- 1Capital One - Center for Machine Learning, McLean, VA, United States
- 2Earth and Planetary Sciences, Johns Hopkins University, Baltimore, MD, United States
- 3Industrial Engineering, Purdue University, West Lafayette, IN, United States
- 4Environmental & Ecological Engineering, Purdue University, West Lafayette, IN, United States
Access to fresh water is critical for human well-being, economic activity and, in some cases, political stability. Data from the Gravity Recovery and Climate Experiment (GRACE) has been used to monitor variability and trends in total water storage. This makes it possible to associate changes in water storage with both climate variability and large scale water management. Recent research has shown that these trends can be associated, globally, with rainfall, irrigation, and climate model predictions. This research indicates a need for further investigation into specific human predictors of trends in terrestrial water storage. This paper presents the first global scale analysis of GRACE trends focused on national scale socio-economic predictors of terrestrial water storage. We show that rainfall, irrigation, agricultural characteristics, and energy practices all contribute to GRACE trends, and the importance of each differs by country and region. Additionally, this work suggests that other factors such as GDP, population density, urbanization, and forest cover do not explain GRACE trends at a national level. Identifying these key predictors aids in understanding trends in water availability and for informing water management policy in a changing climate.
1. Introduction
The global distribution of accessible fresh water resource is in flux. Rising temperatures affect the extent and seasonality of water storage in snow and glaciers. Changes in the distribution and intensity of precipitation influence the reliability of river flows and recharge to shallow aquifers. Construction or removal of major dams alters human ability to appropriate surface water flows. Perhaps most significantly, extensive exploitation of groundwater reserves is rapidly depleting aquifers in some of the world's most important food basket regions (Liu et al., 2011; Kumar et al., 2012; Scanlon et al., 2012). Against this backdrop, the need to monitor, understand, and, when possible, project the future distribution of water resources is a recognized research priority.
One area that has seen dramatic progress in the past decade is our ability to monitor changes in water resources using satellite-derived observations. Global monitoring of rainfall, soil moisture, snow cover, and even evapotranspiration is now possible, albeit with significant uncertainties that are the subject of active research (Greatrex et al., 2014; Wanders et al., 2014). The Gravity Recovery and Climate Experiment (GRACE) satellite mission has been instrumental in providing data that allows us to monitor total fresh water resources. With GRACE observations, estimates of anomalies in total terrestrial water storage can be computed. This has allowed for remote monitoring of water storage change—particularly groundwater storage change—in a manner never before possible. Using this data researchers have investigated the global scale relationships between GRACE trends, climate models, precipitation, and irrigation. They show that once these signals are removed, irrigation, and potentially other human causes may explain, in part, GRACE observed TWS trends (Rodell et al., 2018). At a basin scale, GRACE has been applied to quantify trends in water storage in critical breadbasket regions around the world (Tiwari et al., 2009; Voss et al., 2013; Richey et al., 2015; Li and Rodell, 2016; Lo et al., 2016; Girotto et al., 2017; Nie et al., 2017). This research indicates that common terrestrial water storage components do not explain these trends, suggesting that they may be caused by unsustainable human use (Rodell et al., 2009; Famiglietti et al., 2011).
Globally, irrigated agriculture continues to be the largest user of surface water and groundwater resources. However, all irrigated agriculture is not equal. This is true hydrologically—different crops require different amounts of water, and some regions have greater access to renewable irrigation water resource than others—and it is also true economically. The value of crops differs dramatically, as was repeatedly emphasized during the recent California drought (e.g., high value almond vs. low value alfalfa), and the drivers of production differ as well (Swegal, 2017). The percentage of agricultural production sent to international markets has increased significantly in recent decades (Kastner et al., 2014), such that domestic water resources are now strongly influenced by international virtual water flow for many countries. This influences local economic opportunities, national trade strategies, and risks to water resources. Beyond agriculture, urban and industrial activity have a smaller but growing influence on surface water and groundwater resources.
Given these multiple drivers of water use, it is important to investigate observed trends in terms of their climatic and societal drivers. Doing so can help to characterize and map diverse human influences on water resources, distinguishing between regions in which water shortages are a function of climate variability and those in which particular economic or demographic activities might be most directly responsible for changing water availability. In this paper, we compile extensive data on climatic and socio-economic variables that have been shown in the literature to influence water storage and leverage statistical learning theory to identify the key predictors of observed GRACE trends at a national scale.
2. Data Preparation and Processing
In order to explore drivers of GRACE trends at a country level we compiled a dataset with 47 covariates. Covariates were chosen for their potential to impact groundwater use in a country. Specifically, the covariates were selected based on a review of existing literature of potential drivers of groundwater storage trends as well as data availability at a national level over the time period of the GRACE observations. These included annual or monthly metrics for precipitation data, agricultural data (e.g., irrigation, crop yields, area under cultivation), economic and trade data (e.g., imports, exports, GDP, employment), population data (e.g., population density, percent urbanized population), and land-use data (e.g., forested area, agricultural land, urban land). A full list and data sources of country level indicators can be found in Appendix Table A1.
GPCC and GRACE data are made available by NOAA and NASA respectively. Other data was gathered from the World Bank's “World Development Indicators” and the United Nations Food and Agriculture Organization's “FAOSTAT” database. This analysis was conducted on 88 countries. Country inclusion was limited according to the following criteria.
• Latitude: This study does not address high latitude cryospheric effects, so analysis was limited to the latitudes 50°S–50°N. Countries that lie entirely below 50°S or above 50°N were excluded from analysis. For countries that lie partially outside this latitude range the GRACE and GPCC variables were extracted only for the portion of the country below 50°N (or above 50°S). While there are cryospheric trends between 50°S and 50°N, limiting our analysis to these latitudes excludes Greenland and Antarctica, where GRACE trends are dominated by ice sheet dynamics. Excluding high latitude regions of countries that cross the 50th parallel introduces some inconsistency with national-scale covariates, but it is consistent with the fact that most major water use activities in these countries occurs at lower latitudes.
• Area: Accuracy for GRACE is known to diminish at smaller scales, such as smaller countries. Therefore, countries smaller than 100,000 km2 were not included. Additionally, significant coastline can also affect the accuracy of GRACE measurements therefore small island nations and peninsular countries were excluded.
• Data Availability: Some countries lacked the measures for several of the other covariates. These countries were also excluded.
Raw source data from the World Development Indicators and FAOSTAT are provided as time series with varying time-steps for each variable and for each country. To transform the data the raw data was processed in two distinct ways:
• Trends: calculating a historic average for each country for the years available prior to 2002 (corresponding to the first year of the GRACE data), then that number was subtracted from each year between 2002 and 2015. A simple linear regression was fit to those deviations from the historic average and this was deemed the trend over that time period.
• Averages: averaging the values between 2002 and 2015, the time period roughly corresponding to the GRACE observations.
Data Visualization
GRACE trends were calculated in 88 countries at national scale and depicted in Figure 1 (top graph). As has been reported in previous studies (Rodell et al., 2009; Voss et al., 2013) strong negative trends in the Middle East and Central Asia are evident in the top graph in Figure 1. Additionally, positive trends can be seen in Equatorial West Africa and the northern regions of South America. Precipitation trends were also depicted in Figure 1 (bottom graph). Trends in precipitation over the period of GRACE record align with the GRACE trends in some regions, but in many regions there is no clear association between water storage trend and precipitation trend.
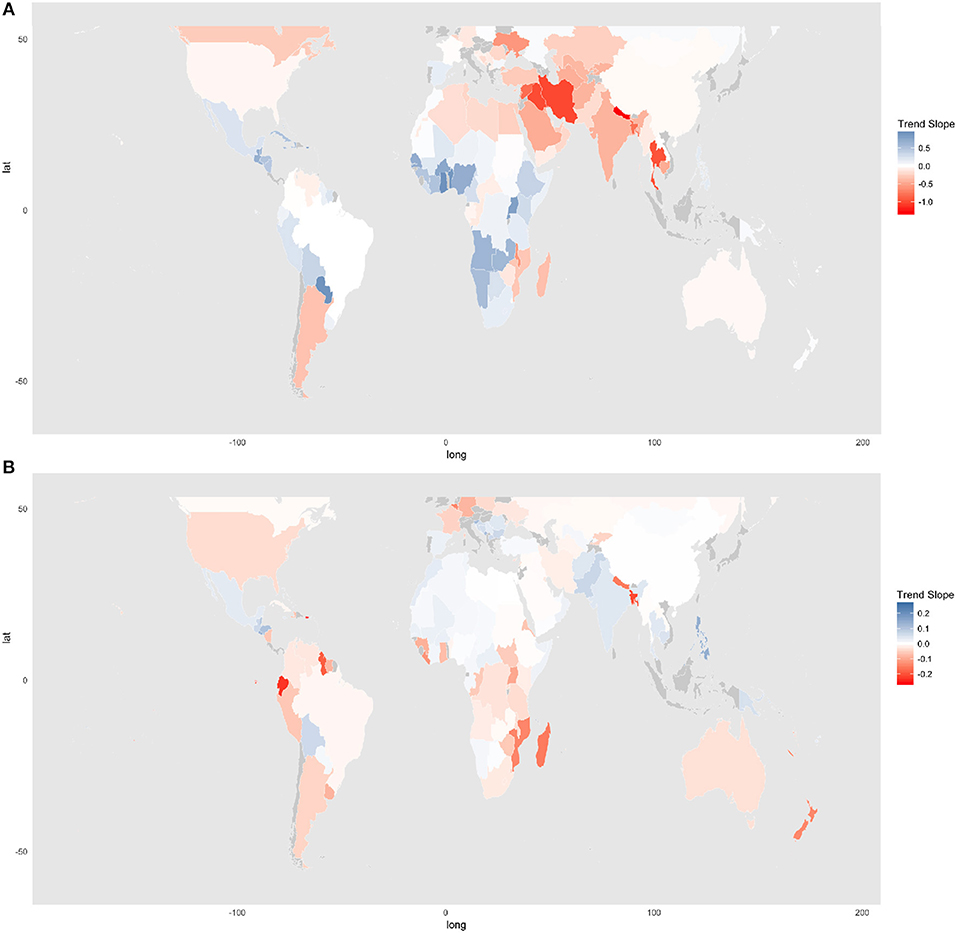
Figure 1. (A) Seasonally Adjusted Country Level GRACE Trends (top), (B) Seasonally Adjusted Country Level GPCC Trends (bottom).
As another exploratory analysis of the input data we leveraged Principal component analysis (PCA)-biplot which is a powerful visualization technique for high dimensional multivariate data (Gower et al., 2011). A PCA-biplot can help create a low-dimensional representation of multivariate data using the first two principle components. In a PCA-biplot, vector lengths approximate standard deviations, and the cosines of their angles are proportional to the correlation between the variables. It can be seen in the biplot of the first two principal components of leading predictor variables, Figure 2A, that countries generally cluster by continent, with expected economic outliers, and there is a tendency for countries with negative GRACE water storage trends to cluster relative to countries with positive trends (Figure 2B). For instance, it is interesting to note from Figure 2 (top) that the African countries (in red) tend to cluster around the increasing trends in land equipped for irrigation; while some of those countries (such as Republic of Congo) are already experiencing negative GRACE anomalies (Figure 2, bottom). Of additional note, in the first two principal components, Land Equipped for Irrigation (Average) and GPCC have nearly opposite vector directions. Suggesting that these two indicators may be inversely related to one another.
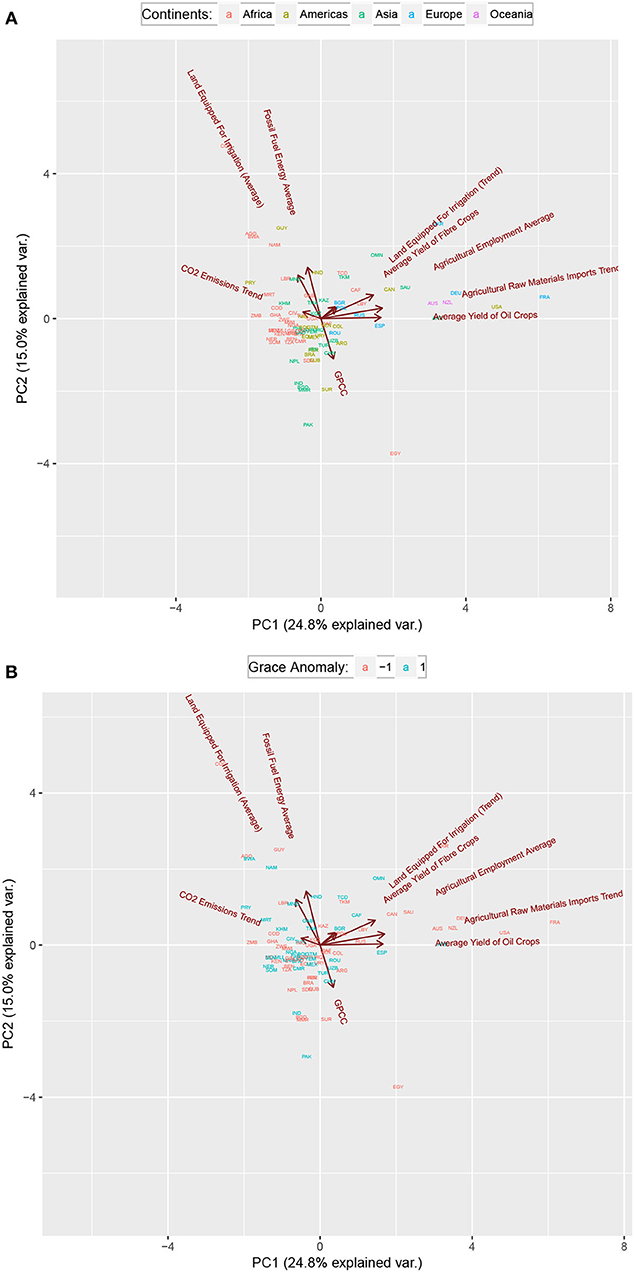
Figure 2. (A) PCA-Biplot of input data, the countries are color-coded by continents, (B) PCA Biplots of input data, with color red indicating negative and blue indicating positive GRACE anomalies.
3. Methodology
Supervised machine learning is used to approximate an unknown function f to predict the response variable Y as a function of independent variables x. Mathematically, it be summarized as Y = f(X) + ϵ; where ϵ is referred to as “irreducible error.” The goal of supervised learning is to use the training data to approximate the function such that the loss function of interest is minimized over the entire domain of input data; where ω(X) stands for a weight function and Δ denotes a measure of distance (Friedman, 1991; Hastie et al., 2001). Global parametric modeling—such as GLM—has been the most widely used technique for approximating f(X). Global parametric models are popular due to their ease of computation and interpretability. However, such an approach is “rigid” (due to assumptions such as linearity, etc.). Its limited flexibility means that it often fails to approximate the true function accurately (Hastie et al., 2001).
To estimate GRACE anomalies and identify the most important predictors of global ground water storage trends, we tested the predictive performance of several classes of models such as generalized linear models (GLM) (McCullagh, 1984), generalized additive models (GAM) (Hastie and Tibshirani, 1990), artificial neural network (ANN) (Hastie et al., 2001), multivariate adaptive regression splines (MARS), support vector machines (SVM) (Drucker et al., 1997) and ensemble tree-based approaches such as Random Forest (RF) (Breiman, 2001), and Bayesian Additive Regression Trees (BART) (Chipman et al., 2010; Kapelner and Bleich, 2013). BART (Chipman et al., 2010; Kapelner and Bleich, 2013) was selected as our final best model due to its robust predictive performance. More specifically, the predictive models developed based on the BART algorithm outperformed the other models (i.e., GLM, GAM, MARS, RF, SVM, and ANN) in terms of both out-of-sample performance (i.e., based on 30-fold random holdout cross-validation tests on the data) and goodness-of-fit (i.e., based on in-sample errors and R2 values). Each model was compared in terms of mean squared error on a held out set of data for each of the 30-folds of the cross-validation. The BART model had a Bonferroni corrected statistically significant mean squared error lower than the rest of the models. Moreover, BART's ability to yield probabilistic inferences and adequately characterize the uncertainties was another attribute of the algorithm that made it desirable for this analysis.
Bayesian Additive Regression Trees (BART)
BART is a Bayesian, ensemble, tree-based approach, capable of capturing complex interactions and non-linearities (Chipman et al., 2010). A BART model can be represented as the summation of the estimate from M small(shallow) trees:
Where Y represents the response variable, g() denotes a regression tree. For each of the tree structures denoted by Tj, and its associated terminal node parameter denoted by Mj = (μ1, μ2, …, μj). The function g assigns the conditional mean value μ to the vector of covariates, where μ can represents the main effects or interaction effects depending on the number of covariate(s). The trees are fitted via an MCMC algorithm (Chipman et al., 2010; Kapelner and Bleich, 2013). What makes BART different from other ensemble, tree-based methods is the Bayesian component; where prior probabilities are imposed on each tree such that all individual trees are “weak learner.” This means that no one tree will dominate the final estimate. The application of a regularization prior prohibits the trees from growing too deep and over-fitting the data.
Model Inference
To conduct model inferencing based on BART, variable importance ranking (to facilitate variable selection) and partial dependence plots (PDP) can be generated. The ranking of the most important variable in BART can be identified by tracking the number of times a predictor has been used in a given tree and therefore contributing to the final prediction. Moreover, since BART is a non-parametric model, the relationship between the response and the predictors can be depicted via partial dependence plots (PDP). PDPs show the influence of the predictor of interest on the response, while the effect of all other variables are averaged as shown in the equation below.
Where xj is the predictor of interest and x−j, i are all the predictors in the model other than xj and represents the BART model. Partial dependence plots provide the effect of the predictors at different quantiles and provide a 95% confidence interval at each quantile. PDPs show the overall picture of how the predictor contributes to the model as it increases in quantile.
4. Results
We developed predictive models of GRACE trends—using the BART algorithm described above—to identify the key predictors of water storage trends a national scale. The response variable in all models is a linear trend computed from GRACE Terrestrial Water Storage anomalies. This data was extracted from the 0.5x 0.5 degree gridded RL05 Mascon solutions from the Center for Space Research (Himanshu et al., 2016), downloaded from http://www.csr.utexas.edu/grace.
Given the stochastic nature of the BART algorithm, to achieve stability and convergence, The predictive models OF GRACE trends were fitted over 3,000 times, and in each instance the variable importance for the model was calculated. Analyses were repeated with Spherical Harmonics GRACE solutions and the character of results did not change. The features that consistently demonstrated high importance in these 3000 models are as follows:
• Rainfall (Trend): monthly total rainfall derived from the Global Precipitation Climatology Centre (GPCC) 0.5 x 0.5 degree monthly Full Data Product (V7). The Full Data Product is considered to be the most reliable GPCC product, and is available from 1901 to 2013. Gridded fields are generated using quality-controlled data from 67,200 stations with records of 10 years/longer (Schneider et al., 2016). GPCC Precipitation data provided by the NOAA/OAR/ESRL PSD, Boulder, Colorado from their website1.
• Land Equipped for Irrigation (Trend and Average): This is the % agricultural land in a country that is equipped for irrigating crops. This does not mean that the entire area is actually irrigated at any point in time.
• Agricultural Raw Material Imports (Trend): This represents the percentage of imports to a country comprised primarily of crude agricultural products such as vegetable oils, wood, cotton, raw animal, and vegetable products as defined in Standard International Trade Classification (SITC) section 2. Of note, it excludes crude fertilizers and minerals.
• Fiber Crops Yield (Average): This consists of the total land used in fibre crop production and the annual yield of fibre crop per country. Fibre crops include cotton, jute and kenaf, hemp, flax, coir, sisal, henequen, and abaca. It has been estimated that per ton of fibre crop produced 3,837 cubic meters of water are used, nearly 10 times higher than that required for one ton of vegetables (Van Dam and Bos, 2004).
• Oil Crops Yield (Average): This includes the yields and total land area in production of oil crops. The most important of these crops are oil-palm, soybean, sunflower, and rapeseed. Oil-crops are known to have a very high water footprint (2,400 m3/ton).
• Agricultural Employment (% Average): This indicator represents the percent of employed persons who work in the agricultural sector. Employment here means people of working age working to earn money. Agricultural sector includes farming, hunting, fishing, and forestry.
• CO2 Emissions (Trend): This indicator measures changes in CO2 emissions within a country during the time period in question. CO2 emissions are measured in KiloTons. The World Bank collects this data from the Carbon Dioxide Information Analysis Center, Environmental Sciences Division, at Oak Ridge National Laboratory.
• Fossil Fuel Energy Use (% Average): This indicator represents the average percent of total energy comprised of Fossil Fuels. Fossil fuels here refer to coal, petroleum, natural gas, and oil products. Total energy, according to the World Bank “refers to the use of primary energy before transformation to other end-use fuels (such as electricity and refined petroleum products).”
These consistently most important variables can be clustered into four main groups: Climate, Irrigation, Agriculture, and Energy. These groupings and the variables associated are:
Investigating the partial dependence plots for these variables in Figure 3 provides further insight into the relationship between these variables and GRACE trends.
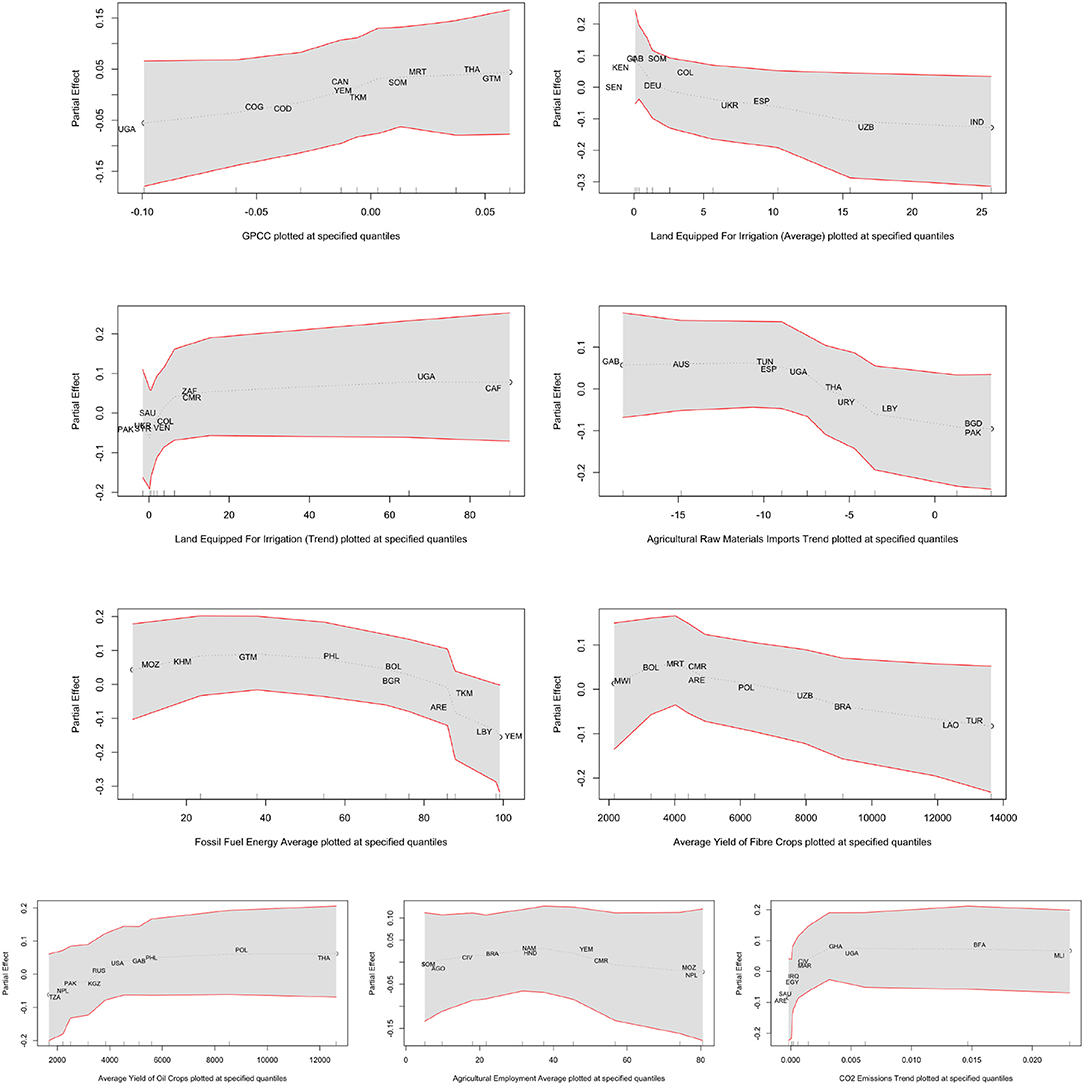
Figure 3. Partial dependence plots for most important predictors. Country codes appearing on the plots relate to which countries have a value closest to that particular quintile.
GPCC: Intuitively, countries that experienced an increase in precipitation over the period of GRACE record tend to show increases in total water storage. It is a reasonably symmetric relationship, in which increased (decreased) precipitation is associated with increased (decreased) water storage up to a threshold, beyond which additional precipitation increase (decrease) has no significant impact. Another rainfall measure, the Tropical Rainfall Measurement Mission (TRMM) Multisensor Precipitation Analysis (TMPA) (Huffman et al., 2007), was also tested with consistent results.
Average Land Equipped for Irrigation: The negative relationship between land equipped for irrigation and water storage indicates that the capability to irrigate is associated with reductions in water storage. The relationship indicates that countries with a low percentage of irrigated land have positive water storage trends. However, countries where greater percentages of agricultural land relies on irrigation, have increasingly negative water storage trends.
Trend in Land Equipped for Irrigation: Interestingly, increases in irrigated area over the GRACE record are associated with an increase in total water storage. This is counter-intuitive, and could reflect the presence of time lags between the implementation of irrigation and potential long-term impacts on water resources. Countries that are actively adding irrigation infrastructure currently have adequate water resources to supply them in the short term.
Trend in Agricultural Raw Material Imports: Countries with rapid growth in their agricultural sectors see increases in the amount of agricultural raw materials required to maintain this growth. This variable distinguishes between countries that are rapidly decreasing raw materials imports (near zero on partial dependence) with those in the more normal range. Within this normal range the more a country increases its agricultural raw materials imports the more likely it is to be experiencing water storage declines. This can be seen as an indicator of how fast a country is growing its agricultural sector or how much a country is decreasing its reliance on domestic agriculture.
Average Yield of Major Crop Types: Interestingly, there are systematic differences in the relationship between water storage trend and yield of various crop types. For oil crops the relationship is positive, where higher yields occur in countries with positive water storage trends, possibly because high yielding oil crops are planted in humid areas that are not prone to water deficit. For fibre, higher yield is associated with water depletion. This could be because fibre crops tend to be irrigated and are very water intensive.
Fossil Fuel Use: This variable indicates that countries that are heavily reliant (greater than 80%) on fossil fuel as a sole source of energy for example, United Arab Emirates (ARE) and Khazakstan (KAZ) are more likely to be experiencing ground water storage declines. For the rest of the countries there isn't a clear relationship. Intuitively there should be no direct relationship between fossil fuel use and groundwater depletion. However, countries that have high percent of energy from fossil fuels might be have a lower cost of fossil fuels than other countries (e.g., fossil fuel providers) therefore it could be cheaper to use said fossil fuels in water pumping systems. Further investigation into the local economies would be required to show this effect more precisely.
Average Percent Agricultural Employment: Countries with low percent and high percent of their workforce in the agricultural sector tend to have negative GRACE trends. However, the effect is not great in any of the quintiles. Nonetheless this feature is consistently important across model iterations. One possible explanation is that this feature is interacting with other features in a meaningful manner. Perhaps this is because this feature is a proxy for several indicators. On the one hand it can be a sign of the net agricultural capacity of a country. If a country has little agricultural capacity, very few people will be employed in agriculture. At the same time, highly technological countries can also have low agricultural employment even if its net output is high.
CO2 Emissions Trend: This variable may indicate a growth in industry and development over the period of GRACE observations. Particularly, this was highest in countries where there was little CO2 emissions at the beginning of period of observation. Those countries appear to be in places where water resources have been increasing. This appears to be the case for countries in West Africa that both experienced a rapid economic growth and an increase in rainfall during this same period. However, effect is fairly limited across all quintiles and it is likely that this feature is interacting with other features more than it is directly contributing to explaining GRACE trends.
5. Discussion
Changes in climate, population growth, urbanization, and industrialization have made fresh water access a global concern. Countries are increasingly relying on groundwater for population and agricultural needs. However, given groundwater storage system complexity, it has been difficult to ascertain the impacts of these withdrawals. Data from the Gravitational Recovery and Climate Experiment satellites have been used at local scales (individual countries and watersheds) to demonstrate that subsurface groundwater can be effectively monitored. Researchers had long hypothesized that where groundwater shortages had been identified, human use played a large role (Rodell et al., 2009). Recent research using GRACE data has shown that land equipped for irrigation helps to explain variability in GRACE observed trends not explained exclusively by rainfall and climate model predictions (Rodell et al., 2018). The work presented here extends this research, confirms the impact of these human factors and further defines the specific anthropogenic factors that contribute the most to these trends.
The food-water-energy nexus is a valuable paradigm through which to view the inter-relatedness of these human and environmental systems2. Within this paradigm, water access, energy prices, and crop choice play a significant and highly interconnected role. Our research further demonstrates this approach. Of the top most important anthropogenic variables used to predict water storage trends, crop choice, fossil fuel energy use and irrigation play the biggest role. Not surprisingly, irrigation leads these factors, as agricultural and irrigation accounts for 69% percent of global water withdrawals, with higher percentages in countries with limited surface water3. Additionally, with fossil fuel energy, deep groundwater extraction requires significant energy as pumps are largely driven by electricity or fossil fuels. Water scarce countries that also rely on fossil fuels for a significant portion of their electricity may be more likely to have energy subsidies in place and therefore it can be theorized that there is a lower barrier for the extraction of deep aquifers (Zhu et al., 2007) to enable economic development in their agricultural sectors.
Due to different water requirements, crop choice plays a logical role in how much water is required by a nation's agricultural system (Mekonnen and Hoekstra, 2011). Furthermore, specific crops show a generally negative relationship with water storage trends, such as fibre crop yields. Fibre crops are resource intensive, and farmers may switch to fibre crops if they command higher market prices than alternatives (Yang et al., 2017). Furthermore, we show that commonly thought drivers such as population density, forested land and GDP do not contribute significantly to models predicting terrestrial water storage trends. These variables might show correlation with water storage when considered independently, but they do not offer explanatory power when variables related to agriculture and energy are also taken into account.
While this study provides good evidence for the anthropogenic factors in water storage trends, there are several limitations. First, the country level statistics used for covariates could contain errors or inconsistencies due to collection methods as well as missing data due to geopolitical changes. Second, there is an obvious mismatch between the gridded scale of the GRACE and GPCC data with the country level statistics. This was resolved by taking the average GRACE and GPCC signal at the country level across grid-cells. This is particularly problematic for large countries with internal variance of water storage trends (i.e., USA, Russia, and China). Third, the means by which variables are selected by the BART model favors those variables which give the cleanest lift to the overall model. This means if two variables are correlated to one another, the one with the stronger signal will be overweighed while the other will be dampened. This impacts interpretation of variable importance. While the top variables are consistent, it's important to avoid over-attributing meaning or policy decisions to some of the features with lower effects. Fourth, we were limited in taking a truly global view because we had to avoid high latitude countries due to cryosphere effects. It is known that snow and mountain glaciers can affect TWS and snow melt was not addressed by this study. Fifth, while data-driven models do not directly account for physical processes, they can be complementary to dynamical systems models. This is because data driven models—such as the one proposed in this study—are more computationally efficient; and by mining dependency mechanisms between highly-dimensional complex data, they can help formulate additional hypotheses to be tested through dynamical systems modeling frameworks. Lastly, while the number of covariates was extensive, we do not rule out that there are other significant drivers of water storage not explored here.
Further research is needed to explore additional variables and their impact on water storage trends. For instance, livestock data was not included in this analysis, as their direct consumption of water is limited. However, the virtual water required per pound of meat is much higher than per pound of plant matter. Additionally, incorporating hydrological information and downscaling from country level to watershed levels would help researchers get an even better understanding of how climate, hydrology and human-landscape interactions affect water storage. While this is difficult given the lack of granular data on local agriculture, other remote sensing techniques could be combined with the GRACE satellite data to estimate agricultural production and irrigation at finer grid scales and move away from country level statistics.
In brief, this work extends existing research into the observable changes in groundwater storage trends globally by bridging the gap between global scale analysis and local basin scale. By looking at national trends we are able to utilize commonly collected statistics by non-Governmental Organizations. Using the statistical methods outlined above we are able to model the complex relationship between different types of human factors related to groundwater trends and show that certain human factors related to the food-water-energy nexus have a stronger relationship to groundwater storage trend than others. This effort lays a foundation for additional follow-up case studies in specific countries with the appropriate data available that would both validate the statistical results laid out here as well as provide more detail required for resource management decisions.
Author Contributions
CB completed data collection, preprocessing, analysis, and inferencing. BZ implemented data collection, guided the study, and assisted in result analysis. RN contributed to the analysis. All authors reviewed and edited the manuscript.
Conflict of Interest Statement
CB was employed by company Capital One.
The remaining authors declare that the research was conducted in the absence of any commercial or financial relationships that could be construed as a potential conflict of interest.
Acknowledgments
The authors would like to acknowledge NSF (#1555582), NSF HDBE (#1826161), NASA (NNX16AF12G), Purdue Climate Change Research Center (PCCRC), and Purdue University Libraries Open Access Publishing Fund.
Supplementary Material
The Supplementary Material for this article can be found online at: https://www.frontiersin.org/articles/10.3389/fenvs.2019.00085/full#supplementary-material
Table A1. Complete list and data sources of country level indicators used as inputs to BART model.
Footnotes
1. ^https://www.esrl.noaa.gov/psd/ (accessed November 30, 2017).
2. ^http://www.unwater.org/water-facts/water-food-and-energy/ (accessed December 04, 2017).
3. ^http://www.fao.org/nr/water/aquastat/water_use/index.stm (accessed December 04, 2017).
References
Chipman, H. A., George, E. I., and McCulloch, R. E. (2010). Bart: Bayesian additive regression trees. Ann. Appl. Stat. 4, 266–298. doi: 10.1214/09-AOAS285
Drucker, H., Burges, C. J., Kaufman, L., Smola, A. J., and Vapnik, V. (1997). “Support vector regression machines,” in Advances in Neural Information Processing Systems (Denver, CO), 155–161.
Famiglietti, J., Lo, M., Ho, S., Bethune, J., Anderson, K., Syed, T., et al. (2011). Satellites measure recent rates of groundwater depletion in california's central valley. Geophys. Res. Lett. 38:L03403. doi: 10.1029/2010GL046442
Friedman, J. H. (1991). Multivariate adaptive regression splines. Ann. Stat. 19, 1–67. doi: 10.1214/aos/1176347963
Girotto, M., De Lannoy, G. J., Reichle, R. H., Rodell, M., Draper, C., Bhanja, S. N., et al. (2017). Benefits and pitfalls of grace data assimilation: a case study of terrestrial water storage depletion in india. Geophys. Res. Lett. 44, 4107–4115. doi: 10.1002/2017GL072994
Gower, J. C., Lubbe, S. G., and Le Roux, N. J. (2011). Understanding Biplots. West Sussex, UK: John Wiley & Sons.
Greatrex, H., Grimes, D., and Wheeler, T. (2014). Advances in the stochastic modeling of satellite-derived rainfall estimates using a sparse calibration dataset. J. Hydrometeorol. 15, 1810–1831. doi: 10.1175/JHM-D-13-0145.1
Hastie, T., Tibshirani, R., and Friedman, J. (2001). The Elements of Statistical Learning. New York, NY: Springer New York Inc.
Himanshu, S., Bettadpur, S., and Tapley, B. D. (2016). High resolution csr grace rl05 mascons. J. Geophys. Res. Solid Earth 121, 7547–7569. doi: 10.1002/2016JB013007
Huffman, G. J., Bolvin, D. T., Nelkin, E. J., Wolff, D. B., Adler, R. F., Gu, G., et al. (2007). The trmm multisatellite precipitation analysis (tmpa): quasi-global, multiyear, combined-sensor precipitation estimates at fine scales. J. Hydrometeorol. 8, 38–55. doi: 10.1175/JHM560.1
Kapelner, A., and Bleich, J. (2013). bartmachine: machine learning with bayesian additive regression trees. arXiv preprint arXiv:1312.2171.
Kastner, T., Erb, K.-H., and Haberl, H. (2014). Rapid growth in agricultural trade: effects on global area efficiency and the role of management. Environ. Res. Lett. 9:034015. doi: 10.1088/1748-9326/9/3/034015
Kumar, M. D., Sivamohan, M., and Narayanamoorthy, A. (2012). The food security challenge of the food-land-water nexus in india. Food Secur. 4, 539–556. doi: 10.1007/s12571-012-0204-1
Li, B., and Rodell, M. (2016). “Monitoring drought with grace data assimilation,” in Geoscience and Remote Sensing Symposium (IGARSS), 2016 IEEE International (Beijing: IEEE), 2815–2817.
Liu, J., Cao, G., and Zheng, C. (2011). “Sustainability of groundwater resources in the North China Plain,” in Sustaining Groundwater Resources (Dordrecht: Springer), 69–87.
Lo, M.-H., Famiglietti, J. S., Reager, J. T., Rodell, M., Swenson, S., and Wu, W.-Y. (2016). “GRACE-based estimates of global groundwater depletion,” in Terrestrial Water Cycle and Climate Change: Natural and Human-Induced Impacts, eds Q. Tang and T. Oki, 137–146.
McCullagh, P. (1984). Generalized linear models. Eur. J. Oper. Res. 16, 285–292. doi: 10.1016/0377-2217(84)90282-0
Mekonnen, M., and Hoekstra, A. (2011). National Water Footprint Accounts: The Green, Blue and Grey Water Footprint of Production and Consumption. UNESCO-IHE Institute for Water Education, 1.
Nie, W., Zaitchik, B. F., Kumar, S., and Rodell, M. (2017). “Groundwater withdrawals under drought: reconciling grace and models in the united states high plains aquifer,” in AGU Fall Meeting Abstracts (New Orleans, LA).
Richey, A. S., Thomas, B. F., Lo, M.-H., Reager, J. T., Famiglietti, J. S., Voss, K., et al. (2015). Quantifying renewable groundwater stress with grace. Water Resour. Res. 51, 5217–5238. doi: 10.1002/2015WR017349
Rodell, M., S. Famiglietti, J., Wiese, D., Reager, J., K. Beaudoing, H., Landerer, F., et al. (2018). Emerging trends in global freshwater availability. Nature 557, 651–659. doi: 10.1038/s41586-018-0123-1
Rodell, M., Velicogna, I., and Famiglietti, J. (2009). Satellite-based estimates of groundwater depletion in india. Nature 460, 999–1002. doi: 10.1038/nature08238
Scanlon, B. R., Faunt, C. C., Longuevergne, L., Reedy, R. C., Alley, W. M., McGuire, V. L., et al. (2012). Groundwater depletion and sustainability of irrigation in the us high plains and central valley. Proc. Natl. Acad. Sci. U.S.A. 109, 9320–9325. doi: 10.1073/pnas.1200311109
Schneider, U., Becker, A., Finger, P., Meyer-Christoffer, A., Rudolf, B., and Ziese, M. (2016). GPCC Full Data Reanalysis Version 7.0: Monthly Land-Surface Precipitation from Rain Gauges Built on GTS Based and Historic Data. Research Data Archive at the National Center for Atmospheric Research, Computational and Information Systems Laboratory. doi: 10.5065/D6000072
Swegal, H. (2017). “The rise and fall of almond prices: Asia, drought, and consumer preference,” in Beyond the Numbers: Global Economy, vol. 6 (U.S. Bureau of Labor Statistics).
Tiwari, V., Wahr, J., and Swenson, S. (2009). Dwindling groundwater resources in northern india, from satellite gravity observations. Geophys. Res. Lett. 36, 184–201. doi: 10.1029/2009GL039401
Van Dam, J. E. G., and Bos, H. L. (2004). The Environmental Impact of Fibre Crops in Industrial Applications. Hintergrundpapier.
Voss, K. A., Famiglietti, J. S., Lo, M., Linage, C., Rodell, M., and Swenson, S. C. (2013). Groundwater depletion in the middle east from grace with implications for transboundary water management in the tigris-euphrates-western iran region. Water Resour. Res. 49, 904–914. doi: 10.1002/wrcr.20078
Wanders, N., Bierkens, M. F., de Jong, S. M., de Roo, A., and Karssenberg, D. (2014). The benefits of using remotely sensed soil moisture in parameter identification of large-scale hydrological models. Water Resour. Res. 50, 6874–6891. doi: 10.1002/2013WR014639
Yang, L., Liu, M., Lun, F., Yuan, Z., Zhang, Y., and Min, Q. (2017). An analysis on crops choice and its driving factors in agricultural heritage systems–A case of honghe hani rice terraces system. Sustainability 9:1162. doi: 10.3390/su9071162
Keywords: terrestrial water stress, GRACE satellite data, global water storage variability, irrigated agriculture, crop-choice
Citation: Bruss CB, Nateghi R and Zaitchik BF (2019) Explaining National Trends in Terrestrial Water Storage. Front. Environ. Sci. 7:85. doi: 10.3389/fenvs.2019.00085
Received: 25 June 2018; Accepted: 27 May 2019;
Published: 19 June 2019.
Edited by:
Jill A. Engel-Cox, National Renewable Energy Laboratory (DOE), United StatesReviewed by:
Nitin Kaushal, World Wide Fund for Nature, IndiaSushel Unninayar, Morgan State University, United States
Copyright © 2019 Bruss, Nateghi and Zaitchik. This is an open-access article distributed under the terms of the Creative Commons Attribution License (CC BY). The use, distribution or reproduction in other forums is permitted, provided the original author(s) and the copyright owner(s) are credited and that the original publication in this journal is cited, in accordance with accepted academic practice. No use, distribution or reproduction is permitted which does not comply with these terms.
*Correspondence: C. Bayan Bruss, YmF5YW4uYnJ1c3NAY2FwaXRhbG9uZS5jb20=