- 1Department of Natural Resources and the Environment, University of New Hampshire, Durham, NH, United States
- 2Stream Biofilm and Ecosystem Research Laboratory, École Polytechnique Fédérale de Lausanne, Lausanne, Switzerland
- 3Department of Earth Sciences, University of New Hampshire, Durham, NH, United States
Inland waters are the largest natural source of methane (CH4) to the atmosphere, yet the contribution from small streams to this flux is not clearly defined. To fully understand CH4 emissions from streams and rivers, we must consider the relative importance of CH4 emission pathways, the prominence of microbially-mediated production and oxidation of CH4, and the isotopic signature of emitted CH4. Here, we construct a complete CH4 emission budgets for four lowland headwater streams by quantifying diffusive CH4 emissions and comparing them to previously published rates of ebullitive emissions. We also examine the isotopic composition of CH4 along with the sediment microbial community to investigate production and oxidation across the streams. We find that all four streams are supersaturated with respect to CH4 with diffusive emissions accounting for approximately 78–100% of total CH4 emissions. Isotopic and microbial data suggest CH4 oxidation is prevalent across the streams, depleting approximately half of the dissolved CH4 pool before emission. We propose a conceptual model of CH4 production, oxidation, and emission from small streams, where the dominance of diffusive emissions is greater compared to other aquatic ecosystems, and the impact of CH4 oxidation is observable in the emitted isotopic values. As a result, we suggest the CH4 emitted from small streams is isotopically heavy compared to lentic ecosystems. Our results further demonstrate streams are important components of the global CH4 cycle yet may be characterized by a unique pattern of cycling and emission that differentiate them from other aquatic ecosystems.
Introduction
Since the start of the industrial revolution, atmospheric concentrations of methane (CH4) have increased nearly threefold (Saunois et al., 2020). This atmospheric enrichment of CH4 is caused predominantly by anthropogenic activities; however, aquatic ecosystems comprise roughly half of all global CH4 emissions (Rosentreter et al., 2021). Most research into CH4 emissions and cycling in freshwater systems has occurred in wetlands, lakes, and impoundments, but recent studies have started to highlight the role of rivers and streams in the global CH4 budget (Stanley et al., 2016; Zhang et al., 2020). Rosentreter et al. (2021) estimate that streams and rivers emit approximately 30.5 Tg CH4 yr−1 globally, which represents about 20% of the total annual emissions from lakes (151 Tg CH4 yr−1) or wetlands (149 Tg CH4 yr−1; Saunois et al., 2020). Methane cycling in small headwater streams, which comprise a majority of river network length (Bishop et al., 2008), represents a gap in our understanding of aquatic CH4 dynamics. Headwater streams are known to be disproportionately important in the emissions of carbon dioxide within river networks (Hotchkiss et al., 2015; Marx et al., 2017), yet their relative importance regarding CH4 remains undefined. Additionally, most investigations of CH4 focus on quantifying CH4 concentration or flux rather than how CH4 cycles through the ecosystem (Stanley et al., 2016). As a result, more comprehensive measurements of CH4 emissions and an improved understanding of CH4 cycling are needed to accurately include streams in regional and global CH4 budgets.
The fundamental controls of CH4 cycling are consistent across aquatic ecosystems, thus we can apply much of what is known about lentic systems to study stream ecosystems. In general, CH4 is produced via methanogenesis by archaea under anoxic conditions (Chowdhury and Dick, 2013), and the inundated sediments of aquatic environments are generally well-suited for this because of their low redox conditions (e.g., Wik et al., 2018). A key process that consumes CH4 in surface waters is biological oxidation by bacterial and archaeal methanotrophs, which can function under oxic or anoxic conditions (Conrad, 2009). Oxidation of CH4 is prevalent in aquatic ecosystems because methanotrophs are generally more productive where there are strong opposing gradients of CH4 and oxygen, common to aquatic ecosystems (Chowdhury and Dick, 2013). The oxidation of CH4 can be an important process mitigating CH4 emissions from aquatic systems (Bastviken et al., 2008; Sawakuchi et al., 2016).
Streams are largely differentiated from lentic waterbodies by the presence of flow, which may affect CH4 cycling via turbulent mixing and oxygenation of the water column and benthic environment (Trimmer et al., 2010). Previously, the mixing of oxic water into stream sediments was thought to limit methanogenesis, but stream sediments have been shown to support methanogenesis (Sanders et al., 2007; Bodmer et al., 2020). High rates of CH4 oxidation have also been observed in streams (Shelley et al., 2017), attributed to the strong redox gradient at the sediment-water interface and the introduction of oxygenated waters into the sediments via hyporheic flow (Hampton et al., 2020). However, the relatively rapid mixing of streams and rivers due to flow compared to lentic systems may reduce the proportion of CH4 oxidized before emissions (Sawakuchi et al., 2016). That is, higher turbulence and gas exchange in streams and rivers may result in less depletion of the CH4 pool via oxidation because of reduced transport time. This may explain why complete oxidation of the dissolved CH4 pool has been observed in lakes (Bastviken et al., 2008), but not in streams and rivers (Sawakuchi et al., 2016).
The relative importance of CH4 production and oxidation also affects the isotopic composition of CH4 (Chanton, 2005; Chanton et al., 2006). The δ13C-CH4 and δD-CH4 signature of emitted CH4 from a particular source (e.g., wetlands, landfills) can be used in atmospheric mixing models to constrain source contributions to bulk atmospheric CH4 (Schwietzke et al., 2016). For example, CH4 oxidation exerts a fractionation pattern of δ13C-CH4 and δD-CH4 enrichment compared to the source signature of the CH4 (Chanton et al., 2005). While the exact fractionation can vary significantly (Conrad, 2005), the change in δ13C-CH4 and δD-CH4 values typically range from approximately 5–20‰ and 50–250‰, respectively (Whiticar, 1999; Wang et al., 2016). The emission pathway by which gaseous CH4 leaves aquatic environment also has implications for the isotopic values of the emitted gas (Chanton, 2005). For example, Chanton et al. (1989) established that the δ13C-CH4 values found within released bubbles were not significantly different from those of the reservoir of CH4 held within the sediment. Meanwhile, CH4 that diffuses through sediments and into the water column is more susceptible to oxidation (e.g., Shelley et al., 2017). Thus, the balance of CH4 emission pathways can be important in determining the isotopic signature of a particular ecosystem (e.g., Sawakuchi et al., 2016) and how that ecosystem is included in atmospheric mixing models (Fisher et al., 2017; Saunois et al., 2020).
To properly understand the role of stream ecosystems in the global CH4 cycle, there is a need to not only quantify CH4 fluxes, but also investigate how CH4 cycles through these systems, using isotopic and microbial analyses. In this paper, we 1) quantify the relative contributions of diffusive and ebullitive emissions of CH4 from four headwater streams; 2) examine the δ13C-CH4 and δD-CH4 values of CH4 associated with these two emission pathways; and 3) explore the CH4-associated microbial community in the stream sediments to provide context for CH4 cycling. Integrating measurements of CH4 emissions with an investigation of production and oxidation is needed to understand CH4 cycling at the ecosystem level. By comparing our results to studies of other aquatic ecosystems such as larger rivers and lakes, we explore how CH4 cycling in streams may differ and what implications this has for aquatic CH4 budgets.
Materials and Methods
Site Description
This study was conducted in four lowland headwater streams in southeastern New Hampshire and northwestern Massachusetts, United States (Figure 1). Watersheds were selected to contrast CH4 dynamics in the two predominant land use conditions in this region (developed versus forested). These streams have been the focus of previous studies that examined nutrient and carbon cycling and the impact of land use (e.g., Wollheim et al., 2005; Wollheim et al., 2015; Wollheim et al., 2017), and were all monitored for CH4 ebullition from May through October 2019 (Robison et al., 2021). The watersheds of two streams, Sawmill Brook (D1) and College Brook (D2), are characterized by a relatively developed, suburban landscape, while the other two, Cart Creek (F1) and Dube Brook (F2), are predominantly forest-covered. We group these streams into these two watershed land cover classes for comparison, but recognize the limitation of statistical inference with such a small sample size and mixed land use.
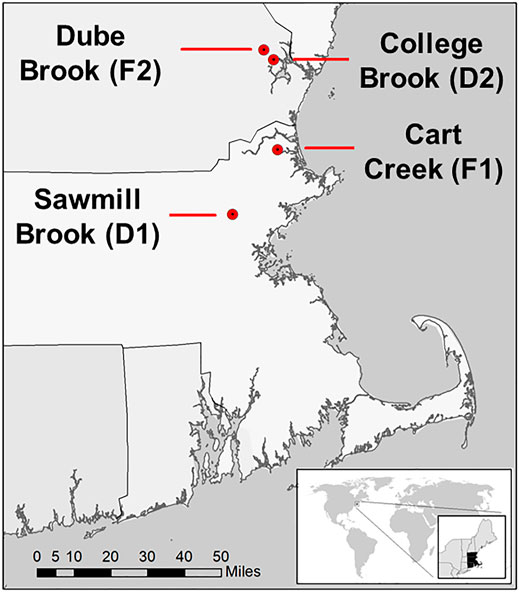
FIGURE 1. Locations of four study stream reaches in northeast Massachusetts and southeast New Hampshire, United States (Robison et al., 2021).
The watersheds range in size from 2.3 to 4.1 km2, and all streams exhibit relatively shallow slopes (Table 1). Mean annual rainfall is 1,280 mm yr−1 and mean annual air temperature is 8.9°C (Wollheim et al., 2017). Mean discharge ranges from 25.5 L s−1 at D2 to 36.7 L s−1 at D1. Stream chemistry is measured as part of the Plum Island Ecosystems LTER project (Morse and Wollheim, 2014; Wollheim et al., 2015). Stream reach characteristics were examined in a concomitant study (Robison et al., 2021). Mean reach depth at baseflow ranged from 7.2 cm at D2 to 14.5 cm at F1, and mean reach width at baseflow ranged from 1.94 m at D2 to 2.83 m at D1. All reaches exhibit shallow slopes of approximately 2 m km−1 or less. Benthic substrates included sand, silt, and fine organic matter, while rocks comprised less than 10% of benthic surface area across all sites. Macrophytes were absent from all reaches in this study, eliminating the possibility of CH4 efflux via plant-mediated transport.
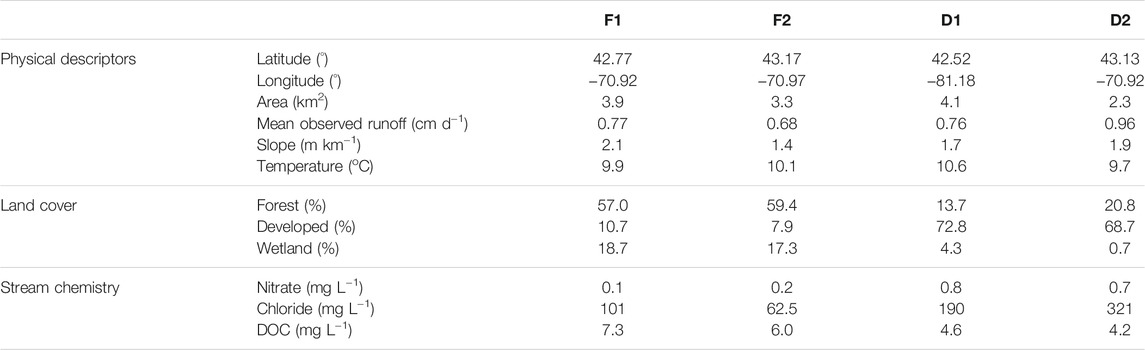
TABLE 1. Location, land use, and water quality characteristics of the four streams included in this study. Mean values of runoff, temperature, and stream chemistry are from the period of record.
Dissolved CH4 Sampling and Diffusive Efflux Estimation
Water samples for dissolved CH4 analysis were collected at each stream using 60 ml syringes fitted with three-way stopcocks. Samples were collected at variable frequencies ranging from multiple samples a week to roughly weekly between June 1 and October 31, 2019. On average, a sample was collected every 6 days at each site. Syringes were rinsed with stream water prior to sample collection. To collect water samples, syringes were filled with approximately 60 ml of stream water from 5 to 10 cm depth below the stream water surface. Syringes were cleared of air bubbles by inverting and expelling bubbles and water until 30 ml of sample water remained. Samples were stored on ice until returned to the laboratory within 6 h. In the laboratory, 30 ml of ambient air was added to each syringe to achieve a 1:1 ratio of sample water to air. Syringes were then shaken for 2 min to equilibrate gases between water and headspace (Magen et al., 2014). The water was then dispelled from the syringe, and the remaining headspace gas was saved for analysis. If the gas samples were not analyzed immediately, they were stored in evacuated glass vials sealed with a rubber septum until analyzed.
Samples were analyzed for CH4 concentration in the Trace Gas Biogeochemistry Laboratory at the University of New Hampshire. The CH4 concentration in parts per million by volume (ppmv) was determined using a Shimadzu Gas Chromatograph Flame Ionization Detector. Concentration was standardized using the average area response of ten injections of a standard CH4 mixture (Northeast Airgas, 2.006 ppmv or Maine Oxy, 1,000 ppmv) to determine instrument precision (Frolking and Crill, 1994). If multiple samples were collected from a single site, the mean measured concentration was used.
Diffusive fluxes of CH4 to the atmosphere (FCH4, mmol CH4 m−2 d−1) were calculated as:
where kCH4 (m d−1) is the gas transfer velocity for CH4 and ΔCH4 is the difference in CH4 concentration (g m−3) between the water and the air corrected for Henry’s Law. We used a constant air CH4 concentration of 1.94 ppmv based on measurements at the nearby Global Monitoring Laboratory site at the Isle of Shoals, New Hampshire during the monitoring period in 2019 (Earth System Research Laboratory, G. M. D., 2021). The gas exchange rate for CH4, kCH4, was calculated as:
where
where V is the water velocity at time of sampling (m s−1) and S is the channel slope (unitless). The mean slope of each stream reach was found using StreamStats (Ries et al., 2017). Mean daily discharge (Q; L s−1) was available from the long-term monitoring projects (Morse and Wollheim, 2014; Wollheim et al., 2017). Predictive relationships for V from Q were made using the equations for scaling stream geometry at a site (Knighton, 1998):
Two or fewer direct measurements of the gas exchange velocity were also made at each site, although generally under relatively low flow conditions (< 50 L s−1). These measurements were made using argon as a conservative gas tracer (Hall and Ulseth, 2020). We used these measurements to roughly evaluate the accuracy of our estimates of k600 (Supplementary Figure S1).
Ebullitive Sampling and Flux Estimation
Ebullitive CH4 fluxes were measured during the same time frame as diffusive flux by Robison et al. (2021). Briefly, stationary bubble traps were deployed in triplicate at three to four locations in each stream. Traps were visited at least weekly from June 1 to October 31, 2019, during which the volume of gas collected in each trap was measured and collected for analysis of CH4 concentration. The flux at each trap was then calculated as the mass of CH4 emitted over the observation period normalized per unit area under the trap (mmol CH4 m−2 d−1). For this study, mean rates of ebullitive CH4 flux per measurement period at each stream were used, based on the total number of funnels at a stream.
Isotopic Sampling and Analysis
Gas samples were collected for CH4 isotopic analysis in the first week of August 2019. Additional samples from F1 and D1 from the first week of September 2018 were also included in analysis. Dissolved CH4 samples from the surface water were collected in the same manner described above. Additional benthic gas samples were collected by physically disturbing the sediment and collecting released bubbles. A minimum of three sediment gas samples and three surface water dissolved gas samples were collected at each site. All samples were stored in evacuated glass vials sealed with a rubber septum for analysis.
Samples were analyzed for δ13C-CH4 and δD-CH4 using an Aerodyne dual tunable infrared laser direct absorption spectrometer (TILDAS; Aerodyne Research Inc, Massachusetts, United States ) at the University of New Hampshire. These instruments use high resolution infrared spectrometry to quantify trace gases such as CH4 (Mcmanus et al., 2011; Nelson and Roscioli, 2015). The TILDAS used here is configured with two 8 µm quantum cascade lasers (QCLs, Alpes Lasers, Switzerland) and a 200 m multipass absorption cell to simultaneously monitor 12CH4, 13CH4, and CH3D. The instrument was regularly calibrated with three standard tanks with known isotopic mixing ratios for 13CH4/12CH4 and CH4/CH3D. The isotopic composition of the standards was determined using an Aerodyne calibration system. The spectroscopic isotope ratios of four Isometric (now Airgas) CH4 standards were measured at diluted concentrations ranging from < 1 to 12 ppmv. Keeling plot analysis was used to determine the relationship between the spectroscopic and standard isotope ratios of the Isometric standards. The linear relationship was made to the corresponding measured spectroscopic isotopic ratios of the UNH calibration tanks. The TILDAS is configured with an automated sampling system designed to measure small (≤ 5 ml) injections of high concentration samples by diluting them within the instrument to a target CH4 mixing ratio of eight ppmv with ultra-zero air. The instrument precision was 0.1‰ for δ13C-CH4 and 3‰ for δD-CH3D at the target CH4 mixing ratio. Samples with very low concentration (<0.01 mM CH4) can result in erroneous isotopic measurement, and these datapoints (five of 59 samples) were removed prior to statistical analysis.
The percent of CH4 oxidized between sediment generation and surface water evasion was estimated using the equation:
where
Microbial Sample Collection and Analysis
Sediment cores were collected from the streams for 16S rRNA analysis. A modified Multi Stage Soil Core Sampler (AMS Inc, Idaho, United States ), consisting of a stainless steel cylinder and a plastic liner (5 cm diameter), was manually driven into the stream sediments using a sliding weight stand (Wik et al., 2018). Sediment cores were collected the first 2 weeks of July 2019 and processed in the field. Cores were collected at patches in the stream near each set of bubble traps as described in Robison et al. (2021). Four patches at each stream were sampled, except D2 where only three patches were sampled. At each patch, triplicate cores were collected. Sediment collected in the top 2 cm from each core was combined into a composite “surface” sediment sample in a Whirl-Pak sample bag. Similarly, sediment collected between 9 and 11 cm depth was combined into a composite “subsurface” sample. As a result, a total of four composite surface and four composite subsurface samples were collected at each stream except D2, where only three composite samples of each were collected. All tools used to collect cores, including the coring equipment, were sterilized with 70% ethanol between samples and sediment layers to minimize contamination. The depth of 9–11 cm was chosen as the subsurface sample depth because buried rocks or clay layers prevented collection of sediment at depths greater than 12–15 cm in some places. Thus, while 9–11 cm does not represent the maximum or mean depth of the sediment in portions of these streams, it represents a depth layer that could be collected across all sites and is deep enough where complete depletion of oxygen is likely (Crawford et al., 2014). Sediment samples were frozen on-site with liquid nitrogen, stored on ice, and returned to the laboratory within 4 h. Samples were stored in a −80°C freezer until analyzed.
Genomic DNA was extracted from sediment samples using a Qiagen DNeasy PowerSoil kit (Qiagen, Hilden, Germany) with minor changes to the manufacturer’s protocol (see Doherty et al., 2020). DNA was amplified by polymerase chain reaction (PCR) using the primers 515f–806r of the V4 region of the 16S rRNA gene to profile bacterial and archaeal communities (Apprill et al., 2015; Parada et al., 2016). Each reaction contained 6 µl DreamTaq Hot Start Green (Thermo Fisher Scientific, Waltham, MA, United States ), 2.6 µl sterile water, 0.7 µl forward primer (5 µM), 0.7 µl reverse primer (5 µM), and 2 µl template DNA (10× diluted). DNA amplification was performed using a T100 Thermal Cycler (Bio-Rad, Hercules, CA, United States). The 16S rRNA conditions were: enzyme activation at 95°C for 3 min; followed by 35 cycles of denaturation at 95 °C for 30 s, annealing at 55°C for 30 s, and extension at 72°C for 60 s; then a final extension at 72°C for 12 min.
Gel electrophoresis was used to confirm the presence of the PCR product, which was then quantified using a Quant-iT dsDNA High-Sensitivity Assay Kit and a Qubit 3.0 fluorometer (Thermo Fisher Scientific, Massachusetts, United States). Resulting concentrations ranged from 0.5 to 28 ng μL−1, with sandier sediment samples typically having lower concentrations. PCR products were sent to the Hubbard Center for Genome Studies (University of New Hampshire, New Hampshire, United States) for sequencing by Illumina HiSeq2500 (250 bp reads) with Rapid Run© SBS V2 chemistries (Illumina, San Diego, CA, United States). Sequence reads were demultiplexed using CASAVA.
Sequences were analyzed using QIIME 2 (version 2020.1; Bolyen et al., 2019) on the Premise high performance computing cluster at the University of New Hampshire. Cutadapt was used to remove primers (Martin, 2011). Using DADA2 (Callahan et al., 2016), sequences were truncated at the length where the median Phred score (quality score) fell below 30 (200 bp for forward read; 225 bp for reverse read) and unique sequences were designated as amplicon sequence variants (ASVs). Each sample was then rarefied to 3,400 sequences per sample. Rarefication depths were chosen to ensure at least three samples remained for each depth at each stream. Taxonomy was assigned to ASVs using the SILVA database (release SILVA 138 SSU; Quast et al., 2013; Glöckner et al., 2017). The relative abundances of taxa were calculated after removing chloroplasts, mitochondria, and taxa present in less than 5% of samples.
In this study, we focused primarily on taxa associated with CH4 production and CH4 oxidation. Following Hough et al. (2020), we identified methanogens based on classification into the archaeal orders: Methanocellales, Methanobacteriales, and Methanomicrobiales, and the families Methanosarcinaceae and Methanosaetaceae, of order Methanosarcinales (Evans et al., 2019). Similarly, we identified methanotrophs as those from the bacterial orders Methylococcales and Methylomirabilales and the archaeal family Methanoperedenaceae, order Methanosarcinales (Smith and Wrighton, 2019). Taxa from the order Methylococcales are classical aerobic methanotrophs, while those from the order Methylomirabilales are capable of CH4 oxidation following reduction of nitrate or nitrite (Wu et al., 2011); that is, they are capable of generating oxygen for CH4 oxidation when in an anoxic environment. Finally, Methanoperedenaceae are a methanotrophic archaea family (formerly known as ANME-2d) known to be capable of CH4 oxidation via alternative electron donors like nitrate, iron, or manganese (Ettwig et al., 2016; Leu et al., 2020). Despite the indefinite nature of assigning organismal function using 16S sequencing, traits associated with CH4 cycling are typically conserved and well defined (Martiny et al., 2015), allowing for confidence in these assignments. The relative abundance of individual samples is calculated, from which a mean and standard deviation from all samples collected at a sediment depth in a stream is determined.
Statistical Analyses
All calculations and statistical analyses were performed in MATLAB and Statistics Toolbox Release 2020a (The MathWorks, Inc, Massachusetts, United States), and the significant level was set at α = 0.05. Uncertainty in the dissolved CH4 concentration for each site was determined by the ratio of the standard error (SE) of the measured concentrations to the median concentration (Hojo and Pearson, 1931):
which resulted in a unique percent uncertainty at each site. Uncertainty in the gas exchange rate was determined by the standard deviation included in the factors of Eq. 3. Simple linear interpolation was used to estimate the rate of diffusive efflux on days without a dissolved CH4 concentration measurement. Uncertainty in the diffusive CH4 flux was then estimated by propagating the uncertainty from dissolved CH4 concentration and gas exchange rates. We summarized CH4 concentration, gas exchange, and rates of diffusion as medians because of the non-normal distribution of the data. Differences in CH4 concentration, gas exchange, and diffusive emissions between streams were analyzed using the nonparametric Wilcoxon rank sum test. Trends in CH4 concentration and diffusion across time were analyzed using the nonparametric Kendall rank correlation coefficient. Isotopic and microbial data were summarized as means because of their small sample sizes and unknown distributions. Differences between streams, depths for microbial samples, and type of sample for isotopes (i.e., benthic or surface water) were compared using two-sample t-test or one-way ANOVA.
Results
Dissolved CH4 Concentration and Diffusive Fluxes
Measured concentrations of dissolved CH4 in surface water were above saturation (roughly 0.003 µmol CH4 L−1 at 10°C) in all collected samples, with an overall median ±SE of 1.1 ± 0.4 µmol CH4 L−1 (Table 2; Figure 2A). F2 and D2 had the highest median concentration of CH4 (1.6 ± 0.9 µmol CH4 L−1) and F1 had the lowest (0.9 ± 0.3 µmol CH4 L−1). There was no statistical difference in the median dissolved concentration of CH4 between watershed land cover classes (p > 0.05). We also observed no clear pattern in the dissolved concentration across time at any individual stream (p = 0.54). The median kCH4 across all sites was 4.8 ± 0.4 m d−1, ranging from 3.4 ± 0.3 m d−1 at F2 to 5.9 ± 0.6 m d−1 at D1 (Table 2; Figure 2B). The watershed land cover classes did differ in the estimated gas exchange rates (p < 0.01), where the two streams draining developed landscapes, D1 and D2, exhibited significantly higher kCH4. Calculated rates of gas exchange were similar to limited direct measurements in each of the streams (Supplementary Figure S1) using short-term continuous injections of a volatile gas tracer (Hall and Ulseth, 2020). Median diffusive CH4 flux was 5.7 ± 2.3 mmol CH4 m−2 d−1 overall (Table 2; Figure 2C). In individual streams, the median diffusive flux ranged from 4.1 ± 2.1 to 7.8 ± 4.8 mmol CH4 m−2 d−1 at F1 and D2, respectively, with the developed watershed class exhibiting a higher rate compared to the forested watershed class (p < 0.01). We did not observe any clear temporal pattern in diffusive CH4 emissions across our monitoring period at any individual site (p > 0.05). High diffusive CH4 fluxes were more strongly associated with high measurements of dissolved CH4 than with high gas exchange rates (Figure 2). The maximum daily rate of diffusive CH4 efflux was 70.8 ± 31.4 mmol CH4 m−2 d−1 at D2.
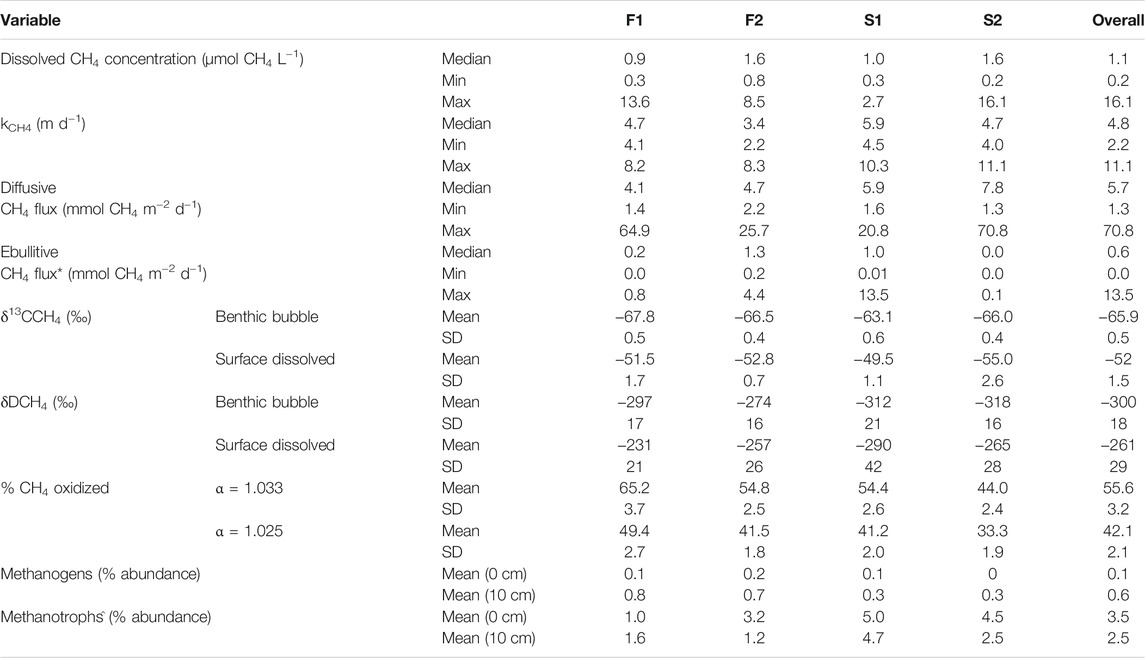
TABLE 2. Summary of measured dissolved methane concentration, estimated methane-specific gas exchange rate, calculated diffusive and ebullitive emissions, measured δ13C-CH4 and δD-CH4 values, estimated percent of the dissolved CH4 oxidized before emission, and the percent abundance of methanogens and methane oxidizing bacteria in the total detected community for the four streams in this study. SD indicates the standard deviation. *Ebullitive methane flux data is from Robison et al. (2021). ^Methanotrophs refers to taxa capable of CH4 oxidation, whether aerobic or anaerobic.
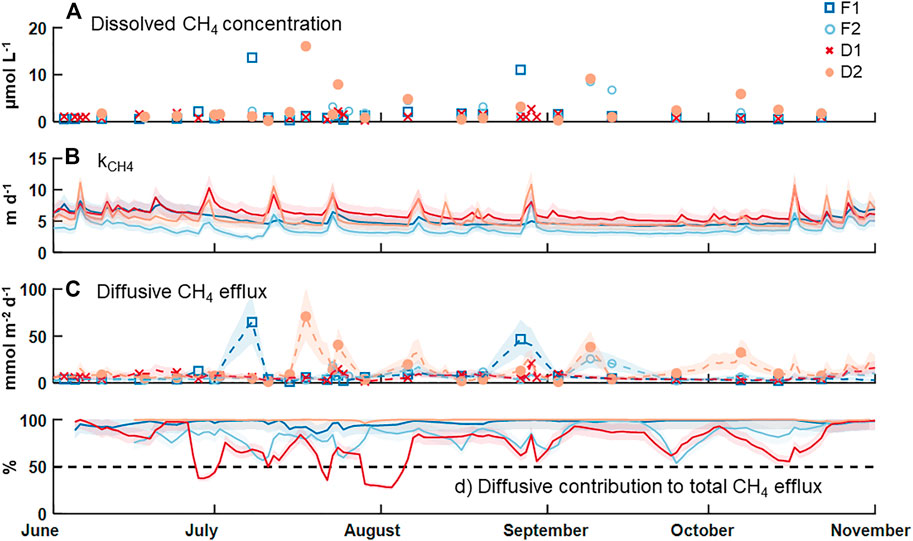
FIGURE 2. Time series of (A) measured dissolved CH4 concentration, (B) estimated gas exchange rate for CH4, (C) estimated diffusive flux rate for CH4 on days with a concentration measurement (markers) and the days between via linear interpolation (dashed lines), and (D) the percent contribution of diffusion to the total CH4 efflux budget. The shaded areas indicate uncertainty in estimating the gas exchange rate an in linear interpolation of the diffusive emission rates.
Diffusive fluxes of CH4 were compared to ebullitive fluxes of CH4 from Robison et al. (2021) during the same period of record (Table 2; Figure 2D). Across all four streams, the median diffusive CH4 flux (5.7 ± 2.3 mmol CH4 m−2 d−1) was greater than the median ebullitive CH4 flux (0.6 ± 0.1 mmol CH4 m−2 d−1). Diffusive emissions of CH4 comprised approximately 90% of total CH4 emissions from these four streams over the entire period, ranging from 78% at F2 to nearly 100% at D2. Ebullitive CH4 flux exceeded diffusive flux only at D1 for short periods (Figure 2D). Diffusion accounted for the majority of CH4 emissions at the other three sites during the entire monitoring period.
Stable Isotopic Composition of CH4
Benthic gas and surface water gas samples exhibited clear differences in δ13C-CH4 and δD-CH4 values (Table 2; Figure 3). Generally, there was more variability between the type of sample (i.e., benthic or surface water) than between streams. Benthic gas δ13C-CH4 and δD-CH4 values averaged across all streams were −65.9 ± 2.0‰ and −300 ± 18‰, respectively. There was some variation in the benthic δ13C-CH4 values between streams, where D1 exhibited a significantly higher mean δ13C-CH4 (−63.1 ± 0.6‰) than F1 (−67.8 ± 0.5‰, p < 0.01) and F2 (−66.5 ± 0.4‰, p = 0.02). More variation was noted in measured δD-CH4 values, with F2 having the highest mean value of −257 ± 16‰ and D2 having the lowest value of −318 ± 16‰, with only these two sites being significantly different (p = 0.01). Dissolved CH4 δ13C-CH4 and δD-CH4 values averaged across all four streams were −52 ± 1.5‰ and −261 ± 29‰, respectively. These were slightly more varied across streams than the benthic samples. For example, δ13C-CH4 values ranged from −49.5 ± 0.6‰ at D1 to -55.0 ± 2.6‰ at D2 in dissolved CH4 samples, although no site was significantly different from the others. Meanwhile, dissolved δD-CH4 ranged from −257 ± 26‰ at F2 to −290 ± 42‰ at D1, with only these two sites being significantly different (p = 0.04). There was no difference in measured isotopic values between watershed land cover classes when comparing either δ13C-CH4 or δD-CH4 between benthic gas and dissolved samples.
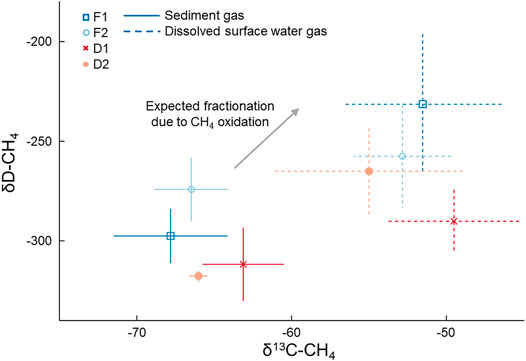
FIGURE 3. Mean (points) and standard deviation (lines) of δ13C-CH4 and δD-CH4 values of samples from sediment gas and dissolved gas in the surface water collected in August 2019. Sediment gas was collected by disturbing the sediment and collecting released bubbles. Points indicate mean values and bars represent the standard deviation. The arrow depicts the expected isotopic fractionation of CH4 due to oxidation (Chanton et al., 2005).
Dissolved CH4 in surface water was more 13C- and D-enriched relative to the sediment gas overall (p < 0.01; Figure 3). This amounted to a 14 ± 1.5‰ and 39 ± 29‰ shift towards the heavier isotope for δ13C-CH4 and δD-CH4, respectively. The δ13C-CH4 values for dissolved CH4 were all significantly higher than sediment CH4 at each stream, and the δD-CH4 values were significantly higher than sediment CH4 at F1 (p = 0.01) and D1 (p = 0.04). Given this enrichment in δ13C-CH4 values, we estimated the mean proportion of dissolved CH4 oxidized was 42.1 ± 2.1% when using α = 1.033, and 55.6 ± 2.8% when using α = 1.025 (Table 2). The percentage of dissolved CH4 oxidized in individual streams ranged from a minimum of 33.3 ± 3.1% at D2 using α = 1.025 to 65.2 ± 2.6% at D1 given α = 1.033.
Methane-Associated Microbial Community
Taxa identified as methanogens and methanotrophs were detected across all four streams (Figure 4A, Supplementary Table S1). Methanogen taxa were detected in 10 of 15 surface sediment samples, and 14 of 15 subsurface samples (Figure 4B). These included the archaeal orders Methanosarcinales (families Methanosaetaceae and Methanosarcinaceae), Methanobacteriales (family Methanobacteriacea), Methanomicrobiales (families Methanomicrobiaceae, Methanoregulaceaea, and rice cluster II), and Methanocellales (family Methanocellaceae). As a percent of the total community, methanogens comprised roughly 0.1% of the surface sediment community and 0.6% of the subsurface community, on average. Methanogens comprised a larger percentage of the microbial community in the forested watershed streams than in the developed streams (p = 0.04).
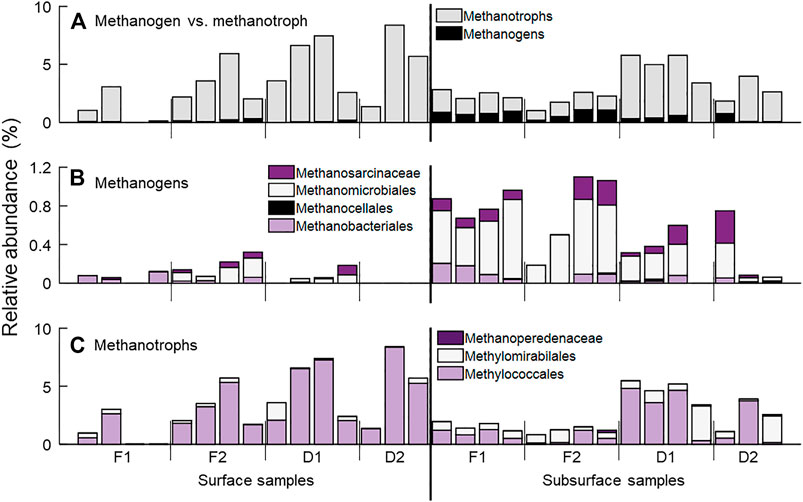
FIGURE 4. Relative abundance of CH4-associated taxa at sampling locations. (A) All taxa associated with CH4 production (methanogens, black) and oxidation (methanotrophs, gray). Here, we use the simplified term methanotrophs to refer to those taxa who are capable of CH4 oxidation through any of a variety of biochemical pathways. (B) Archaeal taxa identified as methanogens detected in sediment samples, including the orders Methanomicrobiales, Methanocellales and Methanobacteriales, and the families Methanosarcinales and Methanosaetaceae, both of the order Methanosarcinales. (C) Taxa associated with CH4 oxidation, separated into categories based on potential biochemical pathways. The bacterial order Methylococcales are canonical, aerobic CH4 oxidizing bacteria. The bacterial order Methylomirabilales are proposed to be capable of CH4 oxidation following the reduction of nitrite. Finally, methanotrophic archaea from the order Methanosarcinales, family Methanoperedenaceae (formerly known as ANME-2d) are also capable of CH4 oxidation following the reduction of nitrate, iron, or manganese.
Taxa classified as methanotrophs were detected in 14 of 15 surface samples and all subsurface samples (Figure 4C). Taxa from the bacterial orders Methylococcales (families Methylococcaceae and Methylomonaceae), Methylomirabilales (family Methylomirabilaceae), as well as the archaeal order Methanosarcinales (family Methanoperedenaceae) were detected. Methanotrophs comprised a larger fraction of the total microbial community in all samples compared to methanogens, but this may be a result in part of the bias of our primers for bacteria over archaea (Walters et al., 2016). As a percent of the total community, methanotrophs comprised on average 4.3 and 3.6% in surface and subsurface samples, respectively. Aerobic methanotrophs, i.e., Methylococcales, were significantly more abundant in surface sediment samples (p = 0.02), while taxa capable of CH4 oxidation in anoxic conditions (Methylomirabiales and Methanoperedenaceae) were higher in relative abundance in subsurface samples than in surface samples (p = 0.02). Additionally, methanotroph relative abundance was greater in the two streams draining developed watersheds than the two draining forested watersheds (p < 0.01).
Discussion
Diffusion via turbulent mixing was the dominant pathway of CH4 emissions from the stream reaches in this study (Figure 2D; Table 2). This appears more common in small streams compared to larger rivers or lentic ecosystems, where ebullition tends to be more important. The enrichment of CH4 isotopic values from the benthos to the surface water is likely a result of oxidation, which is supported by the presence of a diverse microbial community capable of CH4 oxidation. Emitted CH4 from these streams thus exhibits isotopic values more characteristic of the dissolved CH4 pool, i.e., heavier than the benthic gas pool. Together, our results suggest CH4 emission and isotopic patterns in small streams may be unique amongst aquatic ecosystems, and thus may distinguish how small streams should be included in global CH4 models.
Diffusive Emissions Dominate CH4 Efflux Budget
Diffusive emissions were the dominant efflux pathway of CH4 in these four lowland stream reaches. The median diffusive flux is comparable to a summary of 385 streams and rivers (8.22 ± 25.50 mmol CH4 m−2 d−1; Stanley et al., 2016). Total CH4 emissions were greater than the median of a recent analysis of streams and rivers globally (0.9 mmol CH4 m−2 d−1), but within the 90% range (0.0–35.8 mmol CH4 m−2 d−1; Rosentreter et al., 2021). When compared to lentic waterbodies, the rate of emissions from these four streams is similar on a per area basis (e.g., Holgerson and Raymond, 2016; Sanches et al., 2019). For example, in a variety of lakes and ponds of northern latitudes, total CH4 emissions ranged from 2.8 to 12.5 mmol CH4 m−2 d−1 (Wik et al., 2016b).
The efflux of CH4 from small streams may be a significant, yet overlooked pathway of carbon fluxes from inland waters. The total aquatic carbon flux for inland waters across North America is estimated to be 24 g C m−2 yr−1 (Butman et al., 2018), where the flux is normalized for total land area. However, this flux does not include CH4, as scaling was limited by significant data gaps at the time of estimation. Following scaling methods published previously (Robison et al., 2021), the streams in this study emit roughly 0.08 g CH4-C m2 watershed area yr−1 when both diffusion and ebullition are considered, less than 1% of the total estimated for inland waters. If the greater radiative forcing of CH4 relative to CO2 is taken into account (Saunois et al., 2020), the CO2-equivalent rate increases to 2.2 g C m2 watershed area yr−1. Thus, ignoring small stream methane emissions may underestimate watershed carbon emissions by approximately 10%, a significant factor.
Relatively few studies exist with which to compare diffusion and ebullition in lotic systems (Supplementary Table S2). Summarizing 26 streams and rivers which considered both pathways, diffusion delivered 68% of total CH4 emissions on average, but this ranged from 14 to 94% (Stanley et al., 2016). However, it appears smaller streams tend to favor diffusive emissions. For example, diffusion comprised roughly 90% of CH4 emissions in a small stream in Wisconsin, United States (Crawford et al., 2014). In contrast, studies of larger rivers in the Amazon (Sawakuchi et al., 2014), on the East Qinghai–Tibet Plateau (Zhang et al., 2020), and in urban China (Wang et al., 2021) found diffusion accounted for 64, 21, and 20% of total CH4 emissions, respectively. Diffusive fluxes generally account for a smaller fraction of the total CH4 budget in lentic systems too, e.g., 48% for northern lakes and ponds (Wik et al., 2016b).
Land use may affect diffusive CH4 emissions, where development promotes higher rates of emission. This pattern follows previous studies which linked low oxygen and high organic carbon delivery to higher rates of CH4 production in urban streams (Stanley et al., 2016; Wang et al., 2021). With only four streams studied and mixed land use within each watershed, the strength of this comparison is narrow. Individual samples from each stream exhibited relatively high concentrations of dissolved CH4. The median and maximum of dissolved CH4 concentrations were similar to those found in a yearlong survey of gas concentrations in streams in New Hampshire (0.63 and 13.5 µmol CH4 L−1; Herreid et al., 2020). Our dataset precludes analysis of what causes these high concentrations, but we have no reason to discard them from our analysis as they fall within ranges observed in streams and rivers locally and globally (Stanley et al., 2016).
The estimation of diffusive CH4 efflux is limited by the relatively sparse measurements of dissolved CH4 concentrations and the estimation of gas exchange. The similarity of direct gas exchange measurements to estimates used in this study suggests this latter uncertainty is modest during low flow conditions (Supplementary Figure S1). Similarly, while our results likely suffer in accuracy at fine temporal scales (i.e., daily), it does not necessarily indicate the summarized results are inaccurate. Uncertainty due to sampling frequency on estimating seasonal rates of CH4 emissions in lakes has been examined previously (Wik et al., 2016a); here, a minimum of 17 measurements of dissolved CH4 concentration were needed to accurately represent seasonal emission patterns from lakes. The minimum number of measurements of dissolved CH4 concentration in this study is 25. While the standard for lakes may not be the same for lotic ecosystems, we believe our results reflect the typical seasonal behavior at these streams in which diffusion is the dominant emission pathway for CH4.
Evidence for In-Stream CH4 Production and Oxidation From Isotopic and Microbial Data
The isotopic and microbial results provide evidence for active CH4 processing in these streams, with production and oxidation ubiquitous. For example, the presence of ebullition and the detection of methanogens suggests active CH4 production in the sediment of all four streams. The δ13C-CH4 and δD-CH4 values of the sediment-derived gas suggest a combination of acetoclastic (−60‰ to −50‰ δ13C-CH4 and −400‰ to −250‰ δD-CH4) and hydrogenotrophic (−110‰ to −60‰ δ13C-CH4 and −250‰ to −170‰ δD-CH4) methanogenesis (Whiticar, 1999; Chanton et al., 2005), which is common in aquatic environments (Shelley et al., 2015; Wik et al., 2020). The presence of methanotrophs and an enrichment in both δ13C-CH4 and δD-CH4 values between benthic and surface samples suggests CH4 oxidation was prevalent across the four streams as well, representing a potentially significant control on emissions. The estimated oxidation proportions are similar, but generally lower than the 57–82% range estimated for large rivers in the Amazon River basin (Sawakuchi et al., 2016) or the 57–100% estimated for lakes in Sweden (Bastviken et al., 2002). The lower oxidation proportion in the streams could be a result of relatively faster turnover of the water column. That is, while the efficiency of CH4 oxidation could be similar across all aquatic ecosystems, relative fast mixing in small streams may limit the time for CH4 oxidation to occur. Regarding microbial evidence for CH4 production and oxidation, detection of microbial taxa via 16S rRNA sequencing can recover dormant or dead microbes (Oliver, 2005), thus the detections do not directly indicate activity. However, combined with the magnitude of CH4 fluxes and isotopic values, we can confidently infer the presence of microbial CH4 production and oxidation in the streams.
Alternative explanations for the change in isotopic values are possible. The difference could result from dissolved CH4 inputs from a different source in the watershed, e.g., the riparian zone (Crawford et al., 2013). If the balance of isotopic values of this other source is distinct from that in the stream sediments, the isotopic values of the dissolved CH4 in the streams may be confounded by the mixed sources. Additionally, diffusion exerts a fractionation effect (< 3‰ δ13C-CH4) on CH4, where lighter CH4 is preferentially emitted (Knox et al., 1992). This fractionation is much smaller than that of oxidation and the time for diffusion is relative fast in these streams, limiting the impact of this effect. Because isotopic and microbial sampling occurred in August and July, respectively, the results of our oxidation analysis may reflect the conditions of this warmer period of the year. For example, CH4 isotopes in aquatic ecosystems may be heaviest in the summer (Atkins et al., 2017), possibly as a result of more active microbial oxidation (Shelley et al., 2015). If so, this would indicate we may have measured conditions under which oxidation is most efficient. However, considering the isotopic results in the context of the detection of methane oxidizing microbes, we are confident CH4 oxidation is prevalent in these streams with the greatest uncertainty around the temporal dynamics of oxidation efficiency.
Mechanisms Driving CH4 Emission Pathway and Oxidation Potential in Headwater Streams
The dominance of diffusive CH4 emissions and the relatively lower proportion of CH4 oxidation appear to distinguish small streams from other aquatic ecosystems. Identifying the factors that drive this difference is thus key to understanding how CH4 dynamics may differ in small streams. We propose two characteristics that are fundamental in differentiating small streams from larger rivers and from lentic systems: flow and depth. We hypothesize limited bubble formation and CH4 oxidation results from the unique physical and biogeochemical conditions of small streams caused by increased water exchange between the water column and sediments (hyporheic flow) and short residence time of CH4 in the water column (gas exchange).
Flow provides lotic ecosystems with consistent turbulent mixing, and the relatively shallow nature of small streams propagates this turbulence to the sediment–water interface. These likely limit bubble formation broadly via changes to sediment quality and depth. Because bubble formation is greatly affected by the grain size and physical structure of aquatic sediments (Liu et al., 2016), processes like turbulence which affect the sediment will in turn affect ebullition. For example, the erosional nature of streams (Knighton, 1998) may inhibit ebullition by increasing mean grain size or reducing deposition of organic matter, both of which have been shown to limit ebullition in streams (Crawford et al., 2014; Bodmer et al., 2020). Turbulence also enhances exchange at both the sediment–water and air–water interface within streams (Boano et al., 2014), affecting both bubble formation and oxidation rates. Advective water inputs into and through stream sediments via hyporheic flow (Packman and Salehin, 2003) create patches of oxic and anoxic conditions in close proximity (MahmoodPoor Dehkordy et al., 2019; Nelson et al., 2019). The CH4 produced in anoxic zones can be transported through sediments by this advective flow (Sobczak and Findlay, 2002), limiting bubble formation further, exposing CH4 to oxidizing conditions, and decreasing the residence time of CH4 within the sediment. This advective exchange may ultimately limit the overall potential for CH4 oxidation. For example, in Amazonian rivers, the extent of CH4 oxidation was positively related to residence time in surface sediments (Sawakuchi et al., 2016). Oxidation within the water column is also limited by higher rates of gas exchange in streams and rivers compared to lentic ecosystems (Looman et al., 2021), as does the shallower depth of streams compared to larger rivers (Raymond et al., 2012). For example, 51–100% of the CH4 in lakes originating in sediments in deeper portions of lakes was oxidized in the water column, while only 24–40% of the CH4 from shallower areas was oxidized due to the relatively shorter transit time (Bastviken et al., 2008).
We propose a conceptual model of CH4 production, transport, oxidation, and emission unique to small streams, where CH4 is emitted primarily through diffusive fluxes and oxidation is relatively limited (Figure 5). In this model, 1) CH4 is primarily produced by methanogens in anoxic sediments. While bubble formation is generally limited in small streams, 2) some CH4 is emitted via ebullition. The bulk of CH4 production, plus inputs from the upslope catchment via groundwater flow, remains dissolved. In small streams, dissolved CH4 3) diffuses through the sediments entering hyporheic flow paths, 4) although small amounts of vertical diffusion through the sediments may also occur. Hyporheic flow, which remains primarily anoxic in many systems, moves dissolved CH4 back to the water column. We propose 5) oxidation primarily occurs near the sediment–water interface, where the redox gradient is greatest and the highest abundance of methanotrophs are detected. In the surface water, CH4 oxidation is likely limited due to relatively high gas exchange rates. After oxidation, 6) the remaining dissolved CH4 emitted via diffusion is enriched isotopically relative to that emitted via ebullition. The relative importance of each of these processes will depend on stream characteristics such as slope, water velocity, water depth, and sediment characteristics (i.e., depth, grain size) that influence the degree of hyporheic exchange, hyporheic residence time, redox conditions, and microbial activity. Some of the proposed mechanisms described in this conceptual model were not directly measured (e.g., hyporheic exchange) and require further exploration and testing. We specifically urge the examination of CH4 cycling and transport within the benthic environment in relation to subsurface flow and redox conditions.
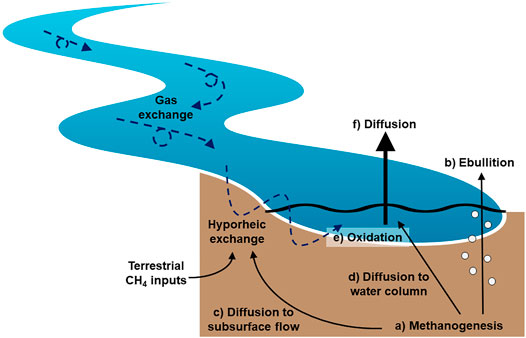
FIGURE 5. Proposed conceptual model of CH4 production, transport, oxidation, and emissions in stream ecosystems. The inherent flow and shallow nature of streams promotes hyporheic exchange and high gas exchange rates. This serves to limit bubble formation and promote the transport of dissolved CH4 out of the sediments via diffusion and subsurface flow. While the dissolved CH4 pool is exposed to oxidation, the proportion of CH4 that is oxidized is limited by the relatively rapid exchange of water and gases in stream ecosystems. Most CH4 is emitted via diffusion across the water-air interface in streams, and the limited exposure of this CH4 pool results in relatively heavy dissolved CH4 isotopic values. Because diffusive CH4 emissions dominate the overall emission budget, the mean isotopic value of emitted CH4 reflects this heavier signature and CH4 isotopes in streams are enriched relative to other aquatic ecosystems.
Lotic Ecosystems May Be Isotopically Enriched Relative to Lentic Systems
One important implication of this conceptual model is how streams are considered in global CH4 models (Dean et al., 2018), particularly with regards to the isotopic values of emitted CH4. For example, given both the imbalance of emission pathways in this study, as well as the isotopic values of ebullitive and diffusive gases, we calculate mean isotopic values of −55.7 ± 2.1‰ δ13C-CH4 and −260 ± 36‰ δD-CH4 for the total CH4 emitted. The δ13C-CH4 reflects relatively heavy values compared to global averages of approximately −62‰ δ13C-CH4 used for aquatic systems (Schwietzke et al., 2016). Considering streams and rivers account for roughly 8% of the global aquatic CH4 budget (Rosentreter et al., 2021), this difference could be enough to alter the global isotopic signature used for aquatic CH4 sources.
Very few studies which combine measurements of CH4 isotopes and emission pathways are available in lotic systems, limiting comparison of our results (Supplementary Table S2). The most extensive data in rivers comes from the Amazon, where consideration of both ebullitive and diffusive emissions provides mean δ13C-CH4 values for total emitted CH4 of −49.6‰ (Sawakuchi et al., 2016) and −38.4‰ (Sawakuchi et al., 2021). In these large tropical rivers, diffusion comprises a smaller fraction of the total emission budget, but a greater proportion of dissolved CH4 is oxidized and thus the emitted CH4 is more isotopically enriched. Our proposed conceptual model allows for these differences, where the higher efficiency of oxidation in larger rivers is enough to counter the higher prevalence of ebullition (Sawakuchi et al., 2021). Further, hyporheic flow is relatively less important in large rivers (Battin et al., 2008), potentially resulting in a greater proportion of CH4 accumulating in bubbles in river sediments compared to streams. Even fewer studies exist with which to compare δD-CH4 values. The streams in this study (−260‰) are δD-CH4 enriched relative to Swedish lentic ecosystems (−310‰; Wik et al., 2020), which further supports the preferred emissions of oxidized CH4 from streams. More closely comparing lotic δ13C-CH4 signatures to wetlands of similar latitudes further supports relative isotopic enrichment in lotic systems. Latitudinal patterns have been observed in aquatic δ13C-CH4 signatures, with enriched values typically characteristic of more tropical environments (Ganesan et al., 2018). The values measured in the present study are enriched relative to wetlands at a similar latitude (approx. −67‰; Fisher et al., 2017). Similarly, δ13C-CH4 values measured in fluvial portions of the Amazon River basin (Sawakuchi et al., 2016; Sawakuchi et al., 2021) are enriched relative to other tropical wetlands (approx. −60‰; Brownlow et al., 2017). There remains uncertainty in how much aquatic CH4 emissions are contributing to variation in atmospheric δ13C-CH4 values (Turner et al., 2019), thus expanding measurement of aquatic CH4 isotopes remains a valuable endeavor. While many additional measurements across ecosystems will be needed to confirm our proposed model, the possibility of a unique isotopic signature for streams and rivers is significant.
Conclusion
This study demonstrates how small streams can emit CH4 at similar rates to other aquatic ecosystems on a per area basis, but with distinct isotopic signatures compared to lentic systems. The dominance of diffusive emissions to the total CH4 efflux appears to be characteristic of small streams. Still, the presence of methanogens and methanotrophs highlight the active production and oxidation of CH4 within stream ecosystems. While oxidation of dissolved CH4 is limited by the relatively rapid transport of dissolved CH4 out of the ecosystem, the dominance of diffusive emissions to the overall CH4 emissions budget ensures the isotopic signature of emitted CH4 is enriched relative to lentic waterbodies but similar to larger rivers. Consideration of a distinct lotic CH4 isotopic signature may be critical for accurately incorporating streams and rivers in regional and global atmospheric mixing CH4 models. Our proposed conceptual model provides a framework on which to further examine CH4 production and emissions from streams and how the relative importance of each process varies as a function of stream characteristics. At a global scale, concurrent examination of CH4 production, oxidation, isotopic values, emissions, and associated microbial communities is needed to determine the generality of our observed patterns in streams. The resultant mechanistic perspectives are required to robustly understand the contribution of lotic ecosystems to the global CH4 cycle.
Data Availability Statement
The datasets presented in this study can be found in online repositories. The names of the repository/repositories and accession number(s) can be found below: genetic data: https://www.ncbi.nlm.nih.gov/, PRJNA757371. All other data: https://pie-lter.ecosystems.mbl.edu/data
Author Contributions
All authors contributed significantly to this manuscript. AR, WW, AC, JM, RV, and JE designed the project. AR, AC, and PC led field sampling and laboratory analyses. CP and RV led isotopic analyses. AC, JM, and JE led microbial laboratory preparation. AR, CP, and AC led data analyses. AR led manuscript development with input from all authors.
Funding
Funding for this project comes from the Plum Island Ecosystems LTER NSF Award OCE-1637630, the National Aeronautics and Space Administration Interdisciplinary Science award NNX17AK10G, and the University of New Hampshire College of Life Sciences and Agriculture’s Paine Fund. Partial funding was provided by the New Hampshire Agricultural Experiment Station. This is Scientific Contribution Number 2923. This work was supported by the USDA National Institute of Food and Agriculture Hatch Projects NH00659 and NH00667.
Conflict of Interest
The authors declare that the research was conducted in the absence of any commercial or financial relationships that could be construed as a potential conflict of interest.
Publisher’s Note
All claims expressed in this article are solely those of the authors and do not necessarily represent those of their affiliated organizations, or those of the publisher, the editors, and the reviewers. Any product that may be evaluated in this article, or claim that may be made by its manufacturer, is not guaranteed or endorsed by the publisher.
Acknowledgments
We thank the members of the Water Systems Analysis Group and the Trace Gas Biogeochemistry Laboratory at the University of New Hampshire for their assistance in project and manuscript development. We thank Eliza Balch, Sarah Bower, and Christopher Whitney for their support in field work, and Apryl Perry for her assistance in laboratory analyses. We also thank Dr. Joanne Shorter from Aerodyne Research Inc. for access to the dual tunable infrared laser direct absorption spectrometer (TILDAS).
Supplementary Material
The Supplementary Material for this article can be found online at: https://www.frontiersin.org/articles/10.3389/fenvs.2021.791305/full#supplementary-material
Supplementary Figure S1 | Comparison of measured and estimated gas exchange rates (k600) in the four streams. Gas exchange is measured directly by addition of Argon as a conservative gas tracer. Gas exchange is estimated using Equations 3 and 4 as described in the text. The 1:1 line is displayed as the dashed line.
Supplementary Table S1 | Mean relative abundance (%) of methanogen and methanotroph archaeal and bacterial orders in sediments of the four streams as classified using the SILVA database. Samples are from the surface sediments (0-2 cm depth) or subsurface (9-11 cm depth). nd, no detection. In the order Methanosarcinales the Methanosarcinaceae and Methanosaetaceae families are is classified as methanogens, and the Methanoperedenaceae family are classified as methanotrophs.
Supplementary Table S2 | Rates of diffusive and total CH4 emissions from a variety of aquatic ecosystems, and δ13C-CH4 and δD-CH4 values of emitted CH4. ^Emitted isotope values are inferred from source signatures and both diffusive and ebullitive emission pathway balances.
References
Apprill, A., Mcnally, S., Parsons, R., and Weber, L. (2015). Minor Revision to V4 Region SSU rRNA 806R Gene Primer Greatly Increases Detection of SAR11 Bacterioplankton. Aquat. Microb. Ecol. 75, 129–137. doi:10.3354/ame01753
Atkins, M. L., Santos, I. R., and Maher, D. T. (2017). Seasonal Exports and Drivers of Dissolved Inorganic and Organic Carbon, Carbon Dioxide, Methane and δ13C Signatures in a Subtropical River Network. Sci. Total Environ. 575, 545–563. doi:10.1016/j.scitotenv.2016.09.020
Bastviken, D., Cole, J. J., Pace, M. L., and Van de Bogert, M. C. (2008). Fates of Methane from Different lake Habitats: Connecting Whole-lake Budgets and CH4emissions. J. Geophys. Res. 113, 1–13. doi:10.1029/2007JG000608
Bastviken, D., Ejlertsson, J., and Tranvik, L. (2002). Measurement of Methane Oxidation in Lakes: A Comparison of Methods. Environ. Sci. Technol. 36, 3354–3361. doi:10.1021/es010311p
Battin, T. J., Kaplan, L. A., Findlay, S., Hopkinson, C. S., Marti, E., Packman, A. I., et al. (2008). Biophysical Controls on Organic Carbon Fluxes in Fluvial Networks. Nat. Geosci 1, 95–100. doi:10.1038/ngeo101
Bishop, K., Buffam, I., Erlandsson, M., Fölster, J., Laudon, H., Seibert, J., et al. (2008). Aqua Incognita: the Unknown Headwaters. Hydrol. Process. 22, 1239–1242. doi:10.1002/hyp.7049
Boano, F., Harvey, J. W., Marion, A., Packman, A. I., Revelli, R., Ridolfi, R., et al. (2014). Hyporheic Flow and Transport Processes: Mechanisms, Models, and Bioghemical Implications. Rev. Geophys. 52, 1–77. doi:10.1002/2012RG000417.Received
Bodmer, P., Wilkinson, J., and Lorke, A. (2020). Sediment Properties Drive Spatial Variability of Potential Methane Production and Oxidation in Small Streams. J. Geophys. Res. Biogeosci. 125, 1–15. doi:10.1029/2019JG005213
Bolyen, E., Rideout, J. R., Dillon, M. R., Bokulich, N. A., Abnet, C., Al-Ghalith, G. A., et al. (2018). QIIME 2: reproducible, interactive, scalable, and extensible microbiome data science. PeerJ 6, e27295v2–354.
Brownlow, R., Lowry, D., Fisher, R. E., France, J. L., Lanoisellé, M., White, B., et al. (2017). Isotopic Ratios of Tropical Methane Emissions by Atmospheric Measurement. Glob. Biogeochem. Cycles 31, 1408–1419. doi:10.1002/2017GB005689
Butman, D., Striegl, R. G., Stackpoole, S. M., Del Giorgio, P. A., Prairie, Y. T., Pilcher, D. J., et al. (2018). Chapter 14: Inland Waters, in Second State of the Carbon Cycle Report (SOCCR2): A Sustained Assessment Report. Washington D. C: U.S. Global Change Research Program. doi:10.7930/SOCCR2.2018.Ch14
Callahan, B. J., McMurdie, P. J., Rosen, M. J., Han, A. W., Johnson, A. J. A., and Holmes, S. P. (2016). DADA2: High-Resolution Sample Inference from Illumina Amplicon Data. Nat. Methods 13, 581–583. doi:10.1038/nmeth.3869
Chanton, J., Chaser, L., Glasser, P., and Siegel, D. (2005). “Carbon and Hydrogen Isotopic Effects in Microbial, Methane from Terrestrial Environments,” in Stable Isotopes and Biosphere - Atmosphere Interactions (San Diego, CA, USA: Elsevier Inc.), 85–105. doi:10.1016/B978-012088447-6/50006-4
Chanton, J. P., Fields, D., and Hines, M. E. (2006). Controls on the Hydrogen Isotopic Composition of Biogenic Methane from High-Latitude Terrestrial Wetlands. J. Geophys. Res. 111, 1–9. doi:10.1029/2005JG000134
Chanton, J. P., Martens, C. S., and Kelley, C. A. (1989). Gas Transport from Methane-Saturated, Tidal Freshwater and Wetland Sediments. Limnol. Oceanogr. 34, 807–819. doi:10.4319/lo.1989.34.5.0807
Chanton, J. P. (2005). The Effect of Gas Transport on the Isotope Signature of Methane in Wetlands. Org. Geochem. 36, 753–768. doi:10.1016/j.orggeochem.2004.10.007
Chowdhury, T. R., and Dick, R. P. (2013). Ecology of Aerobic Methanotrophs in Controlling Methane Fluxes from Wetlands. Appl. Soil Ecol. 65, 8–22. doi:10.1016/j.apsoil.2012.12.014
Conrad, R. (2005). Quantification of Methanogenic Pathways Using Stable Carbon Isotopic Signatures: A Review and a Proposal. Org. Geochem. 36, 739–752. doi:10.1016/j.orggeochem.2004.09.006
Conrad, R. (2009). The Global Methane Cycle: Recent Advances in Understanding the Microbial Processes Involved. Environ. Microbiol. Rep. 1, 285–292. doi:10.1111/j.1758-2229.2009.00038.x
Crawford, J. T., Stanley, E. H., Spawn, S. A., Finlay, J. C., Loken, L. C., and Striegl, R. G. (2014). Ebullitive Methane Emissions from Oxygenated Wetland Streams. Glob. Change Biol. 20, 3408–3422. doi:10.1111/gcb.12614
Crawford, J. T., Striegl, R. G., Wickland, K. P., Dornblaser, M. M., and Stanley, E. H. (2013). Emissions of Carbon Dioxide and Methane from a Headwater Stream Network of interior Alaska. J. Geophys. Res. Biogeosci. 118, 482–494. doi:10.1002/jgrg.20034
Dean, J. F., Middelburg, J. J., Röckmann, T., Aerts, R., Blauw, L. G., Egger, M., et al. (2018). Methane Feedbacks to the Global Climate System in a Warmer World. Rev. Geophys. 56, 207–250. doi:10.1002/2017RG000559
Doherty, S. J., Barbato, R. A., Grandy, A. S., Thomas, W. K., Monteux, S., Dorrepaal, E., et al. (2020). The Transition from Stochastic to Deterministic Bacterial Community Assembly during Permafrost Thaw Succession. Front. Microbiol. 11, 2923. doi:10.3389/fmicb.2020.596589
Earth System Research Laboratory, G. M. D. (2021). Carbon Cycle Gases Offshore Portsmouth, New Hampshire (Isles of Shoals), United States. Available at: https://gml.noaa.gov/dv/iadv/index.php?code=nha (Accessed July 1, 2021).
Ettwig, K. F., Zhu, B., Speth, D., Keltjens, J. T., Jetten, M. S. M., and Kartal, B. (2016). Archaea Catalyze Iron-dependent Anaerobic Oxidation of Methane. Proc. Natl. Acad. Sci. USA 113, 12792–12796. doi:10.1073/pnas.1609534113
Evans, P. N., Boyd, J. A., Leu, A. O., Woodcroft, B. J., Parks, D. H., Hugenholtz, P., et al. (2019). An Evolving View of Methane Metabolism in the Archaea. Nat. Rev. Microbiol. 17, 219–232. doi:10.1038/s41579-018-0136-7
Fisher, R. E., France, J. L., Lowry, D., Lanoisellé, M., Brownlow, R., Pyle, J. A., et al. (2017). Measurement of the 13 C Isotopic Signature of Methane Emissions from Northern European Wetlands. Glob. Biogeochem. Cycles 31, 605–623. doi:10.1002/2016GB005504
Frolking, S., and Crill, P. (1994). Climate Controls on Temporal Variability of Methane Flux from a Poor Fen in southeastern New Hampshire: Measurement and Modeling. Glob. Biogeochem. Cycles 8, 385–397. doi:10.1029/94gb01839
Ganesan, A. L., Stell, A. C., Gedney, N., Comyn‐Platt, E., Hayman, G., Rigby, M., et al. (2018). Spatially Resolved Isotopic Source Signatures of Wetland Methane Emissions. Geophys. Res. Lett. 45, 3737–3745. doi:10.1002/2018GL077536
Glöckner, F. O., Yilmaz, P., Quast, C., Gerken, J., Beccati, A., Ciuprina, A., et al. (2017). 25 Years of Serving the Community with Ribosomal RNA Gene Reference Databases and Tools. J. Biotechnol. 261, 169–176. doi:10.1016/j.jbiotec.2017.06.1198
Hall, R. O., and Ulseth, A. J. (2020). Gas Exchange in Streams and Rivers. WIREs Water 7, 1–19. doi:10.1002/wat2.1391
Hampton, T. B., Zarnetske, J. P., Briggs, M. A., MahmoodPoor Dehkordy, F., Singha, K., Day-Lewis, F. D., et al. (2020). Experimental Shifts of Hydrologic Residence Time in a sandy Urban Stream Sediment-Water Interface Alter Nitrate Removal and Nitrous Oxide Fluxes. Biogeochemistry 149, 195–219. doi:10.1007/s10533-020-00674-7
Herreid, A. M., Wymore, A. S., Varner, R. K., Potter, J. D., and McDowell, W. H. (2020). Divergent Controls on Stream Greenhouse Gas Concentrations across a Land-Use Gradient. Ecosystems 24, 1299–1316. doi:10.1007/s10021-020-00584-7
Hojo, T., and Pearson, K. (1931). Distribution of the Median, Quartiles and Interquartile Distance in Samples from a Normal Population. Biometrika 23, 315–363. doi:10.2307/2332422
Holgerson, M. A., and Raymond, P. A. (2016). Large Contribution to Inland Water CO2 and CH4 Emissions from Very Small Ponds. Nat. Geosci 9, 222–226. doi:10.1038/ngeo2654
Hotchkiss, E. R., Hall Jr, R. O., Sponseller, R. A., Butman, D., Klaminder, J., Laudon, H., et al. (2015). Sources of and Processes Controlling CO2 Emissions Change with the Size of Streams and Rivers. Nat. Geosci 8, 696–699. doi:10.1038/ngeo2507
Hough, M., McClure, A., Bolduc, B., Dorrepaal, E., Saleska, S., Klepac-Ceraj, V., et al. (2020). Biotic and Environmental Drivers of Plant Microbiomes across a Permafrost Thaw Gradient. Front. Microbiol. 11, 1–18. doi:10.3389/fmicb.2020.00796
Knighton, D. (1998). Fluvial Forms and Processes: A New Perspective. First. New York, New York: Hodder Arnold.
Knox, M., Quay, P. D., and Wilbur, D. (1992). Kinetic Isotopic Fractionation during Air-Water Gas Transfer of O2, N2, CH4, and H2. J. Geophys. Res. 97, 20335. doi:10.1029/92jc00949
Leu, A. O., Cai, C., McIlroy, S. J., Southam, G., Orphan, V. J., Yuan, Z., et al. (2020). Anaerobic Methane Oxidation Coupled to Manganese Reduction by Members of the Methanoperedenaceae. ISME J. 14, 1030–1041. doi:10.1038/s41396-020-0590-x
Liu, L., Wilkinson, J., Koca, K., Buchmann, C., and Lorke, A. (2016). The Role of Sediment Structure in Gas Bubble Storage and Release. J. Geophys. Res. Biogeosci. 121, 1992–2005. doi:10.1002/2016JG003456
Looman, A., Maher, D. T., and Santos, I. R. (2021). Carbon Dioxide Hydrodynamics along a wetland-lake-stream-waterfall Continuum (Blue Mountains, Australia). Sci. Total Environ. 777, 146124. doi:10.1016/j.scitotenv.2021.146124
Magen, C., Lapham, L. L., Pohlman, J. W., Marshall, K., Bosman, S., Casso, M., et al. (2014). A Simple Headspace Equilibration Method for Measuring Dissolved Methane. Limnol. Oceanogr. 12, 637–650. doi:10.4319/lom.2014.12.637
MahmoodPoor Dehkordy, F., Briggs, M. A., Day-Lewis, F. D., Singha, K., Krajnovich, A., Hampton, T. B., et al. (2019). Multi-scale Preferential Flow Processes in an Urban Streambed under Variable Hydraulic Conditions. J. Hydrol. 573, 168–179. doi:10.1016/j.jhydrol.2019.03.022
Martin, M. (2011). Cutadapt Removes Adapter Sequences from High-Throughput Sequencing Reads. EMBnet j. 17, 10–2809. doi:10.14806/ej.17.1.200
Martiny, J. B. H., Jones, S. E., Lennon, J. T., and Martiny, A. C. (2015). Microbiomes in Light of Traits: A Phylogenetic Perspective. Science 350, 350. doi:10.1126/science.aac9323
Marx, A., Dusek, J., Jankovec, J., Sanda, M., Vogel, T., van Geldern, R., et al. (2017). A Review of CO2and Associated Carbon Dynamics in Headwater Streams: A Global Perspective. Rev. Geophys. 55, 560–585. doi:10.1002/2016RG000547
Mcmanus, J. B., Zahniser, M. S., and Nelson, D. D. (2011). Dual Quantum cascade Laser Trace Gas Instrument with Astigmatic Herriott Cell at High Pass Number. Appl. Opt. 50, A74–A85. doi:10.1364/AO.50.000A74
McManus, J. B., Zahniser, M. S., Nelson, D. D., Shorter, J. H., Herndon, S. C., Jervis, D., et al. (2015). Recent Progress in Laser-Based Trace Gas Instruments: Performance and Noise Analysis. Appl. Phys. B 119, 203–218. doi:10.1007/s00340-015-6033-0
Morse, N. B., and Wollheim, W. M. (2014). Climate Variability Masks the Impacts of Land Use Change on Nutrient export in a Suburbanizing Watershed. Biogeochemistry 121, 45–59. doi:10.1007/s10533-014-9998-6
Nelson, A. R., Sawyer, A. H., Gabor, R. S., Saup, C. M., Bryant, S. R., Harris, K. D., et al. (2019). Heterogeneity in Hyporheic Flow, Pore Water Chemistry, and Microbial Community Composition in an alpine Streambed. J. Geophys. Res. Biogeosci. 124, 3465–3478. doi:10.1029/2019JG005226
Oliver, J. D. (2005). The Viable but Nonculturable State in Bacteria. J. Microbiol. 43 Spec No, 93–100.
Packman, A. I., and Salehin, M. (2003). Relative Roles of Stream Flow and Sedimentary Conditions in Controlling Hyporheic Exchange. Hydrobiologia 494, 291–297. doi:10.1023/A:1025403424063
Parada, A. E., Needham, D. M., and Fuhrman, J. A. (2016). Every Base Matters: Assessing Small Subunit rRNA Primers for marine Microbiomes with Mock Communities, Time Series and Global Field Samples. Environ. Microbiol. 18, 1403–1414. doi:10.1111/1462-2920.13023
Quast, C., Pruesse, E., Yilmaz, P., Gerken, J., Schweer, T., Yarza, P., et al. (2013). The SILVA Ribosomal RNA Gene Database Project: Improved Data Processing and Web-Based Tools. Nucleic Acids Res. 41, D590–D596. doi:10.1093/nar/gks1219
Raymond, P. A., Zappa, C. J., Butman, D., Bott, T. L., Potter, J., Mulholland, P., et al. (2012). Scaling the Gas Transfer Velocity and Hydraulic Geometry in Streams and Small Rivers. Limnol. Oceanogr. 2, 41–53. doi:10.1215/21573689-1597669
Ries, K. G., Newson, J. K., Smith, M. J., Guthrie, J. D., Steeves, P. A., Haluska, T. L., et al. (2017). StreamStats, Version 4. In U.S. Geological Survey Fact Sheet 2017–3046. doi:10.3133/fs20173046
Robison, A. L., Wollheim, W. M., Turek, B., Bova, C., Snay, C., and Varner, R. K. (2021). Spatial and Temporal Heterogeneity of Methane Ebullition in lowland Headwater Streams and the Impact on Sampling Design. Limnol. Oceanogr. 66, 4063–4076. doi:10.1002/lno.11943
Rosentreter, J. A., Borges, A. V., Deemer, B. R., Holgerson, M. A., Liu, S., Song, C., et al. (2021). Half of Global Methane Emissions Come from Highly Variable Aquatic Ecosystem Sources. Nat. Geosci. 14, 225–230. doi:10.1038/s41561-021-00715-2
Sanches, L. F., Guenet, B., Marinho, C. C., Barros, N., and de Assis Esteves, F. (2019). Global Regulation of Methane Emission from Natural Lakes. Sci. Rep. 9, 1–10. doi:10.1038/s41598-018-36519-5
Sanders, I. A., Heppell, C. M., Cotton, J. A., Wharton, G., Hildrew, A. G., Flowers, E. J., et al. (2007). Emission of Methane from Chalk Streams Has Potential Implications for Agricultural Practices. Freshw. Biol 52, 1176–1186. doi:10.1111/j.1365-2427.2007.01745.x
Saunois, M., Stavert, A., Poulter, B., Bousquet, P., Canadell, J., Jackson, R., et al. (2020). The Global Methane Budget 2000–2017. Earth Syst. Sci. Data 12, 1561–1623. doi:10.5194/essd-12-1561-2020
Sawakuchi, H. O., Bastviken, D., Enrich-prast, A., Ward, N. D., Camargo, P. B., and Richey, J. E. (2021). Low Diffusive Methane Emissions from the Main Channel of a Large Amazonian Run-Of-The-River Reservoir Attributed to High Methane Oxidation. Front. Environ. Sci. 9, 1–13. doi:10.3389/fenvs.2021.655455
Sawakuchi, H. O., Bastviken, D., Sawakuchi, A. O., Krusche, A. V., Ballester, M. V. R., and Richey, J. E. (2014). Methane Emissions from Amazonian Rivers and Their Contribution to the Global Methane Budget. Glob. Change Biol. 20, 2829–2840. doi:10.1111/gcb.12646
Sawakuchi, H. O., Bastviken, D., Sawakuchi, A. O., Ward, N. D., Borges, C. D., Tsai, S. M., et al. (2016). Oxidative Mitigation of Aquatic Methane Emissions in Large Amazonian Rivers. Glob. Change Biol. 22, 1075–1085. doi:10.1111/gcb.13169
Schwietzke, S., Sherwood, O. A., Bruhwiler, L. M. P., Miller, J. B., Etiope, G., Dlugokencky, E. J., et al. (2016). Upward Revision of Global Fossil Fuel Methane Emissions Based on Isotope Database. Nature 538, 88–91. doi:10.1038/nature19797
Shelley, F., Abdullahi, F., Grey, J., and Trimmer, M. (2015). Microbial Methane Cycling in the Bed of a Chalk River: Oxidation Has the Potential to Match Methanogenesis Enhanced by Warming. Freshw. Biol. 60, 150–160. doi:10.1111/fwb.12480
Shelley, F., Ings, N., Hildrew, A. G., Trimmer, M., and Grey, J. (2017). Bringing Methanotrophy in Rivers Out of the Shadows. Limnol. Oceanogr. 62, 2345–2359. doi:10.1002/lno.10569
Smith, G. J., and Wrighton, K. C. (2019). Metagenomic Approaches Unearth Methanotroph Phylogenetic and Metabolic Diversity. Curr. Issues Mol. Biol. 33, 57–84. doi:10.21775/cimb.033.057
Sobczak, W. V., and Findlay, S. (2002). Variation in Bioavailability of Dissolved Organic Carbon Among Stream Hyporheic Flowpaths. Ecology 83, 3194–3209. doi:10.1890/0012-9658(2002)083[3194:vibodo]2.0.co;2
Stanley, E. H., Casson, N. J., Christel, S. T., Crawford, J. T., Loken, L. C., and Oliver, S. K. (2016). The Ecology of Methane in Streams and Rivers: Patterns, Controls, and Global Significance. Ecol. Monogr. 86, 146–171. doi:10.1890/15-1027.1
Trimmer, M., Maanoja, S., Hildrew, A. G., Pretty, J. L., and Grey, J. (2010). Potential Carbon Fixation via Methane Oxidation in Well-Oxygenated River Bed Gravels. Limnol. Oceanogr. 55, 560–568. doi:10.4319/lo.2009.55.2.056010.4319/lo.2010.55.2.0560
Turner, A. J., Frankenberg, C., and Kort, E. A. (2019). Interpreting Contemporary Trends in Atmospheric Methane. Proc. Natl. Acad. Sci. USA 116, 2805–2813. doi:10.1073/pnas.1814297116
Tyler, S. C., Bilek, R. S., Sass, R. L., and Fisher, F. M. (1997). Methane Oxidation and Pathways of Production in a Texas Paddy Field Deduced from Measurements of Flux, δl3C, and δD of CH4. Glob. Biogeochem. Cycles 11, 323–348. doi:10.1029/97gb01624
Walters, W., Hyde, E. R., Berg-Lyons, D., Ackermann, G., Humphrey, G., Parada, A., et al. (2016). Improved Bacterial 16S rRNA Gene (V4 and V4-5) and Fungal Internal Transcribed Spacer Marker Gene Primers for Microbial Community Surveys. mSystems 1, 1–10. doi:10.1128/msystems.00009-15
Wang, D. T., Welander, P. V., and Ono, S. (2016). Fractionation of the Methane Isotopologues 13CH4, 12CH3D, and 13CH3D during Aerobic Oxidation of Methane by Methylococcus Capsulatus (Bath). Geochimica et Cosmochimica Acta 192, 186–202. doi:10.1016/j.gca.2016.07.031
Wang, G., Xia, X., Liu, S., Zhang, L., Zhang, S., Wang, J., et al. (2021). Intense Methane Ebullition from Urban Inland Waters and its Significant Contribution to Greenhouse Gas Emissions. Water Res. 189, 116654. doi:10.1016/j.watres.2020.116654
Whiticar, M. J. (1999). Carbon and Hydrogen Isotope Systematics of Bacterial Formation and Oxidation of Methane. Chem. Geology. 161, 291–314. doi:10.1016/S0009-2541(99)00092-3
Wik, M., Johnson, J. E., Crill, P. M., DeStasio, J. P., Erickson, L., Halloran, M. J., et al. (2018). Sediment Characteristics and Methane Ebullition in Three Subarctic Lakes. J. Geophys. Res. Biogeosci. 123, 2399–2411. doi:10.1029/2017JG004298
Wik, M., Thornton, B. F., Bastviken, D., Uhlbäck, J., and Crill, P. M. (2016a). Biased Sampling of Methane Release from Northern Lakes: A Problem for Extrapolation. Geophys. Res. Lett. 43, 1256–1262. doi:10.1002/2015GL066501.Received
Wik, M., Thornton, B. F., Varner, R. K., McCalley, C., and Crill, P. M. (2020). Stable Methane Isotopologues from Northern Lakes Suggest that Ebullition Is Dominated by Sub‐Lake Scale Processes. J. Geophys. Res. Biogeosci. 125, 1–13. doi:10.1029/2019JG005601
Wik, M., Varner, R. K., Anthony, K. W., MacIntyre, S., and Bastviken, D. (2016b). Climate-sensitive Northern Lakes and Ponds Are Critical Components of Methane Release. Nat. Geosci 9, 99–105. doi:10.1038/ngeo2578
Wollheim, W. M., Mulukutla, G. K., Cook, C., and Carey, R. O. (2017). Aquatic Nitrate Retention at River Network Scales across Flow Conditions Determined Using Nested In Situ Sensors. Water Resour. Res. 53, 9740–9756. doi:10.1002/2017WR020644
Wollheim, W. M., Pellerin, B. A., Vörösmarty, C. J., and Hopkinson, C. S. (2005). N Retention in Urbanizing Headwater Catchments. Ecosystems 8, 871–884. doi:10.1007/s10021-005-0178-3
Wollheim, W. M., Stewart, R. J., Aiken, G. R., Butler, K. D., Morse, N. B., and Salisbury, J. (2015). Removal of Terrestrial DOC in Aquatic Ecosystems of a Temperate River Network. Geophys. Res. Lett. 42, 6671–6679. doi:10.1002/2015GL064647
Wu, M. L., Ettwig, K. F., Jetten, M. S. M., Strous, M., Keltjens, J. T., and Niftrik, L. v. (2011). A New Intra-aerobic Metabolism in the Nitrite-dependent Anaerobic Methane-Oxidizing Bacterium Candidatus 'Methylomirabilis Oxyfera'. Biochem. Soc. Trans. 39, 243–248. doi:10.1042/BST0390243
Zhang, G., Yu, H., Fan, X., Ma, J., and Xu, H. (2016). Carbon Isotope Fractionation Reveals Distinct Process of CH4 Emission from Different Compartments of Paddy Ecosystem. Sci. Rep. 6, 1–10. doi:10.1038/srep27065
Keywords: methane, streams, isotopes, methanogens, methane oxidation, emissions
Citation: Robison AL, Wollheim WM, Perryman CR, Cotter AR, Mackay JE, Varner RK, Clarizia P and Ernakovich JG (2022) Dominance of Diffusive Methane Emissions From Lowland Headwater Streams Promotes Oxidation and Isotopic Enrichment. Front. Environ. Sci. 9:791305. doi: 10.3389/fenvs.2021.791305
Received: 08 October 2021; Accepted: 30 December 2021;
Published: 31 January 2022.
Edited by:
Daniel F. McGinnis, Université de Genève, SwitzerlandReviewed by:
Mark Alexander Lever, ETH Zürich, SwitzerlandChanghui Wang, Shanxi Agricultural University, China
Copyright © 2022 Robison, Wollheim, Perryman, Cotter, Mackay, Varner, Clarizia and Ernakovich. This is an open-access article distributed under the terms of the Creative Commons Attribution License (CC BY). The use, distribution or reproduction in other forums is permitted, provided the original author(s) and the copyright owner(s) are credited and that the original publication in this journal is cited, in accordance with accepted academic practice. No use, distribution or reproduction is permitted which does not comply with these terms.
*Correspondence: Andrew L. Robison, YW5kcmV3LnJvYmlzb25AZXBmbC5jaA==