- 1International Institute for Applied Systems Analysis, Laxenburg, Austria
- 2Institute for Integrated Energy Systems, University of Victoria, Victoria, BC, Canada
Approaches that integrate feedback between climate, land, energy and water (CLEW) have progressed significantly in scope and complexity. The so-called nexus approaches have shown their usefulness in assessing strategies to achieve the Sustainable Development Goals in the contexts of increasing demands, resource scarcity, and climate change. However, most nexus analyses omit some important inter-linkages that could actually be addressed. The omissions often stem from technical and practical considerations, but also from limited dissemination of new open-source frameworks incorporating recent advances. We review and present a set of models that can meet the needs of decision makers for analysis tools capable of addressing a broad range of nexus questions. Particular attention is given to model accessibility, usability and community support. The other objective of this review is to discuss research gaps, and critical needs and opportunities for further model development from a scientific viewpoint. We explore at different scales where and why some nexus interactions are most relevant. We find that both very small scale and global models tend to neglect some CLEW interactions, but for different reasons. The former rarely include climate impacts, which are often marginal at the local level, while the latter mostly lack some aspects because of the complexity of large full CLEW systems at the global level.
1 Introduction
Interconnections between hydrology and water systems, natural and human land use, energy resources and climate have attracted increasing attention in the last decade. The so called Climate-Land-Energy-Water (CLEW, often in the literature referred to as Water-Energy-Food (WEF), without mentioning the climate component) nexus perspective aims at understanding complex issues that are intrinsically interconnected in our world and addressing the sustainable development objectives (see Figure 1 for a simplified diagram) Waughray (2011), IEA (2009), Liu et al. (2018a). Neglecting some of the nexus interactions has already lead to several policy choices then proved wrong. One famous example occurred in the early 2000s when a significant portion of US corn started being used for ethanol production. This lead to immediate consequences for Mexico, which relied on US corn export. The country suffered from the sudden increase in corn prices that afflicted the poor and lead to hunger in the country. Wise (2012). Liu et al. (2018a) also mention the case of the Aral Sea, where water flowing into the sea was diverted to create irrigated desert croplands but also led to a substantial loss in biodiversity and fishery as the lake shrank Liu et al. (2018a); Jin et al. (2017). Numerous cases are also related to water bodies, such as lakes or rivers shared among countries, which are often cause of disputes and, in some cases, of international conflicts on resource management (e.g., between Tajikistan and Uzbekistan or Pakistan and India in the Indus basin Nakayama et al. (2015); Keskinen et al. (2016); Vinca et al. (2020b)).
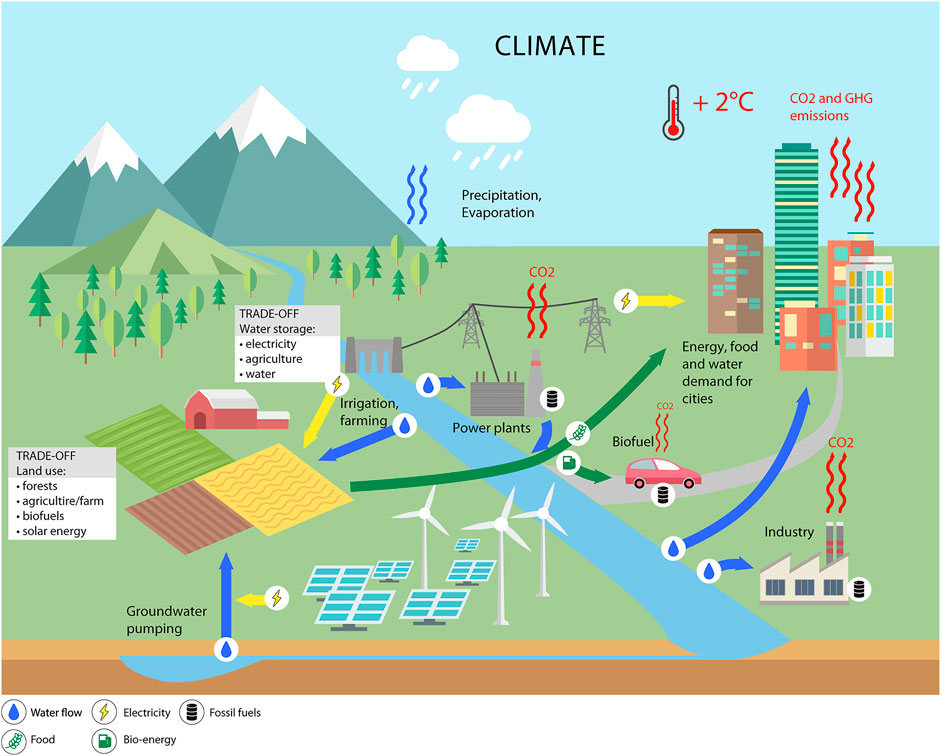
FIGURE 1. Schematic of the main interconnections between climate land energy and water systems. This cover has been designed using resources from Freepik.com, design from Nucleartist.
Advances in analytical modeling and computational capacity have allowed the development of increasingly comprehensive tools for quantitative analysis to better inform policy makers on the benefits and trade-offs between sectors Albrecht et al. (2018). A large number of models focusing on bilateral linkages (e.g., water-energy, water-land) have been developed and widely reviewed Albrecht et al. (2018); Endo et al. (2015); Khan et al. (2017). Recent reviews highlight the issue of having tools that embrace the Water-Energy-Food Nexus complexity and at the same time are useful for stakeholders Dai et al. (2018); Kaddoura and El Khatib (2017). For instance, Dai et al., 2018 state that: “none can or do provide a singular framework for performing a “exus study”” Dai et al. (2018). However, as of today some models have been successfully used in nexus studies where also climate is considered an important system as much as energy, water and land Daher and Mohtar (2015). The most recent studies incorporating all CLEW sectors have shown that restricted bilateral perspectives (and models) omit important feedback and should therefore be updated with comprehensive methodologies Graham et al. (2020); Vinca et al. (2020a).
Some of the outcomes of this paper have been to some extent touched by previous reviews, such as the limitations of model-linking and spatial resolution Khan et al. (2017); Johnson et al. (2019). This are expanded with reflections on community effort oriented at defining new CLEW standards or integrating with other models to better include socioeconomic aspects. Moreover, the above-mentioned reviews cover mostly global models (also included in this article), and no consideration on different scales, as a determining factor in the development approach Khan et al. (2017); Johnson et al. (2019). In some cases, mostly at small spatial and temporal scales, neglecting some interactions does not particularly affect the outcomes. However, at the country, basin, or even larger scales, and when considering long-term perspectives, responses between CLEW sectors are always present and should therefore be considered. The major barrier to using models that include all CLEW linkages are the complexity of methodology, and the difficulty of gathering data and expertize from different sectors, which limits numbers of groups that have the resources to build and maintain broad cross-sector capacity, both at the academic and institutional level Kaddoura and El Khatib (2017); Ramos et al. (2020). This review identifies research gaps and provides a perspective on some of the required developments for full CLEW models from a scientific point of view.
Ramos et al. (2020) review stands out by presenting models that actually focus on equal importance to all the CLEW interactions across scale sRamos et al. (2020). The paper however only focuses on the models within the CLEWs framework Howells et al. (2013), excluding a notable number of available models that provide a comprehensive coverage of the CLEW nexus, both as upgrades of pre-existing models and as totally new developments. This article reviews these models with the primary aim of guiding decision makers in selecting the analysis tool best suited to their needs. In addition to previous reviews, particular attention is given to factors that reinforce models’ longevity and usefulness, such as community support, accessibility and availability.
The paper starts with an overview of the CLEW nexus and the evolution of modeling streams (this is complemented with a basic introduction to Nexus models in the Supplementary Material). This is followed by a detailed comparison of a selection of models; an assessment of pros and cons; and a discussion of research gaps. This review concludes with a summary of recommendations for prospective users and modellers.
2 Climate, Land (and Food), Energy and Water Nexus Modeling Background
It has been recognized for some time that unraveling the synergies and trade-offs between climate, land (or food), energy and water is of fundamental importance to understanding the complexity of our planet, and to formulating effective policies for sustainable development Liu et al. (2018a); Endo et al. (2015). The United Nations’ Sustainable Development Goals (SDGs), are intrinsically linked to CLEW sectors and involve numerous interdependencies Liu et al. (2015); Nilsson et al. (2016); McCollum et al. (2018); Fader et al. (2018). The concept of CLEW nexus modeling has been first published as an overview of concepts in the World Economic Forum 2011 Waughray (2011) and has been incorporated into the analysis frameworks conceptualized at the International Atomic Energy Agency (IAEA), the Food and Agriculture Organization (FAO) and the Royal Institute of Technology in Stockholm (KTH) IAE (2009); Howells et al. (2013). The generic modules in the CLEW framework have been linked in various combinations and applied to several case studies for integrated assessment of the SDGs. Ramos et al. (2020) presents the history of the CLEWs framework and a detailed review of various models and application to 2019 Ramos et al. (2020). Concurrently, more research efforts have been directed at water-energy, energy-land or water-land bilateral interactions, and increasingly to linkages among water, energy and land (WEL) resources Khan et al. (2017); Liu et al. (2018b); Albrecht et al. (2018). The major focus has remained on balancing WEL interactions across multiple sectors and a better understanding of the synergies and trade-offs in future scenarios. However, the climate dimension has not been considered at the same level of detail as the sectoral representations Bazilian et al. (2011); Biggs et al. (2015); Liu et al. (2018a).
Another stream of CLEW nexus analyses is represented by the development of globally integrated assessment models (IAMs). These models have also been used in the last decade to inform the Intergovernmental Panel for Climate Change (IPCC) on the correlations between human energy use and production with climate, and their scope has been expanded in recent years to also incorporate a representation of land and water systems Parkinson et al. (2019); Bauer et al. (2020); Calvin et al. (2019); Bijl et al. (2018a); Van Vuuren et al. (2019). Decarbonization analyses have shown that nexus modeling is needed at multiple scales to better assess the interaction with policies impacting different sectors and administrative levels Sattler et al. (2012); Hejazi et al. (2015); Snyder et al. (2020). Spatial and temporal scales are therefore important factors that affect no only the structure of nexus models and the representations of energy, water and food supply but also the couplings and feedbacks between sectors Bijl et al. (2018b); Kahil et al. (2018); Köberle et al. (2020).
A number of studies have demonstrated that system integration can identify strategies that avoid or minimize trade-offs and achieve synergies Buras (1979); Lall and Mays (1981); Matsumoto and Mays (1983); Huang et al. (2017); Kernan et al. (2017); Santhosh et al. (2014); Pereira-Cardenal et al. (2016); Dodder et al. (2016); Oikonomou and Parvania (2018). Most of these studies have focused on, or considered interactions between, two or three of the CLEW systems, for instance, quantifying the economic benefits brought by joint water-energy system planning Howells et al. (2013); Dubreuil et al. (2013); Parkinson et al. (2016); Zhang and Vesselinov (2017); Khan et al. (2018); Bieber et al. (2018); Wang et al. (2018); Li et al. (2019); Vakilifard et al. (2019). Other examples of focused nexus interactions are studies analyzing impacts of energy decisions on land use, including bioenergy supply chain interactions Mesfun et al. (2018); Akhtari et al. (2018); de Carvalho Köberle (2018).
More recent studies, using models that attempt to integrate all salient CLEW nexus interactions, have shown that virtually all interaction matters, and are often related to the representation scales. Recent multi-scale studies with country or basin scale resolution show the importance of considering simultaneous CLEW interactions for instance in water reservoir management for power generation and storage for agriculture Vinca et al. (2020a); Payet-Burin et al. (2019); Sridharan et al. (2020). Conversely, global estimations of future water requirements for cooling power plants and those on virtual water related to future food trade both lack the other side of the medal and would benefit from a full CLEW system representation that takes into account all synergies to provide better assessments Parkinson et al. (2019); Dalin et al. (2017); Graham et al. (2018).
The Integrated Multisector Multiscale Modeling project (IM3) is a great example of coupling open-source models to cover nexus interlinkages and study for instance relation of changes in the water cycle for thermal power plants Zhang et al. (2020). Some models operate at the urban scale and show that for specific questions, such as heatwave exposure in an urban environment, some components of the CLEW nexus are not that relevant Vahmani et al. (2019). However, when considering only water-energy nexus when studying climate-change impacts at the national level, neglecting future changes in water requirements from other sectors might compromise the reliability of the results Voisin et al. (2020).
There is clearly a need to develop more competencies and technical solutions to build and run models that better represent the complexity of the natural and socioeconomic system we live in. This manuscript reviews some of the models with this potential and attempts to unveil the underlying complexity to different users.
3 Methods, Review Approach
The models compared in this review have been selected by using some systematic research approach complemented with manual selection to narrow on the main focus (full CLEW nexus models), direct interaction with the researchers and developers of the selected models. A first systemic review on the SCOPUS database using the CLEW keywords, together with “Nexus modeling”, “Integrated analysis”, and “Nexus scale” resulted in a substantial list of models. This list has been drastically cut to only include models with the representation of all the climate, land, energy and water sectors. Next, during the information validation phase, all main authors and developers of the selected models were engaged to validate the information presented in this manuscript. During this phase, is was also asked to the developers suggestion to expand the list with other novel CLEW models that we had not yet identified. This further expanded the final list with additional models and subsequent contact with their authors/developers.
During the validation phase the following questions were asked:
1. Would you agree on the following classification concerning your model [Table extract] If no, how would you change it?
2. Could you please suggest the most recent references for: (a) Documentation, online data/code repository; (b) Published application studies
3. Which of the following Nexus linkages are included in the model?
a. Water to Energy: water for power plant cooling; water for hydropower, storage
b. Water to Land: agriculture water use evapotranspiration
c. Water to climate: any
d. Energy to water: energy (or just electricity use) for water distribution, pumping, desalination
e. Energy to Land: energy (or just electricity use) use in land preparation or food chain production
f. Energy to climate: GHG emissions
g. Land to water: irrigation, canal, water reservoirs
h. Land to Energy: biomass production from crop or crop residues
i. Land to climate: de- and afforestation impact on GHG
j. Climate impacts on Water: change in ET rates and evaporation, precipitations, changes in demand
k. Climate impacts on energy: power plant cooling requirement, energy demand change, energy efficiency
l. Climate impacts on Land: crop yields
4. Could you name any other recently developed model, not from you or your team, that you think has the most relevance to the above-mentioned models?
Other models, listed below, were mentioned by developers or initially considered by the author and in the end not included in the review for one of the above-mentioned reasons. This however does not make the models or framework less worth being referred to, examined or used.
• IM3 framework more references in the Supplementary Material.
• ELENA Bataille et al. (2020).
• PRIMA Kraucunas et al. (2015).
• AWASH Rising (2020).
• TIMES-MARKAL Loulou et al. (2004).
4 CLEW Nexus Models
This Section provides a high-level description and assessment of the models currently available and maintained (to 2020). In particular, we examine the scales (spatial and temporal) at which various sectors are represented and resolved; the level of detail/complexity with which CLEW sector are represented; the cross-sector linkages and geophysical biases; the scope of model uses and levels of integration; and we finally provide an evaluation of models accessibility and usability.
4.1 Modeling Scale and Resolution
The scale of a model defines the spatial or geographical delineation of the real system that the model represents. Examples of different scales are a province/state or a country, a national or transboundary hydrological basin or agro-ecological zone, or simply a physical area defined in hectares or in terms of the elements that characterize it. Similarly, temporal scales range from seconds to years or decades. The scales determine the spatial or temporal scope within the modeling work. Up to a certain extent, temporal and spatial scales are often correlated Li et al. (2018). For instance, it is unlikely for a model representing CLEW dynamics in an urban district to have a ten-year time scale because the uncertainties related to boundary conditions (what happen at the interface of the modeled system with the outside) and future projections would become extremely high. On the other hand, global models are hardly ever resolved at an hourly scale, since mapping hourly dynamics for the entire world would be computationally prohibitive and require too much data. However, both global and district scale models can have a yearly time-step.
Scale and resolution are sometimes confused or used interchangeably, especially regarding the temporal dimension, where the two often converge into the same concept. In this paper we use the term scale to refer to the overall delineation of the system, and with the term resolution we refer to the smallest spatial or temporal unit of the model at which inputs are defined and outputs and model variables determined/updated.
Complex models such as CLEW models include different sub-models or sectors which are often best studied at different resolutions, while still representing the same scale. For instance, hydrological models and agricultural and land use models often require a very fine spatial resolution, defined with grid maps (e.g., portion of Decimal Degrees (DD)) predicated either by the gradients of underlying physical processes (e.g., heat transfer) or of the represented physical environment (e.g., soil humidity). Energy system models often have a simplified resolution consisting of a few discrete points for a country. The discrepancy in resolution or in scale is one of the major challenges when working with interconnected sectors in a single modeling framework, or with linked models (see Section 4.5).
This is most commonly addressed using concepts from global climate models: via up-scaling (aggregating) or downscaling some of the information Khan et al. (2020); Li et al. (2018); Chen et al. (2019). Other strategies identify spatial and temporal definitions that intersect existing ones (in ECHO, GCAM and other models, river basins are crossed by political border maps and agro-ecological zones). It should be noted that trade-offs on resolution are also adopted in single-discipline models; for example renewable energy modeling the representation of intermittent energy production, which presents features ranging from seconds to seasonal Broeer et al. (2014), is often simplified IAMs and linked to energy system model with multi-year temporal resolution Johnson et al. (2017).
Local and regional models, which are generally more oriented to implementation analyses, present a higher level of detail (or resolution) than others, but include less interconnection across sectors. This is noticeable in Figure 2, where all the local scale models and some country models include weak interlinkages to the climate sector. On the other hand, global models, which grant a higher level of aggregation and coarse representations, include all the CLEW sectors, but with limited interconnections compared to national models. This is due to the large amount of data that would need to cover all interlinkages and still poses computational challenges. The models with the highest number of interlinkages are those at the country or river basin scale.
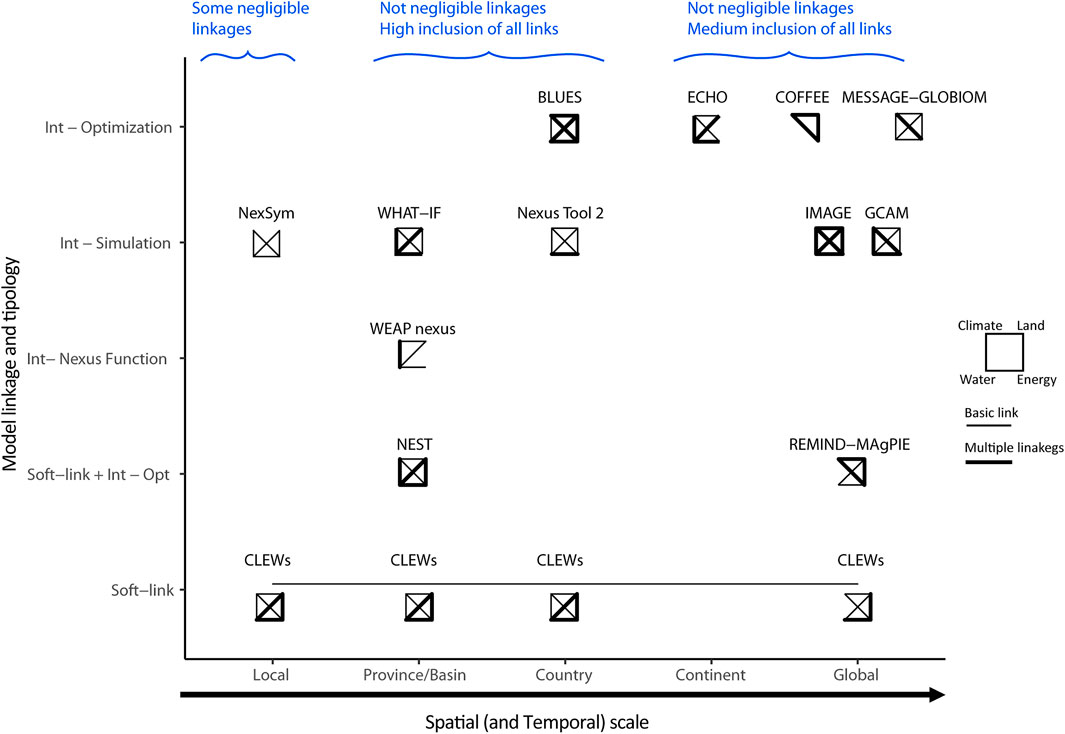
FIGURE 2. Increasing scale of the CLEW model considered and structure and nexus integration. Methods distinguish between soft-linked and integrated modules and simulation and optimization models. The squared symbols represent the interlinkages between climate, land, energy and water sectors, as displayed on the right panel (more detail in Supplementary Table S3).
An important limitation at small scales and fine resolutions is to collect data across multiple sectors with the same level of detail Engström et al. (2019). Local data collection is particularly critical in areas that lack official surveys or research from governments or institutions. Local data scarcity is often overcome with assumption through extrapolation or downscaling of data at a larger scale or coarse resolution Khan et al. (2020); Li et al. (2018); Chen et al. (2019). Another limitation for small-scale models is the assumptions needed to represent any possible interaction with outside the model scope Martinez-Hernandez et al. (2017). Boundary conditions are critical also at larger scales, such as basin and national and it is particularly relevant when import and export of resources are involved. To overcome it, price curves of external resources are often used, which require additional information on the boundaries Vinca et al. (2020a); Payet-Burin et al. (2019).
4.2 Sectoral Coverage: State of the Art and Compromises
Models are used to represent in a simplified way real systems and interactions. The level of detail of this representation usually depends on the research scope and questions, and this is particularly evident for newly developed CLEW nexus models. It is however not the case of the global models showed in this review, as most of them originated from energy-climate IAMs. Some of these models show a large disparity between the detail of land and water sectors, compared to the energy sector. Some models have invested or are currently investing important resources to fill this gap, for instance integrating linkages to land use and agriculture models (MESSAGE-GLOBIOM, REMIND-MAgPIE), or hydrological models (IMAGE, GCAM). Almost every model, including newly developed models, show some bias on detail in sectoral representation, often because of the main research questions and expertize. Consequently, most models include state-of-the-art features of the CLEW system, but applying simplifications to deal with nexus interactions. Below are reported the status of state-of-the-art modeling and cases of simplification. Table 1 includes a brief description of sectoral representation.
4.2.1 Energy
Depending on the scale of analysis, energy system models include detail on primary energy, such as fossil fuel supply, a number of energy supply and processing technologies, and representation of demand, often driven by individual choices Bauer et al. (2016); Grubler et al. (2018). Focus on specific generation technologies has lead, for instance, to incorporate spatial data of solar and wind renewable energy, hydropower and seasonal pump storage into models Pfenninger and Staffell (2016); Vinca et al. (2020a); Gernaat et al. (2017); Hunt et al. (2020). Most models at local or national scale deal with the problem of dispatchable electricity by linking with unit commitment models, which explore the feasibility of projected energy system in meeting realistic hourly electricity demand variations Welsch et al. (2014). When considering the global scale, with a division of the globe in macro-regions and yearly or multi-year time steps, the validation of the electricity system is often simplified, using curves that dispatchability to solar and wind installed capacity Johnson et al. (2017).
Other important sectors are usually included with more or less detail in energy-oriented IAMs, but then simplified or neglected in most of the CLEWs models. For example, transports, industry, and the residential sectors offer less connection to the land sector and get often reduced into energy and water demands Hejazi et al. (2014).
4.2.2 Water
A large portion of the models includes hydrological assessment, with the possibility to evaluate the impacts of human withdrawals and climatic changes on the water cycle, water demands and resources. Highly complex physical dynamics, such as soil water transfer, evaporation and evapotranspiration (ET) are often included with nonlinear systems of equations Burek et al. (2019); Schaphoff et al. (2018). The major aspects often neglected or simplified, which also represent growing research areas, are related to groundwater, alternative water sources (wastewater, desalination) and water quality Gleeson and Richter (2018); Jones et al. (2020); Strokal et al. (2019). In this context, water models are used to understand the impact of human demands on water resources and quality, as well as possible climate change impacts on human requirements. Interactions with other models, linking for instance hydropower and water for power plant cooling and crop growth models, allow making such assessments more reliable and robust. Some common simplifications of hydrology in CLEW models are aggregated annual water availability and constraints on demand to reflect, for instance, environmental limitations.
4.2.3 Land
Land use, vegetation, modeling of crops and forest growth, are intrinsically connected to water. Therefore, some models embed hydrology with nutrients models and plant life-cycle representation Schaphoff et al. (2018). Other frameworks include forestry modeling or link to food and wood industry, relating to socioeconomic factors Dietrich et al. (2019); Havlík et al. (2018). Such models can inform on how policies targeting hunger and sustainable food production could be pursued, and what are climate change implications on crop yields, or possible advancement that could help guarantee a balance between human needs and the ecosystem. In CLEW models, these dynamics are sometimes reduced to simple energy and water requirements per crop or food type. Or, in particular in global models that have an important energy component, the main focus has been so far on biomass availability at given energy prices.
4.2.4 Climate
Climate is a complex system and Atmosphere-Ocean General Circulation Models (AOGCM) still present a high level of uncertainty. Most of the CLEW models include climate dynamics in a simplified way, by linking to reduced-complexity models within the Coupled Model Intercomparison Project (CMIP6) IPCC (2014); Eyring et al. (2016). Models such as MAGICC, Hector and FaIR mimic climate response to input emissions of greenhouse gases to estimate radiative forcing, global mean atmospheric GHG concentrations and global mean temperature Eyring et al. (2016); Hartin et al. (2015). These models are sometimes used iteratively with CLEW integrated assessment models, or just used as exogenous pathways of GHG concentration and global temperature. A set of Representative Concentration Pathways (RCP) describes some possible climate scenarios that can be closely related to the future level of anthropocentric emissions, but also to implications on the water system, such as changes in precipitations, runoff and evapotranspiration for plants Van Vuuren et al. (2011). All these aspects are more or less included in the CLEW models that link land use and vegetation modules to other sectors.
Figure 3 (and Supplementary Table S1) show the balance between the level of detail for each sector in the considered models and offer a view on where there is potential for further development. It appears evident that most of the models adopt consistent simplification or lack of detail particularly in the water sector, followed by land. Smaller-scale models also reduce the scope in the energy sector. On the other hand, no models are lacking water and energy advanced features simultaneously, indicating that most models have a strong water or energy basis.
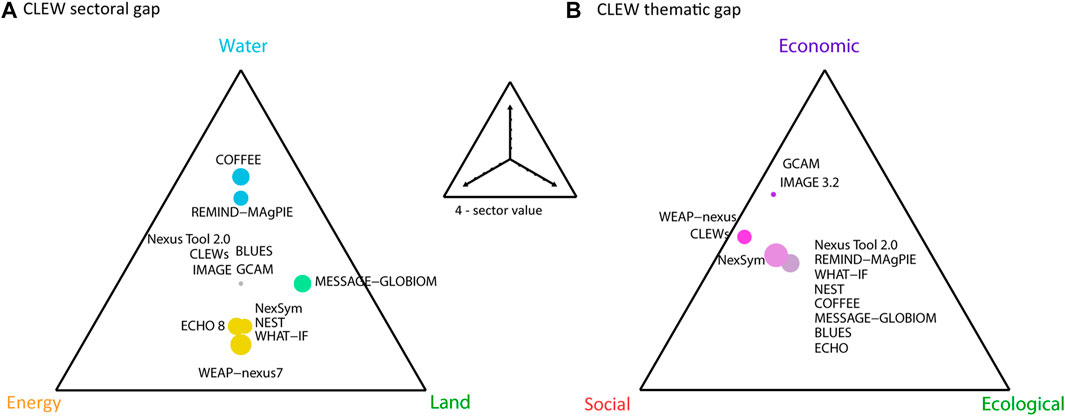
FIGURE 3. Sector gap, summary of simplifications of water, energy and land systems compared to advanced sectoral models (A). Thematic gap, simplifications or omission of possible social, economic and ecological aspects (B). Size depending on the total gap considering CLEW sectors (The explanatory diagram in the center refers to sector value in Supplementary Tables S1, 2).
4.3 Cross-Sector Interlinkages
As extensively mentioned above and in other literature, the innovation in CLEW models stands in the cross-sector interactions that have often been neglected in the past but are intrinsic in our system. It was also mentioned that there is a correlation between scales and interlinkages. While small-scale models tend to focus on specific nexus interactions that are present in the analyzed system (often neglecting climate aspects), national and basin models are those that integrate most interlinkages. Because of a relatively detailed resolution in hydrology and crop modeling, interlinkages of country and basin models are also quite detailed (Figure 2 and Supplementary Table three). Global models need to deal with a much larger scope and adding a high number of interconnection might increase significantly the complexity. In addition, soft-linked models require approximation of flows, costs, or commodity levels that are exchanged between modules. While integrated models have often reduced representation thereby making it difficult to endogenize nexus interactions.
Accounting for energy used in pumping and water distribution technologies, water for energy purposes (hydropower or cooling) and water for agriculture are the most represented nexus linkages (Figure 4). This is followed by biomass for energy production and accounting for GHG emissions from the energy sector. Also climate impacts, especially on the water cycle, are well embedded in many models. A slightly less explored aspects are the energy impacts in agriculture and land use, aside from biomass competition for food crops, and their consequences on the climate.
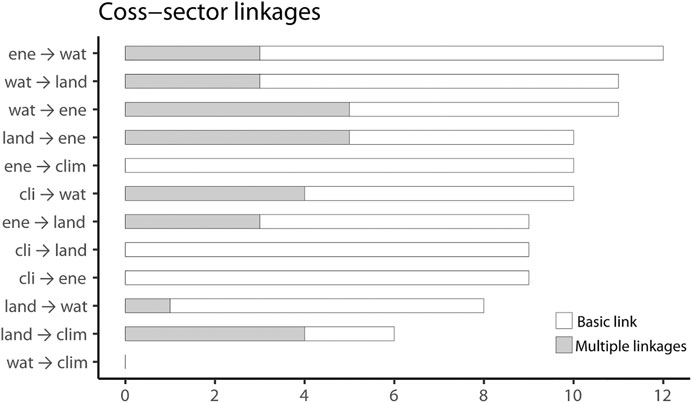
FIGURE 4. Number of models including interlinkages across sectors with level of detail based on Supplementary Table S3.
What is ignored by all the models is any possible influence of changes in water management, such as creation of new storage reservoirs, river diversion, or increased irrigation on local and global climate.
4.4 Geophysical Bias
In conclusion to the previous paragraphs, it appears evident that the spatial and temporal scope, the resolution and the detail of sectoral representation, and the cross-sector interlinkages are all closely related to the scale of models. This influences the research questions that can be properly answered. Most of the models considered have strong geophysical representations, which means natural systems such as water bodies and vegetation are represented with high detail. Also human interactions with such systems are clearly well represented in all of the CLEW sectors as a force driving change.
Ecosystem services, biodiversity loss are often included in nexus analysis, but only in a limited number of cases, and lacking detail, for instance of species loss and aquatic ecosystem Holland et al. (2018); Hülsmann et al. (2019); Díaz et al. (2020).
Comprehensively, CLEW models are well suited for dealing with environmental questions, the relation between our society and the Earth system, primary markets (e.g., electricity, heat, food), and, to some extent, ecosystem challenges. This is confirmed by looking at Table 2 where modellers were asked to state what is the best use for their models (“Question best answer” column) and all referred to the relationship between human development and the geophysical system. Liu et al. (2018a) also point out that the CLEW approach is centered on natural systems and it is harder to relate with other social and economic aspects of our society, such as education, equality or institutions Liu et al. (2018a).
Nevertheless, several studies have focused on inequality implications such as hunger, energy access and water access, also under the perspective of climate change Rosenberg et al. (2020); Fujimori et al. (2019); Cetrulo et al. (2020). But IAM still struggle to fully represent inequality aspects, and this challenge increases when multiple sectors are under scope Rao et al. (2017). Similarly, other social aspects, that often dominate the daily political discussion, and therefore are strong drivers of policy and change, are also poorly embedded in CLEW models. Aspects such as public acceptance or environmental policies and investments, or climate-induced migration are widely explored in the literature, also with quantitative methods, but indirectly considered in most CLEW studies Davis et al. (2018); Stigka et al. (2014). The major input for social aspects in CLEW models comes from stakeholders that are often engaged to shape appropriate scenarios.
Economic aspects, such as decisions or behavior of individuals and companies, governments and finance, are not at all represented in the models here considered. In particular, while assessing a large range of environmental and development policies, CLEW models do not focus on how such policies could be financed. This is an enormous implementation barrier that is explored in the literature, contemplating microfinancing, and financing for international agreements. For example, the IAM community often represents climate mitigation policies as perfectly implemented through global carbon prices, which in reality is far from being achievable. Li et al. (2017) developed an Agent Based Model (ABM) that mimics the interactions between governments, individuals and companies with regards to water, energy and food consumption Li et al. (2017). Hyun et al. (2019) developed the ABM–RiverWare that connects a hydrological model to an ABM to study the change of farmers’ water withdrawals under different climate-change related risk and socioeconomic scenarios Hyun et al. (2019); Yang et al. (2020).
Figure 3B shows this asymmetry in terms of inclusion of societal and economic problems. Most of the models here considered link to macro-economic indicators of country or global GDP under the scenario framework of the Shared Socioeconomic Pathways Riahi et al. (2017) to assess investments, cost of resources or commodities (i.e., water, equivalent carbon). Clearly, additional approaches, such as linking with ABM could complement the CLEW analysis, improving feasibility, acceptability and implementation aspects of policy results.
4.5 Model Structure and Nexus Integration
The models considered in this article present mostly two types of solution methods, simulation and optimization. Simulation models are used to predict possible effects and implications across different variables and sectors, based on input assumptions that could simulate policies. For instance, given exogenous input demands, costs and share of technology use, the models can simulate the functioning of the system and calculate outputs, in terms of resource consumption, cross-sectoral demands, and so on.
Optimization models normally perform dynamic optimization that minimizes or maximizes a specific utility function and find the optimal solution of the system under exogenous inputs and constraints, which could mimic specific policies. Such models tend to find the “optimal” system configuration as a unique solution and are often used to assess possible capacity expansion portfolios, investment costs, and also assess resource used across sectors.
Several solution methods and algorithms exist, are used and changed by the developers’ teams. However, it does not seem to be a major issue of discussion among the modeling limitation of CLEWs models. Various approaches are therefore interchangeable and often compared in literature (see column Structure in Table 2Hanssen et al. (2020). One limitation for large optimization models that process a large amount of data, might be to find free advanced solvers that also guarantee reduced solution time.
A different aspect that can be related to uncertainty and limitations, especially in the CLEW system, is the way different sectors are connected. Here, the majority of cases differ between soft-linked separate models, fully integrated models, or a hybrid of the two (see column Nexus integration in Table 2).
Having soft-linked models allows the use of pre-existing models, likely one-sector models, that might also have a high level of sectoral detail. However, to make different models work together, an exchange of information is required through an iterative process to convergence. For instance, a hydrological model would pass information, probably in aggregated form, to a crop-growth model, which would take water availability and evapotranspiration as input, and would determine crop production. These results would then need to iterate back to the water model to update the previous runs with the new water demand assumptions, which would then give new hydrological output. This iterative process can become particularly complicated when more than two models are linked, such as energy system or climate models, and requires substantial work in setting up the input-output exchange and the iterative procedure.
Integrated models, on the other hand, incorporate all the possible representations in one unique large system, with the advantage of reducing effort and simplifications related to transferring information across modules and avoiding iterations. This also reduces particularly the uncertainty around the connection between different modules, which is in this case endogenized. However, integrated models might become very large models, heavy to work with and requiring high multi-disciplinary competencies. This limitation might result in a more simplified or unbalanced representation of each module. This is for instance noticeable in some of the global IAM models, historically energy-climate models, which have later added water and land features, initially simplified, and then further improved. This trade-off of integrated models is less of an issue in CLEW models where the focus is more on the cross-sectoral dynamics than on the detailed processes within each sector.
4.6 Availability, Accessibility and Usability
In recent years the scientific community and modeling teams have pushed significantly toward open-access information, both in terms of publications and making the tools available for free to external users. This has allowed spreading the use of models to different teams without the need to dedicate new funds and time to re-develop complex models. These spillovers sometimes resulted in new versions of the models (e.g., COFFE and BLUES are modified version of the MESSAGE-GLOBIOM model, and from those, the model ELENA was developed Bataille et al. (2020)) or the creation of proper international communities that also contribute to the development of the tools (an example of the OSeMOSYS energy system model, part of the CLEWS framework that has been adopted and developed in numerous country studies Howells et al. (2011)).
Previous nexus model intercomparison studies assessed the complexity of models by looking at both methodological aspects (data, sector coverage) and accessibility, aspects Dargin et al. (2019). For this article, we disentangle those aspects thinking that also very complex models can be made easily available and usable for different audiences. Here, we consider only models that are currently available, maintained, or still actively used, so to better focus on the factors that could contribute to further development.
The rationale for more open-access research and tools is to make them available, accessible, understandable and reusable. In the modeling world, this means having an easy-to-find online repository where the model is downloadable and having clear documentation that explains the model in detail and how to use it. Additional tutorials, testing infrastructure and community forums to engage with developers are also assets that make a significant difference. In fact, the open-access trend has often lead to simple online repositories where code and data are stored without thorough documentation and therefore not particularly useful for external users. This is an issue in particular in small projects or early research, where time and resources are scarce Allen and Mehler (2019).
Scanning through the models considered in the review, it is noticeable that several models completely lack online repository and documentation (Figure 5). Only a few are fully accessible, documented and usable for free. One of the main limitations, especially for large global models, is the lack of public model data, which shareability is often constraint by third parties. Modeling teams that can afford it (mostly teams maintaining global models), find solutions like graphic interfaces (IMAGE, GCAM) or data and results explorers (MESSAGE, CLEWs) to enable the users to run the models or access data stored on the developers’ servers.
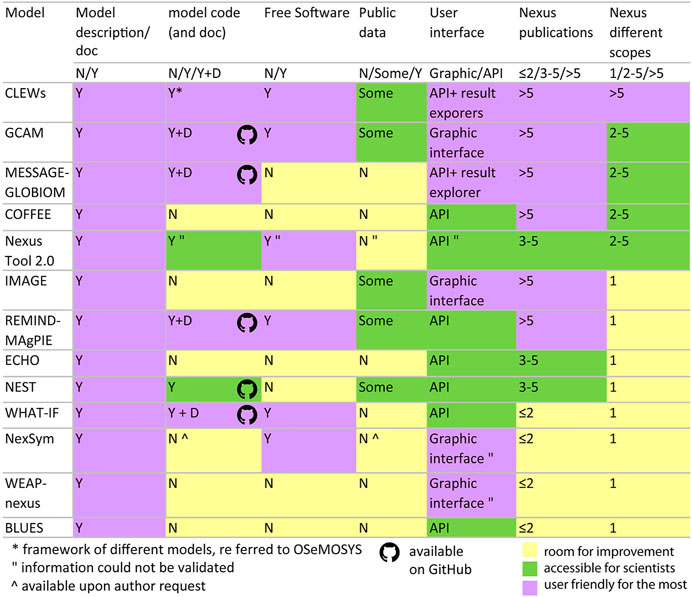
FIGURE 5. Summary of aspects that define accessibility of the model, data and software, as well as proxies of previous applications. The colors highlight features that are user-friendly for a generic audience to those requiring more scientific and technical skills.
Considering the number of publications and the number of applications of those nexus models to different scopes, we notice that actually open-access is not the primary factor for widespread utilization. Although Figure 5 shows that open-access models (mostly global models, with the exception of CLEWs) are also quite well known and utilized (last two columns on the right). It must be noted that in most of the case the modeling teams have moved to open-access only in the last couple of years. Or, in the case of the CLEWs framework, not all model configurations associated with individual studies are openly shared, and a large amount of the resulting research is not peer-reviewed but is rather gray literatureRamos et al. (2020). For these reasons, the correlation between model scale and accessibility might change in the future as small-scale models spread more widely. Nonetheless, those models that grant high visibility, and that have been utilized widely around the world can be probably associated with the large community support that has been promoted over years, together with capacity building activities, and engagement with stakeholders. In conclusion, while open-access is important and need to be promoted with adequate time and resources, other factors like stakeholder engagement and capacity building are driving forces in connecting modellers groups, and shaping existing model into new prototypes, or case studies.
5 Discussion: Emerging Gaps and Way Forward
The comparison of the thirteen CLEW models (or family of models) presented above allows identification of areas that would most benefit from new developments, while providing a transparent assessment of usability and availability for scientists and non-scientist users.
The survey of CLEW models at different scales also highlighted some of the main limitations regarding methodology, sectoral coverage and nexus interlinkages (summarized in Table 3), and socioeconomic components.
5.1 Methodological Limitations
The major methodological limitation among CLEW models are the following.
• Soft-linked modules suffer mostly from the simplification of the data and scope of information exchanged between models, often reduced to one or two variables (e.g., price) and from long iteration time.
• Integrated models, particularly at the global scale, tend to simplify some nexus interlinkages and/or the sectoral representation; although continuous progress in computing power has allowed increasingly higher model complexity.
• Boundary conditions are a critical source of uncertainty for country, basin and local-scale models.
The trend for integrated models is to increase detail in each sector with more detailed spatial data and increased resolution.
5.2 Sectoral Representation and Nexus Interlinkages
Depending on the scale of representation we noticed that the details of the sectoral representation or nexus interlinkages vary, sometimes rationally with the model scope and questions that can be addressed, but in other cases in a relatively ad-hoc approach to address complexity issues. Below follows a list of the major simplifications in sectoral representation.
• Local models tend to neglect or simplify interactions with climate and focus only on the main nexus feedbacks relevant to the system.
• At the basin and national scale all CLEW interactions become relevant. In fact models at these scales include the largest number of interconnections and allow for detailed spatial representation of water and land systems.
• Full CLEW representation is also highly relevant for global models, but to make the models tractable complexity trade-offs that complicate the modeling are necessary. Only a few teams can have the necessary coverage of expertize, data and computing power to build and run such large integrated models.
• Major gaps in sectoral implementation are in hydrology assessment (particularly groundwater), and in primary energy linkage to natural resources. The water-energy nexus is represented quite adequately in most of the models.
5.3 CLEW Linkage to Sustainable Development Goals and Challenges
Several studies in the literature show how models on climate, land, energy and water nexus can inform planning, policy exploration and risk assessment in regards to the water, food, energy and climate SDGs Liu et al. (2018a); Ramos et al. (2020). Depending on the specific model, some have a stronger focus on water or food scarcity, environmental protection, or soil and air pollution. Therefore, linkages to other SDGs such as industry and infrastructure, poverty, economic growth and life on land can also be explored with existing tools.
Social aspects such as equity, health and human rights, as well as economic aspects, e.g., governments and finance, are not included in any of the existing CLEW models, although there is some evidence these are also related to the CLEW sectors. Other types of models already explore some of these dimensions, but they are not yet linked to proper geophysical assessment tools like the CLEW models discussed here.
In terms of heterogeneity of CLEW model applications, most of the recently developed models focus on an area where climate change impacts are projected to be high and CLEW interlinkages are highly relevant. Several models explore case studies in Africa (ECHO, CLEWS, WHAT-IF), south Asia (NEST) and South America (BLUES, ELENA Bataille et al. (2020)). Moreover, current global models do now provide tools to zoom in the analysis to single countries or basins (IMAGE, GCAM).
5.4 The Lack of Common Assumptions
To better address socioeconomic and climate uncertainty, the IAM community has agreed on common scenarios called Shared socioeconomic Pathways (SSP) Riahi et al. (2017). Originally the SSPs defined five storylines with different trends of population and GDP growth, and assumptions concerning technologies in the energy sector. The SSPs represent different challenges for mitigation and adaptation to climate change. Almost all the studies mentioned in this article refer to SSPs, providing a multi basis for result comparisons.
The same community has subsequently introduced assumptions on global land use Popp et al. (2017) with the intention of homogenizing assumptions on other sectors besides energy. Similarly, some teams have proposed SSP assumptions for water technologies Graham et al. (2018). But a holistic CLEW shared nexus perspective remains to be formulated within the SSPs. For example Momblanch et al., 2019 make assumptions for cropland and environmental flows that are not agreed upon within a community (as is for example the case for GDP and other indicators); this complicates the comparison of results with other models Momblanch et al. (2019). In addition, the SSPs provide publicly available databases with population and GDP trends that were originated at the macro-regional level. When focusing on national or regional scales, downscaling and further assumptions are required. Some recent work addresses this issue and proposes approaches in this direction Frame et al. (2018). Nonetheless, there is a need for high-resolution database for all SSP assumptions that cover all water, energy and land sectors that can be formally approved/agreed upon and referenced by all researchers working in this area.
5.5 Way Forward for Scientists and Modellers
Both global models and those focusing at smaller scales are increasingly reaching higher levels of detail in representing CLEW systems and cross-sector interlinkages. Parallel efforts should be directed to the reduction of existing uncertainties related to geographical boundary conditions, interactions between sub-modules and downscaling.
Socioeconomic aspects need to be better incorporated or connected to CLEW models to improve assessment of the feasibility and impact of policies and understanding of the social implications of future investments. Stakeholder engagement during scenario development and results analysis is currently used for validating scenarios analyzed with CLEW models. Further linkings with social-science experts, ABM representing household-companies-governments interactions, or finance risk models would be avenues to be explored in the future and provide further assets to the relevance of CLEW models.
5.6 The Right Model for the Right User
There is not a better or worse model but, based on the research questions, the users, the scale, accessibility and data some models are more suitable to be used Daher et al. (2017). In this review we discussed models at different scales, highlighting the scope applications they can be used for. We also surveyed public availability in terms of source code, data, open-access software and appropriate documentation, as well as the strength of the online community supporting maintenance and new developments (Figure 5). It is clear that open access and transparency are important factors for model dissemination and use. However, other factors, such as a strong community and stakeholder engagement network can help spread the use of models and frameworks. Since CLEW models are new and in undergoing continuous development, it might be hasty to draw conclusions largely on accessibility visibility.
These are important considerations for scientists looking for state-of-the-art models to use or compare. These considerations could also help decision makers, governments institution, and other research and development institutions identify useful opportunities to pursue new climate land energy and water analyses to face regional, national, or global challenges and possibilities.
Author Contributions
AV and ND conceived of the manuscript research questions. AV led the literature review and analysis, the preparation of figures, data tables, and the writing of the manuscript. All the authors contributed providing feedback and to review the manuscript.
Funding
Some authors received funding from the European Union’s Horizon 2020 research and innovation program under grant agreements no. 821124 (NAVIGATE). This work was supported in part by the Natural Sciences and Engineering Research Council of Canada.
Conflict of Interest
The authors declare that the research was conducted in the absence of any commercial or financial relationships that could be construed as a potential conflict of interest.
Acknowledgments
The Authors would like to acknowledge all the researchers and modellers who have helped validate and improve the information supporting this review by answering a questionnaire and providing further information: Pedro Rochedo, Raphaël Payet-Burin, Katherine Calvin, Jennie Rice, Eunice Pereira Ramos, Detlef van Vuuren, Elias Martinez Hernandez and Zarrar Khan.
Supplementary Material
The Supplementary Material for this article can be found online at: https://www.frontiersin.org/articles/10.3389/fenvs.2021.691523/full#supplementary-material
References
Akhtari, S., Sowlati, T., and Griess, V. C. (2018). Integrated Strategic and Tactical Optimization of forest-based Biomass Supply Chains to Consider Medium-Term Supply and Demand Variations. Appl. Energ. 213, 626–638. doi:10.1016/j.apenergy.2017.10.017
Albrecht, T. R., Crootof, A., and Scott, C. A. (2018). The Water-Energy-Food Nexus: A Systematic Review of Methods for Nexus Assessment. Environ. Res. Lett. 13, 043002. doi:10.1088/1748-9326/aaa9c6
Allen, C., and Mehler, D. M. A. (2019). Open Science Challenges, Benefits and Tips in Early Career and beyond. Plos Biol. 17, e3000246. doi:10.1371/journal.pbio.3000246
Bataille, C., Waisman, H., Briand, Y., Svensson, J., Vogt-Schilb, A., Jaramillo, M., et al. (2020). Net-zero Deep Decarbonization Pathways in Latin America: Challenges and Opportunities. Energ. Strategy Rev. 30, 100510. doi:10.1016/j.esr.2020.100510
Bauer, N., Hilaire, J., Brecha, R. J., Edmonds, J., Jiang, K., Kriegler, E., et al. (2016). Assessing Global Fossil Fuel Availability in a Scenario Framework. Energy 111, 580–592. doi:10.1016/j.energy.2016.05.088
Bauer, N., Klein, D., Humpenöder, F., Kriegler, E., Luderer, G., Popp, A., et al. (2020). Bio-energy and CO2 Emission Reductions: an Integrated Land-Use and Energy Sector Perspective. Climatic Change 163, 1675–1693. doi:10.1007/s10584-020-02895-z
Bazilian, M., Rogner, H., Howells, M., Hermann, S., Arent, D., Gielen, D., et al. (2011). Considering the Energy, Water and Food Nexus: Towards an Integrated Modelling Approach. Energy Policy 39, 7896–7906. doi:10.1016/j.enpol.2011.09.039
Bieber, N., Ker, J. H., Wang, X., Triantafyllidis, C., van Dam, K. H., Koppelaar, R. H. E. M., et al. (2018). Sustainable Planning of the Energy-Water-Food Nexus Using Decision Making Tools. Energy Policy 113, 584–607. doi:10.1016/j.enpol.2017.11.037
Biggs, E. M., Bruce, E., Boruff, B., Duncan, J. M. A., Horsley, J., Pauli, N., et al. (2015). Sustainable Development and the Water-Energy-Food Nexus: A Perspective on Livelihoods. Environ. Sci. Pol. 54, 389–397. doi:10.1016/j.envsci.2015.08.002
Bijl, D. L., Biemans, H., Bogaart, P. W., Dekker, S. C., Doelman, J. C., Stehfest, E., et al. (2018a). A Global Analysis of Future Water Deficit Based on Different Allocation Mechanisms. Water Resour. Res. 54, 5803–5824. doi:10.1029/2017WR021688
Bijl, D. L., Bogaart, P. W., Dekker, S. C., and van Vuuren, D. P. (2018b). Unpacking the Nexus: Different Spatial Scales for Water, Food and Energy. Glob. Environ. Change 48, 22–31. doi:10.1016/j.gloenvcha.2017.11.005
Broeer, T., Fuller, J., Tuffner, F., Chassin, D., and Djilali, N. (2014). Modeling Framework and Validation of a Smart Grid and Demand Response System for Wind Power Integration. Appl. Energ. 113, 199–207. doi:10.1016/j.apenergy.2013.06.058
Buras, N. (1979). “Determining the Feasibility of Incorporating Water Resource Constraints into Energy Models,” in NASA STI/Recon Technical Report N 80, August 1979, (CA, United States: Department of Operations Research, Final Report Stanford University).
Burek, P., Satoh, Y., Kahil, T., Tang, T., Greve, P., Smilovic, M., et al. (2019). Development of the Community Water Model (CWatM v1.04) A High-Resolution Hydrological Model for Global and Regional Assessment of Integrated Water Resources Management. Geoscientific Model. Develop. Discuss. 13, 1–49. doi:10.5194/gmd-2019-214
Calvin, K., Patel, P., Clarke, L., Asrar, G., Bond-Lamberty, B., Cui, R. Y., et al. (2019). GCAM v5.1: Representing the Linkages between Energy, Water, Land, Climate, and Economic Systems. Geosci. Model. Dev. 12, 677–698. doi:10.5194/gmd-12-677-2019
Cetrulo, T. B., Marques, R. C., Malheiros, T. F., and Cetrulo, N. M. (2020). Monitoring Inequality in Water Access: Challenges for the 2030 Agenda for Sustainable Development. Sci. Total Environ. 727, 138746. doi:10.1016/j.scitotenv.2020.138746
Chen, M., Vernon, C. R., Huang, M., Calvin, K. V., and Kraucunas, I. P. (2019). Calibration and Analysis of the Uncertainty in Downscaling Global Land Use and Land Cover Projections from GCAM Using Demeter (v1.0.0). Geosci. Model. Dev. 12, 1753–1764. doi:10.5194/gmd-12-1753-2019
Daher, B., Mohtar, R., Lee, S.-H., and Assi, A. (2017). Modeling the Water-Energy-Food Nexus: A 7-Question Guideline. TX, United States: Texas A&M University System (TAMUS).
Daher, B. T., and Mohtar, R. H. (2015). Water-energy-food (WEF) Nexus Tool 2.0: Guiding Integrative Resource Planning and Decision-Making. Water Int. 40, 748–771. doi:10.1080/02508060.2015.1074148
Dai, J., Wu, S., Han, G., Weinberg, J., Xie, X., Wu, X., et al. (2018). Water-energy Nexus: A Review of Methods and Tools for Macro-Assessment. Appl. Energ. 210, 393–408. doi:10.1016/J.APENERGY.2017.08.243
Dalin, C., Wada, Y., Kastner, T., and Puma, M. J. (2017). Groundwater Depletion Embedded in International Food Trade. Nature 543, 700–704. doi:10.1038/nature21403
Dargin, J., Daher, B., and Mohtar, R. H. (2019). Complexity versus Simplicity in Water Energy Food Nexus (WEF) Assessment Tools. Sci. Total Environ. 650, 1566–1575. doi:10.1016/j.scitotenv.2018.09.080
Davis, K. F., Bhattachan, A., D’Odorico, P., and Suweis, S. (2018). A Universal Model for Predicting Human Migration under Climate Change: Examining Future Sea Level Rise in Bangladesh. Environ. Res. Lett. 13, 064030. doi:10.1088/1748-9326/aac4d4
de Carvalho Köberle, A. (2018). “Implementation of Land Use in an Energy System Model to Study the Long-Term Impacts of Bioenergy in Brazil and its Sensitivity to the Choice of Agricultural Greenhouse Gas Emission Factors,” (Rio de Janeiro: Universidade Federal do Rio de Janeiro). Ph.D. thesis.
Díaz, S., Zafra-Calvo, N., Purvis, A., Verburg, P. H., Obura, D., Leadley, P., et al. (2020). Set Ambitious Goals for Biodiversity and Sustainability. Science 370, 411–413. doi:10.1126/science.abe1530
Dietrich, J. P., Bodirsky, B. L., Humpenöder, F., Weindl, I., Stevanović, M., Karstens, K., et al. (2019). MAgPIE 4 - a Modular Open-Source Framework for Modeling Global Land Systems. Geosci. Model. Dev. 12, 1299–1317. doi:10.5194/gmd-12-1299-2019
Dodder, R. S., Barnwell, J. T., and Yelverton, W. H. (2016). Scenarios for Low Carbon and Low Water Electric Power Plant Operations: Implications for Upstream Water Use. Environ. Sci. Technol. 50, 11460–11470. doi:10.1021/acs.est.6b03048
Dubreuil, A., Assoumou, E., Bouckaert, S., Selosse, S., and Maïzi, N. (2013). Water Modeling in an Energy Optimization Framework - the Water-Scarce Middle East Context. Appl. Energ. 101, 268–279. doi:10.1016/j.apenergy.2012.06.032
Endo, A., Burnett, K., Orencio, P., Kumazawa, T., Wada, C., Ishii, A., et al. (2015). Methods of the Water-Energy-Food Nexus. Water 7, 5806–5830. doi:10.3390/w7105806
Engström, R., Destouni, G., Howells, M., Ramaswamy, V., Rogner, H., Bazilian, M., et al. (2019). Cross-Scale Water and Land Impacts of Local Climate and Energy Policy-A Local Swedish Analysis of Selected SDG Interactions. Sustainability 11, 1847. doi:10.3390/su11071847
Eyring, V., Bony, S., Meehl, G. A., Senior, C. A., Stevens, B., Stouffer, R. J., et al. (2016). Overview of the Coupled Model Intercomparison Project Phase 6 (CMIP6) Experimental Design and Organization. Geosci. Model. Dev. 9, 1937–1958. doi:10.5194/gmd-9-1937-2016
Fader, M., Cranmer, C., Lawford, R., and Engel-Cox, J. (2018). Toward an Understanding of Synergies and Trade-Offs between Water, Energy, and Food SDG Targets. Front. Environ. Sci. 6, 112. doi:10.3389/fenvs.2018.00112
Frame, B., Lawrence, J., Ausseil, A.-G., Reisinger, A., and Daigneault, A. (2018). Adapting Global Shared Socio-Economic Pathways for National and Local Scenarios. Clim. Risk Manage. 21, 39–51. doi:10.1016/J.CRM.2018.05.001
Fujimori, S., Hasegawa, T., Krey, V., Riahi, K., Bertram, C., Bodirsky, B. L., et al. (2019). A Multi-Model Assessment of Food Security Implications of Climate Change Mitigation. Nat. Sustain. 2, 386–396. doi:10.1038/s41893-019-0286-2
Gernaat, D. E. H. J., Bogaart, P. W., Vuuren, D. P. v., Biemans, H., and Niessink, R. (2017). High-resolution Assessment of Global Technical and Economic Hydropower Potential. Nat. Energ. 2, 821–828. doi:10.1038/s41560-017-0006-y
Gleeson, T., and Richter, B. (2018). How Much Groundwater Can We Pump and Protect Environmental Flows through Time? Presumptive Standards for Conjunctive Management of Aquifers and Rivers. River Res. Applic 34, 83–92. doi:10.1002/rra.3185
Graham, N. T., Davies, E. G. R., Hejazi, M. I., Calvin, K., Kim, S. H., Helinski, L., et al. (2018). Water Sector Assumptions for the Shared Socioeconomic Pathways in an Integrated Modeling Framework. Water Resour. Res. 54, 6423–6440. doi:10.1029/2018WR023452
Graham, N. T., Hejazi, M. I., Kim, S. H., Davies, E. G. R., Edmonds, J. A., and Miralles-Wilhelm, F. (2020). Future Changes in the Trading of Virtual Water. Nat. Commun. 11, 1–7. doi:10.1038/s41467-020-17400-4
Grubler, A., Wilson, C., Bento, N., Boza-Kiss, B., Krey, V., McCollum, D. L., et al. (2018). A Low Energy Demand Scenario for Meeting the 1.5 °C Target and Sustainable Development Goals without Negative Emission Technologies. Nat. Energ. 3, 515–527. doi:10.1038/s41560-018-0172-6
Hanssen, S. V., Daioglou, V., Steinmann, Z. J. N., Frank, S., Popp, A., Brunelle, T., et al. (2020). Biomass Residues as Twenty-First century Bioenergy Feedstock-A Comparison of Eight Integrated Assessment Models. Climatic Change 163, 1569–1586. doi:10.1007/s10584-019-02539-x
Hartin, C. A., Patel, P., Schwarber, A., Link, R. P., and Bond-Lamberty, B. P. (2015). A Simple Object-Oriented and Open-Source Model for Scientific and Policy Analyses of the Global Climate System - Hector v1.0. Geosci. Model. Dev. 8, 939–955. doi:10.5194/gmd-8-939-2015
Havlík, P., Valin, H., Mosnier, A., Frank, S., Lauri, P., Leclère, D., et al. (2018). GLOBIOM Documentation. Laxenburg, Austria: International Institute for Applied Systems Analysis.
Hejazi, M., Edmonds, J., Clarke, L., Kyle, P., Davies, E., Chaturvedi, V., et al. (2014). Long-term Global Water Projections Using Six Socioeconomic Scenarios in an Integrated Assessment Modeling Framework. Technol. Forecast. Soc. Change 81, 205–226. doi:10.1016/j.techfore.2013.05.006
Hejazi, M. I., Voisin, N., Liu, L., Bramer, L. M., Fortin, D. C., Hathaway, J. E., et al. (2015). 21st century united states Emissions Mitigation Could Increase Water Stress More Than the Climate Change it Is Mitigating. Proc. Natl. Acad. Sci. USA 112, 10635–10640. doi:10.1073/pnas.1421675112
Holland, R. A., Beaumont, N., Hooper, T., Austen, M., Gross, R. J. K., Heptonstall, P. J., et al. (2018). Incorporating Ecosystem Services into the Design of Future Energy Systems. Appl. Energ. 222, 812–822. doi:10.1016/j.apenergy.2018.04.022
Howells, M., Hermann, S., Welsch, M., Bazilian, M., Segerström, R., Alfstad, T., et al. (2013). Integrated Analysis of Climate Change, Land-Use, Energy and Water Strategies. Nat. Clim Change 3, 621–626. doi:10.1038/nclimate1789
Howells, M., Rogner, H., Strachan, N., Heaps, C., Huntington, H., Kypreos, S., et al. (2011). OSeMOSYS: The Open Source Energy Modeling System. Energy Policy 39, 5850–5870. doi:10.1016/j.enpol.2011.06.033
Huang, W., Ma, D., and Chen, W. (2017). Connecting Water and Energy: Assessing the Impacts of Carbon and Water Constraints on China's Power Sector. Appl. Energ. 185, 1497–1505. doi:10.1016/j.apenergy.2015.12.048
Hülsmann, S., Sušnik, J., Rinke, K., Langan, S., van Wijk, D., Janssen, A. B., et al. (2019). Integrated Modelling and Management of Water Resources: the Ecosystem Perspective on the Nexus Approach. Curr. Opin. Environ. Sustainability 40, 14–20. doi:10.1016/j.cosust.2019.07.003
Hunt, J. D., Byers, E., Wada, Y., Parkinson, S., Gernaat, D. E. H. J., Langan, S., et al. (2020). Global Resource Potential of Seasonal Pumped Hydropower Storage for Energy and Water Storage. Nat. Commun. 11, 1–8. doi:10.1038/s41467-020-14555-y
Hyun, J.-Y., Huang, S.-Y., Yang, Y.-C. E., Tidwell, V., and Macknick, J. (2019). Using a Coupled Agent-Based Modeling Approach to Analyze the Role of Risk Perception in Water Management Decisions. Hydrol. Earth Syst. Sci. 23, 2261–2278. doi:10.5194/hess-23-2261-2019
IEA (2009). Seeking Sustainable Climate, Land, Energy and Water (CLEW) Strategies. Tech. rep., International Atomic Energy Agency.
IPCC (2014). “Climate Change 2014: Mitigation of Climate Change,” in Contribution of Working Group III to the Fifth Assessment Report of the Intergovernmental Panel on Climate Change. Editors O. Edenhofer, R. Pichs-Madruga, Y. Sokona, E. Farahani, S. Kadner, K. Seybothet al. (Cambridge, United Kingdom and New York, NY, United States: Cambridge University Press).
Jin, Q., Wei, J., Yang, Z.-L., and Lin, P. (2017). Irrigation-induced Environmental Changes Around the Aral Sea: An Integrated View from Multiple Satellite Observations. Remote Sensing 9, 900. doi:10.3390/rs9090900
Johnson, N., Burek, P., Byers, E., Falchetta, G., Flörke, M., Fujimori, S., et al. (2019). Integrated Solutions for the Water-Energy-Land Nexus: Are Global Models Rising to the Challenge? Water 11, 2223. doi:10.3390/w11112223
Johnson, N., Strubegger, M., McPherson, M., Parkinson, S. C., Krey, V., and Sullivan, P. (2017). A Reduced-form Approach for Representing the Impacts of Wind and Solar PV Deployment on the Structure and Operation of the Electricity System. Energ. Econ. 64, 651–664. doi:10.1016/j.eneco.2016.07.010
Jones, E. R., van Vliet, M. T. H., Qadir, M., and Bierkens, M. F. P. (2020). Spatially-explicit Estimates of Global Wastewater Production, Collection, Treatment and Re-use. Earth Syst. Sci. Data Discuss., 1–29. doi:10.5194/essd-2020-156
Kaddoura, S., and El Khatib, S. (2017). Review of Water-Energy-Food Nexus Tools to Improve the Nexus Modelling Approach for Integrated Policy Making. Environ. Sci. Pol. 77, 114–121. doi:10.1016/J.ENVSCI.2017.07.007
Kahil, T., Parkinson, S., Satoh, Y., Greve, P., Burek, P., Veldkamp, T. I. E., et al. (2018). A Continental‐Scale Hydroeconomic Model for Integrating Water‐Energy‐Land Nexus Solutions. Water Resour. Res. 54, 7511–7533. doi:10.1029/2017WR022478
Kernan, R., Liu, X., McLoone, S., and Fox, B. (2017). Demand Side Management of an Urban Water Supply Using Wholesale Electricity price. Appl. Energ. 189, 395–402. doi:10.1016/j.apenergy.2016.12.082
Keskinen, M., Guillaume, J., Kattelus, M., Porkka, M., Räsänen, T., Varis, O., et al. (2016). The Water-Energy-Food Nexus and the Transboundary Context: Insights from Large Asian Rivers. Water 8, 193. doi:10.3390/w8050193
Khan, Z., Linares, P., and García-González, J. (2017). Integrating Water and Energy Models for Policy Driven Applications. A Review of Contemporary Work and Recommendations for Future Developments. Renew. Sustain. Energ. Rev. 67, 1123–1138. doi:10.1016/j.rser.2016.08.043
Khan, Z., Linares, P., Rutten, M., Parkinson, S., Johnson, N., and García-González, J. (2018). Spatial and Temporal Synchronization of Water and Energy Systems: Towards a Single Integrated Optimization Model for Long-Term Resource Planning. Appl. Energ. 210, 499–517. doi:10.1016/j.apenergy.2017.05.003
Khan, Z., Wild, T., Vernon, C., Miller, A., Hejazi, M., Clarke, L., et al. (2020). Metis - A Tool to Harmonize and Analyze Multi-Sectoral Data and Linkages at Variable Spatial Scales. J. Open Res. Softw. 8, 10. doi:10.5334/jors.292
Köberle, A. C., Rochedo, P. R. R., Lucena, A. F. P., Szklo, A., and Schaeffer, R. (2020). Brazil's Emission Trajectories in a Well-Below 2 °C World: the Role of Disruptive Technologies versus Land-Based Mitigation in an Already Low-Emission Energy System. Climatic Change 162, 1823–1842. doi:10.1007/s10584-020-02856-6
Kraucunas, I., Clarke, L., Dirks, J., Hathaway, J., Hejazi, M., Hibbard, K., et al. (2015). Investigating the Nexus of Climate, Energy, Water, and Land at Decision-Relevant Scales: the Platform for Regional Integrated Modeling and Analysis (PRIMA). Climatic Change 129, 573–588. doi:10.1007/s10584-014-1064-9
Lall, U., and Mays, L. W. (1981). Model for Planning Water-Energy Systems. Water Resour. Res. 17, 853–865. doi:10.1029/wr017i004p00853
Li, G., Wang, Y., Huang, D., and Yang, H. (2017). Water-energy-food Nexus in Urban Sustainable Development: an Agent-Based Model. Int. J. Crowd Sci. 1, 121–132. doi:10.1108/IJCS-08-2017-0014
Li, X., Vernon, C. R., Hejazi, M. I., Link, R. P., Huang, Z., Liu, L., et al. (2018). Tethys - A Python Package for Spatial and Temporal Downscaling of Global Water Withdrawals. J. Open Res. Softw. 6, 9. doi:10.5334/jors.197
Li, X., Yang, L., Zheng, H., Shan, Y., Zhang, Z., Song, M., et al. (2019). City-level Water-Energy Nexus in Beijing-Tianjin-Hebei Region. Appl. Energ. 235, 827–834. doi:10.1016/j.apenergy.2018.10.097
Liu, J., Hull, V., Godfray, H. C. J., Tilman, D., Gleick, P., Hoff, H., et al. (2018a). Nexus Approaches to Global Sustainable Development. Nat. Sustain. 1, 466–476. doi:10.1038/s41893-018-0135-8
Liu, J., Mao, G., Hoekstra, A. Y., Wang, H., Wang, J., Zheng, C., et al. (2018b). Managing the Energy-Water-Food Nexus for Sustainable Development. Appl. Energ. 210, 1–4. doi:10.1016/j.apenergy.2017.10.064
Liu, J., Mooney, H., Hull, V., Davis, S. J., Gaskell, J., Hertel, T., et al. (2015). Systems Integration for Global Sustainability. Science 347, 1258832. doi:10.1126/science.1258832
Loulou, R., Goldstein, G., and Noble, K. (2004). Documentation for the MARKAL Family of Models, (Paris, France: Energy Technology Systems Analysis Program).
Martinez-Hernandez, E., Leach, M., and Yang, A. (2017). Understanding Water-Energy-Food and Ecosystem Interactions Using the Nexus Simulation Tool NexSym. Appl. Energ. 206, 1009–1021. doi:10.1016/J.APENERGY.2017.09.022
Matsumoto, J., and Mays, L. W. (1983). Capacity Expansion Model for Large-Scale Water-Energy Systems. Water Resour. Res. 19, 593–607. doi:10.1029/wr019i003p00593
McCollum, D. L., Echeverri, L. G., Busch, S., Pachauri, S., Parkinson, S., Rogelj, J., et al. (2018). Connecting the Sustainable Development Goals by Their Energy Inter-linkages. Environ. Res. Lett. 13, 033006. doi:10.1088/1748-9326/aaafe3
Mesfun, S., Leduc, S., Patrizio, P., Wetterlund, E., Mendoza-Ponce, A., Lammens, T., et al. (2018). Spatio-temporal Assessment of Integrating Intermittent Electricity in the EU and Western Balkans Power Sector under Ambitious CO2 Emission Policies. Energy 164, 676–693. doi:10.1016/j.energy.2018.09.034
Momblanch, A., Papadimitriou, L., Jain, S. K., Kulkarni, A., Ojha, C. S. P., Adeloye, A. J., et al. (2019). Untangling the Water-Food-Energy-Environment Nexus for Global Change Adaptation in a Complex Himalayan Water Resource System. Sci. Total Environ. 655, 35–47. doi:10.1016/J.SCITOTENV.2018.11.045
Nakayama, M., Salewicz, K. A., Khatib, S. T. E., Kikuchi, R., and Sakamoto, M. (2015). Water-Energy-Agriculture Nexus in Vakhsh River Basin of Tajikistan. Tokyo, Japan: Graduate School of Frontier Sciences, The University of Tokyo.
Nilsson, M., Griggs, D., and Visbeck, M. (2016). Policy: Map the Interactions between Sustainable Development Goals. Nature 534, 320–322. doi:10.1038/534320a
Oikonomou, K., and Parvania, M. (2019). Optimal Coordination of Water Distribution Energy Flexibility with Power Systems Operation. IEEE Trans. Smart Grid 10, 1101. doi:10.1109/TSG.2018.2824308
Parkinson, S. C., Djilali, N., Krey, V., Fricko, O., Johnson, N., Khan, Z., et al. (2016). Impacts of Groundwater Constraints on Saudi Arabia's Low-Carbon Electricity Supply Strategy. Environ. Sci. Technol. 50, 1653–1662. doi:10.1021/acs.est.5b05852
Parkinson, S., Krey, V., Huppmann, D., Kahil, T., McCollum, D., Fricko, O., et al. (2019). Balancing Clean Water-Climate Change Mitigation Trade-Offs. Environ. Res. Lett. 14, 014009. doi:10.1088/1748-9326/aaf2a3
Payet-Burin, R., Kromann, M., Pereira-Cardenal, S., Strzepek, K. M., and Bauer-Gottwein, P. (2019). WHAT-IF: an Open-Source Decision Support Tool for Water Infrastructure Investment Planning within the Water-Energy-Food-Climate Nexus. Hydrol. Earth Syst. Sci. 23, 4129–4152. doi:10.5194/hess-23-4129-2019
Pereira-Cardenal, S. J., Mo, B., Gjelsvik, A., Riegels, N. D., Arnbjerg-Nielsen, K., and Bauer-Gottwein, P. (2016). Joint Optimization of Regional Water-Power Systems. Adv. Water Resour. 92, 200–207. doi:10.1016/j.advwatres.2016.04.004
Pfenninger, S., and Staffell, I. (2016). Long-term Patterns of European PV Output Using 30 Years of Validated Hourly Reanalysis and Satellite Data. Energy 114, 1251–1265. doi:10.1016/j.energy.2016.08.060
Popp, A., Calvin, K., Fujimori, S., Havlik, P., Humpenöder, F., Stehfest, E., et al. (2017). Land-use Futures in the Shared Socio-Economic Pathways. Glob. Environ. Change 42, 331–345. doi:10.1016/J.GLOENVCHA.2016.10.002
Ramos, E. P., Howells, M., Sridharan, V., Engström, R. E., Taliotis, C., Mentis, D., et al. (2020). The Climate, Land, Energy, and Water Systems (CLEWs) Framework: a Retrospective of Activities and Advances to 2019. Environ. Res. Lett. 16, 033003. doi:10.1088/1748-9326/abd34f
Rao, N. D., Van Ruijven, B. J., Riahi, K., and Bosetti, V. (2017). Improving Poverty and Inequality Modelling in Climate Research. Nat. Clim Change 7, 857–862. doi:10.1038/s41558-017-0004-x
Riahi, K., van Vuuren, D. P., Kriegler, E., Edmonds, J., O’Neill, B. C., Fujimori, S., et al. (2017). The Shared Socioeconomic Pathways and Their Energy, Land Use, and Greenhouse Gas Emissions Implications: An Overview. Glob. Environ. Change 42, 153–168. doi:10.1016/J.GLOENVCHA.2016.05.009
Rising, J. (2020). Decision-making and Integrated Assessment Models of the Water-Energy-Food Nexus. Water Security 9, 100056. doi:10.1016/j.wasec.2019.100056
Rosenberg, M., Armanios, D. E., Aklin, M., and Jaramillo, P. (2020). Evidence of Gender Inequality in Energy Use from a Mixed-Methods Study in India. Nat. Sustain. 3, 110–118. doi:10.1038/s41893-019-0447-3
Santhosh, A., Farid, A. M., and Youcef-Toumi, K. (2014). Real-time Economic Dispatch for the Supply Side of the Energy-Water Nexus. Appl. Energ. 122, 42–52. doi:10.1016/j.apenergy.2014.01.062
Sattler, S., Macknick, J., Yates, D., Flores-Lopez, F., Lopez, A., and Rogers, J. (2012). Linking Electricity and Water Models to Assess Electricity Choices at Water-Relevant Scales. Environ. Res. Lett. 7, 045804. doi:10.1088/1748-9326/7/4/045804
Schaphoff, S., Von Bloh, W., Rammig, A., Thonicke, K., Biemans, H., Forkel, M., et al. (2018). LPJmL4 - A Dynamic Global Vegetation Model with Managed Land - Part 1: Model Description. Geosci. Model. Dev. 11, 1343–1375. doi:10.5194/gmd-11-1343-2018
Snyder, A., Calvin, K., Clarke, L., Edmonds, J., Kyle, P., Narayan, K., et al. (2020). The Domestic and International Implications of Future Climate for U.S. Agriculture in GCAM. PLOS ONE 15, e0237918. doi:10.1371/journal.pone.0237918
Sridharan, V., Shivakumar, A., Niet, T., Ramos, E. P., and Howells, M. (2020). Land, Energy and Water Resource Management and its Impact on GHG Emissions, Electricity Supply and Food Production- Insights from a Ugandan Case Study. Environ. Res. Commun. 2, 085003. doi:10.1088/2515-7620/abaf38
Stigka, E. K., Paravantis, J. A., and Mihalakakou, G. K. (2014). Social Acceptance of Renewable Energy Sources: A Review of Contingent Valuation Applications. Renew. Sustain. Energ. Rev. 32, 100–106. doi:10.1016/j.rser.2013.12.026
Strokal, M., Spanier, J. E., Kroeze, C., Koelmans, A. A., Flörke, M., Franssen, W., et al. (2019). Global Multi-Pollutant Modelling of Water Quality: Scientific Challenges and Future Directions. Curr. Opin. Environ. Sustainability 36, 116–125. doi:10.1016/j.cosust.2018.11.004
Vahmani, P., Jones, A. D., and Patricola, C. M. (2019). Interacting Implications of Climate Change, Population Dynamics, and Urban Heat Mitigation for Future Exposure to Heat Extremes. Environ. Res. Lett. 14, 084051. doi:10.1088/1748-9326/ab28b0
Vakilifard, N., A. Bahri, P., Anda, M., and Ho, G. (2019). An Interactive Planning Model for Sustainable Urban Water and Energy Supply. Appl. Energ. 235, 332–345. doi:10.1016/j.apenergy.2018.10.128
Van Vuuren, D. P., Bijl, D. L., Bogaart, P., Stehfest, E., Biemans, H., Dekker, S. C., et al. (2019). Integrated Scenarios to Support Analysis of the Food-Energy-Water Nexus. Nat. Sustain. 2, 1132–1141. doi:10.1038/s41893-019-0418-8
Van Vuuren, D. P., Edmonds, J., Kainuma, M., Riahi, K., Thomson, A., Hibbard, K., et al. (2011). The Representative Concentration Pathways: An Overview. Climatic change 109, 5–31. doi:10.1007/s10584-011-0148-z
Vinca, A., Parkinson, S., Byers, E., Burek, P., Khan, Z., Krey, V., et al. (2020a). The NExus Solutions Tool (NEST) v1.0: an Open Platform for Optimizing Multi-Scale Energy-Water-Land System Transformations. Geosci. Model. Dev. 13, 1095–1121. doi:10.5194/gmd-13-1095-2020
Vinca, A., Parkinson, S., Riahi, K., Byers, E., Siddiqi, A., Muhammad, A., et al. (2020b). Transboundary Cooperation a Potential Route to Sustainable Development in the Indus basin. Nat. Sustain. 4, 331–339. doi:10.1038/s41893-020-00654-7
Voisin, N., Dyreson, A., Fu, T., O'Connell, M., Turner, S. W. D., Zhou, T., et al. (2020). Impact of Climate Change on Water Availability and its Propagation through the Western U.S. Power Grid. Appl. Energ. 276, 115467. doi:10.1016/j.apenergy.2020.115467
Wang, X., Guo, M., Koppelaar, R. H. E. M., van Dam, K. H., Triantafyllidis, C. P., and Shah, N. (2018). A Nexus Approach for Sustainable Urban Energy-Water-Waste Systems Planning and Operation. Environ. Sci. Technol. 52, 3257–3266. doi:10.1021/acs.est.7b04659
Waughray, D. (2011). Water Security: The Water-Food-Energy-Climate Nexus. Washington, Covelo, London: The World Economic Forum.
Welsch, M., Deane, P., Howells, M., Ó Gallachóir, B., Rogan, F., Bazilian, M., et al. (2014). Incorporating Flexibility Requirements into Long-Term Energy System Models - A Case Study on High Levels of Renewable Electricity Penetration in Ireland. Appl. Energ. 135, 600–615. doi:10.1016/j.apenergy.2014.08.072
Wise, T. (2012). The Cost to Mexico of U.S. Corn Ethanol Expansion — Observatorio Económico Latinoamericano OBELA, (Medford, MA, United States: Tufts University).
Yang, Y. C. E., Son, K., Hung, F., and Tidwell, V. (2020). Impact of Climate Change on Adaptive Management Decisions in the Face of Water Scarcity. J. Hydrol. 588, 125015. doi:10.1016/j.jhydrol.2020.125015
Zhang, X., Li, H. Y., Leung, L. R., Liu, L., Hejazi, M. I., Forman, B. A., et al. (2020). River Regulation Alleviates the Impacts of Climate Change on U.S. Thermoelectricity Production. J. Geophys. Res. Atmos. 125, e2019JD031618. doi:10.1029/2019JD031618
Keywords: climate land energy water nexus, model comparison, multi-sector perspective, sustainable development, multi-scale models
Citation: Vinca A, Riahi K, Rowe A and Djilali N (2021) Climate-Land-Energy-Water Nexus Models Across Scales: Progress, Gaps and Best Accessibility Practices. Front. Environ. Sci. 9:691523. doi: 10.3389/fenvs.2021.691523
Received: 07 April 2021; Accepted: 21 June 2021;
Published: 02 July 2021.
Edited by:
Pete Falloon, Hadley Center, United KingdomReviewed by:
Rabi Mohtar, American University of Beirut, LebanonAlex Oriel Godoy, Universidad del Desarrollo, Chile
Copyright © 2021 Vinca, Riahi, Rowe and Djilali. This is an open-access article distributed under the terms of the Creative Commons Attribution License (CC BY). The use, distribution or reproduction in other forums is permitted, provided the original author(s) and the copyright owner(s) are credited and that the original publication in this journal is cited, in accordance with accepted academic practice. No use, distribution or reproduction is permitted which does not comply with these terms.
*Correspondence: Adriano Vinca, dmluY2FAaWlhc2EuYWMuYXQ=