- 1Institute of Atmospheric Sciences and Climate, National Research Council (CNR-ISAC), Turin, Italy
- 2Copernicus Institute of Sustainable Development, Utrecht University, Utrecht, Netherlands
- 3Senckenberg Biodiversity and Climate Research Centre, Frankfurt, Germany
- 4Department of Physical Geography and Ecosystem Sciences, Lund University, Lund, Sweden
- 5Department of Environment, Land and Infrastructure Engineering (DIATI), Politecnico di Torino, Turin, Italy
Africa is largely influenced by fires, which play an important ecological role influencing the distribution and structure of grassland, savanna and forest biomes. Here vegetation strongly interacts with climate and other environmental factors, such as herbivory and humans. Fire-enabled Dynamic Global Vegetation Models (DGVMs) display high uncertainty in predicting the distribution of current tropical biomes and the associated transitions, mainly due to the way they represent the main ecological processes and feedbacks related to water and fire. The aim of this study is to evaluate the outcomes of two state-of-the–art DGVMs, LPJ-GUESS and JSBACH, also currently used in two Earth System Models (ESMs), in order to assess which key ecological processes need to be included or improved to represent realistic interactions between vegetation cover, precipitation and fires in sub-Saharan Africa. To this end, we compare models and remote-sensing data, analyzing the relationships between tree and grass cover, mean annual rainfall, average rainfall seasonality and average fire intervals, using generalized linear models, and we compare the patterns of grasslands, savannas, and forests in sub-Saharan Africa. Our analysis suggests that LPJ-GUESS (with a simple fire-model and complex vegetation description) performs well in regions of low precipitation, while in humid and mesic areas the representation of the fire process should probably be improved to obtain more open savannas. JSBACH (with a complex fire-model and a simple vegetation description) can simulate a vegetation-fire feedback that can maintain open savannas at intermediate and high precipitation, although this feedback seems to have stronger effects than observed, while at low precipitation JSBACH needs improvements in the representation of tree-grass competition and drought effects. This comparative process-based analysis permits to highlight the main factors that determine the tropical vegetation distribution in models and observations in sub-Saharan Africa, suggesting possible improvements in DGVMs and, consequently, in ESM simulations for future projections. Given the need to use carbon storage in vegetation as a climate mitigation measure, these models represent a valuable tool to improve our understanding of the sustainability of vegetation carbon pools as a carbon sink and the vulnerability to disturbances such as fire.
Introduction
Understanding the ecological processes and feedbacks between biotic and abiotic factors that determine vegetation distributions and structure is essential for estimating vegetation responses to climate and environmental changes. Dynamic global vegetation models (DGVMs) aim at simulating the dynamical responses of vegetation to past, present, and future climate through the representation of several natural processes within terrestrial ecosystems (including vegetation geography, physiology, biochemistry, biophysics, dynamics) as well as the human influence on land use (Prentice et al., 2007; Hurtt et al., 2011; Bonan and Doney, 2018). Given the importance of vegetation feedbacks for the dynamics of the climate system (Bonan, 2008; Swann et al., 2018), DGVMs are more and more included in state-of-the-art Earth System Models (ESMs), used for historical simulations and climate projections, to represent the active role of the biosphere in the Earth system (Bonan, 2008; Bonan and Doney, 2018). Several DGVMs include a representation of fire processes (Rabin et al., 2017), which are crucial in shaping regional vegetation cover, but also have a strong influence on carbon cycle and climate (Bowman et al., 2009). The level of understanding and, consequently, implementation of wildfires in Earth-system models is still limited with respect to the many aspects in which fires influence the Earth system, for instance the effects of aerosols, peatland fires, or vegetation traits (Lasslop et al., 2019). Since anthropogenic land-use change is an important forcing for the observed climate change (IPCC, 2013), especially for CO2 emissions, many DGVMs implement not only natural ecosystems but also land-use change due to human activity, such as pastoralism and agriculture. These models are therefore a major tool to understand the relative contributions of different drivers such as climate, vegetation, and humans on fire occurrence and to quantify the effects of fire on vegetation and on the carbon cycle. Results of such models are useful to inform the general public but also policy makers. However, many DGVMs display high uncertainties in predicting the distribution of current tropical vegetation biomes, and especially of grasslands and savannas, possibly due to the way they represent the natural ecological mechanisms and feedbacks between vegetation, climate and fire (Baudena et al., 2015; Lasslop et al., 2018).
Climate-vegetation-fire relationships and vegetation structure differ between continents (Lehmann et al., 2014; Lasslop et al., 2018). We here focus on Africa (following Baudena et al., 2015; D’Onofrio et al., 2018), where most of the global annual burned area is observed (about 68%, Roy et al., 2008) and most of the tropical rainforest and many areas of savannas could be at risk of biome changes (Staver et al., 2011b).
In Africa, tropical grasslands and savannas, so-called tropical grassy biomes (TGBs), cover about one third of the land surface (Parr et al., 2014). They are characterized by a continuous layer of C4 grasses with possibly an overstory of shade-intolerant, fire-tolerant trees with varying density (Ratnam et al., 2011; Parr et al., 2014). At the wetter end of the TGB distribution range savannas transition into tropical forests (TFs), which cover about 11% of Africa (Parr et al., 2014) and are the world’s second largest tropical forest after the Amazon (Malhi et al., 2013). Tropical forests are characterized by a closed canopy with shade-tolerant, fire-intolerant species (Ratnam et al., 2011). The current ecological understanding identifies mean annual rainfall (MAR) as the main factor determining the distributions of TGBs and TFs and the transitions between them, followed by rainfall seasonality: MAR drives vegetation processes directly, by limiting the vegetation cover, and indirectly, by modulating the role of other factors (Hirota et al., 2011; Lehmann et al., 2011; Staver et al., 2011b; Case and Staver, 2018; D’Onofrio et al., 2018). Fire has an important ecological role influencing tropical vegetation (Bond et al., 2005; Higgins et al., 2007; Staver et al., 2011b). It is especially relevant for mesic savannas, where C4 grasses promote fires and maintain open canopies (Sankaran et al., 2005). In areas with similar climatic conditions fire has been suggested to maintain savannas and forests as alternative stable states through a positive vegetation-fire feedback (Hirota et al., 2011; Staver et al., 2011b; Staver and Levin, 2012). Furthermore, fire has important effects not only on vegetation dynamics but also on atmospheric composition, and Africa, along with South America, provides the largest fire emissions (Ward et al., 2012; Voulgarakis and Field, 2015; Veira et al., 2016). Since savannas are subject to frequent fires, which are rare in forests, these two biomes contribute differently to the emissions of carbon and aerosols from the burning of biomass (Grace et al., 2006). TFs are well known for their extremely high net primary productivity (NPP) and carbon stock (worldwide, about a half of the world’s carbon stored in terrestrial vegetation, e.g., Hubau et al., 2020). Although less data are available for TGBs, globally they have especially large carbon storage in their soils (up to a third of the world carbon in soil; Grace et al., 2006).
Vegetation influences the climate through biogeophysical fluxes (e.g., of water and energy) and biogeochemical fluxes (e.g., of CO2) (Bonan, 2008; Brovkin et al., 2009; Bonan and Doney, 2018). Changes in the ecosystem structure (e.g., due to deforestation in tropical forests or woody encroachment in savannas) or shifts between these biome states can alter the exchanges between the ecosystems and the atmosphere and thus may impact the climate. The direction of these changes is unclear, and predictions require accurate mechanistic modeling.
Furthermore, ongoing and expected increasing temperature and CO2 levels, altered precipitation regimes, land-use change (IPCC, 2013) and the observed decline in fire activity (Andela et al., 2017) could have large impacts on vegetation ecosystems. A complex set of interactions between these drivers could induce changes in vegetation structure and function (Midgley and Bond, 2015), possibly leading to biome shifts (Gonzalez et al., 2010; Hirota et al., 2011; Staver et al., 2011b). Shifts in vegetation connected to changes in climate, CO2 or fires were observed over the past 28,000 years in West Africa (Shanahan et al., 2016). Over the past decades, woody encroachment was observed in African savannas: one of the possible drivers is the increase of atmospheric CO2, which can enhance C3 tree growth rate (and regrowth after fire), decreasing the advantage of C4 grasses over trees (Bond, 2008; Buitenwerf et al., 2012; Mitchard and Flintrop, 2013). At the same time deforestation of African forests was observed in the 20th century (Aleman et al., 2018), and it is continuing in the new century, although at a lower rate than in other continents (Malhi et al., 2013 and references therein).
The inclusion in DGVMs of appropriate parameterizations of natural ecological processes is essential for obtaining reliable simulations and reducing the uncertainty of current and future projections of vegetation and climate states (Baudena et al., 2015; Bonan and Doney, 2018). In this study we analyze and evaluate two state-of-the-art DGVMs: LPJ-GUESS (Smith et al., 2001; Thonicke et al., 2001) and JSBACH (Lasslop et al., 2014). These two models, currently implemented in the EC-Earth ESM (Hazeleger et al., 2010, 2012) and the MPI ESM (Mauritsen et al., 2019), respectively, are characterized by different spatial resolutions (0.5° for LPJ-GUESS and 1.875° for JSBACH in this study) and complexity of the representation of vegetation and fire processes. LPJ-GUESS is a “second generation” DGVM (Fisher et al., 2010) with representation of vegetation demographics, coupled with the simple empirical First Global Fire Model (Glob-FIRM; Thonicke et al., 2001), which is commonly used in Earth system models (Kloster and Lasslop, 2017). The JSBACH version used here includes a simple representation of vegetation with grid-cell, areal-mean plant functional types, coupled with the complex process-based, rate-of-spread model SPITFIRE (Thonicke et al., 2010; Lasslop et al., 2014). In contrast to the simple fire model of LPJ-GUESS, this model includes for instance a representation of human influences and differentiation of different fuel types. In this study, LPJ-GUESS simulates only potential natural vegetation, while JSBACH includes vegetation changes due to human land use and land cover change.
The aims of this study are threefold: (1) to evaluate the relationships and interactions between climate, vegetation and fire from LPJ-GUESS and JSBACH in Sub-Saharan Africa, at different spatial resolutions; (2) to assess for which changes of environmental conditions the modeled results are reliable and (3) to assess which key ecological mechanisms need to be improved or included within these models, at different levels of complexity. To this end, we compare the relationships of tree and grass cover with MAR, rainfall seasonality and fire and the patterns of TGB and TF from models against remote-sensing data, building up on the DGVM evaluation used in the studies of Baudena et al. (2015) and Lasslop et al. (2018) and using the current knowledge of the main factors and mechanisms determining the sub-Saharan African vegetation distribution (Lehmann et al., 2011; Staver et al., 2011a,b; D’Onofrio et al., 2018). Hereby we extend the existing approaches by complementing the visual comparison of the relationships with quantifications based on generalized linear models (GLMs), and we deepen the analysis of Lasslop et al. (2018), which analyzed the performance of JSBACH in all the tropical areas, by including an evaluation of the model ability to reproduce TGB and TF distributions and characteristics following the observational analysis of D’Onofrio et al. (2018).
Materials and Methods
We evaluate the model vegetation-climate-fire interactions in sub-Saharan Africa (between 35° S and 15° N, comprising a little area of Arabian peninsula) by analyzing and comparing the relationships of percentages of tree (T) and grass cover (G) with mean annual rainfall (MAR [mm year–1]), average rainfall seasonality index (SI) (Walsh and Lawler, 1981) and average fire intervals (AFI [year]). The analysis is performed for both model data and observations. Additionally we investigate the ability of models to simulate tropical grassy biomes (TGB) and tropical forest biomes (TF) by comparing their modeled and observed distributions and characteristics. The following subsections report the model descriptions and simulation setup (“DGVMs: Main Characteristics and Experimental Setup”), the information about the observational datasets (“Observational Datasets”), the descriptions of the variables, and the methods applied to derive them for the comparison (“Variables for the Comparison”) and the statistical analysis (“Statistical Analysis for Model-Observation Comparison”).
DGVMs: Main Characteristics and Experimental Setup
JSBACH
JSBACH [Jena Scheme for Biosphere–Atmosphere Coupling in Hamburg (Raddatz et al., 2007)] includes the DYNVEG module for natural vegetation dynamics (Brovkin et al., 2009; Reick et al., 2013), a component for anthropogenic land use change (Reick et al., 2013) based on the Harmonized Protocol by Hurtt et al. (2011) and the SPITFIRE model for fire dynamics (Thonicke et al., 2010) with modifications described in Lasslop et al. (2016). Natural vegetation comprises eight plant functional types (PTFs), five of which represent tropical vegetation: deciduous and evergreen trees, C3 and C4 grasses, and raingreen shrub. C3 grasses typically dominate the temperate regions, but there can still be a mixture in tropical areas. The competition between natural PFTs of the same group (i.e., woody or grass classes) is based on NPP, whereas intergroup competition for uncolonized habitable land is driven by disturbances (fire and windthrow). In addition to natural PFTs JSBACH includes crops and pastures as agricultural land cover PFTs, both with C3 and C4 photosynthetic pathways. The transitions between natural and anthropogenic vegetation classes follow simple rules described in detail in Reick et al. (2013). The interaction between fires and vegetation is simulated by coupling the vegetation module with the complex process-based fire model SPITFIRE. Using information about vegetation composition, fuel amount of different fuel size classes and characteristics (such as fuel bulk density and surface area to volume ratio), and soil moisture from JSBACH, SPITFIRE computes burnt area and plant mortality that reduce litter carbon, vegetation biomass and cover fraction. Pasture PFTs are handled as grassland by SPITFIRE but have a slightly higher fuel bulk density with respect to natural grass, whereas croplands are excluded from fire dynamics. Further details on the implementation of the JSBACH-SPITFIRE coupling can be found in Lasslop et al. (2014; 2016; 2018).
LPJ-GUESS
LPJ-GUESS (Lund-Potsdam-Jena General Ecosystem Simulator; Smith et al., 2001, to which we refer for a detailed description) is a stand-alone 2nd generation DGVM that includes the Glob-FIRM (Thonicke et al., 2001) module for fire dynamics. It simulates vegetation distribution from plant specific environmental limits and the competition for light, space, and soil resources. Global natural plants are described by 12 PFTs, which include C4 grass, a raingreen deciduous tree and two types of evergreen trees that represent tropical vegetation. Only natural PFTs were considered in this study. Vegetation dynamics are simulated within a number of replicate patches representing cohorts of different time-since-last-disturbance within each grid cell. These multiple patches are simulated to account for variation in vegetation dynamics due to stochastic processes such as establishment, mortality and disturbance. In this study the model was run in “cohort mode,” in which, for woody PFTs, individuals within a cohort (age class) in the same patch are represented by a single average individual. To estimate burned area and fire effects on vegetation within LPJ-GUESS the fire-model Glob-FIRM has been applied, which simulates fire occurrence based on temperature, fuel load (litter), and moisture. In a fire both live and litter biomass are consumed following a PFT-depending mortality, where each PFT has a specific fire-resistance parameter defining the minimum percentage of a cohort surviving a fire.
Experimental Setup and Model Outputs
JSBACH was run in offline mode, forced by climatological data from a historical simulation of the MPI-ESM (version 1.1) over the period 1850–2005, with a horizontal resolution of 1.875°× 1.875°. The model was forced with climate model outputs, because it is usually used in a coupled mode and therefore vegetation parameters, for instance climatic limits, are not tuned for observed meteorological forcing. The land-use transition data were taken from Hurtt et al. (2011). The simulation used in this study was the same as used in Lasslop et al. (2018) to which we refer to for more detail.
LPJ-GUESS was run in the period 1901–2015, with a horizontal resolution of 0.5°× 0.5°. In this run 25 replicate patches were simulated in each grid cell. Since our aim is to evaluate the ability of models in simulating the main ecological natural processes, which is crucial for studying, e.g., the effects of climate-change mitigation solutions (e.g., Bastin et al., 2019), only natural (potential) vegetation was simulated by LPJ-GUESS (i.e., no anthropogenic land use). The CRU-NCEP5 dataset (Wei et al., 2014) was used as input of daily meteorological data. The simulation (1901–2015) was performed after 500 years of spin-up.
For the comparison with the observations, model variables were obtained from the model outputs (variables T, G, and AFI) and inputs (variables MAR and SI). These were computed over the period 2000–2010 for LPJ-GUESS (as the observational data, see below) and 1996–2005 for JSBACH. The simulations of JSBACH adopted the CMIP5 protocol, where for instance land use forcing ended in the year 2005, therefore the reference period was a compromise between having the same reference period and sufficient years to achieve robust mean values.
Observational Datasets
We compared the inputs/outputs of model simulations with observational variables derived from remote sensing datasets within the period 2000–2010. We use the rainfall product of the tropical rainfall measuring mission (TRMM 3B42), with 0.25° original resolution, to derive MAR and SI. AFI was derived from the monthly MCD45A1 (Collection 5.1) burnt area satellite product, with original 500 m resolution, available from April 2000 (Roy et al., 2002, 2005, 2008). T and G were obtained from the products “percent tree cover,” “percent non-tree vegetation” and “percent non-vegetated” of MODIS vegetation continuous fields (MOD44B VCF, version 051), with original 250 m resolution (Townshend et al., 2011). Notice that for year 2000 we substituted the original non-vegetated cover data with 100% – “non-tree vegetation cover” – “tree cover” of the same year, following the VCF layer definition, because of the presence of anomalous values of the non-vegetated product in the African western part. To identify tropical grassy and forest biomes we used the ESA global land cover map (ESA CCI-LC, v 1.6.1; 5-year-averaged dataset centered in 2010, with original 300 m resolution). These are the same observational data described in D’Onofrio et al. (2018), to which we refer to for more details. Observational data were aggregated in space to the resolution of LPJ-GUESS (0.5°) and of JSBACH (1.875°).
Variables for the Comparison
Rainfall Seasonality Index
The variable SI is the rainfall seasonality index proposed by Walsh and Lawler (1981), which we obtained as the averages over the years of the annual index defined as for year i, where xn,i is the rainfall of month n, and Ri is the annual rainfall. This index can vary from 0, when annual rainfall is uniformly distributed within the year, to 1.83, when annual rainfall occurs in 1 month.
Average Fire Intervals
As fire variable we used the average fire intervals (AFI), which is the expected return time of fire at any point in a grid cell (Johnson and Van Wagner, 1985). This was obtained as the inverse of the average annual burnt area fraction (BA [year–1]) in each grid cell (AFI = 1/BA). For the observational dataset, BA was computed as in D’Onofrio et al. (2018) (using a method derived from Lehsten et al., 2010). First, we converted the monthly maps to annual maps setting to one all the 500 m pixels classified as burned one or more times during the year, and to zero valid pixels that did not experience any fire. Then, for each year we computed the annual burned area fraction as the mean of the “burned” pixels within each large-scale grid cell (0.5° and 1.875°). Finally, we averaged over the years. For both models AFI was obtained using the annual burnt area outputs.
In the analysis we used the decadal logarithm of AFI, log10(AFI), which corresponds to −log10(BA), because AFI values covered different orders of magnitude. In order to avoid infinite values when BA = 0, we added to modeled and observed BA a small constant (i.e., AFI = −log10(BA+a), where a = 0.0001 year–1), such that maximum AFI is equal to 10000 years.
Vegetation Cover
For the observational datasets, we derived T and G averaging in time and space the yearly percentage of tree and non-tree vegetation cover MODIS products. Since MODIS does not detect tree cover in the presence of trees smaller than 5 m (Bucini and Hanan, 2007), assuming that these are mostly shrubs, we used the ESA global land cover map (ESA CCI-LC, v 1.6.1; 5-year-averaged dataset centered in 2010) to remove grid cells with equal or more than 50% of the area occupied by shrubland (ESA CCI-LC codes 120, 122) (D’Onofrio et al., 2018). In this way we assumed that the non-tree vegetation cover was representative mostly of the grass cover.
Since LPJ-GUESS was set to simulate only natural vegetation, and in order to mainly focalize on potential vegetation, we used the same procedure to remove grid cells with more than 33% of the areas affected by human activity, such as croplands and urban areas, and/or also covered by inland and coastal water, permanent snow or ice (ESA CCI-LC codes ≤40, 190, 210, 220) to have reliable vegetation cover and fire values. Since our aim is to evaluate the relationships between biotic and abiotic variables, and not the spatial distributions of these variables, we did not seek to have an exact correspondence between observational and model data locations. However, in order to compare datasets with approximately the same number of grid cells (and approximately the same areas), we filtered out from the model datasets the same grid cells excluded from the observation datasets based on the ESA CCI-LC map. We also removed grid cells with MAR larger than 2500 mm year–1 following D’Onofrio et al. (2018) from the observational and model data, as in the observations at 0.5° resolution few grid cells (22 out of the selected 3156) had larger precipitation values.
In order to have comparable vegetation cover between models and observations we rescaled the observed tree cover (Tresc) and consequently the observed grass cover (Gresc). In fact, in the MODIS data the percentage of tree cover represents the percentage of a grid cell covered by canopy, which refers to the fraction of light obstructed by tree canopies equal to or greater than 5 m in height, and reaches a maximum around 80% (Hansen et al., 2003). In JSBACH percent tree cover represents the crown cover which can reach 100%, while in LPJ-GUESS it represents the annual maximum foliar projective cover that can exceed 100% because of individual tree overlap. Thus, for rescaling the observations, given that the MOD44B non-tree vegetation layer (grass) is derived from tree and bare cover (B, the percent non-vegetated product of MOD44B) as G = 100%-T-B, we maintained the bare fraction and required that also the rescaled tree and grass covers satisfy Tresc+B+Gresc = 100%, where Tresc = αT is the tree cover rescaled by a factor α. Notice that in this expression we require Tresc to be between 0 and 100%. We can thus write that for all grid cells Tresc = αT ≤ 100%-B, from which we can find α = min [(100%-B)/T], where the minimum is computed over all the observational data analyzed. The rescaled grass cover is then simply derived as Gresc = 100% – Tresc – B. In the selected grid cells, α was equal to 1.2152 for the data at 1.875° resolution and to 1.1809 for the data at 0.5° resolution. In the following, for the observations, T and G refer to Tresc and Gresc.
For the models, T and G were computed as the sum of the mean cover of tree PFTs and grass PFTs, respectively. For JSBACH we included shrub PFTs in the tree cover because, since shrubs are woody vegetation, they are physiologically more similar to trees than to grasses. In order to exclude croplands as in the observations, for JSBACH we did not include the cropland PFTs in the grass cover. Consequently, we rescaled JSBACH average vegetation cover and average annual burnt area dividing by the area not occupied by croplands, and we removed grid cells where the area occupied by croplands was greater than 1/3. Notice that, although pasture PFTs are anthropogenic land cover types, we included them in the JSBACH grass cover because they are part of real TGBs (Hempson et al., 2017). In LPJ-GUESS, since total vegetation cover can exceed 100%, for each year we rescaled the vegetation cover in each grid cell when this occurred dividing it by the total vegetation (i.e., the sum of all PFT covers) in order to have values between 0 and 100%. With this rescaling we maintain the tree-grass ratio in the grid cell, although there would be grass overlapped by trees (when tree cover exceeds 100%). We argue that this method is more appropriate than setting G = 0 when T ≥ 100%, which, while appropriate for studies involving albedo, would lead to a systematic underestimation of grass cover. However, this approach can potentially lead to an overestimation of the grass cover with respect to observations, since MODIS plausibly cannot detect grass cover below the tree canopy.
The final observational datasets consisted in 3134 grid cells at 0.5° resolution and 209 at 1.875° resolution. Hereafter these two datasets are also called Obs. 0.5° and Obs. 1.875°. The final model datasets consisted in 3141 grid cells for LPJ-GUESS and 208 grid cells for JSBACH. Hereafter we refer to input and output data of the two DGVMs as the LPJ-GUESS dataset and the JSBACH dataset.
Tropical Grassy Biome and Tropical Forest
For the observational datasets we identified grid cells with major presence of TGBs and TFs using the ESA-CCI-LC map. Following D’Onofrio et al., 2018, we classified a grid cell as TGB when ≥50% of its area was covered by deciduous trees and grassland classes (ESA CCI-LC codes 60–62, 130) and TF when covered by evergreen and flooded tree classes (ESA CCI-LC codes 50, 160, 170).
For the model outputs, we classified a grid cell as TGB when the sum of the covers of broadleaved raingreen tree PFTs and of the C4 grass PTF in LPJ-GUESS, and of tropical broadleaved deciduous tree PFT, C4 grass PFT and C4 pasture PFT in JSBACH was ≥50% of the total vegetation in the grid cell. Grid cells were identified as TF in both models when the total cover of tropical broadleaved evergreen tree PFTs was ≥50% of the total vegetation in the grid cell. This step was performed after the rescaling of the data (see above). Notice that LPJ-GUESS has two different PFTs for the tropical broadleaved evergreen trees which differ in the shade tolerance trait and in longevity, which we included in the TF definition because, although forest trees are broadly characterized by shade tolerant trees, forest tree pioneer species typically have a short life and are light demanding, thus shade-intolerant (although not necessarily evergreen) (Ratnam et al., 2011; Gignoux et al., 2016).
Statistical Analysis for Model-Observation Comparison
We analyzed the dependences of T and G on MAR, SI and log10(AFI) using Generalized Linear Models (GLMs) (McCullagh and Nelder, 1989). In order to understand the importance of each abiotic factor separately and to avoid combinations of collinear variables, we computed only univariate GLMs with terms up to the third order. We used a binomial error distribution with a logit link function for fitting tree and grass cover fractions (Dobson, 2002; Schwarz and Zimmermann, 2005). GLMs were classified based on the Akaike information criterion (AIC, Akaike, 1974), such that the best model had the lowest AIC score. We selected only GLMs with AIC smaller than the intercept-only model, while GLMs with AIC larger than the intercept-only GLM were considered not significant. The goodness-of-fit was evaluated with the fraction of deviance explained, R2 (also named D2; Guisan and Zimmermann, 2000; Schwarz and Zimmermann, 2005).
The prevalent mechanisms determining observed biome occurrence and distribution change with MAR (Sankaran et al., 2005; Lehmann et al., 2011) and in particular they vary in three mean annual rainfall ranges (Accatino et al., 2010; D’Onofrio et al., 2018). We thus performed the GLM analysis also separately for three intervals of MAR, recalculated from the observational datasets following the approach of D’Onofrio et al., 2018: low (R1: MAR ≤ 590 mm year–1), intermediate (R2: 590 mm year–1 < MAR < 1200 mm year–1) and high (R3: MAR ≥ 1200 mm year–1) annual rainfall. The ranges were identified from the changes of the relative tree-grass dominance (represented by T-G) in its dependence on MAR in the observational data (see Supplementary Figure S1A,C, in Supplementary Material also for details on the threshold selection). We found these thresholds to be quite similar for the observational datasets at both resolutions and to be fairly close to those of D’Onofrio et al. (2018). In order to evaluate the DGVM performances with respect to the observations (that we assumed to represent reality), we used the same intervals for both observed and model data. In the analyses within each of the three ranges, we computed univariate GLMs with terms only at the first order. The GLM analysis performed separately for the three MAR ranges was complemented with the comparison of the variable distributions through box plots and of the correlations between the abiotic variables (using Pearson’s r coefficient).
In R1 there were 1247 grid cells for Obs. 0.5°, 82 for Obs 1.875°, 1186 for LPJ-GUESS and 58 for JSBACH; in R2 there were 953 grid cells for Obs. 0.5°, 64 for Obs 1.875°, 699 for LPJ-GUESS and 52 for JSBACH; in R3 there were 934 grid cells, Obs. 0.5°; 63 grid cells for Obs 1.875°,1256 grid cells for LPJ-GUESS and 98 grid cells for JSBACH.
Results
Overall Dependence of Vegetation Cover
Overall, the best predictor for observed T was MAR (as in D’Onofrio et al., 2018), and this was captured by both JSBACH and LPJ-GUESS (Figure 1 and Table 1). However, modeled T grew over the entire MAR domain, although with a reduced steepness at higher MAR (Figures 1B,D), where closed forest was attained, while the fit for observed T reached a saturation at lower rainfall values (around ca. 1700–2000 mm year–1, Figures 1A,C), probably due to the larger spread of observed tree cover values above these rainfall levels with respect to the models.
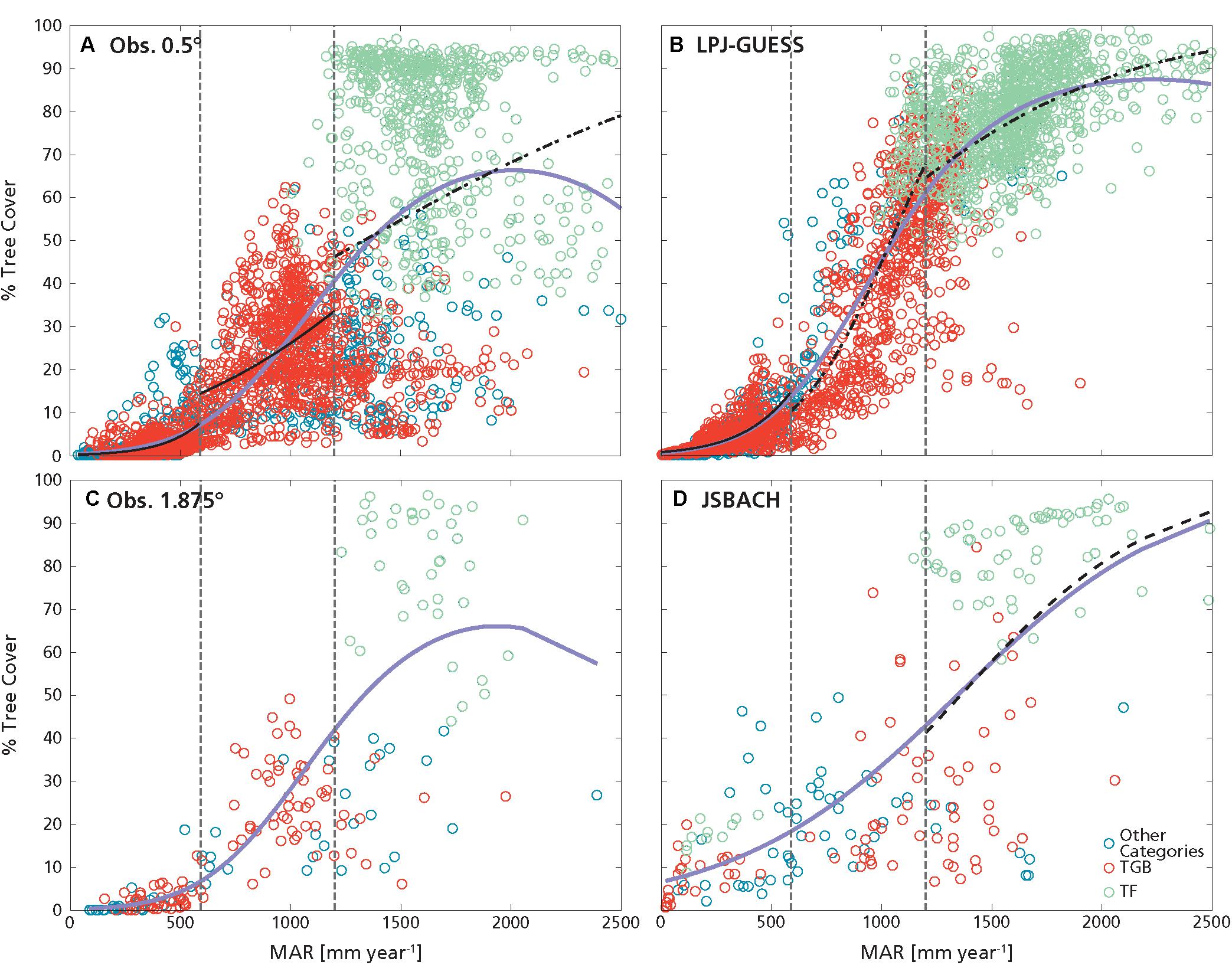
Figure 1. Percentage tree cover as a function of mean annual rainfall (MAR): (A) obs. 0.5°, (B) LPJ GUESS, (C) obs. 1.875°, and (D) JSBACH. Dashed vertical lines delimit the ranges of low MAR (R1: MAR ≤ 590 mm year–1), intermediate MAR (R2: 590 mm year–1 < MAR < 1200 mm year–1) and high MAR (R3: MAR ≥ 1200 mm year–1). Black lines are the GLM fit of tree cover with MAR (Table 2 and Supplementary Figures S6–S8). Lilac lines represent the GLM fit performed over all data (Table 1). Lines are continuous when the fits are the best GLMs explaining tree cover variation within the MAR range (i.e., with minimum AIC). If no fits are shown in a MAR range it means that there was no significant dependence of tree cover on MAR in that range. Green circles are grid cells with predominance of forest (TF), red circles with predominance of tropical grassy biome (TGB). Blue circles are grid cells with other or no predominant PFTs/biome.
The best predictor for observed G was log10(AFI) (as in D’Onofrio et al., 2018), at all resolutions, and overall G decreased with fire intervals, i.e., it increased with fire frequency, and this relationship had a predictive power (deviance explained) of 55% (Figures 2A,C and Table 1). JSBACH data display the same decrease, although steeper and with narrower spread with respect to the observations (Figures 2C,D), and this GLM explained 90% of the deviance. In LPJ-GUESS data the best predictor for G was MAR, whereas MAR was the least important factor explaining G in the observations (Table S4), albeit with a similar relationship (Figures 3A,B). In this model log10(AFI) was the second-best predictor for G (Supplementary Table S4): modeled G decreased with log10(AFI) up to about 100 years, with a steeper slope than in the observations (Figures 2A,B). Furthermore, the climatological average of fire intervals in LPJ-GUESS did not present fires with average intervals smaller than ca. 3 year (i.e., with average burned area greater than ca. 0.33 year–1), but it also had few grid cells with AFI greater than 1000 years. Still, we verified that in individual years also lower or higher values of fire intervals could be found.
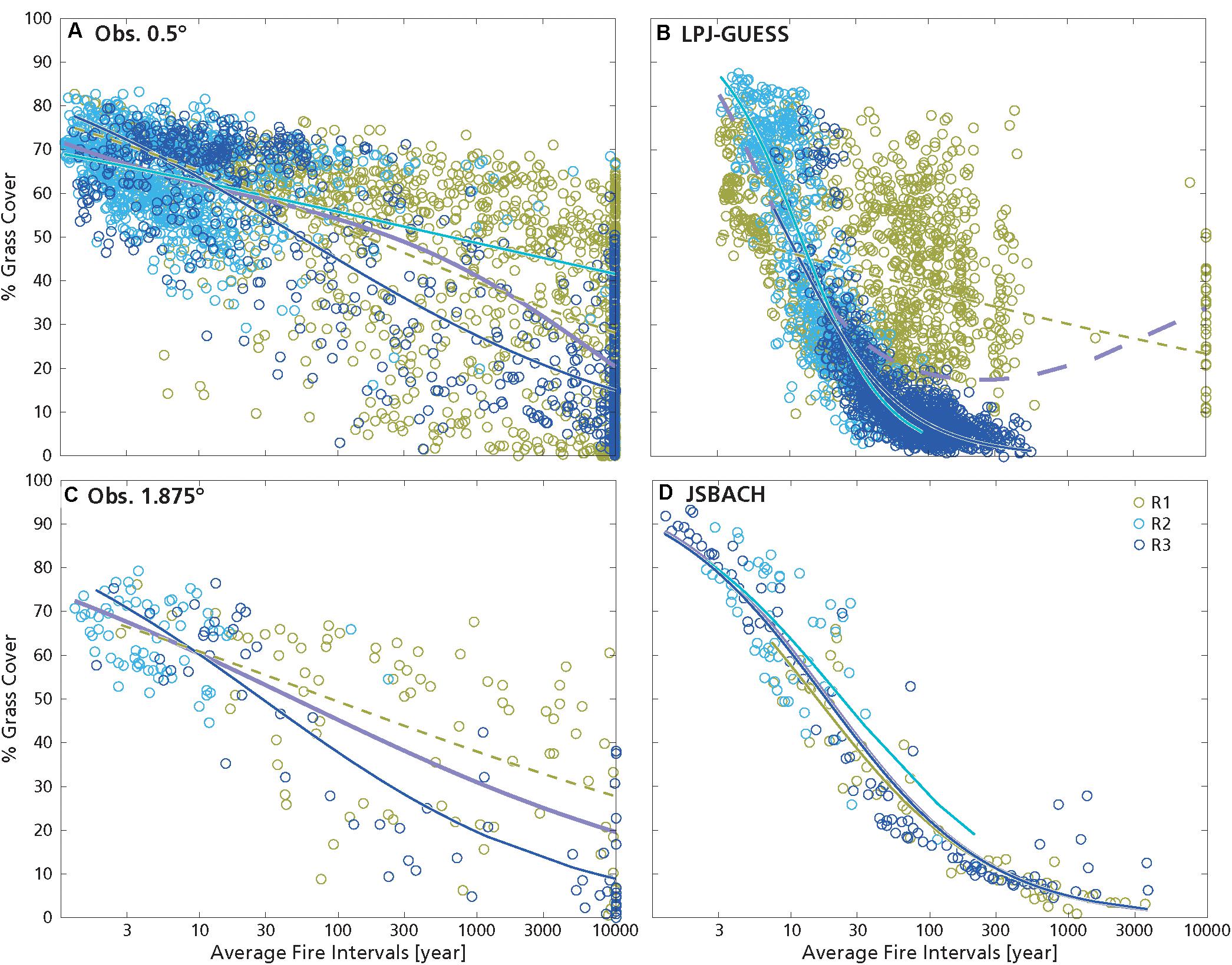
Figure 2. Grass cover as a function of average fire intervals (in logarithmic scale): (A) obs. 0.5°, (B) LPJ-GUESS, (C) obs. 1.875°, and (D) JSBACH. Green circles: grid cells in R1 (MAR ≤ 590 mm year–1); Light blue circles: grid cells in R2 (590 mm year–1 < MAR < 1200 mm year–1); blue circles: grid cell in R3 (MAR ≥ 1200 mm year–1). Lines are the GLM fits of grass cover with log10(AFI) within the three MAR ranges (with the same colors as the circles) and over all data (lilac line). Only significant fits are shown. Lines are continuous when the fits are the best GLMs explaining grass cover variation (i.e., with minimum AIC, Table 2).
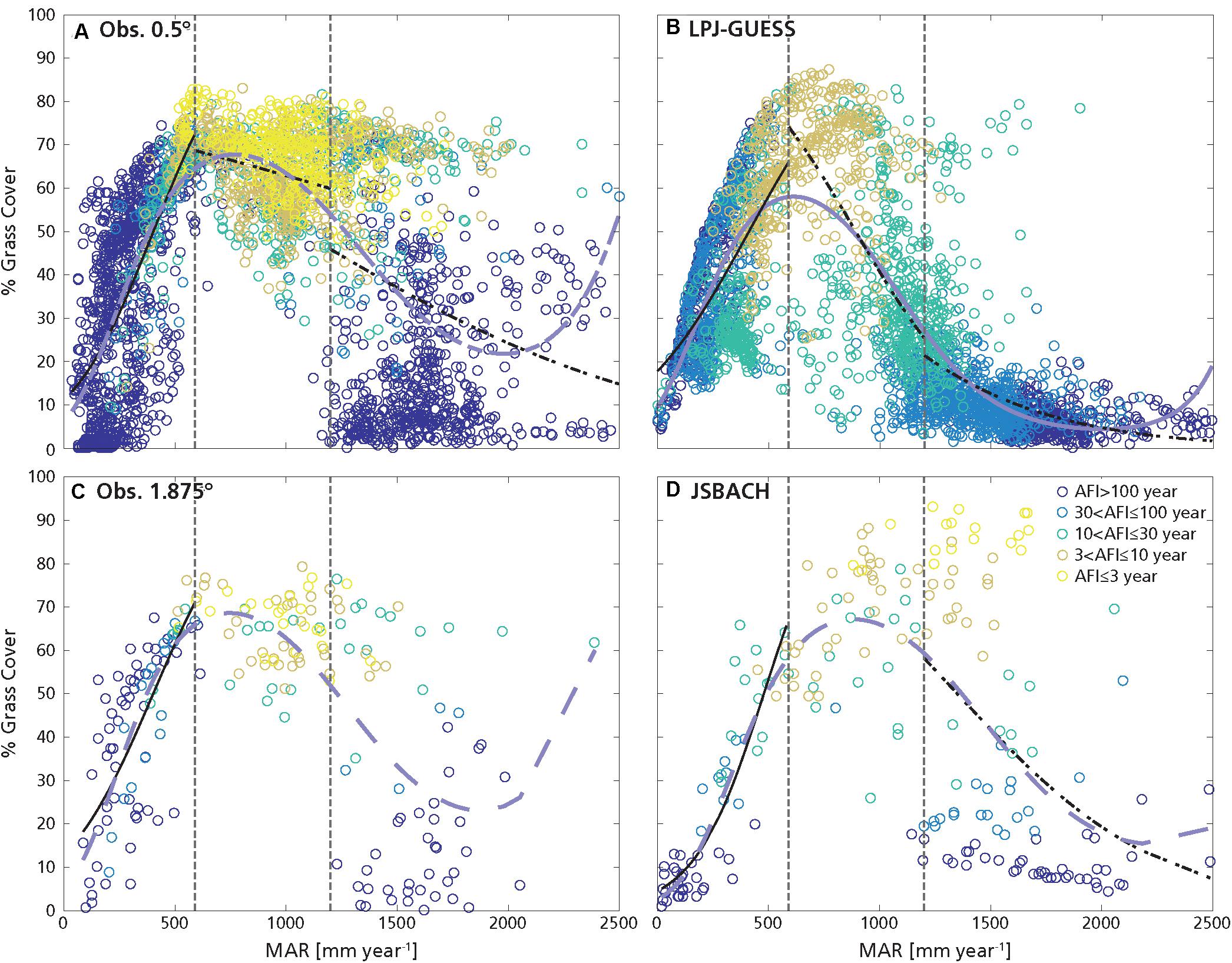
Figure 3. Same as Figure 1 but for grass cover. The colors of the circles indicate the average fire intervals (AFI, see legend for values). Panels: (A) obs. 0.5°, (B) LPJ-GUESS, (C) obs. 1.875°, and (D) JSBACH.
Mean annual rainfall was the best predictor for the total vegetation cover (i.e., T+G) in all datasets and both models simulated the observed sigmoidal-like relationship (Figure 4 and Table 1 and D’Onofrio et al., 2018). Especially the JSBACH relationship was in good agreement with observations (Figures 4C,D). Total vegetation cover from LPJ-GUESS grew with MAR with larger spread than the observations, especially above ca. 500 mm year–1 (Figures 4A,B). Furthermore, it showed a marked upper bound, which was noisier in the observations (Figures 4A,B).
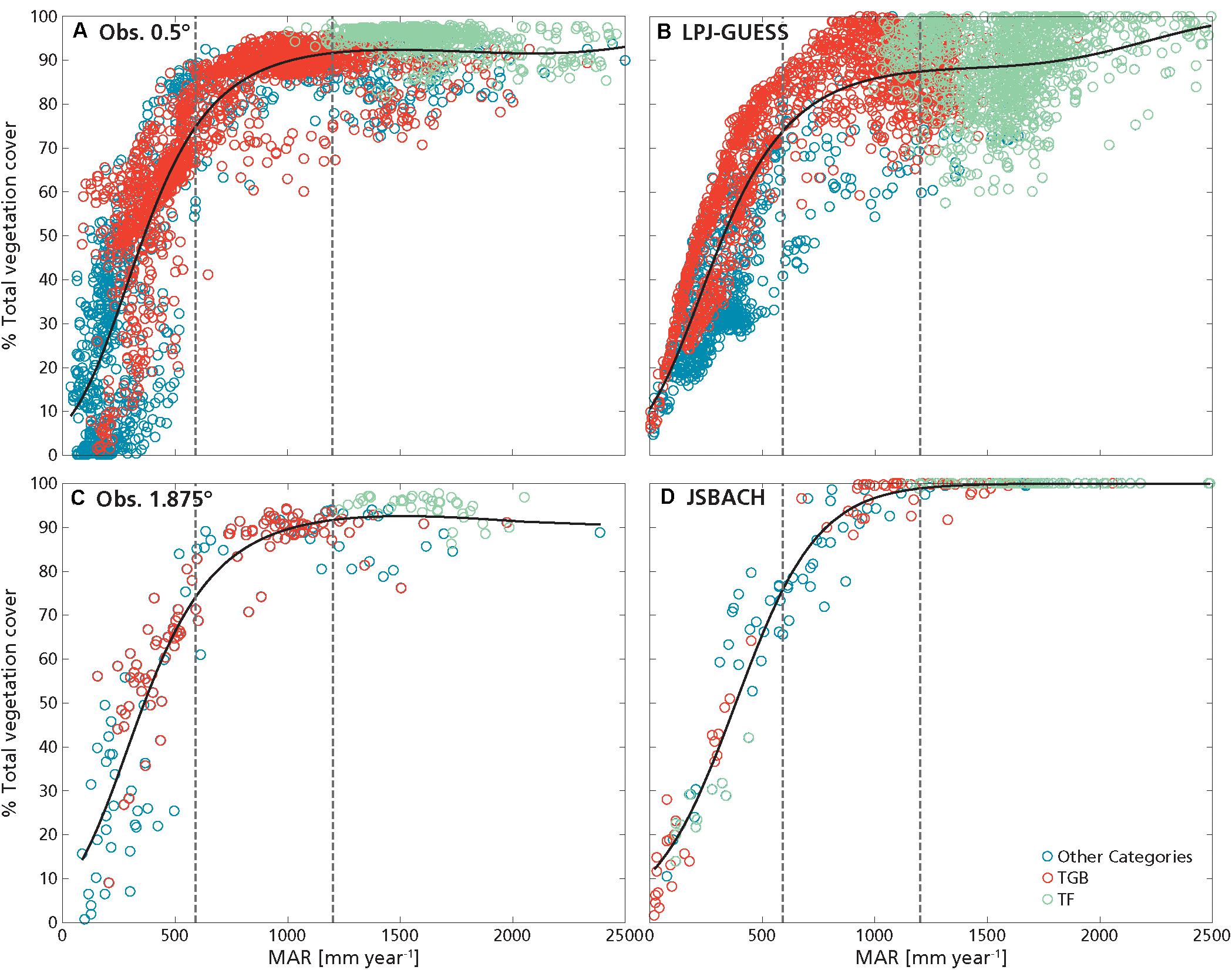
Figure 4. Total vegetation cover (tree cover+grass cover) as a function of MAR: (A) obs. 0.5°, (B) LPJ-GUESS, (C) obs. 1.875°, and (D) JSBACH. Continuous lines are the best GLM fits of total vegetation cover with MAR (that was the best predictors for all datasets, see Table 1). Note that for Obs 1.875° we show the fit with MAR at third order (the second best fit after the one with MAR at the second order, ΔAIC = 1.55), because they have similar predictive power but the fit with MAR3 looks better in agreement with the observations (Supplementary Table S5). Green circles are grid cells with predominance of tropical forest (TF), red circles with predominance of tropical grassy biome (TGB). Blue circles are grid cells with other or no predominant PFT/biome.
In the following, we report the results of the analysis performed in the three MAR ranges separately. As explained in the methods section, we used the MAR thresholds obtained from the observational datasets. This choice was reasonable as in the models the relative tree-grass dominance showed qualitatively similar changes in the dependence on MAR occurring at similar MAR thresholds as in the observations (Supplementary Figure S1).
Low Mean Annual Rainfall
At low annual precipitation (MAR ≤ 590 mm year–1) in the observations grasses always dominated over trees (i.e., G > T), and in most of the grid cells on average rainfall seasonality was marked with a long dry season and fires were rare (Figure 5; see also D’Onofrio et al., 2018). There was a fairly good agreement especially between LPJ-GUESS data and observations, and this model was generally able to simulate the main relationships of increasing tree and grass cover with MAR (Figures 1A,B, 3A,B, 6, 7), although it overestimated fire frequency. Overall, JSBACH underestimated grass cover and overestimated tree cover (Figure 5), but it was able to simulate the observed increase of grass cover with MAR, although the best predictor for modeled grass cover was fire (Figures 7C,D).
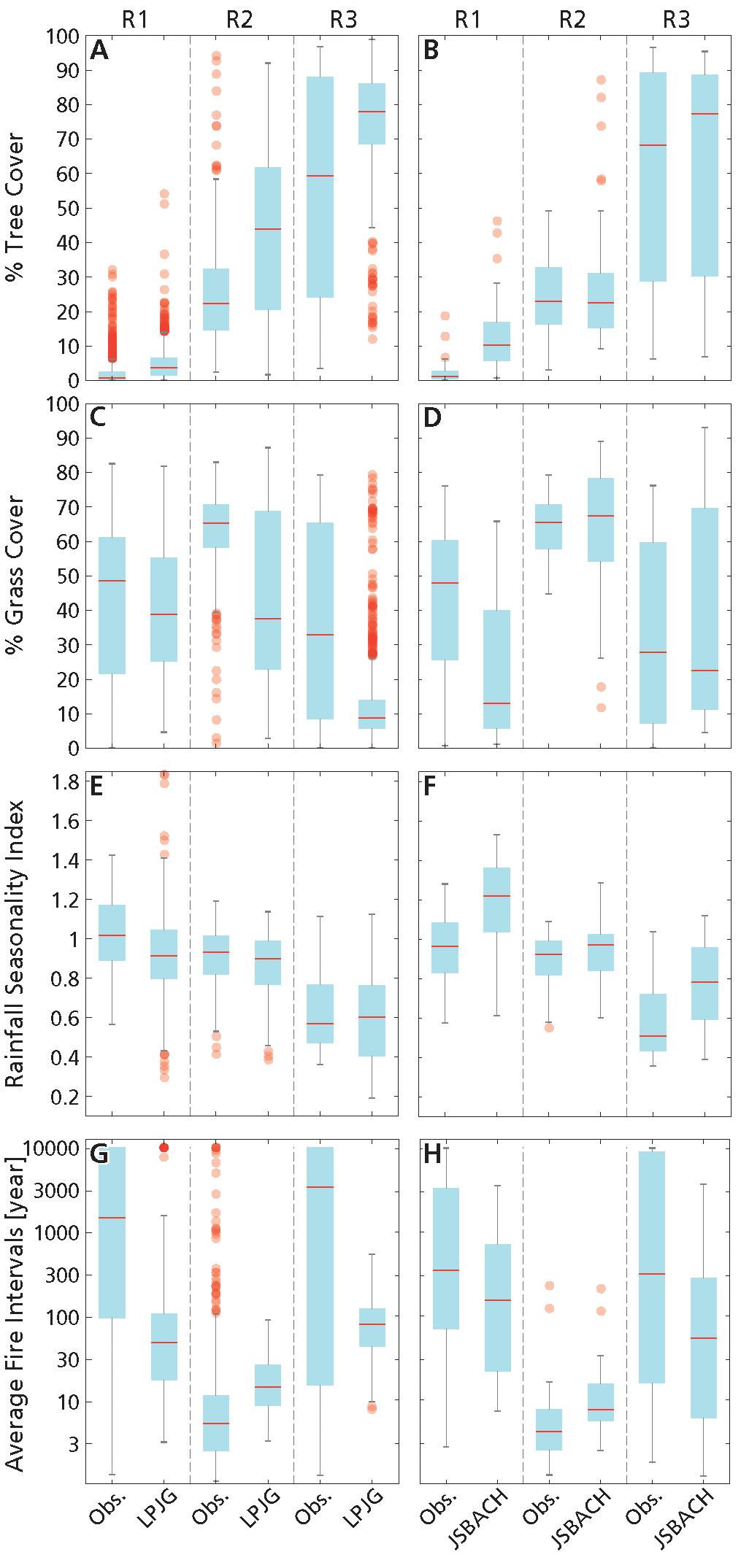
Figure 5. Box plots of vegetation cover and abiotic variables in the three mean annual rainfall ranges: (A,B) percentage tree cover, (C,D) percentage grass cover, (E,F) rainfall seasonality index, (G,H) average fire intervals (in logarithmic scale) from obs. 0.5° and LPJ-GUESS (left column) and from obs. 1.875° and JSBACH (right column). Vertical lines delimit the ranges of low MAR (R1: MAR ≤ 590 mm year–1), intermediate MAR (R2: 590 mm year–1 < MAR < 1200 mm year–1) and high MAR (R3: MAR ≥ 1200 mm year–1). Red lines are the medians. The top and bottom of each box are the 25th and 75th percentiles, respectively. The extremes of the whiskers correspond to the highest and lowest values within a range defined by the 75th and 25th quartiles +/–, respectively, 1.5 times their interquartile range. Red symbols are outliers.
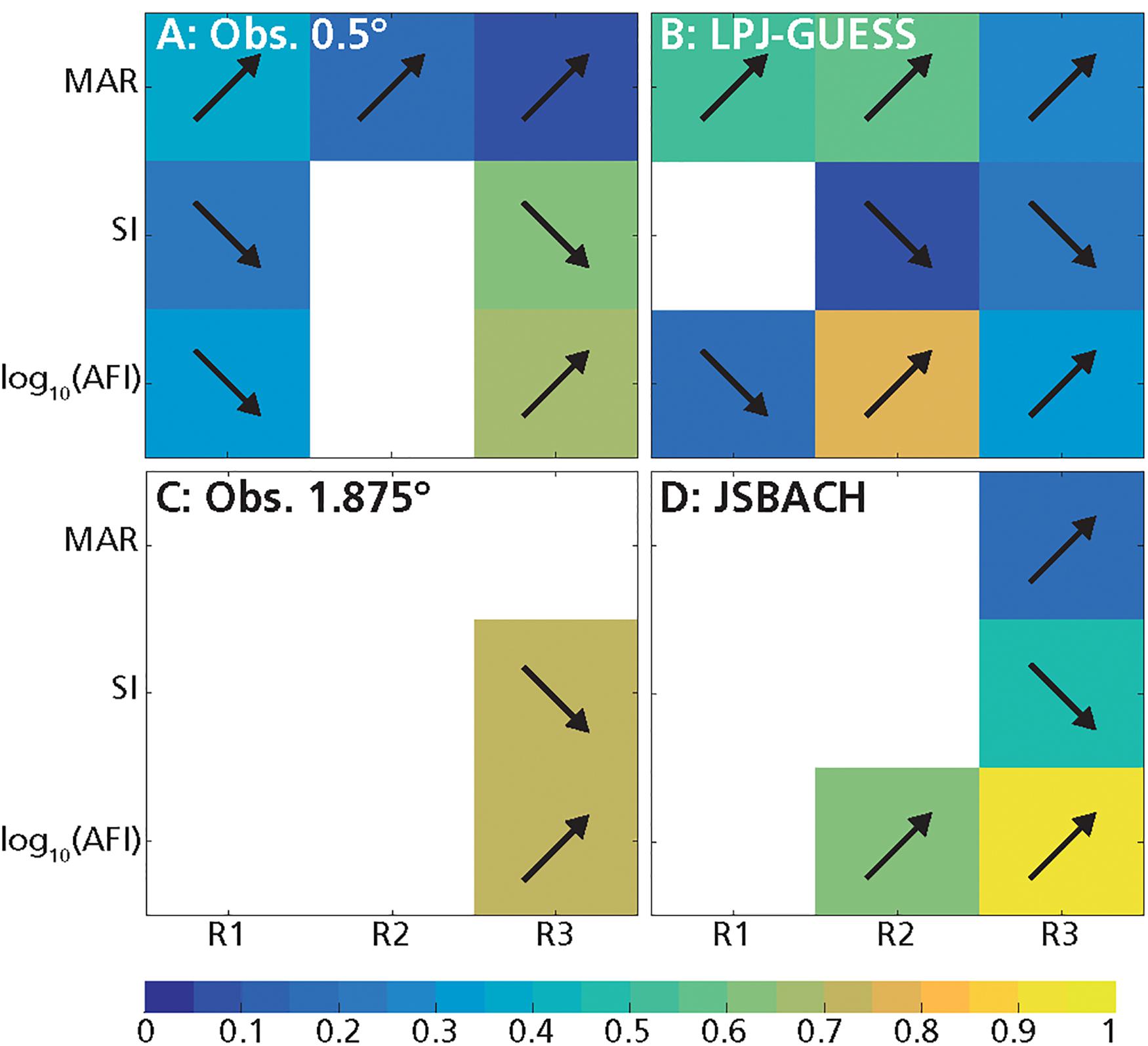
Figure 6. Deviance explained (R2; color scale) of the GLMs of tree cover with the three abiotic predictors at low mean annual rainfall (MAR) (R1: MAR ≤ 590 mm year–1), intermediate MAR (R2: 590 mm year–1 < MAR < 1200 mm year–1) and high MAR (R3: MAR ≥ 1200 mm year–1): (A) obs. 0.5°, (B) LPJ-GUESS, (C) obs. 1.875°, (D) JSBACH. Arrows indicate the tendencies of the relationships between tree cover and abiotic factors (increasing or decreasing). White grid cells indicate that there were no significant relationships.
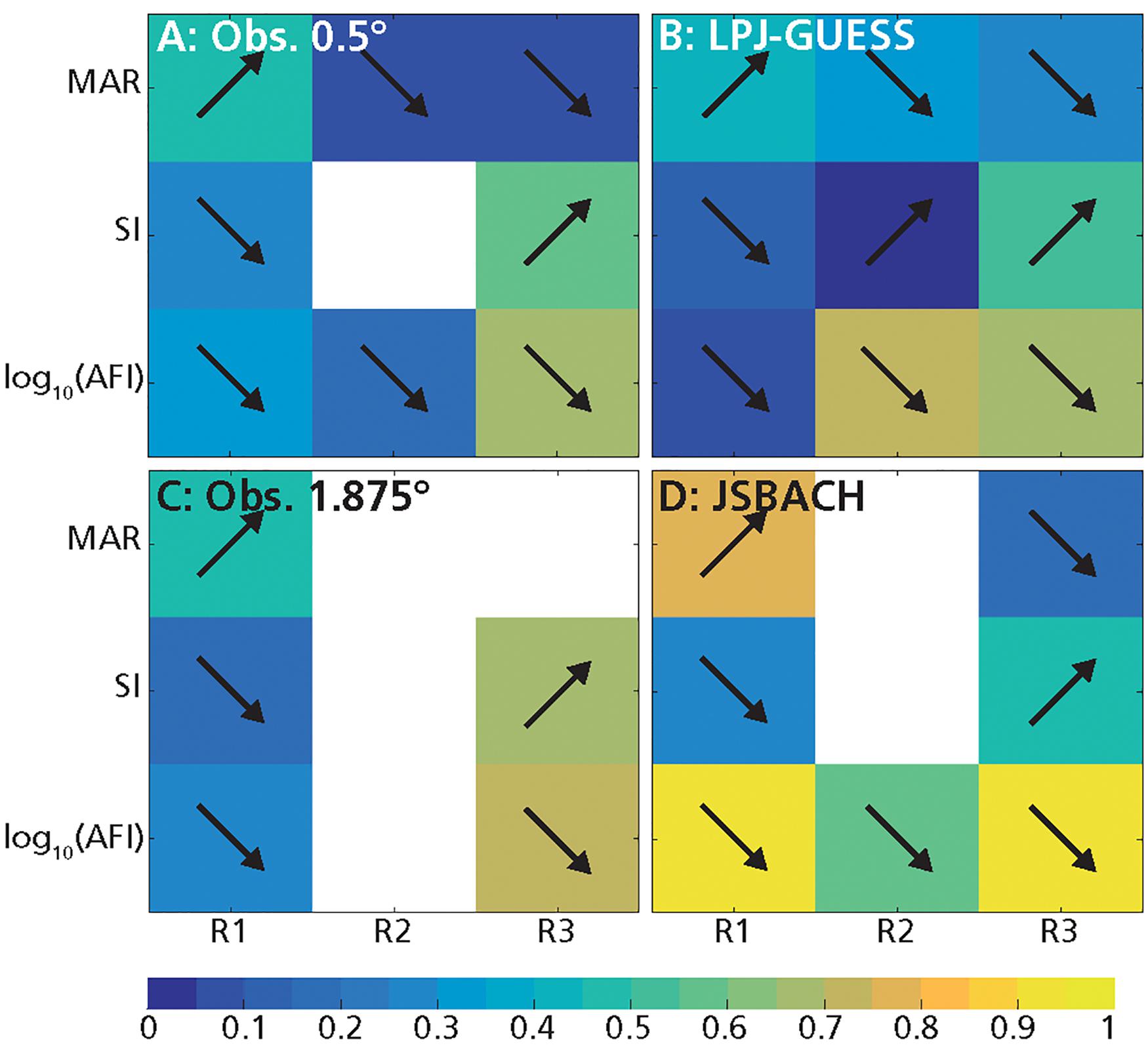
Figure 7. Same as Figure 6 but for grass cover.
For the grid cells in this MAR range, observed grass cover was larger than tree cover, which was very low. This was simulated reasonably well by both models (Figures 5A–D). However, in the models, the medians and ranges of grass distributions were generally underestimated, while those of tree cover distributions were overestimated with respect to the observations. These discrepancies were stronger for JSBACH data, whose grass and tree cover medians were very close to each other, and in some grid cells, T was even larger than G (Supplementary Figure S1D). For both the observations and the models the grid cells in this precipitation range had the highest rainfall seasonality indices and generally rare fires (Figures 5E–H). In LPJ-GUESS, fires were generally more frequent than in the observations for most of the grid cells (Figure 5G). With respect to the observations, average rainfall seasonality index was overestimated in JSBACH data (Figure 5F), while SI from LPJ-GUESS dataset compared relatively well (Figure 5E).
There was a quite good agreement between the correlation coefficients between MAR, SI and log10(AFI) of model and observational datasets, except for the correlation between log10(AFI) and SI that was not significant for the observations (r = 0.01, p-value > 0.05), but significant for JSBACH data (r = 0.35, p-value < 0.05) (Supplementary Table S2).
When comparing the land cover type of grid cells, TGBs were largely present in the observations and in the models, but in JSBACH some grid cell had vegetation with predominance of evergreen trees (Figure 1).
For the observations at 0.5° resolution, G and T mainly depended on MAR, and increased with it (Figures 1A, 3A, 6A, 7A and Table 2; D’Onofrio et al., 2018). G and T also decreased with SI and log10(AFI) (Figures 6A, 7A), whereas at 1.875° resolution only the relationships for grass cover were significant (Table 2 and Figures 3C, 7C). LPJ-GUESS simulated the main relationships of increasing tree and grass cover with annual rainfall, but for modeled T this increase was steeper and explained higher deviance (R2 = 0.51) than in the observations (R2 = 0.35; Figures 1, 6 and Table 2). LPJ-GUESS also simulated the observed decrease of T with log10(AFI) and of G with log10(AFI) and SI (although with much less predictive power than in the observations), but it did not capture the observed decrease of T with rainfall seasonality, whose GLM in the observations explained a deviance of 0.24. In JSBACH the best predictor for G was log10(AFI): grass cover decreased with fire intervals, and this fit had a very large explanatory power (R2 = 0.91) (Figures 3D, 7D). Although MAR was the second factor determining JSBACH grass cover variation (Figure 7D), it explained a large deviance of G (R2 = 0.78), even larger than found in the observations (R2 = 0.49). As for the observations at 1.875°, T in JSBACH did not depend significantly on any abiotic variable (Figure 6D).
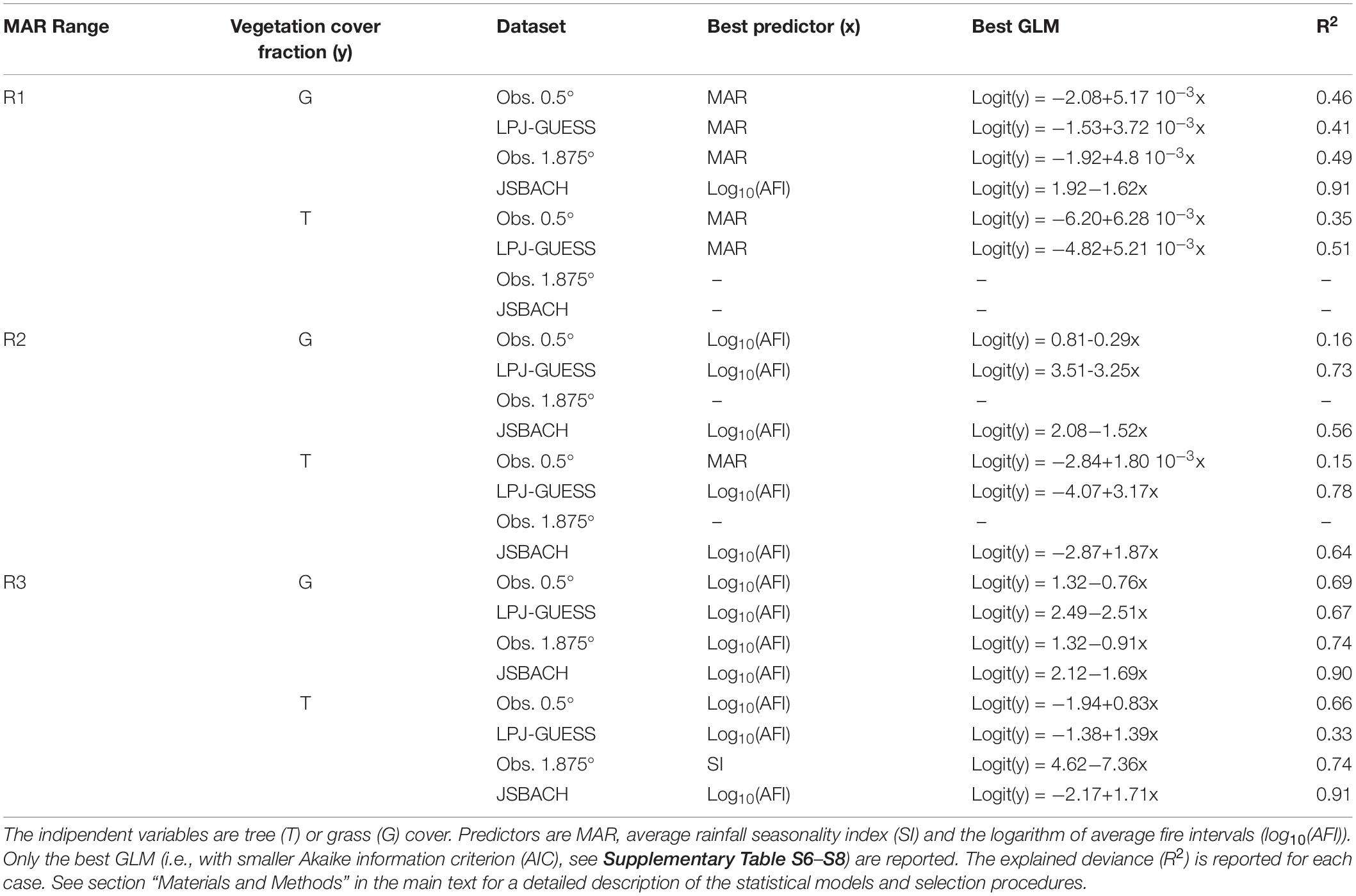
Table 2. Results of the GLM analyses in the three mean annual rainfall (MAR) ranges: low MAR (R1, MAR ≤ 630 mm year–1), intermediate MAR (R2, 630 mm year–1 < MAR < 1200 mm year–1) and high MAR (R3, MAR ≥ 1200 mm year–1) for obs. 0.5°, LPJ-GUESS, obs. 1.875° and JSBACH datasets.
Intermediate Mean Annual Rainfall
At intermediate annual rainfall (590 mm year–1 < MAR < 1200 mm year–1), in the observations TGB was the predominant vegetation type and most of the grid cells had frequent fires (see also D’Onofrio et al., 2018). In the observations at 0.5° resolution, according to the best GLMs, trees depended weakly on MAR and grasses depended weakly on fire (Table 2 and D’Onofrio et al., 2018) and there were no significant dependencies in the observations at 1.875° resolution (Figures 6, 7). In LPJ-GUESS data, fire was the most important factor for grasses and trees, explaining a large deviance (R2 = 0.73 for grass and 0.78 for trees), but high-frequency fires were underestimated and annual rainfall had stronger importance than in the observations for both trees and grasses (Figures 6A,B, 7A,B). JSBACH simulated the fire occurrence better than LPJ-GUESS, but both tree and grass cover depended too strongly on fire compared to the observations (Figures 6C,D, 7C,D).
In the observations, at both resolutions grass cover still was mostly larger than tree cover (Figures 5A–D, Supplementary Figures S1A,C). While there was quite a good agreement between vegetation cover distributions from the observations and JSBACH (Figures 5B,D, but notice the broader modeled G distribution with respect to the observations), vegetation cover distributions from LPJ-GUESS were very different from the observations at 0.5°: modeled T and G had larger spread in values, modeled T and G medians were higher and lower, respectively, and closer to each other (Figures 5A,C), and there was a larger number of grid cells with T > G (Supplementary Figures S1A,B).
In the observations, in most of the grid cells fires occurred more frequently than in the other MAR ranges (Figures 5G,H). Overall, both model datasets displayed this feature. However, in LPJ-GUESS data, the log10(AFI) distribution was shifted toward higher values of AFI, thus fires were mostly rarer than in the observations, although the range of modeled log10(AFI) distribution was narrower than in the observations (Figure 5G). SI between the models and the observations were quite comparable (Figures 5E,F).
In this MAR range, the explanatory variables from the observations displayed very small correlation (Supplementary Table S1). The correlation coefficients from the observation and LPJ-GUESS datasets disagreed (Supplementary Table S1): in LPJ-GUESS there was a positive and large correlation between log10(AFI) and MAR (r = 0.69), which had a smaller absolute value (although significant, p < 0.05) and was negative in the observations (r = −0.16). The Pearson’s r between abiotic variables from JSBACH data were smaller than in the other MAR ranges, as in the observations. However, in JSBACH log10(AFI) was negatively correlated significantly with SI (with quite a large absolute value, r = −0.41, whereas this correlation was not significant in the observations, p > 0.05; Supplementary Table S2).
Analyzing the biome types, most of the grid cells from the observational datasets were identified as TGBs (85% at 0.5° resolution and 78% at 1.875°). The TGB predominance was quite well simulated by LPJ-GUESS, with 75% of the grid cells classified as TGB, whereas in JSBACH data this percentage was lower (48%).
In the observations at 0.5°, T depended mainly on MAR and G on log10(AFI) (Table 2 and D’Onofrio et al., 2018): T increased with annual rainfall and G decreased with fire intervals, but these relationships had very low explanatory power (R2 = 0.15 for G and R2 = 0.16 for T best GLMs, Figures 6A, 7A) especially if compared with the best GLMs in the other MAR ranges. G also slightly decreased with MAR, but the fit had very low explained deviance (Figure 7A, R2 = 0.05), and the ΔAIC between this GLM and the intercept-only model was smaller than 1 (ΔAIC = 0.27, Supplementary Table S6): therefore, this dependence could be considered negligible (Burnham and Anderson, 2002). In the observations at 1.875°, G and T didn’t depend on any of the factors which we considered (Figures 6C, 7C and Table 2). The best predictor for both G and T from LPJ-GUESS was log10(AFI), followed by MAR. However, the increase of T and decrease of G with these MAR and log10(AFI), respectively, were steeper than in the observations (Figures 1A,B, 2A,B), and the explained deviances of these GLMs, especially for T, were larger (Figures 6B,7B). Differently from the observations, G and T depended significantly on SI in LPJ-GUESS (although weakly) and on log10(AFI) in JSBACH, where this was the best and only predictor (Table 2 and Figures 6D, 7D).
High Mean Annual Rainfall
At high precipitation (MAR ≥ 1200 mm year–1), in the observations both TGB and TF occurred, with dominance of TFs. The latter were characterized by higher tree cover, lower grass cover, lower rainfall seasonality and rare fires than TGBs. Considering all the grid cells in the range, tree and grass cover were highly determined by rainfall seasonality and fire intervals (Figures 6A,C, 7A,C; D’Onofrio et al., 2018). For LPJ-GUESS data, fires and rainfall seasonality seemed to have a strong impact on grass cover, but a weak impact on tree cover. However, modeled tree and grass cover had narrower spread in values than in the observations: the number of grid cells with closed TFs was overestimated and with open TGBs underestimated, while TFs and TGBs were not associated to really different AFI as in the observations (Supplementary Figure S5). JSBACH was able to simulate the presence of closed TF and open TGB with both different rainfall seasonality and fire intervals. However, compared to the observations, fire had a greater importance in determining the variation of both tree and grass cover, while rainfall seasonality had a lower predictive power (Figures 6, 7) in JSBACH; in the presence of frequent fires the values for grass cover and tree cover were generally overestimated and underestimated, respectively (Figures 2C,D and Supplementary Figures S2C,D).
Overall, in the observations tree cover dominated over grasses, although there were many grid cells with more grass cover than tree cover (Figures 5A–D and Supplementary Figures S1A,C, see also D’Onofrio et al., 2018). There was a quite good agreement between JSBACH and observed vegetation cover distributions, although maximum values of modeled grass cover were overestimated (Figure 5B). Conversely, in LPJ-GUESS tree cover was generally overestimated and grass cover underestimated, and their distributions were narrower than in the observations (Figures 5A,C and Supplementary Figure S1B).
For grid cells in this MAR range, the rainfall regime was less seasonal than in the other MAR ranges for the observations as well as for the forcing used in LPJ-GUESS and JSBACH (Figure 5E), although for the latter the seasonality index distribution was shifted toward greater values than in the observations, i.e., toward more seasonal rainfall regimes [notice that, on the contrary, when using daily metrics of seasonality, precipitation in MPI-ESM was found to underestimate seasonality of precipitation on average for all tropical areas for high precipitation (Lasslop et al., 2018)]. As in the first MAR range, fires were mostly rare, but frequent fires occurred (Figures 5G,H), except for LPJ-GUESS. The correlation coefficients between explanatory variables showed a quite good agreement between observed and model datasets, although in both JSBACH and LPJ-GUESS the absolute values of the correlation coefficients between log10(AFI) and MAR and SI and MAR are greater than in the observations (Supplementary Tables S1, S2).
In the observations, the threshold of 1200 mm year–1 represented the transition to the forest biome, and the grid cells classified as TF had typically more tree cover than grass cover, whereas TGB grid cells had the opposite features (Supplementary Figures S5A–D and D’Onofrio et al., 2018). TF grid cells were also characterized by a less seasonal rainfall regime and less frequent fires with respect to TGB grid cells (Supplementary Figures S5E–H). The limit of 1200 mm year–1 represented reasonably well the transition to TFs also for JSBACH datasets (Figure 1D), whereas for LPJ-GUESS data this threshold seemed to occur at lower annual rainfall, around 1000 mm year–1, a value in line with other analyses of tree cover from remote sensing datasets (Staver et al., 2011b). JSBACH was somewhat able to simulate the main characteristics of observed TFs and TGBs, although modeled T and G in TGB grid cells varied more (Figures S5B,D). Indeed, some modeled TGB grid cells had T larger than G (Figure 1D). Overall, LPJ-GUESS was able to simulate quite well the tree and grass cover distributions of observed TFs, but not of TGBs, which had lower grass cover and higher tree cover compared to the observations (Supplementary Figures S5A,C). This model also overestimated the percentage of TF grid cells (82% versus 61% in obs. 0.5°) and underestimated that of TGB grid cells (16% versus 23% in obs. 0.5°). Furthermore, fire intervals in modeled TGB grid cells were generally overestimated (Supplementary Figure S5G) and TF fires were less rare than in the observations.
The results of the GLM analysis were somehow expected given the analysis of the characteristics of TGB and TF grid cells: according to the best GLMs for observed vegetation cover, log10(AFI) and SI were the most important predictors (Table 2 and Supplementary Table S8). SI and log10(AFI), which were highly anticorrelated in these high rainfall grid cells (r = −0.74 for obs. 0.5° and r = −0.77 for obs. 1.875°, Supplementary Tables S1, S2), and the GLMs with these variables had similar predictive power for both observed T and G (Figures 6, 7). Specifically, G decreased with log10(AFI) (Figure 2B) and increased with SI, and the opposite dependences occurred for T (Figures 6, 7). Notice that MAR had a really small role for the observations at 0.5° and was not significant for the observations at 1.875° (Figures 6A,C, 7A,C). The same dependencies were simulated by LPJ-GUESS and JSBACH (Figures 6, 7). However, with respect to the observations, in LPJ-GUESS log10(AFI) and SI had a smaller effect on T while MAR had a greater importance for both T and G (although small) (Figures 1B, 6B, 7B and Supplementary Table S8). Conversely, in JSBACH data log10(AFI) had a stronger impact than SI in determining G and T variations. When fires were frequent, modeled G had higher values than in the observations (Figures 2, 3). Unlike the observations at 1.875°, in JSBACH data the dependencies of T and G on MAR were significant, albeit with small predictive power (Figures 6D, 7D).
Discussion
In this study, we evaluated and validated the outcomes of the DGVM LPJ-GUESS and JSBACH in sub-Saharan Africa, using the approach of an observational analysis of the climate-vegetation-fire relationships in this region (D’Onofrio et al., 2018), which includes both grass and tree cover, unlike previous similar analyses that considered only tree cover (e.g., Staver et al., 2011a,b).
Overall, both models were able to simulate the main factors determining the vegetation cover, i.e., the general decrease of grass cover with fire intervals and the general increase of tree cover and total vegetation cover with MAR, with the exception of grass cover in LPJ-GUESS that was found to mainly depend on MAR. In general, the models were able to simulate the distribution of TGB and TF along MAR. However, by analyzing the importance of the different predictors by MAR intervals, we found differences between the observations and the models, which are likely to reflect differences in the main ecological mechanisms at play in model and reality.
At low annual precipitation (MAR ≤ 590 mm year–1), where water availability is the most important factor regulating the vegetation, the eco-hydrological processes are the main mechanisms at play and LPJ-GUESS, which has a more complex representation of vegetation dynamics than JSBACH, showed the best agreement with the observations. In mesic and humid areas (MAR > 590 mm year–1), fire processes became more relevant and are important for maintaining open TGB and for regulating the transition between TGB and TF. At high precipitation (MAR ≥ 1200 mm year–1), JSBACH, which has a more complex representation of fire processes than LPJ-GUESS, was the best model in simulating the observed marked differences in vegetation cover and average fire intervals between TGB and TF.
At low annual precipitation (MAR ≤ 590 mm year–1), grasses dominated over trees, and both vegetation types increased mainly with MAR (only grasses at 1.875° resolution), indicating that their growth was mainly water limited (Scholes et al., 2002; Sankaran et al., 2005; D’Onofrio et al., 2018). In these areas, where fires are generally rare and rainfall seasonality is strong, trees and grasses compete mainly for water, and grasses can be favored because, compared to trees, their roots are closer to the surface, where most of the water is (Ward et al., 2013 and references therein); furthermore, grasses can have a strong competitive impact on tree seedlings (Baudena et al., 2010; February et al., 2013; D’Onofrio et al., 2015). In this MAR range, in general, LPJ-GUESS was able to simulate the predominance of grasses (Figures 7A,B) and the water limitation of vegetation growth (Figures 3A,B), although model data showed a clear MAR-controlled upper bound for grasses that was not as evident in the observations. This represents the optimum between growth-efficiency/death and actual water availability, which depends only on rainfall in the model. This difference between the model and the observations may be partially due to only natural grass being simulated in LPJ-GUESS. In JSBACH, grass cover, which was underestimated by this model, increased with MAR as in the observations below 590 mm year−1, but had a more important relationship with fire than in the observations, whereas tree cover was overestimated [as already reported by Baudena et al. (2015) and Lasslop et al. (2018)]. In JSBACH, trees can be replaced by natural grass only after the occurrence of a fire or of a wind throw, thanks to the faster rate of establishment of natural grass with respect to shrubs and trees (Reick et al., 2013). Trees can also have a disadvantage compared to grass due to the climatological limits to their establishment due to physiological constraints (Reick et al., 2013). However, dry savannas do not strictly depend on fire, as this disturbance can only influence the tree-grass ratio (Sankaran et al., 2005; Accatino et al., 2010). Thus, JSBACH should be revised in order to: (1) weaken the role of fire at low precipitation, for example by explicitly including other mechanisms, related to demography and eco-hydrology, that permit grasses to outcompete trees, such as tree-grass competition for soil water (see also Lasslop et al., 2018), similarly to what represented in e.g., LPJ-GUESS (see below) and/or (2) improve the limitation of tree establishment in very dry regions based on climatological limits (such as precipitation thresholds or drought indices) or related to net primary production. Nevertheless, we must note that the MODIS vegetation cover product displays limitations at low tree cover (Staver and Hansen, 2015). In order to interpret the JSBACH results, we must also consider the mechanisms of land use change: although we removed croplands from observations and JSBACH, we kept pastures in JSBACH, since rangelands are part of African savannas and grasslands (Hanotte, 2002; Hempson et al., 2017). Indeed, in this range the modeled grass cover was mainly composed of pastures (see Supplementary Figure S6), which are included as anthropogenic land-cover change (Reick et al., 2013): pastures first replace natural grasslands, and subsequently, once no natural grasslands are left, the areas covered by trees. Pastures are treated as grassland by SPITFIRE: fire reduces the carbon content of biomass and litter in pastures (according to the combustion completeness, which depends on the moisture) while the pasture cover fraction remains unchanged. Land-use change can modify the relative dominance between trees and grasses. In general, at low precipitation without land-use change (both pastoralism and agriculture), modeled tree cover would be higher than with land-use change, as shown in the paper by Lasslop et al. (2018) with a simulation performed using the land use of 1850 (with low anthropogenic vegetation cover) for the whole simulation. A negative effect of pastoralism on tree cover is in agreement with an observational analysis in sub-Saharan Africa (Aleman et al., 2016).
At intermediate annual rainfall (590 mm year–1 < MAR < 1200 mm year–1), TGBs with predominance of grasses over trees were the main biome and fires were frequent. At the spatial resolutions considered in this study, closed canopy was not observed at these precipitation values, even though it can occur at local scale (Sankaran et al., 2005), and tree cover increased with MAR (at least at 0.5° resolution) indicating that tree cover could be still water limited (Hirota et al., 2011; Staver et al., 2011b; D’Onofrio et al., 2018). Conversely, grass cover was no longer water limited and depended on fire intervals at 0.5°, although really weakly. These relationships were not significant at 1.875° resolution (Figures 6, 7). Within this range the seasonal rainfall regimes can enhance C4 grass-fuel availability in the dry season favoring the occurrence of fires (Archibald et al., 2009; Lehmann et al., 2011), which can maintain open TGBs through a positive vegetation-fire feedback (Beckage et al., 2009) in which savanna trees are also well adapted to fire (Ratnam et al., 2011). In LPJ-GUESS, tree and grass cover were very different from the observations: they had more variations, grasses didn’t dominate over trees, and both varied more steeply with mean annual rainfall (Figures 1, 3). Although fire had an important role in determining modeled tree and grass variation in this MAR range, these patterns could suggest that the grass-fire feedback is not strong enough to keep grass cover as high as in the observations for most of the grid cells. Therefore, one of the main ecological mechanisms regulating the relative modeled tree-grass presence can be related to the dynamics of soil water availability and, for example, to the different water use of the two vegetation forms. Indeed, in LPJ-GUESS grasses are shallow-rooted, with 90% of their roots in the soil layer closest to the surface (0.5 m), whereas trees have a large proportion of their roots in the lower soil layer (40%). Therefore, grasses could take advantage over trees when annual rainfall is low, and soil water can be larger in the shallow soil layer compared to the deeper one (Ward et al., 2013 and references therein), with the opposite possibly occurring at higher annual precipitation. Modeled grass cover can be positively related to fire frequency because it is itself related, in this MAR range, to lower soil-moisture in the first layer, which is the key driver of fire occurrence in GlobFIRM and, moreover, the fire resistance of grasses is higher than that of trees. Yet, our analysis suggests that fires were too rare to have a strong effect on vegetation and to maintain it in a state with more grasses than trees. A possible explanation is that in GlobFIRM the percentage of killed individuals does not consider whether the tree is small or high, which could allow too many trees to grow to a safe height and, thus, avoid the maintenance of open canopies when fire-resistant (deciduous) trees are present. Furthermore, it is important to highlight that the overestimation of modeled tree cover in this intermediate MAR range may also be related to the lack of a representation of pastures in LPJ-GUESS simulations. Pastoralism was observed to negatively correlate with tree cover in sub-Saharan Africa (Aleman et al., 2016) and a large abundance of livestock in sub-Saharan Africa is present in this MAR range (Hempson et al., 2015). However, livestock herbivores may also favor tree cover mainly through suppression of fire (Hempson et al., 2017). Conversely to LPJ-GUESS, JSBACH simulated the occurrence of frequent fires that could maintain low tree cover and high grass cover in most of the grid cells, probably thanks to the modeled positive grass-fire feedback, although fire frequency was a little underestimated in the model with respect to the observations. However, we must note that in JSBACH, in this intermediate MAR range, grass cover, average fire intervals and especially tree cover would show a higher variability if the filtering of the data based on ESA-CCI LC map had not been applied (we used this filtering to have a number of grid cells and locations comparable to the observations); still, this did not qualitatively change the main patterns and conclusions (Supplementary Figure S8). Note that in the observations at 1.875° resolution we can only associate high grass cover with high fire frequency (Figure 5), but we did not find any significant relationship of vegetation cover with precipitation or fire explanatory variables.
The low explanatory power of the GLMs at 0.5°, which became not significant at 1.875°, suggests that the ecological processes shaping the vegetation, such as the vegetation-fire feedback, may operate at a finer scale, as discussed by Pausas and de Dantas (2017). The issue of scale and upscaling in ecology is not resolved (e.g., Staver, 2018) and, thus, may have led to a mismatch between the ecological scale of the fire processes and the spatial resolution of both models, especially in JSBACH. Furthermore, the weak or not significant relationships might also indicate that there are other discarded factors explaining the tree and grass cover variability, such as intra-seasonal rainfall variability (Good and Caylor, 2011; Xu et al., 2018; D’Onofrio et al., 2019) related also to soil texture (Case and Staver, 2018) or herbivores (both livestock and wildlife), which are common in Africa and can have an effect on vegetation comparable to fire and can themselves negatively affect fire occurrence (Hempson et al., 2017).
At high precipitation (MAR ≥ 1200 mm year–1), both forests and savannas occur in the observations and both rainfall seasonality and fires play an important role in determining tree and grass cover (Table 2 and Supplementary Table S8) and, thus, the transition between these two biomes (D’Onofrio et al., 2018). Furthermore, many studies indicate that TGB can occur under similar climatic conditions as TF thanks to the vegetation-fire feedback (Hirota et al., 2011; Staver et al., 2011b; D’Onofrio et al., 2018), which avoids forest formation because forest trees are fire-intolerant (Beckage et al., 2009; Ratnam et al., 2011; Gignoux et al., 2016). By identifying forest and savanna states using the grass and tree PFTs (D’Onofrio et al., 2018), we found that LPJ-GUESS was able to simulate the presence of both TF and TGB biomes at high precipitation, but they were characterized by less marked differences in the distributions of fire frequency, tree cover and grass cover than in the observations (Supplementary Figure S5). Indeed, in this MAR range, in LPJ-GUESS fire frequency was underestimated in TGBs and overestimated in TFs, and, analogously to what we found in the intermediate MAR range, the occurrence of grid cells with open TGBs was underestimated, and vice versa for closed TF. However, we must note that, although in tropical rainforests fires indeed have very low frequency (Cochrane, 2003), satellite products are often not able to detect them, because forest fires in the tropics are usually understory fires and are covered by the canopy (Morton et al., 2011). For tree cover, rainfall seasonality and fire had a low explanatory power, probably due to the much lower variation compared to the observations. Modeled grass cover depended mainly on fire as in the observations, but the fact that there are only few grid cells with high grass cover suggests again that the grass-fire feedback is not strong enough. Indeed, fire is the main factor maintaining open TGBs in humid areas (Bond et al., 2005), whose occurrence is enhanced by rainfall seasonality (Archibald et al., 2009). Thus, although part of the disagreement between the model and the observations may also be related to the pastures not being simulated in LPJ-GUESS, our analysis suggests that changing the fire model in LPJ-GUESS is crucial.
Glob-FIRM is a first-generation fire model, developed before the availability of global-scale satellite fire information data (Hantson et al., 2016), it is based on empirical schemes and it is only driven by soil moisture and vegetation characteristics. Despite its simplicity, Glob-FIRM was able to simulate the positive relationship between grass cover and average fire frequency (in the second and third MAR ranges), but it presented a narrower distribution of the fire variable, with values of average fire intervals never smaller than 3 years and few times greater than 1000 years (Figure 2B).
In contrast, JSBACH simulated the presence of closed TF and open TGB linked to both different rainfall seasonality and fire intervals. In contrast to Glob-FIRM, SPITFIRE includes the main mechanisms and factors permitting the savanna-forest transition in humid areas (Lasslop et al., 2018): as shown by sensitivity simulations with JSBACH-SPITFIRE, the fuel amount and properties are key factors for obtaining the contrast of fire regimes between forests and grasslands in Africa (Figure 8 in Lasslop et al., 2014). However, in the third MAR range, fire had a greater importance and effect than rainfall seasonality in determining the variation of both tree and grass cover in JSBACH compared to the observations. This was reflected in a general overestimation of grass cover and underestimation of tree cover in presence of frequent fires (Lasslop et al., 2018). Thus, in JSBACH, the grass-fire feedback was seemingly stronger than necessary, and it would be even stronger without land use change (Lasslop et al., 2018). We showed that grid cells with higher grass cover, that corresponded to lower average fire intervals, were characterized by lower pasture cover, i.e., the grass cover was mainly composed of natural grass (Supplementary Figure S6). In JSBACH, pastures have a higher fuel bulk density, which reduces the burned area. This is supported by analyses of global datasets that show that pastoralism negatively affects fire occurrence in savannas and grasslands (Andela et al., 2017). As already put forward by Lasslop et al. (2018), many possible solutions for improving the fire-vegetation interactions in JSBACH are possible, such as the amelioration of the fire-tolerance/intolerance of savanna/forest trees, related to the bark thickness, which could decrease the advantage of grass in the presence of fires.
The analysis of sub-Saharan Africa permitted us to identify areas of TGB as grid cells dominated by C4 grass and deciduous trees, and areas of TF as grid cells dominated by evergreen trees, which is a reliable assumption at the spatial resolution which we considered (D’Onofrio et al., 2018). Both models simulated the observed pattern of these biomes in relation to MAR (Figure 1), with TGBs occurring along the entire MAR gradient and TFs appearing above 1000 mm year–1 (Staver et al., 2011b). However, in JSBACH some grid cells with very low precipitation (below ca. 450 mm year–1) had a vegetation cover composed mainly of evergreen trees (with tree cover lower than 25%). In general, it is well-established that evergreen species dominate the humid tropical forest with high rainfall and low seasonality (Walter, 1973; Bowman and Prior, 2005; Murphy and Bowman, 2012). In Africa TGBs are broadly characterized by deciduous trees and evergreen species can occur locally (Scholes et al., 2002), thus, they are not expected to predominate at the JSBACH grid-cell scale. This suggests that in JSBACH the physiological constraints of the evergreen tree PFT, probably related to water stress, should be revised and improved. However, in other continents this may be different than in Africa, as savanna trees may also be evergreen (Scholes and Archer, 1997; Bowman and Prior, 2005). Savanna trees are also typically fire tolerant and shade-intolerant, while forest trees have the opposite characteristics (Ratnam et al., 2011). The tropical raingreen trees included in LPJ-GUESS have these characteristics. However, looking at the predominant type of tropical tree in TGB tree cover (Supplementary Figure S7), we observed that many TGB grid cells in the low and intermediate MAR ranges, where rainfall is seasonal, have more evergreen than deciduous tree cover. Most of these grid cells have very low tree cover, so this mismatch could be also related to variability in the model. This mismatch occurred also in JSBACH (Supplementary Figure S7) and this suggests that also in LPJ-GUESS the characteristics of evergreen trees related to water stress should be revised for the African continent.
Conclusion
The analysis of the two state-of-the-art DGVMs, one including a complex vegetation description but with a simple fire model (LPJ-GUESS), and one with the opposite complexity characteristics (JSBACH), highlighted that a detailed description of either vegetation or fire processes alone is not sufficient to properly simulate the sub-Saharan African vegetation, and that an accurate description of both processes is necessary. Furthermore, our analysis suggests that the importance of the processes depended, as expected, on the MAR level, but also, more interestingly, on the scale, indicating that an increase of resolution, especially for JSBACH, might lead to a better representation of the vegetation-fire feedback.
By identifying the crucial role of vegetation-fire processes and their potential for improving the accuracy of numerical models, our results may provide also added value to inform economists, policy practitioners or decision makers:
Since both LPJ-GUESS and JSBACH are included in Earth System Models, our analysis permits to suggest possible improvements in DGVMs and, consequently, in ESMs for future projections. This is of utmost importance for future land use management under climate change, since DGVMs are already used as support for policy making (e.g., Lee et al., 2015; Daioglou et al., 2017; Sonntag et al., 2018). Finally, several studies propose afforestation in savannas in Africa (Sonntag et al., 2018; Bastin et al., 2019, compare also with Griffith et al., 2017) as a measure to increase carbon stock to remediate anthropogenic carbon emissions. As we showed here, the distribution of vegetation is strongly connected to fire, and thus we maintain that such plans crucially should represent the effects of fires (Bond et al., 2019). The complex dynamics connecting vegetation and fires should be thoroughly evaluated before afforestation is recommended.
Data Availability Statement
The datasets generated for this study are available upon request to the corresponding author.
Author Contributions
DD’O, MB, and JH conceived the original idea and the analyses. DD’O performed the analyses and wrote the first draft of the manuscript. GL provided the JSBACH simulation. LN provided the LPJ-GUESS simulation. JH, MB, GL, LN, and DW contributed to refine the study, to interpret the results, and to further develop the manuscript. All authors contributed to the article and approved the submitted version.
Funding
DD’O and JH acknowledge funding by the European Union Horizon 2020 Research and Innovation Programme under grant agreement no. 641816 (CRESCENDO). GL was funded by the Deutsche Forschungsgemeinschaft (DFG, German Research Foundation) – 338130981.
Conflict of Interest
The authors declare that the research was conducted in the absence of any commercial or financial relationships that could be construed as a potential conflict of interest.
Acknowledgments
LPJ-GUESS-simulations have been performed with the support of the Strategic Research Area MERGE. We thank Victor Brovkin for valuable discussions on this study.
Supplementary Material
The Supplementary Material for this article can be found online at: https://www.frontiersin.org/articles/10.3389/fenvs.2020.00136/full#supplementary-material
References
Accatino, F., De Michele, C., Vezzoli, R., Donzelli, D., and Scholes, R. J. (2010). Tree-grass co-existence in savanna: interactions of rain and fire. J. Theor. Biol. 267, 235–242. doi: 10.1016/j.jtbi.2010.08.012
Akaike, H. (1974). A new look at the statistical model identification. IEEE Trans. Automatic Control 19, 716–723. doi: 10.1109/TAC.1974.1100705
Aleman, J. C., Blarquez, O., and Staver, C. A. (2016). Land-use change outweighs projected effects of changing rainfall on tree cover in Sub-Saharan Africa. Glob. Change Biol. 22, 3013–3025. doi: 10.1111/gcb.13299
Aleman, J. C., Jarzyna, M. A., and Staver, A. C. (2018). Forest extent and deforestation in tropical Africa since 1900. Nat. Ecol. Evol. 2, 26–33. doi: 10.1038/s41559-017-0406-1
Andela, N., Morton, D. C., Giglio, L., Chen, Y., van der Werf, G. R., Kasibhatla, P. S., et al. (2017). A human-driven decline in global burned area. Science 356, 1356L–1362.
Archibald, S., Roy, D. P., van Wilgen, B. W., and Scholes, R. J. (2009). What limits fire? an examination of drivers of burnt area in Southern Africa. Glob. Change Biol. 15, 613–630. doi: 10.1111/j.1365-2486.2008.01754.x
Bastin, J.-F., Finegold, Y., Garcia, C., Mollicone, D., Rezende, M., and Routh, D. (2019). The global tree restoration potential. Science 365, 76–79. doi: 10.1126/science.aax0848
Baudena, M., D’Andrea, F., and Provenzale, A. (2010). An idealized model for tree–grass coexistence in savannas: the role of life stage structure and fire disturbances. J. Ecol. 98, 74–80. doi: 10.1111/j.1365-2745.2009.01588.x
Baudena, M., Dekker, S. C., van Bodegom, P. M., Cuesta, B., Higgins, S. I., Lehsten, V., et al. (2015). Forests, savannas, and grasslands: bridging the knowledge gap between ecology and dynamic global vegetation models. Biogeosciences 12, 1833–1848. doi: 10.5194/bg-12-1833-2015
Beckage, B., Platt, W. J., and Gross, L. J. (2009). Vegetation, fire, and feedbacks: a disturbance-mediated model of savannas. Am. Naturalist 174, 805–818. doi: 10.1086/648458
Bonan, G. B. (2008). Forests and climate change: forcings, feedbacks, and the climate benefits of forests. Science 320, 1444–1449. doi: 10.1126/science.1155121
Bonan, G. B., and Doney, S. C. (2018). Climate, ecosystems, and planetary futures: the challenge to predict life in earth system models. Science 359:eaam8328. doi: 10.1126/science.aam8328
Bond, W., Stevens, N., Midgley, G., and Lehmann, C. (2019). The trouble with trees: afforestation plans for Africa. Trends Ecol. Evol. 34, 963–965. doi: 10.1016/j.tree.2019.08.003
Bond, W. J. (2008). What limits trees in C 4 grasslands and savannas? Annu. Rev. Ecol. Evol. Syst. 39, 641–659. doi: 10.1146/annurev.ecolsys.39.110707.173411
Bond, W. J., Woodward, F. I., and Midgley, G. F. (2005). The global distribution of ecosystems in a world without fire. New Phytol. 165, 525–538. doi: 10.1111/j.1469-8137.2004.01252.x
Bowman, D. M. J. S., Balch, J. K., Artaxo, P., Bond, W. J., Carlson, J. M., Cochrane, M. A., et al. (2009). Fire in the earth system. Science 324, 481–484. doi: 10.1126/science.1163886
Bowman, D. M. J. S., and Prior, L. D. (2005). Why do evergreen trees dominate the australian seasonal tropics? Aust. J. Bot. 53, 379–399. doi: 10.1071/BT05022
Brovkin, V., Raddatz, T., Reick, C. H., Claussen, M., and Gayler, V. (2009). Global biogeophysical interactions between forest and climate. Geophys. Res. Lett. 36, 1–5. doi: 10.1029/2009GL037543
Bucini, G., and Hanan, N. P. (2007). A continental-scale analysis of tree cover in African savannas. Glob. Ecol. Biogeogr. 16, 593–605. doi: 10.1111/j.1466-8238.2007.00325.x
Buitenwerf, R., Bond, W. J., Stevens, N., and Trollope, W. S. W. (2012). Increased tree densities in South African Savannas: 50 years of data suggests CO2 as a driver. Glob. Change Biol. 18, 675–684. doi: 10.1111/j.1365-2486.2011.02561.x
Burnham, K. P., and Anderson, D. R. (2002). Model Selection and Multimodel Inference: A Practical Information-Theoretic Approach (2nd Ed). Ecological Modelling, Vol. 172. Berlin: Springer. doi: 10.1016/j.ecolmodel.2003.11.004
Case, M. F., and Staver, A. C. (2018). Soil texture mediates tree responses to rainfall intensity in African savannas. New Phytol. 219, 1363–1372. doi: 10.1111/nph.15254
Daioglou, V., Doelman, J. C., Stehfest, E., Müller, C., Wicke, B., Faaij, A., et al. (2017). Greenhouse gas emission curves for advanced biofuel supply chains. Nat. Clim. Change 7, 920–924. doi: 10.1038/s41558-017-0006-8
Dobson, A. J. (2002). An Introduction to Generalized Linear Models / Annette J. Dobson, 2nd Edn. London: Chapman & Hall.
D’Onofrio, D., Baudena, M., D’Andrea, F., Rietkerk, M., and Provenzale, A. (2015). Tree-grass competition for soil water in arid and semiarid savannas: the role of rainfall intermittency. Water Resour. Res. 51, 169–181. doi: 10.1002/2014wr015515
D’Onofrio, D., Sweeney, L., von Hardenberg, J., and Baudena, M. (2019). Grass and tree cover responses to intra-seasonal rainfall variability vary along a rainfall gradient in African tropical grassy biomes. Sci. Rep. 9:2334. doi: 10.1038/s41598-019-38933-9
D’Onofrio, D., von Hardenberg, J., and Baudena, M. (2018). Not only trees: grasses determine African tropical biome distributions via water limitation and fire. Global Ecol. Biogeogr. 27, 714–725. doi: 10.1111/geb.12735
February, E. C., Higgins, S. I., Bond, W. J., and Swemmer, L. (2013). Influence of competition and rainfall manipulation on the growth responses of savanna trees and grasses. Ecology 94, 1155–1164. doi: 10.1890/12-0540.1
Fisher, R., McDowell, N., Purves, D., Moorcroft, P., Sitch, S., Cox, P., et al. (2010). Assessing uncertainties in a second-generation dynamic vegetation model caused by ecological scale limitations. New Phytologist 187, 666–681. doi: 10.1111/j.1469-8137.2010.03340.x
Gignoux, J., Konaté, S., Lahoreau, G., Le Roux, X., and Simioni, G. (2016). Allocation strategies of savanna and forest tree seedlings in response to fire and shading: outcomes of a field experiment. Sci. Rep. 6:38838. doi: 10.1038/srep38838
Gonzalez, P., Neilson, R. P., Lenihan, J. M., and Drapek, R. J. (2010). Global patterns in the vulnerability of ecosystems to vegetation shifts due to climate change. Glob. Ecol. Biogeogr. 19, 755–768. doi: 10.1111/j.1466-8238.2010.00558.x
Good, S. P., and Caylor, K. K. (2011). Climatological determinants of woody cover in Africa. Proc. Natl. Acad. Sci. U.S.A. 108, 4902–4907. doi: 10.1073/pnas.1013100108
Grace, J., José, J. S., Meir, P., Miranda, H. S., and Montes, R. A. (2006). Productivity and carbon fluxes of tropical savannas. J. Biogeogr. 33, 387–400. doi: 10.1111/j.1365-2699.2005.01448.x
Griffith, D. M., Lehmann, C. E. R., Strömberg, C. A. E., Parr, C. L., Pennington, R. T., Sankaran, M., et al. (2017). Comment on ‘The Extent of Forest in Dryland Biomes.’ Science 358:eaao1309. doi: 10.1126/science.aao1309
Guisan, A., and Zimmermann, N. E. (2000). Predictive habitat distribution models in ecology. Ecol. Model. 135, 147–186. doi: 10.1016/S0304-3800(00)00354-9
Hanotte, O. (2002). African pastoralism: genetic imprints of origins and migrations. Science 296, 336–339. doi: 10.1126/science.1069878
Hansen, M. C., DeFries, R. S., Townshend, J. R. G., Carroll, M., Dimiceli, C., and Sohlberg, R. A. (2003). Global percent tree cover at a spatial resolution of 500 meters: first results of the MODIS vegetation continuous fields algorithm. Earth Interactions 7, 1–15. doi: 10.1175/1087-3562(2003)007<0001:GPTCAA>2.0.CO;2
Hantson, S., Arneth, A., Harrison, S. P., Kelley, D. I., Prentice, C., Rabin, S. S., et al. (2016). The status and challenge of global fire modelling. Biogeosciences 13, 3359–3375. doi: 10.5194/bg-13-3359-2016
Hazeleger, W., Severijns, C., Semmler, T., Ştefanescu, S., Yang, S., Wang, X., et al. (2010). EC-earth: a seamless earth-system prediction approach in action. Bull. Am. Meteorol. Soc. 91, 1357–1363. doi: 10.1175/2010BAMS2877.1
Hazeleger, W., Wang, X., Severijns, C., Ştefanescu, S., Bintanja, R., Sterl, A., et al. (2012). EC-earth V2.2: description and validation of a new seamless earth system prediction model. Climate Dyn. 39, 2611–2629. doi: 10.1007/s00382-011-1228-5
Hempson, G. P., Archibald, S., and Bond, W. J. (2015). A continent-wide assessment of the form and intensity of large mammal Herbivory in Africa. Science 350, 1056–1061. doi: 10.1126/science.aac7978
Hempson, G. P., Archibald, S., and Bond, W. J. (2017). The consequences of replacing wildlife with livestock in Africa. Sci. Rep. 7, 1–10. doi: 10.1038/s41598-017-17348-4
Higgins, S. I., Bond, W. J., February, E. C., Bronn, A., Euston-Brown, D. I. W., Enslin, B., et al. (2007). Effects of four decades of fire manipulation on woody vegetation structure in savanna. Ecology 88, 1119–1125. doi: 10.1890/06-1664
Hirota, M., Holmgren, M., Van Nes, E. H., and Scheffer, M. (2011). Global resilience of tropical forest and savanna to critical transitions. Science 334, 232–235. doi: 10.1126/science.1210657
Hubau, W., Lewis, S., Phillips, O. L., Affum-Baffoe, K., Beeckman, H., cuni sanchez, A., et al. (2020). Asynchronous carbon sink saturation in african and amazonian tropical forests. Nature 579, 80–87. doi: 10.1038/s41586-020-2035-0
Hurtt, G. C., Chini, L. P., Frolking, S., Betts, R. A., Feddema, J., Fischer, G., et al. (2011). Harmonization of land-use scenarios for the period 1500-2100: 600 years of global gridded annual land-use transitions, wood harvest, and resulting secondary lands. Clim. Change 109, 117–161. doi: 10.1007/s10584-011-0153-2
IPCC (2013). “Climate change 2013: the physical science basis,” in Contribution of Working Group I to the Fifth Assessment Report of the Intergovernmental Panel on Climate Change (Cambridge, United Kindom and New York: Cambridge University Press).
Johnson, E. A., and Van Wagner, C. E. (1985). The theory and use of two fire history models. Can. J. Forest Res. 15, 214–220. doi: 10.1139/x85-039
Kloster, S., and Lasslop, G. (2017). Historical and future fire occurrence (1850 to 2100) simulated in CMIP5 earth system models. Glob. Planet. Change 150, 58–69. doi: 10.1016/j.gloplacha.2016.12.017
Lasslop, G., Brovkin, V., Reick, C. H., Bathiany, S., and Kloster, S. (2016). Multiple stable states of tree cover in a global land surface model due to fire-vegetation feedback. Geophys. Res. Lett. 43, 6324–6331. doi: 10.1002/2016GL069365
Lasslop, G., Coppola, A. I., Voulgarakis, A., Yue, C., and Veraverbeke, S. (2019). Influence of fire on the carbon cycle and climate. Curr. Clim. Change Rep. 5, 112–123. doi: 10.1007/s40641-019-00128-9
Lasslop, G., Moeller, T., D’Onofrio, D., Hantson, S., and Kloster, S. (2018). Tropical climate–vegetation–fire relationships: multivariate evaluation of the land surface model JSBACH. Biogeosciences 15, 5969–5989. doi: 10.5194/bg-15-5969-2018
Lasslop, G., Thonicke, K., and Kloster, S. (2014). SPITFIRE within the MPI earth system model: model development and evaluation. J. Adv. Model. Earth Syst. 6, 740–755. doi: 10.1002/2013MS000284
Lee, C., Schlemme, C., Murray, J., and Unsworth, R. (2015). The cost of climate change: ecosystem services and wildland fires. Ecol. Econ. 116, 261–269. doi: 10.1016/j.ecolecon.2015.04.020
Lehmann, C. E. R., Anderson, T. M., Sankaran, M., Higgins, S. I., Archibald, S., Hoffmann, W. A., et al. (2014). Savanna vegetation-fire-climate relationships differ among continents. Science 343, 548–553. doi: 10.1126/science.1247355
Lehmann, C. E. R., Archibald, S. A., Hoffmann, W. A., and Bond, W. J. (2011). Deciphering the distribution of the savanna biome. New Phytologist 191, 197–209. doi: 10.1111/j.1469-8137.2011.03689.x
Lehsten, V., Harmand, P., Palumbo, I., and Arneth, A. (2010). Modelling burned area in Africa. Biogeosciences 7, 3199–3214. doi: 10.5194/bg-7-3199-2010
Malhi, Y., Adu-bredu, S., Asare, R. A., Lewis, S. L., and Mayaux, P. (2013). African rainforests: past, present and future. Philos. Trans. R. Soc. B 368:20120312. doi: 10.1098/rstb.2012.0312
Mauritsen, T., Bader, J., Becker, T., Behrens, J., Bittner, M., Brokopf, R., et al. (2019). Developments in the MPI−M earth system model version 1.2 (MPI−ESM1.2) and its response to increasing CO 2. J. Adv. Model. Earth Syst. 11, 998–1038. doi: 10.1029/2018MS001400
McCullagh, P., and Nelder, J. A. (1989). Generalized Linear Models, Second Edition. Abingdon: Taylor & Francis.
Midgley, G. F., and Bond, W. J. (2015). Future of African terrestrial biodiversity and ecosystems under anthropogenic climate change. Nat. Clim. Change 5, 823–829. doi: 10.1038/nclimate2753
Mitchard, E. T. A., and Flintrop, C. M. (2013). Woody encroachment and forest degradation in Sub-Saharan Africa’s woodlands and savannas 1982 – 2006. Philos. Trans. R. Soc. Lond. B Biol. Sci. 368:20120406. doi: 10.1098/rstb.2012.0406
Morton, D. C., DeFries, R. S., Nagol, J., Souza, C. M., Kasischke, E. S., Hurtt, G. C., et al. (2011). Mapping canopy damage from understory fires in amazon forests using annual time series of landsat and MODIS data. Remote Sensing Environ. 115, 1706–1720. doi: 10.1016/j.rse.2011.03.002
Murphy, B. P., and Bowman, D. M. J. S. (2012). What controls the distribution of tropical forest and savanna? Ecol. Lett. 15, 748–758. doi: 10.1111/j.1461-0248.2012.01771.x
Parr, C. L., Lehmann, C. E. R., Bond, W. J., Hoffmann, W. A., and Andersen, A. N. (2014). Tropical grassy biomes: misunderstood. Neglected, and under Threat. Trends Ecol. Evol. 29, 205–213. doi: 10.1016/j.tree.2014.02.004
Pausas, J. G., and de Dantas, V. (2017). Scale matters: fire-vegetation feedbacks are needed to explain tropical tree cover at the local scale. Glob. Ecol. Biogeogr. 26, 395–399. doi: 10.1111/geb.12562
Prentice, I. C., Bondeau, A., Cramer, W., Harrison, S. P., Hickler, T., Lucht, W., et al. (2007). “Dynamic global vegetation modeling: quantifying terrestrial ecosystem responses to large-scale environmental change,” in Terrestrial Ecosystems in a Changing World, eds J. G. Canadell, D. E. Pataki, and L. F. Pitelka (Berlin: Springer Berlin Heidelberg), 175–192. doi: 10.1007/978-3-540-32730-1_15
Rabin, S. S., Melton, J. R., Lasslop, G., Bachelet, D., Forrest, M., and Hantson, S. (2017). The fire modeling intercomparison project (FireMIP), phase 1 : experimental and analytical protocols with detailed model descriptions. Geosci. Model Dev. 10, 1175–1197. doi: 10.5194/gmd-10-1175-2017
Raddatz, T. J., Reick, C. H., Knorr, W., Kattge, J., Roeckner, E., Schnur, R., et al. (2007). Will the tropical land biosphere dominate the climate-carbon cycle feedback during the twenty-first century? Clim. Dyn. 29, 565–574. doi: 10.1007/s00382-007-0247-8
Ratnam, J., Bond, W. J., Fensham, R. J., Hoffmann, W. A., Archibald, S., Lehmann, C. E. R., et al. (2011). When Is a ‘forest’ a savanna, and why does it matter? Glob. Ecol. Biogeogr. 20, 653–660. doi: 10.1111/j.1466-8238.2010.00634.x
Reick, C. H., Raddatz, T., Brovkin, V., and Gayler, V. (2013). Representation of natural and anthropogenic land cover change in MPI-ESM. J. Adv. Model. Earth Syst. 5, 459–482. doi: 10.1002/jame.20022
Roy, D., Lewis, P., and Justice, C. (2002). Burned area mapping using multitemporal\rmoderate spatial resolution data-a bi-directional reflectance\rmodel-based expectation approach. Remote Sensing Environ. 83, 263–286. doi: 10.1016/s0034-4257(02)00077-9
Roy, D. P., Boschetti, L., Justice, C. O., and Ju, J. (2008). The collection 5 MODIS burned area product – global evaluation by comparison with the MODIS active fire product. Remote Sensing Environ. 112, 3690–3707. doi: 10.1016/j.rse.2008.05.013
Roy, D. P., Jin, Y., Lewis, P. E., and Justice, C. O. (2005). Prototyping a global algorithm for systematic fire-affected area mapping using MODIS time series data. Remote Sensing Environ. 97, 137–162. doi: 10.1016/j.rse.2005.04.007
Sankaran, M., Hanan, N. P., Scholes, R. J., Ratnam, J., Augustine, D. J., Cade, B. S., et al. (2005). Determinants of woody cover in African savannas. Nature 438, 846–849.
Scholes, R. J., and Archer, S. R. (1997). Tree-grass interactions in savannas. Annu. Rev. Ecol. Syst. 28, 517–544. doi: 10.1146/annurev.ecolsys.28.1.517
Scholes, R. J., Dowty, P. R., Caylor, K., Parsons, D. A. B., Frost, P. G. H., and Shugart, H. H. (2002). Trends in savanna structure and composition along an aridity gradient in the Kalahari. J. Veg. Sci. 13, 419–428. doi: 10.1111/j.1654-1103.2002.tb02066.x
Schwarz, M., and Zimmermann, N. E. (2005). A new GLM-based method for mapping tree cover continuous fields using regional MODIS reflectance data. Remote Sensing Environ. 95, 428–443. doi: 10.1016/j.rse.2004.12.010
Shanahan, T. M., Hughen, K. A., McKay, N. P., Overpeck, J. T., Scholz, C. A., Gosling, W. D., et al. (2016). CO2and fire influence tropical ecosystem stability in response to climate change. Sci. Rep. 6:29587. doi: 10.1038/srep29587
Smith, B., Prentice, I. C., and Sykes, M. T. (2001). Representation of vegetation dynamics in modelling of terrestrial ecosystems: comparing two contrasting approaches within European climate space. Global Ecol. Biogeogr. 10, 621–637. doi: 10.1046/j.1466-822X.2001.t01-1-00256.x
Sonntag, S., González, M. F., Ilyina, T., Kracher, D., Nabel, J. E. M. S., Niemeier, U., et al. (2018). Quantifying and comparing effects of climate engineering methods on the earth system. Earth’s Future 6, 149–168. doi: 10.1002/2017EF000620
Staver, A. C. (2018). Prediction and scale in savanna ecosystems. New Phytologist 219, 52–57. doi: 10.1111/nph.14829
Staver, A. C., Archibald, S., and Levin, S. (2011a). Tree cover in sub-saharan Africa: rainfall and fire constrain forest and savanna as alternative stable states. Ecology 92, 1063–1072. doi: 10.1890/10-1684.1
Staver, A. C., Archibald, S., and Levin, S. A. (2011b). The global extent and determinants of savanna and forest as alternative biome states. Science 334, 230–232. doi: 10.1126/science.1210465
Staver, A. C., and Hansen, M. C. (2015). Analysis of stable states in global savannas: is the CART pulling the horse? – a comment. Glob. Ecol. Biogeogr. 24, 985–987. doi: 10.1111/geb.12285
Staver, A. C., and Levin, S. A. (2012). Integrating theoretical climate and fire effects on savanna and forest systems. Am. Naturalist 180, 211–224. doi: 10.1086/666648
Swann, A. L. S., Laguë, M. M., Garcia, E. S., Field, J. P., Breshears, D. D., Moore, D. J. P., et al. (2018). Continental-scale consequences of tree die-offs in North America: identifying where forest loss matters most. Environ. Res. Lett. 13:055014. doi: 10.1088/1748-9326/aaba0f
Thonicke, K., Spessa, A., Prentice, I. C., Harrison, S. P., Dong, L., and Carmona-Moreno, C. (2010). The influence of vegetation, fire spread and fire behaviour on biomass burning and trace gas emissions: results from a process-based model. Biogeosciences 7, 1991–2011. doi: 10.5194/bg-7-1991-2010
Thonicke, K., Venevsky, S., Sitch, S., and Cramer, W. (2001). The role of fire disturbance for global vegetation dynamics: coupling fire into a Dynamic Global Vegetation Model. Global Ecol. Biogeogr. 10, 661–677. doi: 10.1046/j.1466-822X.2001.00175.x
Townshend, J. R., Carroll, M., DiMiceli, C., Sohlberg, R., Hansen, M., and DeFries, R. (2011). Vegetation Continuous Fields MOD44B, 2000-2010 Percent Tree Cover, Percent Non-Tree Vegetation, Collection 5, Version 051, University of Maryland, College Park, Maryland. Available online at: Https://Earthdata.Nasa.Gov/ (accessed December 8, 2016).
Veira, A., Lasslop, G., and Kloster, S. (2016). Wildfires in a warmer climate: emission fluxes, emission heights, and black carbon concentrations in 2090-2099. J. Geophys. Res. Atmos. 121, 3195–3223. doi: 10.1002/2015JD024142
Voulgarakis, A., and Field, R. D. (2015). Fire influences on atmospheric composition, air quality and climate. Curr. Pollut. Rep. 1, 70–81. doi: 10.1007/s40726-015-0007-z
Walsh, R. P. D., and Lawler, D. M. (1981). RAINFALL SEASONALITY: DESCRIPTION, SPATIAL PATTERNS AND CHANGE THROUGH TIME. Weather 36, 201–208. doi: 10.1002/j.1477-8696.1981.tb05400.x
Walter, H. K. (1973). Vegetation of the Earth in Relation to Climate and the Eco-Physiological Conditions. London: English Universities Press.
Ward, D., Wiegand, K., and Getzin, S. (2013). Walter’s two-layer hypothesis revisited: back to the roots! Oecologia 172, 617–630. doi: 10.1007/s00442-012-2538-y
Ward, D. S., Kloster, S., Mahowald, N. M., Rogers, B. M., Randerson, J. T., and Hess, P. G. (2012). The changing radiative forcing of fires: global model estimates for past, present and future. Atmos. Chem. Physics 12, 10857–10886. doi: 10.5194/acp-12-10857-2012
Wei, Y., Liu, S., Huntzinger, D. N., Michalak, A. M., Viovy, N., Post, W. M., et al. (2014). The North American carbon program multi-scale synthesis and terrestrial model intercomparison project – Part 2: environmental driver data. Geosci. Model Dev. 7, 2875–2893. doi: 10.5194/gmd-7-2875-2014
Keywords: dynamic global vegetation models, sub-Saharan Africa, tree and grass cover, fire, precipitation, tropical forest, savanna, tropical grassy biomes
Citation: D’Onofrio D, Baudena M, Lasslop G, Nieradzik LP, Wårlind D and von Hardenberg J (2020) Linking Vegetation-Climate-Fire Relationships in Sub-Saharan Africa to Key Ecological Processes in Two Dynamic Global Vegetation Models. Front. Environ. Sci. 8:136. doi: 10.3389/fenvs.2020.00136
Received: 02 August 2019; Accepted: 22 July 2020;
Published: 14 August 2020.
Edited by:
Dagmar Haase, Humboldt University of Berlin, GermanyReviewed by:
Elzbieta Antczak, University of Łódź, PolandHeath Beckett, Stellenbosch University, South Africa
Copyright © 2020 D’Onofrio, Baudena, Lasslop, Nieradzik, Wårlind and von Hardenberg. This is an open-access article distributed under the terms of the Creative Commons Attribution License (CC BY). The use, distribution or reproduction in other forums is permitted, provided the original author(s) and the copyright owner(s) are credited and that the original publication in this journal is cited, in accordance with accepted academic practice. No use, distribution or reproduction is permitted which does not comply with these terms.
*Correspondence: Jost von Hardenberg, am9zdC5oYXJkZW5iZXJnQHBvbGl0by5pdA==