- 1South China Sea Institute of Oceanology, Chinese Academy of Sciences, Guangzhou, China
- 2Daya Bay Marine Biology Research Station, Chinese Academy of Sciences, Shenzhen, China
- 3Southern Marine Science and Engineering Guangdong Laboratory (Guangzhou), Guangzhou, China
- 4Putian Institute of Aquaculture Science of Fujian Province, Putian, China
- 5Nansha Marine Ecological and Environmental Research Station, Chinese Academy of Sciences, Sansha, China
The role of microbial ecology in aquaculture is becoming increasingly significant; however, studies on the changes in microbial ecology driven by the culture environment are limited. In the present study, high-throughput sequencing and chemical analysis was used to explore changes in physicochemical factors, bacterial communities, and their relationships between a water source (Bay) and an aquaculture area located in a reclaimed area. Statistical analysis results revealed that operational taxonomic units levels in inlet water and pond water varied significantly (p < 0.05). Non-metric multidimensional scaling (NMDS) analysis revealed the distribution characteristics of bacterial communities with water properties. The abundance of Alphaproteobacteria, Actinobacteria, and Flavobacteria in pond water increased significantly when compared to inlet water. The abundance of heterotrophic bacteria, such as Candidatus Actinomarina, Candidatus Aquiluna, Marivita, and Vibrio genera in pond water was significantly higher (Welch’s t-tests, p < 0.05) than inlet water. Functional prediction analysis primarily revealed an increase in the function that was associated with carbon and nitrogen metabolism in the pond environment. Canonical correlation analysis revealed that the bacterial communities was predominantly influenced by inorganic nutrients. Nitrate-nitrogen (N), nitrite-N, ammonium-N, and phosphate-phosphorous (P) were the key factors influencing bacterial communities in pond environment. A significant correlation was observed between inorganic N and phosphorus (P), and dominant bacterial genera (p < 0.05), demonstrating the potential mechanism of regulation of nutrients in bacterial communities. The present study described the microbial ecology of aquaculture ponds in detail and provides a scientific basis for the management of aquacultural environments.
Introduction
Over the last few decades, offshore aquaculture and ocean fishing have not satisfied the dietary requirements of populations due to an increase in demand for seafood globally. In China, offshore aquaculture has developed rapidly, and large-scale aquaculture has become the most widespread offshore fish production system. The number of aquaculture species and levels of production have increased significantly. Aquaculture systems are relatively complex ecosystems with highly diverse bacterial communities, which influence nutrient cycling and energy flow in aquaculture ecosystems (Moriarty, 1997; Austin, 2006).
With the rapid development of coastal aquaculture, water quality is increasingly attracting the attention of stakeholders. During aquaculture activities, only a portion of the nutrients in the feed is consumed and assimilated by the culture animals, and most of the nutrients are retained in the aquaculture water environment (Thakur and Lin, 2003; Sahu et al., 2013). The residual nutrients could induce changes in the aquatic environment, for example, in the form of shifts in pH, dissolved oxygen (DO), and particulate organic matter, in addition to eutrophication (Martin et al., 1998), which could facilitate bacterial settlement and proliferation. Previous studies have revealed that the physical and chemical properties of water [pH, chemical oxygen demand (COD), temperature, salinity, phosphorus (P), and nitrogen (N)] influence bacterial communities in aquaculture environments (Zhang et al., 2016; Li et al., 2017; Alfiansah et al., 2018; Yang et al., 2018). The culture environment has been reported to exert considerable influence on the composition of intestinal bacteria and the occurrence of disease in aquatic species (Wang et al., 2014; Li et al., 2017; Yukgehnaish et al., 2020). Therefore, studying microbial ecology in aquaculture ecosystems is an effective approach to monitor the functioning of aquaculture systems and it provides valuable information that could facilitate the sustainable management of aquaculture systems (Sun et al., 2020b).
Although studies have examined the relationship between the aquaculture environment and bacterial communities (Zhang et al., 2016; Alfiansah et al., 2018; Sun et al., 2019; Sun et al., 2020b), the difference of bacterial communities between water source and aquaculture systems, and the factors driving this difference remain unclear. For aquaculture process, influent water quality and microbe have an important impact on the aquaculture area. Therefore, studying the changes and driving factors of microbial community structure is of great significance for disease prevention and water quality management. In the present study, combination of analytical chemistry and high-throughput sequencing to compare variations in physicochemical parameters and bacterial communities between the bay water and the aquaculture area. Furthermore, this study analyzed the correlation between physicochemical factors and bacterial communities, and finally determined the factors driving bacterial communities in the aquaculture systems.
Materials and Methods
Study Area and Sample Collection
Samples were collected from the Dengfeng mariculture in Fujian, China in December 2018. All ponds had the same water inlet from an adjacent Bay through which water entered the aquaculture area (Supplementary Figure S1). The area of each pond (shrimp-crab polyculture) is 33,300 m2-66,600 m2. Pond water in the reclaimed area was changed every 2 days, and subsequently comes out through the water outlet. The water inlet and outlet were far from each other to prevent water pollution. Six samples were collected near inlet area in the bay and pond water samples (n = 26) were collected from different ponds using a glass water hydrophore at 0.5 m depth. A total of 500 ml of water was filtered through 0.22-µm polycarbonate membranes (EMD Millipore, Billerica, MA, United States). The membranes were placed in 1.5 ml sterile microcentrifuge tubes. Filter membranes were stored in liquid nitrogen for subsequent DNA extraction.
Another 500 ml of each of the remaining water samples was filtered through a 0.45-μm filter and used to analyze inorganic nutrients. Nitrite-nitrogen (N), nitrate-N, ammonium-N, phosphate-phosphorus (P), and DO concentrations were determined according to the procedure described in “The specialties for marine monitoring” (GB17378.4–1998, China). Water salinity and pH were measured in the field using a YSI 6600 V2 Sonde water quality monitoring system (YSI Incorporated, Yellow Springs, OH, United States).
Illumina MiSeq Sequencing of Bacterial Communities
Total DNA was extracted from the filter membranes using a DNA Extraction Kit (Omega Bio-Tek, Norcross, GA, United States) according to the manufacturer’s instructions. The V3 and V4 regions of the 16S rRNA gene were amplified with the 319F (5′- ACTCCTACGGGAGGCAGCAG-3′) and 806R (5′-GGACTACHVG GGTWTCTAAT-3′) primers (Mateos-Rivera et al., 2016). The total volume of the PCR reaction system was 25 μL: 5×FastPfu buffer 4 μL, 2.5 mmol/L dNTPs 2 μL, 5 U/μL FastPfu polymerase 0.5 μL, primer (5.0 μmol/L) 1.0 μL and 10 ng template DNA. PCR reaction conditions were 95°C for 3 min, 30 cycles at 95°C for 30 s, 55°C for 30 s, 72°C for 45 s, and 72°C for 10 min. Different barcodes at both ends of the forward and reverse primers were used to distinguish samples. After amplification, the PCR products were purified and paired-end sequenced (2 × 250) on an Illumina MiSeq platform (Illumina, San Diego, CA, United States) according to standard protocols.
Data Analysis
For pair-ended reads obtained by Illumina MiSeq sequencing, the sequences were first classified according to barcode information and then spliced according to the overlap relationship. Barcodes and primers were trimmed from paired-end sequences and then assembled using FLASH (Magoč and Salzberg, 2011). Low quality sequences with ambiguous bases and average quality scores <30 was removed. All chimeric tags were removed using UCHIME algorithm (Edgar et al., 2011). Tags with sequence similarity greater than 97% were defined as OTUs clusters using the CD-HIT tool (Huang et al., 2010). The RDP classifier used the SILVA database (version 132), which had taxonomic categories predicted at the species level. Alpha diversity indices were calculated in QIIME 1.9.1 (Caporaso et al., 2010) from rarefied samples, and assessed using Shannon’s index and Chao1 index.
T-tests were used to assess the significant differences (p < 0.05) in bacterial OTUs between samples. NMDS (non-metric multi-dimensional scaling) of unweighted unifrac distances was generated in R Vegan package (version 2.5.3) (Oksanen et al., 2015) and plotted in R project ggplot2 (version 2.2.1) package (Kahle and Wickham, 2013). Canonical correspondence analysis (CCA) was executed in R project Vegan package (version 2.5.3) to clarify the influence of environmental factors on bacterial community composition. Pearson correlation analysis between genera abundance and environmental factor was calculated in R project psych package (version 1.8.4). PICRUSt2 (Douglas et al., 2020) was used to predict the functional profile of the bacterial communities in inlet water and pond water based on 16S rRNA sequences. Welch’s t-test (p < 0.05) was calculated in R project Vegan package (version 2.5.3) to determine if there were statistically significant differences in the prediction function of bacterial communities between inlet water and pond water.
Results
Variations in Key Physicochemical Factors Between Inlet Water and Pond Water
Water quality parameters are summarized in Figure 1 and Supplementary Table S1. Overall, t-test showed that pond water quality parameters exhibited distinct variations when compared to inlet water. Notably, nitrite-N (p = 0.001), nitrate-N (p = 0.008), ammonium-N (p = 0.008), phosphate-P (p = 0.003) and DO (p = 0.002) concentrations were significantly higher in pond water than in inlet water, especially ammonium-N concentrations. pH was significantly higher in pond water than in inlet water. However, salinity had no significant difference between pond water and water inlet.
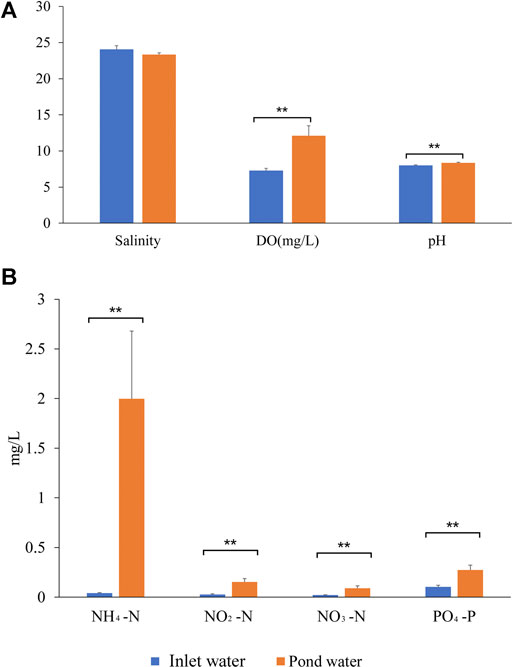
FIGURE 1. Physico-chemical parameters of pond water and inlet water. (A) comparison of salinity DO, and pH between inlet water and pond water; (B) comparison of nitrite-N, nitrate-N, ammonium-N and phosphate-P concentrations between inlet water and pond water; Asterisks represent significance analysis (p < 0.01).
High-Throughput Sequencing Data and OTU Analysis
Based on Illumina sequencing analysis, a total of 2,994 OTUs (n = 32) were observed at a 97% similarity level. t-test analysis revealed that there were statistically significant differences (p < 0.05) in OTU types and abundance between inlet water and pond water (Figure 2A). The relative abundance of 169 OTUs increased, while that of 211 OTUs decreased in pond water when compared to inlet water. The NMDS results revealed similarity in bacterial communities between inlet water and pond water (Figure 2B). Inlet water and pond water samples clustered separately with large distances between each cluster. However, the distances within pond water samples had higher variability compared to inlet water. The Shannon’s diversity and Chao1 index value of bacterial communities in pond water were lower than in inlet water (Figures 2C,D). A Venn diagram revealed that the number of bacterial-specific OTUs was higher in inlet water than in pond water. The total number of common OTUs in inlet water and pond water was 771 (Figure 2E).
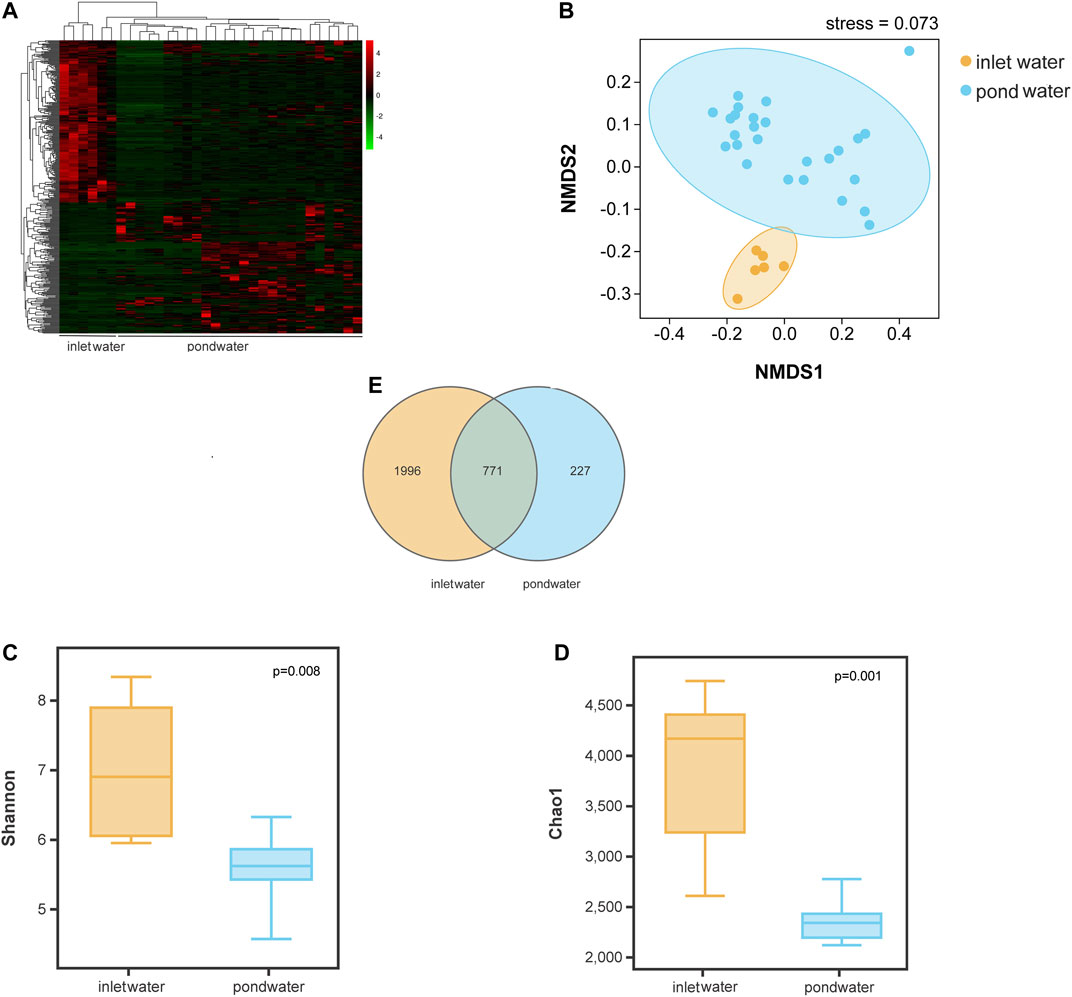
FIGURE 2. Analysis of significant differences in OTUs between inlet water and pond water (A); NMDS analysis (B) of bacterial communities between inlet water and pond water samples; Welch’s t-test analysis of Shannon’s diversity index (C) and Chao1 index (D) of bacterial communities; Venn diagram analysis of OTU numbers in inlet water and pond water (E).
Bacterial Communities in the Aquaculture Environment
The abundance of bacterial taxa corresponding to different samples was compared by annotating sequences information. The dominant taxa in each group at the class and genus levels are presented in Figure 3, Supplementary Table S2 and Supplementary Table S3. The dominant bacterial classes in inlet water and pond water (Figure 3A) were Alphaproteobacteria (20.63 ± 1.74% and 24.00 ± 2.26%), Actinobacteria (3.65 ± 0.58% and 17.57 ± 2.30%), Gammaproteobacteria (20.98 ± 4.18% and 13.05 ± 1.05%), Acidimicrobia (16.63 ± 2.89% and 12.52 ± 2.18%) and Flavobacteriia (4.87 ± 0.62% and 8.77 ± 0.79%). The results of the Welch’s t-tests (p < 0.01, Figure 3B) revealed that the abundances of Actinobacteria and Flavobacteriia were significantly higher in pond water than in inlet water. In contrast, the abundance of Gammaproteobacteria, Betaproteobacteria, and Deltaproteobacteria was significantly lower in pond water than in inlet water.
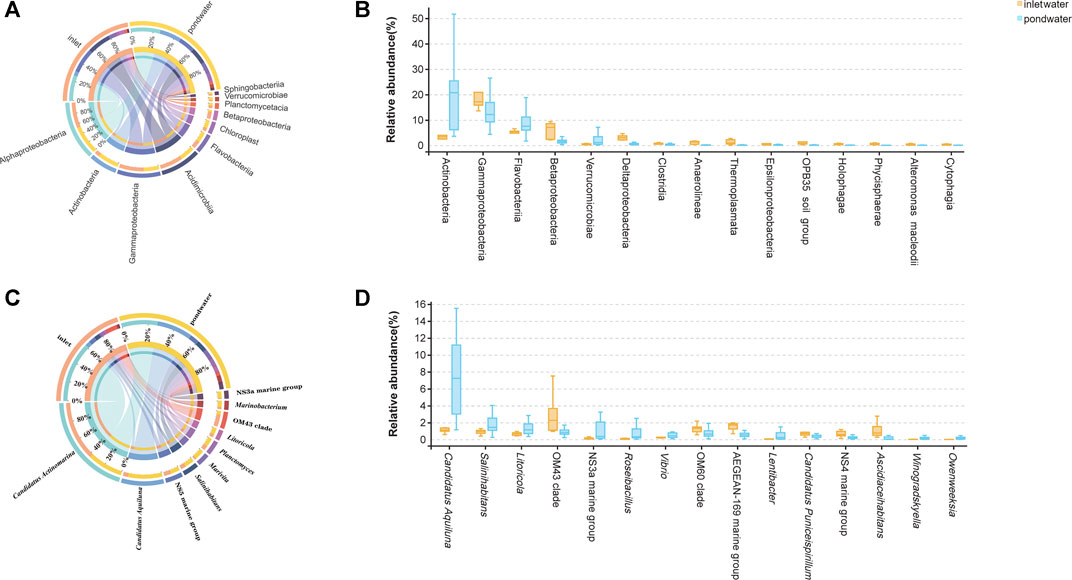
FIGURE 3. Analysis of bacterial communities in inlet water and pond water; dominant bacterial taxa in the samples at the class (A) and genus (C) levels; statistical analysis of significant differences in bacterial class (B) and genera (D) (Wilcoxon rank sum test, p < 0.05).
A significant difference (p < 0.01) was also observed in bacterial genera among the samples, with specific genera observed in inlet water, and pond water (Figures 3C,D). The dominant genera (average abundance >1%) in inlet water were Candidatus Actinomarina (12.41 ± 2.88%), NS5 marine group (1.32 ± 0.20%), Candidatus Aquiluna (1.09 ± 0.12%), and Salinihabitans (0.91 ± 0.13%). The dominant genera (relative abundance >1%) in pond water were Candidatus Actinomarina (8.29 ± 1.80%), Candidatus Aquiluna (7.99 ± 1.19%), Marivita (2.25 ± 0.65%), Salinihabitans (2.21 ± 0.41%), NS5 marine group (2.14 ± 0.41%), and Planctomyces (1.98 ± 0.85%). Furthermore, the results of the Welch’s t-tests revealed that the abundance of Candidatus Aquiluna, Salinihabitans, and Roseibacillus was enriched in pond water than inlet water (Figure 3D).
Potential Functions Prediction of Bacterial Communities in Aquaculture Area
PICRUSt2 analysis revealed variations of prediction functions among samples, which were primarily associated with carbon and nitrogen metabolism (Figure 4). Of all 34 functional families, the majority of them belonged to Amino acid metabolism (13.91 ± 0.06%), Carbohydrate metabolism (13.12 ± 0.07%), Metabolism of cofactors and vitamins (12.30 ± 0.09%), Metabolism of terpenoids and polyketides (9.04 ± 0.07%), Metabolism of other amino acids (8.37 ± 0.04%), Xenobiotics biodegradation and metabolism (6.83 ± 0.11%), Lipid metabolism (6.78 ± 0.08%), and Energy metabolism (5.43 ± 0.07%). Welch’s t-test showed that almost all of these functions of bacterial communities were significantly higher (p < 0.05) in pond water than in inlet water.
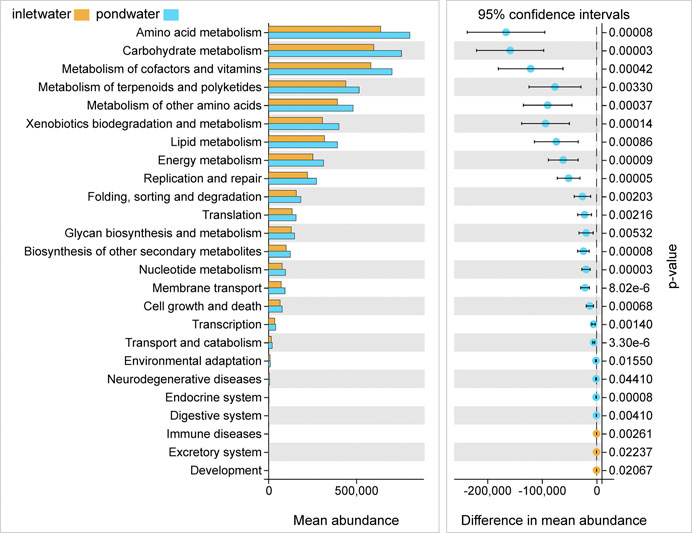
FIGURE 4. Predicted potential functions of bacterial communities and significant difference (p < 0.05) analysis of functions in inlet water and pond water.
Canonical Correlation Analysis Between Physicochemical Factors and Bacterial Communities
Canonical Correlation Analysis (CCA) was conducted to investigate the relationships between physicochemical factors (salinity, pH, DO, nitrate-N, ammonium-N, nitrite-N, and phosphate-P) and bacterial communities in inlet water and pond water (Figure 5A). The results revealed that nitrate-N, nitrite-N, phosphate-P, and ammonium-N were the key factors influencing bacterial communities. The bacterial communities in pond water was largely influenced by nitrate-N, nitrite-N, phosphate-P, and ammonium-N. Analysis of physicochemical factors contribution on bacterial communities exhibited that Nitrite-N (15.60%), nitrate-N (12.34%), phosphate-P (12.20%), and ammonium-N (11.03%) influenced bacterial communities the most in pond water (Figure 5B).
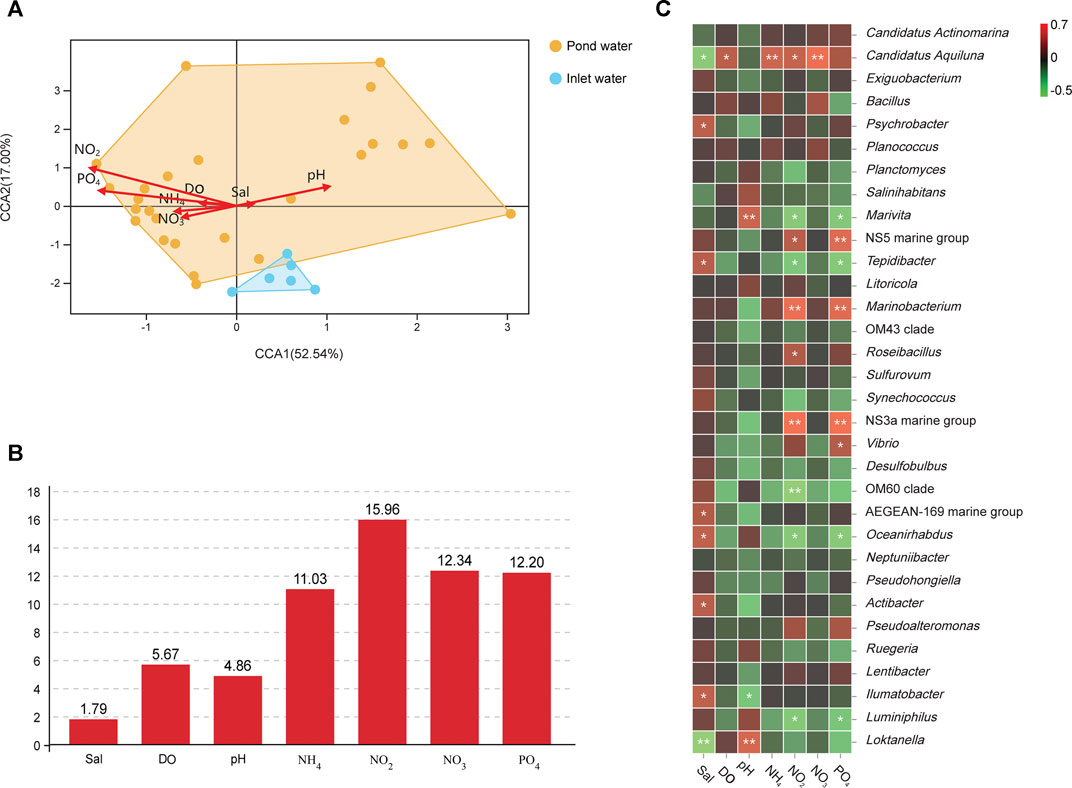
FIGURE 5. CCA analysis of physicochemical factors and bacterial communities (A); environmental contribution analysis (B); correlation analysis between dominant bacterial taxa and physicochemical factors (C).
Figure 5C illustrated the correlation between dominant bacteria genera and physicochemical factors. A significant correlation was observed between inorganic N and P nutrients, and dominant bacterial genera within the aquacultural environment. Nitrate-N, nitrite-N, phosphate-P, and ammonium-N exhibited significant positive correlations with dominant genera, such as Candidatus Aquiluna, NS5 marine group, Marinobacterium, NS3a marine group, and Vibrio (p < 0.05 or p < 0.01); however, they were negatively correlated with Marivita, Tepidibacter, Oceanirhabdus, and Luminiphilus (p < 0.05 or p < 0.01). The types of bacterial genera that were significantly correlated with salinity were quite distinct from bacterial genera correlated with nutrients.
Discussion
The present study investigated variations in physicochemical factors and bacterial communities between inlet water and pond water, and analyzed the relationships between bacterial communities and physicochemical factors. There were distinct bacterial communities and functions between inlet water and pond water, as well as in the physicochemical factors driving the difference in the aquacultural environments.
Aquaculture Decreases the Diversity and Richness of Bacterial Communities in Pond Water
Bacterial diversity and richness significantly decreased in pond water than in inlet water. Previous studies have revealed that bacterial communities in different environmental media (aquaculture water, sediment, and animal intestines) comprise specific bacterial taxa, which is attributed to environmental factors (Sun et al., 2019; Sun et al., 2020b), and suggests that the bacterial communities in such ecosystems is influenced by the environment. Eutrophication in aquaculture ponds water is attributed to animal feeding activities (Thakur and Lin, 2003; Sahu et al., 2013), and environmental factors such as nutrients and organic matter induce significant changes in bacterial communities, with more notable impacts in aquaculture pond environments than in water inlet environments. The relatively rich nutrients in pond water induce rapid propagation of microbe that are susceptible to such environmental changes, which, in turn, decreases diversity and richness of microbial community, as observed in a previous study (Wang et al., 2014). Bacterial diversity increases under relatively low nutrient conditions, while high nutrient levels promote eutrophication, which inhibits the growth of environmentally sensitive bacteria (Chrzanowski et al., 1995), and decreases the diversity of bacterial communities in water. Environmental variation influences the changes of bacterial communities in aquaculture systems, which suggests that microorganisms exhibit sensitive responses to shifts in external environments.
Aquacultural Environment Significantly Alter the Bacterial Communities
Mariculture has a great impact on nutrient concentrations due to the addition of feed, thus shaping the bacterial communities of the pond. A previous study reported that only 36% of N and 33% of P were assimilated and absorbed by cultured animals on average, and the remaining nutrient proportions were lost in various forms in the culture environment (Bouwman et al., 2013). The residual nutrients could induce changes in the aquatic environment, for example, in the form of shifts in pH, dissolved oxygen (DO), high N and Ps concentrations and organic matter. Bacterial community diversity in the eutrophic environment changed significantly compared with those before nitrogen and phosphorus nutrient addition (Haukka et al., 2006). In the present study, the regulation of inorganic nutrients by bacteria in aquaculture ponds was significantly enhanced. Inorganic N and P (ammonium-N, nitrate-N, nitrite-N, and phosphate-P) substantially promoted changes in bacterial communities.
Previous studies have revealed that bacterial communities are influenced by temperature, salinity, chlorophyll a, total N, inorganic N and P, C/N ratio, total P, and feed sources (Xiong et al., 2014; Zhang et al., 2014; Lin et al., 2017; Sun et al., 2019; Sun et al., 2020c). Among the parameters evaluated in the present study, inorganic nutrients, such as N and P, were the primary factors influencing bacterial communities in aquaculture environments, which is consistent with the findings of previous studies (Sun et al., 2019; Sun et al., 2020c). The physicochemical factors could directly alter bacterial communities by impairing microbial physiological activities, or indirectly, by establishing conditions that affect microorganisms adversely.
In addition, there were great variability in physicochemical parameters and bacterial community structure among pond water samples. This is due to differences in farming processes between ponds, such as feed application and feeding amount, which lead to different pond water parameters and nutrient structure. Differences in these physicochemical factors lead to changes in bacterial community structure and diversity. These results further demonstrated the driving effect of environmental parameters on bacterial community in aquaculture.
Correlation analysis of physicochemical factors and bacterial communities suggested that inorganic nitrogen and phosphate exhibited significant positive correlations with Candidatus Aquiluna, NS5 marine group, Marinobacterium, NS3a marine group, and Vibrio. These microorganisms adapt to the eutrophication of aquaculture ponds and their abundance is increased. In the present study, the dominance of heterotrophic bacteria could result in high uptake of inorganic nutrients such as nitrate-N, ammonium-N, and nitrite-N. Candidatus Aquiluna has been previously reported to be the predominant bacterial species in the study area (Lin et al., 2017; Sun F. et al., 2019), and it has the capacity to fix carbon and conduct rhodopsin-based phototrophy (Kang et al., 2012). Marivita abundance is often highest near phytoplankton blooms or in numerous marine and saline environments associated with organic particles (Slightom and Buchan, 2009), and could be a key factor influencing the transformation and removal of N in aquaculture water (Lin et al., 2017). Furthermore, the abundance of Vibrio significantly increased in culture environments in the present study. Vibrio is common genera of marine bacteria, and play important roles in utilizing many carbon, nitrogen, and phosphorus substrates (Roux et al., 2009). Marinobacter contains nitrous oxide reductase (nosZ) genes and has the capacity to reduce nitrite-N and nitrate-N to produce N2 via aerobic denitrification (Liu et al., 2016). These bacteria have the ability to transform inorganic nitrogen and phosphorus, and have the potential to enhance environmental quality, which is crucial in aquaculture.
Aquaculture Increase the Metabolic Capabilities of the Bacterial Communities in Pond Water
Metabolic capabilities of the bacterial communities in pond water increased significantly than in inlet water based on prediction functions analysis. Functional prediction demonstrated that carbon and nitrogen metabolism were the dominant processes in the aquaculture area. Overall, aquacultural environments increase C and N metabolic capacities of bacteria, particularly Amino acid metabolism and Carbohydrate metabolism. Enrichment function involved in carbohydrate and amino acid metabolism indicated that microbes prefer the pond environments with rich in carbohydrates and proteins. Previous studies have revealed that Proteobacteria, Bacteroidetes, and Actinobacteria are the dominant functional phyla associated with N and P removal, and COD reduction in water (Sun et al., 2019; Sun et al., 2020a). Aquacultural environments could increase phytoplankton diversity and abundance and generate substantial amounts of carbohydrates, amino acids, and other substances. Such substances would promote the growth of bacterial groups such as Rhodobacterales and Flavobacteriales (Osterholz et al., 2016), which increases bacterial metabolic capacity. In addition to nutrient metabolism, bacteria in the ponds also increased their capacity to degrade some harmful substances in the water, such as xenobiotics, terpenoids and polyketides. Actinobacteria could secrete a variety of enzymes and obtain nutrients from a wide variety of nutrient sources, including various complex polysaccharides and refractory biomaterials (Barka et al., 2016).
In conclusion, the present study has demonstrated that aquaculture considerably altered physicochemical factors and induced changes in bacterial community composition and function. Furthermore, this study identified dominant bacterial taxa and potential factors influencing bacterial communities in pond water and established that bacterial communities characteristics were correlated with environmental status. This study was helpful to the water quality management of aquaculture area.
Data Availability Statement
The original contributions presented in the study are publicly available. This data can be found here: NCBI Sequence Read Archive Database with project number PRJNA758780.
Author Contributions
Conceptualization, FS, CW and HY; investigation, CW and FS; methodology, FS; formal analysis, FS; writing original draft, FS; writing-review and editing, FS; data curation, CW and HY; funding acquisition, FS and HY.
Funding
The present study was supported financially by the Strategic Priority Research Program of the Chinese Academy of Sciences (XDA13010103), the Key Research and Development Program of Hainan Province (ZDYF2021XDNY131), the Key Special Project for Introduced Talents Team of Southern Marine Science and Engineering Guangdong Laboratory (GML2019ZD0305 and GML2019ZD0206), the National Natural Sciences Foundation of China (41506061 and 41406130), the Project of Fujian Science and Technology Department (2021N3001), and the Project of Guangdong Science and Technology Department (2017A020216008).
Conflict of Interest
The authors declare that the research was conducted in the absence of any commercial or financial relationships that could be construed as a potential conflict of interest.
Publisher’s Note
All claims expressed in this article are solely those of the authors and do not necessarily represent those of their affiliated organizations, or those of the publisher, the editors and the reviewers. Any product that may be evaluated in this article, or claim that may be made by its manufacturer, is not guaranteed or endorsed by the publisher.
Supplementary Material
The Supplementary Material for this article can be found online at: https://www.frontiersin.org/articles/10.3389/fenvs.2021.709541/full#supplementary-material
References
Alfiansah, Y. R., Hassenrück, C., Kunzmann, A., Taslihan, A., Harder, J., and Gärdes, A. (2018). Bacterial Abundance and Community Composition in Pond Water from Shrimp Aquaculture Systems with Different Stocking Densities. Front. Microbiol. 9, 15. doi:10.3389/fmicb.2018.02457
Austin, B. (2006). The Bacterial Microflora of Fish, Revised.Scientific World J. 6, 931–945. doi:10.1100/tsw.2006.181
Barka, E. A., Vatsa, P., Sanchez, L., Gaveau-Vaillant, N., Jacquard, C., Klenk, H.-P., et al. (2016). Taxonomy, Physiology, and Natural Products of Actinobacteria. Microbiol. Mol. Biol. Rev. 80 (1), 1–43. doi:10.1128/mmbr.00019-15
Bouwman, L., Goldewijk, K. K., Van Der Hoek, K. W., Beusen, A. H. W., Van Vuuren, D. P., Willems, J., et al. (2013). Exploring Global Changes in Nitrogen and Phosphorus Cycles in Agriculture Induced by Livestock Production over the 1900-2050 Period. Proc. Natl. Acad. Sci. 110 (52), 20882–20887. doi:10.1073/pnas.1012878108
Caporaso, J. G., Kuczynski, J., Stombaugh, J., Bittinger, K., Bushman, F. D., Costello, E. K., et al. (2010). QIIME Allows Analysis of High-Throughput Community Sequencing Data. Nat. Methods 7 (5), 335–336. doi:10.1038/nmeth.f.303
Chrzanowski, T. H., Sterner, R. W., and Elser, J. J. (1995). Nutrient Enrichment and Nutrient Regeneration Stimulate Bacterioplankton Growth. Microb. Ecol. 29 (3), 221–230. doi:10.1007/bf00164886
Douglas, G. M., Maffei, V. J., Zaneveld, J., Yurgel, S. N., Brown, J. R., Taylor, C. M., et al. (2020). PICRUSt2: An Improved and Customizable Approach for Metagenome Inference. bioRxiv, 672295. doi:10.1101/672295
Edgar, R. C., Haas, B. J., Clemente, J. C., Quince, C., and Knight, R. (2011). UCHIME Improves Sensitivity and Speed of Chimera Detection. Bioinformatics 27 (16), 2194–2200. doi:10.1093/bioinformatics/btr381
Haukka, K., Kolmonen, E., Hyder, R., Vakkilainen, K., and Kairesalo, T., (2006). Effect of Nutrient Loading on Bacterioplankton Community Composition in Lake Mesocosms. Microb. Ecol. 51 (2), 137–146. doi:10.1007/s00248-005-0049-7
Huang, Y., Niu, B., Gao, Y., Fu, L., and Li, W. (2010). CD-HIT Suite: a Web Server for Clustering and Comparing Biological Sequences. Bioinformatics 26 (5), 680–682. doi:10.1093/bioinformatics/btq003
Kahle, D., and Wickham, H. (2013). Ggmap: Spatial Visualization with Ggplot2. R. J. 5 (1), 144–161. doi:10.32614/rj-2013-014
Kang, I., Lee, K., Yang, S.-J., Choi, A., Kang, D., Lee, Y. K., et al. (2012). Genome Sequence of “Candidatus Aquiluna” Sp. Strain IMCC13023, a Marine Member of the Actinobacteria Isolated from an Arctic Fjord. J. Bacteriol. 194 (13), 3550–3551. doi:10.1128/jb.00586-12
Le Roux, F., Zouine, M., Chakroun, N., Binesse, J., Saulnier, D., Bouchier, C., et al. (2009). Genome Sequence ofVibrio Splendidus: an Abundant Planctonic marine Species with a Large Genotypic Diversity. Environ. Microbiol. 11 (8), 1959–1970. doi:10.1111/j.1462-2920.2009.01918.x
Li, T., Li, H., Gatesoupe, F.-J., She, R., Lin, Q., Yan, X., et al. (2017). Bacterial Signatures of "Red-Operculum" Disease in the Gut of Crucian Carp (Carassius auratus). Microb. Ecol. 74 (3), 510–521. doi:10.1007/s00248-017-0967-1
Lin, G., Sun, F., Wang, C., Zhang, L., and Zhang, X. (2017). Assessment of the Effect of Enteromorpha Prolifera on Bacterial Community Structures in Aquaculture Environment. PLoS One 12 (7), e0179792. doi:10.1371/journal.pone.0179792
Liu, Y., Ai, G.-M., Miao, L.-L., and Liu, Z.-P. (2016). Marinobacter Strain NNA5, a Newly Isolated and Highly Efficient Aerobic Denitrifier with Zero N2O Emission. Bioresour. Techn. 206, 9–15. doi:10.1016/j.biortech.2016.01.066
Magoc, T., and Salzberg, S. L. (2011). FLASH: Fast Length Adjustment of Short Reads to Improve Genome Assemblies. Bioinformatics 27 (21), 2957–2963. doi:10.1093/bioinformatics/btr507
Martin, J., Veran, Y., Guelorget, O., and Pham, D. (1998). Shrimp Rearing: Stocking Density, Growth, Impact on Sediment, Waste Output and Their Relationships Studied through the Nitrogen Budget in Rearing Ponds. Aquaculture 164 (1), 135–149. doi:10.1016/s0044-8486(98)00182-3
Mateos-Rivera, A., Yde, J. C., Wilson, B., Finster, K. W., Reigstad, L. J., and Øvreås, L. (2016). The Effect of Temperature Change on the Microbial Diversity and Community Structure along the Chronosequence of the Sub-arctic Glacier Forefield of Styggedalsbreen (Norway). FEMS Microbiol. Ecol. 92 (4), fnw038. doi:10.1093/femsec/fiw038
Moriarty, D. J. (1997). The Role of Microorganisms in Aquaculture Ponds. Aquaculture 151 (1), 333–349. doi:10.1016/s0044-8486(96)01487-1
Oksanen, J., Blanchet, F. G., Kindt, R., Legendre, P., Minchin, P., O’Hara, R. B., et al. (2015). Vegan: Community Ecology Package, 2. R package vegan, vers., 2–1. Available at: http://CRAN.R-project.org/package=vegan
Osterholz, H., Singer, G., Wemheuer, B., Daniel, R., Simon, M., Niggemann, J., et al. (2016). Deciphering Associations between Dissolved Organic Molecules and Bacterial Communities in a Pelagic marine System. ISME J. 10 (7), 1717–1730. doi:10.1038/ismej.2015.231
Sahu, B. C., Adhikari, S., and Dey, L. (2013). Carbon, Nitrogen and Phosphorus Budget in Shrimp (Penaeus monodon) Culture Ponds in Eastern India. Aquacult Int. 21 (2), 453–466. doi:10.1007/s10499-012-9573-x
Slightom, R. N., and Buchan, A. (2009). Surface Colonization by Marine Roseobacters: Integrating Genotype and Phenotype. Appl. Environ. Microbiol. 75 (19), 6027–6037. doi:10.1128/aem.01508-09
Sun, F.-L., Fan, L.-L., Wang, Y.-S., and Huang, L.-Y. (2019b). Metagenomic Analysis of the Inhibitory Effect of Chromium on Microbial Communities and Removal Efficiency in A2O Sludge. J. Hazard. Mater. 368, 523–529. doi:10.1016/j.jhazmat.2019.01.076
Sun, F., Wang, C., Chen, H., and Zheng, Z. (2020a). Metagenomic Analysis of the Effect of Enteromorpha Prolifera Bloom on Microbial Community and Function in Aquaculture Environment. Curr. Microbiol. 77, 816–825. doi:10.1007/s00284-019-01862-x10.1007/s00284-019-01862-x
Sun, F., Wang, C., Chen, L., Weng, G., and Zheng, Z. (2020b). The Intestinal Bacterial Community of Healthy and Diseased Animals and its Association with the Aquaculture Environment. Appl. Microbiol. Biotechnol. 104 (2), 775–783. doi:10.1007/s00253-019-10236-z
Sun, F., Wang, C., Wang, Y., Tu, K., Zheng, Z., and Lin, X. (2020c). Diatom Red Tide Significantly Drive the Changes of Microbiome in Mariculture Ecosystem. Aquaculture 520, 734742. doi:10.1016/j.aquaculture.2019.734742
Sun, F., Wang, Y., Wang, C., Zhang, L., Tu, K., and Zheng, Z. (2019a). Insights into the Intestinal Microbiota of Several Aquatic Organisms and Association with the Surrounding Environment. Aquaculture 507, 196–202. doi:10.1016/j.aquaculture.2019.04.026
Thakur, D. P., and Lin, C. K. (2003). Water Quality and Nutrient Budget in Closed Shrimp (Penaeus monodon) Culture Systems. Aquacultural Eng. 27 (3), 159–176. doi:10.1016/s0144-8609(02)00055-9
Wang, C.-z., Lin, G.-r., Yan, T., Zheng, Z.-p., Chen, B., and Sun, F.-l. (2014). The Cellular Community in the Intestine of the Shrimp Penaeus Penicillatus and its Culture Environments. Fish. Sci. 80, 1001–1007. doi:10.1007/s12562-014-0765-3
Xiong, J., Zhu, J., Wang, K., Wang, X., Ye, X., Liu, L., et al. (2014). The Temporal Scaling of Bacterioplankton Composition: High Turnover and Predictability during Shrimp Cultivation. Microb. Ecol. 67 (2), 256–264. doi:10.1007/s00248-013-0336-7
Yang, W., Zhu, J., Zheng, C., Qiu, H., Zheng, Z., and Lu, K. (2018). Succession of Bacterioplankton Community in Intensive Shrimp (Litopenaeus Vannamei) Aquaculture Systems. Aquaculture 497, 200–213. doi:10.1016/j.aquaculture.2018.07.053
Yukgehnaish, K., Kumar, P., Sivachandran, P., Marimuthu, K., Arshad, A., Paray, B. A., et al. (2020). Gut Microbiota Metagenomics in Aquaculture: Factors Influencing Gut Microbiome and its Physiological Role in Fish. Rev. Aquacult 12 (3), 1903–1927. doi:10.1111/raq.12416
Zhang, D., Wang, X., Xiong, J., Zhu, J., Wang, Y., Zhao, Q., et al. (2014). Bacterioplankton Assemblages as Biological Indicators of Shrimp Health Status. Ecol. Indicators 38, 218–224. doi:10.1016/j.ecolind.2013.11.002
Keywords: aquaculture, bacterial community, physicochemical factors, correlation, high-throughput sequencing
Citation: Sun F, Wang C and Yang H (2021) Physicochemical Factors Drive Bacterial Communities in an Aquaculture Environment. Front. Environ. Sci. 9:709541. doi: 10.3389/fenvs.2021.709541
Received: 14 May 2021; Accepted: 08 September 2021;
Published: 04 October 2021.
Edited by:
Changliang Ke, Chinese Academy of Fishery Sciences, ChinaReviewed by:
Jie Lu, Chinese Academy of Fishery Sciences, ChinaXiaoyong Zhang, South China Agricultural University, China
Zhao-Ming Gao, Institute of Deep-Sea Science and Engineering (CAS), China
Copyright © 2021 Sun, Wang and Yang. This is an open-access article distributed under the terms of the Creative Commons Attribution License (CC BY). The use, distribution or reproduction in other forums is permitted, provided the original author(s) and the copyright owner(s) are credited and that the original publication in this journal is cited, in accordance with accepted academic practice. No use, distribution or reproduction is permitted which does not comply with these terms.
*Correspondence: Hongqiang Yang, aHF5YW5nQHNjc2lvLmFjLmNu