- 1The Alliance of Bioversity International and International Center for Tropical Agriculture (CIAT), Cali, Colombia
- 2Ludwig-Maximilians-Universität München, Munich, Germany
- 3University of Copenhagen, Copenhagen, Denmark
Using Colombia as a case study, this analysis provides insights on deforestation dynamics in times of conflict and peace and the different factors driving these dynamics. We performed time series clustering of yearly deforestation data (2001–2018) from 708 out of 1,122 mainland Colombian municipalities (accounting for 98% of the total deforestation areas in Colombia) and produced regression models using a gradient tree boosting framework (XGBoost) to identify drivers that explain varying, local-level deforestation dynamics. Municipalities were characterized by seven categories of deforestation dynamics, with the Amazon region being largely represented by only four categories and the Andes region displaying all categories of deforestation dynamics. Notably, six of the seven representative categories exhibit substantial increases in deforestation in the years following the peace agreement. The regression analysis revealed that coca cultivation area, number of cattle, and municipality area are the top three drivers of deforestation dynamics at national, regional, and category levels. However, the importance of the different variables varied according to the different spatial dimensions. Results provide further understanding on how the drivers of deforestation change not only at a regional scale, as assumed by much of the current literature about drivers of deforestation, but also at a lower scale of analysis (intraregional and intradepartmental variation in the case of Colombia). Insights from this study can be used to understand deforestation dynamics in other countries experiencing times of conflict and peace and will support decision-makers in creating programs that align actions for peacebuilding, climate change mitigation, and biodiversity conservation more effectively.
1 Introduction
Anthropogenic activities are leading to global changes in biodiversity and climate (Ceballos et al., 2015; IPCC, 2019; 2021). The paramount significance of tropical forests in halting biodiversity loss and climate change is widely recognized; tropical forests represent a disproportionate amount of global biodiversity (IPBES, 2019), housing at least two-thirds of the world’s organisms (Raven, 1988). In addition, deforestation and forest degradation of tropical forests account for 0.5–3.5 GtC yr−1 of annual greenhouse gas emissions (IPCC, 2019) resulting from the depletion of vegetation and soil carbon stocks (Don et al., 2011; Baccini et al., 2017). Nonetheless, high deforestation rates in tropical regions persist (Curtis et al., 2018; Ganzenmüller et al., 2019). Concurrently, many tropical forest areas are marked by the presence of armed conflicts (De Jong et al., 2007; Landholm et al., 2019). In these regions, the interplay between conflict and peace shapes an important part of how deforestation trajectories unfold.
The links between tropical forests and armed conflicts are context-specific and non-uniform, resulting in varying deforestation dynamics (Baumann and Kuemmerle, 2016; Castro-Nunez et al., 2017a; Hanson, 2018). Three possible mechanisms are hypothesized in the environmental security literature to explain these links: 1) natural resource scarcity or unequal sharing (Homer-Dixon, 1994); 2) accessibility and competition (Peluso and Watts, 2001); and 3) hidden movements and actions of illegal armed groups in forested areas (Collier and Hoeffler, 2004). These context-specific mechanisms can take effect in tropical forests, which are often characterized by a weak state presence, widespread poverty, disputes over land rights, and an abundance of high-value natural resources available for financing armed groups (Collier and Hoeffler, 2004; Rustad et al., 2008; Rustad and Binningsbø, 2012). This, in turn, also influences how forest cover changes are associated with agricultural expansion, infrastructure development, extractive practices, and underlying demographic, economic, institutional, technological, and cultural drivers (Geist and Lambin, 2002).
Forest cover change in regions with armed conflicts is especially shaped by the strategic forest usage of the involved armed groups (Castro-Nunez et al., 2017b). Deforestation in conflict regions may be fostered through the exploitation of natural resources, such as timber and minerals (Sánchez-Cuervo and Aide, 2013), direct fighting actions (Dudley et al., 2002; Van et al., 2015), cultivation of illicit crops (Davalos et al., 2011), and the resettlement of displaced people (Ordway, 2015). In contrast, armed conflicts can reduce deforestation through forced migration (Sánchez-Cuervo and Aide, 2013; Burgess et al., 2015), disruptions in agriculture and other economic activities (Le Billon, 2000; Hecht et al., 2006; Burgess et al., 2015), and access restrictions that can take diverse forms such as landmines and infrastructure sabotage (Berhe, 2007).
In the aftermath of conflicts, pressures on forest cover changes are often exacerbated (Stevens et al., 2011; Negret et al., 2017; Suarez et al., 2018). Reasons for this pattern include the return of displaced people, an increased demand for agricultural land, and the expansion of economic sectors such as mining or logging (Baptiste et al., 2017; Suarez et al., 2018).
The intensification of deforestation drivers in post-conflict regions highlights the importance of better understanding the different uses of forests in countries emerging from armed conflicts and the political necessity of combining forest conservation policies and peacebuilding programs (Castro-Nunez, 2018; Prem et al., 2020). This suggests that to advance our understanding of how periods of conflict and peace influence forest cover, deforestation dynamics need to be linked with local-level characteristics. Evidence suggests that drivers of deforestation dynamics are highly context-specific at the local scale (Sánchez-Cuervo and Aide, 2013); therefore, the scale of analysis is an important consideration when studying what drives deforestation in countries emerging from armed conflicts. Several studies have been conducted at national and regional scales (e.g., Landholm et al., 2019; Negret et al., 2019; Prem et al., 2020); however, such studies do not fully illustrate the intraregional variation in deforestation dynamics across a country.
Despite numerous studies on deforestation in conflict and post-conflict settings, these insights have not yet been operationalized through spatially-explicit analyses. More specifically, most studies focus on describing the effects of conflict on forest cover and provide insights on conflict-related variables influencing such effects. However, they do not shed light on the diversity of local-level deforestation dynamics observed across conflict-affected countries and the combination of drivers that explain such dynamics.
To address this research gap, we computed time series clustering of yearly forest cover loss data and used gradient tree boosting to identify the combinations of drivers that explain varying, local-level deforestation dynamics in Colombia. We use Colombia as a case study because the nation has endured over 50 years of armed conflicts and has experienced various peace processes, which allow us to discuss deforestation dynamics in times of conflict and peace. Variables were selected based on dominant narratives in the academic literature reporting extensive livestock pastures and coca cultivation as major drivers of deforestation in Colombia, alongside infrastructure development, illegal logging, and mining (Alvarez, 2003; Armenteras et al., 2013; Sánchez-Cuervo and Aide, 2013; Sánchez-Cuervo and Aide, 2013; Castro-Nunez et al., 2017a; González et al., 2018; Landholm et al., 2019). Furthermore, various effects of the armed conflict on forest cover are documented, which we take into consideration by including the variables “conflict events” and “displaced people” in the analysis. Municipality area was also included in the analysis to factor in the large differences in the areas of Colombian municipalities under the assumption that the larger the municipality, the lower the institutional presence. Locally, drivers unfold differently and often influence each other. For example, low institutional presence may favor illegal activities such as coca cultivation, which in turn frequently serves as a precursor for land ownership claims manifested through the establishment of extensive pastures. The goal of this study is to untangle the different drivers of deforestation at various scales ranging from local to national and to determine their effects on deforestation dynamics in Colombia. The insights from this study will support decision-makers in creating programs that align actions for peacebuilding, climate change mitigation, and biodiversity conservation more effectively.
2 History of Deforestation and Conflict in Colombia
Colombia experienced over 50 years of armed conflicts that resulted in nearly 270,000 killed and over 7 million displaced people (RNI Red Nacional de Información, 2019). Decades of violence presented negative impacts on forest cover, with higher deforestation rates present in conflict-affected areas (Landholm et al., 2019). Several academic studies have documented the links between forests, deforestation, and conflict in Colombia (Alvarez, 2003; Davalos et al., 2011; Sánchez-Cuervo and Aide, 2013; Fergusson et al., 2014), and the growing awareness of these links led to the inclusion of environmental considerations in the last peace agreement with the Revolutionary Armed Forces of Colombia (FARC), which brought an end to the longstanding conflict (OACP Oficina del Alto Comisionado para la Paz, 2016). Various scholars have warned of increased pressures on Colombian forests following the peace agreement (Baptiste et al., 2017; Suarez et al., 2018; Eufemia et al., 2019). They argue that the peace agreement opened up areas that were previously controlled and protected by the FARC who had used these areas as hideouts. Subsequently, prevalent land-use conflicts and land grabbing practices, exacerbated by land tenure issues, were seen following the agreement (Murillo-Sandoval et al., 2020, Murillo-Sandoval et al., 2021). These dynamics are expanding the agricultural frontier and placing increased pressure on forests. In particular, land-use conflicts, which have vast and complex implications on forests, soils, and water bodies, are considered to threaten environmental objectives (Suarez et al., 2018). Land-use conflicts can be related to land tenure and grabbing, which in turn are closely linked with extensive cattle grazing when cattle are used to strengthen land ownership claims (Castro-Nunez et al., 2017a).
The origins of the conflict are rooted in the 1960s when several groups accused the Government of Colombia (GoC) of rural neglect and formed guerilla organizations to challenge the state and lead an armed rural insurgency. The most prominent armed group during this time of internal conflict was the FARC. By the late 1990s, armed actors of the FARC had taken control of many areas throughout the country where state presence was weak or absent. Counter to the FARC, right-wing paramilitaries arose in the 1980s in opposition to the guerrilla groups. In 1997, most of the paramilitary groups organized under an umbrella organization called the United Self Defense Forces of Colombia (AUC), which carried out direct military battles against the FARC (Beittel, 2015).
Attempts to negotiate peace with the FARC, paramilitary groups, and other illegal armed forces were made prior to the final peace agreement signed in 2016. Between 1998 and 2002, the Administration of President Andrés Pastrana established a large demilitarized zone approximately the size of Switzerland (∼42,000 square miles) within which negotiations with the FARC were to be held. Negotiations were abandoned, however, in 2002 as the FARC was seen as using the demilitarized zone to regroup and rearm its forces, fueling a continuation of FARC military activity (Beittel, 2015).
In 2003, President Álvaro Uribe signed a peace deal with the AUC, leading to the demobilization of over 31,000 AUC members, and implemented “Plan Patriota,” which commenced a new offensive against the guerrilla forces. This plan brought major advances against the FARC, as FARC ranks and territories were reduced and drug production equipment was seized, but was met with heavy criticism due to the violence that occurred and the large number of civilians displaced. The plan was further criticized for not implementing a strategy to maintain control of territories taken from the FARC and for failing to establish a permanent state presence in these areas. President Uribe continued advances against the FARC into his second term (2006–2010), further successfully weakening their forces (Beittel, 2015).
The final peace negotiations with the FARC that brought an eventual end to the armed conflict took place under the administration of President Juan Manuel Santos. Talks began in 2012 and a final agreement was reached in 2016 with the goal of building lasting peace in the country (Nilsson and González Marín, 2020). In 2017, the FARC officially ceased to be an armed group; however, in 2019, several former combatants called for a return to arms (BBC News, 2019). Today, despite the peace agreement, FARC dissidents and other armed groups are fighting over the control of previously FARC-controlled areas (Nilsson and González Marín, 2020).
3 Methods
3.1 Data Collection
We created a dataset containing information on the area of forest cover loss, our outcome variable, and potential drivers of forest cover loss at the municipality level for the period 2001–2018 (Table 1). Potential drivers were selected based on dominant narratives around the drivers of tropical deforestation in Colombia (Alvarez, 2003; Armenteras et al., 2013; Sánchez-Cuervo and Aide, 2013; Castro-Nunez et al., 2017a; Landholm et al., 2019).
Areas of annual forest cover loss in all 1,122 Colombian municipalities were calculated from Hansen et al. (2013), which define trees as all vegetation taller than 5 m and forest cover loss as a stand-replacement disturbance or a change from a forest to a non-forest state caused by anthropogenic or natural processes. Given that the vast majority of tree cover loss in Latin America is driven by direct anthropogenic modifications (Curtis et al., 2018), the dataset is thus used as a proxy for deforestation. However, we point out that the analyzed trends might include areas of forest cover loss from natural processes, such as forest fires for example.
Information on conflict events, defined as events of organized and lethal violence, were taken from Sundberg and Melander (2013) (UCDP Georeferenced Event Dataset version 19.1). The number of conflict events in each municipality was determined by adding up all georeferenced events within municipality borders in each year (Supplementary Figure S1). Since there is no dataset about the number of cattle at the municipality level covering the whole time period, data were obtained through two different sources. Data from the Federación Colombiana de Ganaderos were used for the years 2001–2011 (FEDEGAN, 2019), while data from the Instituto Colombiano Agropecuario were used for 2008–2018 (ICA Instituto Colombiano Agropecuario).1 For the overlapping years (2008–2011), the mean values of both data sources were taken. When plotting the data for each municipality, several data artifacts were identified. Thus, we applied the following correction procedure: when the number of cattle increased/decreased by more than 25% in 1 year and then decreased/increased by more than 25% in the next year, we adjusted the number of cattle by calculating the mean of the previous and subsequent year (Supplementary Figure S2). Further obvious data artifacts were corrected manually. Instituto Colombiano Agropecuario also provided us with data on the number of cattle farms in each municipality for the period 2008–2018 (ICA Instituto Colombiano Agropecuario)1. For the cattle farm dataset, the same error correction procedure described for the cattle datasets was applied. The area of each municipality was calculated based on a shapefile provided by the Departamento Administrativo Nacional de Estadística (DANE, 2018). Information on displaced people and coca cultivation area was taken from the Red Nacional de Información (RNI, 2019) and Sistema Integrado de Monitoreo de Cultivos Ilícitos/United Nations Office on Drugs and Crime (SIMCI/UNODOC, 2019), respectively.
3.2 Time Series Clustering
To identify typical dynamics of forest cover losses across Colombian municipalities, we conducted a time series clustering analysis. Time series clustering techniques are applied in scientific and non-scientific contexts to find time-specific patterns in large datasets consisting of numerous time series (Aghabozorgi et al., 2015). The goal of time series clustering is the unsupervised assembly of unlabeled time series into coherent and homogeneous groups (Rani and Sikka, 2012). Using this approach, we clustered municipalities into a number of categories determined by shared deforestation dynamics.
For the time series clustering analysis in this study, Colombian municipalities without significant forest cover loss were excluded. We separated municipalities with significant losses from municipalities without by considering only the municipalities that were responsible for 98% of the total forest cover losses nationally. Thereby, 414 municipalities with ≤27.08 ha of mean annual forest cover loss were excluded from the clustering analysis.
For the remaining 708 municipalities, trend values were calculated using the rolling mean with a rolling window of 3 years (Supplementary Figure S3). Thus, the trend value for 1 year is calculated as the mean of the forest cover loss values of the previous, actual, and subsequent years. As a consequence, there are no trend values for the years 2001 and 2018. However, the data from these years are indirectly incorporated in the analysis in the trend values of the years 2002 and 2017. Subsequently, to cluster the municipalities based on the direction of the trends rather than on absolute values, the trend data were normalized by computing the z-scores.
In time series clustering analysis, four components are central: 1) the distance measure, 2) the clustering algorithm, 3) the cluster evaluation, and 4) the prototype definition function (Aghabozorgi et al., 2015). We measured the distance between the trend time series by applying the Dynamic Time Warping (DTW) algorithm. DTW is a widely used technique, originally developed for automatic speech recognition, known for its high accuracy at the expense of computational costs (Müller, 2007; Wang et al., 2013). It is an elastic distance measure and thus has the advantage of dealing well with temporal drifts (Aghabozorgi et al., 2015). The window size was set to 1 year to allow minimum flexibility (Giorgino, 2009; Aghabozorgi et al., 2015). Hierarchical clustering was chosen as the clustering algorithm. The Silhouette index (Rousseeuw, 1987) and the Dunn index (Arbelaitz et al., 2013) served as evaluation criteria for the best number of clusters. Prototypes were defined using a shape-based distance algorithm (Paparrizos and Gravano, 2015).
3.3 Regression Analysis
The importance of potential drivers of forest cover loss was assessed by fitting multiple Extreme Gradient Boosting (XGBoost) models. XGBoost is a machine learning approach and belongs to the group of gradient tree boosting techniques introduced by Friedman (2001). In gradient tree boosting, a tree ensemble model is optimized stepwise to minimize the residuals of the current model (Chen and Guestrin, 2016; Nishio et al., 2018). In many cases, XGBoost outperforms other regression techniques as it uses a superior learning algorithm, is optimized for parallel, distributed and out-of-core computing, and prevents overfitting (Chen and Guestrin, 2016). For a detailed description of the XGBoost algorithm, see Chen and Guestrin (2016). We chose XGBoost over other regression techniques as it provides a time- and memory-efficient way to access non-linear dependencies (Chen and Guestrin, 2016).
For the analysis, we took forest cover loss area as the outcome variable and conflict events, displaced people, coca cultivation area, cattle, cattle farms, and municipality area as predictor variables. Cattle farms were included to reflect recent forest-conflict transition models, suggesting that redistribution of access to land starts just after the colonization and land grabbing processes are completed. Meanwhile, municipality area was included as a predictor variable to consider the large differences in the areas of Colombian municipalities under the assumption that the larger the municipality, the lower the institutional presence. XGBoost models were built for the whole country, each natural region, each municipality category, and each department. Since the departments “Archipielago de San Andres, Providencia y Santa Catalina,” “Bogota, D.C.,” “Guaviare,” and “Vichada” consist of fewer than 5 municipalities, not enough data points were available to build reasonable models. Therefore, these four departments were excluded.
Overall, the driver analysis was conducted for 42 different spatial units (whole country, 5 natural regions, 7 municipality categories, 29 departments). For each of these spatial units, five XGBoost models were selected from 100 models initialized with random parameter settings in a predefined parameter space. The selection of these models was done through a 10-fold adaptive resampling procedure using the Root Mean Square Error (RMSE) as evaluation criteria (Kuhn, 2014). The minimum number of resamples used before models were removed was set to 5 and the number of complete sets of folds to compute was set to 10. Consequently, 21,000 models overall were initialized and evaluated (42 spatial units × 5 rounds × 100 initial models). Results of each unit are presented as mean, minimum, and maximum R2 and variable importance values (Figure 2 and Supplementary Figure S4).
All data processing and analyses were done using R software (R Core Team, 2013).
3.4 Methodological Limitations
This study has a few data and methodological limitations that need to be considered. Firstly, the selected data on forest cover change (Hansen et al., 2013) does not distinguish between primary and secondary forests, e.g., the clearing of an oil palm plantation is classified as deforestation, and the classification of forest and non-forest may be erroneous in certain cases (Tropek et al., 2014). Furthermore, the raw data on forest cover change has not been processed uniformly, as an improved detection process was applied to the years following 2011 (University of Maryland, 2021). Nevertheless, this dataset is the best source available for covering yearly deforestation rates in recent decades. The effects of inaccuracies and misclassifications are limited by taking trend values, which should buffer these inconsistencies. Secondly, full transparency of the data aggregation for the other data sources is not documented. On the other hand, all data were collected from official institutions and are the best sources available. Thirdly, the settings and methods chosen for the time series clustering influence the number of clusters, the grouping of municipalities and the shape of the prototypes. We tried different settings and methods and found the results presented here to be the most reasonable.
4 Results
4.1 Deforestation Dynamics
The time series clustering of yearly deforestation data (2001–2018) from 708 out of 1,122 mainland Colombian municipalities, which account for 98% of the total deforestation areas in Colombia, resulted in seven categories of municipalities (Figure 1) reflecting distinct deforestation dynamics. Six of these categories (A, B, C, D, E, and G) exhibited a moderate to sharp increase in deforestation area during the last third of the evaluated time period (2013–2018). Interestingly, however, four of these six categories (A, D, E, and G) displayed a substantial decline in deforestation during the preceding second third of the period (2007–2012). Only three categories (E, F, and G) exhibited similar or lower forest cover loss at the end of the study period compared to the start of the period.
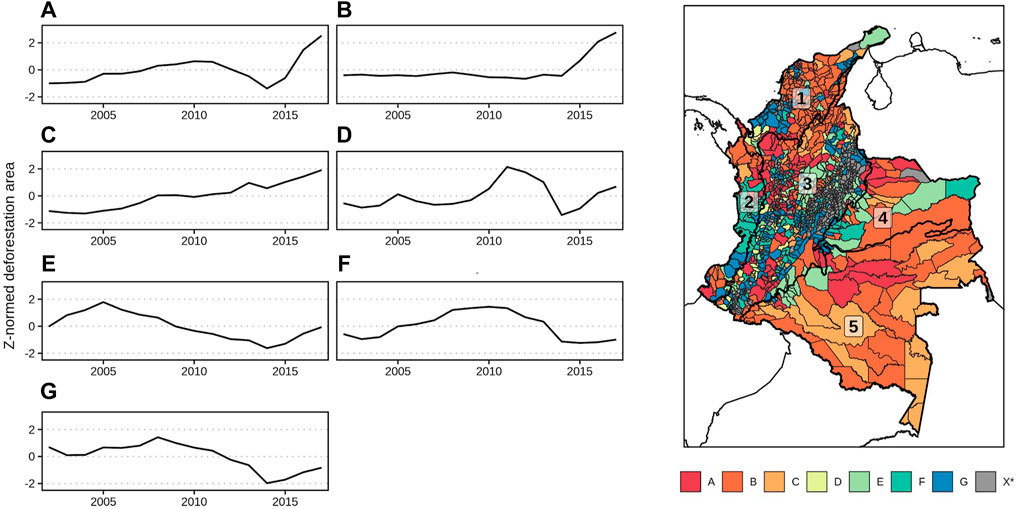
FIGURE 1. Categories and spatial distribution of 708 municipalities. Categories are based on trends in annual forest cover loss for the period 2001–2018. The grey-colored category indicates municipalities that did not have significant losses in forest cover over the time period. Natural regions: (1) Caribe, (2) Pacifico, (3) Andes, (4) Orinoquia, and (5) Amazon.
Category A (n = 129) showed a steady, moderate incline in deforestation that began to decline in the second third of the analysis period before transitioning to a sharp increase in deforestation rates in the last third of the period. Categories B (n = 176) and C (n = 56) were the only categories to not display a significant decline in deforestation rates during the analyzed period; however, category B had a stable rate of deforestation before spiking in the last third of the period, while category C saw an overall steady increase in deforestation rates throughout the period. Deforestation rates in category D (n = 43) fluctuated over time, peaking in the second third of the period before sharply declining and then spiking again in the last third of the period. Category E (n = 69) exhibited the longest period of declining deforestation rates with rates rising only in the first and last years of the analysis period. Category F (n = 74) was characterized by an inverse, U-shaped trend in deforestation rates that reached a low and stabilized in the last third of the period. Lastly, category G (n = 161) displayed a similar trend as category E but saw rates rise during the first half of the period.
4.2 Deforestation Dynamics in Natural Regions
Approximately 75% of the total deforestation accounted for in the study period occurred in the Amazon (38%) and Andes (37%) regions. The greatest percentage of total deforestation in the analyzed period was found in municipalities falling under categories B (34%), A (20%), and C (18%), which were the three most predominant categories in the Amazon region. Table 2 displays the number of municipalities, the area of land, and the percent of total deforestation under each category. It also shows how categories are distributed among the five Colombian natural regions.
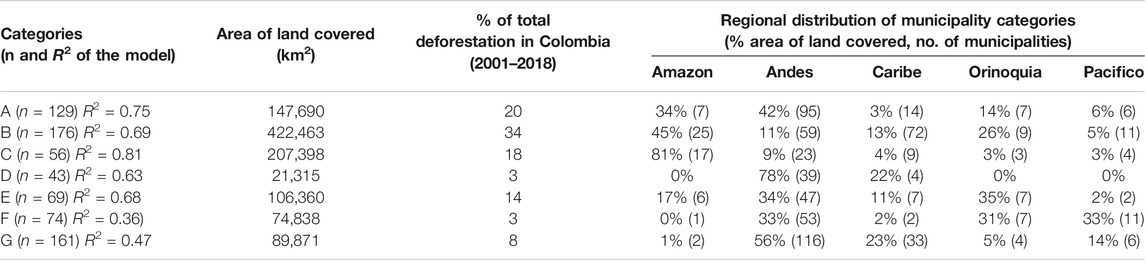
TABLE 2. Summary of categories produced by the time series clustering of yearly deforestation data of 708 municipalities. The following details are displayed for each category: 1) Number of municipalities and the R2 value of the time series clustering model; 2) Area of land covered; 3) Percent of total deforestation in Colombia for the analyzed period; and 4) Distribution of the municipalities among the five natural regions of Colombia with percent of the area covered followed by the number of municipalities in parentheses.
Municipalities in category B characterized the greatest percentage of deforestation, accounted for the greatest area of land, and were distributed across all regions but located predominantly in the Amazon. Categories C, A, and E accounted for the second, third, and fourth greatest areas of land, respectively. Category C was found mostly in the Amazon region, category A in the Amazon and Andes, and category E in the Andes and Orinoquia regions. This last category includes the demilitarized zone established between 1998 and 2002 that was considered as the stronghold of the FARC: La Macarena. Categories G, F, and D covered smaller areas and accounted for only 14% of deforestation. Category G was found mostly in the Andes and, to a lesser extent, Caribe; category F was distributed mostly among the Andes, Pacifico, and Orinoquia regions; and category D was found mostly in the Andes.
In the Amazon region, most municipalities exhibited patterns with increasing deforestation trends. This region was predominated by category B (43% of municipalities in the region), C (29%), and A (12%). The Caribe and Orinoquia regions were also predominated by category B (51 and 24% of municipalities in the regions, respectively), while the Pacifico region was dominated by categories B (26%) and F (26%). The most common dynamics exhibited in the Andes region were categories G (27%) and A (22%), which show relatively similar trends. In addition, most of the municipalities that were excluded from the analysis (n = 413) due to insignificant deforestation rates were located in the Andes region.
Andean municipalities were widely represented in each of the municipality categories. However, the area of municipalities in the Amazon region is substantially larger than the area in the Andes (Supplementary Figure S5). For example, although category C characterized only 8% of the municipalities, it covered the second-largest area compared to the other categories. Therefore, although the Andean region represents all identified patterns of deforestation, the four predominant deforestation patterns in the Amazon region cover a larger area.
4.3 Local Drivers of Deforestation Dynamics
Regression analyses with XGBoost models at the national, regional, and category level show the importance of different variables in influencing deforestation dynamics. At the national level, the combined influence of six variables (conflict events, displaced people, coca area, cattle, cattle farms, municipality area) created strong predictive models for explaining deforestation dynamics (mean R2 = 0.71). At the categorical level, strong predictive models were produced for category C (R2 = 0.81), followed by categories A (mean R2 = 0.75), B (mean R2 = 0.69), E (mean R2 = 0.68), and D (mean R2 = 0.63). Similarly, strong predictive models were built for the Amazon (mean R2 = 0.75), Orinoquia (mean R2 = 0.75), and Andes (mean R2 = 0.71) regions. In contrast, the regression models explained the least amount of variation for categories F (mean R2 = 0.36) and G (mean R2 = 0.47) as well as for the Caribe (mean R2 = 0.47) and Pacifico (mean R2 = 0.53) regions.
As shown in Figure 2, the number of cattle, coca area, and municipality area were the strongest predictor variables for deforestation dynamics at the national level. However, the importance of the predictor variables varied among the five biogeographic regions and the seven municipality categories. Cattle emerged as the top predictor variable for the Amazon and Pacifico regions, while coca area was an influential predictor for the Andes and Orinoquia regions. Likewise, municipality area, cattle, and coca area were the strongest predictor variables for categories A, B, and C, respectively. For categories A and E, municipality area and coca area emerged as the most important predictors. The two strongest predictor variables for category C were coca area and cattle, while for categories B and D cattle and municipality area were the better predictor variables.
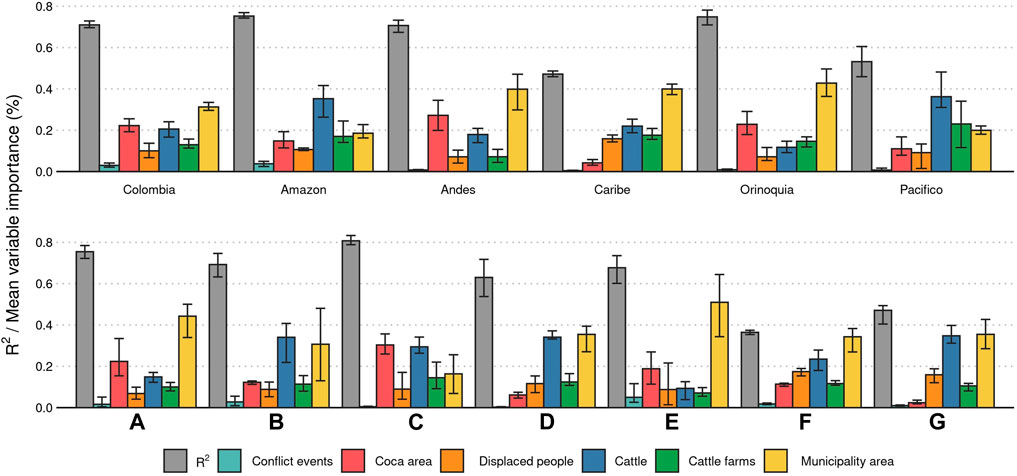
FIGURE 2. R2 and variable importance of Regression Analysis (XGBoost) for Colombia, natural regions, and municipality categories (mean, minimum, and maximum values of five runs with random initialization).
5 Discussion
The results of our analysis indicate that deforestation dynamics have fluctuated over time and differ markedly among and within Colombian natural regions and departments. In recent years, deforestation has increased throughout Colombia, which suggests an undesirable effect of the peace agreement between the GoC and the FARC on forest cover. Accordingly, our results support previous studies reporting that the peace agreement negatively affected deforestation in Colombia, even in places where deforestation was declining before the agreement (Landholm et al., 2019; Negret et al., 2019; Clerici et al., 2020; Murillo-Sandoval et al., 2020, Murillo-Sandoval et al., 2021; Prem et al., 2020). However, a deeper understanding of these effects requires consideration of the multiple dynamics that are influenced by interactions between forest cover and armed conflict variables (Castro-Nunez et al., 2017a). This idea was operationalized in this study resulting in seven categories of deforestation dynamics.
We find that deforestation dynamics change at regional, departmental, and municipal levels and that municipalities located in different regions can share similar deforestation dynamics. Similarly, the results of the municipality-level analysis demonstrate that the drivers of deforestation in conflict and post-conflict settings also vary among and within natural regions. This has been increasingly supported in the Colombian literature (e.g., Sánchez-Cuervo and Aide, 2013; González et al., 2018), highlighting that drivers of deforestation are strongly context-specific at the local scale. Studies from other regions also demonstrated that times of conflict and peace have varied effects on deforestation dynamics, contributing to both increases and decreases in forest cover (Hecht and Saatchi, 2007). In Sierra Leone, for example, deforestation was found to be significantly lower in conflict-afflicted settings compared to more conflict-free settings (Burgess et al., 2015). In the Democratic Republic of the Congo, conflict increased deforestation but also reduced mining activities associated with deforestation (Butsic et al., 2015). Conversely, in El Salvador, the civil war triggered international and urban migration that in part drove forest recovery (Hecht et al., 2006). In Rwanda, however, little difference was found in net trends of forest cover changes between times of conflict and peace. Deforestation dynamics differed spatially, though, which was partly related to resource exploitation near refugee settlements (forest losses) and forced migrations (forest gains) (Ordway, 2015).
Consistently, our results indicate that under certain circumstances, the peace agreement between the GoC and FARC did not significantly change deforestation dynamics, while under others, it exacerbated deforestation. For example, we found that after the peace agreement in 2016, deforestation trends changed from a downward trend to a stabilized trend in the 74 municipalities under category F (which represents 3% of Colombia’s deforestation during the analyzed period). Subsequently, trends changed from a downward to an upward trend in the 402 municipalities belonging to categories A, D, E, and G (45% of Colombia’s deforestation). In the 176 municipalities under category B (34% of Colombia’s deforestation), we see that deforestation dynamics remained stable throughout the study period before spiking around 2014 when negotiations were underway for the final peace agreement. Meanwhile, the 56 municipalities under category C (18% of Colombia’s deforestation) showed a relatively steady incline in forest cover loss throughout the study period, indicating that the peace agreement and past peace negotiations had no effect on either the trajectory or intensity of deforestation trends in these municipalities. These category C municipalities cover an area of 207,398 ha and are mostly located in the Andes and Amazon regions. Four of these municipalities are among the top ten municipalities with the highest deforestation rates in Colombia during the study period.
Our predictive models suggest that both forest dynamics and the peace agreement’s effect on forest cover are influenced by the six selected variables (conflict events, displaced people, coca area, number of cattle, number of cattle farms, and municipality area) in five out of the seven identified municipality categories. Moreover, the effect of these variables change according to temporal and spatial dimensions. The number of conflict events is the weakest predictor and coca, cattle, and municipality area are the strongest predictors at national, regional, and category levels. Combinations of the former three variables are the top three predictors for the four categories where most of Colombia’s deforestation occurs (A, B, C, and E). Municipality area, cattle, and cattle farms are the top three predictors of category D, and municipality area, cattle, and displaced people are the strongest predictors of categories F and G. This is consistent with results reporting that deforestation in Colombia’s forest frontiers is caused by complex interlinkages between land grabbing, cattle, and coca (Castro-Nunez et al., 2017a). However, low R2 values for categories F and G suggest that additional factors are likely driving the overall declining deforestation trends in these two categories. It is striking that these categories are present mostly in certain coastal areas in the Caribe and Pacifico regions and in the Andes close to municipalities without significant deforestation. For the coastal municipalities, their remoteness could be an explanation, while the municipalities in the Andes may already have a low level of forest cover.
Studies from different regions have demonstrated that armed conflicts can influence forest cover dynamics in varied ways through a number of channels (Aide and Grau, 2004; Sánchez-Cuervo and Aide, 2013; Burgess et al., 2015; Butsic et al., 2015; Ordway, 2015), and thus there is no consensus on whether conflict negatively or positively impacts forest cover. Our results also indicate that a variety of factors influence whether the impacts will be positive or negative. Armed conflicts might lead to forest cover losses in areas where armed groups exploit natural resources to finance their military campaigns (Didia, 1997). Alternatively, armed conflicts might reduce deforestation and drive forest cover recovery as armed forces protect dense forest areas to use as cover for their operations (Hecht and Saatchi, 2007). In addition, our results are in accordance with a previous spatially explicit analysis of deforestation and conflict variables (Castro-Nunez et al., 2016). The study reports that municipalities with a high number of armed conflict events and municipalities with a high number of conflict victims geographically overlap with low deforestation rates. In the case of highly forested municipalities where low deforestation rates and conflict events geographically overlap, these areas would be preferred battle sites. Conversely, highly forested municipalities where low deforestation rates overlap with a high number of victims may be preferred by non-state armed groups with hideouts (Castro-Nunez et al., 2017b).
The results of the analysis further support the hypotheses proposed by the forest-conflict transition models for Colombia (Castro-Nunez et al., 2017a). Based on our findings, municipalities in category C correspond to early phases of colonization. These municipalities on average are larger and characterized by a larger area of coca, higher deforestation rates, lower number of cattle considering the area of the municipalities, and lower number of cattle farms. They also have on average a higher number of conflict events. This reflects municipalities that are in the early phases of colonization.
Municipalities in category B on average are the second-largest municipalities, have the second-largest area of coca, the second-highest number of cattle considering the area of municipalities, the second-lowest number of cattle farms, the second-highest number of conflict events, and the highest number of displaced people. These characteristics are indicative of more advanced phases of colonization and illustrate land grabbing processes, given the ratio of the number of cattle to the number of cattle farms. Based on the deforestation graphs, the sharp increase in forest cover loss in these municipalities suggests that the peace agreement facilitated more aggressive land grabbing processes in these areas, thereby exacerbating deforestation in post-conflict years. Such post-conflict forest dynamics have been reported by other studies (e.g., Murillo-Sandoval et al., 2020, Murillo-Sandoval et al., 2021), which suggest a forest-coca-cattle ranching transition followed by land accumulation processes of different non-state actors.
The characteristics of municipalities in categories A and E indicate dynamics that are progressing toward a turning point, after which conflict will decline and deforestation will continue. Category E has the highest number of cattle and cattle farms, a smaller coca area, and a lower number of displaced people, paired with an overall higher number of conflict events and higher deforestation rates compared to other categories. For this category, we see that deforestation rates were declining from 2005 to 2014, which may indicate that several forested areas under this category were being utilized and thereby protected by armed groups to conceal their operations.
In this study, municipality area was included in the analysis to factor in the large differences in the areas of Colombian municipalities under the assumption that the larger the municipality, the lower the institutional presence. This is consistent with previous studies hypothesizing that high levels of conflict, forced displacements and rates of deforestation coincide with frontiers of expanding agriculture (Castro-Nunez et al., 2017a) and arguments that deforestation is largely an institutional and governance issue. Prem et al. (2020) argue that with the discontinuance of FARC activities after the peace agreement, regions previously controlled by the FARC became available for private investors to establish businesses, which in turn exacerbated deforestation. This process would likely be more prevalent in areas with lower institutional presence due to limited capacity to control and regulate such activities. Clerici et al. (2020) and Murillo-Sandoval et al. (2020) report a highly significant increase in the rate of deforestation in Colombia’s protected areas in the years after the peace agreement and partly attribute this to a lack of functional institutional presence by the government in many protected areas.
Due to the scale of analysis, this study provides unique insights into deforestation dynamics that past studies at the national or regional scale have been unable to provide. It highlights local phases of stability and instability of social-ecolocial systems as they are reasoned in Panarchy theory (Chaffin and Gunderson, 2016; Reyers et al., 2018). The different phases of adaptive cycles “exploitation,” “conservation,” “collapse/release,” and “reorganization” theorized by these scholars can be linked to different time periods of the identified municipality categories. For example, the peace negotiations between the GoC and the FARC can be seen as a form of reorganization, which is followed by a phase of exploitation, as both national institutions and international research teams report higher deforestation rates in Colombia for the years after the peace agreement (IDEAM Instituto de Hidrología Meteorología y Estudios Ambientales, 2020; Global Forest Watch, 2021). Furthermore, intertwined cross-scale interactions in social-ecolocial systems are central to Panarchy theory. The results in this study show the effect of certain cross-scale interactions and how the influence of different drivers differs in space and across scales. For example, we found that the importance of some drivers emerged only at the local (department) scale—cattle farms was an important predictor in Arauca, Choco, and Sucre and displaced people was an important predictor in Atlantico, Huila, Tolima, and Valle de Cauca (Supplementary Figure S4)—while other drivers such as municipality area, cattle, and coca were the main drivers at larger scales.
The results of this study can be used to provide insights into drivers of deforestation dynamics in other post-conflict countries as well as countries with ongoing armed conflicts. Furthermore, the conflict in Colombia may continue. FARC dissidents, criminal bands, and competing paramilitary groups continue to fight for control over areas previously dominated by the FARC and have promoted livestock production and coca crops as a means to expand territorial control (Clerici et al., 2020). Thus, a better understanding of how periods of conflict and peace influence forest cover and drivers of deforestation is critical for designing policies that achieve both peacebuilding and environmental conservation objectives. For instance, insights into these dynamics can be used to help blend approaches to achieving peace with those to curbing tropical deforestation, such as those for reducing deforestation and forest degradation (known as REDD+) and those for incentivizing company commitments to green agricultural supply chains. Similarly, such insights can help us understand the role of forest conservation efforts in delivering peace and, likewise, the role of peacebuilding efforts in delivering forest conservation.
Data Availability Statement
All datasets used in this study and their sources are listed in Table 1.
Author Contributions
RG: Methodology, Formal Analysis, Investigation, Writing–Original Draft, Visualization, Writing–Review and Editing; JS: Interpretation of results, Writing–Original Draft, Visualization, Writing–Review and Editing; AC-N: Conceptualization, Interpretation of Results, Writing–Original Draft, Supervision, Project Administration, Funding Acquisition, Writing–Review and Editing.
Funding
This research was funded by the project 18_III_106_COL_A_Sustainable productive strategies. This project is part of the International Climate Initiative (IKI). The Federal Ministry for the Environment, Nature Conservation and Nuclear Safety (BMU) supports this initiative on the basis of a decision adopted by the German Bundestag. The views expressed in this paper cannot be taken to reflect the official opinions of these organizations.
Conflict of Interest
The authors declare that the research was conducted in the absence of any commercial or financial relationships that could be construed as a potential conflict of interest.
Supplementary Material
The Supplementary Material for this article can be found online at: https://www.frontiersin.org/articles/10.3389/fenvs.2022.803368/full#supplementary-material
Footnotes
1ICA Instituto Colombiano Agropecuario. Respuesta Censo Bovinos por Municipio 2008. Bogotá DC, Colombia: Internal communication.
References
Aghabozorgi, S., Seyed Shirkhorshidi, A., and Ying Wah, T. (2015). Time-Series Clustering - A Decade Review. Inf. Syst. 53, 16–38. doi:10.1016/j.is.2015.04.007
Aide, T. M., and Grau, H. R. (2004). Globalization, Migration, and Latin American Ecosystems. Science 305, 1915–1916. doi:10.1126/science.1103179
Álvarez, M. D. (2003). Forests in the Time of Violence. J. Sustain. For. 16, 47–68. doi:10.1300/j091v16n03_03
Arbelaitz, O., Gurrutxaga, I., Muguerza, J., Pérez, J. M., and Perona, I. (2013). An Extensive Comparative Study of Cluster Validity Indices. Pattern Recognition 46, 243–256. doi:10.1016/j.patcog.2012.07.021
Armenteras, D., Cabrera, E., Rodríguez, N., and Retana, J. (2013). National and Regional Determinants of Tropical Deforestation in Colombia. Reg. Environ. Change 13, 1181–1193. doi:10.1007/s10113-013-0433-7
Baccini, A., Walker, W., Carvalho, L., Farina, M., Sulla-Menashe, D., and Houghton, R. A. (2017). Tropical Forests Are a Net Carbon Source Based on Aboveground Measurements of Gain and Loss. Science 358, 230–234. doi:10.1126/science.aam5962
Baptiste, B., Pinedo-Vasquez, M., Gutierrez-Velez, V. H., Andrade, G. I., Vieira, P., Estupiñán-Suárez, L. M., et al. (2017). Greening Peace in Colombia. Nat. Ecol. Evol. 1. doi:10.1038/s41559-017-0102
Baumann, M., and Kuemmerle, T. (2016). The Impacts of Warfare and Armed Conflict on Land Systems. J. Land Use Sci. 11, 672–688. doi:10.1080/1747423X.2016.1241317
BBC News (2019). Colombia Ex-Farc Rebel Iván Márquez Issues Call to Arms. Available at: https://www.bbc.com/news/world-latin-america-49508411 (Accessed October 17, 2020).
Beittel, J. S. (2015). Peace Talks in Colombia. Washington, DC: Library of Congress, Congressional Research Service.
Berhe, A. A. (2007). The Contribution of Landmines to Land Degradation. Land Degrad. Dev. 18, 1–15. doi:10.1002/ldr.754
Burgess, R., Miguel, E., and Stanton, C. (2015). War and Deforestation in Sierra Leone. Environ. Res. Lett. 10, 095014. doi:10.1088/1748-9326/10/9/095014
Butsic, V., Baumann, M., Shortland, A., Walker, S., and Kuemmerle, T. (2015). Conservation and Conflict in the Democratic Republic of Congo: The Impacts of Warfare, Mining, and Protected Areas on Deforestation. Biol. Conservation 191, 266–273. doi:10.1016/j.biocon.2015.06.037
Castro-Nunez, A., Mertz, O., Buritica, A., Sosa, C. C., and Lee, S. T. (2017a). Land Related Grievances Shape Tropical Forest-Cover in Areas Affected by Armed-Conflict. Appl. Geogr. 85, 39–50. doi:10.1016/j.apgeog.2017.05.007
Castro-Nunez, A., Mertz, O., and Quintero, M. (2016). Propensity of Farmers to Conserve Forest within REDD+ Projects in Areas Affected by Armed-Conflict. For. Pol. Econ. 66, 22–30. doi:10.1016/j.forpol.2016.02.005
Castro-Nunez, A., Mertz, O., and Sosa, C. C. (2017b). Geographic Overlaps between Priority Areas for Forest Carbon-Storage Efforts and Those for Delivering Peacebuilding Programs: Implications for Policy Design. Environ. Res. Lett. 12, 054014. doi:10.1088/1748-9326/aa6f20
Castro-Nunez, A. (2018). Responding to Climate Change in Tropical Countries Emerging from Armed Conflicts: Harnessing Climate Finance, Peacebuilding, and Sustainable Food. Forests 9, 621. doi:10.3390/f9100621
Ceballos, G., Ehrlich, P. R., Barnosky, A. D., García, A., Pringle, R. M., and Palmer, T. M. (2015). Accelerated Modern Human-Induced Species Losses: Entering the Sixth Mass Extinction. Sci. Adv. 1, e1400253. doi:10.1126/sciadv.1400253
Chaffin, B. C., and Gunderson, L. H. (2016). Emergence, Institutionalization and Renewal: Rhythms of Adaptive Governance in Complex Social-Ecological Systems. J. Environ. Manage. 165, 81–87. doi:10.1016/j.jenvman.2015.09.003
Chen, T., and Guestrin, C. (2016). “XGboost: A scalable tree boosting system,” in Proceedings of the 22nd ACM SIGKDD International Conference on Knowledge Discovery and Data Mining, August 2016. New York, NY: ACM, 785–794. doi:10.1145/2939672.2939785
Clerici, N., Armenteras, D., Kareiva, P., Botero, R., Ramírez-Delgado, J. P., Forero-Medina, G., et al. (2020). Deforestation in Colombian Protected Areas Increased During Post-Conflict Periods. Sci. Rep. 10, 1–10. doi:10.1038/s41598-020-61861-y
Collier, P., and Hoeffler, A. (2004). Greed and Grievance in Civil War. Oxford Econ. Pap. 56, 563–595. doi:10.1093/oep/gpf064
Curtis, P. G., Slay, C. M., Harris, N. L., Tyukavina, A., and Hansen, M. C. (2018). Classifying Drivers of Global Forest Loss. Science 361, 1108–1111. doi:10.1126/science.aau3445
DANE (Departamento Administrativo Nacional de Estadística) (2018). Nivel Geográfico Municipio. Available at: https://geoportal.dane.gov.co/servicios/descarga-y-metadatos/descarga-mgn-marco-geoestadistico-nacional/ (Accessed March 7, 2020).
Dávalos, L. M., Bejarano, A. C., Hall, M. A., Correa, H. L., Corthals, A., and Espejo, O. J. (2011). Forests and Drugs: Coca-Driven Deforestation in Tropical Biodiversity Hotspots. Environ. Sci. Technol. 45, 1219–1227. doi:10.1021/es102373d
De Jong, W., Donovan, D., and Abe, K. (2007). Extreme Conflict and Tropical Forests. Dordrecht, Netherlands: Springer Science & Business Media. doi:10.1007/2F978-1-4020-5462-4
Didia, D. O. (1997). Democracy, Political Instability and Tropical Deforestation. Glob. Environ. Change 7. doi:10.1016/S0959-3780(96)00024-6
Don, A., Schumacher, J., and Freibauer, A. (2011). Impact of Tropical Land-Use Change on Soil Organic Carbon Stocks - a Meta-Analysis. Glob. Change Biol. 17, 1658–1670. doi:10.1111/j.1365-2486.2010.02336.x
Dudley, J. P., Ginsberg, J. R., Plumptre, A. J., Hart, J. A., and Campos, L. C. (2002). Effects of War and Civil Strife on Wildlife and Wildlife Habitats. Conservation Biol. 16, 319–329. doi:10.1046/j.1523-1739.2002.00306.x
Eufemia, L., Bonatti, M., Castro-Nunez, A., Lana, M., Morales, H., and Sieber, S. (2019). Colombia's Inadequate Environmental Goals. Science 364, 444–445. doi:10.1126/science.aax3494
FEDEGAN (Federación Colombiana de Ganaderos) (2019). Inventario bovino por municipios desde 2019. Available at: https://www.fedegan.org.co/estadisticas/inventario-ganadero (Accessed April 4, 2019).
Fergusson, L., Romero, D., and Vargas, J. F. (2014). The Environmental Impact of Civil Conflict: the Deforestation Effect of Paramilitary Expansion in Colombia. SSRN J. doi:10.2139/ssrn.2516512
Friedman, J. H. (2001). Greedy Function Approximation: a Gradient Boosting Machine. Ann. Statist. 29, 1189–1232. doi:10.1214/aos/1013203451
Ganzenmüller, R., Pradhan, P., and Kropp, J. P. (2019). Sectoral Performance Analysis of National Greenhouse Gas Emission Inventories by Means of Neural Networks. Sci. Total Environ. 656, 80–89. doi:10.1016/j.scitotenv.2018.11.311
Geist, H. J., and Lambin, E. F. (2002). Proximate Causes and Underlying Driving Forces of Tropical Deforestation. BioScience 52, 143–150. doi:10.1641/0006-3568(2002)052[0143:pcaudf]2.0.co;2
Giorgino, T. (2009). Computing and Visualizing Dynamic Time Warping Alignments in R: The dtw Package. J. Stat. Soft. 31, 1–24. doi:10.18637/jss.v031.i07
Global Forest Watch, (2021). Dashboards — Colombia. Washington, DC: World Resource Institute. Available at: http://www.globalforestwatch.org/dashboards/country/COL/ (Accessed January 6, 2021).
González, J., Cubillos, A., Chadid, M., Cubillos, A., Arias, M., Zúñiga, E., et al. (2018). Caracterización de las principales causas y agentes de la deforestación a nivel nacional período 2005–2015. Programa ONU-REDD Colombia
Hansen, M. C., Potapov, P. V., Moore, R., Hancher, M., Turubanova, S. A., Tyukavina, A., et al. (2013). High-Resolution Global Maps of 21st-Century Forest Cover Change. Science 342, 850–853. doi:10.1126/science.1244693
Hanson, T. (2018). Biodiversity Conservation and Armed Conflict: a Warfare Ecology Perspective. Ann. N.Y. Acad. Sci. 1429, 50–65. doi:10.1111/nyas.13689
Hecht, S. B., Kandel, S., Gomes, I., Cuellar, N., and Rosa, H. (2006). Globalization, Forest Resurgence, and Environmental Politics in El Salvador. World Dev. 34, 308–323. doi:10.1016/j.worlddev.2005.09.005
Hecht, S. B., and Saatchi, S. S. (2007). Globalization and Forest Resurgence: Changes in Forest Cover in El Salvador. BioScience 57, 663–672. doi:10.1641/B570806
Homer-Dixon, T. F. (1994). Environmental Scarcities and Violent Conflict: Evidence from Cases. Int. security 19, 5–40. doi:10.2307/2539147
IDEAM (Instituto de Hidrología Meteorología y Estudios Ambientales) (2020). Resultados de monitoreo deforestación 2019. Available at: http://www.ideam.gov.co/documents/10182/105413996/presentacionbalancedeforestacion2019/7c9323fc-d0a1-4c95-b1a1-1892b162c067#:∼:text=Para el año 2019 la,2018 cuando fue de 12%25.&text=En la Orinoquía se consolidan,el curso del río Vichada (Accessed January 6, 2021).
IPBES (2019). Global Assessment Report on Biodiversity and Ecosystem Services of the Intergovernmental Science-Policy Platform on Biodiversity and Ecosystem Services. Editors E. S. Brondizio, J. Settele, S. Díaz, and H. T. Ngo (Bonn, Germany: IPBES secretariat).
IPCC (2019). Climate Change and Land: An IPCC Special Report on Climate Change, Desertification, Land Degradation, Sustainable Land Management, Food Security, and Greenhouse Gas Fluxes in Terrestrial Ecosystems. Editors P. R. Shukla, J. Skea, E. Calvo Buendia, V. Masson-Delmotte, H.-O. Pörtner, and D. C. Roberts (Geneva, Switzerland). Available at: https://www.ipcc.ch/srccl
IPCC (2021). Climate Change 2021: The Physical Science Basis. Contribution of Working Group I to the Sixth Assessment Report of the Intergovernmental Panel on Climate Change. Editors V. Masson-Delmotte, P. Zhai, A. Pirani, S. L. Connors, C. Péan, and S. Berger (Cambridge University Press). Available at: https://www.ipcc.ch/report/ar6/wg1/.
Kuhn, M. (2014). Futility Analysis in the Cross-Validation of Machine Learning Models. arXiv preprint http://arxiv.org/abs/1405.6974.
Landholm, D. M., Pradhan, P., and Kropp, J. P. (2019). Diverging Forest Land Use Dynamics Induced by Armed Conflict Across the Tropics. Glob. Environ. Change 56, 86–94. doi:10.1016/j.gloenvcha.2019.03.006
Le Billon, P. (2000). The Political Ecology of Transition in Cambodia 1989-1999: War, Peace and Forest Exploitation. Dev. Change 31, 785–805. doi:10.1111/1467-7660.00177
Müller, M. (2007). Information Retrieval for Music and Motion - Dynamic Time Warping. Berlin, Germany: Information Retrieval for Music and Motion. doi:10.1007/978-3-540-74048-3
Murillo-Sandoval, P. J., Gjerdseth, E., Correa-Ayram, C., Wrathall, D., van den Hoek, J., Dávalos, L. M., et al. (2021). No Peace for the Forest: Rapid, Widespread Land Changes in the Andes-Amazon Region Following the Colombian Civil War. Glob. Environ. Change 69, 102283. doi:10.1016/j.gloenvcha.2021.102283
Murillo-Sandoval, P. J., van Dexter, K., van den Hoek, J., Wrathall, D., and Kennedy, R. (2020). The End of Gunpoint Conservation: Forest Disturbance after the Colombian Peace Agreement. Environ. Res. Lett. 15, 034033. doi:10.1088/1748-9326/ab6ae3
Negret, P. J., Allan, J., Braczkowski, A., Maron, M., and Watson, J. E. M. (2017). Need for Conservation Planning in Postconflict Colombia. Conservation Biol. 31, 499–500. doi:10.1111/cobi.12935
Negret, P. J., Sonter, L., Watson, J. E. M., Possingham, H. P., Jones, K. R., Suarez, C., et al. (2019). Emerging Evidence that Armed Conflict and Coca Cultivation Influence Deforestation Patterns. Biol. Conservation 239, 108176. doi:10.1016/j.biocon.2019.07.021
Nilsson, M., and González Marín, L. (2020). Violent Peace: Local Perceptions of Threat and Insecurity in Post-Conflict Colombia. Int. Peacekeeping 27, 238–262. doi:10.1080/13533312.2019.1677159
Nishio, M., Nishizawa, M., Sugiyama, O., Kojima, R., Yakami, M., Kuroda, T., et al. (2018). Computer-aided Diagnosis of Lung Nodule Using Gradient Tree Boosting and Bayesian Optimization. PLoS ONE 13, e0195875. doi:10.1371/journal.pone.0195875
OACP (Oficina del Alto Comisionado para la Paz) (2016). Final Agreement to End the Armed Conflict and Build a Stable and Lasting Peace. Available at: http://especilales.presidencia.gov.co/Documents/20170620-dejacion-armas/acuerdos/acuerdo-final-ingles.pdf (Accessed June 7, 2019).
Ordway, E. M. (2015). Political Shifts and Changing Forests: Effects of Armed Conflict on forest Conservation in Rwanda. Glob. Ecol. Conservation 3, 448–460. doi:10.1016/j.gecco.2015.01.013
Paparrizos, J., and Gravano, L. (2015). “K-Shape,” in Proceedings of the 2015 ACM SIGMOD International Conference on Management of Data (New York, NY: SIGMOD Rec.) 45, 69–76. doi:10.1145/2949741.2949758
Prem, M., Saavedra, S., and Vargas, J. F. (2020). End-of-conflict Deforestation: Evidence from Colombia's Peace Agreement. World Dev. 129, 104852. doi:10.1016/j.worlddev.2019.104852
Rani, S., and Sikka, G. (2012). Recent Techniques of Clustering of Time Series Data: A Survey. Ijca 52, 1, 9. doi:10.5120/8282-1278
Raven, P. H. (1988). “Our Diminishing Tropical Forests,” in Biodiversity. Editors E. O. Wilson, and F. M. Peter (Washington, DC: National Academy Press).
Reyers, B., Folke, C., Moore, M.-L., Biggs, R., and Galaz, V. (2018). Social-ecological Systems Insights for Navigating the Dynamics of the Anthropocene. Annu. Rev. Environ. Resour. 43, 267–289. doi:10.1146/annurev-environ-110615-085349
RNI (Red Nacional de Información) (2019). Número de personas por municipio y año de ocurrencia. Available at: https://cifras.unidadvictimas.gov.co/Reporteador?pag=2¶metros (Accessed January 23, 2019).
Rousseeuw, P. J. (1987). Silhouettes: a Graphical Aid to the Interpretation and Validation of Cluster Analysis. J. Comput. Appl. Math. 20, 53–65. doi:10.1016/0377-0427(87)90125-7
Rustad, S. A., and Binningsbø, H. M. (2012). A Price Worth Fighting For? Natural Resources and Conflict Recurrence. J. Peace Res. 49, 531–546. doi:10.1016/j.polgeo.2008.09.004
Rustad, S. C. A., Rød, J. K., Larsen, W., and Gleditsch, N. P. (2008). Foliage and Fighting: Forest Resources and the Onset, Duration, and Location of Civil War. Polit. Geogr. 27, 761–782. doi:10.1016/j.polgeo.2008.09.004
Sánchez-Cuervo, A. M., and Aide, T. M. (2013). Consequences of the Armed Conflict, Forced Human Displacement, and Land Abandonment on Forest Cover Change in Colombia: a Multi-Scaled Analysis. Ecosystems 16, 1052–1070. doi:10.1007/s10021-013-9667-y
SIMCI/UNODOC (Sistema Integrado de Monitoreo de Cultivos Ilícitos/United Nations Office on Drugs and Crime) (2019). Sistema de información de drogas de Colombia. Available at: http://www.odc.gov.co/sidco/oferta/cultivos-ilicitos/departamento-municipio (Accessed November 22, 2019).
Stevens, K., Campbell, L., Urquhart, G., Kramer, D., and Qi, J. (2011). Examining Complexities of Forest Cover Change during Armed Conflict on Nicaragua's Atlantic Coast. Biodivers Conserv 20, 2597–2613. doi:10.1007/s10531-011-0093-1
Suarez, A., Árias-Arévalo, P. A., and Martínez-Mera, E. (2018). Environmental Sustainability in Post-Conflict Countries: Insights for Rural Colombia. Environ. Dev. Sustain. 20, 997–1015. doi:10.1007/s10668-017-9925-9
Sundberg, R., and Melander, E. (2013). Introducing the UCDP Georeferenced Event Dataset. J. Peace Res. 50, 523–532. doi:10.1177/2F0022343313484347
Tropek, R., Sedláček, O., Beck, J., Keil, P., Musilová, Z., Šímová, I., et al. (2014). Comment on "High-Resolution Global Maps of 21st-Century Forest Cover Change". Science 344, 981. doi:10.1126/science.1248753
University of Maryland, (2021). Global Forest Change 2000–2018. Available at: https://earthenginepartners.appspot.com/science-2013-global-forest/download_v1.6.html (Accessed January 6, 2021).
Van, T. T., Wilson, N., Thanh-Tung, H., Quisthoudt, K., Quang-Minh, V., Xuan-Tuan, L., et al. (2015). Changes in Mangrove Vegetation Area and Character in a War and Land Use Change Affected Region of Vietnam (Mui Ca Mau) over Six Decades. Acta Oecologica 63, 71–81. doi:10.1016/j.actao.2014.11.007
Keywords: deforestation, conflict, peace agreement, Colombia, time series clustering, XGBoost, land grabbing, climate change
Citation: Ganzenmüller R, Sylvester JM and Castro-Nunez A (2022) What Peace Means for Deforestation: An Analysis of Local Deforestation Dynamics in Times of Conflict and Peace in Colombia. Front. Environ. Sci. 10:803368. doi: 10.3389/fenvs.2022.803368
Received: 27 October 2021; Accepted: 17 January 2022;
Published: 21 February 2022.
Edited by:
Ioan Cristian Ioja, University of Bucharest, RomaniaReviewed by:
Constantina Alina Hossu, University of Bucharest, RomaniaTibor Hartel, Babeș-Bolyai University, Romania
Copyright © 2022 Ganzenmüller, Sylvester and Castro-Nunez. This is an open-access article distributed under the terms of the Creative Commons Attribution License (CC BY). The use, distribution or reproduction in other forums is permitted, provided the original author(s) and the copyright owner(s) are credited and that the original publication in this journal is cited, in accordance with accepted academic practice. No use, distribution or reproduction is permitted which does not comply with these terms.
*Correspondence: Augusto Castro-Nunez, QXVndXN0by5DYXN0cm9AY2dpYXIub3Jn
†These authors have contributed equally to this work