Heavy Metals in Soils From Intense Industrial Areas in South China: Spatial Distribution, Source Apportionment, and Risk Assessment
- 1Guangdong Provincial Key Laboratory of Marine Disaster Prediction and Prevention, Shantou University, Shantou, China
- 2Institute of Marine Sciences, Shantou University, Shantou, China
- 3Key Laboratory of Environment Nanotechnology and Health Effects, Research Center for Eco-Environmental Sciences, Chinese Academy of Sciences, Beijing, China
Heavy metal pollution from various industrial activities has raised global concern. The present study collected 71 surface and 67 subsurface soils from the area with intensive industrial activities in South China. The typical heavy metals, including Cd, As, Hg, Cu, Ni, Pb, Zn, and Cr concentrations, were detected for studying their spatial distribution patterns. Sources apportionment and risks were analyzed using geographical information system and combined approach, including principal component analysis (PCA), positive matrix factorization (PMF), and geo-accumulation index. The concentrations of Cd, As, Hg, Cu, Ni, Pb, and Zn (except Cr) were higher than relevant background levels while exhibiting different pollution degrees in surface and subsurface soils. The PMF results showed that agriculture, industry, transportation, and nature were the four primary contributing sources of heavy metals in soils from the study area. In general, anthropogenic activities were the dominant factors, significantly influencing heavy metal distributions in surface and subsurface soils. Health risks of heavy metals in soil to the population via soil inhalation, skin contact, and ingestion indicated the non-carcinogenic and carcinogenic risks were at an unacceptable level. Each exposure route contribution is higher for adults than for children, whereas there is a relatively high risk of cancer for adults than for children. Therefore, increasing attention shall be needed for monitoring the heavy metal pollution in soils in the areas with dramatic economic development, e.g., South China.
Introduction
Due to the increasing economic development, industrialization, and urbanization in China, it is confronted with a variety of environmental issues (Wang et al., 2020; Zhao et al., 2014). Increasing human activities such as industrial developments release heavy metals into the environment. These pollutants eventually accumulate in the soil, especially in places with rapid industrialization, like in China (Jin et al., 2019; Liu et al., 2020). According to the Chinese bulletin on the State of China’s Ecology and Environment published by the Ministry of Natural Resources of China and the Ministry of Ecology and Environment of China in 2018, soil pollution in the south part of China is heavier than that in the north, soil pollution in the Pearl River Delta and Yangtze River Delta is more prominent and higher than the national related standards (Ministry of Environmental Protection of China, 2018). Because of their significant toxicities, bioaccumulation, and persistence, some heavy metals such as Cd, Cr, As, Hg, Pb, Cu, Zn, and Ni have been identified as priority control contaminants by most countries including China. Soil is a critical element of the Earth’s ecosystem and the physical foundation for human survival and development. It plays a significant role as a sink for polluting heavy metals (Li et al., 2014). Accumulation of heavy metals in soil may result in deteriorating soil quality, reduce soil fertility and crop production, and even threaten human and animal health (Zang et al., 2017; Fei et al., 2020). Thus, it is important for protecting and improving the soil environment.
In the past few decades of research on heavy metal pollution in soils, scholars from all over the world have reported related studies in different regions (Nabulo et al., 2010; Radziemska and Fronczyk, 2015; Rivera et al., 2016) and even in China (Luo et al., 2012; Zhao et al., 2014; Wang et al., 2020). Thanks to scientific and technological advances, methods for assessing heavy metal pollution have become more precise and diverse. Multiple methods, such as geographic information system (GIS), principal component analysis (PCA), and positive matrix factorization (PMF) were used to find the distinct distributions and sources of heavy metals in soils (Zhang et al., 2018; Dong et al., 2019; Jin et al., 2019). Moreover, multiple indicators such as geoaccumulation index (Igeo), potential ecological risk index, and human health risk (non-carcinogenic and carcinogenic risks) have been employed to comprehensively evaluate the heavy metal pollution in soils (Peng et al., 2017; Shi et al., 2018; Tian et al., 2020).
Generally, the heavy metal contaminants in soils often originate from two significant sources, including natural (soil parent materials) and various anthropogenic sources (transportation, industrial activities, and agricultural activities). Therefore, it is crucial to qualitatively and quantitatively discriminate pollution sources for protecting the soil environment (Dong et al., 2019; Fei et al., 2020; Zhao et al., 2021). There are two primary methods for determining soil sources of heavy metals: qualitative source identification and quantitative source distribution. Qualitative identification used in previous studies has included multidimensional statistics. However, there are still limitations on the quantification of contributions from multi-sources in this method (Sun et al., 2013). Thus, the quantitative apportionment of sources has been used extensively in more recent studies because of its capacity to quantify the contributions of various sources of contamination. PMF is a simple and useful multivariate receptor analysis model. Although many publications adopted the PCA and PMF methods, few comprehensive types of research have been conducted for combining qualitative source identification and quantitative source apportionment (Xue et al., 2014; Yadav et al., 2014).
In this study, geostatistical analysis, PCA, and PMF were used to evaluate the spatial distribution pattern, accumulation characteristics, and source apportionment of soil heavy metals, then Igeo, potential ecological risk index, and health risk (non-carcinogenic and carcinogenic risks) were adopted to assess pollutions in soils collected near intense industrial activities from South China. The primary purposes of this study were as follows: 1) to analyze the content and basic properties of heavy metals in soils; 2) to characterize spatial distributions of soil heavy metals; 3) to quantitatively identify potential various heavy metal sources in soils; and 4) to evaluate the potential risks of heavy metals accumulation in soils.
Materials and Methods
Study Area
The selected study area is near a region with intensive industrial activities, in the southern coastal area of China, with an investigated area of 957.03 km2 (Figure 1). As a typical alluvial plain, the study area has a subtropical monsoon climate zone with an average annual temperature of 21–28°C and annual precipitation of 1747.4 mm, respectively. There are various land uses in the study area, such as impervious surface, cropland, water, forest, grassland, and wetland zones. The principal crops cultivated here are rice and vegetables. The area is characterized by lateritic red soil and slightly acidic soil. It is worth noting that this area is one of the largest electronics manufacturing centers in China and an area with many chemicals, printing, and dyeing. There are 98 electroplating factories and other industrial enterprises allocating the study area with high population density and high development levels.
Sampling, Processing, and Analysis
According to the principle of randomness and equivalence, surface soil (0–20 cm depth) and subsurface soil (20–40 cm depth) samples were collected at 16 regions inside the survey zone (Figure 1) following a stratified random to represent sampling across the entire area. In total, 71 surface soils and 67 subsurface soils were collected with the aid of a global positioning system receiver in 2018. The range of 50 m × 50 m grid was extracted 5 soil sub-samples from each sampling location after being mixed thoroughly, and then using the coning and quartering method to obtain a representative sample. All samples are collected with stainless steel shovels, then placed in polyethylene plastic bags, labeled relative to each other, and transported back to the laboratory. Finally, each sample was air-dried at room temperature. Afterward, the samples were gently crushed and ground in a mortar to sieve through 10-mesh and 100-mesh powder for soil pH and heavy metals determinations.
The soil samples referenced to the national standard (HJ803-2016) were digested with aqua regia to determine heavy metals. Cu, Cr, Ni, Zn, Pb, and Cd were detected using an inductively coupled plasma-mass spectrometer (ICP-MS), while As and Hg were measured by using atomic fluorescence spectrometry (AFS). In this study, standard quality assurance and quality control were rigorously maintained throughout the experiment. Recovery rates for heavy metals varied between 70 and 125%, and the results of the experiments are consistent with the quality control standard. In addition, the method detection limits (MDL) for Cu, Cr, Ni, Zn, Pb, Cd, As, and Hg were 0.05, 0.05, 0.02, 0.15, 0.08, 0.001, 0.03, and 0.003 mg/kg, respectively.
Sources Identification of Heavy Metals
Principal Component Analysis Model
PCA is a multi-statistical technique commonly used to reduce the dimensions of many tedious data indicators to obtain a relatively small amount of useful data, performed to identify relationships between heavy metals in the soil and to the analysis of possible sources of a variety of heavy metals (Tian et al., 2020). It provides both qualitative and quantitative information about the source of metal contaminations. Account for relationships between different variables, PCA is used to extract source identification for varied factors whose value is greater than 1 after variant rotation (Liu et al., 2020). PCA has been widely used for studying the sources of contamination in a variety of environmental matrixes around the world (Yadav et al., 2014; Barzegar et al., 2017).
Positive Matrix Factorization Model
PMF is a simple and useful variable receptor analysis model that the USEPA recommends as a general apportionment modeling tool for quantifying contributions based on compositions (Jiang et al., 2017). The PMF model decomposes the data matrix into two matrices: factorial assessments (G) and factorial profiles (F). PMF runs the model using concentration data (including 8 elements) and uncertainty data files and does not require a source profile. In this study, PMF 5.0 was adopted to distribute heavy metal sources into soils (Paatero, 1997). The PMF model can be represented using the formula listed in Supplementary Table S1. The concentration and uncertainty data are first imported into the software during the PMF model in operation. To ensure data analysis accuracy, we minimize the Q value, select varied factors (3, 4, and 5), and run the program 20 times until the model stabilizes. Finally, the results of multiple models run show that when the number of factors is 4, the difference between Qtrue and Qrobust is minimal and relatively stable, with signal-to-noise (S/N) ratios for all heavy metals ranging from 0.6 to 2.4, with prediction residuals normally distributed within between −1.5 and 1.5 and a prediction R2 greater than 0.39.
Assessment of Contamination and Risk
Geoaccumulation Index (Igeo)
The Igeo is the indicator used for assessment of the level of pollution and enriches by heavy metals in sediments and soils (Müller, 1969), and was derived from heavy metal data and conventional laboratory analytical data to assess the accumulation of heavy metals in soil. The Igeo values were calculated according to the formula listed in Supplementary Table S1. In terms of the qualitative scale of pollution intensity, Igeo values are classified as unpolluted (Igeo ≤ 0), unpolluted to moderately polluted (0 < Igeo≤1), moderately polluted (1 < Igeo ≤ 2), moderate to strongly polluted (2 < Igeo ≤ 3), strongly polluted (3 < Igeo ≤ 4), strongly to extremely polluted (4 < Igeo ≤ 5), and extremely polluted (Igeo > 5) (Müller, 1969).
Potential Ecological Risk
The potential ecological risk index (RI), which was proposed by Håkanson (1980), was used to evaluate the overall state of sediment pollution and has been used to assess ecological risk in different fields of research effects on the environment with toxicology (Wang et al., 2018). The RI can be determined by the formulas listed in Supplementary Table S1. Wherein
Human Health Risk
The human health risk evaluation model proposed by USEPA was used which is both non-carcinogenic and carcinogenic (USEPA, 1996; USEPA, 1997; USEPA, 2002) and it establishes a relationship between contaminant levels in the environment and the likelihood of toxic effects to a human population. People have been subjected to heavy metals from the ground, primarily contact through skin, inhalation of dust particles, ingestion directly through the soil, and indirectly through consumption of homegrown produce (Jiang et al., 2017). Exposure risk can be calculated by daily dose, with different daily doses for each heavy metal, and the risk of each exposure pathway can be calculated using a formula listed in Supplementary Table S1 (Li et al., 2015; Ma et al., 2018; Ning et al., 2021).
The Hazard Quotient (HQ) was used to calculate the impact of heavy metals in soil on human non-carcinogenic risk (Zhao et al., 2012). HQ summation over multiple paths gives a corresponding hazard index (HI) (USEPA, 2009; Zhao et al., 2014). Carcinogenic risk (CR) was used to estimate the incremental likelihood of personal exposure to carcinogenic hazards (Zhao et al., 2021). The CR of the three different pathways adds up to the total cancer risk (TCR) listed in Supplementary Table S1. Supplementary Table S3 provides details on the parameters and input values that were used to estimate ADD in the non-cancer risk evaluation. The corresponding reference doses (RfD) and cancer slope factor (SF) values of eight metals are shown in Supplementary Table S4. All variables and their values refer to the USEPA (2016) guidelines, but specific parameters have been adjusted according to the real situation of Chinese residents in this study (Li et al., 2020).
Statistical and GIS Analysis
Statistical analysis software SPSS 26.0 (SPSS, United States) and Microsoft Excel 2016 were used to analyze the data. ArcGIS 10.2 software (ESRI, United States) was used to map the sampling sites and project the spatial distribution of heavy metals. Geostatistical model and ordinary Kriging interpolation were adopted for characterizing the spatial distributions. Source analysis of heavy metals used the PMF model (USEPA PMF 5.0).
Results and Discussion
Descriptive Statistics of Heavy Metals in Soils
The statistical analysis of heavy metal levels in surface and subsurface soils is shown in Table 1. Cr, Ni, Cu, Zn, As, Cd, Pb, and Hg concentrations in surface soils were 3.78–84.62, 1.53–56.00, 3.80–125.05, 20.80–201.39, 4.30–89.92, 0.02–0.92, 15.29–160.07, and 0.01–0.41 mg/kg, with the mean of 40.00, 33.65, 46.98, 119.82, 20.49, 0.44, 43.85, and 0.12 mg/kg, respectively. In comparison to the soil background value (BV), the mean concentrations of most heavy metals (except Cr) were higher than their BVs and the contents of Ni, Cu, Zn, As, Cd, Pb, and Hg were higher than their BVs by approximately 2.34, 2.76, 2.53, 2.30, 7.86, 1.22, and 1.54 times, respectively. This indicated a tendency of metal accumulation in the soils. The heavy metals with the highest multiple, which was for Cd, indicated that Cd would be enriched in the surface soil through human activities (Fei et al., 2020). Based on the analysis using the coefficient of variation (CV), the large CVs for Hg (61.00%), As (63.41%), and Cd (39.34%) suggested that these three elements had relatively high spatial variation, possibly industrial or agricultural activities (Jia et al., 2018; Tume et al., 2018).
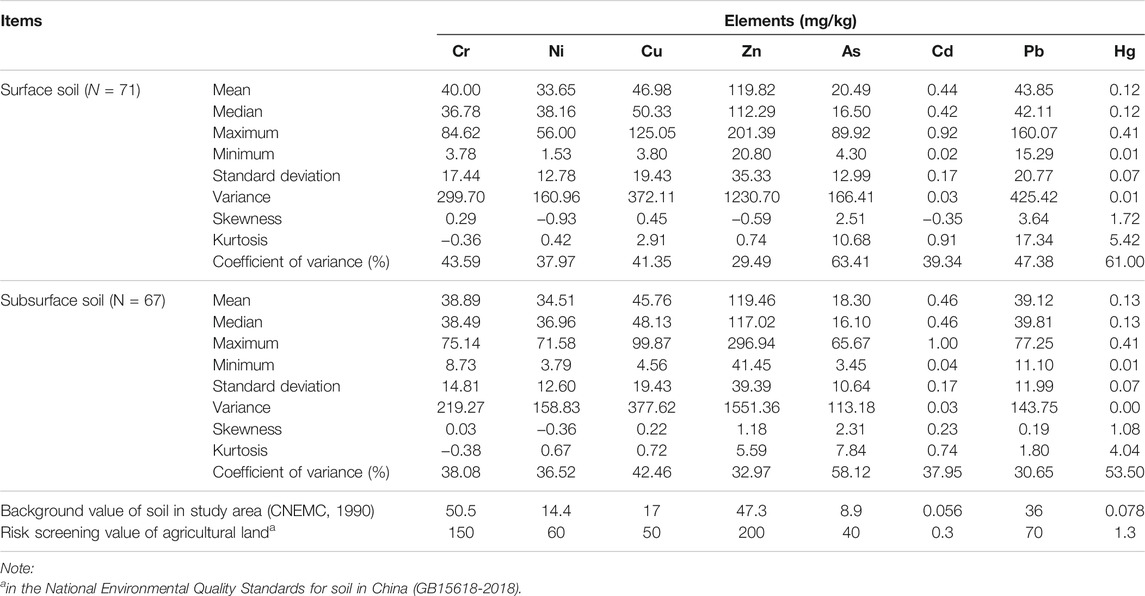
TABLE 1. Summary of statistical result characteristics of heavy metals in surface and subsurface soil in the study area.
As for subsurface soil, the variations of Cr, Ni, Cu, Zn, As, Cd, Pb, and Hg were 8.73–75.14, 3.79–71.58, 4.56–99.87, 41.45–296.94, 3.45–65.67, 0.04–1.00, 11.10–77.25, and 0.01–0.41 mg/kg, respectively. The relevant means were 38.89, 34.51, 45.76, 119.46, 18.30, 0.46, 39.12, and 0.13 mg/kg, respectively. Compared with the corresponding soil BVs, the average levels of most heavy metals (except Cr) are higher than their BVs (Table 1). The Cd in surface and subsurface soils was the dominant element and much higher than its BVs. Compared with the risk screening values of agricultural land defined by the National Environmental Quality Standards for Soils in China (GB 15618-2018), all heavy metals except Cd were significantly less than their corresponding risk screening value.
Spatial Distributions of Heavy Metal in Soils
The spatial distribution of metal concentration is a useful way to identify hotspots with high metal contaminations and thus to define the potential sources. The spatial characteristics of all metals were explained using the ordinary kriging within the ArcGIS 10.2 software (Figure 2). The Cd, Cr, Hg, and Zn in surface soils showed a remarkably similar spatial pattern. The contents decreased significantly from northwest to southeast, wherein Cd was a regional pollutant with the highest level of pollution and coverage (concentration higher than 0.49 mg/kg). The intense industrial and agricultural activities may be the main reasons for the high metal level in surface soils from the northwest area (Wang et al., 2020). In spatial distribution, the levels of Pb and Hg are similar, with higher concentrations mainly distributed in the regions C13, C15, and C16, and significant low levels at junctions of C04 and C07. The high Pb levels in this field can be due to urban traffic. The hotspots of As were to the east and southwest of the C06, C07, C14, C09, and C10, some chemical industries were located around these locations. For Ni, compared with other metals, the degree of pollution is not obvious. To a certain extent, urban soil pollution is becoming more and more serious partly because of more and more industrial activities.
The distribution pattern of heavy metals in subsurface soil was clear through spatial analysis. The subsurface soil had higher contents of Cd similar to the surface soils, with high values mainly located in C02, C03, C04, C05, and C07 (Figure 3). The Cd hotspots were associated with human activities, such as agricultural and industrial activities. Yang et al. (2018) summarized the state of heavy metal pollution in China and demonstrated that Cd plays a leading role in heavy metal pollution of industrial soil. The concentrations of Cu and As are similar in spatial distribution mode through the spatial analysis results. It was found that the area with high concentration of heavy metal is mainly distributed in C9, C10, and C11, whereas significant low concentrations of heavy metal were found in the junction of C06 and C07. The high concentrations in these areas may be caused by the role of associated differentiation of parent material in soil formation. Zn hotspots were to the east and northwest of the C03, C04, C10, and C16. The contamination hotspots of Cr located in C10, C11, and C16 are probably due to human factors (Lv et al., 2013). Hg was also a major contributor to higher levels of heavy metals in C13, C15, and C16, which may be caused by untreated sewage sludge or effluent discharge from industrial activities (Teng et al., 2014; Chen et al., 2015). The concentrations of Pb in soils were lower, with elevated concentrations primarily localized within C10, C15, and C16, which automobile emissions may cause. Ni was extremely focused in the east and southeastern part of the study area, which may be caused by fertilizer use.
Source Apportionment of Heavy Metals in Soils
Source Identification Using PCA
As previously mentioned, the PCA was used to analyze heavy metal relationships and sources for two series of variables: heavy metal levels in surface and subsurface soils. The Kaiser-Meyer-Olkin (KMO) measures of sampling adequacy for the above variables were 0.783 and 0.795, respectively. The KMO values were all well above the threshold of 0.5, showed a remarkably close correlation between them, and PCA produced good and convincing results (Field, 2009). The rotated factors obtained from the PCA are listed in Supplementary Table S5. The four principal components (PCs) were extracted by PCA and cumulatively explained 88.740% and 90.942% of the total variance in surface (PC1: 52.562%, PC2: 14.254%, PC3: 13.797%, PC4: 8.127%) and subsurface (PC1: 59.462%, PC2: 12.466%, PC3: 10.351%, PC4: 8.663%).
PC1 was dominated by Cr, Ni, Cu, Zn, and Cd and accounted for 52.562 and 59.462% of the total variance in surface and subsurface soils. In China, Cu and Zn are widely used in agricultural activities. For example, previous studies found that Zn is a very efficient ingredient of certain bactericidal substances that are used in food and cash crops (Chen et al., 2008; Komarek et al., 2010; Liang et al., 2017; Wang et al., 2020). In particular, the irrigation of wastewater and the application of fertilizers could cause significant Cd contamination in agricultural soils (Wei and Yang, 2010). Therefore, PC1 broadly reflects an anthropogenic source due to agricultural activities on soils.
PC2 accounted for 14.254% of the total variance and was dominated by As (0.955) and Cr (0.639) in surface soils. Moreover, it should be noted that the loadings of As and Cr in PC2 in surface and PC3 in subsurface soils are remarkably similar, suggesting that the PC2 and PC3 in the study area originate from similar sources. The study area is situated in an economically developed region with its intense industrial area and road network. Previous studies indicated that high levels of As in soil might be related to human-made waste and industrial operations, including wastewater sludge and industrial emissions (Zhao et al., 2014; Ma et al., 2018). Emissions from industrial facilities within the study area, such as chemical plants, printing workshops, and an electroplate factory, could contribute to soil pollution. Therefore, the component PC2 on surface and PC3 in subsurface soil were regarded as industrial activities.
PC3 loads heavily on Pb (0.980), and accounted for 13.797% of the total variance in surface soil, while PC2 dominated by Pb (0.889) accounted for 12.466% in the subsurface soil. According to previous studies, Pb may mainly come from the chemical industry and transportation emissions related to human activities (Sun et al., 2010; Zhao et al., 2014). Although Pb gasoline has been banned in China since 2000, tetraethyl Pb is added to gasoline as an anti-knock agent and still discharges Pb into the environment (Komarek et al., 2010; Dong et al., 2019). Furthermore, enormous quantities of emissions and inadequate waste disposal may have been responsible for Pb pollution around the study area as well. For these reasons, the component PC3 on the surface and PC2 in the subsurface soil were considered transportation activities.
PC4 accounted for 8.127% of the total variance in the surface and subsurface soil, and Hg had large loads on PC4 (0.965 and 0.958 in the surface and subsurface, respectively). As far as descriptive statistics are concerned (see Supplementary Table S1), the average values of Hg were close to the soil background values. Combined with the spatial analysis results, the concentrations of Hg at most locations were less than their BVs, showing that the accumulation of this metal was not obvious in the study area. Thus, PC4 represented a natural source, indicating that Hg is mainly derived from the process of soil formation.
Source Apportionment by PMF
To further analyze and quantify the sources and contributions of heavy metals in the soil of the study area, the PMF analysis was completed (Figure 4). Intriguingly, the PMF results corresponded well to the PCA results (Supplementary Table S6). Factor 1 contributed to 9.30% of the heavy metals in the surface soil and included a higher contribution for Pb (39.40%) and Zn (14.40%), respectively. Previous studies found that a large amount of Pb in the human environment comes from traffic emissions, and coal burning is an important manufactured source (Huang et al., 2015; Shi et al., 2019a). Pb may be produced not only in vehicle emissions but also in particulate matter in agricultural activities. Some scholars have found that Pb in atmospheric particulate matter may come from the emission of coal burning and the particulate matter generated by agricultural activities (Huang et al., 2018b; Tao et al., 2018). Similarly, the accumulation and enrichment of Zn in the soil are inextricably linked to the transportation industry. For example, the use of automotive tires has proven to be an important non-point source of Zn accumulation in urban surface soils (Radziemska and Fronczyk, 2015). This result strengthens the Pb and Zn accumulated due to transportation activities. Therefore, Factor 1 can be attributed to transportation.
Factor 2 accounted for 30.56% of the total contribution with As (73.10%), Cr (60.60%), Cu (36.40%), and Ni (26.10%). Chemical, printing and dyeing, electroplating, sewage treatment, and other industrial enterprises were located in the study region, causing the coal-burning emissions from industrial activities (Amir et al., 2020). Similar to the statement above, Cu and Ni were mainly caused by coal-burning emissions from industrial activities. Industrial plants emit enormous quantities of dust containing As, Cr, Cu, and Ni, which causes the soil to be polluted by these elements. Thus, Factor 2 can be associated with industrial activities.
Factor 3 contributed 28.73% of the heavy metals and demonstrated high contribution levels of Cd (55.20%), Cu (30.80%), and Ni (29.20%), and a moderate level of Zn (37.44%). Of note, the contribution of Cd in Factor 3 was 55.20%, indicating that Factor 3 had significant importance on Cd accumulation. Lu et al. (2015) found a positive correlation between the use of chemical fertilizer and the concentration of Cd in grain (including rice, wheat, and corn). In agricultural production, sewage irrigation, fertilizer application, pesticides, and organic fertilizers cause serious pollution to agricultural soil. These agricultural production activities all produce heavy metal pollution element Cd to a certain extent. Therefore, Cd is generally considered as a reference element in agricultural production. According to previously published studies, Cd was derived mainly from anthropogenic activities (Wei and Yang, 2010; Shi et al., 2019b). Hence, Factor 3 represented agricultural activities and significantly affected the accumulation of Cd, Zn, Cu, and Ni.
Factor 4 contributed 31.43% of the heavy metals and was predominated by Hg, Ni, and Zn with loadings of 68.90, 44.50, and 37.40%, respectively. According to the above data analysis results, the average Hg content in the surface soil of the study area is 0.12 mg/kg, close to the local background value (Table 1). Thus, we can infer that Hg is mainly affected by natural geological background except for human factors (Fei et al., 2020). Therefore, the fourth factor may represent natural sources, such as soil parent materials.
Likewise, Factor 1 contributed to 40.01% of the heavy metals in subsurface soil and included a higher contribution for Hg (80.80%) and Cu (64.00%), respectively. The mean value of Hg content in subsurface soil in the study area was 0.13 mg/kg which is close to the local background value (Table 1). Therefore, the first source factor may represent natural sources, such as soil parent materials and pedogenic processes. Factor 2 accounted for 13.56% of the total contribution with Pb (40.60%), As (19.10%), and Zn (14.40%). Pb was distributed along the road in farmland, and the exhaust gases from motor vehicles and agricultural machinery and equipment contain Pb, which caused pollution to the soil (Luo et al., 2015; Jiang et al., 2017). Thus, Factor 2 is associated with transportation.
Factor 3 contributed 19.84% of the heavy metals and demonstrated strong contribution rates of Cd (43.30%), Zn (36.10%), Pb (30.30%), and Ni (19.00%). When growing and developing crops, fertilizer (mineral fertilizers, organic fertilizers, and/or manures) was applied, it not only provided nutrients for crop growth but was also a significant source of Cd, Pb, and Zn in agricultural soils (Sun Q. B. et al., 2013; Hu et al., 2018; Shi et al., 2019a). Hence, Factor 3 represented agricultural activities. Factor 4 contributed 26.61% of the heavy metals and was predominated by Cr, As, and Ni with loadings of 73.20, 49.60, and 31.30%, respectively. Men et al. (2018) found that fuel combustion and industrial products contributed the most to the concentration of Cr in soil. Therefore, Factor 4 may represent industrial activities.
Assessment Risk of Heavy Metals in Soils
Geoaccumulation Index (Igeo) Based Risks
The Igeo assessment was used for estimating the level of pollution from metals and assessed the degree of cumulative heavy metal contamination in soil. Supplementary Table S7 shows the categorical distribution of heavy metal geoaccumulation indices based on the classification system. The Igeo values of Cd, Cr, Ni, Cu, Zn, As, Pb, and Hg ranged from −1.91–3.45, −4.32–0.16, −3.82–1.37, −2.75–2.29, −1.77–1.51, −1.63–2.75, −1.82–1.57, and −4.18–1.79 in surface soils, respectively. The mean Igeo of Cd (2.18), Cu (0.70), Zn (0.66), Ni (0.42), and As (0.37) was greater than zero, while other heavy metals (Cr, Pb, and Hg) were less than zero, suggesting that these metals were related to the possible contamination in the surface soil in the study area. The Igeo values show that all sampling sites for Cr were below class 2, with nearly 99% falling into class 0. More than 70% of the sampling sites are lower than class 2 for As, Ni, Zn, Pb, and Hg (Supplementary Table S7). However, the Igeo values for Cd were above class 2 in about 80% of the study area, indicating moderate contamination of Cd in the surface soil. The Igeo for heavy metals in surface soil was illustrated in Supplementary Figure S1A. The lowest contamination levels were found in Cr, Pb, and Hg, with most Igeo below 0. Meanwhile, the Igeo of Cd, As, and Cu shows higher than other heavy metals. It is noteworthy that the Igeo of Cd was higher than all other heavy metals in the topsoil.
As for subsurface soils, the Igeo of Cd, Cr, Ni, Cu, Zn, As, Pb, and Hg was −0.90–3.57, −3.12–(−0.01), −2.51–1.73, −2.48–1.97, −0.78–2.07, −1.95–2.30, −2.28–0.52, and −3.76–1.80, respectively, (Supplementary Table S7). The mean Igeo of Cd (2.32), Cu (0.68), Zn (0.67), Ni (0.54), and As (0.26) was greater than 0, implying these metals were associated with the likely contaminant in the subsurface soils. Therein, Cd was the worst polluter among all heavy metals because it had only 1% of unpolluted, 19% moderately polluted, and 70% moderate to strongly polluted. Pb belongs to class 1, or uncontaminated to moderately unpolluted in terms of other heavy metals. It can be seen from Supplementary Figure S1B, Cr and Pb, most of which had values less than 0, indicate that these metals were unpolluted at most subsurface sites in this area. The Igeo of Ni, Cu, and Zn was greater than 0 in half of the total subsurface sampling sites, indicating that half of the sites were in a polluted status.
Potential Ecological Risks of Heavy Metals in Soils
The potential ecological risk assessment was performed by comparing relevant regional soil background values as an evaluation reference. The evaluation criteria for the ecological risk index RI and single metal (
RI represents the sensitivity of various biomes to toxic substances and demonstrates the ecological risks that heavy metals can pose. In terms of RI spatial distribution, sites with moderate or considerable RI values occupied most of the area in surface soils, wherein C03, C04, C05, and C06 represented very high risks (Supplementary Figure S2A). The RI comprehensive index values gradually reduced from northwest to southeast in surface soils. On the other hand, compared to surface soil, high ecological risks were observed in the C09 and C10 in subsurface soils (Supplementary Figure S2B). RI values were associated with the degree of anthropogenic disturbance and differed significantly through the sampling sites.
Health Risks Assessment of Heavy Metals in Soils
The health risks (non-carcinogenic and carcinogenic risks) were calculated for adults and children from heavy metals in soils from this area through different exposure pathways, including dermal contact, inhalation, and ingestion (Supplementary Table S10). The total hazard index (THI) and the TCR values were shown in Figure 5. Their carcinogenic risk indices were not evaluated because of the lack of corresponding SF values for Hg, Pb, Cu, and Zn. The THI values were 2.63 and 2.09, the TCR was 1.70E-03 and 1.63E-03 for adults and children, respectively. When 1.00E-06 < CR ≤ 1.00E-04, it means acceptable risk, CR > 1.00E-04 means the risk is unacceptable, while CR ≤ 1.00E-06 represents no significant risk. All hazard indexes (HIs) are greater than 1, indicating the potential for significant non-cancer effects to the public health risks associated with heavy metals (Ma et al., 2018). At the same time, the HI and CR values of Cd are shown in Figure 5. The relevant HI values were 2.54E-02 and 2.41E-02, the CR was 3.56E-06 and 3.03E-06 for adults and children, respectively.
The HI values were in the following order: As > Pb > Cr > Cd > Ni > Cu > Hg > Zn, showing that As was potentially highly deleterious to health (Supplementary Table S10). The As total exposure HI values for different population groups (adults and children) were 1.52E+00 and 1.51E+00, indicating that the public may experience significant non-carcinogenic health risks. It is well known that As in the ambient environment was mainly attributed to industrial activities. Moreover, the non-carcinogenic risks of heavy metals in this area were all lower than 1, except for As. As resulted in the highest HQdermal (Supplementary Table S10) for children and adults, and HQ values greater than 1 suggested that As had the highest non-carcinogenic risk. Among Ni, Zn, and Cu, only Zn had the lowest inhalation HQ values for children (2.61E-06) and adults (1.91E-06), HINi and HICu for children and adults based on total contents of metal were lower than the safe levels (HI ≤ 1). The HQs for all heavy metals in humans exposed to soil reduced in the order of dermal contact, ingestion, and inhalation. It suggested that dermal contact of soil was the most important exposure pathway, which was in line with a recent study (Li et al., 2017; Li et al., 2020).
Based on the current USEPA cancer slope factors, As, Ni, Cr, and Cd (considering ingestion, inhalation pathways, and dermal absorption) were estimated deeply (Supplementary Table S9). The CR toward adults and children was in the following order: Ni > Cr > As > Cd, and dermal absorption is higher than ingestion and inhalation pathways. The inhalation CR was below the reference value of USEPA (1.00E-04) (US EPA, 2002). However, the results of CR for single metal illustrated that ingestion exposure was mainly attributed to Cr, Ni, and As, even higher than the acceptable level. The CR of Cd (3.03E-06 for children and 3.56E-06 for adults) was generally higher than 1.00E-06 (accepted level), but lower than 1.00E-04, implying that carcinogenic risks could be considered acceptable. The total CR was several folds higher than the acceptable level (1.00E-06 < CR ≤ 1.00E-04) reaching 1.70E-03 for adults and 1.63E-03 for children. Therefore, people living in the study area were likely under higher carcinogenic risks.
Conclusion
The Cd was much higher than its BVs and national risk screening values for agricultural land, indicating that Cd would be enriched in the soil through human activities. Cd would pose contamination in soils with Igeo value and showed strong enrichment characteristics.
The areas of Cd concentrations that exceed the national risk screening value (0.3 mg/kg) in surface and subsurface soils were 833.17 and 903.06 km2, respectively, indicating up to 87% of the study area was contaminated by Cd.
Based on PCA analysis, the four PCs were cumulatively explained as 88.740% and 90.942% of the total variance in surface and subsurface soil. Four possible sources were determined and identified by the PMF model including natural sources, industrial activities, agricultural activities, and transportation.
The non-carcinogenic risks were all below the risk threshold for eight detected heavy metals. While total cancer risks for adults and children were 1.70E-03 and 1.63E-03, exceeding the acceptable limit. Therefore, there may be potential health risks associated with soil to the residents living in the study area.
Data Availability Statement
The original contributions presented in the study are included in the article/Supplementary Material, further inquiries can be directed to the corresponding author.
Author Contributions
CS: Formal analysis, data curation, writing–review and editing. JM: Investigation, formal analysis, and methodology. YZ: Investigation, formal analysis. RB: Formal analysis, writing–review and editing. ZC: Investigation, data curation. JD: Formal analysis. ZH: Formal analysis. ZK: Formal analysis, data curation. TW: Conceptualization, methodology, writing–review and editing, project administration, supervision.
Funding
This study was supported by the National Key R&D Program of China (Grant No. 2018YFC1800301), the National Natural Science Foundation of China (Grant Nos. 41877509, 41807493), and the STU scientific research foundation for talents (NTF20001).
Conflict of Interest
The authors declare that the research was conducted in the absence of any commercial or financial relationships that could be construed as a potential conflict of interest.
Publisher’s Note
All claims expressed in this article are solely those of the authors and do not necessarily represent those of their affiliated organizations, or those of the publisher, the editors, and the reviewers. Any product that may be evaluated in this article, or claim that may be made by its manufacturer, is not guaranteed or endorsed by the publisher.
Supplementary Material
The Supplementary Material for this article can be found online at: https://www.frontiersin.org/articles/10.3389/fenvs.2022.820536/full#supplementary-material
References
Amir, M., Bhuiyan, M., Karmaker, S., Bodrud-Doza, M., and Saha, B. B. (2020). Enrichment, Sources and Ecological Risk Mapping of Heavy Metals in Agricultural Soils of Dhaka District Employing Som, Pmf and Gis Methods. Chemosphere 263 (4), 128339. doi:10.1016/j.chemosphere.2020.128339
Barzegar, R., Asghari Moghaddam, A., Soltani, S., Fijani, E., Tziritis, E., and Kazemian, N. (2017). Heavy Metal(loid)s in the Groundwater of Shabestar Area (NW Iran): Source Identification and Health Risk Assessment. Expo. Health 11 (4), 251–265. doi:10.1007/s12403-017-0267-5
Chen, H., Teng, Y., Lu, S., Wang, Y., and Wang, J. (2015). Contamination Features and Health Risk of Soil Heavy Metals in China. Sci. Total Environ. 512-513, 143–1561. doi:10.1016/j.scitotenv.2015.01.025
Chen, T., Liu, X., Zhu, M., Zhao, K., Wu, J., Xu, J., et al. (2008). Identification of Trace Element Sources and Associated Risk Assessment in Vegetable Soils of the Urban-Rural Transitional Area of Hangzhou, China. Environ. Pollut. 151 (1), 67–78. doi:10.1016/j.envpol.2007.03.004
CNEMC (China National Environmental Monitoring Centre) (1990). The Backgrounds of Soil Environment in China. Beijing, China: China Environmental Science Press.
Dong, B., Zhang, R., Gan, Y., Cai, L., Freidenreich, A., Wang, K., et al. (2019). Multiple Methods for the Identification of Heavy Metal Sources in Cropland Soils from a Resource-Based Region. Sci. Total Environ. 651 (Pt 2), 3127–3138. doi:10.1016/j.scitotenv.2018.10.130
Fei, X., Lou, Z., Xiao, R., Ren, Z., and Lv, X. (2020). Contamination Assessment and Source Apportionment of Heavy Metals in Agricultural Soil through the Synthesis of PMF and GeogDetector Models. Sci. Total Environ. 747, 141293. doi:10.1016/j.scitotenv.2020.141293
Håkanson, L. (1980). An Ecological Risk index for Aquatic Pollution Control: a Sediment Ecological Approach. Water Res. 14, 975–1001. doi:10.1016/0043-1354(80)90143-8
Hu, W., Wang, H., Dong, L., Huang, B., Borggaard, O. K., Bruun Hansen, H. C., et al. (2018). Source Identification of Heavy Metals in Peri-Urban Agricultural Soils of Southeast China: An Integrated Approach. Environ. Pollut. 237, 650–661. doi:10.1016/j.envpol.2018.02.070
Huang, R.-J., Cheng, R., Jing, M., Yang, L., Li, Y., Chen, Q., et al. (2018b). Source-specific Health Risk Analysis on Particulate Trace Elements: Coal Combustion and Traffic Emission as Major Contributors in Wintertime Beijing. Environ. Sci. Technol. 52, 10967–10974. doi:10.1021/acs.est.8b02091
Huang, Y., Li, T., Wu, C., He, Z., Japenga, J., Deng, M., et al. (2015). An Integrated Approach to Assess Heavy Metal Source Apportionment in Peri-Urban Agricultural Soils. J. Hazard. Mater. 299, 540–549. doi:10.1016/j.jhazmat.2015.07.041
Jia, Y., Wang, L., Qu, Z., and Yang, Z. (2018). Distribution, Contamination and Accumulation of Heavy Metals in Water, Sediments, and Freshwater Shellfish from Liuyang River, Southern China. Environ. Sci. Pollut. Res. 25 (7), 7012–7020. doi:10.1007/s11356-017-1068-x
Jiang, Y., Chao, S., Liu, J., Yang, Y., Chen, Y., Zhang, A., et al. (2017). Source Apportionment and Health Risk Assessment of Heavy Metals in Soil for a Township in Jiangsu Province, China. Chemosphere 168, 1658–1668. doi:10.1016/j.chemosphere.2016.11.088
Jin, Y., O'Connor, D., Ok, Y. S., Tsang, D. C. W., Liu, A., and Hou, D. (2019). Assessment of Sources of Heavy Metals in Soil and Dust at Children's Playgrounds in Beijing Using GIS and Multivariate Statistical Analysis. Environ. Int. 124, 320–328. doi:10.1016/j.envint.2019.01.024
Komárek, M., Čadková, E., Chrastný, V., Bordas, F., and Bollinger, J.-C. (2010). Contamination of Vineyard Soils with Fungicides: A Review of Environmental and Toxicological Aspects. Environ. Int. 36, 138–151. doi:10.1016/j.envint.2009.10.005
Li, H.-H., Chen, L.-J., Yu, L., Guo, Z.-B., Shan, C.-Q., Lin, J.-Q., et al. (2017). Pollution Characteristics and Risk Assessment of Human Exposure to Oral Bioaccessibility of Heavy Metals via Urban Street Dusts from Different Functional Areas in Chengdu, China. Sci. Total Environ. 586, 1076–1084. doi:10.1016/j.scitotenv.2017.02.092
Li, L., Holm, P. E., Marcussen, H., and Bruun Hansen, H. C. (2014). Release of Cadmium, Copper and lead from Urban Soils of Copenhagen. Environ. Pollut. 187, 90–97. doi:10.1016/j.envpol.2013.12.016
Li, N., Kang, Y., Pan, W., Zeng, L., Zhang, Q., and Luo, J. (2015). Concentration and Transportation of Heavy Metals in Vegetables and Risk Assessment of Human Exposure to Bioaccessible Heavy Metals in Soil Near a Waste-Incinerator Site, South China. Sci. Total Environ. 521-522, 144–151. doi:10.1016/j.scitotenv.2015.03.081
Li, Y., Yuan, Y., Sun, C., Sun, T., Liu, X., Li, J., et al. (2020). Heavy Metals in Soil of an Urban Industrial Zone in a metropolis: Risk Assessment and Source Apportionment. Stoch Environ. Res. Risk Assess. 34 (2), 435–446. doi:10.1007/s00477-020-01779-z
Liang, J., Feng, C., Zeng, G., Gao, X., Zhong, M., Li, X., et al. (2017). Spatial Distribution and Source Identification of Heavy Metals in Surface Soils in a Typical Coal Mine City, Lianyuan, China. Environ. Pollut. 225, 681–690. doi:10.1016/j.envpol.2017.03.057
Liu, P., Hu, W., Tian, K., Huang, B., Zhao, Y., Wang, X., et al. (2020). Accumulation and Ecological Risk of Heavy Metals in Soils Along the Coastal Areas of the Bohai Sea and the Yellow Sea: A Comparative Study of China and South Korea. Environ. Int. 137, 105519. doi:10.1016/j.envint.2020.105519
Lu, Y., Song, S., Wang, R., Liu, Z., Meng, J., Sweetman, A. J., et al. (2015). Impacts of Soil and Water Pollution on Food Safety and Health Risks in China. Environ. Int. 77, 5–15. doi:10.1016/j.envint.2014.12.010
Luo, X.-S., Xue, Y., Wang, Y.-L., Cang, L., Xu, B., and Ding, J. (2015). Source Identification and Apportionment of Heavy Metals in Urban Soil Profiles. Chemosphere 127, 152–157. doi:10.1016/j.chemosphere.2015.01.048
Luo, X.-s., Yu, S., Zhu, Y.-g., and Li, X.-d. (2012). Trace Metal Contamination in Urban Soils of China. Sci. Total Environ. 421-422, 17–30. doi:10.1016/j.scitotenv.2011.04.020
Lv, J., Liu, Y., Zhang, Z., and Dai, J. (2013). Factorial Kriging and Stepwise Regression Approach to Identify Environmental Factors Influencing Spatial Multi-Scale Variability of Heavy Metals in Soils. J. Hazard. Mater. 261 (13), 387–397. doi:10.1016/j.jhazmat.2013.07.065
Ma, W., Tai, L., Qiao, Z., Zhong, L., Wang, Z., Fu, K., et al. (2018). Contamination Source Apportionment and Health Risk Assessment of Heavy Metals in Soil Around Municipal Solid Waste Incinerator: A Case Study in North China. Sci. Total Environ. 631-632, 348–357. doi:10.1016/j.scitotenv.2018.03.011
Men, C., Liu, R., Wang, Q., Guo, L., and Shen, Z. (2018). The Impact of Seasonal Varied Human Activity on Characteristics and Sources of Heavy Metals in Metropolitan Road Dusts. Sci. Total Environ. 637-638, 844–854. doi:10.1016/j.scitotenv.2018.05.059
Ministry of Environmental Protection of China (MEP) (2018). Ministry of Land and Resources of China (MLR)National Soil Pollution Investigation Bulletin. Available at http://www.mee.gov.cn/ywdt/tpxw/201905/t20190529_704841.shtml.
Nabulo, G., Young, S. D., and Black, C. R. (2010). Assessing Risk to Human Health from Tropical Leafy Vegetables Grown on Contaminated Urban Soils. Sci. Total Environ. 408 (22), 5338–5351. doi:10.1016/j.scitotenv.2010.06.034
Ning, Z., Liu, E., Yao, D., Xiao, T., Ma, L., Liu, Y., et al. (2021). Contamination, Oral Bioaccessibility and Human Health Risk Assessment of Thallium and Other Metal(loid)s in farmland Soils Around a Historic Tl Hg Mining Area. Sci. Total Environ. 758, 143577. doi:10.1016/j.scitotenv.2020.143577
Paatero, P. (1997). Least Squares Formulation of Robust Non-negative Factor Analysis. Chemometr. Intell. Lab. 37, 23e35. doi:10.1016/s0169-7439(96)00044-5
Peng, X., Shi, G., Liu, G., Xu, J., Tian, Y., Zhang, Y., et al. (2017). Source Apportionment and Heavy Metal Health Risk (HMHR) Quantification from Sources in a Southern City in China, Using an ME2-HMHR Model. Environ. Pollut. 221, 335–342. doi:10.1016/j.envpol.2016.11.083
Radziemska, M., and Fronczyk, J. (2015). Level and Contamination Assessment of Soil along an Expressway in an Ecologically Valuable Area in Central Poland. Ijerph 12 (10), 13372–13387. doi:10.3390/ijerph121013372
Rivera, M. B., Giraldez, M. I., and Fernandez-Caliani, J. C. (2016). Assessing the Environmental Availability of Heavy Metals in Geogenically Contaminated Soils of the Sierra de Aracena Natural Park (SW Spain). Is There a Health Risk?. Sci. Total Environ. 560–561, 254–265. doi:10.1016/j.scitotenv.2016.04.029
Shi, T., Ma, J., Zhang, Y., Liu, C., Hu, Y., Gong, Y., et al. (2019a). Status of lead Accumulation in Agricultural Soils across China (1979-2016). Environ. Int. 129, 35–41. doi:10.1016/j.envint.2019.05.025
Shi, T., Zhang, Y., Gong, Y., Ma, J., Wei, H., Wu, X., et al. (2019b). Status of Cadmium Accumulation in Agricultural Soils across China (1975-2016): From Temporal and Spatial Variations to Risk Assessment. Chemosphere 230, 136–143. doi:10.1016/j.chemosphere.2019.04.208
Shi, Y., Xu, X., Li, Q., Zhang, M., Li, J., Lu, Y., et al. (2018). Integrated Regional Ecological Risk Assessment of Multiple Metals in the Soils: A Case in the Region Around the Bohai Sea and the Yellow Sea. Environ. Pollut. 242 (Pt A), 288–297. doi:10.1016/j.envpol.2018.06.058
Sun, C., Liu, J., Wang, Y., Sun, L., and Yu, H. (2013). Multivariate and Geostatistical Analyses of the Spatial Distribution and Sources of Heavy Metals in Agricultural Soil in Dehui, Northeast China. Chemosphere 92 (5), 517–523. doi:10.1016/j.chemosphere.2013.02.063
Sun, Q. B., Yin, C. Q., Deng, J. F., and Zhang, D. F. (2013a). Characteristics of Soil-Vegetable Pollution of Heavy Metals and Health Risk Assessment in Daye Mining Area. Environ. Chem. 32, 671–677.
Sun, Y., Zhou, Q., Xie, X., and Liu, R. (2010). Spatial, Sources and Risk Assessment of Heavy Metal Contamination of Urban Soils in Typical Regions of Shenyang, China. J. Hazard. Mater. 174, 455–462. doi:10.1016/j.jhazmat.2009.09.074
Tao, S., Ru, M. Y., Du, W., Zhu, X., Zhong, Q. R., Li, B. G., et al. (2018). Quantifying the Rural Residential Energy Transition in China from 1992 to 2012 through a Representative National Survey. Nat. Energ. 3, 567–573. doi:10.1038/s41560-018-0158-4
Teng, Y., Wu, J., Lu, S., Wang, Y., Jiao, X., and Song, L. (2014). Soil and Soil Environmental Quality Monitoring in China: a Review. Environ. Int. 69, 177–199. doi:10.1016/j.envint.2014.04.014
Tian, K., Wu, Q., Liu, P., Hu, W., Huang, B., Shi, B., et al. (2020). Ecological Risk Assessment of Heavy Metals in Sediments and Water from the Coastal Areas of the Bohai Sea and the Yellow Sea. Environ. Int. 136, 105512. doi:10.1016/j.envint.2020.105512
Tume, P., Roca, N., Rubio, R., King, R., and Bech, J. (2018). An Assessment of the Potentially Hazardous Element Contamination in Urban Soils of Arica, Chile. J. Geochemical Exploration 184, 345–357. doi:10.1016/j.gexplo.2016.09.011
USEPA (1997). Exposure Factors Handbook. Washington DC: Office ofresearch and development, national center for environmental assessment.
USEPA (2016). Regional Screening Levels (RSLs) User’s Guide. Available on https://www.epa.gov/expobox/about-exposure-factors-handbook.
USEPA (2009). Risk Assessment Guidance for Superfund Volume I. Washington, D.C: Human Health Evaluation Manual.
USEPA (2002). Supplemental Guidance for Developing Soil Screening Levels for Superfund Sites. Washington, DC: Environmental Protection Agency.
Wang, H., Wu, Q., Hu, W., Huang, B., Dong, L., and Liu, G. (2018). Using Multi-Medium Factors Analysis to Assess Heavy Metal Health Risks along the Yangtze River in Nanjing, Southeast China. Environ. Pollut. 243 (Pt B), 1047–1056. doi:10.1016/j.envpol.2018.09.036
Wang, Y., Duan, X., and Wang, L. (2020). Spatial Distribution and Source Analysis of Heavy Metals in Soils Influenced by Industrial enterprise Distribution: Case Study in Jiangsu Province. Sci. Total Environ. 710, 134953. doi:10.1016/j.scitotenv.2019.134953
Wei, B., and Yang, L. (2010). A Review of Heavy Metal Contaminations in Urban Soils, Urban Road Dusts and Agricultural Soils from China. Microchemical J. 94 (2), 99–107. doi:10.1016/j.microc.2009.09.014
Xue, J.-l., Zhi, Y.-y., Yang, L.-p., Shi, J.-c., Zeng, L.-z., and Wu, L.-s. (2014). Positive Matrix Factorization as Source Apportionment of Soil lead and Cadmium Around a Battery Plant (Changxing County, China). Environ. Sci. Pollut. Res. 21 (12), 7698–7707. doi:10.1007/s11356-014-2726-x
Yadav, I. C., Devi, N. L., and Singh, S. (2014). Spatial and Temporal Variation in Arsenic in the Groundwater of Upstream of Ganges River Basin, Nepal. Environ. Earth Sci. 73 (3), 1265–1279. doi:10.1007/s12665-014-3480-6
Yang, Q., Li, Z., Lu, X., Duan, Q., Huang, L., and Bi, J. (2018). A Review of Soil Heavy Metal Pollution from Industrial and Agricultural Regions in China: Pollution and Risk Assessment. Sci. Total Environ. 642, 690–700. doi:10.1016/j.scitotenv.2018.06.068
Zang, F., Wang, S., Nan, Z., Ma, J., Zhang, Q., Chen, Y., et al. (2017). Accumulation, Spatio-Temporal Distribution, and Risk Assessment of Heavy Metals in the Soil-Corn System Around a Polymetallic Mining Area from the Loess Plateau, Northwest China. Geoderma 305, 188–196. doi:10.1016/j.geoderma.2017.06.008
Zhang, P., Qin, C., Hong, X., Kang, G., Qin, M., Yang, D., et al. (2018). Risk Assessment and Source Analysis of Soil Heavy Metal Pollution from Lower Reaches of Yellow River Irrigation in China. Sci. Total Environ. 633, 1136–1147. doi:10.1016/j.scitotenv.2018.03.228
Zhao, H., Xia, B., Fan, C., Zhao, P., and Shen, S. (2012). Human Health Risk from Soil Heavy Metal Contamination under Different Land Uses Near Dabaoshan Mine, Southern China. Sci. Total Environ. 417-418, 45–54. doi:10.1016/j.scitotenv.2011.12.047
Zhao, L., Xu, Y., Hou, H., Shangguan, Y., and Li, F. (2014). Source Identification and Health Risk Assessment of Metals in Urban Soils Around the Tanggu Chemical Industrial District, Tianjin, China. Sci. Total Environ. 468, 654–662. doi:10.1016/j.scitotenv.2013.08.094
Keywords: heavy metals, spatial distribution, source apportionment, risk assessment, soil
Citation: Su C, Meng J, Zhou Y, Bi R, Chen Z, Diao J, Huang Z, Kan Z and Wang T (2022) Heavy Metals in Soils From Intense Industrial Areas in South China: Spatial Distribution, Source Apportionment, and Risk Assessment. Front. Environ. Sci. 10:820536. doi: 10.3389/fenvs.2022.820536
Received: 23 November 2021; Accepted: 10 January 2022;
Published: 14 February 2022.
Edited by:
Peter S. Hooda, Kingston University, United KingdomReviewed by:
Guodi Zheng, Institute of Geographic Sciences and Natural Resources Research (CAS), ChinaAn Liu, Shenzhen University, China
Copyright © 2022 Su, Meng, Zhou, Bi, Chen, Diao, Huang, Kan and Wang. This is an open-access article distributed under the terms of the Creative Commons Attribution License (CC BY). The use, distribution or reproduction in other forums is permitted, provided the original author(s) and the copyright owner(s) are credited and that the original publication in this journal is cited, in accordance with accepted academic practice. No use, distribution or reproduction is permitted which does not comply with these terms.
*Correspondence: Tieyu Wang, wangt@stu.edu.cn