- 1Department of Earth Sciences, University of Gothenburg, Gothenburg, Sweden
- 2Department of Geography, McGill University, Montreal, QC, Canada
- 3Department of Land and Water Resources Engineering, Royal Institute of Technology (KTH), Stockholm, Sweden
- 4Finnish Meteorological Institute, Helsinki, Finland
- 5INAR Institute for Atmospheric and Earth System Research/Physics, Faculty of Science, University of Helsinki, Helsinki, Finland
- 6Department of Forest Sciences, University of Helsinki, Helsinki, Finland
Nutrient-rich peat soils have previously been demonstrated to lose carbon despite higher photosynthesis and litter production compared to nutrient-poor soils, where instead carbon accumulates. To understand this phenomenon, we used a process-oriented model (CoupModel) calibrated on data from two closely located drained peat soil sites in boreal forests in Finland, Kalevansuo and Lettosuo, with different soil C/N ratios. Uncertainty-based calibrations were made using eddy-covariance data (hourly values of net ecosystem exchange) and tree growth data. The model design used two forest scenarios on drained peat soil, one nutrient-poor with dense moss cover and another with lower soil C/N ratio with sparse moss cover. Three vegetation layers were assumed: conifer trees, other vascular plants, and a bottom layer with mosses. Adding a moss layer was a new approach, because moss has a modified physiology compared to vascular plants. The soil was described by three separate soil organic carbon (SOC) pools consisting of vascular plants and moss litter origin and decomposed organic matter. Over 10 years, the model demonstrated a similar photosynthesis rate for the two scenarios, 903 and 1,034 g C m−2 yr−1, for the poor and rich site respectively, despite the different vegetation distribution. For the nutrient-rich scenario more of the photosynthesis produce accumulated as plant biomass due to more trees, while the poor site had abundant moss biomass which did not increase living aboveground biomass to the same degree. Instead, the poor site showed higher litter inputs, which compared with litter from vascular plants had low turnover rates. The model calibration showed that decomposition rate coefficients for the three SOC pools were similar for the two scenarios, but the high quantity of moss litter input with low decomposability for the nutrient poor scenario explained the major difference in the soil carbon balance. Vascular plant litter declined with time, while SOC pools originating from mosses accumulated with time. Large differences between the scenarios were obtained during dry spells where soil heterotrophic respiration doubled for the nutrient-rich scenario, where vascular plants dominated, owing to a larger water depletion by roots. Where moss vegetation dominated, the heterotrophic respiration increased by only 50% during this dry period. We suggest moss vegetation is key for carbon accumulation in the poor soil, adding large litter quantities with a resistant quality and less water depletion than vascular plants during dry conditions.
Introduction
Worldwide, natural peatlands and other organic soils cover only 3% of the land area but contain 30% of the soil carbon (Gorham, 1991; FAO, 2014). The peat have accumulated after the last glaciation period where the northern peatlands carbon stock have been estimated to contain 500 ± 100 Gtonnes C (Yu, 2012). The peat soil is formed by a litter production slightly larger than its decomposition (Frolking et al., 2001), and many factors influence the balance of soil accumulation and decomposition, mainly climate and water conditions, alongside soil fertility forming the plant community (Waddington and Roulet, 1996; Alm et al., 1997; Laiho et al., 2003). Climate warming and soil drain operations dry out the peat causing decomposition and large increase in greenhouse gas emissions, why there is an urgent need to manage the peatlands in a way that preserve the carbon (Huang et al., 2021), where the main tool is to raise the soil water table (Evans et al., 2021). The photosynthesis is an important part of the carbon budget, where mosses and lichens should not to be neglected besides vascular plants in model studies of cool climate and nutrient limiting conditions (Chadburn et al., 2017). When restoring disturbed peatlands, mosses have been shown to be important to regain carbon accumulation, thus peat mosses need to be preserved or introduced by moss fragments (Huth et al., 2021).
Rewetting is expected to result in reduced CO2 emission but increased methane (CH4) emission, where climate warming by CO2 have been shown the most important to mitigate (Günther et al., 2020). However, the resulting soil carbon (C) balance is not easy to predict owing to the complexity of soil processes and influencing factors, where systems that look similar at first have been demonstrated to act differently, as either a large carbon dioxide (CO2) source or a small sink (Ojanen et al., 2013). High losses have been displayed for fertile peat soil ecosystems, for example in Skogaryd Sweden, where the combined Net Ecosystem Exchange (NEE) measured by Eddy Covariance (EC), and measured forest growth, revealed a net carbon soil loss of 630 g C m−2 yr−1 despite high spruce tree growth of 830 g C m−2 yr−1 including fine roots (Meyer et al., 2013). Similarly, the loss from a fertile peatland forest soil in southern Finland averaged 200 g C m−2 yr−1 (Korkiakoski, 2020), whereas a nutrient-poor peatland forest soil nearby was a sink of 60 g C m−2 yr−1 (Minkkinen et al., 2018). Other poor peat soil sites in Finland were also revealed to be small CO2 sinks, while fertile peat soils were sources (Ojanen et al., 2013; Ojanen and Minkkinen, 2019). Since fertile peat soil sites have larger plant production than poor soil sites, it is counter-intuitive that the poor sites accumulate C rather than the fertile sites. The higher emissions from fertile soils have earlier been hypothesized to be caused by more nutrients for plants and decomposers or a higher topsoil bulk density in fertile soils where more carbon are available for decomposition (Minkkinen and Laine, 1998; Ojanen et al., 2013). Besides the soil properties, the plant composition and function have also been demonstrated to have influence on the soil water table where a forest vegetation keeps the soil drained even in wet years (Leppä et al., 2020), with more oxygen in the soil increasing soil processes. Plant composition is important for how the photosynthesis fixed C is stored, as a living biomass or litter. Vascular plants, having roots, adds litter both on top of the soil and as root litter, while mosses only adds litter in the top soil below the living moss. Nutrient-poor conditions favor mosses over vascular plants, which could be fundamental for the ecosystem function (Van Breemen, 1995; Pedrotti et al., 2014). In fertile sites with deciduous trees like birch, moss growth could be hindered by a thick leaf litter layer (Laine and Vanhamajamaa, 1992), preventing the formation of a new peat layer.
It is a need to investigate why nutrient-poor and rich peat soils show different soil decomposition rate. By combining field NEE data with process-based models, linking ecological theory and data, we can examine and identify the processes most important for gain and losses of carbon. An earlier model study suggested the quantity of litter input from vascular and nonvascular plants, together with decomposability and input location, at the soil surface or deeper layers by roots, to be decisive for peat accumulation (Frolking et al., 2001). Litter input results in a mixture of different organic substances in continuous transition into decomposed organic materials, simplified by assuming all organic matter as a litter decomposing by time (Frolking et al., 2001; St-Hilaire et al., 2010). The soil organic carbon (SOC) have also been separated into two organic matter pools, one with a fast decomposition which C partly ends up into a slow pool, with rates overall faster for nutrient-rich peat soils than poor soils (Metzger et al., 2015).
In this investigation we use the Coupled Heat and Mass Transfer Model for Soil–Plant-Atmosphere Systems (CoupModel, www.coupmodel.com), which been used previously for peat soil greenhouse gas flux investigations (Metzger et al., 2015; He et al., 2016a; Metzger et al., 2016; Kasimir et al., 2018) and is designed to include a wide range of ecosystems and soils. The model was first presented by (Jansson and Halldin, 1979) for forest soils and later developed for agricultural soils by (Johnsson et al., 1987). A recent development of the model have been to include phosphorous (He et al., 2021). Model development and applications is provided in (Jansson, 2012). The model can provide simplified views and conceptualize our understanding of the system function. However, the degree of simplification and conceptualization of the system is a challenge. In earlier use of the model, peatland vegetation has been modeled either as one simplified explicit big leaf, including grass and mosses (Metzger et al., 2015; Metzger et al., 2016) or by assuming two canopies with two “simple big leaves”, one for the trees and another for smaller vascular plants like grass (He et al., 2016a; He et al., 2016b). The specific role of mosses has not been the focus (Metzger et al., 2016), although it has been described and included as part of the system when calibrating the model. In this study, we explore the importance of mosses and their influence on the ecosystem by explicitly modeling their particular morphology and physiology and compare two systems having either large or small quantities of moss cover. We also in the CoupModel separate the litter pool into two pools, generated by vascular and moss plants. We based the present study on empirical studies that have demonstrated vascular leaf litter to decompose faster than moss litter (Dorrepaal et al., 2005; Lang et al., 2009; Strakova et al., 2011). The approach used these new concepts describing the systems to calibrate the CoupModel on measured data from two forested drained peatlands, having contrasting soil fertility (Supplementary Table S1).
We aim to answer the question: how a sparser tree canopy with a more developed moss layer and low soil fertility can result in a higher soil carbon sequestration than a forest with a dense tree canopy and a sparse moss layer on fertile peat soil?
The specific purposes of the study were:
1) To present a new model explicitly including moss litter production and decomposition.
2) To develop a common model set-up and design valid for both nutrient-rich and nutrient-poor peatland forest soils.
3) To discuss the role of vascular plants versus mosses on C soil balance.
Materials and Methods
Site Description
We used data from two forest sites located 80 km northwest of Helsinki, and approximately 20 km from each other in Southern Finland, Kalevansuo and Lettosuo, to set up the model. Both sites are on drained peat soil but have contrasting soil fertility expressed as C/N, where the nutrient-poor site, Kalevansuo, (60°38′ N, 24°21′ E, elevation 123 m a.s.l.), have C/N = 40 in the top 50 cm soil, and the nutrient-rich site, Lettosuo (60°38′ N, 23°57′ E; 111 m a.s.l), have C/N = 27. Both sites have the same long term annual mean precipitation of 722 mm and annual mean air temperature of 5°C. The first draining took place during the 1930s by manually dug, widely spaced ditches. Then, Lettosuo in 1969 and Kalevansuo in 1971 were drained by 1 m deep ditches spaced 45 m apart and fertilized with phosphorus and potassium. No cleaning of ditches has taken place at Kalevansuo; conversely, various ditches were cleaned at Lettosuo during the 1990s. Ditches at both sites are still functional but are now partly overgrown with Sphagnum mosses. At Kalevansuo, the trees have grown bigger, and mire species coverage has decreased while common forest species have taken over in the field and bottom layers. Scots pine (Pinus sylvestris), on average 120 years old in 2008, dominates the tree stand (forming 98% of the stand volume), and small pubescent birch (Betula pubescent) trees grow alongside ditches (Lohila et al., 2011). The basal area of the tree stand is 18.4 m2 ha−1, with a tree stand C stock of 4,600 g C m−2, and tree stand C sequestration of 170 g C m−2 yr−1 (above and below ground, excluding roots <1 cm) (Minkkinen et al., 2018). The field layer is dominated by Ledum palustre, Vaccinium uliginosum, and others (Supplementary Table S1). The bottom layer covers approximately 90% of the land surface and is dominated by forest mosses with several Sphagnum mosses on the wetter spots. At Lettosuo, Scots pine and pubescent birch dominated the canopy, with an understory of small-sized Norway spruce and pubescent birch. The trees’ total basal area was 27.5 m2 ha−1, with a tree stand C stock of 8,000 g C m−2, and a C sequestration rate of 270 g C m-2 years−1 (above and below ground, excluding roots <1 cm; unpublished data). Because the tree stand is larger than in Kalevansuo, the forest floor is more shaded and has patchy vegetation. The field layer consists of Dryopteris carthusiana and Vaccinium myrtillus. In the patchy bottom layer, forest mosses can be found alongside Sphagnum in moist patches (Bhuiyan et al., 2017).
The topsoil at Kalevansuo is Sphagnum-dominated peat with a mixture of Eriophorum vaginatum and shrub constituents, while fen peat (i.e., sedges and herbs) is present in the deep layers (Mathijssen et al., 2017). Remains of earlier forest fires (charcoal particles) can be found, especially at a depth of 30–50 cm. After drainage, the oxic peat layer to a depth of approximately 10–30 cm below the surface has decomposed to a high degree. In the topmost 10 cm of the soil, remnants of forest mosses and woody roots can be observed. The peat soil at Lettosuo is sedge-peat with a mixture of Sphagnum and wood, namely, typical peat for a treed fen. The peat in the topsoil is more decomposed than at Kalevansuo.
Data Used for the Current Study
The turbulent fluxes of CO2 (net ecosystem exchange NEE), water vapor (H2O), and sensible/latent heat were measured with the eddy covariance technique on top of 21.5-m telescopic masts. Supporting meteorological measurements included wind speed, relative humidity, incoming short-wave radiation, total net radiation, air and soil temperatures, precipitation, soil moisture, and water table (WT) depth. The eddy covariance system, footprint calculation, and flux data handling for Kalevansuo were described in detail by Lohila et al. (2011) and Minkkinen et al. (2018). For Kalevansuo, the data covers 2005–2008, and for Lettosuo, 2009–2012. The daily mean was estimated upon data measured hourly, with no gap-filling. When measured data had gaps, the simulation also had the same gaps to make sure that we had no bias because of the different temporal resolution. For the partition of NEE, into gross primary production (GPP) and ecosystem respiration (Reco), tree growth data was used. In this paper, we use the convention that a positive value of NEE indicates a flux from the ecosystem to the atmosphere, while a negative value means uptake of CO2 by the ecosystem.
The WT depth was continuously recorded close to the EC mast. In Kalevansuo, WT data were recorded daily from 2005 to 2008 and hourly from 2010 to 2016. In Lettosuo, hourly WT data were obtained from 2010 to 2016. Soil temperatures were recorded from four plots in 16 points with temperature loggers from the depths of 5 cm, 15 cm, and 30 cm below the soil surface at intervals of 30 min. Peat C stock was estimated based on average peat layer thickness (Lohila et al., 2011) and average carbon density in peat (Mathijssen et al., 2017). Tree diameter at breast height was measured in spring 2005 and fall 2008 for Kalevansuo, and in fall 2003 and 2008 for Lettosuo to estimate the biomass growth (Ojanen et al., 2012). Most of the publications cited here describe the measurements at Kalevansuo (Minkkinen et al., 2018), and the same methods were applied at Lettosuo, making data from both sites comparable.
Modeling Approach
The CoupModel is an ecosystem model platform designed to simulate water and heat fluxes alongside C and nitrogen (N) cycles in terrestrial soil-plant ecosystems based on well-established equations to represent all major processes and components (Jansson, 2012). The main model structure is a one-dimensional layered soil profile including plants, containing all main flows of water, heat, C, and N dynamics between the atmosphere, plants, and soil, based on detailed descriptions of soil and plant physical and biogeochemical processes. A general description of the model and how the components are linked to each other can be found in Jansson (2012); Metzger et al. (2015) and He et al. (2016a). The model main equations used in this study are described in the Supplementary Table S2.
Two systems named K and L were thus modeled based on Kalevansuo and Lettosuo data, describing a poor scenario with a large moss cover and a nutrient-rich scenario with low moss cover, respectively (Figure 1). In the model, vascular plants are represented by three components: root, stem, and leaf. Photosynthetic assimilates (mobile C and N pools) are transformed to the biomass of the three components based on tissue partitioning and nutrient demand and availability. Nutrient partitioning is defined with the highest priority for the roots, followed by the leaf, and finally, the remaining for the stem. Optimal C/N ratios are defined by parameters governing together with the C allocation the N demand from the soil. Thus, roots are a relatively large fraction of the normal growth for vascular plants. Vascular plants feed the soil with litter from above ground components to the soil surface and from root litter to different horizons. Vascular plants extract water and nutrients through roots, exponentially distributed by depth. In contrast, mosses have no roots, only the leaf and stem act as primary targets for the photosynthesis products. Here we introduce an explicit description of mosses, new for the model, where mosses are defined as plants without roots, a living component located partly inside the topsoil layer where uptake of water and nutrients occurs. When mosses grow, the old parts are covered by new parts from above, and the old parts of the moss die. Moss litter from the stem and leaf takes place as a continuous flux directly to a position inside the soil and not to the soil surface. For this application, we assumed that mosses die in the modeled soil layer 2, 5–15 cm soil depth. Moreover, N fixation was assumed for moss plants only.
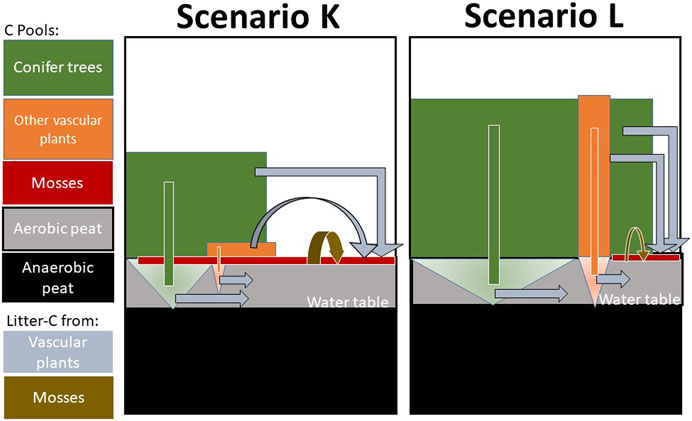
FIGURE 1. Overview of the two simulated systems K and L, where K is nutrient-poor. Vascular plants, Vegetation1 (conifers) dominate at L, both in height and coverage. Vegetation2 consists also of vascular plants, mainly of small shrubs but also tall birch trees at L. Mosses are incorporated in the soil surface and cover the soil at K but are sparse at L. Note that mosses have no roots and add litter only to the soil layer just below the mosses. The soil column is divided into 10 layers to 2.5 m depth, each with three soil organic carbon components with different decomposition rates. The surface soil is oxic, in average to a depth of 0.3 m, where most roots can be found.
Three vegetation layers were constructed for the model to represent the major differences in light, water, and N availability: Vegetation1 consisted of the coniferous tree canopy, Vegetation2 of deciduous trees of all sizes, and smaller evergreen vascular plants, and Vegetation3 the bottom layer with moss plants. The vegetation can be understood by the model as three big leaves allowing the competition of light, water, and nutrients. A big leaf can intercept light from a certain height down to the soil surface. The interception of light follows the exponential Beers law as a function of the Leaf Area Index (LAI) and a corresponding uniform distribution of leaf with height. The light extinction coefficients were assumed to be the same for the vascular plants, 0.5, but for the mosses, the coefficient of two was used, a number often used for field layer plants. The vegetation of each height segment share light, regulated both by the degree of cover and LAI. The LAI, maximal height, and degree of soil cover estimated for K and L scenarios based on the two sites Kalevansuo and Lettosuo (Supplementary Table S3). The model calculates photosynthesis to be proportional to the global radiation absorbed by the canopy; however, it is limited by unfavorable temperature, water conditions, and lack of N in the leaf. On average, 92% of the total incoming global radiation was intercepted by vegetation at K and 93% at L. The conifers (Vegetation1) of the poor scenario intercepted only 53% of the light, while 88% was intercepted at L. At K, the Vegetation2 plant type was composed mainly of dwarf shrubs, while at L deciduous trees dominated. The mosses (Vegetation3) differed most, intercepting 35% of the light at K but only 2% at L (Supplementary Table S3).
Vascular plants access water through roots in the uppermost 50 cm of the soil, with most roots in the surface 20 cm according to the measured root biomass data (Bhuiyan et al., 2017). Besides larger access to water, vascular plants are able to keep the cell water potential quite stable by stomata regulation. Conversely, mosses access only water in the soil surface and have no leaf stomata why they lose water by evaporation from the leaf surface, regulated by the resistance between the soil and atmosphere. Mosses are thus unable to control tissue water as vascular plants, which have stomata, why mosses need to cope periods of low tissue water content (Proctor et al., 2007). The model approach for moss water evaporation was a resistance based on leaf moisture only and no stomata control as used in vascular plants.
The model set-up assumes a soil structure with three soil organic matter components and 10 soil layers to describe the differences down to 2.5 m depth focusing on the uppermost 50 cm of the soil profile (Supplementary Table S4). The soil of the top 5 cm was assumed composed of both SOC and living mosses. The SOC component design was: two litter pools SOC1 and SOC2, originating from vascular plants and mosses, respectively. The third pool, SOC3, was produced from decomposed SOC1 and SOC2. The initial soil organic C of each layer was divided into SOC1, SOC2, and SOC3, assuming a C/N of 45 for SOC1, 65 for SOC2 (Kuhry and Vitt, 1996), and 20 for SOC3 (Svensson et al., 2008). The distribution of each layer of SOC1, SOC2, and SOC3 is presented in the Supplementary Table S4. This pool separation mainly aimed to link the soil processes to the plant functional roles. Soil physical properties are presented in Supplementary Table S5. All water was assumed to be added through precipitation only.
Decomposition was simulated as a first-order equation, controlled by the decomposition rate coefficient RateCoefSOC, combined with response functions for soil temperature and moisture around an optimal range with the assumption of zero decomposition at full saturation. The decrease from an optimal to zero decomposition rate was assumed to follow a simple expression accounting only for the air-filled pore space described by the parameters ThetaUpperRange, describing the percentage of air-filled soil pores when decomposition starts to slow down, together with ThetaPowerCoef describing unlinearity. These parameters demonstrate the range of air-filled pores where decomposition is negatively impacted by air-filled soil pores and the rate of decrease. In general, a higher value of ThetaUpperRange and ThetaPowerCoef means a smaller moisture response, thus a lower simulated soil decomposition. Temperature functions were not calibrated since they were assumed to be well described by scenario-independent parameters.
Calibration and Sensitivity
Measured data from the two sites represent two different periods in time and could therefore not be compared directly due to the between-year variability. However, the data could be used for calibration and extended to cover the same period and weather conditions. A common climate forcing dataset covering 10 years was used for calibration and simulation. We used measured characteristics as independent inputs and assumed that the vegetation cover characteristics and the soil properties displayed relatively slow changes during this period. In contrast, the EC flux data displays high variability within a day, between seasons, and between years depending on the specific climate variability. The approach was only to use measured, hourly quality checked data (Vesala et al., 2008) to find the best possible parameter representation of the model when fitting to the NEE data. The calibration protocol was using a Monte-Carlo based approach using a stepwise design of a selection of parameters, with appropriate uncertainty ranges, to make sure that we generated a high amount of more than 10,000 candidates that was used for selection of posterior parameter distributions.
To make sure we did not obtain a systematic bias between our two systems, especially in the dynamics of the WT, we used many parameters values based on previous applications of the model and to a common value for both systems (Supplementary Table S6), and we initially calibrated the model to represent good enough abiotic conditions and seasonal variability in WT, and atmospheric fluxes of latent and sensible heat flows. The first step of calibration used subjective criteria and literature values for most parameters and calibrated a few parameters (hydraulic conductivity function, the spacing between the ditches, and approximate drainage depths). The resulting model outputs were compared with measured WT dynamics and latent and sensible heat flux. After this, the model was constrained to represent the carbon flux dynamics. First, we tested using only NEE flux data but found it was necessary to include and fit the model with measured data on tree growth. In principle, we wanted to investigate all different parameters possibly influencing the dynamics of NEE in forest ecosystems. However, a parameter-rich model has many problems with possible overparameterizations and equifinality in the results. Calibrated parameters were thus selected to have an expected strong impact on the NEE fluxes. Initially, we tried to include details on the N cycle, to calibrate N mineralization, N uptake, internal N allocation within the plants, and N fixation. However, because of the many uncertainties, especially for within-year dynamics, we decided to keep only one parameter representing N of the leaf, N_PhotoFactor. This single parameter represents the N control on photosynthesis rate for fixed light adsorption of a vegetation layer, meaning the N supply needed to fit a reasonable photosynthesis level. To clarify, N processes are still included and modeled. Moreover, radiation efficiency, meaning the CO2 fixed per quantum light, was assumed to be similar for both sites and for each of the vegetation layers concerning plant function type, namely, vascular plants or mosses (Supplementary Table S6).
Another choice of simplification was that vascular plants of layer one and two were assumed to have a similar N control; thus vascular plants were assigned a common N_PhotoFactor, and moss plants a separate N_PhotoFactor. Transpiration/evaporation regulation was calibrated on the parameter CriticalThresholdDry, which describes at which soil water potential, expressed in cm water column (hekto Pascal), photosynthesis starts to be negatively impacted by drought. Again, we assumed the moss layer would have a different sensitivity compared with vascular plants. Acclimation of photosynthesis from winter conditions into spring is necessary for a forest in the boreal region, reflected by the parameter TF SUM Start, which was included in the calibration scheme, undifferentiated by vegetation type.
Decomposition of the three soil organic pools (SOC1, SOC2, and SOC3) was assumed controlled by the decomposition rate coefficient, RateCoefSOC, assigned an assumed fixed range of sensitivity with decreasing sensitivity from SOC1 to SOC3. The decomposition rate of vascular plant litter (SOC1) was assumed to be much higher than that of mosses (SOC2) (Hobbie et al., 2000). Moreover, decomposition is controlled by oxygen availability which decreases when the soil water content becomes high. This was included by the two parameters, ThetaUpperRange and ThetaPowerCoef.
In the calibration, each of the selected parameters was allowed to randomly vary within a range according to Table 1 and Supplementary Table S7. To avoid the noise from the high resolution of the 30 min measured flux data, hourly mean values were cumulated each year. A prior representation of the two sites was made by 15,000 simulations, out of which the best acceptable ensemble of 100 candidates was selected using several criteria (Supplementary Table S11A). The least-square criteria was applied since it focused on the seasonal dynamic of the carbon fluxes. It also reduced the bias between simulated and measured values to a low level. A full set of statistical performance indicators was estimated both on untransformed and on transformed cumulative data. Details of those are presented in the Supplementary Table S8.
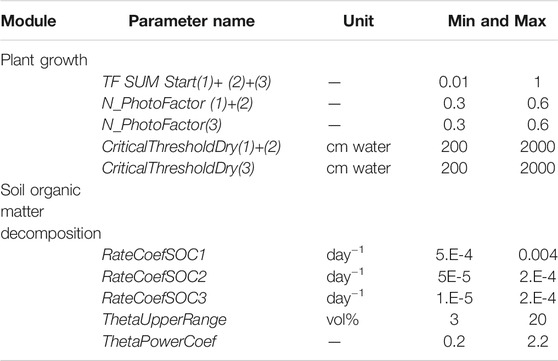
TABLE 1. Parameter setting, prior range. Plant growth parameters 1–3 refer to the three vegetation layers. SOC pool one and two refer to litter pools originating from vascular and moss plants respectively, and the decomposed organic matter SOC3.
Results
Calibrated Model Parameters and Performance
Vegetation
The calibrated rate coefficients controlling photosynthesis, N_PhotoFactor, revealed no major differences for vascular plants and mosses at K (Figure 2). The N_PhotoFactor could not be constrained for mosses at L owing to an exceedingly low proportion of this vegetation. Thus, the calibration resulted in similar and narrower parameter ranges compared to the prior min and max values of Table 1, for both K and L alongside the vegetation types. Besides light and N control on photosynthesis, plant water availability was also important as regulated by the parameter CriticalThresholdDry; although this displayed minor changes following calibration, vascular plants at K were somewhat more sensitive to dry conditions than at L. Mosses displayed the same sensitivity as vascular plants. The TF SUM Start, which describes the seasonality of photosynthesis, namely, the transition to full photosynthesis after the dormant winter conditions, resulted in a lower value for K but not for L. This low value suggested a need for a long start-up period for the plants at K.
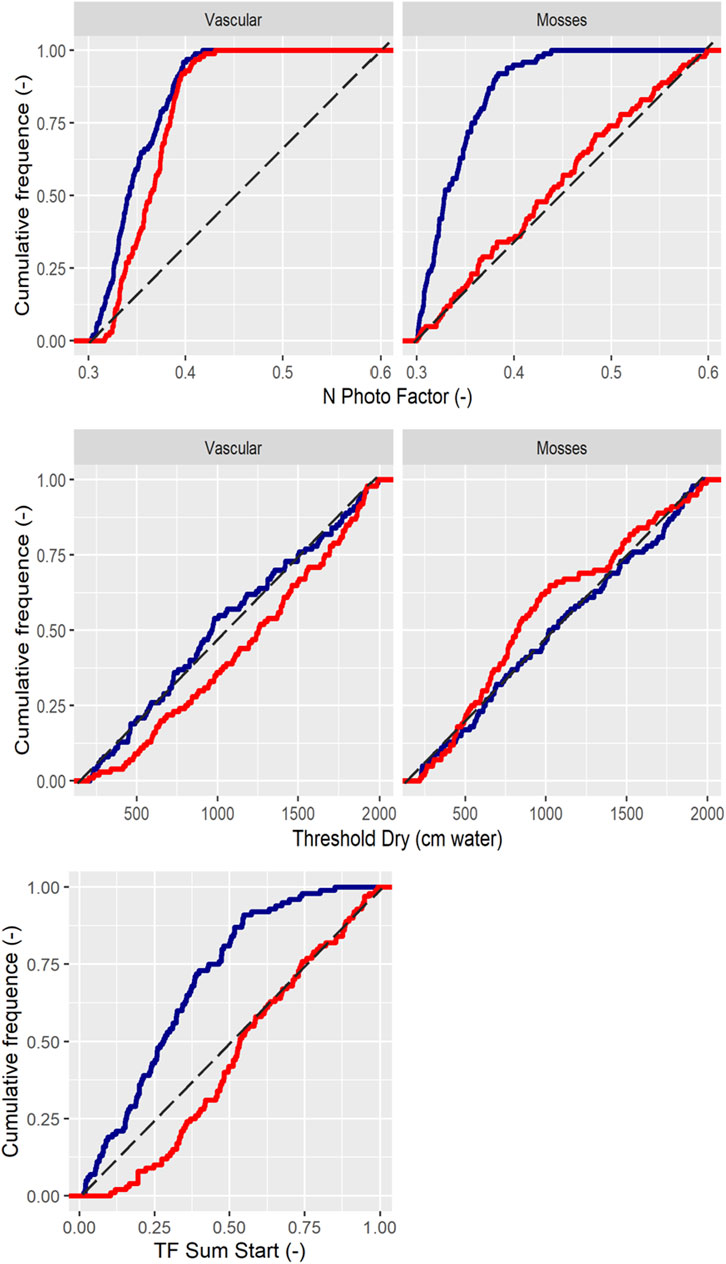
FIGURE 2. The cumulative frequency distribution of accepted parameter values associated with plant growth. K = blue and L = red.
Soil
Calibrated rate coefficients for SOC decomposition were similar for both systems, but all three RateCoefSOC were constrained to higher values than the prior values. The threshold for the lowest posterior values shifted to higher values, especially for the L scenario, however, comparing mean or median values indicated no differences. Both scenarios also displayed similar sensitivity to soil anaerobicity, ThetaUpperRange, alongside the nonlinearity of this parameter, ThetaPowerCoeff (Figure 3).
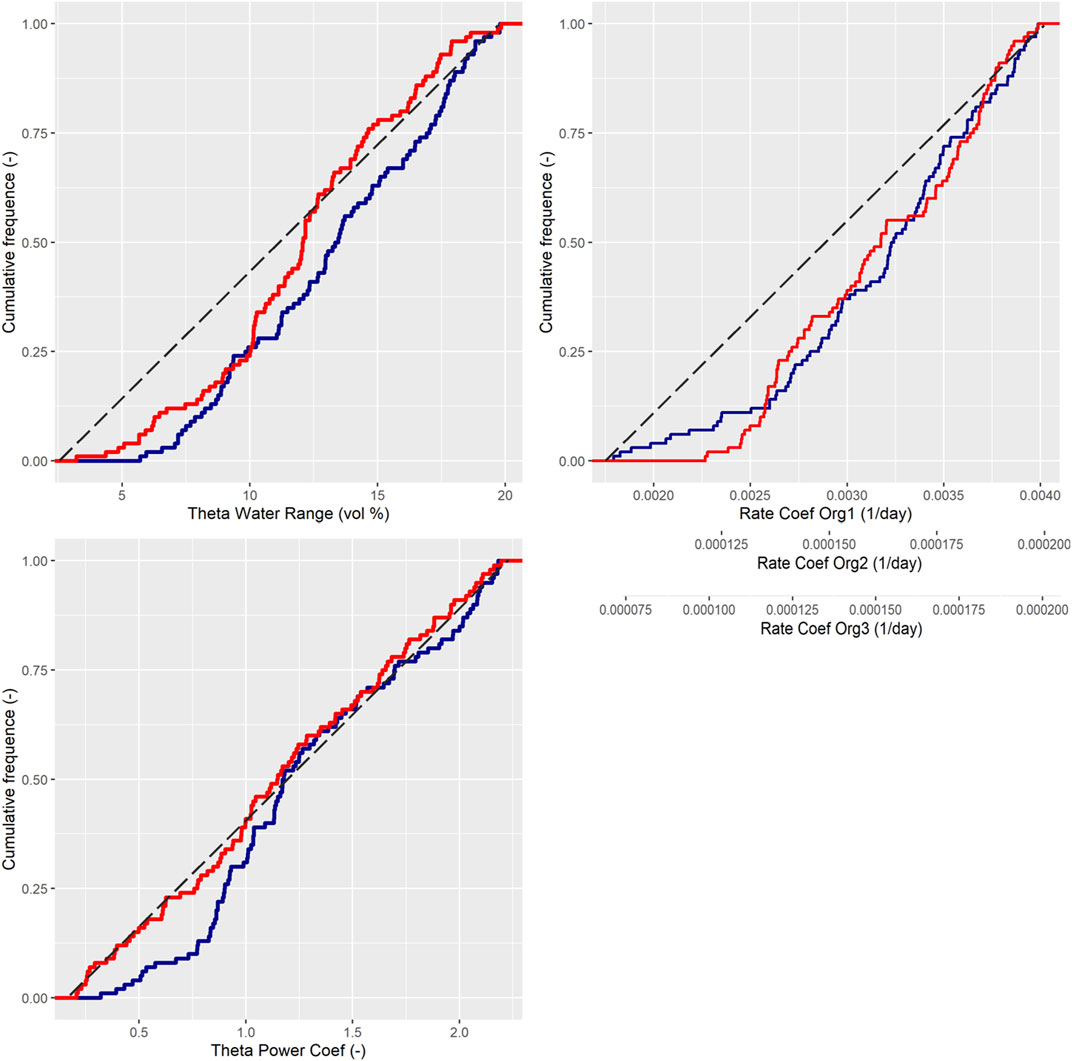
FIGURE 3. The cumulative frequency distribution of the accepted parameter values associated with soil processes. K = blue and L = red.
Overall, the calibration showed that the parameters for both scenarios were quite similar concerning photosynthesis and soil decomposition. The largest change compared to the prior setting was found for the N_PhotoFactor and TF SUM Start parameters.
Performance
The Kalevansuo measured NEE data was on average −0.74 g C m−2 day−1 between September 2004 and March 2009 (n = 28,862), whereas the mean of the K simulated ensemble was −0.77 g C m−2 day−1 with a simulated range from −0.88 to −0.53 g C m−2 day−1. Measurements at Lettosuo were conducted later, from September 2009 to December 2012, with an average NEE of −0.17 g C m−2 day−1 (n = 22,145), whereas the L simulated ensemble was -0.19 g C m−2 day−1 with a range from −0.21 to −0.08. In the growing season, both the modeled and measured NEE data fluctuated, with the model displaying a good performance overall (Figure 4). The simulated and measured winter NEE fluxes were small and demonstrated good agreement. One systematic deviation with overestimations of NEE appeared in the late summer and autumn of 2006 at K. Similar overestimation tendencies for NEE appeared in late summer during 2010 and 2011 at L. Measurements displayed high, meteorology-driven day-to-day variability at both sites, but this short-term variability was difficult for the model to fully capture. The scatter plot representation (Figure 5) of the daily mean values displays similar patterns for the two systems/sites, without systematic data noise patterns.
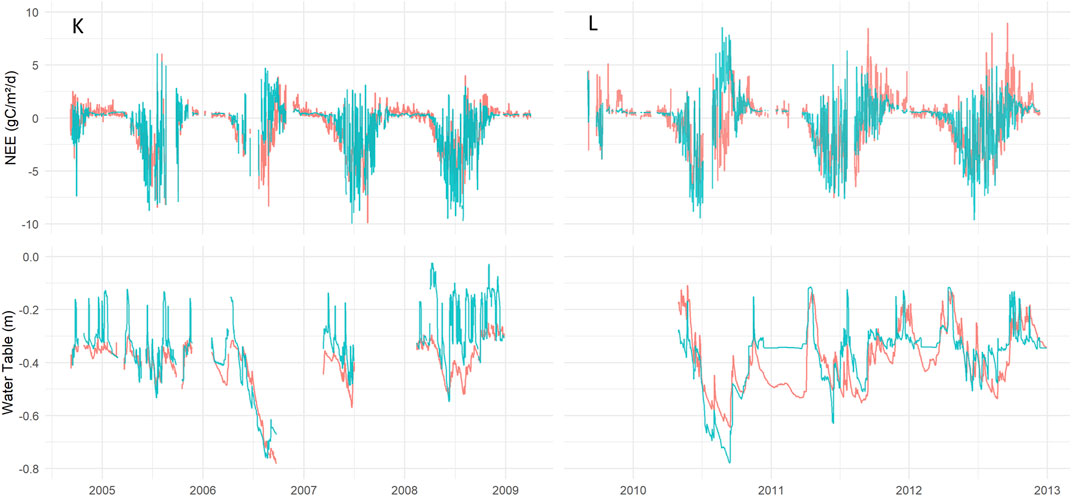
FIGURE 4. Daily measured and simulated average NEE, g C m−2 day−1 and WT, m, expressed over the measurement period. On the left measured Kalevansuo data (red) together with simulated K (blue-green). On the right the same for Lettosuo and L data.
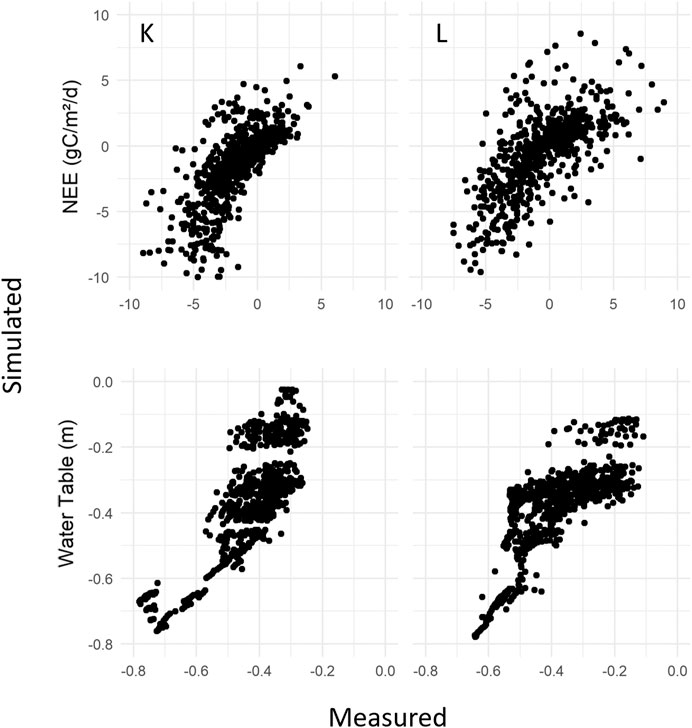
FIGURE 5. 1:1 plots of daily measured vs. simulated average NEE, g C m−2 day−1 and WT, m. On the left measured Kalevansuo data together with simulated K. On the right Lettosuo and L data.
The time series of simulated WT for K demonstrates frequent occasions with a higher (wetter) water saturation level than the measured data. Besides this, the dry summer of 2006 was revealed to be a key period for K, where modeled WT drop followed measured Kalevansuo data. Unfortunately, WT measurements were not done deep enough to allow a comparison for the deeper levels. The model was able to reflect the normal seasonal variability and between-year variability. No deviating systematic patterns could be seen between measured and simulated WT for the two systems/sites. The mean measured and simulated WT levels were similar (Figure 5). However, one similar systematic pattern was apparent for shallow WT. The simulated values were lacking representation of values around −0.2 m depth, which was similar for both systems. This reflects a problem with a continuous representation of the WT when a discrete representation of the compartment is used in the numerical representation of the model. Additionally, the model demonstrated higher WT in 2008 for the K site and 2011 for the L site (Figure 4).
Modeled 10-years Balance
The modeled 10-years balance of NEE demonstrated the nutrient-poor K to be a sink, −232 g C m−2 yr−1, and L to be a source, +7 g C m−2 yr−1. On many occasions, both K and L had the same NEE, but the L scenario also had many occasions with overall C-loss when K demonstrated uptake (Figure 6). In early summer, net uptake dominated for both K and L, while in late summer and autumn, many days with losses occurred, most pronounced for the fertile L which displayed the largest flux variability. During winter, NEE was small overall with low variability for both.
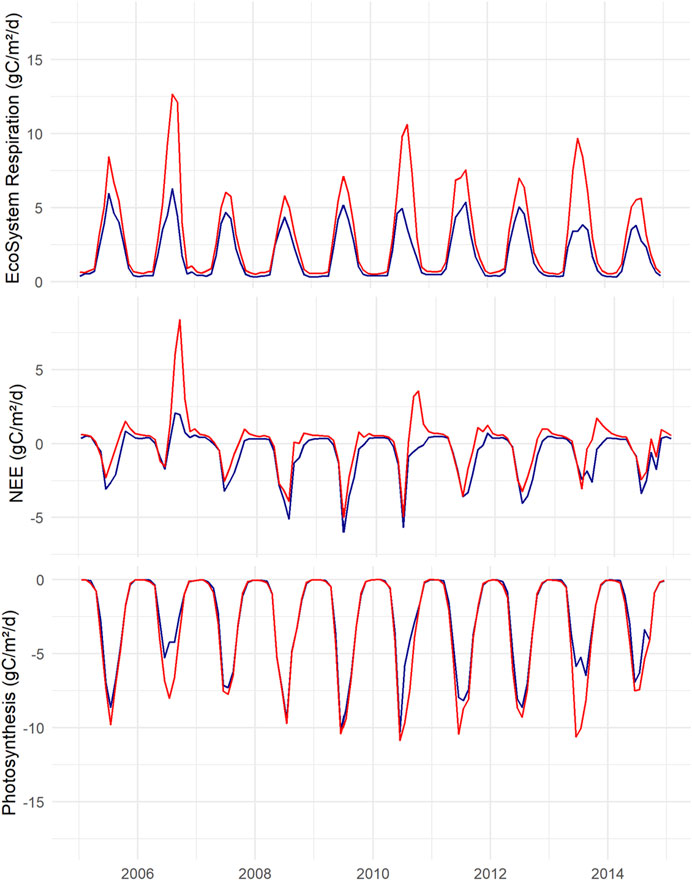
FIGURE 6. Top, modelled ecosystem respiration (Reco) for K (blue) and L (red). Middle, net ecosystem exchange (NEE). Bottom, gross primary production (GPP). Negative numbers show uptake, monthly averages expressed as g C m−2 day−1.
Net ecosystem exchange is composed of two large terms, photosynthesis and ecosystem respiration (Reco), where Supplementary Table S9-a presents selected carbon flux outputs with uncertainty range of the two systems. Neither can be measured directly but it is possible to disentangle them from various models. The seasonal pattern of GPP follows that of light and temperature, being large in summer and almost zero in winter (Figure 6). On most occasions, both systems had quite similar GPP for the total vegetation. The average GPP was −920 g C m−2 yr−1 for the K scenario, slightly lower than the L-scenario, −1,060 g C m−2 yr−1. The largest difference between the scenarios was the Vegetation3, mosses, which were responsible for a large part of the GPP at K while the moss GPP was barely visible for L. However, at L, Vegetation2 (tall birch trees and vascular plants in the field layer) displayed a certain importance while it was very small at K. The carbon fixed in the GPP is either lost in autotrophic respiration or stored in living and dead biomass (litter). The average litter production was the largest for K, 474 g C m−2 yr−1 compared to 330 g C m−2 yr−1 at L, despite a smaller GPP at K. Of the total litter production, mosses contributed 54% at K while only 7% at L. This is fundamental for the next term, Reco, since litter quality differs between vascular plants and mosses. The Reco over the 10 years on average was 688 for K and 1,068 g C m−2 yr−1 for L. Every year the Reco was larger for L than for K, and this was most pronounced during dry years (Figure 6).
Over the 10 years, plant growth accumulated more C at L than at K (Figure 7). Owing to competition for light and nutrients, the vegetation layers two and three did not grow much during this time, which is also inherent in their life-form as shrubs and moss. Only the birches grew in Vegetation2 at L. Despite, or because of, the lack of biomass growth, mosses at K were almost as essential as the trees adding litter carbon to the system. More C accumulated as biomass at L than K, but for the soil accumulation, the opposite was found, with a loss for the L scenario and a gain for K (Figure 8). The modeled overall soil C-loss at L was from all three soil components. In K, net losses were from SOC1, while the C-pool increased for SOC2 and SOC3.
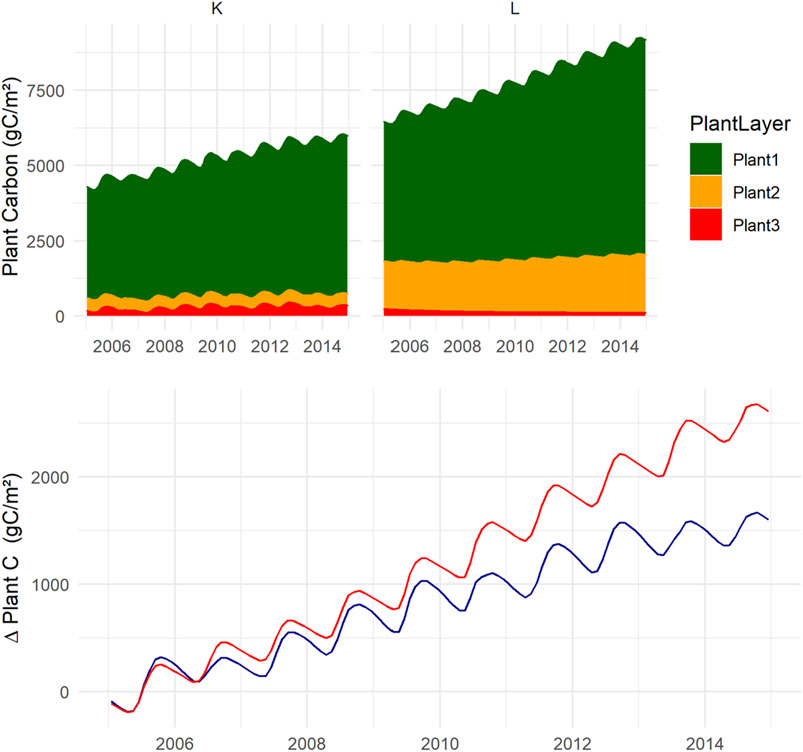
FIGURE 7. Plant carbon accumulation (positive numbers) over 10 years, top panel shows C accumulated in vegetation and its change over the years. Vegetation1 (conifer trees) = dark green, Vegetation2 (deciduous trees and field layer vascular plants) = yellow and Vegetation3 (mosses) = red. Bottom panel shows total plant C accumulation of the two scenarios where K = blue and L = red. Mean of 100 accepted model runs.
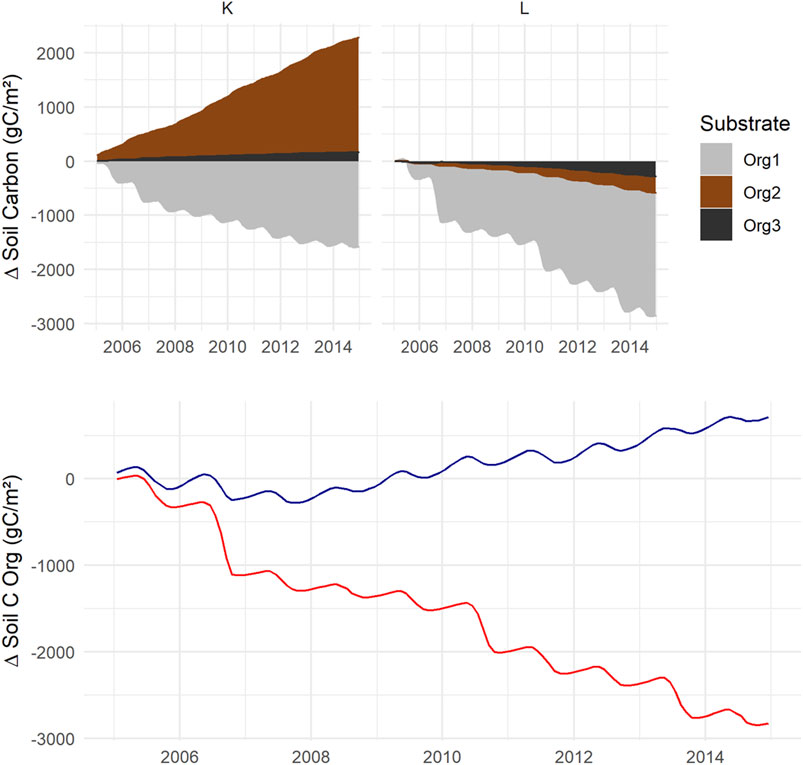
FIGURE 8. Simulated change in soil C pools where positive numbers shows the accumulation. Top panel shows the change in SOC1 = grey, SOC2 = brown and SOC3 = black. Bottom panel shows the total soil C accumulation for scenario K = blue and L = red. Mean of 100 accepted model runs (Supplementary Table S10-a).
All data on fluxes and carbon stock changes above were displayed as the average of the 100 accepted simulation runs. The accepted 100 model runs demonstrated a narrow distribution range for the C stock change of both for vegetation and soil and for K and L (Figure 9). No overlap for the scenarios was found, indicating a significant difference. While the L vegetation gained carbon, this did not compensate for the high soil C-loss For K, both the plants and the soil gained C for all the accepted simulations, where the soil accumulated C in the range from 0 to 130 g C m−2 yr−1 (Figure 9).
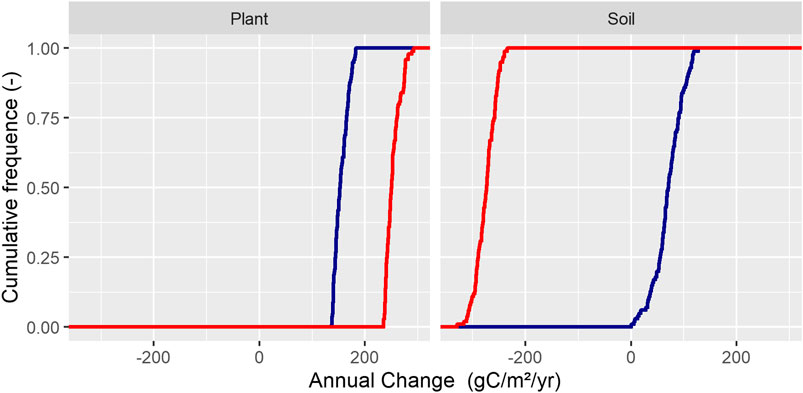
FIGURE 9. Variation within the 100 accepted model runs is shown as the frequency distribution of annual carbon change in vegetation and soil, for K (blue) and L (red). A positive number here shows accumulation.
Simulated Impact of Drought
During the 10 simulated years, three dry years occurred: 2006, 2010, and 2013. These explained most of the difference between K and L (Figures 6, 8). In 2007, the WT depth was approximately 0.3 m, similar for both sites, but in the dry year 2006, the WT drop was larger for L, reaching below 0.9 m, while for K, the WT dropped to 0.75 m (Figure 10). The model predicted that the especially dry year 2006 impacted L the most, with a NEE of +137 g C m−2 yr−1 for K and +599 g C m−2 yr−1 for L. In contrast, in a more “normal” year represented by 2007, the NEE demonstrated an overall uptake for both scenarios, −226 C m−2 yr−1 for K and −45 g C m−2 yr−1 for L.
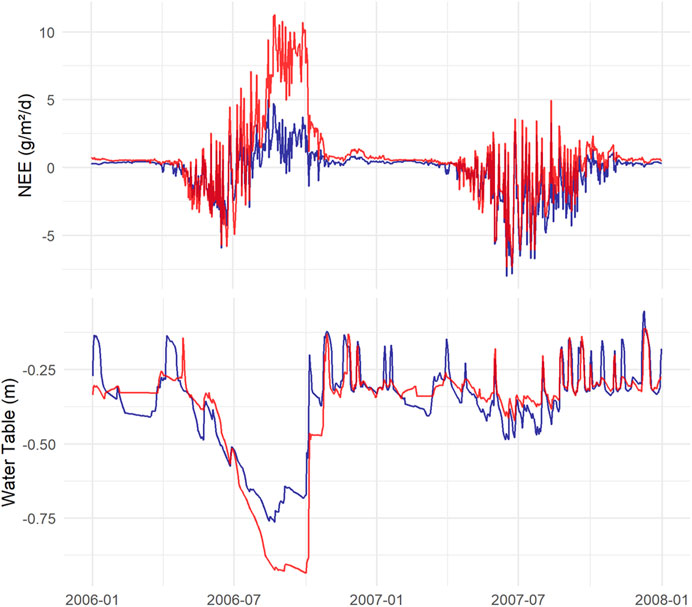
FIGURE 10. Simulated NEE, where a negative number shows uptake, and WT fluctuations during the dry year 2006 and the following more normal year 2007, for K (blue) and L (red).
The photosynthesis rate for the L-scenario was relatively unaffected despite dry conditions in 2006, while that for K photosynthesis became smaller (Figure 11). At K, the Vegetation1 (conifers) photosynthesis rate in 2006 was 95% compared of that in 2007. This was the least affected vegetation compared to Vegetation2 (birch and field layer), 79%, and Vegetation3 (mosses), which reached only 44% of the photosynthesis rate compared a more normal year. One explanation is that vascular plants with deep roots are less negatively impacted due to access to water resources deeper down compared to mosses living in the soil surface with no roots. Mosses at L had lower photosynthesis rate in 2006, 86% of the normal rate; however, mosses at L generally had a lower coverage and lived in a more shaded environment than at K. It was also possible to see a transpiration increase for Vegetation1 by 8 and 15% for K and L, respectively, in 2006, while evaporation from mosses decreased for K and L by 48 and 14% of a normal year evaporation. Thus, vascular plants mediated a lowered WT, whereby the soil in the L scenario with more vascular plants dried out more than in the K site. An effect of the lowered WT was a larger aerobic soil volume and consequently increased heterotrophic respiration: 50 and 140% for the K and L scenario, respectively (Figure 11), displayed by the red line, and adding the autotrophic respiration gives the total respiration delineated by the top brown line.
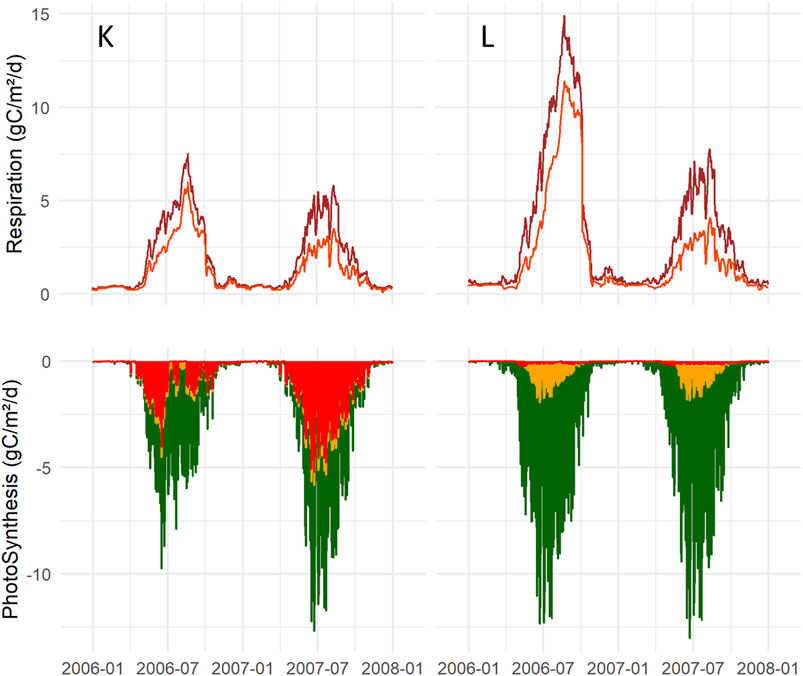
FIGURE 11. Top panel shows total ecosystem respiration (Reco) brown line, and heterotrophic respiration delineated by the red line at K (left) and L (right) during the dry (2006) and normal year (2007). Bottom panel, stacked diagram of GPP including three vegetation layers, Vegetation1 = dark green, Vegetation2 = yellow and Vegetation3 = red.
The simulation shows that K and L had different drought vulnerabilities: photosynthesis was the most sensitive at the K scenario because of a dominant moss layer, while at L, it increased the decomposition owing to a larger soil aerobic volume, which caused the soil to lose carbon. The soil C loss was the most decisive concerning C balance.
Discussion
This study aimed to examine how a sparsely forested nutrient-poor site could sequester more carbon than a fertile and dense forest, as observed previously by flux measurements. We hypothesized this could be explained by differences in the moss layer coverage, litter production, and decomposition. In many studies, the focus has been on vascular plants like trees, while the importance of mosses as a vital part of the ecosystem has not been recognized. Moreover, investigations of carbon balances with micrometeorological methods are complicated since many components interact with uncertain storage terms and are not easy to separate. Data coverage is never 100%, thus measured field data must be complemented with a modeling approach to fill data gaps and close the carbon balance, namely, the GPP and Re partitioning by using NEE data. Since the micrometeorological technique measures the net CO2 flux of the whole ecosystem, it is not possible to understand the role of separate vegetation layers without additional biomass growth measurements. In this study, we attempted to disentangle the importance of the many ecosystem components by using the CoupModel constrained with the best available measured field data. Our model keeps track of all major carbon fluxes and is based on the law of mass conservation, although there can still be uncertainties in partitioning. Thus, it is important to have a realistic model structure where the major components are identified. This was the background to why we developed the model also to describe the special morphology and physiology of mosses which in previous peat soil studies utilizing the COUP-model were not separated from vascular plants (Metzger et al., 2015; He et al., 2016a; He et al., 2016b; Metzger et al., 2016). The model was conceptualized with three vegetation layers to include and separate conifer trees, other vascular plants, and mosses. The soil description separated moss plant litter from vascular plant litter, both of which decomposed into a common third pool of decomposed soil organic matter. With these descriptions, the aim was to obtain a fitted model for drained peat soil, which could be used as a tool to investigate differences in carbon fluxes at different peatland ecosystems. In the following sections, we discuss the major findings and remaining challenges to explicitly describe the different vegetation functional roles.
Model Calibration and Uncertainties
Of the 15,000 calibration simulations, 100 were selected, and many different criteria were tested to obtain the reduced uncertainty for the simulation. The specific choice was made to produce the same type of error for both sites and to reduce errors for general impacts on the carbon balance. High-resolution uncertainty was smoothed out but not disregarded within a daily resolution.
When forcing the long-term mean value to be without a bias, this increased performance indicator (RMSE) that emphasizes the short-term dynamics. Objective use of the Bayesian approach has been tested, with different error model assumptions, which displayed different problems, especially when high numbers of data were relatively close to zero compared to a few peaks with either high negative or positive values.
The final selection of using RMSE on yearly accumulated numbers was taken because of its simplicity and robustness. Normally this indicator displayed similarity with other indicators like the Nash-Sutcliffe efficiency. We also tested our calibration of the quality checked gap-filled data for the Kalevansuo site, however, this resulted in a less good performance based on our statistical indicator. Thus, we rejected the use of gap-filled data since it also added another model to the procedure.
To reduce the general uncertainty, we also considered using a multi-criteria approach considering different data: sensible heat flux, latent heat flux, and chamber-based gas flux measurement for various treatments of the areas. However, a multi-criteria approach would add even more subjectivity, especially for the representativity error of the measurements. Thus, the first choice was not to include other data than the NEE. However, we found it necessary to constrain the model by estimated total plant biomass accumulation (i.e., tree stand growth) of the two sites. Without this constraint, the partitioning between the soil and the plant was uncertain. Additional criteria may be added for future studies to further test the uncertainty and role of the different components of the ecosystem. It is possible to reduce the uncertainties in the simulated plant vegetation with good empirical data.
Parameter Results and Performance
In many aspects, K and L were similar since most parameters changed in the same direction. The parameter controlling photosynthesis, N_PhotoFactor, displayed the same importance since the range after calibration became narrower and lower than the prior range. Several differences between the sites can be seen in the parameter TF SUM Start, with a slower start of photosynthesis after the winter for K. Moreover, vascular plants at K were revealed to be somewhat more sensitive to dry conditions than vascular plants at L, displayed by the parameter change of CriticalThresholdDry. For mosses, this parameter did not change. The central parameter, RateCoeffSOC, that described soil organic matter decomposition, was constructed to require small overall differences in soil decomposability between K and L. A prior proportionality of 1:5:50 (SOC1: SOC2: SOC3) for the minimum and 1:20:20 for the maximum values for the three SOC pools were used to meet this requirement (Table 1). After calibration, RateCoeffSOC1, 2, and three increased but were similar for both K and L as required. With this setting, we were able to describe with the model the measured NEE and biomass growth. However, it could be asked if our prior parameter setting was realistic since we had a large difference between decomposability of litter originating from vascular plants or mosses. Compared to experimental data compiled in Hobbie et al. (2000) we can see our SOC1 max value may have been a little high. However, the rate coefficient for SOC2 was similar to the measured values in Hobbie et al. (2000). By calibrating SOC1 and two independently but with the same prior range, the calibration resulted in different decomposability for the same litter quality at K and L, especially for the SOC2 (Supplementary Table S11A,B). The RateCoeffSOC2 became lower after calibration, although not as low as in the main setting, and higher compared to data in Hobbie et al. (2000). In this setting, SOC3 was accumulating for both K and L, rather than the SOC2 pool, as was the result of the main approach. Since the three pools act in the model as communicating vessels, the model in the independent calibration showed that decomposed matter accumulated instead of litter. Thus, to fit data with overall soil loss at Lettosuo, the simulation in this alternative calibration displayed losses mostly from the SOC2 and the SOC1 pools. With rapid litter decomposition, the effect of the moss litter addition is more difficult to disentangle since C ends up in the decomposed pool, however, the same large moss litter addition occurred. We think our main setting where each of the SOC pools showed a similar rate coefficients for the same SOC quality for the two scenarios could explain more regarding the soil processes than rapid litter decomposition into a large soil C-pool. Since the experiments also displayed a difference in decomposability between leaf-litter from vascular plants compared with mosses, we find it more realistic to describe the soil and litter qualities with distinct different rate coefficients for these litters.
The EC-data of CO2 exchange collected at Kalevansuo over 4 years used in this study was in an earlier study presented as gap-filled data resulting in a similar NEE, −234 g C m−2 yr−1 (Minkkinen et al., 2018). Using this gap-filled data in model calibration demonstrated less good performance which is why our choice was to use non-gap-filled NEE-data in our study. The modeled data reflected the measured NEE and biomass growth data quite well. Deviations between simulated and measured data were most pronounced during summer when gas exchange activities are high with large day-to-day fluctuations.
Simulation Covering Wet and dry Years
Over the 10 simulated years, including both wet and dry years, the NEE was on average −232 g C m−2 yr−1 for K and +7 g C m−2 yr−1 for L. These numbers were close to the measured NEE on which the model was calibrated, −272 g C m−2 yr−1 and −61 g C m−2 yr−1 for Kalevansuo and Lettosuo, respectively, however, these numbers were measured in two different periods. The L system shifted from a small net sink in normal years into a source during the dry years. This variation between years is common, where some years are sources and other sinks, like for a pristine bog in Canada where NEE varied between +14 g C m−2 yr−1 and −89 g C m−2 yr−1 (Roulet et al., 2007).
The NEE was separated into photosynthesis and respiration, anchored on biomass accumulation data of trees. The overall photosynthesis was quite similar for K and L, with a somewhat lower overall annual GPP for K (−903 g C m−2 yr−1) than L (−1,034 g C m−2 yr−1). Interestingly, our model suggests that mosses contribute approximately 40% of the total GPP at K, while at L, the mosses were of minor importance, which is a crucial difference between the scenarios. Mosses have the same biomass each year, meaning that all production goes into the litter, which is decomposed at a much slower rate than vascular plant litter. With a high moss composition for K, and despite a lower overall GPP, the model simulated K to accumulate carbon into the soil. In earlier studies at Kalevansuo, using chamber data sampled during the summer of 2008 and 2009, the measured moss GPP contributed 19–27% of the ecosystem GPP (Badorek et al., 2011), and moss litter production was 20% of the total (Minkkinen et al., 2018), where the importance of mosses was somewhat smaller than modeled here. However, photosynthesis and biomass production can vary between years and results influenced by the methodology used. The aim of this modeling study was not to primarily fit all the model processes against measurements but to display possible influence of mosses and vascular plants on the ecosystem C-balance.
The model was conceptualized with three initial SOC components, quantities that did not change much over the 10 years in relation to each other. However, the overall stock increased for the K scenario and decreased for L. With the three components, it was possible to see that the SOC2 pool of K increased the most, defined as moss litter which was assumed to be added only in the second soil layer (no2), 5–15 cm soil depth, a layer which most times experienced aerobic conditions. In K, we also noticed a small increase in SOC3. In L, all the three SOC pools decreased over time. However, when we used the other concept for the RateCoeffSOC calibration, with a similar RateCoeffSOC independent of litter type, this demonstrated the SOC3 pool to accumulate for both K and L, whereas the SOC2 soil component decomposed the most for L. Thus, understanding how to conceptualize the ecosystem into a model may be of importance for seeking answers on the function. However, by assuming similar decomposability of vascular plant and moss litter, more C ended up in the decomposed material. If one litter type (here mosses) decomposes slower, the only difference was that more undecomposed SOC2 accumulated in the soil. And it was the litter quantity per se that decided whether the soil accumulated C or not.
The WTs in K and L were generally relatively high, typically around 0.3-m depth, but the level fluctuated with seasons and weather conditions, with water saturation sometimes even close to the soil surface. In the dry summer of 2006, the WT was lower, reaching deeper than 0.9 m at L and 0.75 m at K. Despite K having wetter conditions in the dry summers the photosynthesis rate was affected more than for L. This we think can be explained by vegetation abundance differences, between the K and L, where the latter site with a higher relative abundance of vascular plants with roots can reach and access water at depths, thus able to continue photosynthesis. Thus, the L scenario with domination of vascular plants continued to deliver litter but this did not compensate for the soil losses. Where vascular plants can use the soil water for transpiration, mosses instead desiccate and survive the dry conditions waiting for the rain. It is known that mosses on the other hand are able to cope drought, becoming considerably dry and regain function when water becomes available (Proctor et al., 2007), with reduced growth during dry conditions (Norby et al., 2019). Thus where mosses dominates the ecosystem the photosynthesis is hampered by dry conditions, and the dry moss plants influence the soil water content less. The earlier added moss litter do also withstand oxygenated soil condition better than vascular plant litter, thus the model shows the K scenario SOC2 pool not to be as affected by the drought as the SOC1 pool. Thus the draught influence both photosynthesis and soil decomposition, where vascular plants increase the soil water withdrawal and an otherwise water-saturated soil became air-filled with increased soil decomposition. Besides draughts, warmer summers has also been demonstrated to affect the moss growth negatively (Gunnarsson et al., 2004); however, warmer conditions also induce desiccation, and it is difficult to separate a temperature effect from a water effect. In coming years dry conditions is expected to be more common why our understanding of the ecosystems on peatland soils is important for a management protecting the carbon.
Comparison With Other Studies
Ecosystem processes are influenced by many variables. It is a challenge to disentangle and explain the many ecosystem functions where carbon is simultaneously released and accumulated. In our simulation, the resulting GPP for the two scenarios were similar to GPP earlier estimated for forests in Finland and Sweden (Lagergren et al., 2008) as well as in Canada (Zha et al., 2013), at approximately −1,000 g C m−2 yr−1. Similarly Minkkinen et al. (2018) in a study made at Kalevansuo found an average GPP of trees and ground vegetation of −1,037 g C m−2 yr−1 and Re of 807 g C m−2 yr−1, where NEE was partitioned into photosynthesis based upon light, CO2 in the air, and the water vapor deficit, and respiration upon air temperature. The authors here used an independent tree-growth model, Stand Photosynthesis Program (SPP), suggesting 70% of the overall GPP was produced by trees, leaving 30% for the ground vegetation. Another study at Kalevansuo, concluded share of mosses was 19–27% of measured total GPP (Badorek et al., 2011). In our modeling scenario for K, based on Kalevansuo, the GPP shares for the different vegetation layers 1, 2, and three were 56, 5, and 38%, respectively, which can explain different results.
The photosynthetic efficiency of mosses compared to vascular plants is uncertain. Several studies have demonstrated mosses to be less efficient compared to vascular plants (Whitehead and Gower, 2001; Yuan et al., 2014), while others have shown them to be similar when relating rates to chlorophyll content (Martin and Adamson, 2001). For simplicity, we assumed moss photosynthesis to have similar light use efficiency as vascular plants. In contrast to static models, the CoupModel estimates photosynthesis and respiration based on many influencing and interacting factors, like water and nitrogen limitation as well as the temperatures of both air and each of the soil layers, which change over time. Thus, detailed simulations can be made using the CoupModel based on each vegetation layer, simulated separately with many respiration components, with different temperature Q10 functions for the maintenance respiration for tissues above and below ground, and each soil layer which is influenced by the surroundings. There will always be certain discrepancies between how the system is described in the model and the reality. Both the model and measured data have uncertainties, where measured data are compiled during a short period with uncertainties in the methods.
Experimental data have demonstrated decomposability to be lowest in Sphagnum moss and woody litter, somewhat higher for forest moss litter, and being the highest in the leaf-litter of vascular plants (Hobbie et al., 2000; Strakova et al., 2011). For simplicity, in the model, we only assumed that leaf litter was added to the soil with no woody litter, and different decomposition rates were assumed only for the leaf litter of vascular plants and mosses. No differentiation was made between Sphagnum and forest mosses. As the field layer in Kalevansuo, besides mosses, also produces small shrubs which add woody litter among the growing mosses (Minkkinen et al., 2018), this could, together with moss litter addition, also explain the accumulation of C in the Kalevansuo peatland. However, the above suggestion of the importance of shrubs does not contradict the model results; namely, that mosses can contribute to C-accumulation since they are plants producing a litter with low decomposability and no living plant C-accumulation, where all the produce goes to litter.
Another explanation for the C-losses at Lettosuo could be a larger soil carbon content in the top 50 cm, where the L scenario lost more C than K during drought spells. Other studies have also, like our study, demonstrated increased soil respiration during dry conditions, which increased the most at spots with vascular plants in contrast to Sphagnum moss spots (Munir et al., 2017). In our study, we could explain soil decomposition size by 1) litter quality and quantity, 2) the soil aerobic volume, and 3) the soil carbon density, which are all connected with site fertility (Ojanen et al., 2013). The explicit inclusion and separation of mosses in this modeling study demonstrated the importance of these plants which contribute a relatively large part (38% in K) of the ecosystem GPP. Whereas trees direct their production to growing biomass, the biomass of mosses remains the same, and all primary production is directed to litter with low decomposability.
In wet conditions, Sphagnum mosses are known as ecosystem engineers, forming peatlands (Van Breemen, 1995). In drained peatlands, mosses can still thrive at nutrient-poor sites, which remain relatively open compared to nutrient-rich sites, although species dominance may change from peat mosses to forest mosses, as is the case in Kalevansuo. In contrast to nutrient-poor sites, post-drainage change at nutrient-rich sites is rapid and often completely towards forest vegetation (Laine et al., 1995). A lowered WT favors trees at the expense of wetland-adapted plants, where a dominating tree layer takes hold on available light and nutrients out-competing field and ground plants (Laiho et al., 2003), which also affects soil C balance. In our study, mosses are suggested to be significant for soil C sequestration. This was also concluded in a literature review and modeling study, where more mosses facilitated soil C-accumulation owing to slow decomposition (Turetsky et al., 2012). Additionally, when moss vegetation was lost, C-accumulation in an ombrotrophic bog was reduced (Larmola et al., 2013).
Alongside the common suggestion of rewetting drained peat soils, to restore the C-accumulation, could be an attempt to keep a dense moss vegetation covering the soil. A WT as high as possible (30 cm) both decreased soil organic matter decomposition (Ojanen and Minkkinen, 2019) and favored moss plants. Although mosses are key for C sequestration in peatlands, changed silvicultural management could increase moss coverage. This could be reached by moving from even-aged rotation forestry with clear-cuts to continuous-cover forestry, where the tree stands are relatively open, allowing continuous regeneration of the trees. Our aim was here to discuss the role of vegetation and environment on C balances, to keep the carbon in the soil, but a continuous forestry management have also been suggested out of an aim to avoid ditch cleaning, as the remaining vegetation will still keep the site dry enough (Leppä et al., 2020). This shows that the trees need to be sparse enough to reduce evapotranspiration, to be able to raise the WT (Sarkkola et al., 2010). There is a large need to stop unsustainable land use and reduce global GHG emissions and thus the aim should be to raise the WT high enough, at least to a level of 30 cm but best would be 10 cm below the surface, for obtaining minimized GHG losses (Kasimir et al., 2018; Evans et al., 2021). Thus for protection of peat, both rewetting and more wetland-adapted plants are of need.
By using models, our shortfall of ecosystem understanding could be revealed and indicate where there is a need for better knowledge and numbers. Thus, the model results can be used to design new studies. Our modeling demonstrates a need to continue examining the impact of vegetation on the C-balance of ecosystems and soils; the impact by mosses needs to be especially highlighted. Additionally, the effect of tree types, conifers, and/or deciduous trees, alongside vegetation composition and tree density, should be examined. Moreover, we have not touched upon whether a dense leaf litter layer could hinder moss growth and peat accumulation or perhaps seal the soil surface (Laine and Vanhamajamaa, 1992), possibly reducing air exchange and causing anoxic soil conditions, which can be suggested for future investigations.
Using the model, we are confident that the set-up of the CoupModel made in this study may be used as a role-model for investigations of drained peat soils of slightly different use, as managed grasslands and forests. An advantage of the model is that it takes into account properties of different vegetation types and soil qualities and dynamically follows the processes of energy, water, carbon, and nutrients. However, it should be noted we only assumed water to enter the soil by precipitation, and care should be taken if vertical water addition is to be expected. In this study, we also used data from two sites close by and of relatively similar types. For model improvements and examination of processes, there is a need for long-term data from systems with distinctly different qualities and fluxes. Also, it is of high interest to further develop the uncertainty based calibration methods and to find common protocol designed for various purposes (Van Oijen et al., 2011; Wallach et al., 2021).
Conclusion
• In a dry spell, moss photosynthesis is reduced in contrast to trees and other vascular plants. For the latter, water access maintains photosynthesis and transpiration, causing the ground-water level to drop.
• Of moss net primary production, most goes into litter which, together with lower decomposability, results in carbon addition to the soil.
• Explicit consideration of differences between mosses and vascular plants is highly recommended for future studies.
• This study can be used as a base for continued studies on how C-balances in wetlands are influenced by hydrology and vegetation. However, there is still parameter uncertainty, and care should be taken before the model is applied for various peatlands.
Data Availability Statement
The original contributions presented in the study are included in the article/Supplementary Material, further inquiries can be directed to the corresponding author.
Authors Contributions
ÅK led the work and wrote the text; HH made the initial set up on the Finnish data and made the initial calibrations; PEJ led the model design, calibrations, and made most figures; AL and KM contributed to the description of the sites and EC-data for calibration and commented on the manuscript.
Funding
We would like to acknowledge funding from FORMAS Research Council, grant nos 942-5015-1287 and 2019-01991; “Climate smart management practices on Norwegian organic soils” (MYR), funded by the Research Council of Norway, grant no 281109; the Swedish strategic research area “Biodiversity and Ecosystem Services in a Changing Climate” (BECC).
Conflict of Interest
The authors declare that the research was conducted in the absence of any commercial or financial relationships that could be construed as a potential conflict of interest.
Publisher’s Note
All claims expressed in this article are solely those of the authors and do not necessarily represent those of their affiliated organizations, or those of the publisher, the editors and the reviewers. Any product that may be evaluated in this article, or claim that may be made by its manufacturer, is not guaranteed or endorsed by the publisher.
Acknowledgments
We acknowledge Paavo Ojanen and Juha Heiskanen, Luke Finland, for help accessing data. The model and all the data used for this study is available by request from the corresponding authors and stored in an archive linked to the CoupModel home page (www.coupmodel.com).
Supplementary Material
The Supplementary Material for this article can be found online at: https://www.frontiersin.org/articles/10.3389/fenvs.2021.680430/full#supplementary-material
References
Alm, J., Talanov, A., Saarnio, S., Silvola, J., Ikkonen, E., Aaltonen, H., et al. (1997). Reconstruction of the Carbon Balance for Microsites in a Boreal Oligotrophic Pine Fen, Finland. Oecologia 110, 423–431. doi:10.1007/s004420050177
Badorek, T., Tuittila, E.-S., Ojanen, P., and Minkkinen, K. (2011). Forest Floor Photosynthesis and Respiration in a Drained Peatland forest in Southern Finland. Plant Ecol. Divers. 4, 227–241. doi:10.1080/17550874.2011.644344
Bhuiyan, R., Minkkinen, K., Helmisaari, H.-S., Ojanen, P., Penttilä, T., and Laiho, R. (2017). Estimating Fine-Root Production by Tree Species and Understorey Functional Groups in Two Contrasting Peatland Forests. Plant Soil 412, 299–316. doi:10.1007/s11104-016-3070-3
Chadburn, S. E., Krinner, G., Porada, P., Bartsch, A., Beer, C., Belelli Marchesini, L., et al. (2017). Carbon Stocks and Fluxes in the High Latitudes: Using Site-Level Data to Evaluate Earth System Models. Biogeosciences 14, 5143–5169. doi:10.5194/bg-14-5143-2017
Dorrepaal, E., Cornelissen, J. H. C., Aerts, R., Wallén, B., and Van Logtestijn, R. S. P. (2005). Are Growth Forms Consistent Predictors of Leaf Litter Quality and Decomposability across Peatlands along a Latitudinal Gradient? J. Ecol. 93, 817–828. doi:10.1111/j.1365-2745.2005.01024.x
Evans, C. D., Peacock, M., Baird, A. J., Artz, R. R. E., Burden, A., Callaghan, N., et al. (2021). Overriding Water Table Control on Managed Peatland Greenhouse Gas Emissions. Nature 593 (7860), 548–552. doi:10.1038/s41586-021-03523-1
Frolking, S., Roulet, N. T., Moore, T. R., Richard, P. J. H., Lavoie, M., and Muller, S. D. (2001). Modeling Northern Peatland Decomposition and Peat Accumulation. Ecosystems 4, 479–498. doi:10.1007/s10021-001-0105-1
Gorham, E. (1991). Northern Peatlands: Role in the Carbon Cycle and Probable Responses to Climatic Warming. Ecol. Appl. 1, 182–195. doi:10.2307/1941811
Gunnarsson, U., Granberg, G., and Nilsson, M. (2004). Growth, Production and Interspecific Competition in Sphagnum : Effects of Temperature, Nitrogen and sulphur Treatments on a Boreal Mire. New Phytol. 163, 349–359. doi:10.1111/j.1469-8137.2004.01108.x
Günther, A., Barthelmes, A., Huth, V., Joosten, H., Jurasinski, G., Koebsch, F., et al. (2020). Prompt Rewetting of Drained Peatlands Reduces Climate Warming Despite Methane Emissions. Nat. Commun. 11, 1644. doi:10.1038/s41467-020-15499-z
He, H., Jansson, P.-E., and Gärdenäs, A. I. (2021). CoupModel (v6.0): An Ecosystem Model for Coupled Phosphorus, Nitrogen, and Carbon Dynamics - Evaluated against Empirical Data from a Climatic and Fertility Gradient in Sweden. Geosci. Model. Dev. 14, 735–761. doi:10.5194/gmd-14-735-2021
He, H., Jansson, P.-E., Svensson, M., Björklund, J., Tarvainen, L., Klemedtsson, L., et al. (2016b). Forests on Drained Agricultural Peatland Are Potentially Large Sources of Greenhouse Gases - Insights from a Full Rotation Period Simulation. Biogeosciences 13, 2305–2318. doi:10.5194/bg-13-2305-2016
He, H., Jansson, P.-E., Svensson, M., Meyer, A., Klemedtsson, L., and Kasimir, Å. (2016a). Factors Controlling Nitrous Oxide Emission from a Spruce Forest Ecosystem on Drained Organic Soil, Derived Using the CoupModel. Ecol. Model. 321, 46–63. doi:10.1016/j.ecolmodel.2015.10.030
Hobbie, S. E., Schimel, J. P., Trumbore, S. E., and Randerson, J. R. (2000). Controls over Carbon Storage and Turnover in High-Latitude Soils. Glob. Change Biol. 6, 196–210. doi:10.1046/j.1365-2486.2000.06021.x
Huang, Y. Y., Ciais, P., Luo, Y. Q., Zhu, D., Wang, Y. P., Qiu, C. J., et al. (2021). Tradeoff of CO2 and CH4 Emissions from Global Peatlands under Water-Table Drawdown. Nat. Clim. Change 11, 618–622. doi:10.1038/s41558-021-01059-w
Huth, V., Günther, A., Bartel, A., Gutekunst, C., Heinze, S., Hofer, B., et al. (2021). The Climate Benefits of Topsoil Removal and Sphagnum Introduction in Raised Bog Restoration. Restoration Ecol., e13490. doi:10.1111/rec.13490
Jansson, P.-E., and Halldin, S. (1979). “Model for Annual Water and Energy Flow in a Layered Soil,” in Comparison of Forest and Energy Exchange. Editor S. Halldin (Copenhagen: Society for Ecological Modelling), 145–163. doi:10.1016/b978-0-444-41844-9.50017-2
Jansson, P. E. (2012). Coupmodel: Model Use, Calibration, and Validation. Trans. Asabe 55, 1335–1344. doi:10.13031/2013.42245
Johnsson, H., Bergström, L., Jansson, P.-E., and Paustian, K. (1987). Simulated Nitrogen Dynamics and Losses in a Layered Agricultural Soil. Agric. Ecosyst. Environ. 18, 333–356. doi:10.1016/0167-8809(87)90099-5
Kasimir, Å., He, H., Coria, J., and Nordén, A. (2018). Land Use of Drained Peatlands: Greenhouse Gas Fluxes, Plant Production, and Economics. Glob. Change Biol. 24, 3302–3316. doi:10.1111/gcb.13931
Korkiakoski, M. (2020). The Short-Term Effect of Partial Harvesting and Clearcutting on Greenhouse Gas Fluxes and Evapotranspiration in a Nutrient-Rich Peatland forest. Helsinki: Helsingin yliopisto.
Kuhry, P., and Vitt, D. H. (1996). Fossil Carbon/Nitrogen Ratios as a Measure of Peat Decomposition. Ecology 77, 271–275. doi:10.2307/2265676
Lagergren, F., Lindroth, A., Dellwik, E., Ibrom, A., Lankreijer, H., Launiainen, S., et al. (2008). Biophysical Controls on CO2fluxes of Three Northern Forests Based on Long-Term Eddy Covariance Data. Tellus B 60, 143–152. doi:10.1111/j.1600-0889.2006.00324.x
Laiho, R., Vasander, H., Penttila, T., and Laine, J. (2003). Dynamics of Plant-Mediated Organic Matter and Nutrient Cycling Following Water-Level Drawdown in Boreal Peatlands. Glob. Biogeochem. Cycles 17, 11. doi:10.1029/2002gb002015
Laine, J., and Vanhamajamaa, I. (1992). Vegetation Ecology along a Trophic Gradient on Drained Pine Mires in Southern Finland. Ann. Botanici Fennici 29, 213–233.
Laine, J., Vasander, H., and Laiho, R. (1995). Long-Term Effects of Water Level Drawdown on the Vegetation of Drained pine Mires in Southern Finland. J. Appl. Ecol. 32, 785–802.
Lang, S. I., Cornelissen, J. H. C., Klahn, T., Van Logtestijn, R. S. P., Broekman, R., Schweikert, W., et al. (2009). An Experimental Comparison of Chemical Traits and Litter Decomposition Rates in a Diverse Range of Subarctic Bryophyte, Lichen and Vascular Plant Species. J. Ecol. 97, 886–900. doi:10.1111/j.1365-2745.2009.01538.x
Larmola, T., Bubier, J. L., Kobyljanec, C., Basiliko, N., Juutinen, S., Humphreys, E., et al. (2013). Vegetation Feedbacks of Nutrient Addition lead to a Weaker Carbon Sink in an Ombrotrophic Bog. Glob. Change Biol. 19, 3729–3739. doi:10.1111/gcb.12328
Leppä, K., Korkiakoski, M., Nieminen, M., Laiho, R., Hotanen, J.-P., Kieloaho, A.-J., et al. (2020). Vegetation Controls of Water and Energy Balance of a Drained Peatland forest: Responses to Alternative Harvesting Practices. Agric. For. Meteorology 295, 108198. doi:10.1016/j.agrformet.2020.108198
Lohila, A., Minkkinen, K., Aurela, M., Tuovinen, J.-P., Penttilä, T., Ojanen, P., et al. (2011). Greenhouse Gas Flux Measurements in a Forestry-Drained Peatland Indicate a Large Carbon Sink. Biogeosciences 8, 3203–3218. doi:10.5194/bg-8-3203-2011
Martin, C. E., and Adamson, V. J. (2001). Photosynthetic Capacity of Mosses Relative to Vascular Plants. J. Bryology 23, 319–323. doi:10.1179/jbr.2001.23.4.319
Mathijssen, P. J. H., Kähkölä, N., Tuovinen, J.-P., Lohila, A., Minkkinen, K., Laurila, T., et al. (2017). Lateral Expansion and Carbon Exchange of a Boreal Peatland in Finland Resulting in 7000 Years of Positive Radiative Forcing. J. Geophys. Res. Biogeosci. 122, 562–577. doi:10.1002/2016jg003749
Metzger, C., Jansson, P.-E., Lohila, A., Aurela, M., Eickenscheidt, T., Belelli-Marchesini, L., et al. (2015). CO2 Fluxes and Ecosystem Dynamics at Five European Treeless Peatlands - Merging Data and Process Oriented Modeling. Biogeosciences 12, 125–146. doi:10.5194/bg-12-125-2015
Metzger, C., Nilsson, M. B., Peichl, M., and Jansson, P.-E. (2016). Parameter Interactions and Sensitivity Analysis for Modelling Carbon Heat and Water Fluxes in a Natural Peatland, Using CoupModel V5. Geosci. Model. Dev. 9, 4313–4338. doi:10.5194/gmd-9-4313-2016
Meyer, A., Tarvainen, L., Nousratpour, A., Björk, R. G., Ernfors, M., Grelle, A., et al. (2013). A fertile Peatland forest Does Not Constitute a Major Greenhouse Gas Sink. Biogeosciences 10, 7739–7758. doi:10.5194/bg-10-7739-2013
Minkkinen, K., and Laine, J. (1998). Long-term Effect of forest Drainage on the Peat Carbon Stores of pine Mires in Finland. Can. J. For. Res. 28, 1267–1275. doi:10.1139/x98-104
Minkkinen, K., Ojanen, P., Penttilä, T., Aurela, M., Laurila, T., Tuovinen, J.-P., et al. (2018). Persistent Carbon Sink at a Boreal Drained Bog forest. Biogeosciences 15, 3603–3624. doi:10.5194/bg-15-3603-2018
Munir, T. M., Khadka, B., Xu, B., and Strack, M. (2017). Partitioning Forest-Floor Respiration into Source Based Emissions in a Boreal Forested Bog: Responses to Experimental Drought. Forests 8, 17. doi:10.3390/f8030075
Norby, R. J., Childs, J., Hanson, P. J., and Warren, J. M. (2019). Rapid Loss of an Ecosystem Engineer: Sphagnum Decline in an Experimentally Warmed Bog. Ecol. Evol. 9, 12571–12585. doi:10.1002/ece3.5722
Ojanen, P., Minkkinen, K., Lohila, A., Badorek, T., and Penttilä, T. (2012). Chamber Measured Soil Respiration: A Useful Tool for Estimating the Carbon Balance of Peatland forest Soils? For. Ecol. Manage. 277, 132–140. doi:10.1016/j.foreco.2012.04.027
Ojanen, P., Minkkinen, K., and Penttilä, T. (2013). The Current Greenhouse Gas Impact of Forestry-Drained Boreal Peatlands. For. Ecol. Manage. 289, 201–208. doi:10.1016/j.foreco.2012.10.008
Ojanen, P., and Minkkinen, K. (2019). The Dependence of Net Soil CO2 Emissions on Water Table Depth in Boreal Peatlands Drained for Forestry. Mires and Peat 24, 8. doi:10.19189/MaP.2019.OMB.StA.1751
Pedrotti, E., Rydin, H., Ingmar, T., Hytteborn, H., Turunen, P., and Granath, G. (2014). Fine-scale Dynamics and Community Stability in Boreal Peatlands: Revisiting a Fen and a Bog in Sweden after 50 Years. Ecosphere 5, 24. doi:10.1890/es14-00202.1
Proctor, M. C. F., Oliver, M. J., Wood, A. J., Alpert, P., Stark, L. R., Cleavitt, N. L., et al. (2007). Desiccation-tolerance in Bryophytes: a Review. The Bryologist 110, 595–621. doi:10.1639/0007-2745(2007)110[595:dibar]2.0.co;2
FAO (2014). “Towards Climate-Responsible Peatlands Management,” in Mitigation of Climate Change in Agriculture SERIES. Editors R Biancalani, and A. Avagyan (Rome: Food and Agriculture Organization of the United Nations (FAO)).
Roulet, N. T., Lafleur, P. M., Richard, P. J. H., Moore, T. R., Humphreys, E. R., and Bubier, J. (2007). Contemporary Carbon Balance and Late Holocene Carbon Accumulation in a Northern Peatland. Glob. Change Biol. 13, 397–411. doi:10.1111/j.1365-2486.2006.01292.x
Sarkkola, S., Hökkä, H., Koivusalo, H., Nieminen, M., Ahti, E., Päivänen, J., et al. (2010). Role of Tree Stand Evapotranspiration in Maintaining Satisfactory Drainage Conditions in Drained Peatlands. Can. J. For. Res. 40, 1485–1496. doi:10.1139/x10-084
St-Hilaire, F., Wu, J., Roulet, N. T., Frolking, S., Lafleur, P. M., Humphreys, E. R., et al. (2010). McGill Wetland Model: Evaluation of a Peatland Carbon Simulator Developed for Global Assessments. Biogeosciences 7, 3517–3530. doi:10.5194/bg-7-3517-2010
Straková, P., Niemi, R. M., Freeman, C., Peltoniemi, K., Toberman, H., Heiskanen, I., et al. (2011). Litter Type Affects the Activity of Aerobic Decomposers in a Boreal Peatland More Than Site Nutrient and Water Table Regimes. Biogeosciences 8, 2741–2755. doi:10.5194/bg-8-2741-2011
Svensson, M., Jansson, P.-E., and Berggren Kleja, D. (2008). Modelling Soil C Sequestration in spruce forest Ecosystems along a Swedish Transect Based on Current Conditions. Biogeochemistry 89, 95–119. doi:10.1007/s10533-007-9134-y
Turetsky, M. R., Bond‐Lamberty, B., Euskirchen, E., Talbot, J., Frolking, S., Mcguire, A. D., et al. (2012). The Resilience and Functional Role of moss in Boreal and Arctic Ecosystems. New Phytol. 196, 49–67. doi:10.1111/j.1469-8137.2012.04254.x
Van Breemen, N. (1995). How Sphagnum Bogs Down Other Plants. Trends Ecol. Evol. 10, 270–275. doi:10.1016/0169-5347(95)90007-1
Van Oijen, M., Cameron, D. R., Butterbach-Bahl, K., Farahbakhshazad, N., Jansson, P.-E., Kiese, R., et al. (2011). A Bayesian Framework for Model Calibration, Comparison and Analysis: Application to Four Models for the Biogeochemistry of a Norway spruce forest. Agric. For. Meteorology 151, 1609–1621. doi:10.1016/j.agrformet.2011.06.017
Vesala, T., Kljun, N., Rannik, Ü., Rinne, J., Sogachev, A., Markkanen, T., et al. (2008). Flux and Concentration Footprint Modelling: State of the Art. Environ. Pollut. 152, 653–666. doi:10.1016/j.envpol.2007.06.070
Waddington, J. M., and Roulet, N. T. (1996). Atmosphere-Wetland Carbon Exchanges: Scale Dependency of CO2and CH4exchange on the Developmental Topography of a Peatland. Glob. Biogeochem. Cycles 10, 233–245. doi:10.1029/95gb03871
Wallach, D., Palosuo, T., Thorburn, P., Hochman, Z., Andrianasolo, F., Asseng, S., et al. (2021). Multi-Model Evaluation of Phenology Prediction for Wheat in Australia. Agric. For. Meteorology 298, 10. doi:10.1016/j.agrformet.2020.108289
Whitehead, D., and Gower, S. T. (2001). Photosynthesis and Light-Use Efficiency by Plants in a Canadian Boreal forest Ecosystem. Tree Physiol. 21, 925–929. doi:10.1093/treephys/21.12-13.925
Yu, Z. C. (2012). Northern Peatland Carbon Stocks and Dynamics: A Review. Biogeosciences 9, 4071–4085. doi:10.5194/bg-9-4071-2012
Yuan, W., Liu, S., Dong, W., Liang, S., Zhao, S., Chen, J., et al. (2014). Differentiating moss from Higher Plants Is Critical in Studying the Carbon Cycle of the Boreal Biome. Nat. Commun. 5, 4270. doi:10.1038/ncomms5270
Keywords: drained peat soils, vascular plants, mosses, coupmodel, boreal forest, litter, drought
Citation: Kasimir Å, He H, Jansson P-E, Lohila A and Minkkinen K (2021) Mosses are Important for Soil Carbon Sequestration in Forested Peatlands. Front. Environ. Sci. 9:680430. doi: 10.3389/fenvs.2021.680430
Received: 17 March 2021; Accepted: 23 August 2021;
Published: 29 September 2021.
Edited by:
Gilles Colinet, University of Liège, BelgiumReviewed by:
Na Li, Northeast Institute of Geography and Agroecology, Chinese Academy of Science, ChinaJeroen Meersmans, University of Liège, Belgium
Copyright © 2021 Kasimir, He, Jansson, Lohila and Minkkinen. This is an open-access article distributed under the terms of the Creative Commons Attribution License (CC BY). The use, distribution or reproduction in other forums is permitted, provided the original author(s) and the copyright owner(s) are credited and that the original publication in this journal is cited, in accordance with accepted academic practice. No use, distribution or reproduction is permitted which does not comply with these terms.
*Correspondence: Å. Kasimir, YXNhLmthc2ltaXJAZ3Uuc2U=