- 1Oxford Martin Programme on the Future of Food, University of Oxford, Oxford, United Kingdom
- 2School of Geography and the Environment, Environmental Change Institute, University of Oxford, Oxford, United Kingdom
- 3Ecosystem Services and Management Program, International Institute for Applied Systems Analysis, Laxenburg, Austria
Growing competition for land, water and energy call for global strategies ensuring affordable food production at minimum environmental impacts. Economic modelling studies suggest trade-off relationships between environmental sustainability and food prices. However, evidence based on empirical cost-functions supporting such trade-offs remains scarce at the global level. Here, based on cost engineering modelling, we show that optimised spatial allocation of 10 major crops, would reduce current costs of agricultural production by approximately 40% while improving environmental performance. Although production inputs per unit of output increase at local scales, a reduction of cultivated land of 50% overcompensates the slightly higher field-scale costs enabling improved overall cost-effectiveness. Our results suggest that long-run food prices are bound to continue to decrease under strong environmental policies. Policies supporting sustainability transitions in the land sector should focus on managing local barriers to the implementation of high-yield regenerative agricultural practices delivering multiple regional and global public goods.
Introduction
Increasing competing demands for land, water and energy (Steffen et al., 2015) along with increasing world population call for strategies to minimise environmental impacts while producing adequate food for 10 billion people (United Nations, 2017; Ramankutty et al., 2018). The Sustainable Development Goals (SDGs) agenda of the United Nations articulates conditions for the sustainable management of these challenges, through the environmental sustainability pillar and SDG-2, that aims to achieve food security and promote sustainable agriculture (UN, 2015)–objectives vital to the success of the entire agenda (FAO, 2016a). In this context, SDG-2 targets “doubling agricultural productivity” (Target 2.3), yet this is not globally applicable as in different regions, with certain yield limitations (Tumushabe, 2018), this would contrast the goal of sustainable agriculture (Gil et al., 2019). In this context, the debate of land sparing vs. land sharing has emerged assessing balances between environmental conservation and agricultural yields (Lamb et al., 2016). Land sparing entails setting aside land utilised for high-yield agricultural production on a small land footprint to allow for biodiversity conservation on non-agricultural land (Balmford et al., 2015; Kremen, 2015; Phalan, 2018). On the contrary, land sharing advocates integration of environmental conservation and food production incentives on the same plots of land through low-intensity systems on a larger land footprint (Kremen, 2015). In the present analysis, agricultural intensification exceeds the traditional perspective of high-yield farming resulting from high-input high-output relationships but rather, is reconciled with natural restoration though spatially optimised land-sparing that enables closing current “yield gaps” (the difference between observed and attainable yields in a given location) (Grassini et al., 2015).
The land sparing-sharing model has received both positive and criticizing reviews (Phalan, 2018). Criticism in land sparing stems from the intensive use of agricultural land that is required to achieve high yields (Law and Wilson, 2015) which has led to the development of arguments suggesting trade-off relationships between productivity and socioeconomic goals (Kremen, 2015; Frison, 2016; Mehrabi et al., 2018), productivity and environmental conservation (Lamb et al., 2016) or the necessity of demand-side adjustments to meet environmental and food security goals (Erb et al., 2016). Kremen (2015), points out that sustainable intensification provides the means to spare land in a capital- and input-intensive way which fails to address global hunger due to the existing inequalities in resource distribution. Egli et al. (2018), suggest that the high-input agriculture associated with attempts to close yield-gaps on existing croplands and increase food security, negatively affect multiple dimensions of biodiversity. On the contrary, research has shown that land sparing can resolve such trade-offs by producing adequate volumes of food and also by improving environmental performance of agricultural production, through yield gaps closure that results in reduced emissions, irrigation and fertilisation (Cannon et al., 2019; Feniuk et al., 2019). The added value of the land sparing concept has resulted in the development of incentives providing evidence in production practices that reduce emissions (Folberth et al., 2020) and generate cost-effective solutions (Desquilbet et al., 2017). Recent studies have highlighted the importance of integrated approaches studying policies for land sparing taking into consideration economic mechanisms and feasibility (Lamb et al., 2016; Salles et al., 2017) yet, agricultural production costs in the land sparing context have been overlooked by the literature.
Due to its links with environmental and food security objectives, the global agricultural transition in cost-effective production systems is a great planetary challenge. As economics drive agricultural systems decisions (Marinoni et al., 2012), policy solutions will need to analyze the quantitative relationships between food production goals, environmental targets and agricultural production costs on which we focus in this paper. Here we provide an initial and fundamental estimation of the global costs of agricultural production to test the basic hypothesis that production of current food baskets at global scales is less costly in land-sparing scenarios than in present conventional production practices. We accomplish that by comparing actual business-as-usual plans including current cropland allocation and production practices (BAU) to land sparing strategies designed to minimise cropland expansion by approximately 50% of the current cropland extent while maintaining present food production volumes (MLS and TLS). We conduct financial estimations at sub-national scales (in varying spatial simulation units of ∼ 9.26 km × ∼ 9.26 km–∼ 55.56 km × ∼ 55.56 km, see Methods) that were aggregated to estimate national and global costs of agricultural production (Figure 1). While the focus of the present analysis was to investigate cost functions in agricultural production under current and two land sparing production scenarios, we also quantified crop-specific food supply implications through metrics that include global supply curves as well as costs of energetic values per unit of output across the three scenarios. The estimation of financial implications of land sparing production scenarios is based on hypothetical crop land use allocation while implementation barriers such as transaction costs, lack of access to knowledge and best available technologies are left aside. Our results, nonetheless, deliver insights, addressing policy makers, on systems cost functions under land-sparing targets. This novel effort to synthesise cost implications on agriculture at global scales, provides direct information on cost-competitiveness of alternative production scenarios thus, highlights their financial attractiveness–knowledge critical to the development of policies designing the ways in which food production systems could evolve and be managed in the future.
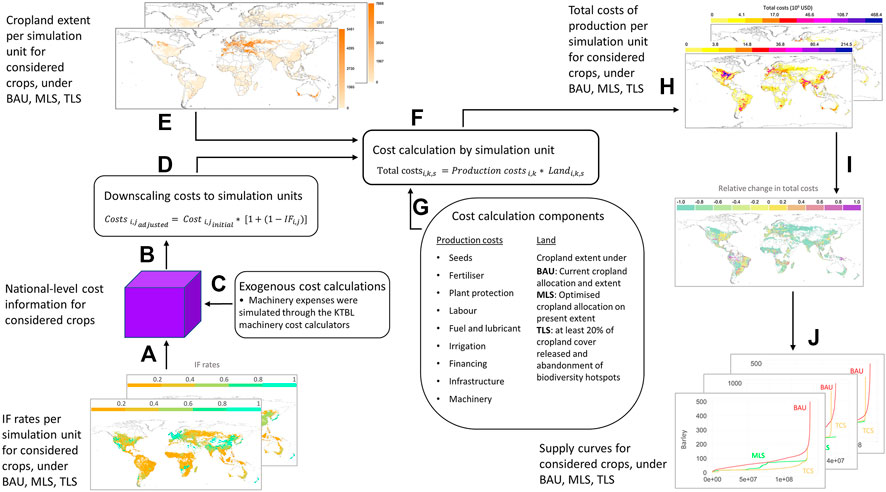
FIGURE 1. Schematic design of the study, (A–J). Intensification factor (
We seek to contribute to the examination of the longstanding hypothesis that trade-off relationships link agricultural productivity and environmental performance as well as agricultural productivity and social (food security) and economic (cost effectiveness) objectives suggesting whether this hypothesis can be rejected at a global scale. We apply a novel bottom-up cost engineering assessment at sub-national scales for 154 countries to provide crop-specific information on cost-effectiveness gains from two land-sparing case study production scenarios for the production of ten major crops that include barley, groundnut, maize, potato, sugar beet, rice, sunflower, sorghum, soybean and wheat (Supplementary Table S1). The ten crops considered herein provided 52% of total direct human calorie intake and 63% of plant-based direct human calorie intake in the year 2015 (FAO, 2016b). Evaluating the potential of current global agricultural systems to adapt land-sparing strategies provides insights about reductions in production costs and land requirements for the supply of sufficient food while addressing environmental performance objectives. The global perspective of the analysis enables us to identify broad trends of cost-effectiveness and spared cropland while the sub-national cost accounting capabilities of our framework allow the identification of increased cost patterns at the field scale, as a result of production intensity. In turn, this provides critical inputs to national research priorities that seek to evaluate the cost-competitiveness of such strategies across different crops, cropping systems and locations to promote their implementation.
This paper has five sections. Section “Introduction” provides an overview of land-sparing as a strategy to reach environmental and food security SDGs and briefly introduces the main methodological steps followed in this assessment. Section “Literature review on land-sparing trade-offs and production costs” discusses previous research on land-sparing and highlights the research gaps identified and aimed to be addressed in the present analysis. Section “Methods” describes in detail the study design, cost engineering framework as well as physical and financial modelling set up. In the next section, “Results” we analyze global agricultural production costs and present the main findings in the context of cost effectiveness gains between different production scenarios, the spatial distribution of costs and the global food supply implications. Finally, Section ‘‘Discussion and conclusion’’ concludes with main lessons from the cost implications of the case study land-sparing strategies.
Literature Review on Land-Sparing Trade-offs and Production Costs
Global-scale studies on land-sparing were sparked around 1996 (Waggoner, 1996) and have ever since examined the environmental and economic responses of agricultural production systems from different production strategies aiming to produce given amounts of food with least harm to biodiversity. Recent empirical evidence from Balmford et al. (2015), Finch et al. (2019), suggest that high-yield agricultural production separated from conservation of nonfarm ecosystems (land-sparing) has greater potentials than wildlife-friendly farming over expanded areas (land-sharing) to limit the ecological cost emerging from the production of food. Cannon et al. (2019), investigate ecological implications of competing land-use strategies and find that land-sparing agriculture conserves great functional diversity of species supplying key ecological functions. However, several approaches have identified a number of substantive concerns for biodiversity conservation, associated with the high intensity and specialisation of production on the parts of land that are being devoted for food production (Emmerson et al., 2016; Landis, 2017; Egli et al., 2018). Along these lines, Kremen and Merenlender (2018), suggest that we must join biodiversity conservation objectives into the landscapes we use in order to avoid mass extinction and ecosystem destruction. In this context, Grass et al. (2019) highlight that such solutions are not mutually exclusive, as both are required to harmonise management choices for the multifunctionality of agricultural landscapes (Seppelt et al., 2016).
Phalan, (2018) reviews the conceptual and analytical strengths of the land sparing-sharing framework suggesting that while it is a model of biophysical, and not economic relationships, it provides a method to produce economic measures such as opportunity costs. To that end, Zabel et al. (2019), examine the implications of cropland expansion and agricultural intensification as ways to respond to the increasing demand patterns and find that while both would negatively affect biodiversity, increased food production will reduce crop prices under these scenarios. Pannell et al. (2014), investigate the economics of land conservation approaches, as strategies that address food security and land degradation, identifying the economic drivers that have been influencing the adoption of conservation agriculture practices. Runting et al. (2019), explore economic returns in wood products under land sparing-sharing strategies and best practice implementation in tropical forests suggesting that sparing provides better environmental benefits than sharing and also leads in lower costs than in better management.
Although the literature provides an extensive discussion of the relationships between productivity and biodiversity in the context of land restoration, it rarely examines the economic implications triggered by such production alternatives through analytical methods (Ephraim et al., 2016). Owing to the systems inherent complexity but also lack of consistent and adequate crop-specific financial information at global scales, to the extent of our knowledge, there are no analytical approaches investigating crop-specific and spatially explicit global-scale costs of production under existing and land-sparing production scenarios. The latter emerges as a significant research gap because it is necessary to evaluate the crop- and location-specific financial attractiveness of sustainable practices (Piñeiro et al., 2020) as higher costs essentially trigger higher risk to farmer livelihoods, and this is one of the aspects that we aim to improve alongside food security and environmental sustainability. Providing such an analytical framework, addresses this need for knowledge which is essential for investigating the cost-competitiveness of different agricultural production strategies.
Material and Methods
Study Design and Intensification Scenarios for Land Sparing
The study investigated the implications on costing mechanisms within agricultural production on the basis of land-sparing production scenarios. We conducted an engineering cost assessment over current practices to compare them with land sparing strategies. To achieve that, we developed the present global costing framework in such way that has the capacity to assess cost-effectiveness in the land sparing scenarios under consideration.
Here we develop a cost accounting method to investigate potential cost-effectiveness in land sparing production scenarios. We estimate costs for three production scenarios namely, i) actual business-as-usual plans including current cropland allocation and production practices (BAU), ii) maximum land sparing (MLS) where cropland extent is optimised allowing the entire present cropland in each simulation unit or pixel to remain occupied after crop reallocation if it is a solution of the optimisation and iii) targeted land sparing (TLS) where cropland extent has been optimised with an enforced uniform release of at least 20% of cropland cover in each simulation unit or pixel and the abandonment of biodiversity hotspots by simultaneously achieving attainable yields of 10 major crop commodities (optimisation modelling developed by Folberth et al., 2020). The optimisation method modelled spatial allocation of agricultural systems at global scales while maintaining crop-specific production volumes reported by FAO for the years of 2011–2015 (FAO, 2016b). In this study MLS represents a reference point of what degree of land sparing scenarios are technically feasible given attainable yields and current agricultural technologies. Additionally, TLS provides a reference point for a global scenario combining habitat restoration of threatened species and introducing systematically distributed landscape slots as wildlife habitats (Feniuk et al., 2019) or zones to compensate for negative impacts of intensive agriculture (Schulte et al., 2017).
The harvested area of the ten crops considered encompasses presently 62% of total cropland. Several of these crops play an important role in livestock feed supply (FAO, 2016b). However, this is challenging to fully quantify at global scales as crop uses are reported in FAOSTAT only for the primary step, which is in the case of feed stuffs in some cases processing. To provide an overview of major uses for the crops selected for this study, we compiled major crop uses in Supplementary Table S2.
Cost Accounting Modelling Set Up
This method enables geographically explicit calculation of agricultural production costs for the various crop commodities and management methods. To simulate production costs, our framework includes direct (variable) and indirect (overhead) costs of production. A full list of costing elements and disaggregated items is presented in Supplementary Table S3 Production costs (per hectare) and total costs of production for cell
and,
Where
Physical and Financial Data
To estimate cost functions, we look at the intersection of biophysical and economic functions (Figure 1) This analysis integrates current knowledge of high-yield farming and optimised land use strategies to estimate the economic consequences of changes in global agricultural production resulting from the two land use scenarios. For physical information, current yields and harvested area were derived from SPAM 2010 v1.1 (International Food Policy Research Institute, 2019) this data was scaled up and to match food production volumes during the reference period (2011–2015) using FAO reported values on production of the ten crops. Attainable yields and corresponding nutrient requirements were derived from the established global gridded crop model EPIC-IIASA (Balkovič et al., 2014). Information was derived explicitly for sub-national grid cells that vary in sizes of ∼ 9.26 km × ∼ 9.26 km–∼ 55.56 km × ∼ 55.56 km (5′ × 5′ to 30′ × 30′ arc minutes at the equator). This grid reference is a result of EPIC-IIASA integrating the process-based agronomic model ‘Environmental Policy Integrated Climate (Williams et al., 1989; Izaurralde et al., 2006) (EPIC) to a global data infrastructure referenced at 5′ x 5′ spatial resolution. These five arcmin grid cells belong to the same topography classes, have identical soil texture and are located within the same 30′ x 30′ climate grid and administrative region cells that were then aggregated to simulations units. As a result, we have approximately 120,000 simulation units in varying sizes corresponding to surface areas from ∼ 69 to ∼ 2,500 km2 near the equator conditional to input data heterogeneity.
Financial information is organised in a cost engineering framework, using a bottom-up cost assessment formula that includes direct costs and overheads at the field-scale, following the cost accounting system of the Farm Accountancy Data Network (FADN)1 and the Agri-benchmark2 network. We compiled a novel global data set containing information on production costs (reflecting costs from the perspective of the farmer) at national scales for 10 major crops derived from agricultural data surveys and platforms, the scientific literature as well as official statistical data. In such way, we developed a baseline of countries representing a range of production practices that was used to extrapolate national-scale financial information on production costs from data-rich countries in locations where no information was available (further description on data sources for listed production costs is available in Supplementary Tables S3, S4). To create this baseline of countries, we introduced a classification of countries based on technological adoption per country using the global cropland field size index developed by Fritz et al. (2015). Our intuition on the latter is based on evidence suggesting a relationship between field size and technology adoption (Mittal and Mehar, 2016; Brown et al., 2018) which we tested as an assumption with the number of tractors in use per country indicator provided by FAOSTAT (Supplementary Figure S1A). Furthermore, to test this relationship we examined the relationship between GDP (as an indicator of incomes and expenditure on goods and services) and field sizes finding that higher GDP per capita is related to larger field sizes (Supplementary Figure S1B). This set of relationships has been examined by Dethier and Effenberger (2012) who find that lack of credit leads in either low technological adoption directly or in the need for a loan to withstand the initial investments. Collateral then is required for the poorer farmers and when they also lack land ownership (or own very small parts), they are restricted from taking a loan and thus, physical and economic size could then determine levels of technology adoption. Furthermore, Schimmelpfennig (2016) investigates the ways and whether farm managers decide to adopt new technologies highlighting that farm size (determined from total cropland area) is a driver influencing adoption based on the finding that farms adopting agricultural technologies tend to be of larger size than those that do not. Schimmelpfennig’s study offers support to relevant approaches that similarly suggest smaller farm sizes have an inverse relationship with technological adoption (Cavallo et al., 2014; Mottaleb et al., 2016; Das V. et al., 2019).
Furthermore, we utilised the established online plant process cost calculator developed by the agricultural advisory board for engineering and building (KTBL) (KTBL, 2020) to estimate country-level and crop specific machinery expenses, based on respective technology adoption and soil properties. The online plant process cost calculator simulates costs of machinery, for a range of crops, as a function of machinery power (
Following the extrapolation, pricing information was equalised per country with the use of the purchasing power parities (PPP) indicator provided by the World Bank (World Development Indicators database, 2019a). Specifically, as shown in the basic equation bellow, cost of input
Spatial Explicit Cost Estimates and Intensification Factor
Our assessment quantified the intensity of production (Intensification Factor ratio -
With
Where
For production systems with
Moreover, even though the present study estimates production costs in such granular spatial scales, it is imperative that, in order to understand cost functions, we compare trends between total costs of different scenarios and assess cost-effectiveness among them rather than focus on absolute estimates at a simulation unit basis.
Reference Period
In this study we conduct a cost engineering assessment to increase the understating around agricultural costing mechanisms and thus, for our estimations we use prices on the basis of a uniform reference period to indicate production inputs real prices. As such, in our cost model prices on production inputs were equalised in financial contexts around the year 2000 with the use of inflation rates and specifically the consumer price index (World Bank, World Development Indicators database, 2019b). Furthermore, to allow a global assessment, a monetary consistency was of essence for which we used the purchasing power parities (PPP) metrics obtained from the World Bank (World Development Indicators database, 2019a) to transform prices from local currencies to United States dollars. Finally, to then bring cost estimates from 2000 at the food production reference period (2011–2015) we inflated prices to an average of these years. The latter enables the study to derive estimations directly for various years by inflating or deflating costs without rerunning all processing.
Model Estimations Evaluation
To assess the validity of our estimations, we cross-referenced the costs per tonne for the 10 crops to FAOSTAT reported producers’ prices per tonne which are the prices at the farm gate per country and crop commodity. For each of the crop commodities we derive costs per tonne through a fraction of total costs (costs per hectare multiplied by the corresponding yield) over produced tonnes (attainable or current yield multiplied by cropland extent) and find that estimated costs per tonne are consistently bellow the reported producers’ prices which assures us that our estimations follow the pattern globally and fall within expected numerical regions (Supplementary Figure S5).
Furthermore, we conducted cross validations on modelled technological costs as well as fertiliser costs. Specifically, we analyzed the composition of total costs by investigating cost analogues for the aforementioned elements. We are particularly interested in analogues rather than absolute costs as the purpose of this analysis is to compare cost trends between the three production scenarios and assess potential cost effectiveness between them. We do this by comparing reported and modelled cost analogues and find that for machinery expenses the extrapolation method produces an estimated analogue very close to the reported when looking at US costs of production (Supplementary Figure S6). Concerning fertiliser cost analogues, we cross validated modelled values with data derived from FADN on 20 EU countries. The data provided is in an aggregated farm type format covering the categories of cereals and root plants. Results of the validation demonstrate that for most of the countries the differences in cost analogues are less than 10% (Supplementary Table S5). Exceptions are countries of small or very small field sizes which used information from the baseline from developing countries (e.g., India, Georgia and Azerbaijan). This points out that there are intra-classes within our classification system that could be further developed in the future to increase accuracy of modelled values (i.e. field size classes break down based on regions or continent).
Results
Total Costs of Production Under Business-As-Usual, Maximum Land Sparing and Targeted Land Sparing Scenarios
Our results demonstrate a clear pattern of differentiation in global production costs for crop commodities between the BAU, MLS, and TLS production scenarios (Figure 2) that is strongly driven by the reduction of cropland extent by 50%. Globally, we estimate that total costs of agricultural production extend to $255, $149, and $166 bn for BAU, MLS, and TLS, respectively, indicating a cost-effectiveness of ∼41.3 and ∼34.8% for MLS and TLS, respectively. First, a negative impact in cost-effectiveness is expected due to higher localised inputs imposed by closing yield gaps through the supply of sufficient nutrients to meet plant requirements. Particularly, in cases where production requires higher levels of inputs, we find that on average, costs per hectare for all crops increase by 5.3 and 4.9% globally in the MLS and TLS scenario respectively, compared to business as usual costs. Nevertheless, in particular cases, MLS and TLS production systems can be less intensive than in, business as usual due to decreased production inputs and there we observe reductions of costs per hectare by 3.7 and 3.6%, respectively, (Figure 3 and Supplementary Figure S3). Such cost fluctuations are expected as we observe that the optimisation of cropland allocation shifted the intensity of production, and in particular, production systems are generally more intensive in MLS than in BAU and TLS (Figure 3; Supplementary Figure S2).
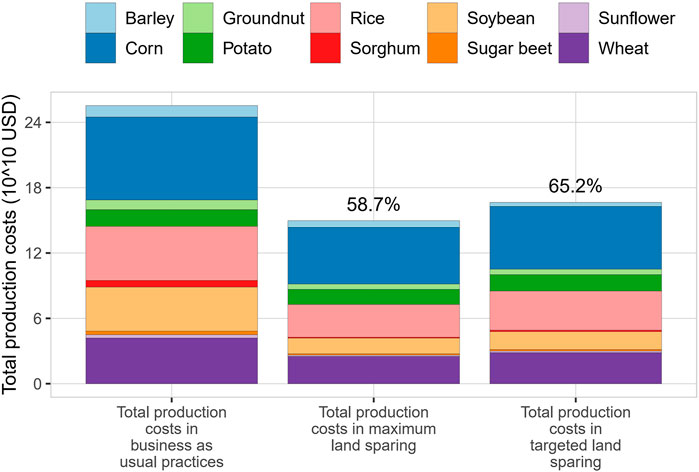
FIGURE 2. Global costs of production in the business as usual, and the two land sparing scenarios. The stacked bars demonstrate the estimated total costs for the production of 10 major crops in actual business as usual for the reference period of 2011–2015 (left bar), estimated total costs for the production in the maximum land sparing (MLS) scenario (middle bar) and sparing of at least 20% of cropland in each simulation unit and entirely abandoning biodiversity hotspots (TLS) (right bar). On top of the middle and right bar, percentage values regard total costs of production for the 10 considered crops relative to the total costs in the business as usual scenario. In a global scale, total costs of production in BAU are approximately $255.39 bn while in the sparing scenarios costs are approximately $149.74 and $166.49 bn in MLS and TLS, respectively.
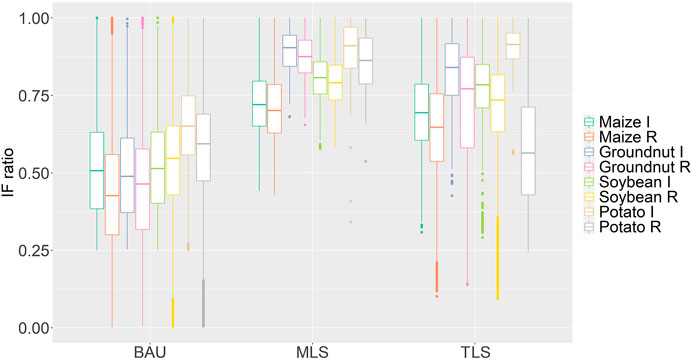
FIGURE 3. Intensification factor per crop, simulation unit and water regime (rainfed – R and sufficiently irrigated—I) in the business as usual and the land sparing production scenarios. Boxplots display the distribution of intensity of production index (IF) in the business as usual and the two land sparing production scenarios for selected crops. Here we present IF estimates for maize, groundnut, soybean, and potato each as a representative crop, based on the FAOSTAT reported global production volumes, of the crop categories considered in this study (cereals, legumes, oil and protein crops and root vegetables, respectively). The IF index comprises of four basic components estimating intensity of production based on input (N and P fertiliser) and output (current and attainable yields) relationships (see Methods). Globally, production systems of low intensity in both MLS and TLS (
Second, the spatial occurrence of cropland use in MLS and to a lesser extent TLS is more prominent in regions with more favourable agroclimatic conditions, and thus, higher attainable yields, for each of the examined crops (Folberth et al., 2020). Thus, we observe that global scale
Mapping Total Agricultural Costs
Regions with significant reductions in total costs of production in MLS compared to BAU (Figure 4A) include areas with unfavourable biophysical properties such as the West coast of the United States and parts of central Asia but also in more productive regions including South Asia and South Russia. Likewise, in MLS globally, costs remain high in the areas of Central North America, East Latin America, North West Europe and some parts of South Asia. In TLS, the geographical distribution of cropland spans more widely and thus, we observe a similar pattern to the cost distribution of MLS with the addition of concentration of higher costs in Northern North America, West Europe and a significant spatial expansion of production-cost hotspots in South Asia (Figure 4B). Collectively, on a simulation unit basis and across the three scenarios we observe that total production costs in BAU extend to a smaller scale but to a significantly larger geographical extent. MLS and TLS estimate increased local costs compared to BAU due to higher intensity of production but in smaller land extent that ultimately results in greater cost effectiveness at global scales (Supplementary Figure S4).
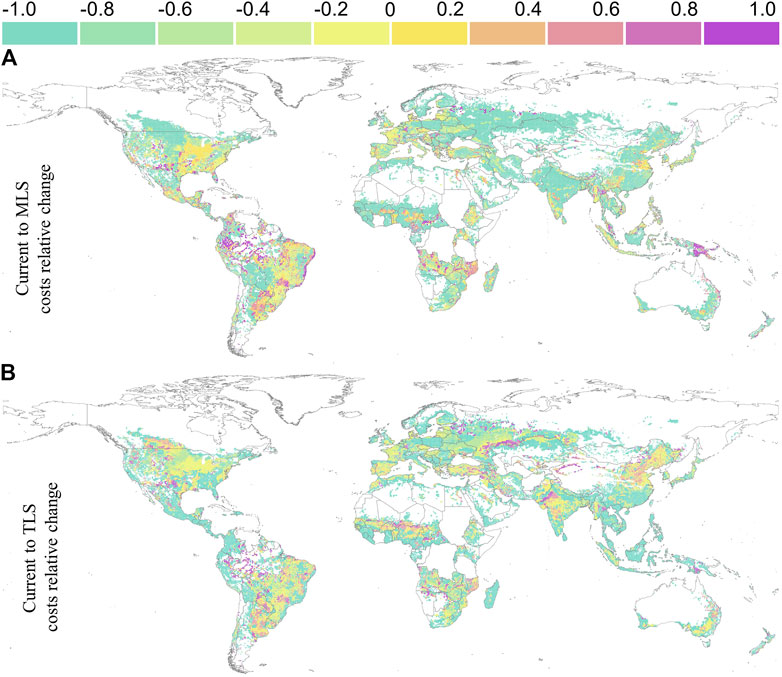
FIGURE 4. Relative change in total costs of agricultural production for the ten major crops. (A). Relative change in costs between current production costs and costs under the maximum land sparing production scenario (MLS) (B). Relative change in costs between current production costs and costs under sparing of at least 20% of cropland in each simulation unit and entirely abandoning biodiversity hotspots (TLS).
Global Food Supply Implications
Herein we investigate the implications on food supply at a global scale and also assess cost fluctuations between the three production scenarios BAU, MLS, and TLS (Figure 5). We find that while the magnitude of food baskets remains the same across the scenarios, total costs of production per tonne and crop commodity is consistently lower in the land sparing scenarios in any given geographical part. With this finding we demonstrate that food demand can be met at a lower cost of production per tonne–a primordial and critical factor to the performance of supply chains (Gold et al., 2017; Validi et al., 2014). We further assess food security implications and investigate costs of energy produced for the 10 crops considered. In this context, food prices were calculated by keeping everything else equal without taking into consideration any dynamic interactions in response to demand or income and employment. Under the present land sparing scenarios, no energy intake loss is due to occur as costs per Mcal produced decrease (Figure 6). This is of essence to the current consensus where studies attempt to enhance global food security through the utilisation of crop-based solutions (Aiking, 2011; Day, 2013; Young and Skrivergaard, 2020). Naturally, as the optimisation spatially reallocated agricultural systems globally, parts of the globe would rely on trade to achieve production inputs sufficiency (fertiliser) along with feed sufficiency – factors that affect food security in far deeper ways than merely yield gaps (Savary et al., 2012), which calls for robust global supply chains to assure resilience and constant food supply (Seekell et al., 2017; Cole et al., 2018; Kinnunen et al., 2020). Interestingly, MLS and TLS supply curves often intersect, indicating which of the two strategies would result in the most economically efficient system at any given simulation unit. The latter signifies the potential value of a crop-specific, spatially targeted integration of land sparing strategy to best facilitate increased food production at the minimal economic and environmental cost.
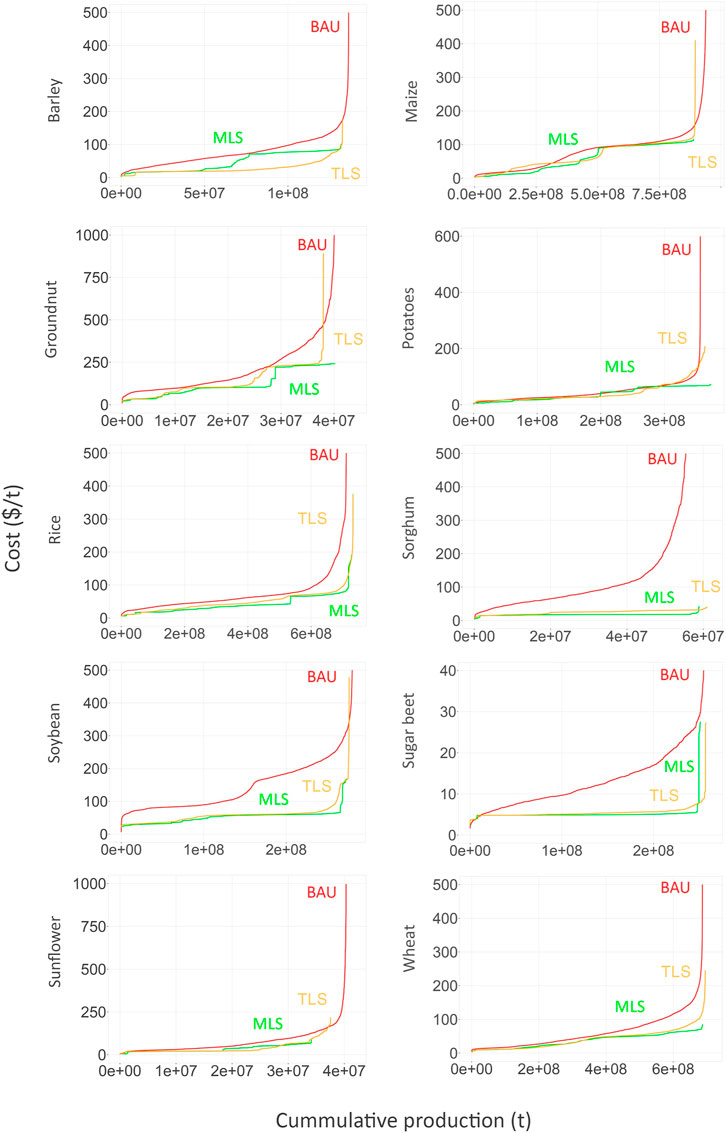
FIGURE 5. Supply Curves for the 10 crops around the years 2011–2015. The graphs illustrate supply curves representing cumulative production per simulation unit, expressed in tonnes (
Discussion and Conclusion
The effects of environmental conservation on agricultural commodity prices have been highly disputed in the academic literature where it has been indicated that trade-off relationships exist between food security and strategies for conservation (Tscharntke et al., 2012; Pannell et al., 2014; Frank et al., 2017). Here, we develop a cost engineering framework that combines biophysical and financial information of agricultural production systems for ten major crops and compare cost-effectiveness gains between current production practices (BAU) and two land-sparing alternative scenarios (MLS and TLS). Our analysis shows that through a global lens, land-sparing production practices would enable yield-gap closure and thus, allow almost 50% of current cropland extent to be spared which results in lower agricultural production costs than the existing production practices. Findings in the present study demonstrate that the examined land sparing production scenarios reduce aggregate food costs by up to 40% at a global scale.
Closing yield gaps is subject to technical and knowledge requirements with emerging externalities mostly across social dimensions. Concerning the former, our study provides a closed system cost assessment of best available technologies (BAT) (OECD, 2018) where new food production technologies (Herrero et al., 2020) are not accounted for, while the additional yield improvements are not due to better genetic material or plant protection. Specifically, increased yields per unit of land result from intensified application of sufficient nutrients to meet plant requirements and the optimal spatial reallocation of production systems that takes advantage of biophysical characteristics. Moreover, lack of essential knowledge poses a significant barrier in implementing efficient production practices to close yield gaps (Lobell et al., 2009) while lack of credit to respond in production inputs requirements limit agricultural production (Tittonell and Giller, 2013). Seasonal forecasts are not yet good enough to supply farmers with the confidence they need in a highly variable (and therefore risky) environment. As Hochman et al. (2013) demonstrate, risk-averse farmers are hesitant to supply crops with enough N fertiliser unless they are convinced they will earn a good return on investment at harvest, which results in many farmers underapplying N. Therefore, across developed countries with expert farmers, the problem is related to lack of knowledge for best crop rotation (Hochman et al., 2020), based on their location, rather than lack of essential knowledge as well as uncertainty due to high seasonal climate variability. Performance based payments could be motivated as a suitable policy (instrument) option incentivising yield gap closure or it could even be part of sustainability contracts in business practice. The respective environmental accounting standards will need to be developed as the benefits of yield gap closure are accrued through indirect land use effects of local intensification. Regarding social externalities, studies have already addressed the diverse social implications from such strategies (Schleicher et al., 2019) that may affect local food self-sufficiency (Folberth et al., 2020) but also trigger contractions in agricultural incomes, and adversely affect economically local rural populations, imposed by inherently unequally distributed agro-economic efficiencies (Grau et al., 2013). In this context, a range of policy measures have been proposed to financially compensate for abandoned cropland, best manage re-established vegetation as well as exchange knowledge and co-operate infrastructure in order to incentivise implementation of cropland sparing (Phalan et al., 2016).
We contest the longstanding hypothesised trade-off relationships between food security and strategies for conservation and point out that release of cropland does not necessarily entail expansion of costs for the production of agricultural commodities and thus, does not lead in increased food prices. Even though the latter is a result of many factors, long-run food prices are bound to continue to decrease along with historical trends if systems produce more efficiently and close yield gaps by switching where (agro-climatically favourable locations) and how (high-yield farming) food is produced. There is no reason for food prices to escalate in the long-run, neither under business as usual production strategies nor with the implementation of strong policies promoting land sparing.
Policies promoting land sparing could be productivity-based policies that switch subsidies from decoupled payments to a subsidy system that rewards higher yields and environmental goods thus, promoting economic growth from practices enhancing sustainability rather than diminishing natural capital (Tanentzap et al., 2015). In that context, owners of fertile land are encouraged to take up effective reward-by-result options that further promote improvements of farming efficiencies in food production systems (Merckx and Pereira, 2015). Through this location-specific focus, outcome-based payments are being spatially targeted and this improves their economic efficiency, as different locations will have different cost-effectiveness in delivering any given environmental benefit (Reed et al., 2014). Result-based payments provide opportunities for achieving biodiversity objectives effectively, allowing flexibility for the farmers in the management practices chosen to achieve the environmental goals thus, encouraging farm innovation and cost-efficiency (Matzdorf and Lorenz, 2010; Magda et al., 2015; Russi et al., 2016). To achieve environmental outcomes, high-yield farming associated with land sparing strategies needs to be combined with allocation of land for conservation elsewhere (Phalan et al., 2016; Finch et al., 2019). Therefore, the marginal (less productive) land is going to be spared for ecological restoration therein, production systems existing on such landscapes, would be encouraged financially to take up ecosystem services options such as compensation for land left out of production and for planting woodland clusters (Rey Benayas and Bullock, 2012; Zahawi et al., 2013). Land governance, is a rather complicated process where multiple dynamics compete with each other to produce food, conserve natural values or achieve both at an optimally minimal trade-off between the two (Hodgson et al., 2010; Garnett and Godfray, 2012). Thus, to bridge the global targets it is imperative that strategies will lead to effective environmental conservation without delivering unequal socio-economic burdens (Ellis, 2019) and this probably emerges as one of the most significant challenges for the land sparing strategies implementation (Phalan et al., 2016; Folberth et al., 2020).
This analysis provides an evidence-based comparison of how land-sparing production strategies affect agricultural production costs at a global level. However, our framework does not account for the other dimensions of systems transition relative to local constraints, and specifically technical, knowledge, and financial capital limitations. While it is unrealistic to assume that systems change would be independent of these parameters, it can be rationally hypothesized that best available technologies and management practices applied locally will enhance this process. In addition, our model ignores the effects of global cropland reallocation on food trade and while large shares of the worldwide population depend on food imports (26–64%) (Kinnunen et al., 2020), we may underestimate the implications for particular regions where the existing trade balances would change under land-sparing alternatives. The analysis of such limitations and effects in a partial equilibrium model will be the subject of future research. Furthermore, our assessment neglects potential impacts of income and prices on food demand patterns. Relevant empirical studies have assessed such implications on food security suggesting that increases in food commodity prices would decrease food consumption or switch demand to less expensive food products but also finding that increase of market prices by 20% would reduce food consumption by 3% by 2050 (Hasegawa et al., 2014, 2018; Baldos and Hertel, 2014; Nelson et al., 2014). Therefore, the relationship between food demand and market prices is found to be less elastic and thus, these effects would have small-scale impacts on our results. Despite these caveats, the land sparing strategies under consideration seem to have the capacity to enhance food availability at a societal level. Further technological and institutional interventions would be of essence to ensure a meaningful transition for the global poor farming systems providing off-farm and diversification options as alternatives to deemphasize or abandon agriculture as the principal livelihood activity (Ritzema et al., 2017; Thornton et al., 2018).
In conclusion, our results suggest that land-sparing production strategies can reduce global food production costs by up to 40%. Achieving such agricultural landscape organisation and the associated cost-effectiveness requires steps to inform policy making and stakeholders about the economic, environmental and food security benefits. Our study could also be extended to explore subnational production systems variability and technological adoption as well as heterogeneity of soil types and properties – factors very critical to the determination of cost functions within agricultural production systems. Yet, the global and empirical approach of our study is imperative for understanding the cost functions and enable the economic evaluation of the optimised spatial rearrangement of food production as a global strategy. Our cost engineering estimations of production of ten basic crops can also enable the discussion of real options for farmers and landholders as well as policy design to enhance food security in a win-win strategy for the economy and the environment.
Data Availability Statement
The original contributions presented in the study are included in the article/Supplementary Material, further inquiries can be directed to the corresponding author. Datasets required for reproducing key results of the crop production cost engineering model are available from https://ora.ox.ac.uk/objects/uuid:933edc45-43f5-43ed-870a-fe0077916923. Data pre-processing, analysis and estimations were carried out in R (R Core Team, 2014) using the package “dplyr” (Wickham et al., 2020), where visualisations and plots were created using the packages “ggplot2” (Wickham, 2016) and “raster” (Hijmans et al., 2020). Geographical visualizations of results and inputs were produced with ESRI ArcGIS 10.6.1 (ESRI, 2018).
Author Contributions
YV and MO contributed to conception and design of the study. YV and CF organized the database. YV performed the analysis and wrote the first draft of the manuscript. SCB, CF, MO, and YV wrote sections of the manuscript. All authors contributed to manuscript revision, read, and approved the submitted version.
Funding
This research was funded by the Wellcome Trust, Our Planet Our Health (Livestock, Environment and People–LEAP), award number 205212/Z/16/Z.
Conflict of Interest
The authors declare that the research was conducted in the absence of any commercial or financial relationships that could be construed as a potential conflict of interest.
Supplementary Material
The Supplementary Material for this article can be found online at: https://www.frontiersin.org/articles/10.3389/fenvs.2021.672663/full#supplementary-material
Footnotes
1For further information on data collection methods and definitions see https://ec.europa.eu/agriculture/fadn_en
2For further information on data collection methods and definitions see http://www.agribenchmark.org/home.html
References
Aiking, H. (2011). Future Protein Supply. Trends Food Sci. Technol. 22, 112–120. doi:10.1016/j.tifs.2010.04.005
Baldos, U. L. C., and Hertel, T. W. (2014). Global Food Security in 2050: the Role of Agricultural Productivity and Climate Change. Aust. J. Agric. Resour. Econ. 58, 554–570. doi:10.1111/1467-8489.12048
Balkovič, J., van der Velde, M., Skalský, R., Xiong, W., Folberth, C., Khabarov, N., et al. (2014). Global Wheat Production Potentials and Management Flexibility under the Representative Concentration Pathways. Glob. Planet. Change 122, 107–121. doi:10.1016/j.gloplacha.2014.08.010
Balmford, A., Green, R., and Phalan, B. (2015). Land for Food & Land for Nature? Daedalus 144, 57–75. doi:10.1162/DAED_a_00354
Brown, W. M., Ferguson, S. M., and Viju, C. (2018). Farm Size, Technology Adoption and Agricultural Trade Reform: Evidence from Canada (SSRN Scholarly Paper No. ID 3200345). Rochester, NY: Social Science Research Network.
Cannon, P. G., Gilroy, J. J., Tobias, J. A., Anderson, A., Haugaasen, T., and Edwards, D. P. (2019). Land‐sparing Agriculture Sustains Higher Levels of Avian Functional Diversity Than Land Sharing. Glob. Change Biol. 25, 1576–1590. doi:10.1111/gcb.14601
Cavallo, E., Ferrari, E., Bollani, L., and Coccia, M. (2014). Strategic Management Implications for the Adoption of Technological Innovations in Agricultural Tractor: the Role of Scale Factors and Environmental Attitude. Technol. Anal. Strateg. Manag. 26, 765–779. doi:10.1080/09537325.2014.890706
Cole, M. B., Augustin, M. A., Robertson, M. J., and Manners, J. M. (2018). The Science of Food Security. Npj Sci. Food 2, 14. doi:10.1038/s41538-018-0021-9
Das, V., J., Sharma, S., and Kaushik, A. (2019). Views of Irish Farmers on Smart Farming Technologies: An Observational Study. AgriEngineering 1, 164–187. doi:10.3390/agriengineering1020013
Day, L. (2013). Proteins from Land Plants - Potential Resources for Human Nutrition and Food Security. Trends Food Sci. Technol. 32, 25–42. doi:10.1016/j.tifs.2013.05.005
Desquilbet, M., Dorin, B., and Couvet, D. (2017). Land Sharing vs Land Sparing to Conserve Biodiversity: How Agricultural Markets Make the Difference. Environ. Model. Assess. 22, 185–200. doi:10.1007/s10666-016-9531-5
Dethier, J.-J., and Effenberger, A. (2012). Agriculture and Development: A Brief Review of the Literature. Econ. Syst. 36, 175–205. doi:10.1016/j.ecosys.2011.09.003
Egli, L., Meyer, C., Scherber, C., Kreft, H., and Tscharntke, T. (2018). Winners and Losers of National and Global Efforts to Reconcile Agricultural Intensification and Biodiversity Conservation. Glob. Change Biol. 24, 2212–2228. doi:10.1111/gcb.14076
Ellis, E. C. (2019). Sharing the Land between Nature and People. Science 364, 1226–1228. doi:10.1126/science.aax2608
Emmerson, M., Morales, M. B., Oñate, J. J., Batáry, P., Berendse, F., Liira, J., et al. (2016). “How Agricultural Intensification Affects Biodiversity and Ecosystem Services,” in Advances in Ecological Research, Large-Scale Ecology: Model Systems to Global Perspectives. Editors A. J. Dumbrell, R.L. Kordas, and G. Woodward (Academic Press), 43–97. doi:10.1016/bs.aecr.2016.08.005
Ephraim, N., Alisher, M., and von Braun, J. (2016). Economics of Land Degradation and Improvement: A Global Assessment for Sustainable Development (Cham: Springer Open).
Erb, K.-H., Lauk, C., Kastner, T., Mayer, A., Theurl, M. C., and Haberl, H. (2016). Exploring the Biophysical Option Space for Feeding the World without Deforestation. Nat. Commun. 7, 11382. doi:10.1038/ncomms11382
ESRI (2018). ArcGIS Desktop: Release 10.6.1. Redlands, CA: Environmental Systems Research Institute [WWW Document]. URL https://www.esri.com/en-us/home (Accessed 18 11, 20).
FAO (2016b). FAOSTAT Statistical Database. Rome: Food and Agriculture Organization of the United Nations. URL http://www.fao.org/faostat/en/#home (Accessed 11, 27. 19).
FAO (2016a). Food and Agriculture: Key to Achieving the 2030 Agenda for Sustainable Development. Rome: Food and Agriculture Organization of the United Nations.
Feniuk, C., Balmford, A., and Green, R. E. (2019). Land Sparing to Make Space for Species Dependent on Natural Habitats and High Nature Value farmland. Proc. R. Soc. B. 286, 20191483. doi:10.1098/rspb.2019.1483
Finch, T., Gillings, S., Green, R. E., Massimino, D., Peach, W. J., and Balmford, A. (2019). Bird Conservation and the Land Sharing‐sparing Continuum in farmland‐dominated Landscapes of lowland England. Conservation Biol. 33, 1045–1055. doi:10.1111/cobi.13316
Fischer, G., Nachtergaele, F. O., Prieler, S., Teixeira, E., Toth, G., van Velthuizen, H., et al. (2012). Global Agro-Ecological Zones (GAEZ v3.0)- Model Documentation [WWW Document]. URL http://www.fao.org/soils-portal/soil-survey/soil-maps-and-databases/harmonized-world-soil-database-v12/en/(Accessed 30 3, 20).
Folberth, C., Khabarov, N., Balkovič, J., Skalský, R., Visconti, P., Ciais, P., et al. (2020). The Global Cropland-Sparing Potential of High-Yield Farming. Nat. Sustain. 3, 281–289. doi:10.1038/s41893-020-0505-x
Frank, S., Havlík, P., Soussana, J.-F., Levesque, A., Valin, H., Wollenberg, E., et al. (2017). Reducing Greenhouse Gas Emissions in Agriculture without Compromising Food Security? Environ. Res. Lett. 12, 105004. doi:10.1088/1748-9326/aa8c83
Frison, E. A. (2016). From Uniformity to Diversity: a Paradigm Shift from Industrial Agriculture to Diversified Agroecological Systems (Report). IPES.
Fritz, S., See, L., McCallum, I., You, L., Bun, A., Moltchanova, E., et al. (2015). Mapping Global Cropland and Field Size. Glob. Change Biol. 21, 1980–1992. doi:10.1111/gcb.12838
Garnett, T., and Godfray, H. C. J. (2012). Sustainable Intensification in Agriculture. Navigating a Course through Competing Food System Priorities. Food Climate Research Network and the Oxford Martin Programme on the Future of Food. UK: University of Oxford.
Gil, J. D. B., Reidsma, P., Giller, K., Todman, L., Whitmore, A., and van Ittersum, M. (2019). Sustainable Development Goal 2: Improved Targets and Indicators for Agriculture and Food Security. Ambio 48, 685–698. doi:10.1007/s13280-018-1101-4
Gold, S., Kunz, N., and Reiner, G. (2017). Sustainable Global Agrifood Supply Chains: Exploring the Barriers. J. Ind. Ecol. 21, 249–260. doi:10.1111/jiec.12440
Grass, I., Loos, J., Baensch, S., Batáry, P., Librán‐Embid, F., Ficiciyan, A., et al. (2019). Land‐sharing/‐sparing Connectivity Landscapes for Ecosystem Services and Biodiversity Conservation. People Nat. 1, 262–272. doi:10.1002/pan3.21
Grassini, P., van Bussel, L. G. J., Van Wart, J., Wolf, J., Claessens, L., Yang, H., et al. (2015). How Good Is Good Enough? Data Requirements for Reliable Crop Yield Simulations and Yield-gap Analysis. Field Crops Res. 177, 49–63. doi:10.1016/j.fcr.2015.03.004
Grau, R., Kuemmerle, T., and Macchi, L. (2013). Beyond 'land Sparing versus Land Sharing': Environmental Heterogeneity, Globalization and the Balance between Agricultural Production and Nature Conservation. Curr. Opin. Environ. Sustainability 5, 477–483. doi:10.1016/j.cosust.2013.06.001
Hasegawa, T., Fujimori, S., Havlík, P., Valin, H., Bodirsky, B. L., Doelman, J. C., et al. (2018). Risk of Increased Food Insecurity under Stringent Global Climate Change Mitigation Policy. Nat. Clim Change 8, 699–703. doi:10.1038/s41558-018-0230-x
Hasegawa, T., Fujimori, S., Shin, Y., Takahashi, K., Masui, T., and Tanaka, A. (2014). Climate Change Impact and Adaptation Assessment on Food Consumption Utilizing a New Scenario Framework. Environ. Sci. Technol. 48, 438–445. doi:10.1021/es4034149
Herrero, M., Thornton, P. K., Mason-D’Croz, D., Palmer, J., Benton, T. G., Bodirsky, B. L., et al. (2020). Innovation Can Accelerate the Transition towards a Sustainable Food System. Nat. Food 1, 266–272. doi:10.1038/s43016-020-0074-1
Hijmans, R. J., Etten, J. van., Sumner, M., Cheng, J., Baston, D., Bevan, A., et al. (2020). Raster: Geographic Data Analysis and Modeling. doi:10.1093/oso/9780198826491.003.0099
Hochman, Z., Carberry, P. S., Robertson, M. J., Gaydon, D. S., Bell, L. W., and McIntosh, P. C. (2013). Prospects for Ecological Intensification of Australian Agriculture. Eur. J. Agron. 44, 109–123. doi:10.1016/j.eja.2011.11.003
Hochman, Z., Horan, H., Navarro Garcia, J., Hopwood, G., Whish, J., Bell, L., et al. (2020). Cropping System Yield Gaps Can Be Narrowed with More Optimal Rotations in Dryland Subtropical Australia. Agric. Syst. 184, 102896. doi:10.1016/j.agsy.2020.102896
Hodgson, J. A., Kunin, W. E., Thomas, C. D., Benton, T. G., and Gabriel, D. (2010). Comparing Organic Farming and Land Sparing: Optimizing Yield and Butterfly Populations at a Landscape Scale. Ecol. Lett. 13, 1358–1367. doi:10.1111/j.1461-0248.2010.01528.x
International Food Policy Research Institute, (2019). Global Spatially-Disaggregated Crop Production Statistics Data for 2010 Version 1.1. doi:10.7910/DVN/PRFF8V
Izaurralde, R. C., Williams, J. R., McGill, W. B., Rosenberg, N. J., and Jakas, M. C. Q. (2006). Simulating Soil C Dynamics with EPIC: Model Description and Testing against Long-Term Data. Ecol. Model. 192, 362–384. doi:10.1016/j.ecolmodel.2005.07.010
Kinnunen, P., Guillaume, J. H. A., Taka, M., D’Odorico, P., Siebert, S., Puma, M. J., et al. (2020). Local Food Crop Production Can Fulfil Demand for Less Than One-Third of the Population. Nat. Food 1, 229–237. doi:10.1038/s43016-020-0060-7
Kremen, C., and Merenlender, A. M. (2018). Landscapes that Work for Biodiversity and People. Science 362, eaau6020. doi:10.1126/science.aau6020
Kremen, C. (2015). Reframing the Land-Sparing/land-Sharing Debate for Biodiversity Conservation. Ann. N.Y. Acad. Sci. 1355, 52–76. doi:10.1111/nyas.12845
KTBL (2020). Online Verfahrensrechner des Kuratorium für Technik und Bauwesen in der Landwirtschaft [Online calculator of Association for Technology and Structures in Agriculture] [WWW Document]. URL https://daten.ktbl.de/vrpflanze/home.action (Accessed 18 11, 20).
Lamb, A., Green, R., Bateman, I., Broadmeadow, M., Bruce, T., Burney, J., et al. (2016). The Potential for Land Sparing to Offset Greenhouse Gas Emissions from Agriculture. Nat. Clim Change 6, 488–492. doi:10.1038/nclimate2910
Landis, D. A. (2017). Designing Agricultural Landscapes for Biodiversity-Based Ecosystem Services. Basic Appl. Ecol. 18, 1–12. doi:10.1016/j.baae.2016.07.005
Law, E. A., and Wilson, K. A. (2015). Providing Context for the Land-Sharing and Land-Sparing Debate. Conservation Lett. 8, 404–413. doi:10.1111/conl.12168
Lobell, D. B., Cassman, K. G., and Field, C. B. (2009). Crop Yield Gaps: Their Importance, Magnitudes, and Causes. Annu. Rev. Environ. Resour. 34, 179–204. doi:10.1146/annurev.environ.041008.093740
Magda, D., de Sainte Marie, C., Plantureux, S., Agreil, C., Amiaud, B., Mestelan, P., et al. (2015). Integrating Agricultural and Ecological Goals into the Management of Species-Rich Grasslands: Learning from the Flowering Meadows Competition in France. Environ. Manage. 56, 1053–1064. doi:10.1007/s00267-015-0553-6
Marinoni, O., Navarro Garcia, J., Marvanek, S., Prestwidge, D., Clifford, D., and Laredo, L. A. (2012). Development of a System to Produce Maps of Agricultural Profit on a continental Scale: An Example for Australia. Agric. Syst. 105, 33–45. doi:10.1016/j.agsy.2011.09.002
Matzdorf, B., and Lorenz, J. (2010). How Cost-Effective Are Result-Oriented Agri-Environmental Measures?-An Empirical Analysis in Germany. Land Use Policy 27, 535–544. doi:10.1016/j.landusepol.2009.07.011
Mehrabi, Z., Ellis, E. C., and Ramankutty, N. (2018). The challenge of Feeding the World while Conserving Half the Planet. Nat. Sustain. 1, 409–412. doi:10.1038/s41893-018-0119-8
Merckx, T., and Pereira, H. M. (2015). Reshaping Agri-Environmental Subsidies: From Marginal Farming to Large-Scale Rewilding. Basic Appl. Ecol. 16, 95–103. doi:10.1016/j.baae.2014.12.003
Mittal, S., and Mehar, M. (2016). Socio-economic Factors Affecting Adoption of Modern Information and Communication Technology by Farmers in India: Analysis Using Multivariate Probit Model. The J. Agric. Edu. Extension 22, 199–212. doi:10.1080/1389224X.2014.997255
Mottaleb, K. A., Krupnik, T. J., and Erenstein, O. (2016). Factors Associated with Small-Scale Agricultural Machinery Adoption in Bangladesh: Census Findings. J. Rural Stud. 46, 155–168. doi:10.1016/j.jrurstud.2016.06.012
Nelson, G. C., Valin, H., Sands, R. D., Havlík, P., Ahammad, H., Deryng, D., et al. (2014). Climate Change Effects on Agriculture: Economic Responses to Biophysical Shocks. Proc. Natl. Acad. Sci. USA 111, 3274–3279. doi:10.1073/pnas.1222465110
OECD (2018). Best Available Techniques (BAT) for Reventing and Controlling Industrial Pollution, Activity 2: Approaches to Establishing Best Available Techniques around the World, Environment, Health and Safety, Louvain-la-Neuve: Environment Directorate, OECD.
OECD, Eurostat (2012). Eurostat-OECD Methodological Manual on Purchasing Power Parities. 2012 Edition.
Pannell, D. J., Llewellyn, R. S., and Corbeels, M. (2014). The Farm-Level Economics of Conservation Agriculture for Resource-Poor Farmers. Agric. Ecosyst. Environ. 187, 52–64. doi:10.1016/j.agee.2013.10.014
Phalan, B., Green, R. E., Dicks, L. V., Dotta, G., Feniuk, C., Lamb, A., et al. (2016). How Can Higher-Yield Farming Help to Spare Nature? Science 351, 450–451. doi:10.1126/science.aad0055
Phalan, B. (2018). What Have We Learned from the Land Sparing-Sharing Model? Sustainability 10, 1760. doi:10.3390/su10061760
Piñeiro, V., Arias, J., Dürr, J., Elverdin, P., Ibáñez, A. M., Kinengyere, A., et al. (2020). A Scoping Review on Incentives for Adoption of Sustainable Agricultural Practices and Their Outcomes. Nat. Sustain. 3, 809–820. doi:10.1038/s41893-020-00617-y
R Core Team (2014). R: The R Project for Statistical Computing. Vienna, Austria: R Foundation for Statistical Computing. [WWW Document]. URL https://www.r-project.org/ (Accessed 18 11, 20).
Ramankutty, N., Mehrabi, Z., Waha, K., Jarvis, L., Kremen, C., Herrero, M., et al. (2018). Trends in Global Agricultural Land Use: Implications for Environmental Health and Food Security. Annu. Rev. Plant Biol. 69, 789–815. doi:10.1146/annurev-arplant-042817-040256
Reed, M. S., Moxey, A., Prager, K., Hanley, N., Skates, J., Bonn, A., et al. (2014). Improving the Link between Payments and the Provision of Ecosystem Services in Agri-Environment Schemes. Ecosystem Serv. 9, 44–53. doi:10.1016/j.ecoser.2014.06.008
Rey Benayas, J. M., and Bullock, J. M. (2012). Restoration of Biodiversity and Ecosystem Services on Agricultural Land. Ecosystems 15, 883–899. doi:10.1007/s10021-012-9552-0
Ritzema, R. S., Frelat, R., Douxchamps, S., Silvestri, S., Rufino, M. C., Herrero, M., et al. (2017). Is Production Intensification Likely to Make Farm Households Food-Adequate? A Simple Food Availability Analysis across Smallholder Farming Systems from East and West Africa. Food Sec. 9, 115–131. doi:10.1007/s12571-016-0638-y
Runting, R. K., Ruslandi, R., Griscom, B. W., Griscom, M. J., Satar, M., Meijaard, E., et al. (2019). Larger Gains from Improved Management over Sparing-Sharing for Tropical Forests. Nat. Sustain. 2, 53–61. doi:10.1038/s41893-018-0203-0
Russi, D., Margue, H., Oppermann, R., and Keenleyside, C. (2016). Result-based Agri-Environment Measures: Market-Based Instruments, Incentives or Rewards? the Case of Baden-Württemberg. Land Use Policy 54, 69–77. doi:10.1016/j.landusepol.2016.01.012
Salles, J.-M., Teillard, F., Tichit, M., and Zanella, M. (2017). Land Sparing versus Land Sharing: an Economist's Perspective. Reg. Environ. Change 17, 1455–1465. doi:10.1007/s10113-017-1142-4
Savary, S., Ficke, A., Aubertot, J.-N., and Hollier, C. (2012). Crop Losses Due to Diseases and Their Implications for Global Food Production Losses and Food Security. Food Sec. 4, 519–537. doi:10.1007/s12571-012-0200-5
Schimmelpfennig, D. (2016). Farm Profits and Adoption of Precision Agriculture. U.S. Department of Agriculture, Economic Research Service. doi:10.22004/ag.econ.249773
Schleicher, J., Zaehringer, J. G., Fastré, C., Vira, B., Visconti, P., and Sandbrook, C. (2019). Protecting Half of the Planet Could Directly Affect over One Billion People. Nat. Sustain. 2, 1094–1096. doi:10.1038/s41893-019-0423-y
Schulte, L. A., Niemi, J., Helmers, M. J., Liebman, M., Arbuckle, J. G., James, D. E., et al. (2017). Prairie Strips Improve Biodiversity and the Delivery of Multiple Ecosystem Services from Corn-Soybean Croplands. Proc. Natl. Acad. Sci. USA 114, 11247–11252. doi:10.1073/pnas.1620229114
Seekell, D., Carr, J., Dell’Angelo, J., D’Odorico, P., Fader, M., Gephart, J., et al. (2017). Resilience in the Global Food System. Environ. Res. Lett. 12, 025010. doi:10.1088/1748-9326/aa5730
Seppelt, R., Beckmann, M., Ceauşu, S., Cord, A. F., Gerstner, K., Gurevitch, J., et al. (2016). Harmonizing Biodiversity Conservation and Productivity in the Context of Increasing Demands on Landscapes. BioScience 66, 890–896. doi:10.1093/biosci/biw004
Steffen, W., Richardson, K., Rockström, J., Cornell, S. E., Fetzer, I., Bennett, E. M., et al. (2015). Planetary Boundaries: Guiding Human Development on a Changing Planet. Science 347, 1259855. doi:10.1126/science.1259855
Tanentzap, A. J., Lamb, A., Walker, S., and Farmer, A. (2015). Resolving Conflicts between Agriculture and the Natural Environment. PLOS Biol. 13, e1002242. doi:10.1371/journal.pbio.1002242
Thornton, P. K., Kristjanson, P., Förch, W., Barahona, C., Cramer, L., and Pradhan, S. (2018). Is Agricultural Adaptation to Global Change in Lower-Income Countries on Track to Meet the Future Food Production challenge? Glob. Environ. Change 52, 37–48. doi:10.1016/j.gloenvcha.2018.06.003
Tittonell, P., and Giller, K. E. (2013). When Yield Gaps Are Poverty Traps: The Paradigm of Ecological Intensification in African Smallholder Agriculture. Field Crops Res. 143, 76–90. doi:10.1016/j.fcr.2012.10.007
Tscharntke, T., Clough, Y., Wanger, T. C., Jackson, L., Motzke, I., Perfecto, I., et al. (2012). Global Food Security, Biodiversity Conservation and the Future of Agricultural Intensification. Biol. Conservation 151, 53–59. doi:10.1016/j.biocon.2012.01.068
Tumushabe, J. T. (2018). “Climate Change, Food Security and Sustainable Development in Africa,” in The Palgrave Handbook of African Politics, Governance and Development. Editors S.O. Oloruntoba, and T. Falola (New York: Palgrave Macmillan US), 853–868. doi:10.1057/978-1-349-95232-8_53
UN (2015). Transforming Our World: The 2030 Agenda for Sustainable Development. New York: United Nations, Department of Economic and Social Affairs.
United Nations (2017). World Population Prospects: The 2017 Revision, Key Findings and advance Tables (No. ESA/P/WP/248). New York: United Nations.
Validi, S., Bhattacharya, A., and Byrne, P. J. (2014). A Case Analysis of a Sustainable Food Supply Chain Distribution System-A Multi-Objective Approach. Int. J. Prod. Econ. 152, 71–87. doi:10.1016/j.ijpe.2014.02.003
Wickham, H., François, R., Henry, L., Müller, K., and RStudio, (2020). Dplyr: A Grammar of Data Manipulation.
Williams, J. R., Jones, C. A., Kiniry, J. R., and Spanel, D. A. (1989). The EPIC Crop Growth Model. Trans. ASAE 32, 497–0511.
World Bank (2019a). World Development Indicators Database. PPP conversion factor, GDP (LCU per international $) [WWW Document]. World Bank. URL https://data.worldbank.org/indicator/PA.NUS.PPP (Accessed 18 11, 20).
World Bank (2019b). World Development Indicators Database. Inflation, consumer prices [WWW Document]. World Bank. URL https://data.worldbank.org/indicator/FP.CPI.TOTL.ZG (Accessed 11, 28. 19).
Young, J. F., and Skrivergaard, S. (2020). Cultured Meat on a Plant-Based Frame. Nat. Food 1, 195. doi:10.1038/s43016-020-0053-6
Zabel, F., Delzeit, R., Schneider, J. M., Seppelt, R., Mauser, W., and Václavík, T. (2019). Global Impacts of Future Cropland Expansion and Intensification on Agricultural Markets and Biodiversity. Nat. Commun. 10, 2844. doi:10.1038/s41467-019-10775-z
Keywords: cropland expansion, food security, yield gaps, land sparing, food supply, global economic assessment, optimised land use, cost engineering framework
Citation: Vittis Y, Folberth C, Bundle S-C and Obersteiner M (2021) Restoring Nature at Lower Food Production Costs. Front. Environ. Sci. 9:672663. doi: 10.3389/fenvs.2021.672663
Received: 26 February 2021; Accepted: 07 May 2021;
Published: 25 May 2021.
Edited by:
Ranjan Kumar Ghosh, Indian Institute of Management Ahmedabad, IndiaReviewed by:
Xu Zhao, Shandong University, Weihai, ChinaMuntasir Murshed, North South University, Bangladesh
Javier Navarro, Commonwealth Scientific and Industrial Research Organisation (CSIRO), Australia
Copyright © 2021 Vittis, Folberth, Bundle and Obersteiner. This is an open-access article distributed under the terms of the Creative Commons Attribution License (CC BY). The use, distribution or reproduction in other forums is permitted, provided the original author(s) and the copyright owner(s) are credited and that the original publication in this journal is cited, in accordance with accepted academic practice. No use, distribution or reproduction is permitted which does not comply with these terms.
*Correspondence: Yiorgos Vittis, yiorgos.vittis@ouce.ox.ac.uk