge and water quality were generated with a cosine function to simulate a dominant seasonal signal. In a situation where seasonal fluctuations are much larger than the response to storm events, a monthly sampling during a few years is sufficient (e.g., Dupas et al., 2017, 2018). Because this is not the case in all catchments, a longer monitoring period or higher monitoring frequency would be necessary when interannual or short-term variability is dominant.
Define Export Regime Metrics Based on Local Nutrient Dynamics and Water Quality Targets
In an ideal case of continuous monitoring (like in the synthetic time series used here as an example), the two target metrics of summer base-flow concentration and annual load can be estimated with a high degree of certainty. However, moderate to low frequency monitoring (i.e., weekly to monthly) is much more common, and it is necessary to estimate water quality metrics and their uncertainty. Estimation methods often rely on sub-sampling of high frequency time series to a weekly to monthly frequency, and the best estimation methods are then compared to the original time series to derive estimation of bias and uncertainty ranges (Skeffington et al., 2015; Zhang and Ball, 2017). In addition to the water quality targets, it can be of interest to derive additional export regime metrics to improve understanding of system functioning. Such metrics include the coefficient of variation of concentration (CVc) and discharge (CVq) and the slope of the C-Q relationship (Moatar et al., 2017).
In our simulation, CVc, CVq, and the slope of the C-Q relationship are estimated from the synthetic C and Q time series, in addition to annual load and summer concentration (from Julian day 152 to 212). These metrics are primarily used to interpret water quality time series in terms of dominant flowpaths and sources heterogeneity in small catchments (e.g., Bieroza et al., 2018). We computed them here to investigate how the spatial distribution of landscape units could influence them in larger catchments.
Landscape Distribution Scenarios and Feasibility of Defined Targets
In a reference scenario S0 where the share of high and low nutrient loading is 50%/50% both in the upstream and downstream parts of the catchment, the mixing model predicted similar loads upstream and downstream (45.9 vs. 45.1 kg/ha, respectively), but very different summer concentrations (1.6 vs. 4.4 mg/l, respectively).
The alternative scenarios S1 and S2 maintain the 50%/50% share of the two land-use types, but relocate 100% of the high nutrient loading land use upstream (S1) or downstream (S2). Scenario S1 reduced summer concentration at the outlet (but not the load) and increased upstream summer concentration and load compared to S0. Scenario S2 reduced both summer concentration and load upstream, but not load and summer concentration at the outlet (Figures 1, 2). It is interesting to note that scenario S2 resulted in a negative C-Q slope at the outlet (Figure 2 and Supplementary Table 2), i.e., opposite to the reference scenario S0. None of the three scenarios S0, S1, S2 was optimal for all four objectives considered, demonstrating how trade-offs between upstream and downstream water bodies and prioritizing either concentration or load will be necessary depending on local considerations such as how far the water bodies are from water quality targets and which water body is more important to protect.
The alternative scenarios S3 and S4 increase the share of land use with low nutrient loading to 75%, locating the remaining 25% with high nutrient loading upstream (S3) or downstream (S4). Scenario S3 exhibited lower summer concentration and annual load upstream, while S4 exhibited lower summer concentration but similar annual load at the outlet. The alternative scenarios S5 and S6 increase the share of land use with high nutrient loading to 75%, locating the remaining 25% with low nutrient loading downstream (S5) or upstream (S6). Scenario S6 exhibited lower summer concentration and annual load upstream, while S5 exhibited lower summer concentration but similar annual load at the outlet. Summer concentrations were generally sensitive to both landscape composition and distribution, while loads were sensitive to landscape composition but not distribution. As in the comparison of the first three scenarios (i.e., S0, S1, and S2), none of these scenarios (S3–S6) was optimal for the four objectives considered.
An interesting feature of the present landscape distribution model is that the scenarios can result in both decreases and increases in variance (CVc/CVq) from upstream to the outlet (Figure 2), similar to recent observations in real catchments (Abbott et al., 2018; Zarnetske et al., 2018). On the contrary, the C-Q slope systematically decreases from upstream to the outlet (Figure 2), agreeing with some real catchments (Creed et al., 2015) but in conflict with others (Moatar et al., 2017). Overall, the landscape redistribution scenarios influenced the two water quality metrics C-Q slope and CVc/CVq, suggesting that these metrics must not be interpreted only in terms of dominant flowpaths in large catchments. In this virtual experiment, seasonal mixing of export regimes with coordinated fluctuations creates strong temporal synchrony (Abbott et al., 2018). However, an emergent summer increase, resulting from non-homogeneous discharge throughout the catchment, was predicted in some situations (e.g., scenarios S2 and S3). Finally, results show that landscape redistribution scenarios influenced intra-annual concentration dynamics (metrics Cs, CVc/CVq, C-Q slope in Figure 2) more than annual loads (metrics L in Figure 2). The large influence of land use spatial distribution results both from spatial variability in Q dynamics and variable travel times in the main river network.
Limits and Perspectives
Changing the spatial distribution of landscape units within large catchments is one management strategy that can potentially achieve water quality targets to limit eutrophication while maintaining crop yields or other socioeconomically favorable activity. This redistribution could complement more commonly used management strategies such as optimizing farming practices or relocating the location or configuration of landscape buffers (extensive pastures, hedgerows, wetlands, etc.) along hillslopes. We argue that consideration of spatial management can lead to cost effective attainment of water quality targets through changes in landscape management where it has the largest effect on water quality (Bol et al., 2018; Helton et al., 2018; Wollheim et al., 2018).
An important weakness of the LUD framework is that the definition of landscape units varies in different geographic settings. Prior knowledge of the landscape variables that predominantly control nutrient dynamics is required, and identifying elementary headwaters representative of these landscape units can be a challenge when a landscape property varies continuously in space (e.g., precipitation gradient) as compared to properties that vary in a more discrete way (e.g., lithology, land use). In the illustrative example, we restricted the typology to four types of terrestrial landscape but it could be extended to river reach types in catchments with heterogeneous river channel properties.
Additionally, as for other short-term assessments of water quality, the LUD framework does not account for long time lags between change in land use and lateral nutrient flux. However, with the assumption of steady-state nutrient state, this framework can provide robust, policy-relevant results without relying on complex and data-intensive mechanistic modeling. When initial water-quality monitoring data are available, this approach should facilitate collaboration with experienced and non-technical stakeholders, encouraging participatory approaches to selecting and implementing effective scenarios. This buy-in is particularly important in identifying priorities and setting expectations because, as the illustrative example showed here, land-use redistribution and other interventions can improve one or several, but often not all dimensions of water quality and aquatic ecosystem function.
Author Contributions
RD and BA wrote the first draft. RD and CM made the figures. RD, BA, CM, and OF wrote the final version of the manuscript.
Funding
RD is supported by the Interreg project Channel Payments for Ecosystem Services, funded through the European Regional Development Fund (ERDF).
Conflict of Interest Statement
The authors declare that the research was conducted in the absence of any commercial or financial relationships that could be construed as a potential conflict of interest.
Supplementary Material
The Supplementary Material for this article can be found online at: https://www.frontiersin.org/articles/10.3389/fenvs.2019.00043/full#supplementary-material
References
Abbott, B. W., Baranov, V., Mendoza-Lera, C., Nikolakopoulou, M., Harjung, A., Kolbe, T., et al. (2016). Using multi-tracer inference to move beyond single-catchment ecohydrology. Earth Sci. Rev. 160, 19–42. doi: 10.1016/j.earscirev.2016.06.014
Abbott, B. W., Gruau, G., Zarnetske, J. P., Moatar, F., Barbe, L., Thomas, Z., et al. (2018). Unexpected spatial stability of water chemistry in headwater stream networks. Ecol. Lett. 21, 296–308. doi: 10.1111/ele.12897
Alexander, R. B., Boyer, E. W., Smith, R. A., Schwarz, G. E., and Moore, R. B. (2007). The role of headwater streams in downstream water quality. J. Am. Water Res. Assoc. 43, 41–59. doi: 10.1111/j.1752-1688.2007.00005.x
Aubert, A. H., Gascuel-Odoux, C., and Merot, P. (2013). Annual hysteresis of water quality: a method to analyse the effect of intra- and inter-annual climatic conditions. J. Hydrol. 478, 29–39. doi: 10.1016/j.jhydrol.2012.11.027
Bieroza, M. Z., Heathwaite, A. L., Bechmann, M., Kyllmar, K., and Jordan, P. (2018). The concentration-discharge slope as a tool for water quality management. Sci. Total Environ. 630, 738–749. doi: 10.1016/j.scitotenv.2018.02.256
Bishop, K., Buffam, I., Erlandsson, M., Folster, J., Laudon, H., Seibert, J., et al. (2008). Aqua incognita: the unknown headwaters. Hydrol. Process. 22, 1239–1242. doi: 10.1002/hyp.7049
Bol, R., Gruau, G., Mellander, P. E., Dupas, R., Bechmann, M., Skarbøvik, E., et al. (2018). Challenges of reducing phosphorus based water eutrophication in the agricultural landscapes of northwest Europe. Front. Mar. Sci. 5:276. doi: 10.3389/fmars.2018.00276
Bouraoui, F., and Grizzetti, B. (2011). Long term change of nutrient concentrations of rivers discharging in European seas. Sci. Total Environ. 409, 4899–4916. doi: 10.1016/j.scitotenv.2011.08.015
Creed, I. F., Mcknight, D. M., Pellerin, B. A., Green, M. B., Bergamaschi, B. A., Aiken, G. R., et al. (2015). The river as a chemostat: fresh perspectives on dissolved organic matter flowing down the river continuum. Can. J. Fish. Aquat. Sci. 72, 1272–1285. doi: 10.1139/cjfas-2014-0400
Doody, D. G., Withers, P. J. A., Dils, R. M., Mcdowell, R. W., Smith, V., Mcelarney, Y. R., et al. (2016). Optimizing land use for the delivery of catchment ecosystem services. Front. Ecol. Environ. 14, 325–332. doi: 10.1002/fee.1296
Dupas, R., Minaudo, C., Gruau, G., Ruiz, L., and Gascuel-Odoux, C. (2018). Multidecadal trajectory of riverine nitrogen and phosphorus dynamics in rural catchments. Water Resour. Res. 54, 5327–5340. doi: 10.1029/2018WR022905
Dupas, R., Musolff, A., Jawitz, J. W., Rao, P. S. C., Jaeger, C. G., Fleckenstein, J. H., et al. (2017). Carbon and nutrient export regimes from headwater catchments to downstream reaches. Biogeosciences 14, 4391–4407. doi: 10.5194/bg-14-4391-2017
Elser, J. J., Bracken, M. E. S., Cleland, E. E., Gruner, D. S., Harpole, W. S., Hillebrand, H., et al. (2007). Global analysis of nitrogen and phosphorus limitation of primary producers in freshwater, marine and terrestrial ecosystems. Ecol. Lett. 10, 1135–1142. doi: 10.1111/j.1461-0248.2007.01113.x
Heathwaite, L., Sharpley, A., and Gburek, W. (2000). A conceptual approach for integrating phosphorus and nitrogen management at watershed scales. J. Environ. Qual. 29, 158–166. doi: 10.2134/jeq2000.00472425002900010020x
Helton, A. M., Hall, R. O., and Bertuzzo, E. (2018). How network structure can affect nitrogen removal by streams. Freshw. Biol. 63, 128–140. doi: 10.1111/fwb.12990
Hrachowitz, M., Benettin, P., Van Breukelen, B. M., Fovet, O., Howden, N. J., Ruiz, L., et al. (2016). Transit times—the link between hydrology and water quality at the catchment scale. Water 3, 629–657. doi: 10.1002/wat2.1155
Le Moal, M., Gascuel-Odoux, C., Ménesguen, A., Souchon, Y., Etrillard, C., Levain, A., et al. (2019). Eutrophication: a new wine in an old bottle. Sci. Total Environ. 651, 1–11. doi: 10.1016/j.scitotenv.2018.09.139
Lewis, W. M., and Wurtsbaugh, W. A. (2008). Control of lacustrine phytoplankton by nutrients: erosion of the phosphorus paradigm. Int. Rev. Hydrobiol. 93, 446–465. doi: 10.1002/iroh.200811065
Mcclain, M. E., Boyer, E. W., Dent, C. L., Gergel, S. E., Grimm, N. B., Groffman, P. M., et al. (2003). Biogeochemical hot spots and hot moments at the interface of terrestrial and aquatic ecosystems. Ecosystems 6, 301–312. doi: 10.1007/s10021-003-0161-9
Minaudo, C., Dupas, R., Gascuel-Odoux, C., Fovet, O., Mellander, P.-E., Jordan, P., et al. (2017). Nonlinear empirical modeling to estimate phosphorus exports using continuous records of turbidity and discharge. Water Resour. Res. 53, 7590–7606. doi: 10.1002/2017WR020590
Minaudo, C., Meybeck, M., Moatar, F., Gassama, N., and Curie, F. (2015). Eutrophication mitigation in rivers: 30 years of trends in spatial and seasonal patterns of biogeochemistry of the Loire River (1980-2012). Biogeosciences 12, 2549–2563. doi: 10.5194/bg-12-2549-2015
Mineau, M. M., Wollheim, W. M., and Stewart, R. J. (2015). An index to characterize the spatial distribution of land use within watersheds and implications for river network nutrient removal and export. Geophys. Res. Lett. 42, 6688–6695. doi: 10.1002/2015GL064965
Moatar, F., Abbott, B. W., Minaudo, C., Curie, F., and Pinay, G. (2017). Elemental properties, hydrology, and biology interact to shape concentration-discharge curves for carbon, nutrients, sediment, and major ions. Water Resour. Res. 53, 1270–1287. doi: 10.1002/2016WR019635
Moatar, F., Meybeck, M., Raymond, S., Birgand, F., and Curie, F. (2013). River flux uncertainties predicted by hydrological variability and riverine material behaviour. Hydrol. Process. 27, 3535–3546. doi: 10.1002/hyp.9464
Musolff, A., Fleckenstein, J. H., Rao, P. S. C., and Jawitz, J. W. (2017). Emergent archetype patterns of coupled hydrologic and biogeochemical responses in catchments. Geophys. Res. Lett. 44, 4143–4151. doi: 10.1002/2017GL072630
Paerl, H. W., Scott, J. T., Mccarthy, M. J., Newell, S. E., Gardner, W. S., Havens, K. E., et al. (2016). It takes two to Tango: when and where dual nutrient (N and P) reductions are needed to protect lakes and downstream ecosystems. Environ. Sci. Technol. 50, 10805–10813. doi: 10.1021/acs.est.6b02575
Rode, M., Halbedel Angelstein, S., Anis, M. R., Borchardt, D., and Weitere, M. (2016). Continuous in-stream assimilatory nitrate uptake from high frequency sensor measurements. Environ. Sci. Technol. 50, 5685–5694. doi: 10.1021/acs.est.6b00943
Schindler, D. W., Hecky, R. E., Findlay, D. L., Stainton, M. P., Parker, B. R., Paterson, M. J., et al. (2008). Eutrophication of lakes cannot be controlled by reducing nitrogen input: Results of a 37-year whole-ecosystem experiment. Proc. Natl. Acad. Sci. U.S.A. 105, 11254–11258. doi: 10.1073/pnas.0805108105
Seitzinger, S. P., Mayorga, E., Bouwman, A. F., Kroeze, C., Beusen, A. H. W., Billen, G., et al. (2010). Global river nutrient export: a scenario analysis of past and future trends. Global Biogeochem. Cycles 24. doi: 10.1029/2009GB003587
Sinha, E., Michalak, A. M., and Balaji, V. (2017). Eutrophication will increase during the 21st century as a result of precipitation changes. Science 357, 405–408. doi: 10.1126/science.aan2409
Skeffington, R. A., Halliday, S. J., Wade, A. J., Bowes, M. J., and Loewenthal, M. (2015). Using high-frequency water quality data to assess sampling strategies for the EU water framework directive. Hydrol. Earth Syst. Sci. 19, 2491–2504. doi: 10.5194/hess-19-2491-2015
Stamm, C., Jarvie, H. P., and Scott, T. (2014). What's more important for managing phosphorus: loads, concentrations or both? Environ. Sci. Technol. 48, 23–24. doi: 10.1021/es405148c
Steffen, W., Richardson, K., Rockstrom, J., Cornell, S. E., Fetzer, I., Bennett, E. M., et al. (2015). Planetary boundaries: guiding human development on a changing planet. Science 347:1259855. doi: 10.1126/science.1259855
Thomas, Z., Abbott, B., Troccaz, O., Baudry, J., and Pinay, G. (2016). Proximate and ultimate controls on carbon and nutrient dynamics of small agricultural catchments. Biogeosciences 13, 1863–1875. doi: 10.5194/bg-13-1863-2016
Van Meter, K. J., Van Cappellen, P., and Basu, N. B. (2018). Legacy nitrogen may prevent achievement of water quality goals in the Gulf of Mexico. Science 360, 427–430. doi: 10.1126/science.aar4462
Vero, S. E., Basu, N. B., Van Meter, K., Richards, K. G., Mellander, P. E., Healy, M. G., et al. (2017). Review: the environmental status and implications of the nitrate time lag in Europe and North America. Hydrogeol. J. 26, 7–22. doi: 10.1007/s10040-017-1650-9
Vinatier, F., Lagacherie, P., Voltz, M., Petit, S., Lavigne, C., Brunet, Y., et al. (2016). An unified framework to integrate biotic, abiotic processes and human activities in spatially explicit models of agricultural landscapes. Front. Environ. Sci. 4:6. doi: 10.3389/fenvs.2016.00006
Withers, P. J. A., Neal, C., Jarvie, H. P., and Doody, D. G. (2014). Agriculture and eutrophication: where do we go from here? Sustainability 6, 5853–5875. doi: 10.3390/su6095853
Wollheim, W. M., Bernal, S., Burns, D. A., Czuba, J. A., Driscoll, C. T., Hansen, A. T., et al. (2018). River network saturation concept: factors influencing the balance of biogeochemical supply and demand of river networks. Biogeochemistry. 141:503. doi: 10.1007/s10533-018-0488-0
Wollheim, W. M., Peterson, B. J., Thomas, S. M., Hopkinson, C. H., and Vorosmarty, C. J. (2008). Dynamics of N removal over annual time periods in a suburban river network. J. Geophys. Res. Biogeosci. 113. doi: 10.1029/2007JG000660
Wollschläger, U., Attinger, S., Borchardt, D., Brauns, M., Cuntz, M., Dietrich, P., et al. (2017). The Bode hydrological observatory: a platform for integrated, interdisciplinary hydro-ecological research within the TERENO Harz/Central German Lowland Observatory. Environ. Earth Sci. 76:29. doi: 10.1007/s12665-016-6327-5
Zarnetske, J. P., Bouda, M., Abbott, B. W., Saiers, J., and Raymond, P. A. (2018). Generality of hydrologic transport limitation of watershed organic carbon flux across ecoregions of the United States. Geophys. Res. Lett.45, 11702–11711. doi: 10.1029/2018GL080005
Keywords: headwater, eutrophication, nutrient, water quality, agriculture, landscape
Citation: Dupas R, Abbott BW, Minaudo C and Fovet O (2019) Distribution of Landscape Units Within Catchments Influences Nutrient Export Dynamics. Front. Environ. Sci. 7:43. doi: 10.3389/fenvs.2019.00043
Received: 20 November 2017; Accepted: 19 March 2019;
Published: 17 April 2019.
Edited by:
Sudhakar Srivastava, Banaras Hindu University, IndiaReviewed by:
Tom Langen, Clarkson University, United StatesJulien Tournebize, National Research Institute of Science and Technology for Environment and Agriculture (IRSTEA), France
Stefanie Lutz, Helmholtz Centre for Environmental Research (UFZ), Germany
Copyright © 2019 Dupas, Abbott, Minaudo and Fovet. This is an open-access article distributed under the terms of the Creative Commons Attribution License (CC BY). The use, distribution or reproduction in other forums is permitted, provided the original author(s) and the copyright owner(s) are credited and that the original publication in this journal is cited, in accordance with accepted academic practice. No use, distribution or reproduction is permitted which does not comply with these terms.
*Correspondence: Rémi Dupas, remi.dupas@inra.fr