Bacterioplankton Richness and Composition in a Seasonal Urban River
- 1Key Lab of Urban Environment and Health, Fujian Key Lab of Watershed Ecology, Institute of Urban Environment, Chinese Academy of Sciences, Xiamen, China
- 2University of Chinese Academy of Sciences, Beijing, China
- 3School of Water Resources and Environment, China University of Geosciences, Beijing, China
- 4School of Earth and Environment, University of Leeds, Leeds, United Kingdom
- 5School of Agriculture and Food, Faculty of Veterinary and Agricultural Sciences, The University of Melbourne, Melbourne, VIC, Australia
Urbanization and seasonality strongly influence the bacterial composition of the soil. However, aquatic environments such as rivers are understudied owing to their high dynamics and therefore rules relating to more static habitats such as lentic or terrestrial environments may be limited. Here, we compared the spatiotemporal patterns of bacterioplankton communities in the Zhangxi river along a gradient of urbanization using 16S ribosomal DNA identification. The alpha and beta diversity of bacterioplankton showed no significant response to watershed urbanization. A significant difference in predicted functional profiles of the bacterioplankton community was also revealed between the wet and dry seasons. The bacterioplankton community assembly was driven by both deterministic and stochastic processes. Stochasticity was one of the most vital processes affecting the bacterioplankton communities in both wet and dry seasons, explaining over 50% variation in the community by the null model analysis. Bacterioplankton co-occurrence patterns in the river changed with the seasons. More notably, the composition of bacterioplankton communities was inconsistent with alternations of the spatial distance offering meaningful implications for interactions between zero-radius operational taxonomic units and the dynamics of the bacterioplankton communities in surface water. In summary, we found clear patterns of seasonal variations in the bacterioplankton community structures.
Introduction
Bacterioplankton plays a key role in global biogeochemical cycles of riverine ecosystems due to their high abundance and turnover rates (Zhu et al., 2017). These microorganisms in surface waters worldwide follow a seasonal succession pattern determined jointly by physicochemical factors like light, climate, and soil properties (Bunse and Pinhassi, 2017). The taxonomic composition of bacterial communities varies widely in rivers at different temporal and spatial scales (Jones et al., 2012; Shade et al., 2013). Two major mechanisms control bacterial communities, that is: i) the dispersion of microorganisms among environments, which determines the bacteria that can be transported and potentially colonize in a given ecosystem, and ii) the environmental selection pressure—that is, adaptive selection—in order to survive the competence stage, species have to adapt to habitat change (Hanson et al., 2012). These major mechanisms are often exposed to seasonal variations or urban stress (Fortunato et al., 2013; Reese et al., 2016; Huber et al., 2020; Liu et al., 2020), highlighting the significance of understanding the response of bacterioplankton diversity and community composition to seasonal variations or urban stress in the watershed microbial ecological systems, as well as the possible link between the bacterioplankton community and heterogeneous habitats. However, all these studies are lacking in systematic research.
The seasonal succession of bacterioplankton species with recurrent patterns is also an essential element influencing bacterioplankton (Bunse and Pinhassi, 2017). Distribution of archaeal and bacterial communities were closely related to the size fraction, habitat, and seasonality (wet and dry seasons) in a subtropical river bay system (Wang et al., 2020). To date, studies using “omics” were mainly focused on marine bacterioplankton communities, and studies on bacterioplankton in freshwater ecosystems are still lacking (Rastogi et al., 2019). On the other hand, the urban stream syndrome is affected primarily by the impervious surface area and urban land use system (Walsh et al., 2005). Increased impervious surface areas can lead to a series of ecological problems, such as water export, peak discharges, and sediment loads, ultimately leading to the loss of microbial biodiversity and ecological function (Li et al., 2020). Since microbes play an essential role in the biogeochemical cycling of trace elements and are closely related to human health, there is a growing concern about the conservation of microbial biodiversity (Reese et al., 2016; Xiao et al., 2020). The core of the microbial communities as a dominant marine holobiont can be modified in response to coastal urbanization (Marzinelli et al., 2018). However, the influence of urbanization on levels of bacteria in rivers is consistently unknown. Hence, looking into the variations in river microbial communities along the gradients consisting of different stages of urban development will provide new insights into anthropogenic impacts on watershed ecosystems.
The mechanisms of microbial community assembly across environmental gradients (e.g., seasonal and urbanization) is a core part of microbial ecology (Hanson et al., 2012; Zhou and Ning, 2017). It is widely accepted that both determinism (e.g., homogeneous and heterogeneous selection) and stochasticity (homogenizing dispersal, dispersal limitation, and undominated processes) contribute to the assembly of the microbial communities (Nemergut et al., 2013; Liu et al., 2020). However, there is still a debate due to the inherent complexity of the microbial community assembly (Zhou and Ning, 2017). It has been shown that the assembly of the bacterioplankton communities was determined by stochasticity (Huber et al., 2020; Wang et al., 2020), while other studies revealed inconsistent results showing it was determined by determinism (Isabwe et al., 2018; Chen et al., 2019). These inconsistent findings underscore the necessity to conduct a comprehensive estimate of the relative contribution of determinism and stochasticity in shaping bacterioplankton communities along various gradients of the environment. Moreover, riverine mobility may also affect the relative contributions of neutral and niche processes to community assembly (Zhou et al., 2014).
In this study, we surveyed the bacterioplankton communities in the surface water of the Zhangxi river, covering an urbanized gradient spanning wet and dry seasons. We adopted the high throughput sequencing of the 16S rRNA gene to analyze the bacterioplankton community composition in a watershed in two sorts of urbanization areas. Additionally, we evaluated the ecological processes dominating the bacterioplankton community assembly semiquantitatively and investigated whether the seasonal variations or urban stress mediate the relationship between their co-occurrence patterns and variations in bacterioplankton community compositions. Overall, this study aimed to 1) quantify the alpha diversity of the bacterioplankton communities across the seasons (wet vs. dry seasons) and the changes along two sorts of urbanization areas, as determined by the percentage of impervious cover; 2) recognize the spatial and environmental factors that explain the changes in bacterioplankton community compositions; 3) quantify the beta diversity, zero-radius operational taxonomic unit (ZOTU), and their co-occurrence patterns across the seasons and two sorts of urbanization areas; and 4) analyze the ecological process of the community assembly. We assume that 1) no matter the urbanization or seasonality, these have caused great influence on the bacterioplankton communities in the Zhangxi river, and 2) the ecological process of the bacterioplankton community assembly is innervated by both determinism and stochasticity, but the proportion was significantly affected by seasonality.
Materials and Methods
Study Area and Sample Collection
The bacterioplankton samples were obtained from the Zhangxi river in Ningbo City, Zhejiang Province, eastern China (Figure 1). There were intense hydraulic exchange and disturbance in the basin as a result of the area’s location in the zone of subtropical monsoon climate, with obvious wet and dry seasons. At the same time, the sampling area is divided into sparsely urbanized area (T1–T5) and densely urbanized area (T6–T9) (Hahs and McDonnell, 2006) (Figure 1; Table 1). Three parallel water samples (0.5-m depth) were collected randomly in each sampling points, and a total of 54 samples were taken [2 (wet and dry seasons in January and July 2016) × 9 (sampling points) × 3 (repetitions), resulting in 54 samples]. Sample collection in each occasion took 2 days to complete. The location of the collection site was recorded using the Handheld Global Positioning System (GPS) (Monterra, GARMIN). On-site fast detection of salinity, temperature, pH, and dissolved oxygen was conducted by the portable multiparameter water quality analyzer (YSI EXO1, United States). The water samples were stored at 4°C in an incubator and taken to the laboratory where pretreatment was conducted immediately. Debris, meroplankton, and microplankton were large enough to be filtered by 200-μm-pore-size sieves, and then a quantity (∼500 ml) of each water sample was transferred onto the membrane in the apparatus and filter. The surface of the mixed cellulose ester microporous membrane (0.22 μm pore size, WX-BD, China) concentrated a large number of microorganisms. All membranes were stored at −80°C until DNA extraction. Physicochemical analysis (e.g., total organic carbon, total phosphorus (TP), chlorophyll-a, nitrate (NO3−), nitrite (NO2−), total nitrogen, and sulfate ion (SO42−)) were conducted as described in our earlier research (Tang et al., 2019).
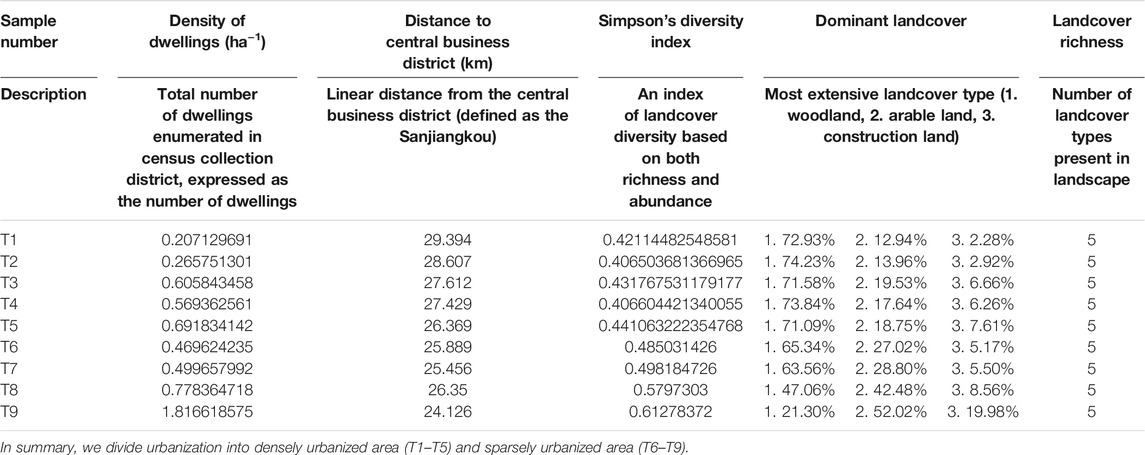
TABLE 1. Description of the six measures of urbanization used to characterize the 2-km radius of the sample site.
DNA Extraction, PCR Amplification, and 16S rRNA Amplicon
DNA of bacterioplankton was extracted directly from the mixed cellulose ester microporous membrane utilizing a FastDNA Spin Kit and in strict accordance with the “FastDNA Spin Kit” operating instructions. PCR amplicon libraries were established for MiSeq sequencing. Briefly, the V4 region of the 16S rRNA gene was amplified with region-specific primers (Caporaso et al., 2012) (515F (5′-GTGCCAGCMGCCGCGGTAA-3′) and 806R (5′-GGACTACHVGGGTWTCTAAT-3′)). Each sample was amplified in triplicate, PCR contained 10 μl genomic DNA (1 ng/μl), 15 μl Phusion Master Mix (2×), and 3.0 μl each of the reverse and forward primers (2 μM). Reactions were held at 98°C for 1 min to pre-degeneration of the genomic DNA, followed by 30 cycles of denaturation at 98°C for 10 s, annealing at 50°C for 30 s, and elongation at 72°C for 30 s and a final extension of 72°C for 5 min.
Sequence Denoising and Diversity Analysis
Raw sequences were modified with VSEARCH (v2.15.0, https://github.com/torognes/vsearch) to remove sequences of primers, barcodes, shorter length (<100 bp), and minimal quality (<20) and merge the high-quality double-end sequences. ZOTUs were deionized in UNOISE3 (Edgar, 2017) at a 100% similarity level. Taxonomical classification based on ZOTUs was performed utilizing the VSEARCH and Silva (Silva 138, https://github.com/jameslz/protocols/blob/master/How-to-generate-USEARCH-compatible-SILVA138%20fasta) databases. Alpha-diversity for each sample was profiled through measurement for the Chao 1 and Shannon_e indices, box plots were described to compare the alpha diversity level of bacterioplankton ZOTUs, and nonparametric linear regression with permutation testing was performed with the lmPerm() package. FAPROTAX was appropriate for function annotation prediction of the biogeochemical cycles with the environmental sample, especially the elements cycling in each ecosystem system, such as carbon, hydrogen, nitrogen, and phosphorus. It is therefore in potential microbial functional profiles that we adhered to methods for the FAPROTAX database version 1.2.3 (http://www.loucalab.com/archive/FAPROTAX/lib/php/index.php?section=Home) (Louca et al., 2016). All raw sequences were deposited in a public database (National Center for Biotechnology Information Sequence Read Archive). The session specification is numbered PRJNA663232.
Null Model
We used the null model evaluation to quantify the relative contributions of different assembly processes of the bacterioplankton, i.e., heterogeneous selection, homogeneous selection, homogenizing dispersal, undominated processes, and dispersal limitation (Stegen et al., 2012). More precise information is provided in the Ecological process analysis section in the Supplemental Material.
Variation Partitioning Analysis and Distance Decay Analysis
Variation partitioning analysis (VPA) was used to quantify the relative role of spatial and environmental factors and their comprehensive effect. Environmental factors that were important to explain community changes of the redundancy analysis (RDA) were applied to conduct further analysis. By using R statistical software, via the principal coordinates of neighbor matrices (PCNM) analysis, a set of spatial factors were automatically generated. The spatial factors were selected via the same method as used for environmental factors for the VPA. Pairwise geographic distances between samples drew the conjugated Bray–Curtis dissimilarities using the “ggplot2” package in the R statistical software and were computed from the longitude and latitude coordinates using the geosphere library. The Spearman’s rank correlation between geographic distances and Bray–Curtis dissimilarities was estimated.
Network Analysis
The bacterioplankton co-occurrence network was established based on the ZOTUs table, representing bacterioplankton co-occurrence relationships. To minimize complexity, only ZOTUs with a proportion less than 0.01% or 1% across all samples, and existing in more than 20% of the samples, were employed to construct the co-occurrence network. Pairwise Spearman’s correlations between ZOTUs were computed, with a p-value < 0.05 and a correlation coefficient > |0.6| being deemed as an intimate connection. Network-level (betweenness centralization, clustering coefficient, mean node degree, average path length, degree centralization, diameter, density, and modularity) and node-level (closeness centrality, betweenness centrality, transitivity, and degree) topological characteristics of a network were calculated. The network diagram was visualized in Gephi (v. 0.9.2, https://gephi.org).
Results
Bacterioplankton Community Composition, Diversity, and Abundance
A total of 2,626,733 filtered sequences were acquired after rarefication and quality control and were deionized into 3,247 ZOTUs at a 100% similarity level. The wet season samples were found to have the highest level of Chao 1 (128.8 on 25 degrees of freedom; Pseudo-F = 0.9292; p = 0.3443) and Shannon_e (0.2579 on 25 degrees of freedom; Pseudo-F = 0.9908; p = 0.3291) indices; however, these were not very significant (Figures 2A,B). The sparsely urbanized area was discovered to have the highest level of Chao 1 and Shannon_e indices regardless of the season, though the differences were not statistically significant (112.5 on 8 degrees of freedom; Pseudo-F = 2.441; p = 0.1392), except for the Shannon_e index from the dry season (0.2316 on 8 degrees of freedom; Pseudo-F = 2.278; p<0.05) (Supplementary Figures S2A, B).
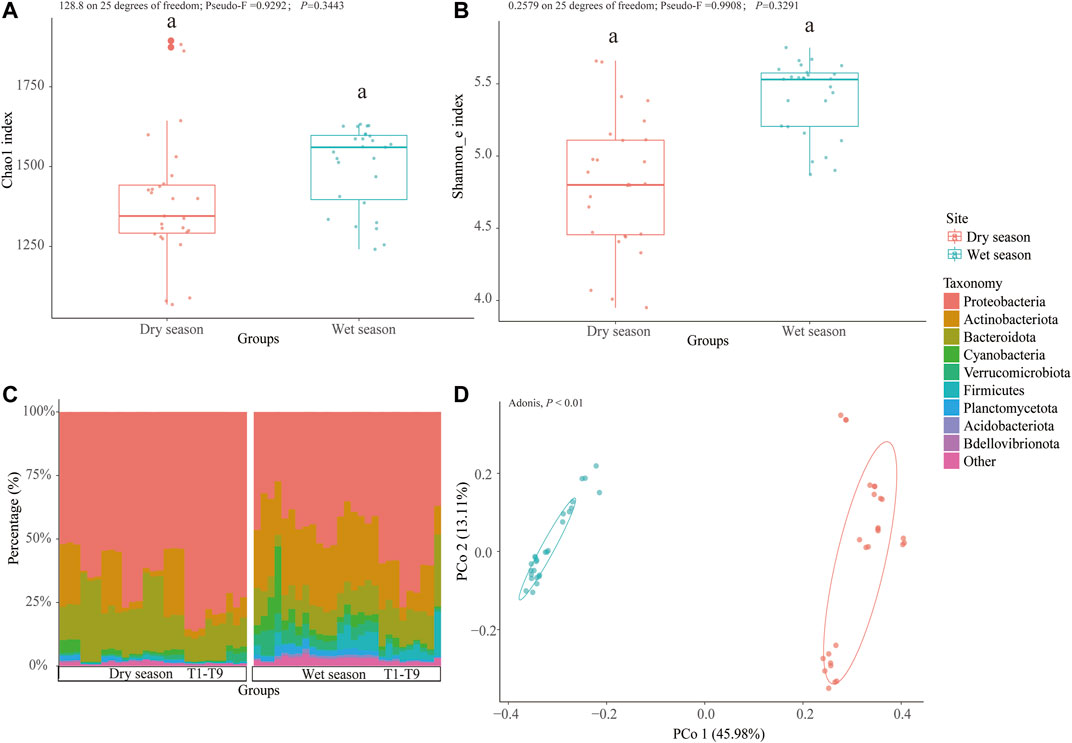
FIGURE 2. Seasonal changes in bacterioplankton diversity and community composition. Chao 1 index (A) and Shannon_e index (B) of the bacterioplankton communities of surface water from the Zhangxi river. Taxa composition at the phylum level among the wet and dry seasons (C). PCoA of Bray–Curtis dissimilarity on community composition in different seasons (D).
The bacterioplankton communities in the wet season were mainly dominated by Proteobacteria (27.2–76.87%), followed by Actinobacteria (5.24–38.60%) and Bacteroidota (4.47–28.20%). However, the dry season bacterioplankton community was dominated by Proteobacteria (51.40–86.33%), followed by Bacteroidota (9.02–35.01%) and Actinobacteria (0.86–26.90%) (Figure 2C). Following the two sorts of urbanization areas, during the wet season, in the sparsely urbanized area samples, the bacterioplankton community was dominated by Proteobacteria (27.27–53.52%), followed by Actinobacteria (21.18–38.60%), Bacteroidota (4.47–14.68%), Verrucomicrobiota (3.19–10.19%), and Firmicutes (0.344–9.35%), and in the densely urbanized area samples, the bacterioplankton community was dominated by Proteobacteria (37.00–76.87%), followed by Bacteroidota (10.30–28.20%), Actinobacteria (5.24–27.50%), Firmicutes (0.32–17.40%), and Verrucomicrobiota (1.23–4.40%). During the dry season, in the sparsely urbanized area samples, the bacterioplankton community was dominated by Proteobacteria (51.40–77.03%), followed by Bacteroidota (13.09–35.01%) and Actinobacteriota (0.86–24.72%), and in the densely urbanized area samples, the bacterioplankton community was dominated by Proteobacteria (53.59–86.33%), followed by Bacteroidota (9.02–16.20%) and Actinobacteria (2.87–26.90%) (Supplementary Figure S2C).
Significant Differences in Bacterioplankton Communities
The Principal Coordinates Analysis (PCoA) based on the Bray–Curtis distance revealed that the majority of differences between the dry and wet season subcommunities were attributed to seasonality (Adonis, p < 0.01). The results of the constrained principal coordinate analysis showed that the main microbial groups were trying to change the degree of urbanization (Figure 2D and Supplementary Figure S2D). The cladogram drew a map with ZOTU of the linear discriminant analysis (LDA) values more than 2.5 for clarity (Figure 3). There were 134 ZOTUs found to be significantly different between the wet season and dry season groups (Supplementary Figure S4). There were five groups of ZOTUs enriched in the dry season, including Cyanobacteria (from phylum to order), Bacteroidota (from phylum to genus), Proteobacteria (from phylum to genus), and Planctomycetota (from phylum to order) (Figure 3). Thereinto, five good lineages had an LDA value of 4.8 or higher, including Gammaproteobacteria, Proteobacteria, Burkholderiales, Methylotenera, and Methylophilaceae (Supplementary Figure S4). There were 15 groups of ZOTUs enriched in the wet season, including Gammaproteobacteria (from phylum to order), Actinobacteriota (from phylum to genus), Verrucomicrobiota (from phylum to genus), Planctomycetota (from phylum to order), Bacteroidota (from phylum to family), etc. (Figure 3). Within these, five good lineages had an LDA value of 4.8 or higher, including Actinobacteriota, Sporichthyaceae, Frankiales, hgcI_clade, and Actinobacteria (Supplementary Figure S4).
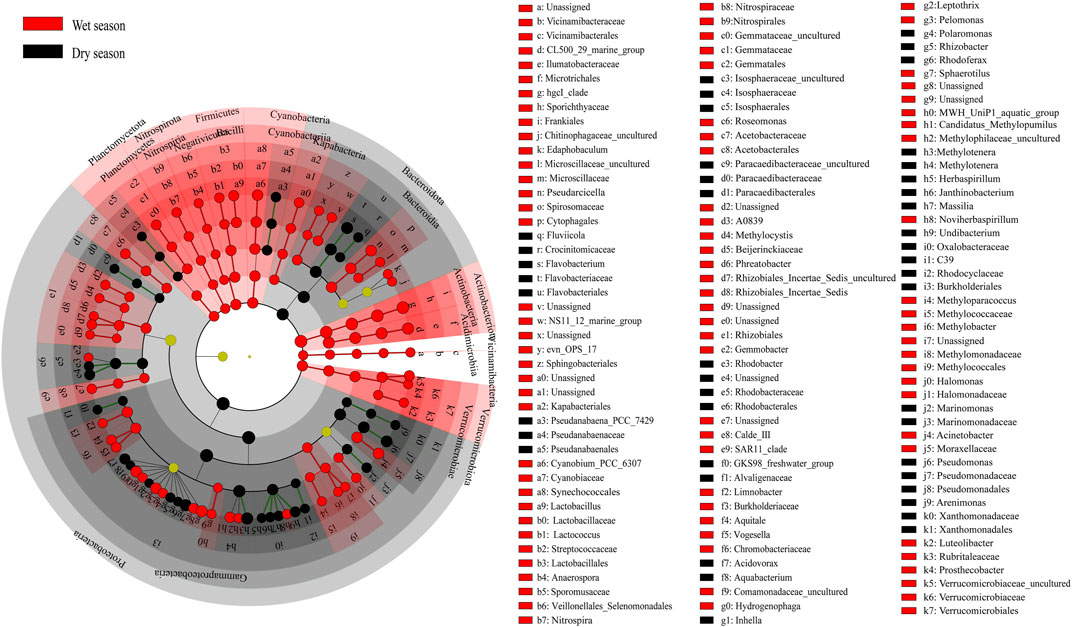
FIGURE 3. LEfSe (linear discriminant analysis effect size) analyses revealed bacteria taxa distribution differences between the wet and dry seasons. The taxa that have the LDA score >3.0 were considered as the biomarker.
Regarding the influence of urbanization on the microbial community, during the wet season, there were 109 ZOTUs found to be significantly different between the densely urbanized areas and sparsely urbanized areas. There were 10 groups of ZOTUs enriched in the sparsely urbanized area, namely, Alphaproteobacteria (from phylum to family), Gammaproteobacteria (from phylum to order), Planctomycetota (from phylum to genus), Actinobacteriota (from phylum to genus), Verrucomicrobiota (from phylum to genus), etc., while only three groups of ZOTUs enriched the densely urbanized area, namely, Gammaproteobacteria (from phylum to genus), Bacteroidota (from phylum to genus), and Rhodobacterales (from phylum to order) (Supplementary Figure S3A). During the dry season, there were 25 ZOTUs found to be significantly different among the densely urbanized area and sparsely urbanized area groups. There were five groups of ZOTUs found to be enriched in the sparsely urbanized area, namely, Alphaproteobacteria (from phylum to family), Gemmatales (from order to genus), Bacteroidota (from phylum to family), Bacteroidota (from phylum to order), and Acidobacteriota (from phylum to order). There was only one group of ZOTU enriched in the densely urbanized area, namely, Gammaproteobacteria (from phylum to class) (Supplementary Figure S3B).
Ecological Processes Governing Community Structure
The null model was used to quantify the relative contributions of each microbial ecological process in the assembly of diverse groups. In both seasons, stochastic processes played an important role in the community structuring. However, in the wet season, undominated processes accounted for a higher turnover, while in the dry season, microbial communities, with a dispersal limitation reaching 52.42% (Figure 4). In the developmental process of urbanization, during the wet season, undominated processes alone dominated 43.81% of the turnover in the community taxonomic composition of densely urbanized areas, while in the sparsely urbanized area, dominated 23.61% of the turnover (Supplementary Figure S7).
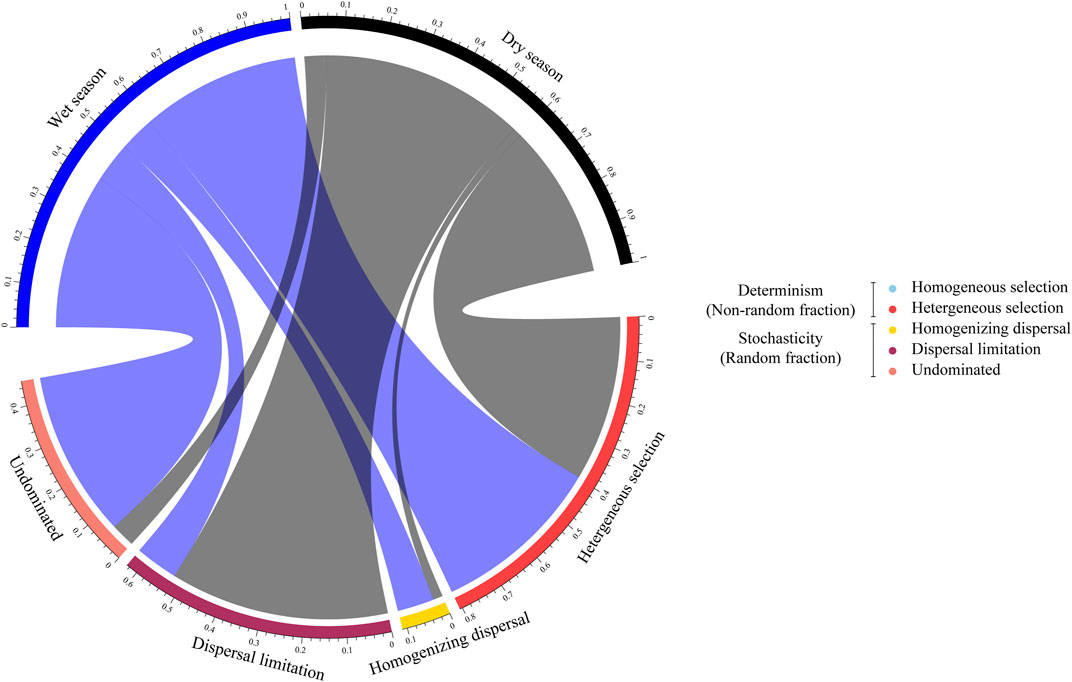
FIGURE 4. Ecological assemblies of bacterioplankton communities. The relative significance of ecological processes dominating the assembly of the bacterioplankton communities in the wet and dry seasons.
We discovered that seasonality and urbanization affect the bacterioplankton ecosystem structure and functionality (Figures 5C,D). For each season, >60% of the community changes could be explained, which indicates relatively simple processes of bacterioplankton community assembly in the river. RDA showed that the significant factors were one environmental factor (temperature) and four spatial factors (PCNM 1, 3, 6, and 7) in the wet season, and four spatial factors (PCNM 1–3 and 7) and three environmental factors (pH, TP, and temperature) in the dry season (Figures 5A,B). The RDA in the wet season shows a positive correlation between the bacterioplankton community (sparsely urbanized area, T1–T5) and temperature, and a negative correlation with spatial factors (PCNM1, PCNM3, PCNM6, and PCNM7), but the bacterioplankton community (densely urbanized area, T6–T9) was the opposite (Figure 5A). However, the bacterioplankton communities were intricately correlated with spatial factors (PCNM1, PCNM2, PCNM3, and PCNM7) and environmental factors (pH, TP, and temperature) in all sample plots (Figure 5B).
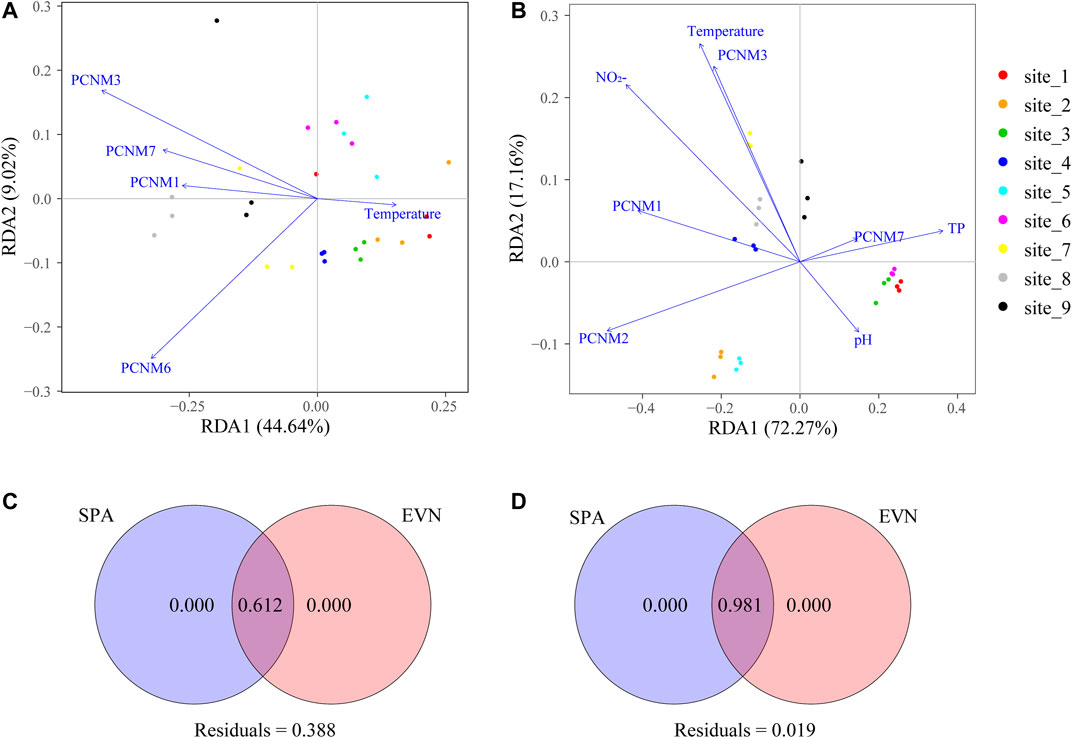
FIGURE 5. Effect of spatial and environmental factors on bacterioplankton communities by RDA and VPA. RDA was performed to show significant environmental and spatial factors in dominating the assembly of the wet (A) and dry (B) season bacterioplankton communities. From a geographic distance matrix, function PCNM computed the PCNM based on distances, in view of spatial eigenfunction analysis. VPA was used to quantify the contribution of environmental and spatial factors to community changes in the wet (C) and dry (D) seasons.
Bacterioplankton Co-Occurrence Networks
The groups of ZOTUs with the abundant ZOTUs (>0.1%, relative abundance (RA) and major ZOTUs (≥1%, RA), we have the following two groups performed network analysis. Figures 6A,B show a correlation-based network, consisting of 567 edges (correlations) and 66 nodes (ZOTUs, RA > 1%) from the wet season bacterioplankton communities, and 474 edges and 77 nodes from the dry season bacterioplankton communities. ZOTUs (dominant bacterial community, RA > 1%) shared between the dry and wet seasons are represented utilizing a bipartite network analysis, and there were only six shared ZOTUs in the bipartite network (Figure 6C).
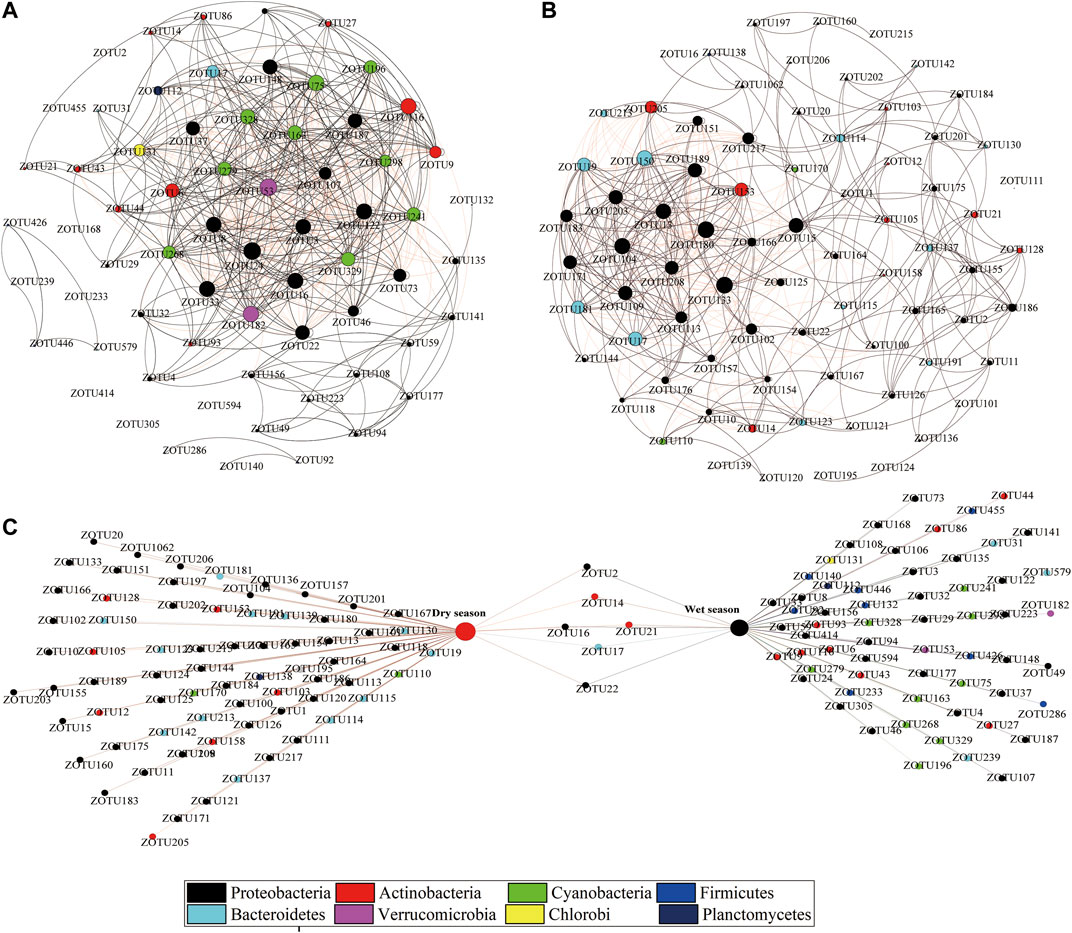
FIGURE 6. Co-occurrence networks of the bacterioplankton communities based on pairwise Spearman’s correlations between ZOTUs. Each connection was associated with a significant level of p < 0.05 and a correlation coefficient >|0.6|. The number of connections varies with the size of each node. The figure shows the network of the wet (A) and dry (B) season samples with ZOTUs colored by taxonomy. The following figure shows the bipartite association network of bacterioplankton taxa with different seasons (C).
To further discern the bacterioplankton co-occurrence patterns of the river bacterioplankton communities based on the previous network analysis, a ZOTU (RA >0.1%) ecological network interaction was constructed. The significant (p < 0.05) and strong (correlation coefficient R > |0.6|) co-occurrence network was constructed consisting of 3,970 positive correlations and 779 negative correlations in the wet season samples (Supplementary Figure S9A), and 2,981 strong positive correlations and 683 negative correlations in the dry season samples, respectively (Supplementary Figure S9B). The network density (the average number of edges per node) was lower in the wet season (17.02) than in the dry season (15.59), suggesting stronger co-occurrence relationships during the wet season. Modularity analysis showed major modules (subcells with highly interconnected nodes) in each season network. In the wet season, many of these modules were made up of a group of ZOTUs with phylum with near homology relationship on evolution (Supplementary Figure S9C). For example, modules 4 and 1 in the wet season network were dominated by Bacteroidetes ZOTUs, Firmicutes ZOTUs, and Chloroflexi ZOTUs, respectively. In contrast, the interaction systems of bacterioplankton communities were more closely linked during the dry season, possibly due to the lack of nutrition for microbial cells. Our findings showed that the taxonomic classification of ZOTUs was critical for the network modular structure (Supplementary Figure S9D).
Functional Prediction
In this study, we utilized FAPROTAX annotation rules to automatically compare each taxon annotated ZOTU. In the bacterial data set, among the 91 functional groups in the FAPROTAX database, 72 groups (78.3%) were associated with at least one of the samples, and 39.17% of the ZOTUs were assigned to at least one group in the FAPROTAX database (Supplementary Table S4). More surprisingly, a few ZOTUs could not be matched with any functional groups. Hence, the ZOTU turnovers inside a functional group were only applicable in the subset of ZOTUs with the specified functions (although this limitation played no role in conclusions of this research). Some ZOTUs were mapped to more than one functional group. For instance, ZOTU_2224 (belonging to the species of Methylosinus) was believed to be involved in methanotrophy, methylotrophy, hydrocarbon degradation, and chemoheterotrophy. Forty functional groups, such as aerobic chemoheterotrophy, chemoheterotrophy, methylotrophy, fermentation, etc., were covered in all water samples. Thirty-eight out of 72 bacterial functional groups, influenced significantly by seasonal cyanobacteria, oxygenic photoautotrophy, photoautotrophy, manganese oxidation, etc., were more abundant, while chemoheterotrophy, methanol oxidation, hydrocarbon degradation, methanotrophy, photoautotrophy, oxygenic photoautotrophs, etc., were less abundant in the wet season (Figure 7A). Nonmetric Multidimensional Scaling (NMDS) and PCoA revealed significant deviation of the RA of functional profiles between the two seasons (Adonis, p < 0.01) (Figures 7B,C). According to the result of cluster analysis, the dissimilarity of functional profiles was further verified using ADONIS, ANOSIM, and MRPP (Supplementary Table S5B).
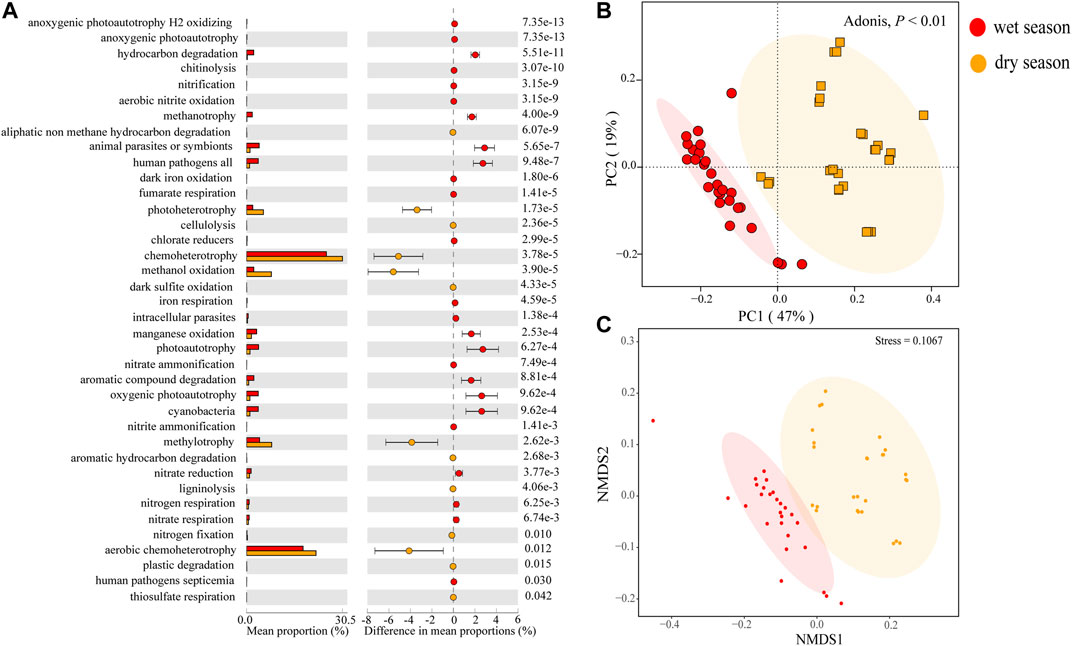
FIGURE 7. Box plots and extended error bar plots (A) of abundant differentially functional groups in surface water samples in the watershed. The PCoA (B) and NMDS (C) functional groups of diverse samples from the wet and dry seasons.
Discussion
Urban Stress and Seasonality Shaping Bacterioplankton Community Structure
In a river microecosystem, the matter and energy of the surroundings input to the river change with the seasons, and therefore shift the bacterioplankton communities accordingly. Our results demonstrated that the bacterioplankton communities largely varied between the dry and wet seasons, exhibiting distinct changes in the diversity and composition. Firstly, the bacterioplankton communities had significantly lower diversity in the dry than in the wet season, which is consistent with previous researches (Fortunato et al., 2013; Chen et al., 2019; Liu et al., 2020). Secondly, there was a succession between the wet and dry seasons in bacterioplankton community composition. This is consistent with the findings that the wet and dry season bacterioplankton communities had very dissimilar compositions (Fortunato et al., 2013; Read et al., 2015). We proposed that the observed pattern in bacterioplankton community composition could be related to i) water residence time (Mcgonigle et al., 2019) (the periodical oscillations of the drought and flood features, and the speed of streams in the wet season is faster than in the dry season); ii) changing resource availability in the wet season (Lynch et al., 2019) (with an increase of rainwater runoff, the runoff coefficient increases); iii) different strong recycling chemicals and minerals between the river and riparian (Bing et al., 2017).
Our data showed that rapid urbanization released the pressure and led to shifts in the bacterioplankton community structure in the watershed, which was also supported by previous studies (Wang et al., 2011; Hosen et al., 2017; Simonin et al., 2019). Indeed, rapid watershed urbanization could transform the physical and chemical conditions in streams, such as pollutant emission, overnourishment, high temperature, changes in channel flow, and river configuration and process, and therefore induce shifts of the bacterioplankton communities (Walsh et al., 2005). Moreover, we found that spatial factors as foremost factors with environmental variables, including temperature, pH, NO2−, and TP, were driving the shifts in bacterioplankton composition (PCNM1-3, 6, and 7) in the watershed. The bacterioplankton community structure shifted with the changes in the land use type but with no significant impacts on alpha diversity assessed through relative diversity indices (Chao 1 index, Shannon_e index), and the findings were not entirely consistent with previous studies (Lear et al., 2011). Artificial interference resulting in a decrease of biological diversity is a widespread phenomenon, especially in densely populated urban areas. However, for the sustainability of the microecosystem, we appeal to maintain the balance and bacterial diversity in riverine ecosystems under global urbanization since i) there were giant inflows of novel bacterial taxa from water supplies and septic systems (sewage in particular), and ii) rainwater may infuse afflux to make up for lost sensitivity taxa of urban conditions (Hosen et al., 2017).
Strong Seasonal Heterogeneity With Varying Contributions From Various Stocks
Remarkable urbanized differences in bacterioplankton community compositions were found in many of the seasonal samples studied. The effects of seasonality on bacterioplankton communities far outweighed the effects of urbanization in this study. The finding has also been supported by previously published studies which found that microeukaryotic (Chen et al., 2019) and bacterioplankton (Pearman et al., 2017; Wu et al., 2017) communities were more variable with the season than with urbanization change. By comparison, in benthic microbial communities, biogeography variations have been found to have an even stronger influence than seasonal variations (Liu et al., 2020). Such a huge gap in the pattern of microbial community dynamics between benthic and pelagic niches indicates lesser temporal habitat stability in the water column than in the sediment (Gilbert et al., 2011). Besides, benthic microbes would have sufficient time to sense and adapt to their environments. For bacterioplankton, with any change, there will be uncertainty risk.
The geographic pattern observed in the bacterioplankton community reflected a clear seasonal variation. There was a significant negative correlation between the Bray–Curtis similarity and geographical distance of bacterioplankton communities in the wet season (p < 0.001, Supplementary Figure S10), while the same finding was not observed in the dry season. However, distance decay regularity was reported to be weaker during the wet season than in the dry season in the Tingjiang river (Chen et al., 2019), which might be attributed to a great variety of Bray–Curtis similarity caused by a flood of sensitive taxa of urban conditions into the river. Inversely, rainfall events are very unusual in the dry season. The decrease of precipitation and surface water and lower river flow might be the principal reasons that result in a higher dispersal limitation of microbes. Distance decay regularity has been found to underlie marine microbial spatial dynamics (Zinger et al., 2014). Nevertheless, bacterioplankton communities may reveal lower attenuation rates with increasing spatial distance compared to benthic bacteria (Zinger et al., 2014). This suggests stronger dispersal abilities for bacterioplankton communities relative to benthic microorganisms.
Stochasticity Plays an Important Part in Bacterioplankton Community Assembly
Plentiful evidence suggest that both deterministic and stochastic processes play a critical role in the turnover of microbial communities along rivers (Zhao et al., 2017; Chen et al., 2019; Kimbrel et al., 2019; Zeng et al., 2019; Xie et al., 2020). Compared with the wet season, slightly enhanced influence of stochasticity on community assembly might be attributed to the device that dry season bacterioplankton communities may have a lower scattered capacity. Coincidentally, leveraging a null model, a slightly greater role of stochasticity than determinism was detected in administering the bacterioplankton community assembly in the Zhangxi river. Furthermore, VPA corroborated this observation by showing that the bacterioplankton community assembly in the Zhangxi river was emphasized by a variety of factors, including spatial factors and environmental factors. Nevertheless, this was not the same as the RDA that revealed a slightly higher influence on spatial factors. Furthermore, heterogeneous selection explained relatively substantial turnover in community compositions for the two seasons. This finding also showed that the variable ambient conditions across time or space (i.e., heterogeneous) at large scales make up for extreme variations in community compositions since heterogeneous selection can increase the revenue of the community structure, leading to communities that are inconsistent in phylogeny, especially in the wet season. But we discovered that community composition was also affected by dispersal limitation, which further determined that the impacts of stochastic and deterministic processes on the assembly of microbial communities are inseparable but act upon each other and exert a synergic impact at large scales (Stegen et al., 2013; Kou et al., 2020).
Drastic environmental changes have an impact on the assembly processes of the ecological community (Chase, 2007; Zhou et al., 2014). Our results indicated that the composition and diversity of bacterioplankton communities were easily discriminated against in the urbanization area in the two seasons. Deterministic and stochastic factors play different roles in different seasons and different urbanized areas. In the wet season, the relative materiality of stochastic processes in dominating the assembly of bacterioplankton communities decreased along two sorts of urbanization areas. In the dry season, however, stochastic processes remained dominant only in densely urbanized areas. The process of receiving nutrition is deemed to decrease niche selection by offering resources and increase stochasticity by enhancing drift (e.g. stochastic processes of extinction, colonization, death, and birth) (Chase, 2010; Zhou et al., 2014). In the wet season, nutritional substances in the rivers mainly come from surface runoffs where human activities play an important role. RDA results also revealed the fact that the beta diversity of bacterioplankton communities was associated with nutritional substance (e.g., NO2− and TP) in the dry season, and therefore, played a key role in the control of community assembly. Apart from community assembly, phosphorus and nitrogen, as the crucial factors (abiotic), observably influenced the composition, diversity, abundance, and species interactions of the microbial community (Zeng et al., 2019). Hence, nutrients, as deterministic factors, deterministically shape the bacterioplankton communities in the urbanized river.
Co-Occur Network: Spatial Dynamics and Unrivalled Seasonality With Community Composition
We probed variations in co-occurrences between taxa by reestablishing network modules and discerned the synchrony of bacteria using K-means–based cluster analysis. The seasonal changes of network-level topological characteristics were researched here to explore whether transformations in bacterioplankton community compositions could identify their co-occurrence patterns. Interactions can include mutualism, parasitism, or competition for resources such as co-metabolic reaction (Parter et al., 2007; Faust et al., 2012). The results showed an obvious seasonal pattern of connection between bacterioplankton taxa; the bacterioplankton ZOTUs were more closely and inherently connected in the wet season than in the dry season. Perhaps this is due to higher wet season spatial connectivity, which was mainly attributable to the strong river surface runoff and discharges. These phenomena may make distinct sampling regions geographically more parted, thus, leading to a whole more dispersive co-occurrence pattern in the dry season. Network module analysis offered an overview of the variation of mutually exclusive and coexistence link between taxa. We identified modules in the network of the wet and dry seasons using MCODE and traced the top eight modules ranked by the average degrees. Previous studies looking at the effect of interference in microbial co-occurrence networks observed an increase in modularity with the increase of disturbance intensity, so far as interference was a short run in nature (Parter et al., 2007). In this study, the modularity level of the wet season network was larger than that of the dry season network, and that the number of keystone-like taxa was quickly disappearing in the dry season network and could also form new keystone-like taxa at the same time. It showed that the abundance of most taxa follows a particular variation pattern rather than an irregular variation of this river with the seasons.
On the other hand, although there was an obvious correlativity between bacterioplankton community composition and geographic distance, no consistent variation in co-occurrence patterns and spatial change was observed (Supplementary Figure S10). The results provide proof for this view that the dynamic change of microbial community co-occurrence patterns and compositions may be out of correlation and sync at times. It is going to help researchers to better understand how microbial ecology evolved with the seasons. Caution is necessary for the evaluated variations in co-occurrence patterns that originated from a method based on network analysis which did not reflect the real inter ZOTU correlations. Hence, extra efforts are required to recognize specific taxa interactions and connect them with further ecological functions and community compositions.
Adaptive Shifts of Bacterioplankton Functional Diversity to Seasonal Variation
Researchers have taken a great interest in the relationship between functional diversity and community structure (Zeglin, 2015; Louca et al., 2016; Maynard et al., 2017; Yang et al., 2019). However, it remains unclear whether the community functions vary according to community composition. Some research has indicated that community composition could result in the stronger variety of community function than functional redundancy, while others suggest explaining changes in community function rely on community composition (Staley et al., 2014). Above all, most of the nitrogen metabolism-related groups (e.g., nitrogen respiration and nitrate respiration), organism degradation-related groups (e.g., hydrocarbon degradation and aromatic compound degradation), and pathogen-related groups (e.g., intracellular parasites, human pathogens, and animal parasites or symbionts) showed a higher RA in the wet season, and most of the bacterial metabolism-related groups (e.g., photoheterotrophy, chemoheterotrophy, methanol oxidation, methylotrophy, and aerobic chemoheterotrophy) showed higher RA in the dry season (Figure 7A). This phenomenon was concordant with other researches in seasonal rivers or streams where linkages between functions and community composition were detected (Luo et al., 2020; Yang et al., 2020). Therefore, the bacterioplankton communities can not only functionally adapt to nutrient pollution but also have the potential to mitigate the negative consequences associated with environmental changes (Jeffries et al., 2015). In the wet season, large volumes of rainwater could carry pathogens, contaminants, and nutrients to the surface water, whereas the pollution was less in the dry season. Since microorganisms are of great significance in biogeochemical cycles, the connection between water quality and dynamics of bacterioplankton communities is worthy to be studied further (Woodward et al., 2012). Therefore, future research should be focused on seasonal and annual monitoring of water quality and microbial diversity.
Conclusion
Based on the marker gene amplicon sequencing, we comprehensively profiled the composition, function, and spatial distribution of the bacterioplankton communities in the Zhangxi river, China. The shift in bacterioplankton community composition was found to be strongly related to seasonal variations (wet vs. dry season). However, when excluding the seasonal impact by comparing the samples from one typical season, we found the shift of bacterioplankton community composition along two sorts of urbanization areas. Our research revealed clear spatiotemporal patterns in bacterioplankton community composition, their co-occurrence relationship, urbanized distribution, and the underlying mechanisms in surface water samples from the Zhangxi river. We also found that the distance decay pattern in the bacterioplankton communities occurred only during the wet season. Bacterioplankton community function diversity in the seasonal variation was notable. Moreover, we provide field evidence of stochasticity as playing a far more important role in structuring the distance decay pattern than determinism. Above all, quantitative evaluation on ecological processes showed that stochasticity (e.g., homogenizing dispersal, dispersal limitation, and undominated processes) exerted a key role in the community assembly of bacterioplankton, which was influenced by seasonal changes. Bacterioplankton co-occurrence relationships in this river changed with seasons. Bacterioplankton community function diversity in the seasonal variation was remarkable. And more notably, their turnover with the spatial distance was inconsistent with that of community compositions, providing novel insights for the inter taxa interactions and the dynamics of bacterioplankton communities in surface water.
Data Availability Statement
The data sets presented in this study can be found in online repositories. The names of the repository/repositories and accession number(s) can be found below: https://www.ncbi.nlm.nih.gov/, PRJNA663232.
Author Contributions
Conception and design of study: ZX, YZ, and GL; acquisition of data: ZX, PB, and JT; analysis and/or interpretation of data: ZX, YX, TR, and GL. Drafting the manuscript: ZX, YZ, GL, KX, and QC; revising the manuscript critically for important intellectual content: ZX, YZ, GL, KX, QC, EFZ, and YX. Approval of the version of the manuscript to be published: ZX, GL, YZ, KX, QC, PB, JT, TR, EFZ, and YX.
Funding
This work was supported financially by the Ministry of Science and Technology of China (MSTC) with National Key Research and Development Program (2017YFE0119000), National Natural Science Foundation of China (NSFC) with Young Scientists Programs (31300397), and Xiamen Municipal Bureau of Science and Technology of China (3502Z20193075). EFZ is supported by the Chinese Academy of Sciences President's International Fellowship for Postdoctoral Researchers (2020PC0002). Any opinions, findings, and conclusions or recommendations expressed in this work are attributable to the authors and do not necessarily reflect the views of MSTC and NSFC.
Conflict of Interest
The authors declare that the research was conducted in the absence of any commercial or financial relationships that could be construed as a potential conflict of interest.
Publisher’s Note
All claims expressed in this article are solely those of the authors and do not necessarily represent those of their affiliated organizations, or those of the publisher, the editors, and the reviewers. Any product that may be evaluated in this article, or claim that may be made by its manufacturer, is not guaranteed or endorsed by the publisher.
Supplementary Material
The Supplementary Material for this article can be found online at: https://www.frontiersin.org/articles/10.3389/fenvs.2021.731227/full#supplementary-material
References
Bunse, C., and Pinhassi, J. (2017). Marine Bacterioplankton Seasonal Succession Dynamics. Trends Microbiol. 25 (6), 494–505. doi:10.1016/j.tim.2016.12.013
Caporaso, J. G., Lauber, C. L., Walters, W. A., Berg-Lyons, D., Huntley, J., Fierer, N., et al. (2012). Ultra-high-throughput Microbial Community Analysis on the Illumina HiSeq and MiSeq Platforms. ISME J. 6 (8), 1621–1624. doi:10.1038/ismej.2012.8
Chase, J. M. (2007). Drought Mediates the Importance of Stochastic Community Assembly. Proc. Natl. Acad. Sci. U S A. 104 (44), 17430–17434. doi:10.1073/pnas.0704350104
Chase, J. M. (2010). Stochastic Community Assembly Causes Higher Biodiversity in More Productive Environments. Science 328 (5984), 1388–1391. doi:10.1126/science.1187820
Chen, W., Ren, K., Isabwe, A., Chen, H., Liu, M., and Yang, J. (2019). Stochastic Processes Shape Microeukaryotic Community Assembly in a Subtropical River across Wet and Dry Seasons. Microbiome 7 (1), 138. doi:10.1186/s40168-019-0749-8
Edgar, R. C. (2017). Updating the 97% Identity Threshold for 16S Ribosomal RNA OTUs. bioRxiv, 192211. doi:10.1101/192211
Faust, K., Sathirapongsasuti, J. F., Izard, J., Segata, N., Gevers, D., Raes, J., et al. (2012). Microbial Co-occurrence Relationships in the Human Microbiome. Plos Comput. Biol. 8 (7), e1002606. doi:10.1371/journal.pcbi.1002606
Fortunato, C. S., Eiler, A., Herfort, L., Needoba, J. A., Peterson, T. D., and Crump, B. C. (2013). Determining Indicator Taxa across Spatial and Seasonal Gradients in the Columbia River Coastal Margin. ISME J. 7 (10), 1899–1911. doi:10.1038/ismej.2013.79
Zinger, L., Amaral-Zettler, L. A., Fuhrman, J. A., Horner-Devine, M. C., Huse, S. M., Welch, D. B. M., et al. (2011). Global Patterns of Bacterial Beta-Diversity in Seafloor and Seawater Ecosystems. PLoS ONE 6 (9), e24570. doi:10.1371/journal.pone.0024570
Hahs, A. K., and McDonnell, M. J. (2006). Selecting Independent Measures to Quantify Melbourne's Urban-Rural Gradient. Landscape Urban Plann. 78 (4), 435–448. doi:10.1016/j.landurbplan.2005.12.005
Hanson, C. A., Fuhrman, J. A., Horner-Devine, M. C., and Martiny, J. B. H. (2012). Beyond Biogeographic Patterns: Processes Shaping the Microbial Landscape. Nat. Rev. Microbiol. 10 (7), 497–506. doi:10.1038/nrmicro2795
Hosen, J. D., Febria, C. M., Crump, B. C., and Palmer, M. A. (2017). Watershed Urbanization Linked to Differences in Stream Bacterial Community Composition. Front. Microbiol. 8, 1452. doi:10.3389/fmicb.2017.01452
Huber, P., Metz, S., Unrein, F., Mayora, G., Sarmento, H., and Devercelli, M. (2020). Environmental Heterogeneity Determines the Ecological Processes that Govern Bacterial Metacommunity Assembly in a Floodplain River System. ISME J. 14, 2951–2966. doi:10.1038/s41396-020-0723-2
Isabwe, A., Yang, J. R., Wang, Y., Liu, L., Chen, H., and Yang, J. (2018). Community Assembly Processes Underlying Phytoplankton and Bacterioplankton across a Hydrologic Change in a Human-Impacted River. Sci. Total Environ. 630, 658–667. doi:10.1016/j.scitotenv.2018.02.210
Jeffries, T. C., Schmitz Fontes, M. L., Harrison, D. P., Van-Dongen-Vogels, V., Eyre, B. D., Ralph, P. J., et al. (2015). Bacterioplankton Dynamics within a Large Anthropogenically Impacted Urban Estuary. Front. Microbiol. 6, 1438. doi:10.3389/fmicb.2015.01438
Jones, S. E., Cadkin, T. A., Newton, R. J., and McMahon, K. D. (2012). Spatial and Temporal Scales of Aquatic Bacterial Beta Diversity. Front. Microbio. 3, 318. doi:10.3389/fmicb.2012.00318
Kimbrel, J. A., Samo, T. J., Ward, C., Nilson, D., Thelen, M. P., Siccardi, A., et al. (2019). Host Selection and Stochastic Effects Influence Bacterial Community Assembly on the Microalgal Phycosphere. Algal Res. 40, 101489. doi:10.1016/j.algal.2019.101489
Kou, Y., Wei, K., Li, C., Wang, Y., Tu, B., Wang, J., et al. (2020). Deterministic Processes Dominate Soil Methanotrophic Community Assembly in Grassland Soils. Geoderma 359, 114004. doi:10.1016/j.geoderma.2019.114004
Lear, G., Dopheide, A., Ancion, P., and Lewis, G. D. (2011). A Comparison of Bacterial, Ciliate and Macroinvertebrate Indicators of Stream Ecological Health. Aquat. Ecol. 45 (4), 517–527. doi:10.1007/s10452-011-9372-x
Li, Y., Fan, L., Zhang, W., Zhu, X., Lei, M., and Niu, L. (2020). How Did the Bacterial Community Respond to the Level of Urbanization along the Yangtze River? Environ. Sci. Process. Impacts 22 (1), 161–172. doi:10.1039/c9em00399a
Liu, J., Zhu, S., Liu, X., Yao, P., Ge, T., and Zhang, X.-H. (2020). Spatiotemporal Dynamics of the Archaeal Community in Coastal Sediments: Assembly Process and Co-occurrence Relationship. ISME J. 14 (6), 1463–1478. doi:10.1038/s41396-020-0621-7
Louca, S., Parfrey, L. W., and Doebeli, M. (2016). Decoupling Function and Taxonomy in the Global Ocean Microbiome. science 353 (6305), 1272–1277. doi:10.1126/science.aaf4507
Luo, X., Xiang, X., Huang, G., Song, X., Wang, P., Yang, Y., et al. (2020). Bacterial Community Structure Upstream and Downstream of cascade Dams along the Lancang River in Southwestern China. Environ. Sci. Pollut. Res. 27, 42933–42947. doi:10.1007/s11356-020-10159-7
Lynch, L. M., Sutfin, N. A., Fegel, T. S., Boot, C. M., Covino, T. P., and Wallenstein, M. D. (2019). River Channel Connectivity Shifts Metabolite Composition and Dissolved Organic Matter Chemistry. Nat. Commun. 10 (1), 459. doi:10.1038/s41467-019-08406-8
Marzinelli, E. M., Qiu, Z., Dafforn, K. A., Johnston, E. L., Steinberg, P. D., and Mayer-Pinto, M. (2018). Coastal Urbanisation Affects Microbial Communities on a Dominant marine Holobiont. NPJ Biofilms Microbiomes 4, 1. doi:10.1038/s41522-017-0044-z
Maynard, D. S., Crowther, T. W., and Bradford, M. A. (2017). Competitive Network Determines the Direction of the Diversity-Function Relationship. Proc. Natl. Acad. Sci. USA 114 (43), 11464–11469. doi:10.1073/pnas.1712211114
Mcgonigle, J. M., Bernau, J. A., Bowen, B. B., and Brazelton, W. J. (2019). Robust Archaeal and Bacterial Communities Inhabit Shallow Subsurface Sediments of the Bonneville Salt Flats. mSphere 4 (4), e00378–19. doi:10.1128/mSphere.00378-19
Nemergut, D. R., Schmidt, S. K., Fukami, T., O'Neill, S. P., Bilinski, T. M., Stanish, L. F., et al. (2013). Patterns and Processes of Microbial Community Assembly. Microbiol. Mol. Biol. Rev. 77 (3), 342–356. doi:10.1128/MMBR.00051-12
Parter, M., Kashtan, N., and Alon, U. (2007). Environmental Variability and Modularity of Bacterial Metabolic Networks. BMC Evol. Biol. 7, 169. doi:10.1186/1471-2148-7-169
Pearman, J. K., Ellis, J., Irigoien, X., Sarma, Y. V. B., Jones, B. H., and Carvalho, S. (2017). Microbial Planktonic Communities in the Red Sea: High Levels of Spatial and Temporal Variability Shaped by Nutrient Availability and Turbulence. Sci. Rep. 7 (1), 6611. doi:10.1038/s41598-017-06928-z
Rastogi, G., Behera, P., and Mohapatra, M. (2019). “Genetic Analysis of the Bacterioplankton Biology and Ecology through Next-Generation High-Throughput Molecular Techniques,” in Microbial Diversity in the Genomic Era. Editors S. Das, and H.R. Dash (Academic Press), 103–115. doi:10.1016/B978-0-12-814849-5.00007-1
Read, D. S., Gweon, H. S., Bowes, M. J., Newbold, L. K., Field, D., Bailey, M. J., et al. (2015). Catchment-scale Biogeography of Riverine Bacterioplankton. ISME J. 9 (2), 516–526. doi:10.1038/ismej.2014.166
Reese, A. T., Savage, A., Youngsteadt, E., McGuire, K. L., Koling, A., Watkins, O., et al. (2016). Urban Stress Is Associated with Variation in Microbial Species Composition-But Not Richness-In Manhattan. ISME J. 10 (3), 751–760. doi:10.1038/ismej.2015.152
Shade, A., Gregory Caporaso, J., Handelsman, J., Knight, R., and Fierer, N. (2013). A Meta-Analysis of Changes in Bacterial and Archaeal Communities with Time. ISME J. 7 (8), 1493–1506. doi:10.1038/ismej.2013.54
Simonin, M., Voss, K. A., Hassett, B. A., Rocca, J. D., Wang, S. Y., Bier, R. L., et al. (2019). In Search of Microbial Indicator Taxa: Shifts in Stream Bacterial Communities along an Urbanization Gradient. Environ. Microbiol. 21 (10), 3653–3668. doi:10.1111/1462-2920.14694
Staley, C., Gould, T. J., Wang, P., Phillips, J., Cotner, J. B., and Sadowsky, M. J. (2014). Core Functional Traits of Bacterial Communities in the Upper Mississippi River Show Limited Variation in Response to Land Cover. Front. Microbiol. 5, 414. doi:10.3389/fmicb.2014.00414
Stegen, J. C., Lin, X., Konopka, A. E., and Fredrickson, J. K. (2012). Stochastic and Deterministic Assembly Processes in Subsurface Microbial Communities. ISME J. 6 (9), 1653–1664. doi:10.1038/ismej.2012.22
Stegen, J. C., Lin, X., Fredrickson, J. K., Chen, X., Kennedy, D. W., Murray, C. J., et al. (2013). Quantifying Community Assembly Processes and Identifying Features that Impose Them. ISME J. 7 (11), 2069–2079. doi:10.1038/ismej.2013.93
Tang, J., Li, X., Cao, C., Lin, M., Qiu, Q., Xu, Y., et al. (2019). Compositional Variety of Dissolved Organic Matter and its Correlation with Water Quality in Peri-Urban and Urban River Watersheds. Ecol. Indicators 104, 459–469. doi:10.1016/j.ecolind.2019.05.025
Walsh, C. J., Roy, A. H., Feminella, J. W., Cottingham, P. D., Groffman, P. M., and Morgan, R. P. (2005). The Urban Stream Syndrome: Current Knowledge and the Search for a Cure. J. North Am. Benthological Soc. 24 (3), 706–723. doi:10.1899/04-028.1
Wang, S.-Y., Sudduth, E. B., Wallenstein, M. D., Wright, J. P., and Bernhardt, E. S. (2011). Watershed Urbanization Alters the Composition and Function of Stream Bacterial Communities. PLoS One 6 (8), e22972. doi:10.1371/journal.pone.0022972
Wang, Y., Pan, J., Yang, J., Zhou, Z., Pan, Y., and Li, M. (2020). Patterns and Processes of Free‐living and Particle‐associated Bacterioplankton and Archaeaplankton Communities in a Subtropical River‐bay System in South China. Limnol Oceanogr 65 (S1), S161–S179. doi:10.1002/lno.11314
Woodward, G., Gessner, M. O., Giller, P. S., Gulis, V., Hladyz, S., Lecerf, A., et al. (2012). Continental-scale Effects of Nutrient Pollution on Stream Ecosystem Functioning. Science 336 (6087), 1438–1440. doi:10.1126/science.1219534
Wu, W., Logares, R., Huang, B., and Hsieh, C. h. (2017). Abundant and Rare Picoeukaryotic Sub‐communities Present Contrasting Patterns in the Epipelagic Waters of Marginal Seas in the Northwestern P Acific O Cean. Environ. Microbiol. 19, 287–300. doi:10.1111/1462-2920.13606
Xiao, J., Fiscella, K. A., and Gill, S. R. (2020). Oral Microbiome: Possible harbinger for Children's Health. Int. J. Oral Sci. 12 (1), 12. doi:10.1038/s41368-020-0082-x
Xie, G., Tang, X., Shao, K., Hu, Y., Liu, H., Martin, R. M., et al. (2020). Spatiotemporal Patterns and Environmental Drivers of Total and Active Bacterial Abundances in Lake Taihu, China. Ecol. Indicators 114, 106335. doi:10.1016/j.ecolind.2020.106335
Xu, B., Wang, X., Liu, J., Wu, J., Zhao, Y., and Cao, W. (2017). Improving Urban Stormwater Runoff Quality by Nutrient Removal through Floating Treatment Wetlands and Vegetation Harvest. Sci. Rep. 7 (1), 7000. doi:10.1038/s41598-017-07439-7
Yang, Y., Gao, Y., Huang, X., Ni, P., Wu, Y., Deng, Y., et al. (2019). Adaptive Shifts of Bacterioplankton Communities in Response to Nitrogen Enrichment in a Highly Polluted River. Environ. Pollut. 245, 290–299. doi:10.1016/j.envpol.2018.11.002
Yang, W., Zheng, S.-Z., Zhou, S.-H., Zhao, L., Zhu, J.-Y., Lukwambe, B., et al. (2020). Structure and Functional Diversity of Surface Bacterioplankton Communities in an Overwintering Habitat for Large Yellow Croaker, Pseudosciaena Crocea, of the Southern East China Sea. Front. Mar. Sci. 7, 472. doi:10.3389/fmars.2020.00472
Zeglin, L. H. (2015). Stream Microbial Diversity in Response to Environmental Changes: Review and Synthesis of Existing Research. Front. Microbiol. 6, 454. doi:10.3389/fmicb.2015.00454
Zeng, J., Jiao, C., Zhao, D., Xu, H., Huang, R., Cao, X., et al. (2019). Patterns and Assembly Processes of Planktonic and Sedimentary Bacterial Community Differ along a Trophic Gradient in Freshwater Lakes. Ecol. Indicators 106, 105491. doi:10.1016/j.ecolind.2019.105491
Zhao, D., Cao, X., Huang, R., Zeng, J., Shen, F., Xu, H., et al. (2017). The Heterogeneity of Composition and Assembly Processes of the Microbial Community between Different Nutrient Loading lake Zones in Taihu Lake. Appl. Microbiol. Biotechnol. 101 (14), 5913–5923. doi:10.1007/s00253-017-8327-0
Zhou, J., and Ning, D. (2017). Stochastic Community Assembly: Does it Matter in Microbial Ecology? Microbiol. Mol. Biol. Rev. 81 (4), e00002–00017. doi:10.1128/MMBR.00002-17
Zhou, J., Deng, Y., Zhang, P., Xue, K., Liang, Y., Van Nostrand, J. D., et al. (2014). Stochasticity, Succession, and Environmental Perturbations in a Fluidic Ecosystem. Proc. Natl. Acad. Sci. USA 111 (9), E836–E845. doi:10.1073/pnas.1324044111
Zhu, Y.-G., Gillings, M., Simonet, P., Stekel, D., Banwart, S., and Penuelas, J. (2017). Microbial Mass Movements. Science 357 (6356), 1099–1100. doi:10.1126/science.aao3007
Keywords: surface water, ZOTUs, co-occurrence network, functional, ecological processes
Citation: Xiao Z, Li G, Zhao Y, Xiao K, Chen Q, Bao P, Tang J, Ruan T, Zama EF and Xu Y (2021) Bacterioplankton Richness and Composition in a Seasonal Urban River. Front. Environ. Sci. 9:731227. doi: 10.3389/fenvs.2021.731227
Received: 14 July 2021; Accepted: 07 September 2021;
Published: 29 October 2021.
Edited by:
Vinicius Fortes Farjalla, Federal University of Rio de Janeiro, BrazilReviewed by:
Renato Tavares Martins, Universidade Federal de Goiás, BrazilMaria Filomena Magalhães, University of Lisbon, Portugal
Copyright © 2021 Xiao, Li, Zhao, Xiao, Chen, Bao, Tang, Ruan, Zama and Xu. This is an open-access article distributed under the terms of the Creative Commons Attribution License (CC BY). The use, distribution or reproduction in other forums is permitted, provided the original author(s) and the copyright owner(s) are credited and that the original publication in this journal is cited, in accordance with accepted academic practice. No use, distribution or reproduction is permitted which does not comply with these terms.
*Correspondence: Yaoyang Xu, yyxu@iue.ac.cn