- 1Department of Chemical Engineering, University of Almería, Almería, Spain
- 2Department of Informatics, University of Almería, Almería, Spain
This paper presents a novel simulation tool to understand and analyze biological models for wastewater treatment processes using microalgae. The models for this type of processes are very complex to be analyzed because of the very different phenomena, variables and parameters involved. The model already included in the tool has been validated at controlled conditions simulating outdoor ones, it being useful to simulate real outdoor cultures. The major contribution of the proposed tool is that these models can be easily and interactively simulated and compared. The tool allows simulating biological models only considering microalgae or including the microalgae-bacteria consortium. Moreover, the simulations can be done only using the solar radiation contribution or by adding the environmental and bacteria effects as cardinal terms. Furthermore, the effects of the wastewater properties or different microalgae strains can be evaluated. The interactive simulations can be performed for selected days as representative of the different year seasons that are already preloaded in the tool. However, the user can also load data from other locations to simulate the models under particular conditions.
Highlights:
Understanding biological models using interactive tools.
Analysis of weather, nutrients and strain type effects on oxygen production.
Studying microalgae-bacteria consortia models with interactivity.
Introduction
Water has become a scarce and limited resource due to its growing consumption in developed industrial countries, contamination of water sources and the lack of efficient technologies for retrieving more usable water (Li et al., 2019). The large volume of wastewater generated should be treated before being discharged into natural watercourses or reused because untreated wastewater discharge pollutes the water bodies and spreads water-related diseases (Singh, 2021). Although the conventional wastewater treatments have shown adequate nutrients removal levels, they imply a high economic cost and a resource waste (Acién et al., 2016). Therefore, in order to solve this situation, some eco-friendly alternatives have appeared for wastewater treatment, which allow obtaining a treated effluent of good quality, an efficient nutrient recovery, and production of energy and/or bioproducts at low cost (Puyol et al., 2017; Patel et al., 2021). From these alternatives, a microalgae-based wastewater treatment process is one of the most promising technologies for the advanced treatment and nutrient recovery of wastewater, and it has attracted more and more attention in recent years. The reason for this increased interest is that the use of microalgae has a dual benefit: economic wastewater treatment and microalgae biomass production, that can be subsequently converted into added-value products such as biofertilizers or animal feed (Craggs et al., 2013; .Guzmán et al., 2020; Acién et al., 2017; Li et al., 2019; Suganya et al., 2016).
Microalgae-based wastewater treatment is performed by complex microalgae-bacteria consortia which varies as a function of the environmental and operational conditions, especially the composition of the wastewater being processed (Acién et al., 2016). Although these interactions have been studied for many years, it is still challenging to understand what are the main processes that occur in microalgae-bacteria systems because most of them take place simultaneously and are strongly interdependent (García et al., 2006). Addressing this challenge, mathematical models have been proposed as a useful tool to understand and optimize biological systems (Bernstein and Carlson, 2012; Klanchui et al., 2012) such as microalgae-bacteria processes (Solimeno and García, 2019; Casagli et al., 2021). During the last decades, different types of mathematical models have been developed for understanding this interaction between microalgae and bacteria for wastewater treatment systems. Since Buhr and Miller (1983) developed the first mathematical model to describe microalgae and bacteria growth in wastewater, more complex models have progressively emerged (Buhr and Miller, 1983; Reichert and Vanrolleghem, 2001; Zambrano et al., 2016; Solimeno et al., 2017). However, due to the complexity of these models and the high number of parameters (more than 50 parameters), it is very difficult to understand and analyze the effect of all of them in a simple way. As a result, many simulations have to be carried out in order to study the effect of all the components involved in the process. This difficulty is evident at both the research level, when a deep analysis of the system is required, and at the understanding level, when the objective is to learn the concepts related to this type of systems.
In recent years, interactive tools and virtual laboratories have been presented as tools that allow simulating highly complex models and control systems quickly and easily. Especially, the interactive tools provide a high potential allowing a real-time interaction between the modification of parameters and the visualization of results (Guzmán et al., 2012). In essence, interactive tools show a graphical interface with dynamic and clickable components, which can be changed in order to visualize the system response immediately, which naturally lends itself to interactivity (Sánchez et al., 2005). These tools have been used successfully in the field of Control Engineering leading to very interesting results (Dormido et al., 2003; Guzmán et al., 2006). Moreover, specific virtual labs have been developed to simulate microalgae growth in photobioreactors, which helps to learn how a microalgae-based system work and to understand how the essential variables are involved in the algae growth (Dormido et al., 2014). However, to the best of the authors' knowledge, no interactive tool or virtual laboratory microalgae-bacteria processes has been developed to date.
Therefore, this work aims to develop an interactive tool based on microalgae mathematical models to visualize the productivity of the system as a function of the main environmental and operational variables that determine microalgal performance. The effect of each parameter is possible to visualize in real time and instantaneously. Specifically, the proposed interactive tool includes four possible models: the microalgae model only with the light effect, the microalgae-bacteria model only with the light effect, and these same two extended models including the effect of other parameters, such as pH, temperature, dissolved oxygen, nitrogen and phosphorous. Furthermore, it is possible to modify the rest of the biological parameters associated with the selected strain as well as the properties of wastewater to be treated. All these analyses can be carried out taking climate and reactor data (solar radiation, pH, dissolved oxygen, and culture temperature) as inputs to the models for different seasons of the year. All the proposed models included in the tool have been validated using experimental data, thus demonstrating its reliability. It is important to remark that the main contribution of this work is the proposed Interactive Tool and the combined implementation of the biological models. The software tool allows to immediately observe the effect of more than 50 parameters interactively, which is not possible in a classical static simulation.
This work is organized as follows. First, the biological models used in the tool are summarized. Next, the models are validated using experimental data obtained at controlled conditions. Then, a detailed description of the interactive tool developed is presented. Afterwards, several illustrative examples are provided to show how the interactive tool can be used under various types of possible scenarios.
Material and Methods
Biological Model
Microalgae wastewater treatment is performed by complex microalgae-bacteria consortia which vary as a function of the environmental and operational conditions (Acién et al., 2016). Under illumination, microalgae perform photosynthesis that turns carbon dioxide (CO2) and water into organic molecules. In this process, microalgae reduce CO2 and split water to release oxygen (O2). This oxygen is essential for the degradation of organic compounds present in wastewater by aerobic bacteria (heterotrophic bacteria). In turn, during bacterial oxidation of organic matter, carbon dioxide is produced and is available for photosynthesis, thereby completing the cycle (Zambrano et al., 2016; Quijano et al., 2017). Apart from heterotrophic bacteria, other bacteria populations appear in wastewater and establish interactions with microalgae, emphasizing the nitrifying bacteria, which perform the nitrification process. During nitrification, nitrifying bacteria transforms ammonium, input from sewage into nitrate, in the presence and with the consumption of oxygen produced through photosynthesis (Vargas et al., 2016) (see Figure 1).
According to the simple scheme proposed, an equilibrium appears in wastewater treatment between the microalgae and the bacteria through the gas exchanges such as oxygen production/consumption. On the one hand, microalgae produce oxygen by photosynthesis and consume part of them for endogenous respiration. Moreover, heterotrophic and nitrifying bacteria consume the released oxygen by respiration. In fact, this makes possible to develop mathematical models based on net oxygen production by microalgae-bacteria consortia in wastewater treatment systems. As a result, and through oxygen production, it is possible to obtain models to predict the biomass productivity in these complex systems.
Mathematical Model Background
Sánchez-Zurano et al. (2021a) developed a dynamic model considering several important environmental parameters (light intensity, temperature, pH, and dissolved oxygen) on microalgae and bacteria growth (Sánchez Zurano et al., 2021b). The model equations were built using experimental data related to the influence of the environmental parameters on the photosynthesis rate and the respiration rate of microalgae-bacteria consortium, distinguishing between microalgae activity, heterotrophic activity, and nitrifying activity and by considering the methodology proposed by (Sánchez-Zurano et al., 2020a). After that, the model was validated using experimental data from a laboratory culture of Scenedesmus sp. growing with wastewater. In reality, three models, one for each microbial population, were developed in order to assemble all of them in one global model. The models were based on measuring the oxygen production rate (PO2) for microalgae and oxygen respiration rate for bacteria (RO2), both under different conditions of light intensity, temperature, pH and dissolved oxygen. The light is considered the decisive parameter which allows obtaining the maximal productivity, and the rest of the parameters (temperature, pH and dissolved oxygen) provide a normalized effect in the models (0–1) and modify the productivity obtained by the light influence. Besides, it must be considered that microalgae-bacteria systems are used to treat wastewaters with different composition, including those from industrial, agricultural and municipal sources. For that, along with environmental conditions, the chemical characteristics of these wastewaters may severely influence microalgae productivity (Kube et al., 2018). Therefore, it is mandatory to include the influence of the main nutrients present in wastewater into the microalgae-bacteria mathematical models.
Apart from light, microalgae require nitrogen (N) and phosphorus (P) for their autotrophic growth, in which they fix inorganic carbon (CO2 and HCO3). Concerning nitrogen, ammonium (NH4+) is the main nitrogen form in wastewater, while nitrate (NO3−) usually appears in a low concentration. Most microalgae can utilize nitrogen in different forms. However, ammonium was known to be preferred by many microalgae because it requires less energy for assimilating (Kim et al., 2016). For that, in the biologic models, the effect of nitrogen on microalgae activity is calculated as a function of the nitrogen in form of ammonium (N-NH4+) present in the culture. In place, only if there is not ammonium in the medium, microalgae consume nitrate and its activity depends on nitrogen in form of nitrate (N-NO3−) concentration. Concerning bacteria activities, the effect of nutrients is considered too. For heterotrophic respiration, the concentration of both N-NH4+ and P-PO43- is included in the model along with the concentration of biodegradable organic matter (Ss), which is calculated as a certain percentage of the total organic matter concentration (COD). During bacterial organic matter oxidation, CO2 is produced, and it is available for photosynthesis and nitrification processes. Moreover, the normalized effect of the N-NH4+ and P-PO43- concentration is taken into account to determine the nitrifying respiration. Therefore, the equations proposed by Sánchez-Zurano et al. (2020c) can be improved by adding the terms related to nitrogen and phosphorous availability (Sánchez-Zurano et al., 2020b), and the effect of the biodegradable organic matter and the inorganic carbon as follows:
Previous models allowed to assemble a global model, ABACO model, to calculate the oxygen production by a microalgae-bacteria consortium in wastewater treatment (Eq. 4):
Finally, ABACO model was calibrated and validated in the laboratory photobioreactors using pig slurry as a nutrient source, demonstrating the validity of the developed model (Sánchez-Zurano et al., 2021b).
Biological Models for the Interactive Tool
As described earlier, processes that occur in microalgae systems are difficult to understand because most of them take place simultaneously and they are strongly interdependent. For that, it is very challenging to understand a microbiological system where a wide variety of metabolic processes coexist and are affected by multiple variables such as solar radiation, temperature, pH, etc. For these reasons, the implementation of an interactive tool that allows the visualization of all these variables and checking their effect on the microalgae-bacteria system is especially useful. The interactive tool proposed in this work includes four different oxygen production models for the microalgae-wastewater treatment problem. A more comprehensive version of the models described in the “Mathematical model background” section is implemented because the effect of the principal nutrients in wastewater treatment (nitrogen and phosphorous) has been also included. The four scenarios proposed in the tool are based on: 1) there are only microalgal cells in wastewater treatment and they are affected by solar radiation; 2) three populations coexist in wastewater treatment (microalga, heterotrophic bacteria and nitrifying bacteria) affected only by solar radiation; 3) the wastewater treatment is performed by microalgae cells and its activity is a function of solar radiation, temperature, pH, dissolved oxygen, nitrogen and phosphorous; 4) finally, the more complex model involves both microalgae and bacteria (heterotrophic and nitrifying bacteria) affected by solar radiation, temperature, pH, dissolved oxygen, nitrogen and phosphorous.
The next sections briefly describe these four different models. Notice that the simulation and comparison of these four different models will allow to obtain a better understanding of the microalgae-bacteria consortium and the effect of all the environmental and operation variables and parameters. More details about the models, the equations and the parameters can be found in Supplementary Table 1.
Light Microalgae Model
The simplest models for describing microalgae growth in different cultivation systems are based on the effect of solar radiation. Despite several light intensity models have been developed to describe microalgae photosynthesis and growth kinetics (Aiba, 1982; Eilers and Peeters, 1988), one of the most accepted models nowadays is the Molina model (Grima et al., 1994) in which the oxygen production is a function of specific maximum photosynthetic rate (PO2,max), average irradiance (Iav), photosynthetic parameter constant (Ik), and a form parameter (n). Using this simple model, it is possible to determine what is the oxygen production by microalgae in a wastewater system according to the following equation:
when only solar radiation is considered. This simplification of the model described by Sánchez-Zurano et al. (2021a) allows to offer an idea of the maximal productivity obtaining in a wastewater bioreactor in which microalgae are the only microbial population and the rest of the operational and environmental parameters do not affect the process.
Light Microalgae-Bacteria Model
Despite the usefulness of the previous model, the actual integrated model considering simultaneous growth of microalgae and bacteria in wastewater treatment should be considered. For this reason, it is recommendable to add the effect of bacteria populations to the model presented in Eq. 6. Thus, if the presence of microalgae and bacteria consortia is considered in wastewater treatment, and only the influence by solar radiation is considered, the new equation is described as follows:
Microalgae Full Model
On the other hand, another comprehensive model for microalgae activity can be obtained by extending the model in Eq. 7 to influence both the environmental parameters (light intensity, temperature, pH and dissolved oxygen) and operational conditions such as culture media composition (nitrogen and phosphorous) and inorganic carbon availability. The inorganic carbon has been calculated according to the equilibrium with carbonate and bicarbonate species by considering a total inorganic carbon (TIC) vale of 100 g L−1 and using the real pH values included in the tool for each specific day. Therefore, a new model only for microalgae cells which oxygen production is determined by solar radiation and the effect of other normalized factors can be obtained:
Microalgae-Bacteria Full Model
Finally, the most complex model for microalgae-bacteria systems can be obtained by including microalgae activity, heterotrophic activity and nitrifying activity. All these microbial populations are affected by the environmental and operational parameters, being solar radiation the decisive factor for determining oxygen production by the consortia. The full model including all the possible parameters and populations is the most realistic model described in this work and the final model used for calculating the real oxygen production of the system (Eq. 8):
Raceway Reactor: Real Dataset
The real data included in the interactive tool for each season (spring, summer, autumn and winter) were collected from a raceway reactor located at the IFAPA Research Centre (Almería, Spain) (Figure 2). The raceway reactor consists of a polypropylene algal pond of two 50 m length channels (0.46 m high × 1 m wide) connected by 180°bends at each end, with a 0.59 m3 sump (0.65 m long × 0.90 m wide × 1 m deep) located 1 m along one of the channels (Barceló-Villalobos et al., 2018). Guide vanes made of polypropylene were placed in the bends of the photobioreactors. In the raceway reactor, the microalgae-bacteria culture is circulated using a rotating paddlewheel actuated by an electric motor. pH is controlled to 8.0 by on-demand injection of pure CO2 in the sump. Also, air is supplied to remove excess dissolved oxygen on demand. Environmental parameters such as pH, temperature and dissolved oxygen in the culture were measured using appropriate probes (5083 T and 5,120, Crison, Barcelona, Spain), connected to an MM44 control-transmitter unit (Crison Instruments, Spain), and data acquisition software (Labview, National Instruments) providing complete monitoring and control of the installation. Also, the solar radiation was collected using an adequate sensor (Pyranometer Kipp & Zonen CM 6B). The reactor is operated in semi-continuous mode throughout the year collecting daily and replaced with fresh wastewater.
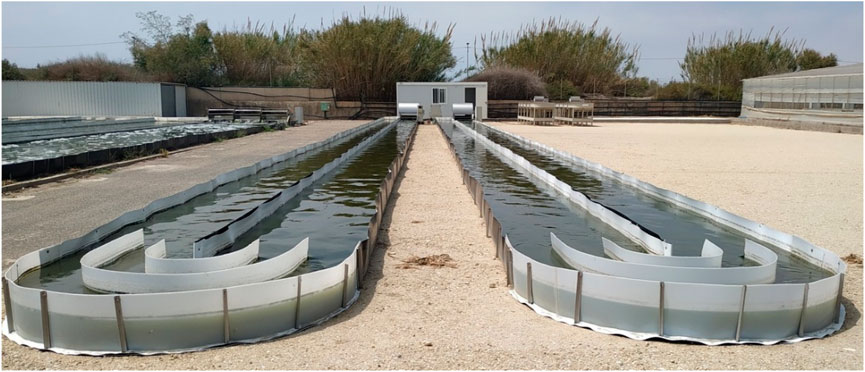
FIGURE 2. Raceway reactor. The real input data for simulating the seasons of the year were collected from the sensor located in it.
By default, representative data from raceway reactors located in Almería (Spain) are preloaded in the tool. However, the user can load his/her own data for a particular analysis. Notice that the dissolved oxygen, pH and temperature balances are not included in the tool, since the objective is the comparison of biological models under the same reactor and weather conditions.
Laboratory Stirred-Tank Reactor: Biologic Model Validation
To validate the model implemented in the proposed interactive tool, experiments were performed in a stirred tank reactor (0.08 m in diameter, 0.2 m in height and with a 1 L capacity) operated at laboratory but simulating outdoor conditions. The reactor was filled with 20% of Scenedesmus sp. inoculum and primary domestic wastewater. The reactor was artificially illuminated using eight 28 W fluorescent tubes (Philips Daylight T5) on a simulated daylight cycle. The culture conditions inside the reactor, such as pH (Crison 5,002, Barcelona, Spain), temperature and dissolved oxygen (Crison 5,002, Barcelona, Spain) were monitored. To prevent the adverse effect of excessive dissolved oxygen accumulation, the dissolved oxygen was controlled and kept below 200%Sat by supplying air on demand. CO2 was likewise injected on demand to control the pH at 8. The oxygen mass transfer coefficient (
Programing Software to Implement the Interactive Tool
Sysquake (Anon), a Matlab-like language with fast execution and excellent facilities for interactive graphics, was used to code the interactive tool for these microalgae-bacteria models. Sysquake allows that the tool is delivered as a stand-alone executable that is readily accessible for free for both professionals and students (Díaz and Dormido, 2015).
Results and Discussion
Full Biologic Model Validation
An experimental validation has been performed to compare the experimental oxygen production by a microalga-bacteria culture and the oxygen production predicted by the full microalgae-bacteria model under particular operational conditions. In practice, the real data obtained from a laboratory stirred-tank reactor, fed with primary domestic wastewater, were compared with the simulated oxygen production by the full microalgae-bacteria model given by Eq. 8, which considers the effect of the whole environmental and operational conditions (light, temperature, pH, dissolved oxygen, nitrogen and phosphorous). For the purpose of this comparative being as precise as possible, the real data from the sensors located in the stirred-tank reactor (radiation, pH, dissolved oxygen and temperature) were introduced in the biologic model to simulate the oxygen production by the microalgae-bacteria consortia. Furthermore, the wastewater properties introduced in the biologic model and used for the simulation were the same nutrient measurements described in “3.1. Laboratory stirred-tank reactor: Tool validation”. Specifically, a five days dataset from the laboratory reactor under different environmental conditions was used. For the validation, the oxygen production was determined as a function of dissolved oxygen in the reactor measured and taking into account the oxygen mass transfer in the reactor. Real data are shown in Figure 3A by dots, while the full model values are represented by a solid line. From this figure, it can be observed that the model highly fits the experimental oxygen produced by the real system for the given days, which confirms the validation of the oxygen production model included in the interactive tool. The maximal oxygen production measured and simulated was 0.3 mgO2/Lmin throughout the test. In Figure 3B, data of radiation, pH, dissolved oxygen and temperature during the 5 days dataset measured in the laboratory stirred-tank reactor are shown, which were the inputs used for the proposed biological model to simulate the data presented in Figure 3A. As observed, these results suggested that the biologic model is capable to reproduce the behavior of the microalgae-bacteria consortia despite the wide environmental variability in parameters as temperature.
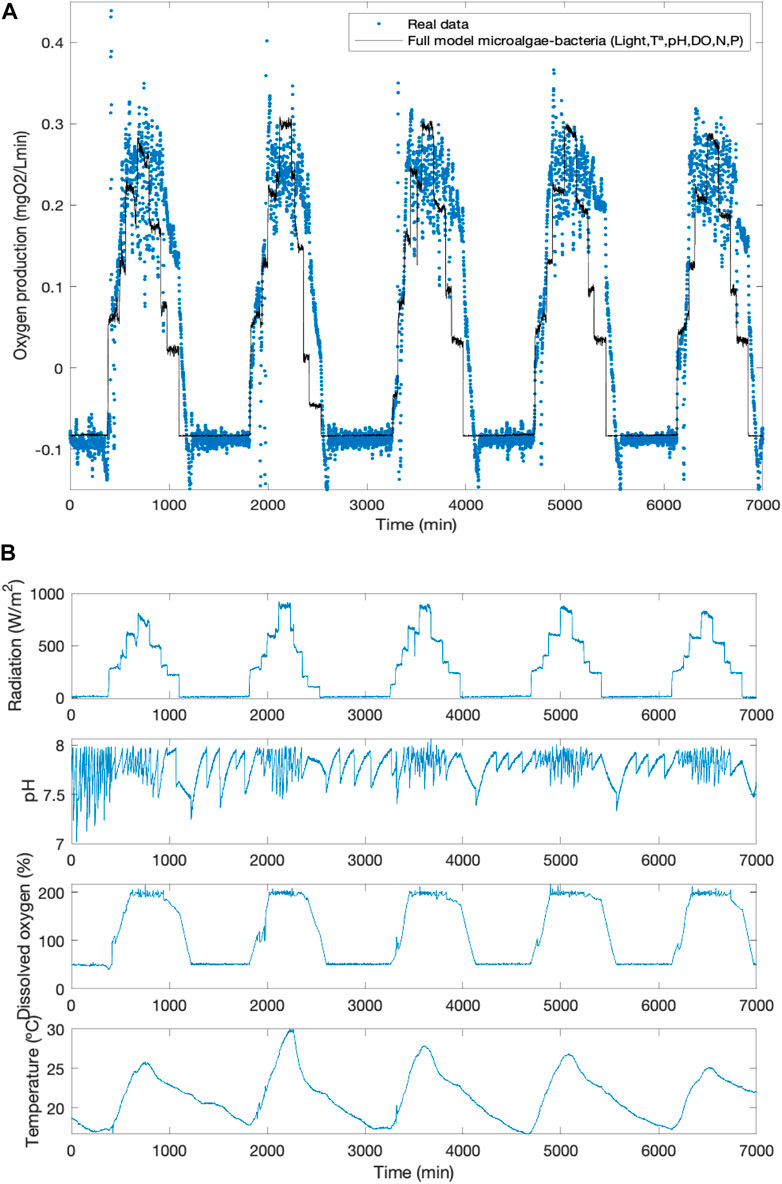
FIGURE 3. Experimental validation of the full microalgae-bacteria model proposed (A), Real input data from the laboratory stirred-tank reactor used for the validation (B).
Interactive Tool Description
Once the theoretical concepts of the microalgae-bacteria models have been summarized, the functionality of the developed tool is described in this section. The tool is freely available through http://www.eu-sabana.eu/ at the Data and Software website section and does not require a Sysquake license to be run. Windows and Mac versions are available for free. On the other hand, a short video tutorial can be found to describe the main capabilities of the tool. Figure 4 shows the main screen of the tool.
The graphic part of the tool has been organized in order to facilitate to the user an understanding of the main biologic components which appear in the models along with the operational and environmental parameters that affected them. Moreover, different microalgae strains and bacteria properties can also be analyzed. The users can easily work simulating all of the proposed models on the screen at the same time, which is very useful for a deeper understanding. Moreover, it is possible to visualize the models throughout the four seasons of the year. All the models are always simulated for 24 h, where real data for pH, dissolved oxygen, solar radiation, and medium temperature are used as input to the models. These real data are modified according to the selected season of the year. Data from the raceway reactor described in Interactive Tool Description is preloaded in the tool by default. However, the user can load his/her own data from the “Load data” available in the tool. Instructions are given at the tool website. In the following, the main features and options of the tool are described.
The left-hand side of the screen is the parameter section, which is divided into two parts: the model parameters part that is located at the upper area of the screen, and the graphical option parameters located at the lower part of the screen. From the model parameters part, it is possible to modify the different wastewater properties such as organic matter concentration (COD), readily biodegradable soluble organic matter (Ss), the nitrogen in form of nitrate N-NO3-, nitrogen in form of ammonium N-NH4+, and phosphorous P-PO4−3 concentrations. Moreover, all the model parameters for microalgae, heterotrophic bacteria and nitrifying bacteria (see Supplementary Tables 2, 3) can be interactively modified in this area. Each parameter value in this area can be changed by using a text field or a slider element. The last one allows easily observing the effect of the corresponding parameter in an interactive manner. On the other hand, the graphical parameter option is focused on switching on or off the graphical results of the models. Two groups of checkboxes are available to show or to hide the plots for the different simulated models or the cardinal elements at the right-hand side of the screen. Furthermore, an option to select the season of the year is shown. Once the season of the year is selected, the real data (that includes pH, dissolved oxygen, solar radiation and medium temperature) of a characteristic day for the selected season is used as input to the models as commented above. Together with this option, a checkbox called “Real Data” is also available to show or to hide the used real data in the plots.
The right-hand side of the screen is devoted to showing the graphical results of the simulated models or the real data used as inputs to the model. When the “Real Data” checkbox is switched on, the real input data is shown in the graphics. The dissolved oxygen and the pH are shown at the top, and the solar radiation and the medium temperature are shown at the bottom. However, when the “Real Data” checkbox is switched off (option by default) the simulation results for the models are presented. As mentioned previously, all the models are simulated for a whole day, where the time scale is shown in minutes. The graphic at the upper part of this area shows the results for the four biological models described by equations 5–8. The plots in the lower graphic show all the cardinal elements involved in the different models. Notice that these two graphics will only show those results that are selected in the graphical parameter option of the tool.
Illustrative Examples
As described above, the multiple options available in the tool allow simulating a large number of possible scenarios with scientific-practical interest. Some of the main ideas that can be analyzed with the tool are listed below:
• To simulate the oxygen production by a microalgae-based system considering the presence or absence of bacteria populations.
• To simulate different microalgae strains with different properties and to observe their behavior under different weather conditions and under the presence/absence of bacteria.
• To evaluate the effect of different biomass concentrations or medium height in the reactor.
• To analyze the differences between the “theoretical oxygen production” (determined by solar radiation parameter) and the more realistic oxygen production, including the environmental and operational variables.
• To take into account and modifying the biological parameters which determining the microalgae and bacteria activities.
• To analyze and to visualize the effect of each factor involved in the biological models. For instance, the effect of each cardinal term.
• To evaluate the biological models in all seasons of the year (summer, spring, autumn and winter).
• To visual the real data used for the simulation tool and to relate it with the effect of the different elements.
In the following, a set of illustrative examples are exposed to show the usefulness of the tool. Notice that the interactive capabilities of the tool are difficult to be shown in a written document. So, we encourage the reader to download it and to evaluate the examples by himself/herself.
Importance of Environmental Factors on Microalgae Productivity
The impact of environmental variables on microalgae growth in outdoor cultivation has been widely reported [33]. Compared to laboratory cultivation, in which most of the environmental conditions are controlled, the diurnal and seasonal fluctuations in irradiance and temperature in outdoor cultures involve potential complications on microalgae activity. This effect has been evaluated in Figure 5 by using the tool, where the four possible biological models presented in this work have been simulated using data from spring and winter seasons. Figure 6 shows the used real input data of pH, dissolved oxygen, medium temperature and solar radiation for these two seasons. The upper plots in Figures 5A,B show the oxygen production by the four biological models for a full day. In this simulation, it can be fully appreciated an oxygen production slight downturn in the models with bacteria compared to biological models which considering only microalgae activity. However, it is remarkable the strong decrease in the oxygen production when environmental and operational factors are considering in microalgae models and microalgae-bacteria models. In the bottom plots of Figures 5A,B, the normalized effect of the environmental parameters (pH, dissolved oxygen and temperature) on the microalgae cells is shown.
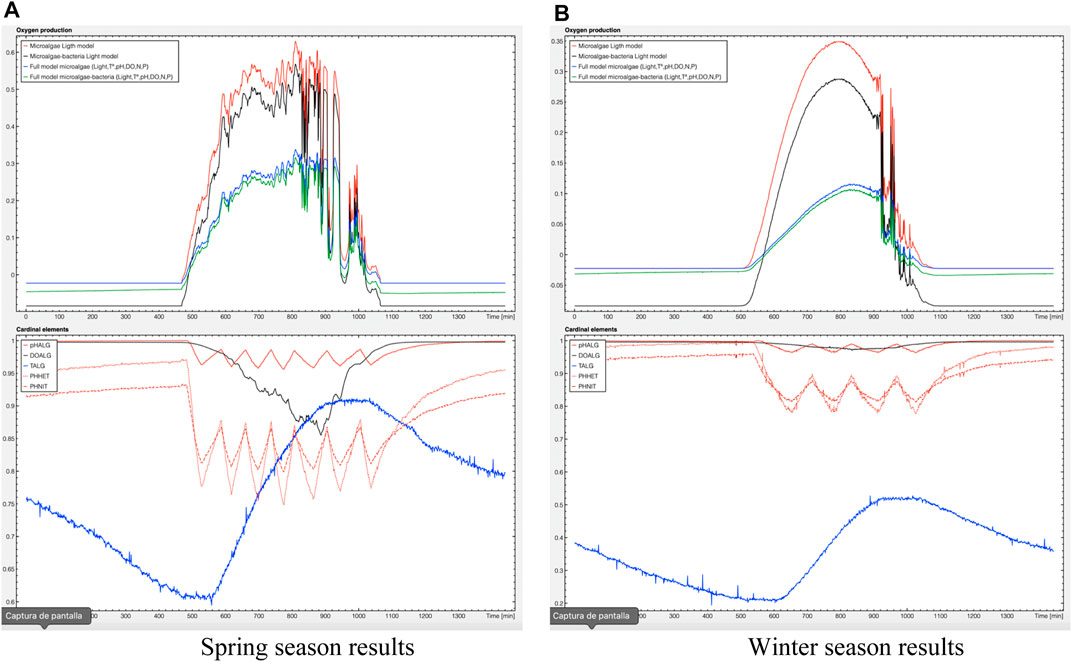
FIGURE 5. Biological models results for different seasonal data to demonstrate the environmental variables effect on the productivity of the microalgae systems.
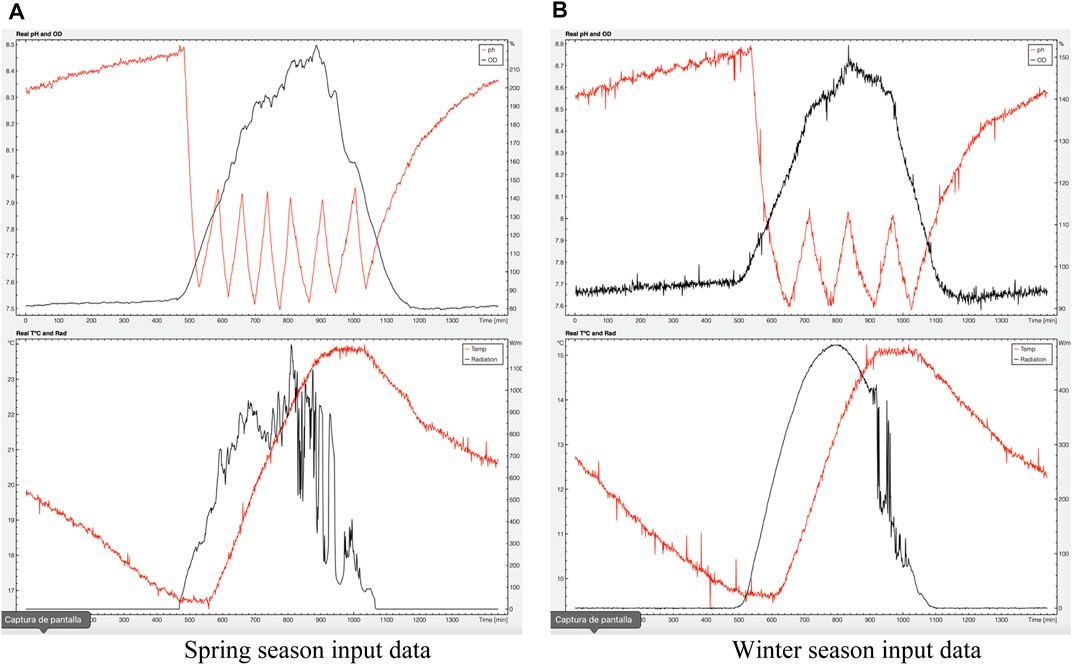
FIGURE 6. Real input data for the examples showed in Figure 2 to demonstrate the environmental variables effect on the productivity of the microalgae systems.
For microalgae growth, pH is one of the most important factors because it deeply influences most of the enzymatic reactions. Despite a majority of microalgae strains have the optimal pH in the neutral to slightly alkaline range, this is characteristic of each one and should be considered in the mathematical models. Moreover, it should be noted that during the photosynthetic activity, an increase in pH is produced and it should be controlled to avoid inhibitory effects. Currently, a possible strategy to mitigate this negative effect on microalgae activity is to control the pH with injection of CO2 on demand (Duarte-Santos et al., 2016). As may be seen in the figure, a good pH control make it possible that microalgae can be cultivated in optimal conditions and their production is not affected by abrupt pH changes due to photosynthetic activity. A similar behavior is observed in the dissolved oxygen because microalgae release a large quantity of oxygen during the light hours by photosynthesis. Part of this oxygen is removing by the oxygen desorption process while the rest remains in the culture reducing the photosynthesis rate and favoring the photorespiration of the culture (Costache et al., 2013; Rubio et al., 1999). To solve this problem, most microalgae systems have an air injection system to remove the oxygen excess. Therefore, if pH and dissolved oxygen are well controlled, they will not have a drastic negative effect on productivity. Finally, the temperature is one of the most crucial factors in the open microalgae-bacteria systems because can modify the microalgae-bacteria growth. To evaluate the temperature effect on microalgae system, three questions should be considered: i) it is not feasible to control the temperature of the culture; ii) optimal temperature depends on each microalgal species (mesophilic, thermophilic and psychrophilic strains); iii) temperature fluctuations depend on geographic localization and season of the year (Ras et al., 2013). In the figure, it is observed that during the spring season, the temperature in the light hours rises up to 24–25°C, which are considering favorable values for the microalgae strain evaluated (Scenedesmus almeriensis). However, when it is evaluating the oxygen production in winter (Figure 3B), the situation is markedly different due to the low temperatures (10–15°C). No different effects with respect to the rest of the variables (dissolved oxygen and pH) between both seasons were observed. As multiple authors have described, the temperature has remarkable effects on microalgae systems which implicated that below or above optimal temperatures, the activity drastically decreases (Costache et al., 2013; Ras et al., 2013). Therefore, biological models coupled with models of the temperature evolution in the reactors could be especially useful for researchers in microalgae fields to predict the weather impact on the microalgae cultures. These predictions/simulations, along with economic strategies such as regulated the weight of the cultures or covered the systems (greenhouse effect), will allow maximizing the biomass productivity.
Influence of Wastewater Composition on Microalgae-Bacteria Systems
Microalgae-based technologies have been used for the treatment of human sewage, industrial wastes, and other wastes such as piggery effluent or effluent from food processing factories (Abdel-Raouf et al., 2012). However, most of the microalgae systems used wastewaters from the wastewater treatment plant, in which different types of wastewater are found, noting wastewaters after primary treatment when the solids and fats are removed or the centrate from anaerobic digestion, which contains a high contaminant concentration (Acién et al., 2016). The composition of these wastewaters varies significantly for some reasons such as the location tested and the variations in water consumption in households or the predominant activities in the surrounding area (agriculture, industry, farms, etc.) (Henze et al., 2015). All these wastewaters contain the main nutrients required for microalgae and bacteria growth: organic matter (COD), nitrogen (N) and phosphorus (P). However, the concentrations of them vary between the wastewater used and have the potential to affect the productivities of microalgae-bacteria cultures significantly. To evaluate the influence of nitrogen, phosphorous and organic matter concentrations on microalgae-bacteria systems, the use of three types of wastewater for microalgae production has been simulated with the tool: primary domestic wastewater, centrate from anaerobic digestion, and pig manure during the summer season (Figure 7). The composition of these wastewaters is shown in Table 1 (Acién et al., 2016). Wastewater from primary treatment contains an adequate level of nutrients for microalgae growth, being ammonium the most frequent nitrogen source with concentrations ranging from 0 to 100 mg L−1, while the concentration of nitrate is significantly less. In Figure 7A, it possible to appreciate the oxygen production expected when primary domestic wastewater is used. With these wastewater properties and using the spring real data, the maximal oxygen production obtained with the full microalgae-bacteria model would be 0.2 mgO2/L min. Given these nutrients values, one may note that both heterotrophic and nitrifying bacteria are in optimal growth conditions ranged the normalized effect of nitrogen, phosphorous and organic matter between 0.9 and 1. For microalgae activity, the nitrogen concentration is remarkable because its normalized effect is 0.7, which corresponds to 30% less than the possible maximal value. However, the use of centrate (Figure 7B) and pig manure (Figure 7C) for microalgae production, involve a strong reduction in oxygen production, being 0.12 and 0.06 mgO2/L min respectively. These data are caused by the high values of ammonium in the medium (506.5 mg L−1 N-NH4+ for centrate and 2,900 mg L−1 N-NH4+ for pig manure). It has been largely reported that ammonium reduces the performance of microalgae cultures, especially at concentrations upper than 100 mg L−1 (Cabanelas et al., 2013). Values above this limit are commonly found in centrate and animal manure, making it necessary to dilute this effluent prior to use as the culture medium inside the reactor (García et al., 2017). For that, knowing the exact composition of the wastewater to be treated is mandatory for an optimal treatment process and biomass production, especially to determine if additional carbon, nitrogen, or phosphorus need to be added when a low nutrient concentration appears. By contrast, the use of wastewaters that contain high nutrients values (such as centrate or animal manure), require a prior dilution to avoid inhibition caused by an excess of ammonium or others micropollutants, such as heavy metals. Moreover, this type of wastewaters should be diluted before use, because of their color could prevent light penetration (Acién et al., 2016). Therefore, this interactive tool allows us to simulate different scenarios, without the need to carry out long experiments, to obtain an approximation of the productivity of the wastewater treatment process based on microalgae-bacteria consortia.
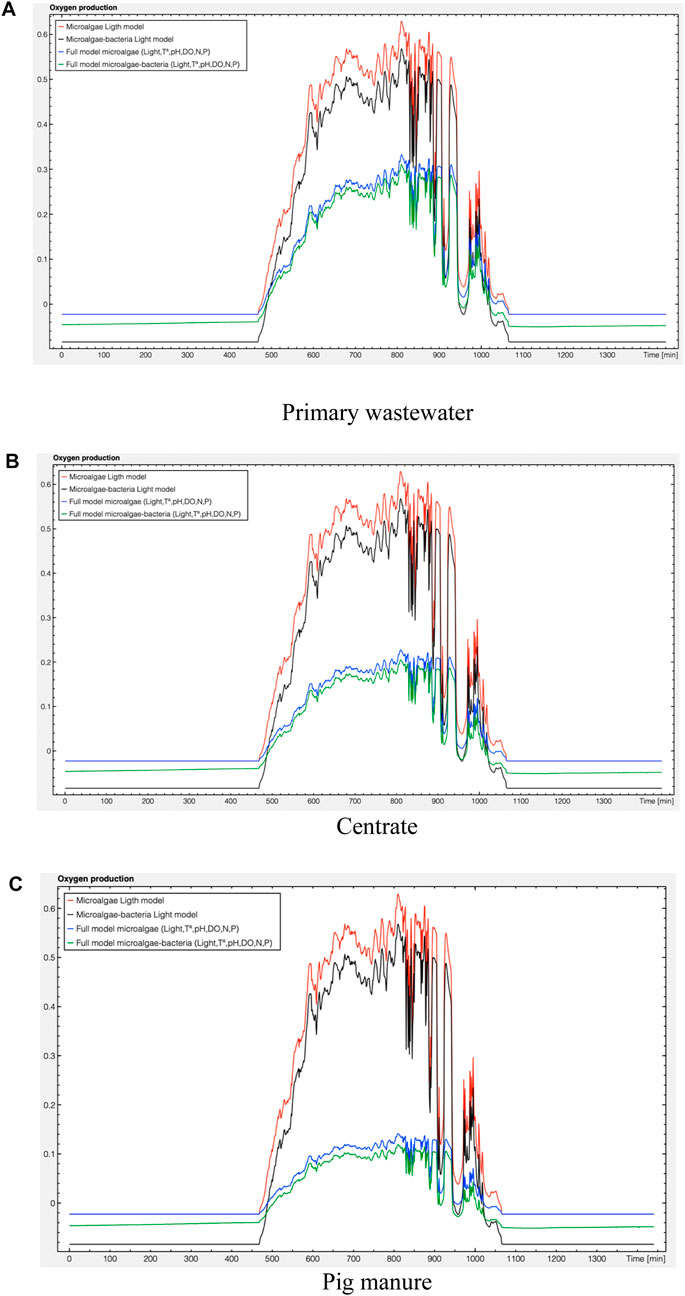
FIGURE 7. Application of the biological models to predict the productivity of the microalgal based system using different types of wastewater: (A) wastewater from primary treatment; (B) centrate from anaerobic digestion; (C) Pig manure.
Production of Specific Microalgae in Wastewater for Industrial Purposes
Currently, the production of some specific microalgal strains has attracted considerable interest worldwide due to their applications in biofuels, animal food ingredients or agriculture. However, a pure production of microalgae may remain costly for animal feed or agricultural purposes. Despite the wastewater cultivation has made microalgae biotechnology sustainable and economically viable, several other questions related to microalgae production should be considered. As previously mentioned, if pH and dissolved oxygen are controlled and an adequate composition is achieved in the influent wastewater, the two determining factors for production would be light and temperature. In Almería, the light conditions are adequate to maintain production throughout the year (San Pedro et al., 2015). However, the temperature is still a challenge. In this example, we propose the production of two strains of microalgae with different industrial/ commercial interests in Almería (using the actual real data registered) and the minimum, maximum and optimal temperatures reported in (Bernard and Rémond, 2012). The proposed strains are Dunaliella tertiolecta (Tamin = 5°C; Tamax = 38.9°C; Taopt = 32.6°C) and Nannochloropsis oceanica (Tamin = -0.2°C; Tamax = 33.3°C; Taopt = 26.7°C). The first one, Dunaliella tertiolecta has been proposed as a potential candidate for biofuels production because of its high oil content and rapid growth rates (Tang et al., 2011). In Figure 8, the oxygen production by Dunaliella tertiolecta is shown in spring (Figure 8A), summer (Figure 8B), autumn (Figure 8C) and winter (Figure 8D) along with the cardinal effect of temperature using its temperature specific parameters. The rest of parameters are the predetermined by the model and the wastewater used is from primary treatment. The model shows the strong influence of temperature on oxygen production by Dunaliella tertiolecta. Due to its optimal temperature is above 30°C, the major productivity is observed in summer by reaching an oxygen production around 0.22 mgO2/Lmin. The oxygen production decreases both in spring and autumn owing to the falling temperature, which a temperature effect on microalgae cells of 0.6–0.7 at noon. Moreover, the microalgae activity has reduced sharply in winter, being the oxygen production 0.03 mgO2/Lmin. This is presumably because of the low temperatures registered in the winter season with a cardinal effect ranged from 0.05 to 0.2. These results reveal that this strain should be produced in summer periods and even so in spring or autumn seasons (accepting the decrease in productivity), but it is not recommendable in winter. Another microalga with special interest because of its significant capacity to accumulate lipids and various bioactive compounds is Nannochloropsis, widely used for biodiesel fuel and as an aquaculture feed (Li et al., 2020). In Figure 9, the production of Nannochloropsis oceanica has been evaluated in Almería during spring (Figure 9A), summer (Figure 9B), autumn (Figure 9C) and winter (Figure 9D). Both the oxygen production and the temperature cardinal effects are shown. In these figures, it is possible to observe that the best period to cultivate Nannochloropsis oceanica is spring with an oxygen production around 0.2 mgO2/Lmin. This productivity decreases in autumn (0.15 mgO2/Lmin), while it is not possible to produce this strain in summer because of the high temperatures such as it can be seen in Figure 9B, with negative values in the temperature cardinal element. Besides, the production in winter is possible but obtaining a low productivity (0.06 mgO2/Lmin). The results from this section reveal the importance of learning about the selected strains before their large-scale production because of the biological aspects of each microalgal strain are crucial to maximize the economic efficiency of the process. In addition, it should be borne in mind that the production of strains has been evaluated using the environmental conditions of Almería, which must be adapted to other locations, since some microalgae cultures in Almería in summer are unfeasible due to high temperatures but may be perfectly valid in the same season but in another location.
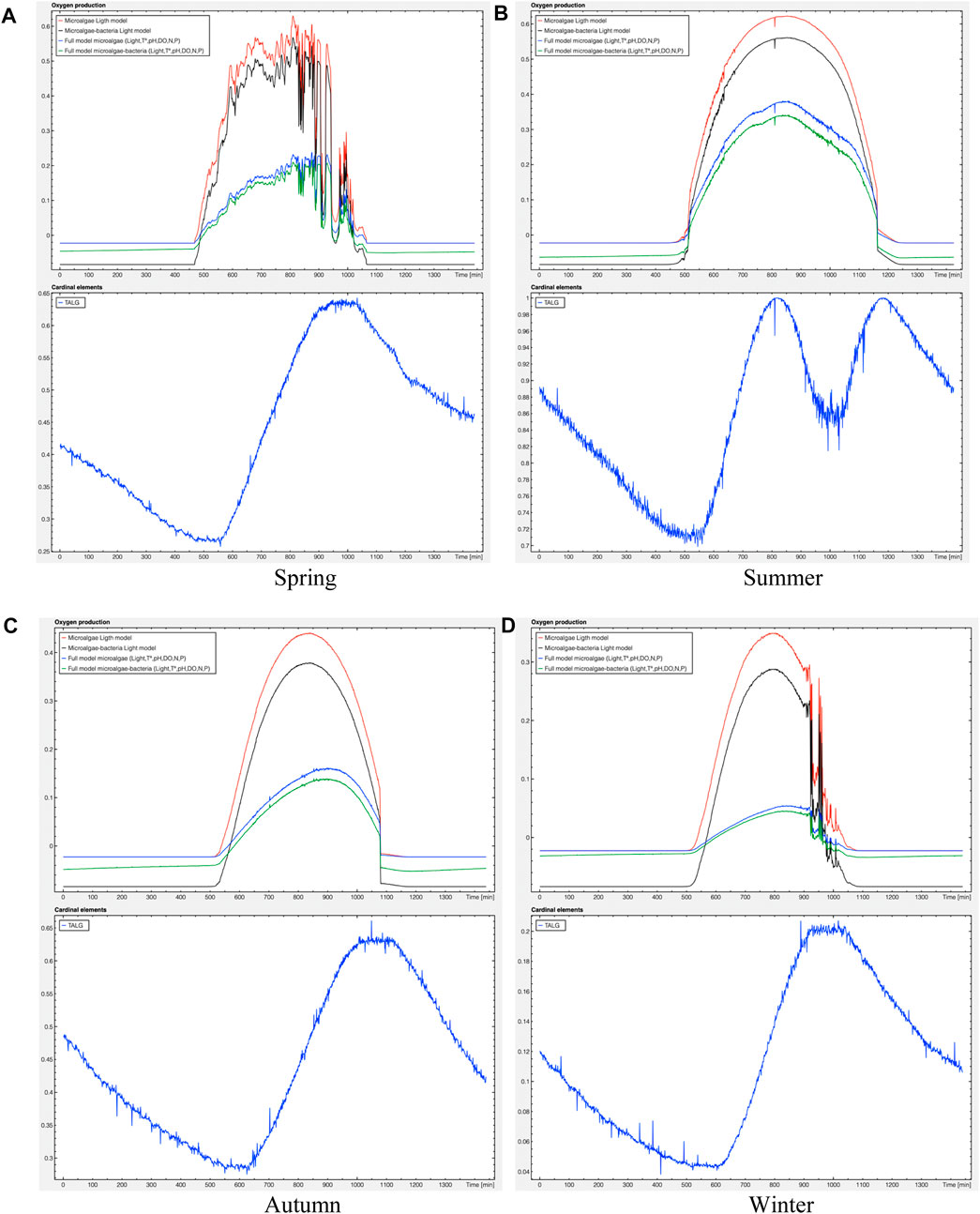
FIGURE 8. Oxygen production by Dunaliella tertiolecta during the seasons of the year using the proposed biological models, along with the temperature effect using its temperature specific parameters: spring (Panel 8A) summer (Panel 8B), autunm (Panel 8C), winter (Panel 8D).
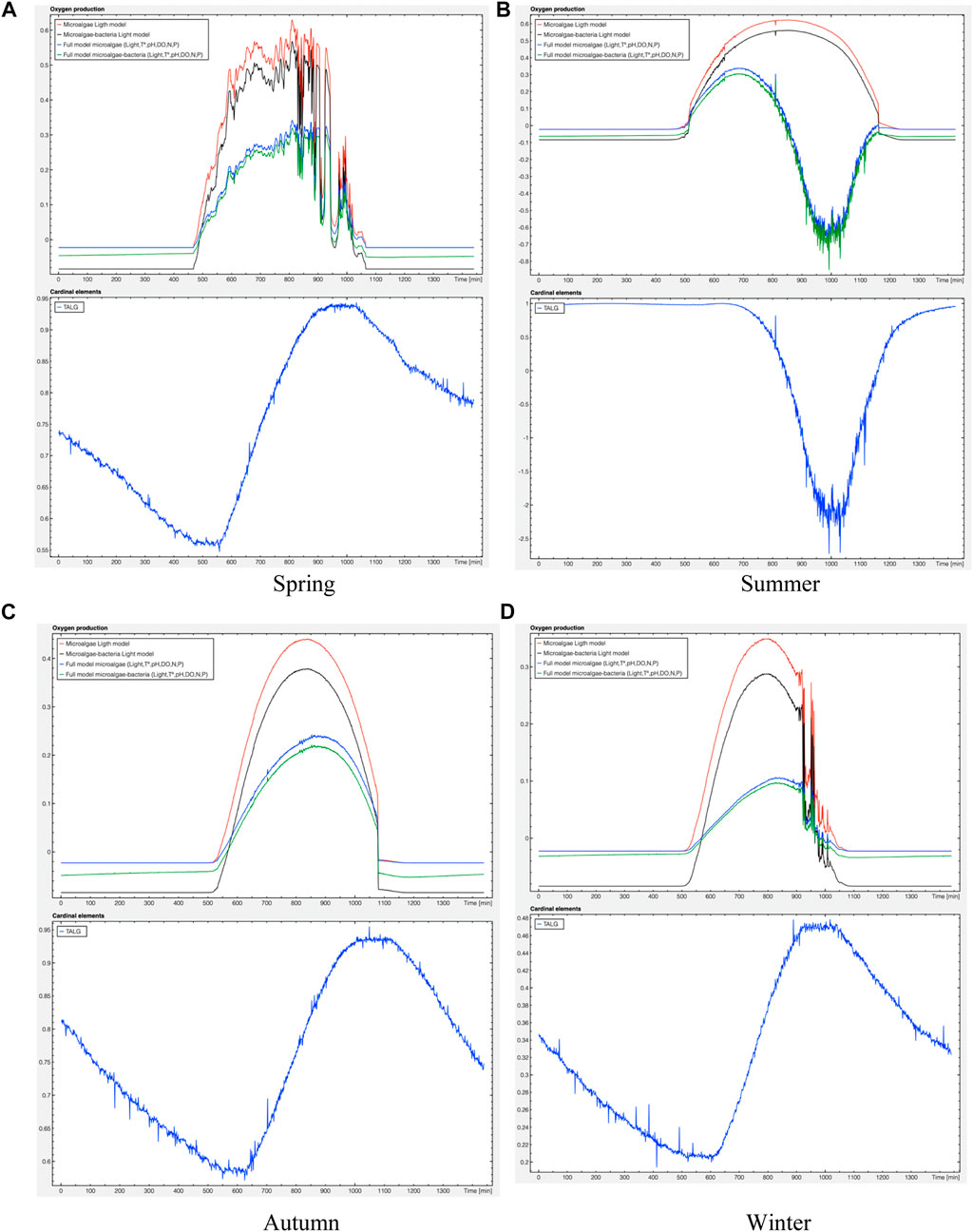
FIGURE 9. Oxygen production by Nannochloropsis oceanica during the seasons of the year using the proposed biological models, along with the temperature effect using its temperature specific parameters: spring (Panel 9A) summer (Panel 9B), autunm (Panel 9C), winter (Panel 9D).
Conclusion
Microalgae-bacteria biological models have been proposed and experimentally validated. These models include many equations and parameters and are difficult to interpret in a simple and practical way. However, the use of interactive tools is presented as a promising alternative not only for understanding microalgae-bacteria processes, but also it is a possible solution to predict the productivity of microalgae-bacteria system and consequently, to avoid long experiments, waiting time, and additional costs. Furthermore, the tool allows to obtain fast simulations and make interactive comparisons that very useful for a deep understanding. Notice that some examples have been included in this paper to show the capabilities of the proposed tool, but many other scenarios can be easily simulated with a high scientific interest.
Data Availability Statement
The original contributions presented in the study are included in the article/Supplementary Material, further inquiries can be directed to the corresponding author.
Author Contributions
AS-Z: investigation, data curation and original draft preparation/writing. JG: Conceptualization, software and reviewing. FA: Conceptualization, supervision, and funding acquisition. JF-S: Editing and funding acquisition.
Funding
This work has been partially funded by the following projects: DPI 2017 84259-C2- 1-R (financed by the Spanish Ministry of Science and Innovation and EU-ERDF funds), the European Union’s Horizon 2020 Research and Innovation Program under Grant Agreement No. 727874 SABANA and the PURASOL project CTQ 2017-84006-C3-3-R (financed by the Spanish Ministry of Economy and Competitiveness). As well as being supported by the Spanish Ministry of Education through the National FPU Program (grant number FPU16/05996).
Conflict of Interest
The authors declare that the research was conducted in the absence of any commercial or financial relationships that could be construed as a potential conflict of interest.
Publisher’s Note
All claims expressed in this article are solely those of the authors and do not necessarily represent those of their affiliated organizations, or those of the publisher, the editors and the reviewers. Any product that may be evaluated in this article, or claim that may be made by its manufacturer, is not guaranteed or endorsed by the publisher.
Supplementary Material
The Supplementary Material for this article can be found online at: https://www.frontiersin.org/articles/10.3389/fenvs.2021.721324/full#supplementary-material
References
Abdel-Raouf, N., Al-Homaidan, A. A., and Ibraheem, I. B. M. (2012). Microalgae and Wastewater Treatment. Saudi J. Biol. Sci. Elsevier. 19 (3), 257–275. doi:10.1016/j.sjbs.2012.04.005
Acién, F. G., Gómez-Serrano, C., Morales-Amaral, M. M., Fernández-Sevilla, J. M., and Molina-Grima, E. (2016). Wastewater Treatment Using Microalgae: How Realistic a Contribution Might it Be to Significant Urban Wastewater Treatment? Appl. Microbiol. Biotechnol. 100, 9013–9022. doi:10.1007/s00253-016-7835-7
Acién, F. G., Molina, E., Reis, A., Torzillo, G., Zittelli, G. C., Sepúlveda, C., et al. (2017). “Photobioreactors for the Production of Microalgae,” in Microalgae-Based Biofuels Bioprod. Editors C. Gonzalez-Fernandez, and R Muñoz (Amsterdam: Woodhead Publishing. Woodhead Publishing Series in Energy), 1–44. doi:10.1016/b978-0-08-101023-5.00001-7
Aiba, S. (1982). Growth Kinetics of Photosynthetic Microorganisms. Berlin, Heidelberg: Springer, 85–156. doi:10.1007/3540116982_3
Barceló-Villalobos, M., Guzmán Sánchez, J. L., Martín Cara, I., Sánchez Molina, J. A., and Acién Fernández, F. G. (2018). Analysis of Mass Transfer Capacity in Raceway Reactors. Algal Res. 35, 91–97. doi:10.1016/j.algal.2018.08.017
Bernard, O., and Rémond, B. (2012). Validation of a Simple Model Accounting for Light and Temperature Effect on Microalgal Growth. Bioresour. Technol. 123, 520–527. doi:10.1016/j.biortech.2012.07.022
Bernstein, H. C., and Carlson, R. P. (2012). Microbial Consortia Engineering for Cellular Factories: In Vitro to In Silico Systems. Comput. Struct. Biotechnol. J. Res. Netw. Comput. Struct. Biotechnol. 3 (4), e201210017. doi:10.5936/csbj.201210017
Buhr, H. O., and Miller, S. B. (1983). A Dynamic Model of the High-Rate Algal-Bacterial Wastewater Treatment Pond. Water Res. 17, 29–37. doi:10.1016/0043-1354(83)90283-x
Cabanelas, I. T. D., Ruiz, J., Arbib, Z., Chinalia, F. A., Garrido-Pérez, C., Rogalla, F., et al. (2013). Comparing the Use of Different Domestic Wastewaters for Coupling Microalgal Production and Nutrient Removal. Bioresour. Technol. 131, 429–436. doi:10.1016/j.biortech.2012.12.152
Casagli, F., Zuccaro, G., Bernard, O., Steyer, J.-P., and Ficara, E. (2021). ALBA: A Comprehensive Growth Model to Optimize Algae-Bacteria Wastewater Treatment in Raceway Ponds. Water Res. 190, 116734. doi:10.1016/j.watres.2020.116734
Costache, T. A., Acién Fernández, F. G., Morales, M. M., Fernández-Sevilla, J. M., Stamatin, I., and Molina, E. (2013). Comprehensive Model of Microalgae Photosynthesis Rate as a Function of Culture Conditions in Photobioreactors. Appl. Microbiol. Biotechnol. 97, 7627–7637. doi:10.1007/s00253-013-5035-2
Craggs, R. J., Lundquist, T. J., and Benemann, J. R. (2013). Wastewater Treatment and Algal Biofuel Production. Algae for Biofuels and Energy. Netherlands: Springer, 153–163. doi:10.1007/978-94-007-5479-9_9
Díaz, J. M., and Dormido, S. (2015). ITADLS: An Interactive Tool for Analysis and Design of Linear Systems. IFAC-PapersOnLine 48, 253–258. doi:10.1016/j.ifacol.2015.11.245
Documentation – Calerga. Available at: https://calerga.com/doc/index.html.
Dormido, S., Dormido-Canto, S., Berenguel, M., and Rodríguez, F. (2003). Interactive Learning of Constrained Generalized Predictive Control. IFAC Proc. 36, 175–180. doi:10.1016/s1474-6670(17)33675-3
Dormido, R., Sánchez, J., Duro, N., Dormido-Canto, S., Guinaldo, M., and Dormido, S. (2014). An Interactive Tool for Outdoor Computer Controlled Cultivation of Microalgae in a Tubular Photobioreactor System. Sensors 14, 4466–4483. doi:10.3390/s140304466
Duarte-Santos, T., Mendoza-Martín, J. L., Acién Fernández, F. G., Molina, E., Vieira-Costa, J. A., and Heaven, S. (2016). Optimization of Carbon Dioxide Supply in Raceway Reactors: Influence of Carbon Dioxide Molar Fraction and Gas Flow Rate. Bioresour. Technol. 212, 72–81. doi:10.1016/j.biortech.2016.04.023
Eilers, P. H. C., and Peeters, J. C. H. (1988). A Model for the Relationship between Light Intensity and the Rate of Photosynthesis in Phytoplankton. Ecol. Model. 42, 199–215. doi:10.1016/0304-3800(88)90057-9
García, J., Green, B. F., Lundquist, T., Mujeriego, R., Hernández-Mariné, M., and Oswald, W. J. (2006). Long Term Diurnal Variations in Contaminant Removal in High Rate Ponds Treating Urban Wastewater. Bioresour. Technol. 97, 1709–1715. doi:10.1016/j.biortech.2005.07.019
García, D., Posadas, E., Grajeda, C., Blanco, S., Martínez-Páramo, S., Acién, G., et al. (2017). Comparative Evaluation of Piggery Wastewater Treatment in Algal-Bacterial Photobioreactors under Indoor and Outdoor Conditions. Bioresour. Technol. 245, 483–490. doi:10.1016/j.biortech.2017.08.135
Grima, E. M., Camacho, F. G., Pérez, J. A. S., Sevilla, J. M. F., Fernández, F. G. A., and Gómez, A. C. (1994). A Mathematical Model of Microalgal Growth in Light-Limited Chemostat Culture. J. Chem. Technol. Biotechnol. 61, 167–173. doi:10.1002/jctb.280610212
Guzmán, J. L., Åström, K. J., Dormido, S., Hägglund, T., and Piguet, Y. (2006). Interactive Learning Modules for PID Control. IFAC Proceedings 39, 7–12. doi:10.3182/20060621-3-es-2905.00003
Guzmán, J. L., Rivera, D. E., Dormido, S., and Berenguel, M. (2012). An Interactive Software Tool for System Identification. Adv. Eng. Softw. 45, 115–123. doi:10.1016/j.advengsoft.2011.09.013
Guzmán, J. L., Acién, F. G., and Berenguel, M. (2020). Modelado y control de la producción de microalgas en fotobiorreactores industriales. Rev. Iberoam. Autom. Inform. Ind. 18, 1–5. doi:10.4995/riai.2020.13604
Henze, M., van Loosdrecht, M. C. M., Ekama, G. A., and Brdjanovic, D. (2015). Biological Wastewater Treatment: Principles, Modelling and Design. Water Intell. Online 7, 9781780401867. doi:10.2166/9781780401867
Kim, G., Mujtaba, G., and Lee, K. (2016). Effects of Nitrogen Sources on Cell Growth and Biochemical Composition of marine Chlorophyte Tetraselmis Sp. For Lipid Production. Algae 31, 257–266. doi:10.4490/algae.2016.31.8.18
Klanchui, A., Vorapreeda, T., Vongsangnak, W., Khannapho, C., Cheevadhanarak, S., and Meechai, A. (2012). Systems Biology and Metabolic Engineering of Arthrospira Cell Factories. Comput. Struct. Biotechnol. J. Res. Netw. Comput. Struct. Biotechnol. 3, e201210015. doi:10.5936/csbj.201210015
Kube, M., Jefferson, B., Fan, L., and Roddick, F. (2018). The Impact of Wastewater Characteristics, Algal Species Selection and Immobilisation on Simultaneous Nitrogen and Phosphorus Removal. Algal Res. 31, 478–488. doi:10.1016/j.algal.2018.01.009
Li, K., Liu, Q., Fang, F., Luo, R., Lu, Q., Zhou, W., et al. (2019). Microalgae-based Wastewater Treatment for Nutrients Recovery: A Review. Bioresour. Technol. 291, 121934. doi:10.1016/j.biortech.2019.121934
Li, T., Chen, Z., Wu, J., Wu, H., Yang, B., Dai, L., et al. (2020). The Potential Productivity of the Microalga, Nannochloropsis Oceanica SCS-1981, in a Solar Powered Outdoor Open Pond as an Aquaculture Feed. Algal Res. 46, 101793. doi:10.1016/j.algal.2020.101793
Patel, A., Arkatkar, A., Singh, S., Rabbani, A., Solorza Medina, J. D., Ong, E. S., et al. (2021). Physico-chemical and Biological Treatment Strategies for Converting Municipal Wastewater and its Residue to Resources. Chemosphere 282, 130881. doi:10.1016/j.chemosphere.2021.130881
Puyol, D., Batstone, D. J., Hülsen, T., Astals, S., Peces, M., and Krömer, J. O. (2017). Resource Recovery from Wastewater by Biological Technologies: Opportunities, Challenges, and Prospects. Front. Microbiol. Front. Media S.A. doi:10.3389/fmicb.2016.02106
Quijano, G., Arcila, J. S., and Buitrón, G. (2017). Microalgal-bacterial Aggregates: Applications and Perspectives for Wastewater Treatment. Biotechnol. Adv. 35, 772–781. doi:10.1016/j.biotechadv.2017.07.003
Ras, M., Steyer, J.-P., and Bernard, O. (2013). Temperature Effect on Microalgae: A Crucial Factor for Outdoor Production. Rev. Environ. Sci. Biotechnol. 12, 153–164. doi:10.1007/s11157-013-9310-6
Reichert, P., and Vanrolleghem, P. (2001). Identifiability and Uncertainty Analysis of the River Water Quality Model No. 1 (RWQM1). Water Sci. Technol. A. J. Int. Assoc. Water Pollut. Res. 43, 329–338. doi:10.2166/wst.2001.0442
Rubio, F. C., Fernández, F. G. A. n., Pérez, J. A. S. n., Camacho, F. G. a., and Grima, E. M. (1999). Prediction of Dissolved Oxygen and Carbon Dioxide Concentration Profiles in Tubular Photobioreactors for Microalgal Culture. Biotechnol. Bioeng. 62, 71–86. doi:10.1002/(sici)1097-0290(19990105)62:1<71::aid-bit9>3.0.co;2-t
Sánchez, J., Dormido, S., and Esquembre, F. (2005). The Learning of Control Concepts Using Interactive Tools. Comput. Appl. Eng. Educ. 13, 84–98. doi:10.1002/cae.20033
San Pedro, A., González-López, C. V., Acién, F. G., and Molina-Grima, E. (2015). Outdoor Pilot Production of Nannochloropsis Gaditana: Influence of Culture Parameters and Lipid Production Rates in Raceway Ponds. Algal Res. 8, 205–213. doi:10.1016/j.algal.2015.02.013
Sánchez-Zurano, A., Gómez-Serrano, C., Acién-Fernández, F. G., and Fernández-Sevilla, J. M. (2020a). Modeling of Photosynthesis and Respiration Rate for Microalgae-Bacteria Consortia. Biotechnol. Bioeng. 118, 952–962. doi:10.1002/bit.27625
Sánchez-Zurano, A., Gómez-Serrano, C., Acién-Fernández, F. G., and Fernández-Sevilla, J. M. (2020b). Influence of Nutrient Availability on the Photosynthesis/respiration Rates and the Nutrient Yield Coefficients of Scenedesmus Almeriensis. Appl. Microbiol. Biotechnol. Under Revi.
Sánchez-Zurano, A., Gómez-Serrano, C., Acién-Fernández, F. G., Fernández-Sevilla, J. M., and Molina-Grima, E. (2020c). A Novel Photo-Respirometry Method to Characterize Consortia in Microalgae-Related Wastewater Treatment Processes. Algal Res. 47, 101858. doi:10.1016/j.algal.2020.101858
Sánchez-Zurano, A., Serrano, C. G., Acién-Fernández, F. G., Fernández-Sevilla, J. M., and Molina Grima, E. (2021a). Modeling of Photosynthesis and Respiration Rate for Microalgae–Bacteria Consortia. Biotechnol. Bioeng. 118, 952. doi:10.1002/bit.27625
Sánchez-Zurano, A., Rodríguez-Miranda, E., Guzmán, J. L., Acién-Fernández, F. G., Fernández-Sevilla, J. M., and Molina Grima, E. (2021b). ABACO: A New Model of Microalgae-Bacteria Consortia for Biological Treatment of Wastewaters. Appl. Sci. 11, 998. doi:10.3390/app11030998
Singh, A. (2021). A Review of Wastewater Irrigation: Environmental Implications. Resour. Conserv. Recycl. 168, 105454. doi:10.1016/j.resconrec.2021.105454
Solimeno, A., and García, J. (2019). Microalgae and Bacteria Dynamics in High Rate Algal Ponds Based on Modelling Results: Long-Term Application of BIO_ALGAE Model. Sci. Total Environ. 650, 1818–1831. doi:10.1016/j.scitotenv.2018.09.345
Solimeno, A., Acíen, F. G., and García, J. (2017). Mechanistic Model for Design, Analysis, Operation and Control of Microalgae Cultures: Calibration and Application to Tubular Photobioreactors. Algal Res. 21, 236–246. doi:10.1016/j.algal.2016.11.023
Suganya, T., Varman, M., Masjuki, H. H., and Renganathan, S. (2016). Macroalgae and Microalgae as a Potential Source for Commercial Applications along with Biofuels Production: A Biorefinery Approach. Renew. Sustain. Energ. Rev. 55, 909–941. doi:10.1016/j.rser.2015.11.026
Tang, H., Abunasser, N., Garcia, M. E. D., Chen, M., Simon Ng, K. Y., and Salley, S. O. (2011). Potential of Microalgae Oil from Dunaliella Tertiolecta as a Feedstock for Biodiesel. Appl. Energ. 88, 3324–3330. doi:10.1016/j.apenergy.2010.09.013
Vargas, G., Donoso-Bravo, A., Vergara, C., and Ruiz-Filippi, G. (2016). Assessment of Microalgae and Nitrifiers Activity in a Consortium in a Continuous Operation and the Effect of Oxygen Depletion. Electron. J. Biotechnol. 23, 63–68. doi:10.1016/j.ejbt.2016.08.002
Keywords: microalage, modelling, bacteria, wastewater, interactive learning environments
Citation: Sánchez-Zurano A, Guzmán JL, Acién FG and Fernández-Sevilla JM (2021) An Interactive Tool for Simulation of Biological Models Into the Wastewater Treatment With Microalgae. Front. Environ. Sci. 9:721324. doi: 10.3389/fenvs.2021.721324
Received: 06 June 2021; Accepted: 13 July 2021;
Published: 28 July 2021.
Edited by:
Alberto Reis, Laboratório Nacional de Energia e Geologia, PortugalReviewed by:
Mohamed Hassaan, National Institute of Oceanography and Fisheries (NIOF), EgyptSamira Nahim Granados, Environmental and Technological Research, Spain
Copyright © 2021 Sánchez-Zurano, Guzmán, Acién and Fernández-Sevilla. This is an open-access article distributed under the terms of the Creative Commons Attribution License (CC BY). The use, distribution or reproduction in other forums is permitted, provided the original author(s) and the copyright owner(s) are credited and that the original publication in this journal is cited, in accordance with accepted academic practice. No use, distribution or reproduction is permitted which does not comply with these terms.
*Correspondence: A. Sánchez-Zurano, YXN6NTYzQHVhbC5lcw==