Research on the Impact of Green Technology Innovation on Energy Total Factor Productivity, Based on Provincial Data of China
- 1Guangzhou Institute of International Finance, Guangzhou University, Guangzhou, China
- 2School of Economics and Statistics, Guangzhou University, Guangzhou, China
Against the background of carbon peaking and carbon neutralization, green technology innovation plays an important role in promoting the energy total factor productivity (TFP). This study verifies the impact of green technology innovation on energy TFP in a complete sample and the subsamples by region, by constructing a panel threshold model, and analyzes its influence mechanism on the basis of the mediating effect test based on annual provincial data of mainland China from 2005 to 2018. The empirical results reveal the following: first, with the level of economic development as the threshold variable, there is a threshold effect in the impact of green technology innovation on the energy TFP; second, green technology innovation has an impact on the energy TFP through industrial structure upgrading; that is, industrial structure has a mediating effect in the influence mechanism; and third, there is heterogeneity in the impact of green technology innovation on the energy TFP among different regions in China, and the threshold effect only exists in the western region, since the central and eastern regions have crossed a certain developmental stage.
Introduction
Energy TFP is usually defined by the ratio of energy input to output (Perez-Lombard et al., 2013). The promotion of energy TFP plays an important role in the sustainable development of the economy. The energy TFP includes the input of a series of means of production such as labor, energy, and capital, and is accompanied by the expected output GDP and the non-expected output carbon dioxide emissions. From the perspective of input, the promotion of energy efficiency avoids excessive energy consumption, and in terms of the output, it reduces the excessive damage to the environment. The energy TFP can reflect the efficiency of comprehensive development and utilization of energy to a large extent, and also helps to identify the state of economic growth, that is, whether economic growth depends on the consumption of energy scale or the improvement of energy use efficiency. A lot of literature works related to energy TFP have discussed ways to improve efficiency. Xie et al. (2014) measured energy TFP in the OECD and BRIC countries and found that the adjustment of the energy structure has a certain impact on energy TFP. Energy consumption can be reduced by restructuring industries so as to improve energy TFP (Xiong et al., 2019; Zhu et al., 2019; Yu, 2020). The efficiency of technological innovation is the main reason for energy TFP improvement in the industrial sector, and the effect of the technological progress on energy TFP is gradually increasing (Fisher-Vanden et al., 2006; Baccarelli et al., 2016; Naranjo et al., 2019). Miao et al. (2018) demonstrate the significant positive driving effect of technology innovation on energy TFP. Hellsmark et al. (2016) believed that industrial technology innovation can rapidly improve energy TFP so as to achieve rapid industrial growth and expansion. From the perspectives of changing energy structure, adjusting industrial structure, and technology innovation, the related literature fully demonstrates the effectiveness of technology innovation in improving energy TFP. However, the adjustment of energy structure and industrial structure is more dependent on the resource endowment of economic subjects, while technological innovation improves the energy utilization efficiency through improvement at the technological level, which has a more realistic value.
Energy TFP measurement needs to focus not only on the desired output but also on the undesired output. Based on the consideration of environmental factors, energy TFP measurement involves two aspects of the output: desired output and undesired output. The desired output refers to the output, such as GDP, which can increase human material products and services to a certain extent. The undesired output refers to additional products that have a negative effect on the human environment and health due to the consumption of energy and other elements in the production process, such as the greenhouse effect caused by carbon dioxide emissions in the production process. Too many studies have mainly focused on the contribution of energy to economic development, with less consideration on the impact of energy consumption on environmental quality, that is, ignoring the issue of the relationship between energy consumption and sustainable development. With the increasing prominence of environmental problems, more and more studies have included environmental factors into the consideration of energy TFP measurement to reduce the deviation in the process of energy TFP measurement (Zhang et al., 2011; He et al., 2013; Yang and Wang, 2013; Simsek, 2014; Vlontzos et al., 2014). Environmental regulation significantly promotes green technology innovation and reduces environmental pollution in the process of economic development (Du et al., 2021). Hu and Wang (2006) constructed a full-factor energy efficiency analysis framework to maximize the energy TFP output to improve energy TFP, which means that improving energy TFP should not only increase the desired output but also pay attention to the weakening of the undesired output. Environmental regulation can reduce the undesired output such as carbon dioxide, while improving energy TFP through technological innovation can play an essential role and have global strategic significance.
Technological innovation promotes the maturity of production technology and the development of new products, thus improving efficiency significantly. From the perspective of the innovation system, the improvement of energy TFP by technological innovation is to reduce the leakage in the process of energy use by means of process transformation, and then to improve the total factor productivity of energy. From the perspective of industrial ecological chain, technological innovation promotes the upgrading of the regional structure and the exchange and cooperation between the industrial structure, and innovation jointly promotes technological innovation and industrial structure upgrading (Greunz, 2004; Motohashi and Yun, 2007; Altenburg et al., 2008), and adjusting industrial structure can improve energy utilization efficiency as well (Zhao et al., 2010; Zhou et al., 2013; Yu et al., 2016; Wang et al., 2020). The technical level of energy TFP production depends on the technological innovation ability; empirical results show that the enhancement of technological innovation ability can effectively improve energy efficiency and reduce energy consumption intensity (Du and Yan, 2009; Zhong and Li, 2020). Wagner et al. (2014) found from innovative activities, with potential environmental impact that the potential of technological innovation is unlimited. Although technological innovation has a strong effect on efficiency, pure technological innovation does not take into account the external effects of the environment, so green technology innovation is more in line with the goal of sustainable development (Li and Liao, 2020). Considering that technological innovation promotes the upgrading of the industrial structure and thus has an impact on energy TFP, this study chooses the upgrading of the industrial structure as a mediating variable to study the indirect impact of green technological innovation on total factor productivity of energy.
Green technology innovation belongs to the scope of technological innovation, which is the general name of management and technology innovation aimed at protecting the environment. In the process of innovation practice, although some innovations can greatly improve productivity, they do not consider the external effects on the environment; for example, technological innovation is simply about increasing the output in energy-intensive industries. As a result, various industries abide by the green principle and pay more and more attention to economic development and environmental problems (Gorelick and Walmsley, 2020; Sukharev, 2020). Green technology follows the ecological principle and the law of ecological economy, considers the saving of resources and energy in the process of innovation, avoids, eliminates or reduces the pollution and damage to the ecological environment in the process of innovation, and maintains the minimum ecological negative effect in technological innovation. Green technology innovation aims to achieve long-term sustainable development; produce economic, environmental, and social benefits; save resources and energy; and eliminate or reduce environmental pollution and degradation (Zhou et al., 2014; Li et al., 2018). Because green technology innovation considers the external efficiency of the environment, there may be conflicts between self-interests and social benefits in the process of enterprises’ implementing green technology innovation, thus reducing the power of green technology innovation (Braun and Wield, 1994; Li et al., 2019a).
The significant effect of technological innovation on energy TFP is based on the consistency of interests which belong to stakeholders, but the impact of green technology innovation on the interests of stakeholders may be different. Green technology innovation may increase the production cost of enterprises, and the improvement of energy TFP requires more consideration of environmental externalities. Therefore, whether green technology innovation has an impact on energy TFP needs to be explored in both theory and practice. Based on this, this article studies the impact of green technology innovation on energy TFP.
This article focuses on the impact of green technology innovation on energy TFP. Its marginal contributions are as follows: first, green technology innovation that will involve subject behavior and external environmental effects related to the subject’s behavior is considered in a framework. Most of the existing literature works only consider green technology innovation or the total factor productivity of energy, and less considers external effects such as environment. In this article, green technology innovation is separated from the innovation system, the undesired output is included in TFP measurement, green technology innovation is included in the behavior of the innovation subject, and external effect of environment is considered in energy TFP measurement. Second, the threshold effect of green technology innovation on energy TFP is studied. In the process of empirical analysis, it is found that green technology innovation does not necessarily have a significant impact on energy TFP, but through the threshold effect model, it is found that when the level of economic development is the threshold variable, there is a threshold effect in the impact of green technology innovation on energy TFP. Third, the mediating effect mechanism of green technology innovation on energy TFP was studied. Through the selection and experiment of different mediating variables, this article empirically tests the mediating effect of the industrial structure in the impact of green technology innovation on energy TFP. Fourth, there is spatial heterogeneity in the impact of green technological innovation on energy TFP. Since China’s economy has very strong regional heterogeneity, according to the basic situation of economic development in the area of space, this article divides the full sample into three subsamples: the eastern, the central, and the western regions, to study the heterogeneity.
The structure of the rest of this article is as follows: the second section is about the measurement of the impact of green technology innovation on energy TFP, including model setting, variables, data sources, and test results. The third section focuses on the influence mechanism analysis of green technology innovation on energy TFP. In terms of the technology of testing the mediating effect, this part estimates the parameters and analyzes the mediating effect by setting the mediating effect model. The fourth section is about the heterogeneity analysis of the impact of green technology innovation on energy TFP. According to the basic situation of economic development, the full sample is divided into three subsamples, and the heterogeneity is analyzed. The fifth section draws the basic conclusion.
Econometric Test of the Impact of Green Technology Innovation on Energy Total Factor Productivity
Panel Threshold Model Setting
The improvement of energy TFP by technological innovation has been proven in a lot of literature works, but whether green technology innovation affects energy TFP needs to be tested with more empirical evidence. From the perspective of the relationship between technological innovation and energy TFP, technological innovation requires costs; the greater uncertainty of green technological innovation means that enterprises are facing greater uncertainty in technological innovation; this uncertainty makes enterprises, as the main body of technological innovation, more inclined to realize their self-interests when making decisions, and then tend to ignore the strategic interests. Accordingly, in the face of various external constraints, enterprises have very great differences in their green technology innovation motivation, so green technology innovation has an impact on total factor productivity, but this impact needs to be verified through econometric tests.
In different stages of economic development, the strength and consciousness of enterprises to support green technology innovation are different. For example, in regions with a high degree of economic development, people have higher demand for products and environmental quality, and the corresponding innovation subjects can bear greater risks of technological innovation and research. Therefore, there is a certain threshold for the impact of green technology innovation on energy TFP in theory.
On that basis, this study assumes that green technology innovation has a significant effect on energy TFP, and this effect is nonlinear and has a threshold effect, and the variable of the core threshold effect is the level of economic development. The threshold effect model can examine the function between the two and the threshold effect (Liu et al., 2020). In this study, the level of economic development is taken as the threshold variable, and the panel threshold model proposed by Hansen is adopted (Hansen, 1999). The basic form of the model is as follows:
where EEit represents the energy TFP, which is used to measure energy efficiency; GTIit represents the green technology innovation; threshold variable EDIit is the economic development level;
After the threshold and slope values are estimated, the significance of the threshold effect should be tested. The basic principle of testing the threshold effect is as follows. Taking single threshold as an example, the null hypothesis and test statistics of the model are obtained as follows:
If the null hypothesis is rejected, there is threshold effect in the impact of green technological innovation on energy TFP. S0 is the sum of squares of residuals obtained under the null hypothesis H0, and
After determining the threshold effect of the economic development level, it is necessary to test whether the threshold estimated value
where the LR distribution is also nonstandard. This study adopts a formula proposed by Hansen (1999); that is, when
Variable and Data Description
Variable Description
Energy TFP is the explained variable, which is measured by the ratio of energy consumption to GDP in many studies. This method of measuring energy efficiency is not responsive to the dynamic change of efficiency (Hang and Tu, 2007; Adom and Kwakwa, 2014). In the continuous research of energy TFP, some methods such as index decomposition analysis (IDA), parametric stochastic Frontier analysis (SFA), and nonparametric data envelopment analysis (DEA) have been proposed to measure energy efficiency or total factor productivity (Zhou et al., 2012; Filippini and Hunt, 2015; Li et al., 2019b; Liao and Drakeford, 2019; Zheng et al., 2020). These methods can dynamically investigate the dynamic changes of energy efficiency or total factor productivity, and then study the effects of other factors on energy TFP, but these methods do not consider the interest correlation between the evaluation subjects. The cross-efficiency evaluation can dynamically investigate the dynamic changes of energy TFP on the basis of self-evaluation and other evaluation so that the evaluation results are comparable, and a complete ranking result can be obtained. Therefore, this study selects the DEA cross-efficiency model to measure energy TFP. The basic form is as follows:
Let S be the number of provinces selected in this study; then the vectors of m energy input indexes and n energy output of the decision-making unit DMUi are expressed as
• The constraints are
where
In the energy TFP calculation, it usually takes into account the input of the means of production, while this study also focuses on the expected and unexpected outputs. Labor, capital stock, and energy consumption are used as input indicators. The number of urban employment is used to measure the labor force, the perpetual inventory is used to estimate capital stock, and the basic equation is
The desired output of energy TFP is measured by GDP, and the undesired output is measured by carbon dioxide emission. The details are shown in Table 1. Data of GDP are converted to real regional GDP with the year 2000 as the base year. Carbon emissions are estimated by the direct method:
The explanatory variable is green technology innovation, expressed by the number of green patent application, including green invention patents and green utility model patents. The control variables of the model include foreign direct investment (FDI), industrial structure (IS), and energy price. FDI which is closely related to economic development is measured by the proportion of foreign direct investment in regional GDP (Li et al., 2019c), IS was measured by the proportion of the output value of secondary industry in GDP, and the energy price is calculated by this formula:
Data Sources
The sample data are from 30 provinces of mainland China (the sample does not include Hong Kong, Macao, Taiwan, and Tibet due to data problems) in 2005–2018. The time frequency of the data is set to year. The green patent data come from CNRDS green patent-GPRD database. The data of urban employment, total energy consumption, and the added value of the primary, secondary, and tertiary industries are derived from the China Energy Database. The output value of the secondary industry and the regional GDP are derived from the annual statistical yearbooks published by the National Bureau of Statistics. Gross fixed capital formation of the whole society comes from the Wind Database. Foreign direct investment data are from the provincial statistical yearbooks. The purchase price indexes of fuel and power come from the China Price Yearbook. The “China Price Yearbook (2004)” has a relatively comprehensive record of various energy prices, so they can be used to calculate the average price of the three energy sources in 2003 and convert into the form of yuan/ton standard coal to get the energy price in 2003. Other variables are shown in Table 2.
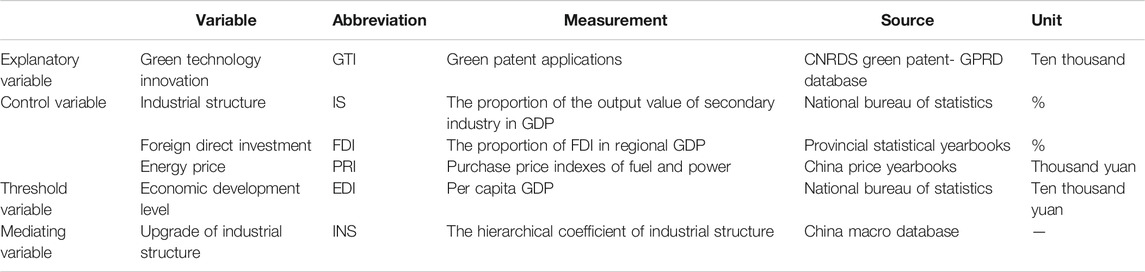
TABLE 2. Explanatory variables, control variables, threshold variables, and mediating variables used in empirical studies.
According to the data source, relevant data are collected and relevant variables are measured. The descriptive statistics of each variable are shown in Table 3.
It can be seen from Table 3 that the variation degree of each variable is quite great, especially the variable of the level of economic development. Table 3 describes the basic characteristics of the data for 30 provinces in China from 2005 to 2018, including the average values, standard deviation, minimum, and maximum values. For the green technology innovation data, there is a large gap between the maximum value 5.603 and the minimum value 0.001, which indicates that there are significant gaps in the level of green technology innovation among different regions. In terms of standard deviation, the standard deviation of energy price is the largest, followed by that of the level of economic development. From the discrete degree of these indicators, it can be seen that there is a large standard deviation in the three variables of the economic development level, energy price, and green technology innovation level, which indicates that heterogeneity exists in the field of green technology innovation and energy technology. Heterogeneity research can be analyzed from different perspectives and methods to explore the development law of things and the internal relations of some influencing factors in a more comprehensive way (Li et al., 2020a; Li et al., 2020b; Li and Zhong, 2020; Li et al., 2021a; Li et al., 2021b).
Empirical Results
On the basis of the constructed threshold model, it is necessary to determine the existence of threshold effect and the number and size of the threshold value. Using sample data, the existence of threshold effect is tested, and the test results are shown in Table 4.
Table 4 shows that with the level of economic development as the threshold variable, green technology innovation has a single threshold effect on energy TFP. The F statistics value of the single threshold effect test is 68.59, passing the significance test at 95% confidence level, while the F statistics value of the double threshold effect is 9.98, failing the significance test. Judging from the F statistics in Table 4, the impact of green technology innovation on energy TFP does cause a single threshold effect based on the level of economic development. The threshold estimate of the variable of the economic development level is 7.248. The single threshold effect model is used to estimate the parameters of the model, and the results are shown in Table 5.
Table 5 shows that with the economic development level as the threshold variable, green technology innovation has a threshold effect on energy TFP. From the regression results of the panel threshold effect, the promotion of green technology innovation to energy TFP is restricted by the threshold effect of the economic development level. When the level of economic development is below the threshold value of 7.248, the influence coefficient of green technology innovation on energy TFP is relatively low, which is 0.113. When the level of economic development crosses the threshold value of 7.248, the influence coefficient of green technology innovation on energy TFP is increased to 0.536. This indicates that the impact of green technology innovation on energy TFP is different at different levels of economic development. Under the low level of economic development, due to extensive economic management, it is difficult for the economic entities to achieve the balance between their own interests and the social benefits in production decision-making, and they pay more attention to their own short-term economic interests; correspondingly, the low level of economic development leads to low promotion of green technology innovation on energy TFP. With the improvement of the level of economic development, the decision-making behavior of economic entities is more focused on strategic development. Local governments gradually implement environmental regulations and other measures to promote the economic transformation of various regions. Therefore, the promotion of green technology innovation on energy TFP is significantly improved.
Mechanism Analysis of the Impact of Green Technology Innovation on Energy Total Factor Productivity
Mediating Effect Model Setting
Green technology innovation has positive promoting effect on economic growth, and this positive role has a threshold effect, so the impact of green technology innovation on energy TFP is not direct, but through other channels. Enterprises are the main body of technological innovation. When technology and capital are combined, they will have a very important impact on the industrial ecology. From the perspective of industrial evolution, technological innovation promotes the upgrading of the industrial structure. In terms of energy consumption, the period of industrialization has greatly increased energy consumption. With the evolution of industrial structure, the industry has gradually developed to the tertiary industry. On the one hand, through the improvement of industrial technology, the comprehensive utilization efficiency of energy has been greatly improved, and then promoting carbon emissions reach the peak faster, which has effectively improved the total factor productivity of energy. On the other hand, the industrial structure gradually shifts from a higher proportion of high-energy industries to a higher proportion of low-energy industries, thus reducing the amount of energy consumption. Green technology innovation needs strategic adjustment. For the micro entity, it is difficult to cover the short-term investment cost of green technology innovation before it reaches a certain level of economic development. Therefore, there is a threshold in the impact of green technology innovation on energy TFP. Based on the above analysis, the impact of green technology innovation on energy TFP is affected by the way of the industrial structure; that is, there is a mediating effect of the industrial structure, but there may be differences among different regions.
In order to study the influence mechanism of green technology innovation on energy TFP and explore the mediating effect, this part introduces industrial structure upgrading as the mediating variable on the basis of the theoretical analysis. The mediating effect models are as follows:
where
In the mediating effect analysis, Model (10) is first regressed to test whether the regression coefficients of energy TFP and green technological innovation are positive, and only when the coefficients are significantly positive the next test can be carried out; otherwise, the test is terminated. Second, Model (11) is regressed to test whether the regression coefficients of the mediating variable industrial structure upgrading and green technology innovation are significantly positive, and if they are significantly positive, green technology innovation supports the upgrading of the industrial structure. Then, Model (12) is regressed, and if the coefficients
Mediating Effect Test and Result Analysis
The parameters in Models (10)–(12) are estimated by using the same sample data, and the results are shown in Table 6.
Table 6 shows that green technology innovation influences energy TFP through the channel of industrial structure upgrading; that is, industrial structure upgrading has a mediating effect in the influence mechanism. The overall regression results show that the regression coefficients of both green technology innovation and industrial structure upgrading on energy TFP are significant, indicating that the total effect is significant. In Model (10), the impact of green technology innovation on energy TFP is verified. The coefficient of GTI is 0.192, which is significantly positive at the level of 1%, indicating that green technology innovation promotes energy TFP. In Model (11), there is a significant positive correlation between green technology innovation and industrial structure upgrading at the level of 1%, which indicates that green technology innovation accelerates industrial structure upgrading. In Model (12), after adding the industrial structure upgrading variable to Model (11), the coefficients of green technology innovation and industrial structure upgrading are significantly positive, and the coefficient of green technology innovation is reduced from 0.192, when there is no mediating variable, to 0.0758. It indicates that industrial structure upgrading plays a partial mediating effect in the impact of green technology innovation on energy TFP.
It can be concluded from Table 7 that the mediating effect of the industrial structure in the influence mechanism of green technology innovation on energy TFP is robust. Compared with the empirical results in Table 6, the significance and direction of parameter estimation in Table 7 have not changed, and the mediating effect has not changed significantly. Through the Bootstrap test, the direct effect value of green technology innovation on energy TFP is 0.12, while the indirect effect value of green technology innovation on energy TFP through industrial structure upgrading is 0.09, and the mediating effect accounts for 42.86% of the total effect, and the effect is significant. According to Table 7, the confidence intervals of direct and indirect effects are observed, excluding 0, indicating that the mediating effect of green technology innovation on energy TFP through industrial structure upgrading is tenable and robust.
Heterogeneity Analysis on the Impact of Green Technology Innovation on Energy Total Factor Productivity
Sample Partition Based on Regions
According to the above test results, green technology innovation has a threshold effect on energy TFP, so to a large extent, the impact of green technology innovation on energy TFP is heterogeneous. It can also be known from the aforementioned descriptive statistics that there are differences in the level of economic development, green technology innovation, and energy TFP in different regions. As a country with extremely uneven economic development, China has significant differences in the level of economic development among different provinces. Therefore, this part analyzes the heterogeneity of the impact of green technology innovation on the energy TFP based on regional differences.
Provincial administrative regions are the main basis of regional division in China. Most of the research literature works on Chinese regions are based on Chinese mainland provincial administrative regions. This study also divides regions into 30 provincial administrative regions (excluding Tibet). According to the descriptive statistics of variables in Table 3, combined with the level of economic development and the practice of most literature, 30 provinces are divided into three regions: the eastern, the central, and the western regions. There are 11 provinces and municipalities in the eastern region, including Beijing, Tianjin, Hebei, Liaoning, Shanghai, Jiangsu, Zhejiang, Fujian, Shandong, Guangdong, and Hainan. The central region includes eight provinces such as Shanxi, Jilin, Heilongjiang, Anhui, Jiangxi, Henan, Hubei, and Hunan. There are 11 provinces and municipalities in the western region, including Inner Mongolia, Guangxi, Chongqing, Sichuan, Guizhou, Yunnan, Shanxi, Gansu, Ningxia, Qinghai, and Xinjiang.
Empirical Result Analysis
According to the division of regions, three subsamples are obtained. The test of the threshold effect shows that there is heterogeneity in the threshold values of the three subsamples. Therefore, the threshold effect of the three subsamples should be tested separately. The specific test results are shown in Table 8.
From Table 8, it can be concluded that with the level of economic development as the threshold variable, there is heterogeneity in the threshold of three subsamples. With the level of economic development as the threshold variable, the green technology innovation in the western region has a single threshold effect on the energy TFP, but there is no threshold effect in the eastern and central regions. The F statistic value of the single threshold effect test is 67.63, which is significant at the level of 1%, and the F statistic value of the double threshold effect test is 12.99, which has not passed the significance test. The F statistic values of the threshold effect test in both the eastern and the central regions do not pass the significance test. Therefore, it can be concluded that the threshold effect of green technology innovation on energy TFP has spatial regional differences with the level of economic development as the threshold variable. At the same time, the results of the threshold effect test show that the central and eastern regions have crossed a certain stage of economic development.
Based on the above analysis, the parameters of the threshold effect in the western region are estimated, and the results are shown in Table 9.
Combining the results in Table 5 and Table 9, it can be seen that the threshold effect range of green technology innovation on the energy TFP in the western region is lower than that of the full sample. In Table 5, the threshold effect values of the full sample are 0.113 and 0.536, and in Table 9, the threshold effect values of the western region are 0.239 and 4.062. The range of the threshold effect value in the western region becomes smaller, while the threshold effect in the eastern and central regions no longer exists, indicating that when economic growth goes beyond a certain limit, the positive effect of green technology innovation on energy TFP will play a promoting role, which can be gradually independent on the level of economic development. According to the estimated results of the parameters in Table 9, when the level of economic development is below the threshold value of 4.062, the influence coefficient of green technology innovation on energy TFP is 0.239. When the level of economic development increases above the threshold value of 4.062, the influence coefficient of green technology innovation on energy TFP is 4.495. This indicates that with the development of the western region, the role of green technology innovation in promoting energy TFP is becoming more and more significant, so in the construction of the western economy, the investment of green technology innovation should be increased, and the improvement of energy TFP should be promoted, so as to achieve a win–win situation.
Conclusion
By constructing the panel threshold effect model and using Chinese mainland provincial data, this study examines the impact of green technology innovation on energy TFP and analyzes its mechanism and heterogeneity. The conclusions are as follows:
First, with the level of economic development as the threshold variable, green technology innovation has heterogeneous threshold effect on energy TFP. Based on the data of the full sample, it is estimated that the impact of green technology innovation on energy TFP is restricted by the level of economic development, and there is a single threshold effect. However, combining with the empirical analysis results, it can be found that this threshold effect does not exist in the central and eastern regions. Green technology innovation has positive effect on energy TFP, which indicates that green innovation must be promoted in order to achieve long-term sustainable development of economy. From the threshold effect, the promotion effect of green technology innovation on energy TFP increases with the improvement of the economic development level.
Second, green technology innovation has an impact on energy TFP through industrial structure upgrading; that is, industrial structure has mediating effect in the influence mechanism. Industrial structure upgrading realizes industrial structure optimization by increasing the proportion of the tertiary industry, improving energy utilization efficiency through technology innovation and product upgrading, and improving energy TFP.
Third, the impact of green technology innovation on energy TFP is heterogeneous in the western, central, and the eastern regions of China, and the threshold effect only exists in the western region, since the economic development of the central and eastern regions has crossed a certain stage. In the eastern and central regions, there is no threshold effect in the impact of green technology innovation on energy TFP, while in the western region, there is a single threshold effect, and the impact of green technology innovation on energy TFP increases significantly with the level of economic development. In the eastern and central regions of China, the effectiveness of green technology has exceeded a certain stage, and green technology innovation has gradually played a strategic role in promoting the total factor productivity of energy.
Data Availability Statement
The original contributions presented in the study are included in the article/Supplementary Material, and further inquiries can be directed to the corresponding author.
Author Contributions
MW: grasp the theme and research direction, YL: empirical research and data, GL: data.
Funding
This research was funded by the Chinese National Funding of Social Sciences, grant number 18ATJ002, and the 13th Five-year Plan of Guangzhou Social Science, grant number 2018GZYB129.
Conflict of Interest
The authors declare that the research was conducted in the absence of any commercial or financial relationships that could be construed as a potential conflict of interest.
Supplementary Material
The Supplementary Material for this article can be found online at: https://www.frontiersin.org/articles/10.3389/fenvs.2021.710931/full#supplementary-material
References
Adom, P. K., and Kwakwa, P. A. (2014). Effects of Changing Trade Structure and Technical Characteristics of the Manufacturing Sector on Energy Intensity in Ghana. Renew. Sustainable Energ. Rev. 35, 475–483. doi:10.1016/j.rser.2014.04.014
Altenburg, T., Schmitz, H., and Stamm, A. (2008). Breakthrough? China's and India's Transition from Production to Innovation. World Development 36, 325–344. doi:10.1016/j.worlddev.2007.06.011
Baccarelli, E., Cordeschi, N., Mei, A., Panella, M., Shojafar, M., and Stefa, J. (2016). Energy-Efficient Dynamic Traffic Offloading and Reconfiguration of Networked Data Centers for Big Data Stream Mobile Computing: Review, Challenges, and a Case Study. IEEE Netw. 30, 54–61. doi:10.1109/mnet.2016.7437025
Braun, E., and Wield, D. (1994). Regulation as a Means for the Social Control of Technology. Technology Anal. Strateg. Management 6, 259–272. doi:10.1080/09537329408524171
Du, K., Cheng, Y., and Yao, X. (2021). Environmental Regulation, green Technology Innovation, and Industrial Structure Upgrading: The Road to the green Transformation of Chinese Cities. Energ. Econ. 98, 105247. doi:10.1016/j.eneco.2021.105247
Du, X. F., and Yan, X. F. (2009). Empirical Study on the Relationship between Regional Technological Innovation Capacity and Regional Energy Consumption Intensity. Los Alamitos: Ieee Computer Soc. doi:10.1109/iciii.2009.168
Filippini, M., and Hunt, L. C. (2015). Measurement of Energy Efficiency Based on Economic Foundations. Energ. Econ. 52, S5–S16. doi:10.1016/j.eneco.2015.08.023
Fisher-Vanden, K., Jefferson, G. H., Jingkui, M., and Jianyi, X. (2006). Technology Development and Energy Productivity in China. Energ. Econ. 28, 690–705. doi:10.1016/j.eneco.2006.05.006
Gorelick, J., Walmsley, N., and Walmsley, N. (2020). The Greening of Municipal Infrastructure Investments: Technical Assistance, Instruments, and City Champions. Green. Financ. 2, 114–134. doi:10.3934/gf.2020007
Greunz, L. (2004). Industrial Structure and Innovation - Evidence from European Regions. J. Evol. Econ. 14, 563–592. doi:10.1007/s00191-004-0234-8
Hang, L., and Tu, M. (2007). The Impacts of Energy Prices on Energy Intensity: Evidence from China. Energy Policy 35, 2978–2988. doi:10.1016/j.enpol.2006.10.022
Hansen, B. E. (1999). Threshold Effects in Nondynamic Panels: Estimation, Testing, and Inference. J. Econom. 93, 345–368. doi:10.1016/s0304-4076(99)00025-1
He, F., Zhang, Q., Lei, J., Fu, W., and Xu, X. (2013). Energy Efficiency and Productivity Change of China's Iron and Steel Industry: Accounting for Undesirable Outputs. Energy Policy 54, 204–213. doi:10.1016/j.enpol.2012.11.020
Hellsmark, H., Mossberg, J., Söderholm, P., and Frishammar, J. (2016). Innovation System Strengths and Weaknesses in Progressing Sustainable Technology: the Case of Swedish Biorefinery Development. J. Clean. Prod. 131, 702–715. doi:10.1016/j.jclepro.2016.04.109
Hu, J.-L., and Wang, S.-C. (2006). Total-factor Energy Efficiency of Regions in China. Energy Policy 34, 3206–3217. doi:10.1016/j.enpol.2005.06.015
Li, T., Huang, Z., Huang, Z., and M Drakeford, B. (2019a). Statistical Measurement of Total Factor Productivity under Resource and Environmental Constraints. Natl. Account. Rev. 1, 16–27. doi:10.3934/nar.2019.1.16
Li, T., and Liao, G. (2020). The Heterogeneous Impact of Financial Development on Green Total Factor Productivity. Front. Energ. Res. 8, 9. doi:10.3389/fenrg.2020.00029
Li, T., Zhong, J., and Huang, Z. (2020a). Potential Dependence of Financial Cycles between Emerging and Developed Countries: Based on ARIMA-GARCH Copula Model. Emerging Markets Finance and Trade 56, 1237–1250. doi:10.1080/1540496x.2019.1611559
Li, Z., Chen, L., and Dong, H. (2021a). What Are Bitcoin Market Reactions to Its-Related Events? Int. Rev. Econ. Finance 73, 1–10. doi:10.1016/j.iref.2020.12.020
Li, Z., Dong, H., Floros, C., Charemis, A., and Failler, P. (2021b). Re-examining Bitcoin Volatility: A CAViaR-Based Approach. Emerging Markets Finance and Trade 19, 1–19. doi:10.1080/1540496x.2021.1873127
Li, Z., Huang, Z., and Dong, H. (2019c). The Influential Factors on Outward Foreign Direct Investment: Evidence from the “The Belt and Road”. Emerging Markets Finance and Trade 55, 3211–3226. doi:10.1080/1540496x.2019.1569512
Li, Z., Liao, G., and Albitar, K. (2019b). Does Corporate Environmental Responsibility Engagement Affect Firm Value? the Mediating Role of Corporate Innovation. Bus Strat Env 29, 1045–1055. doi:10.1002/bse.2416
Li, Z., Liao, G., Wang, Z., and Huang, Z. (2018). Green Loan and Subsidy for Promoting Clean Production Innovation. J. Clean. Prod. 187, 421–431. doi:10.1016/j.jclepro.2018.03.066
Li, Z., Wang, Y., and Huang, Z. (2020b). Risk Connectedness Heterogeneity in the Cryptocurrency Markets. Front. Phys. 8, 13. doi:10.3389/fphy.2020.00243
Li, Z., and Zhong, J. (2020). Impact of Economic Policy Uncertainty Shocks on China's Financial Conditions. Finance Res. Lett. 35, 101303. doi:10.1016/j.frl.2019.101303
Liao, G., Drakeford, B. M., and M. Drakeford, B. (2019). An Analysis of Financial Support, Technological Progress and Energy Efficiency: evidence from China. Green. Financ. 1, 174–187. doi:10.3934/gf.2019.2.174
Liu, Y., Li, Z., and Xu, M. (2020). The Influential Factors of Financial Cycle Spillover: Evidence from China. Emerging Markets Finance and Trade 56, 1336–1350. doi:10.1080/1540496x.2019.1658076
Matei, I. (2020). Is Financial Development Good for Economic Growth? Empirical Insights from Emerging European Countries. Quantitative Finance Econ. 4, 653–678. doi:10.3934/qfe.2020030
Miao, C., Fang, D., Sun, L., Luo, Q., and Yu, Q. (2018). Driving Effect of Technology Innovation on Energy Utilization Efficiency in Strategic Emerging Industries. J. Clean. Prod. 170, 1177–1184. doi:10.1016/j.jclepro.2017.09.225
Motohashi, K., and Yun, X. (2007). China's Innovation System Reform and Growing Industry and Science Linkages. Res. Pol. 36, 1251–1260. doi:10.1016/j.respol.2007.02.023
Naranjo, P. G. V., Pooranian, Z., Shojafar, M., Conti, M., and Buyya, R. (2019). FOCAN: A Fog-Supported Smart City Network Architecture for Management of Applications in the Internet of Everything Environments. J. Parallel Distributed Comput. 132, 274–283. doi:10.1016/j.jpdc.2018.07.003
Pérez-Lombard, L., Ortiz, J., and Velázquez, D. (2013). Revisiting Energy Efficiency Fundamentals. Energy Efficiency 6, 239–254. doi:10.1007/s12053-012-9180-8
Simsek, N. (2014). Energy Efficiency with Undesirable Output at the Economy-wide Level: Cross Country Comparison in OECD Sample. Ajer 2, 9–17. doi:10.12691/ajer-2-1-2
Sukharev, S. (2020). Structural Analysis of Income and Risk Dynamics in Models of Economic Growth. Quantitative Finance Econ. 4, 1–18. doi:10.3934/qfe.202000110.3934/qfe.2020018
Vlontzos, G., Niavis, S., and Manos, B. (2014). A DEA Approach for Estimating the Agricultural Energy and Environmental Efficiency of EU Countries. Renew. Sustainable Energ. Rev. 40, 91–96. doi:10.1016/j.rser.2014.07.153
Wagner, M., Bachor, V., and Ngai, E. W. T. (2014). Engineering and Technology Management for Sustainable Business Development: Introductory Remarks on the Role of Technology and Regulation. J. Eng. Technology Management 34, 1–8. doi:10.1016/j.jengtecman.2014.10.003
Wang, Q.-S., Su, C.-W., Hua, Y.-F., and Umar, M. (2020). Can Fiscal Decentralisation Regulate the Impact of Industrial Structure on Energy Efficiency?. Econ. Research-Ekonomska Istraživanja 25, 1–26. doi:10.1080/1331677x.2020.1845969
Xie, B.-C., Shang, L.-F., Yang, S.-B., and Yi, B.-W. (2014). Dynamic Environmental Efficiency Evaluation of Electric Power Industries: Evidence from OECD (Organization for Economic Cooperation and Development) and BRIC (Brazil, Russia, India and China) Countries. Energy 74, 147–157. doi:10.1016/j.energy.2014.04.109
Xiong, S., Ma, X., and Ji, J. (2019). The Impact of Industrial Structure Efficiency on Provincial Industrial Energy Efficiency in China. J. Clean. Prod. 215, 952–962. doi:10.1016/j.jclepro.2019.01.095
Yang, L., and Wang, K.-L. (2013). Regional Differences of Environmental Efficiency of China's Energy Utilization and Environmental Regulation Cost Based on Provincial Panel Data and DEA Method. Math. Computer Model. 58, 1074–1083. doi:10.1016/j.mcm.2012.04.004
Yu, B. (2020). Industrial Structure, Technological Innovation, and Total-Factor Energy Efficiency in China. Environ. Sci. Pollut. Res. 27, 8371–8385. doi:10.1007/s11356-019-07363-5
Yu, B., Xu, L., and Yang, Z. (2016). Ecological Compensation for Inundated Habitats in Hydropower Developments Based on Carbon Stock Balance. J. Clean. Prod. 114, 334–342. doi:10.1016/j.jclepro.2015.07.071
Zhang, J. (2008). Estimation of China's Provincial Capital Stock (1952-2004) with Applications. J. Chin. Econ. Business Stud. 6, 177–196. doi:10.1080/14765280802028302
Zhang, X.-P., Cheng, X.-M., Yuan, J.-H., and Gao, X.-J. (2011). Total-factor Energy Efficiency in Developing Countries. Energy Policy 39, 644–650. doi:10.1016/j.enpol.2010.10.037
Zhao, M., Tan, L., Zhang, W., Ji, M., Liu, Y., and Yu, L. (2010). Decomposing the Influencing Factors of Industrial Carbon Emissions in Shanghai Using the LMDI Method. Energy 35, 2505–2510. doi:10.1016/j.energy.2010.02.049
Zheng, Y., Chen, S., Chen, S., and Wang, N. (2020). Does Financial Agglomeration Enhance Regional green Economy Development? Evidence from China. Green. Financ. 2, 173–196. doi:10.3934/gf.2020010
Zhong, J., and Li, T. (2020). Impact of Financial Development and its Spatial Spillover Effect on Green Total Factor Productivity: Evidence from 30 Provinces in China. Math. Probl. Eng. 2020, 1–11. doi:10.1155/2020/5741387
Zhou, P., Ang, B. W., and Zhou, D. Q. (2012). Measuring Economy-wide Energy Efficiency Performance: A Parametric Frontier Approach. Appl. Energ. 90, 196–200. doi:10.1016/j.apenergy.2011.02.025
Zhou, X., Zhang, J., and Li, J. (2013). Industrial Structural Transformation and Carbon Dioxide Emissions in China. Energy Policy 57, 43–51. doi:10.1016/j.enpol.2012.07.017
Zhou, Z. F. (2014). On Evaluation Model of Green Technology Innovation Capability of Pulp and Paper Enterprise Based on Support Vector Machines. Amr 886, 285–288. doi:10.4028/www.scientific.net/amr.886.285
Keywords: green technology innovation, energy TFP, threshold effect, mediating effect, heterogeneity
Citation: Wang M, Li Y and Liao G (2021) Research on the Impact of Green Technology Innovation on Energy Total Factor Productivity, Based on Provincial Data of China. Front. Environ. Sci. 9:710931. doi: 10.3389/fenvs.2021.710931
Received: 17 May 2021; Accepted: 03 June 2021;
Published: 25 June 2021.
Edited by:
Qiang Ji, Institutes of Science and Development (CAS), ChinaReviewed by:
Nan Zhao, Beijing Normal University, ChinaHuaiying Lei, Tianjin Polytechnic University, China
Copyright © 2021 Wang, Li and Liao. This is an open-access article distributed under the terms of the Creative Commons Attribution License (CC BY). The use, distribution or reproduction in other forums is permitted, provided the original author(s) and the copyright owner(s) are credited and that the original publication in this journal is cited, in accordance with accepted academic practice. No use, distribution or reproduction is permitted which does not comply with these terms.
*Correspondence: Yanling Li, 2112064100@e.gzhu.edu.cn