- 1Geography Department, Humboldt-Universität zu Berlin, Berlin, Germany
- 2Department of Monitoring and Exploration Technologies, Helmholtz Centre for Environmental Research—UFZ, Leipzig, Germany
- 3Department of Urban and Environmental Sociology, Helmholtz Centre for Environmental Research—UFZ, Leipzig, Germany
In times of urbanization and climate change, urban green spaces and their ecosystem services are pivotal for adapting to extreme weather events such as heat and drought. But what happens to the provision of ecosystem services when green spaces themselves are compromised by heat and drought? In this study, we assessed the air temperature regulation by two structurally distinct inner-city parks in Leipzig, Germany, that were strongly affected by the heat and drought periods in 2018 and 2019. We used a dense network of in-situ measurements and machine learning to create spatially explicit maps of air temperature distribution at a fine-scale neighborhood level for a 24 h period during the summer heat wave in 2019. The results showed that the larger, tree-dominated park could maintain spaces of relative coolness at any time, whereas the other rather open, grass-dominated park was strongly heated during the daytime but provided relatively cool air during the night and early morning. We found a maximum spatially averaged cooling effect of green spaces versus the built-up surroundings of 1.1°C in the morning. In the afternoon, however, when air temperatures peaked at nearly 40°C, cooling was limited to shaded areas, leading to average differences between green spaces and built-up surroundings below 1°C. Our spatially explicit maps indicated that the “cooling distances” of green spaces, i.e., cooling effects that extend beyond the boundaries of a park, are negligible during dry and hot conditions. We conclude that vegetation structure, particularly the configuration of trees, is key to designing heat and drought-resilient green spaces and thus maintaining ecosystem service provision under the challenges of climate change. Urban planning needs to account for larger green spaces that are complemented by decentralized, well-distributed small-scale green infrastructure that intersperses the built infrastructure such as roadside greenery and vegetated backyards.
Introduction
Climate change increases the occurrence of extreme weather events worldwide. Heat waves, dry periods, storms and heavy rain are projected to increase in number and severity in upcoming decades (IPCC, 2021). In Europe, unprecedented heat waves occurred in the recent past, and further increases in frequency and intensity are expected with more hot days exceeding 30°C and tropical nights with nighttime temperatures not falling below 20°C particularly in cities (Guerreiro et al., 2018; Zhang et al., 2020; European Environment Agency (EEA) 2021).
Urban areas are considered hotspots of climate change, simultaneously acting as major drivers of greenhouse gas emissions and being particularly vulnerable to climate change effects (Bulkeley, 2013; McDonald et al., 2018; Kumar, 2021; Nature Editorial, 2021). Globally ongoing urbanization (UN, 2019), with increases in population densities, traffic, impervious surfaces and the loss of open green and blue spaces exacerbates warming trends with mean temperatures in cities often being higher than those in surrounding suburban or rural areas (urban heat island effect) (Oke, 1982; Zhao et al., 2018). The combination of both ongoing urbanization and the more frequent occurrence and severity of extreme weather events, such as heatwaves, have significant impact on human health and wellbeing (Ciscar et al., 2018; Raymond et al., 2020; WHO, 2021). The 2003 heatwave caused more than 70,000 heat-related deaths in Europe with 7,600 cases in Germany alone (Robine et al., 2008; An der Heiden et al., 2019a). For the summer heat period in 2018, 490 heat-related deaths were estimated for the German capital Berlin (An der Heiden et al., 2019b).
To adapt to and mitigate the challenges of climate change and urbanization, cities increasingly rely on urban green spaces and their associated ecosystem services (McDonald et al., 2018; Veerkamp et al., 2021). In particular, urban green spaces help regulate the microclimate through shading, evapotranspiration and air flow regulation (Lehmann et al., 2014; Rahman et al., 2020; Meili et al., 2021). The potential of urban green spaces to regulate the microclimate through cooling was shown to be close to 1°C during the daytime in parks and over 1.5°C in urban forests with dense tree canopies compared to urban surroundings (Bowler et al., 2010; Knight et al., 2021). Dense vegetation cover, however, may reduce wind velocity and heat emission (radiative cooling) at night (Yuan et al., 2017; Knight et al., 2021).
Studies on the microclimate effect of urban green areas can be broadly categorized into empirical and modeling studies (Bartesaghi Koc et al., 2018; Knight et al., 2021). Empirical data are gathered through both remote sensing approaches and in-situ observations. Remote sensing-based assessments are applicable at large spatial scales, provide spatially explicit information, and are easily transferable. However, they are used to analyze surface radiation (and derived surface temperatures); thus, they mostly lack direct measurements of air temperature or other aspects of thermal comfort, such as wind or air humidity (Weber et al., 2014; Kremer et al., 2018; Schwaab et al., 2021). Due to radiometric/physical constraints, temperature data and analyses based on satellite remote sensing have coarse spatial resolutions that are less suitable for fine-scale assessment, e.g., at the neighborhood level.
In addition to remote sensing approaches, in-situ observations have been used in empirical studies to provide direct measurements of meteorological parameters such as air temperature. However, no standardized protocols have been applied or reported, which makes it difficult to compare the findings of different studies (Bartesaghi Koc et al., 2018). For example, Jansson et al. (2007) measured air temperature at 1.2 and 2.5 m heights along (bicycle) routes, whereas Yu and Hien (2006) applied stationary measurements at a 2 m height. Both studies were given a heavily weighted effect size in the meta-analysis by Bowler et al. (2010). There is a limited number of studies converting point or transect observations into spatially explicit maps to allow for air temperature assessments at any point of interest. One urban study on a local scale mapped area-wide air temperatures using regression models, including machine learning (Ho et al., 2014), whereas further examples can be found on a rather large continental scale (e.g., Marando et al., 2022).
Next to empirical studies, urban climate modeling or simulation approaches often use software packages such as ENVI-met or Urban InVEST to produce spatially explicit maps of meteorological parameters based on reference observations or synthetic data (Bartesaghi Koc et al., 2018; Bosch et al., 2021). These models downscale coarse reference data such as official regional radiation or temperature data by using established spatio-physical relationships to link them with land cover and urban structural elements (Lehmann et al., 2014; Gromke et al., 2015). Such modeling approaches use standardized parameters of categorical input data, e.g., transpiration values for the “tree” category, but cannot account for a natural continuum of green in terms of morphology or physiology (Rahman et al., 2020) and hardly consider variations in vegetation health caused by, e.g., extreme weather events.
In addition to the spatial diversity in the microclimate regulation service of urban green spaces, ecosystem service provision by vegetation is known to also vary over time, for example, due to seasonal variations or vegetation growth processes (Willemen, 2020). The cooling potential of vegetation can be substantially compromised in the short term, e.g., when transpiration is reduced during heat or drought events, and in the longer term when stressed vegetation becomes prone to pests and diseases or may even be permanently damaged through the repeated occurrence of heat and drought (Dale and Frank, 2017; Meili et al., 2021; Pace et al., 2021; Schnabel et al., 2021). Marando et al. (2019) and Allen et al. (2021) demonstrated clear effects of drought on vegetation vitality and associated land surface temperatures on a broader city/agglomeration level. However, fine-scale empirical studies that account for the reduction in cooling performance of individual urban green spaces, such as parks, due to heat and drought are scarce. We are aware of one study that assessed drought impacts on two tree species and associated ecosystem services in an urban setting (Rötzer et al., 2021). Therefore, further research is needed at a fine spatial scale to assess the potential of urban green spaces for sustaining microclimate regulation services under heat and drought conditions.
The main aim of this study is to assess the air temperature regulation by two differently structured inner-city parks that were affected by two extreme heat and drought periods in Central Europe in 2018 and 2019. In particular, the objectives are to:
• Use a dense network of standardized in-situ air temperature measurements,
• Apply a machine learning-based modeling technique to create spatially explicit air temperature maps at the fine-scale urban park and neighborhood levels for a 24 h period on one of the hottest days during the summer heat wave in Central Europe in 2019, and
• Derive estimates for the cooling effect of two major, structurally distinct public urban parks.
Materials and Methods
Study Area
We conducted our study in Leipzig, a large city in central Germany. Leipzig is affected by climate change and the resulting extreme weather events. The region is a core area for recent and projected numbers of hot summer days (Tmax >30°C) and drought periods in Germany (Bernhofer et al., 2015; Guerreiro et al., 2018; Potsdam Institute for Climate Impact Research (PIK), 2021). From 2018 to 2020, a perennial drought period occurred with particularly low precipitation in spring and early summer (Boergens et al., 2020; Helmholtz Centre for Environmental Research (UFZ), 2021). The resulting water deficit was accompanied by several unprecedented summer heat waves in 2018 and 2019. In Leipzig, precipitation for the months April to June summed to 107 mm in 2018 and 95 mm in 2019 compared to the long-term mean (1985–2015) of 158 mm for the same period (Figure 1). The mean temperatures for June and July were 20.3 and 21.0°C, respectively, markedly above the long-term mean of 18.1°C. On a national scale, June 2019 was the hottest ever recorded for Germany (Meinert et al., 2019). As a result, urban green spaces were severely damaged: grasslands were degraded, hundreds of mainly mature trees were lost and had to be cut in Leipzig alone, and numerous ponds temporally dried (cf. Figure 1, City of Leipzig, 2019a; City of Leipzig, 2019b).
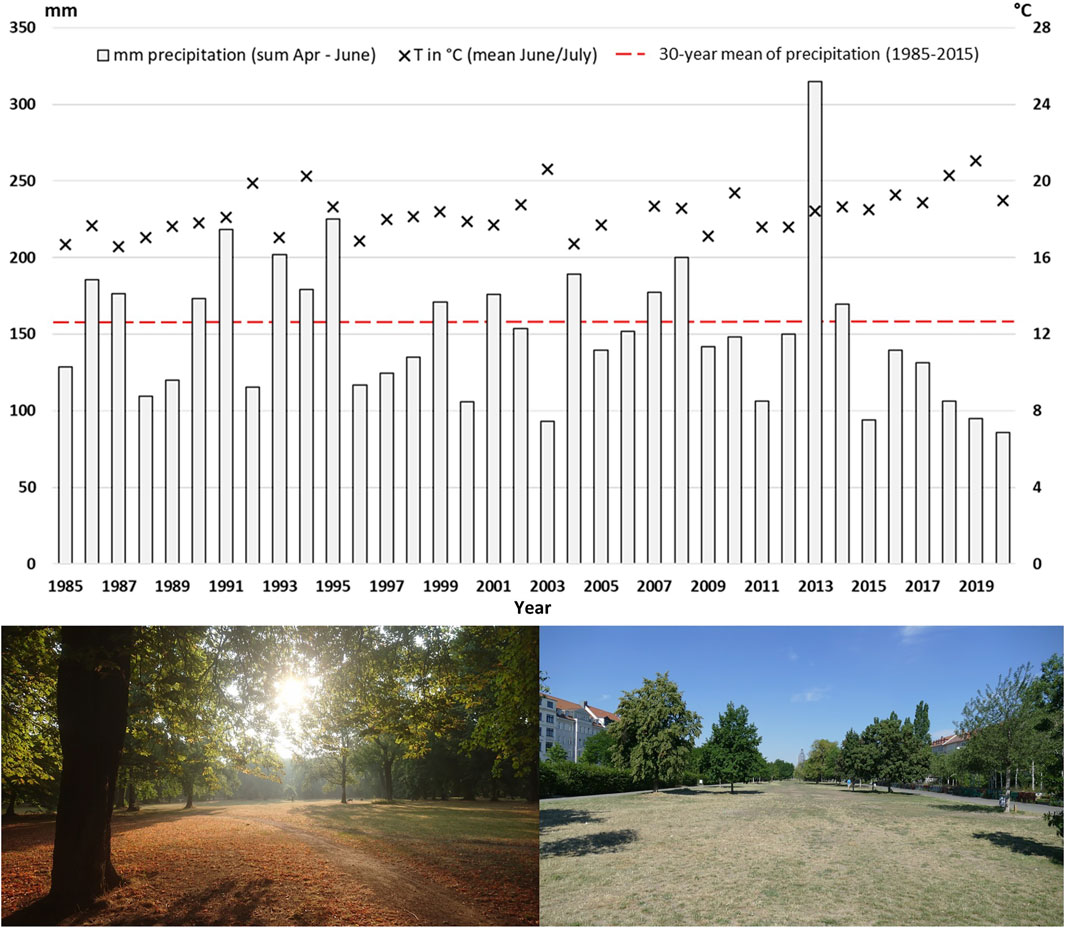
FIGURE 1. Climate data for Leipzig (retrieved from Deutscher Wetterdienst, Climate Data Center (CDC), 2021, station 2928 “Leipzig-Holzhausen”) and impressions from summer 2018 and 2019. Bottom left: early foliage shedding of trees due to drought and heat stress (Photo taken on 23 July 2018 by the authors). Bottom right: degradation of grass cover due to repeated, perennial drought (Photo taken on 6 July 2019, extracted from Kabisch et al., 2021).
Leipzig is a growing city. The population increased from 509.000 in 2010 to 605,000 in 2020, and an ongoing increase is projected for the next decade (Wolff et al., 2016; Heinemann et al., 2019). Nevertheless, Leipzig has a significant share of green and blue spaces. Nearly half of the city’s area is covered by vegetation (45%, 13,415 ha, excluding agricultural areas), of which 36% (16% of the city) is trees taller than 5 m in height (Banzhaf and Kollai, 2018). A ramified network of rivers and channels traverses through the city and former surface mining areas and gravel pits were restored to create numerous lakes and recreational areas in the immediate hinterland.
For our fine-scale air temperature assessment, we focused on the combined area of two inner-city parks—Friedenspark and Lene-Voigt-Park—and their immediate vicinity of 2.5 km (Figure 2). The area is located approximately 650 m southeast of the city center. The parks are distinct in size, shape, vegetation structure and facilities (Kraemer and Kabisch, 2021). The Friedenspark is an old green space that was initially developed as a cemetery in the mid-19th century. The park has a size of 17.5 ha and is dominated by mature trees (61% tree coverage, mean height >13 m). In contrast, the Lene-Voigt-Park, 5.8 ha in size, is a new green space developed on a former railway brownfield that was fully opened to the public in 2004. With 78% total vegetation and 14% tree cover, the park is relatively sparsely vegetated, and trees have an average height of 8 m. Located in a densely populated residential area and hosting several leisure facilities, the Lene-Voigt-Park is heavily used by the public, leading to additional impairment of grass areas (cf. Figure 1).
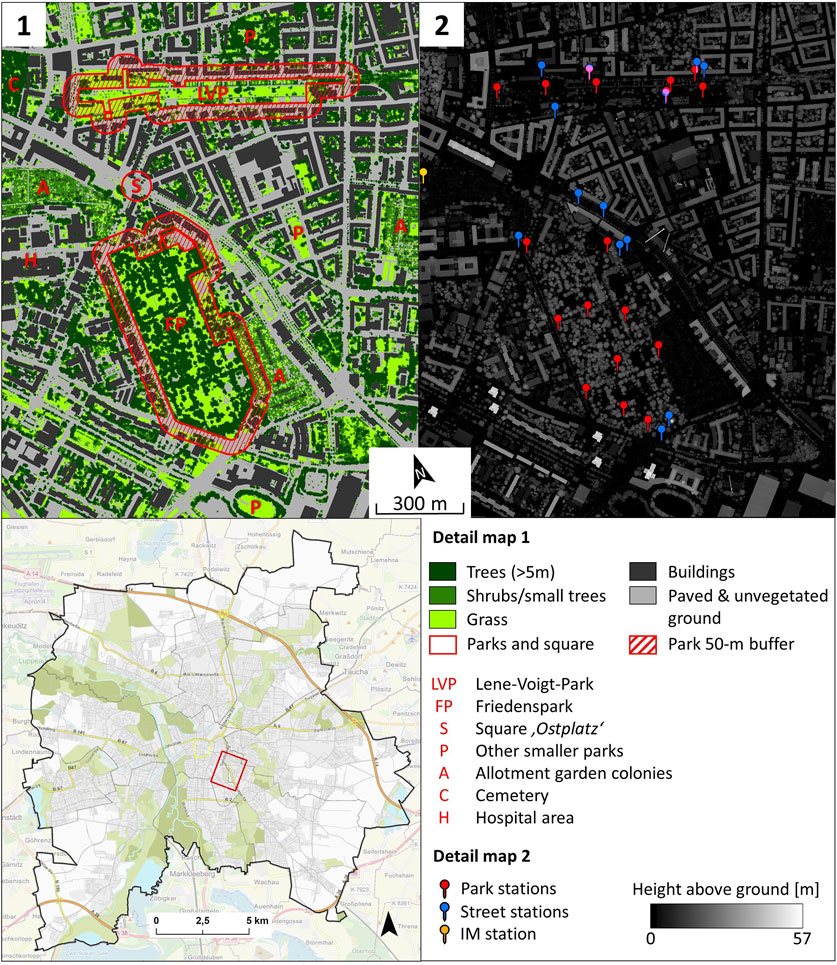
FIGURE 2. Bottom left: Location of the study area (red rectangle) in the city of Leipzig. Detail map 1: Study area with land cover, delineated zones for cooling assessment, and further areas of interest. Detail map 2: Study area with location of meteorological data loggers (for further details of data loggers see Supplementary Material S1). IM—Institute of Meterorology of the Leipzig University. Map data: OpenStreetMap contributors, Stadt Leipzig—Amt für Geoinfromation und Bodenordnung, Banzhaf and Kollai, 2018, GeoSN, 2018.
The total study area comprises 250 ha and covers a variety of urban grey and green structures including other smaller parks, allotment garden colonies, brownfields, one cemetery, Wilhelminian style residential blocks in the northeast (approximately 20 m high) and high-rise buildings over 50 m in height (Figure 2). Approximately 44% of the area is covered by vegetation, of which nearly 50% is trees. Buildings cover one-fifth (50 ha) of the area. The remaining 90 ha or 36% is covered by different non-vegetated surfaces such as bare soils, streets, sidewalks and other artificial surfaces. The terrain of the study area is flat with an absolute south-north gradient of approximately 15 m, corresponding to 6.5 m/km. Air conditioning in buildings is, thus far, not very common in Germany (UBA, 2020) and in our study area can be found on only a few office buildings west of the Friedenspark and the hospital complex in the east (marked with H in Figure 2). All of these air conditioning units are installed on the rooftops of the buildings, thus having only a minor impact on near-surface air temperatures (Jin et al., 2020).
We created a 50-m buffer around the two parks to identify air temperature contrasts between the parks and the adjacent neighborhoods with urban street and building areas (Figure 2). We also selected a highly frequented street area (Ostplatz), a junction of two main roads, located between the two parks to illustrate the potential air temperature difference between the park areas and a dense street environment. We delineated the Ostplatz by applying a 50-m buffer around the approximated center of the junction.
Data
In-Situ Meteorological Data
We collected in-situ data during an extensive field campaign on eight consecutive days, from 26 June to 3 July 2019, as part of the GreenEquityHEALTH research project (Kabisch et al., 2021). During this campaign, we installed a total of 18 (solar-shielded) temperature loggers within both major parks, Friedenspark and Lene-Voigt-Park, and 13 unshielded temperature loggers in nearby street spaces (for details, Supplementary Material S1 and the supplementary material to Kabisch et al., 2021). All sensors were installed at a 2 m height, in accordance with guidelines from the German Meteorological Service (Löffler, 2012) that are in line with international standards (World Meteorological Organization (WMO), 2018). The data-logging interval was 15 min. Data from unshielded sensors were quality checked and cleaned for radiation impact using two unshaded but shielded temperature sensors as references. Cleaning and exclusion of data points are necessary for unshielded sensors because in the case of direct insolation, measured values correspond to the device’s temperature rather than the actual surrounding air temperature. We intercalibrated all sensors directly after the campaign using long-term measurements under equal indoor conditions. We used these laboratory measurements to derive the calibration value for each sensor defined as the deviation of the specific sensor mean from the overall mean across all sensors multiplied by −1 (adverse value).
We also acquired meteorological data from the Institute of Meteorology of the Leipzig University (IM) located in the east of our study area (Figure 2), which provided us with additional air temperature measurements as well as information on wind speed and direction. In total, we acquired air temperature data from 32 locations in our study area of 250 ha, which corresponds to a density of 1 sensor/7.8 ha or 1 sensor/280 × 280 m grid.
The weather conditions during the campaign were characterized by two consecutive heat waves (26–27 June and 29 June–1 July 2019) peaking with daily high temperatures above 35°C and tropical nights, i.e., temperatures not dropping below 20°C (Figure 3). The IM station also recorded notably low values of relative air humidity, dropping below 15% on 30 June. Such dry air may reduce heat stress for people but increases the potential evapotranspiration of soils and vegetation, thus additionally aggravating drought conditions. For our analysis, we chose a 24 h period, from 6:00 h on 30 June to 6:00 h on 1 July, covering the hottest day and one of the warmest nights in 2019, with air temperatures of nearly 40°C during the day and above 20°C at night (Figure 3). Wind conditions were relatively calm throughout, with wind speeds between 4 and 5 m/s (wind force 3, “gentle breeze”) during the day and between 1 and 4 m/s at night. The major wind direction was southwest to northwest.
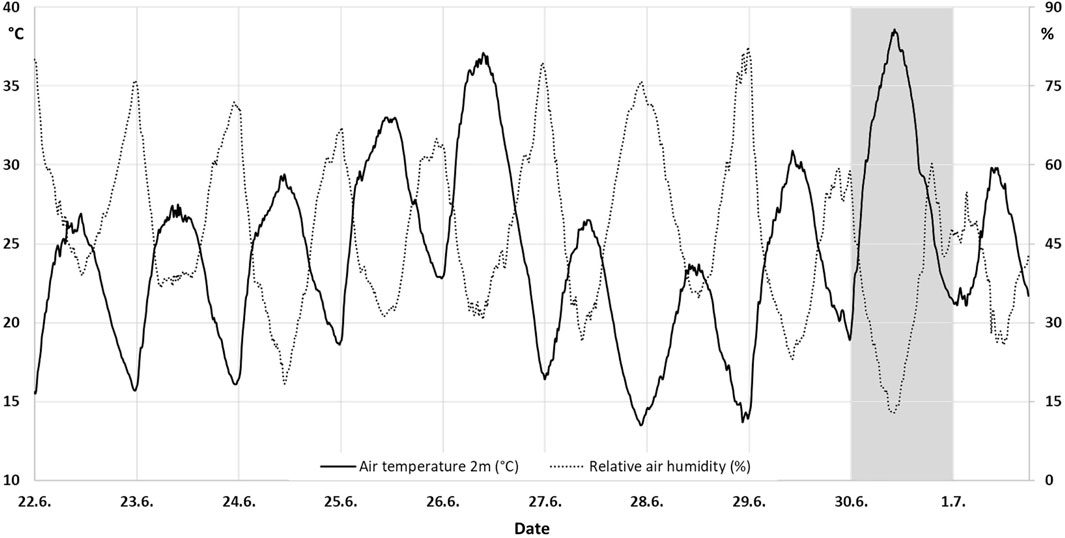
FIGURE 3. Air temperature (left axis) and air humidity (right axis) curves showing two major heat waves in June/July 2019 in Leipzig. Vertical lines are drawn at 6:00 h in the morning, respectively. The grey background indicates the 24 h study period. Data by meteorological station of Leipzig University.
Spatial Data for Predicting Air Temperatures
As spatial predictors for our spatially-explicit air temperature models, we acquired high-resolution remote sensing and land-cover data (Table 1). To obtain true object heights, mainly of trees and buildings, we created a normalized digital surface model (nDSM) by subtracting the digital elevation model (DEM, representing natural landscape elevation above sea level without objects) from the original digital surface model (DSM, representing the landscape elevation above sea level with objects such as trees or buildings).
The land cover data (Banzhaf and Kollai, 2018) were generalized (reclassified) to five land-cover classes, namely, trees, shrubs (incl. small trees <5 m), grass, unvegetated or sealed surfaces and one class comprising all kinds of buildings, from high-rise buildings to garden bowers. To supplement the categorical land cover data for 2012, not distinguishing between dense or healthy and degraded grass cover, and to account for the drought and heat conditions in 2019 (and damages caused by the 2018’s drought) we included airborne imagery (color-infrared digital orthophotos) with fine-grained spectral information recorded in July 2018. To improve vegetation detection, also in shaded areas, we derived the Normalized Difference Vegetation Index (NDVI) using the near-infrared and the red spectral bands (cf. Iovan et al., 2008).
Predicting Air Temperatures Using Random Forest Regression
We used random forest regression, a supervised machine learning approach, to predict hourly spatially explicit maps of air temperature for the study area (Breiman, 2001). Random forests are commonly applied in spatial modelling, often outperforming traditional statistical or other machine learning methods and also proved to be suitable for predicting air temperatures (Li et al., 2011; Ho et al., 2014; Sekulić et al., 2020). For every hour, from 6:00 h on 30 June to 6:00 h on 1 July, one random forest model was fitted using the respective temperature data (measurement points) for training and the land-cover data, the normalized digital surface model, the NDVI (derived from the orthophotos) and the digital elevation model as explanatory variables (predictors). To account for shading, we also included the blue band of the orthophotos. However, since the orthophotos were recorded at one certain point of time (ca. 15:30 h), we included the blue band only for the models from 14:00 to 17:00 h, when shadow effects were similar to those at the recording time. Random forest regression was performed using the “Forest-based Classification and Regression” tool in ArcGIS Pro v2.8 (Esri 2021). In total, we ran 25 models (one model per hour, from 6:00 to 6:00 h) to predict hourly air temperatures at a 2 m height in a regular spatial grid with a 1-m resolution.
Model Validation
For validation, we excluded 25% of the measurement points from training. However, due to the limited number of (valid) measurement points - from a machine-learning perspective - (minimum: 19, maximum: 32) we took data for independent validation only when at least 28 points were available, i.e., when a maximum of four points were excluded due to direct solar radiation (Supplementary Materials S1, S2 for details). Hence, we could validate 12 out of 25 models with only the model performance itself and applied Out-Of-Bag (OOB) statistics, including the mean standard error and R2. We also assessed the absolute temperature offsets of each model by comparing the overall predicted air temperature amplitudes (ranging between the minimum and maximum) with amplitudes measured at the same time in the field. We calculated the total relative offset as the ratio between the absolute offset (difference between measured and predicted amplitudes) and the measured amplitude. Hence, a ratio of one corresponds to a model that underestimates the actual measured temperature amplitude by 100%; i.e., the model itself would have a zero amplitude.
Quantification of Cooling by Urban Green Under Drought and Heat
To assess whether and to what extent urban green spaces regulate the air temperature under conditions of drought and heat, we calculated zonal statistics of predicted air temperatures for both public parks in our study area (Friedenspark and Lene-Voigt-Park), their 50-m buffer zones and for the dense street area (Ostplatz square). To test for significance differences between these five zones, we ran an analysis of variance (ANOVA) with “air temperature” as the independent variable. For the ANOVA, we randomly sampled 500 points/park and buffer and 50 points for the square (minimum distance of 2 m to avoid adjacent cells/pixels) and extracted cell values from the predicted air temperature raster datasets.
Results
Model Selection Based on Evaluation of Random Forest Regressions
Following the performance evaluation of the random forest regressions (for details, Supplementary Material S2), we selected four representative models for our spatial assessment that were well distributed over the 24 h period (Table 2; Figure 4).1 Model T2 at 08:00 h in the morning with highest R2 values and lowest relative offsets, covers the intense heating period during early morning, approximately 3 h after sunrise, when air temperatures rise about 3–4°C/h. The second model at 15:00 h (T9) covers a period shortly before the temperature peak in the afternoon. With the third model at 21:00 h (T15), we cover the evening approximately 30 min before sunset, when air temperatures sharply drop by up to 4°C/h. Finally, the fourth model at 6:00 h (T24) covers the period of minimum air temperatures early in the morning of 1 July.
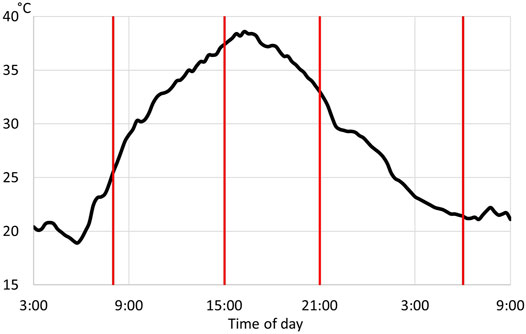
FIGURE 4. Air temperature curve (black line) from 3:00 h on 30 June to 9:00 h on 1 July 2019 and temporal distribution of selected random forest models (red vertical lines). Air temperature profile corresponds to weather station of the Leipzig University (IM station, cf. Figure 2).
Temporal and Spatial Air Temperature Patterns
The results of the first model at 8:00 h showed air temperatures in the study area ranging from 22.8°C up to 25.0°C (23.9°C mean), equaling to a total amplitude of 2.2°C (Table 2; Figure 5, Supplementary Material S3 for absolute values). Compared to the measured values in the field the model underestimated minimum temperatures by 0.35°C and maximum temperatures by −0.51°C. As for an overall spatial gradient, we found the highest air temperatures in the northern part of the study area, which is closest to the city center and where building density is particularly high. In any part of the area, however, coolest spots corresponded to taller, shading vegetation (shrubs and trees) where air temperatures were approximately 0.5°C lower than those in street zones in the vicinity. As spatially extensive “cold spots”, we could identify allotment garden colonies in the southeast (next to Friedenspark) and at the eastern edge of the study area. Here, maximum cooling effects above 1°C compared to surrounding streets were modeled. Additionally, vegetated backyards also appeared as small-scale cool zones in contrast to the sealed and treeless front sides of buildings.
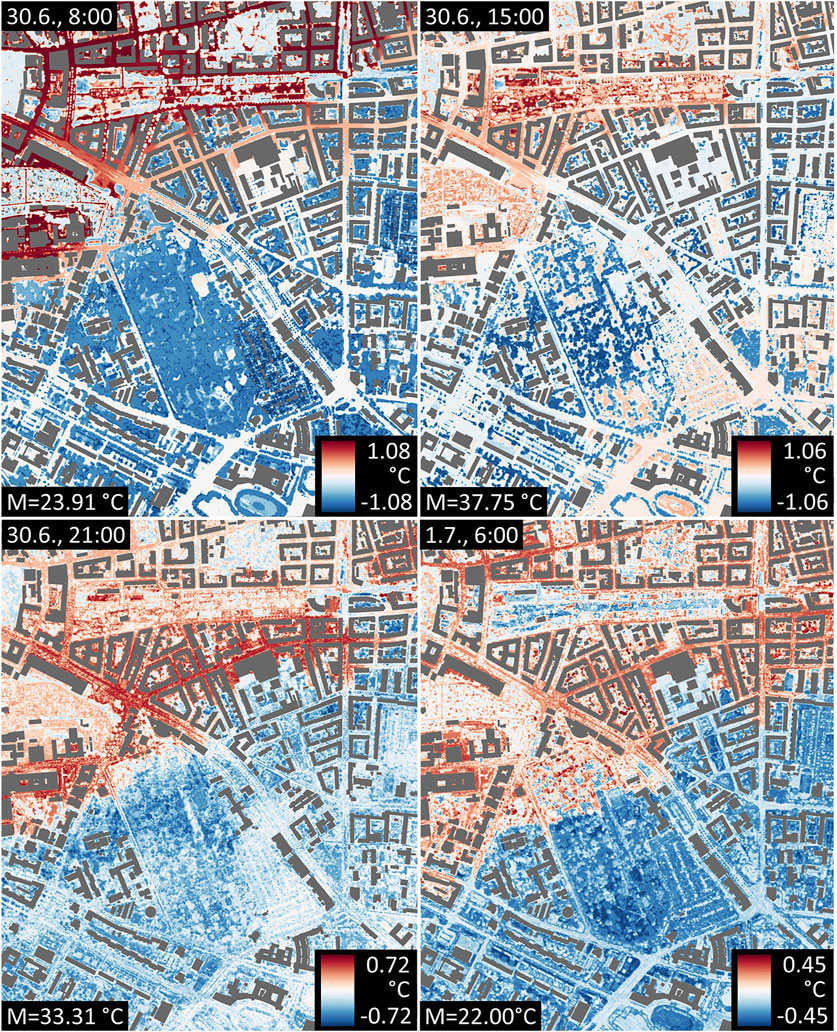
FIGURE 5. Air temperature maps for the four selected points in time depicting deviation from respective mid-range values (central value between minimum and maximum), indicated by M. For representation of absolute values, please see Supplementary Material S3.
For the early afternoon at 15:00 h we modeled air temperatures between 36.7 and 38.8°C (37.7°C mean, 2.1°C amplitude), with values being underestimated by approximately ± 0.5°C, respectively. Temperature gradients were locally confined, with large open spaces being warmer than smaller spaces where shading was prevalent. Notably, the Lene-Voigt-Park and its vicinity appeared as a zone with the highest air temperatures. In fact, we measured values above 39°C during our field campaign only in and around the Lene-Voigt-Park (Supplementary Material S1). In contrast to this hot zone, many vegetated backyards and wider strips of roadside greenery seemed to be sustained as micro-zones of relative coolness. However, significant cooling effects at a larger scale remained only in areas with coherent tree canopies such as in the Friedenspark where air temperatures could be reduced by more than 1°C compared to those in surrounding open spaces. In contrast, areas between trees, e.g., in the Friedenspark, also appeared as traps of warm air, which might be attributable to impaired ventilation.
The results for the evening of 30 June at 21:00 h revealed an overall north-south gradient of air temperatures, similar to the morning model. At 21:00 h, larger scale temperature differences (between the north and the south) dominated compared to fine-scale differences (e.g., between adjacent trees and street areas). Thus, at this time of the day, the vegetation structure seems to have much less impact on spatial air temperature patterns than, e.g., at 15:00 h. In the northern part, the Ostplatz, adjacent streets, and the area around the university hospital (cf. Figure 2) at the western edge appeared as the hottest locations within our study area with air temperatures of approximately 34°C. In contrast, cooler air below 33°C was prevalent in the southern part of the study area, with the coolest zones being concentrated in the middle of the Friedenspark and the westward adjacent botanical garden.
On the morning of 1 July at 6:00 h, the overall pattern (north-south gradient) remained similar to that in the evening condition. Mean temperature, however, dropped to 22°C—a cooling by over 10°C since 21:00 h, and differences between green areas and streets became more prominent again. Notably, air temperatures in the Lene-Voigt-Park decreased more than its surroundings and the coolest zones in the Friedenspark were found in the southern part where grasslands predominate. At the same time, an inflow of warm air might have caused warmer temperatures in the north of the park. However, we also emphasize that temperature differences were marginal with a total modeled amplitude less than 1°C (0.4°C less than measured in the field).
Cooling Effect of Public Parks
When testing for the cooling effect of the two major green spaces in the study area compared to their vicinity, the public parks Lene-Voigt-Park and the Friedenspark, we found major differences between them (Figure 6). On the one hand, the Friedenspark had the lowest average air temperatures among all compared areas at all four times of the day, with maximum differences to respective hottest areas from 0.39°C in the morning of 1 July up to 1.06°C at 8:00 h the day before. Despite its comparably green vicinity including mature trees (cf. Figure 2), the Friedenspark was consistently cooler than its surrounding 50-m buffer zone by 0.1 up to 0.3°C. The smaller and sparsely tree-covered Lene-Voigt-Park, on the other hand, was distinctly warmer throughout the day and in fact constituted the hottest area, followed by its 50-m buffer zone at 15:00 h in the afternoon as already suggested by the spatial patterns in Figure 5. Over the course of the evening and night, however, the Lene-Voigt-Park managed to cool down more effectively than our urban reference area, the Ostplatz (max. ∆T of 0.35°C at 21:00 h), which was the hottest area at this time, and also its immediate surroundings (max. ∆T of 0.11°C at 6:00 h). All average air temperature differences between the five analytical areas were statistically significant at p < 0.001 (for details, Supplementary Material S4).
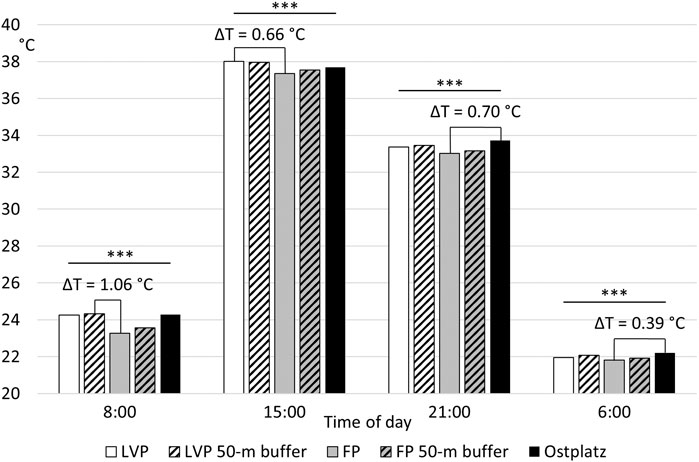
FIGURE 6. Mean air temperatures of the five analytical areas per time of the day that has been selected for spatial assessment. For each point in time we indicated maximum air temperature differences occurring among all five areas. Asterisks above the bars indicate the significance level (of difference) between all areas according to ANOVA (p ≤ 0.001, Supplementary Material S4). Acronyms: LVP, Lene-Voigt-Park; FP, Friedenspark.
Discussion
With this study, we aimed to assess the air temperature regulation by two inner-city parks under drought and heat conditions in Leipzig, a large Central European city. We produced spatially explicit maps of air temperature for a 24 h period (including nighttime) using dense in-situ measurements and random forest regression. The results showed that on a hot summer day with maximum air temperatures above 38°C, following a pronounced dry period, the average cooling by urban green spaces compared to built-up surroundings was approximately 1°C. We found slightly larger cooling effects, i.e., above 1°C, at single time points during the day and on a very fine-scale level that were attributable to coherent tree canopies and corresponding shading and evapotranspiration such as in the Friedenspark or in some vegetated backyards. Privately owned backyards can be often better maintained than public green spaces, e.g., when irrigation is necessary during dry periods.
By comparing two structurally distinct public parks, we conclude that larger, densely vegetated parks seem to better perform in reducing air temperatures under conditions of heat and drought than those of smaller and rather sparsely vegetated parks. In our study area, the dominating grasslands of the Lene-Voigt-Park were largely degraded after consecutive dry seasons in summer 2018 and 2019. As a result, the openness of the park, including several artificial sports areas, and a lack of vital grass cover and shading trees, temporally led to even more severe heat conditions during the daytime than in surrounding built-up areas where buildings provide shade, an effect also observed in previous studies (Bowler et al., 2010). The openness of the Lene-Voigt Park, however, promoted slightly faster cooling of the park area during the night compared to that in its built-up surroundings. In contrast, the larger Friedenspark, dominated by mature trees, showed lower air temperatures on average at any point in time compared with that in built-up areas and also the overall mean of the study area. Hereby, we also demonstrated that structurally diverse parks with a well-balanced combination of mature trees and open grasslands are comparatively cooler at all times of the day and night. The concept of structurally diverse urban green spaces has been called the “savannah approach” (Wittig et al., 2012; Meltzer, 2014; Zardo et al., 2017). The “savannah approach” is meant to explain that due to a diversified composition and configuration of vegetation types the microclimate regulation performs particularly well throughout the day, providing shade during the daytime and open spaces that allow heat emission during the night. However, in our case, consecutive drought and heat in 2018 and 2019 also affected the Friedenspark (cf. Figure 1). We expect the cooling service to be reduced compared to regular, vital conditions, because major parts of the park’s open grassy areas were also degraded, soils were parched and numerous trees died and had to be removed. Repeating our assessments under usual weather conditions and comparing our results with the cooling of unimpaired green space vegetation would be desirable by a follow-up project.
We found that the steepest air temperature gradients (relative coolness) did not necessarily occur between adjacent green and built-up areas but rather within larger zones of the urban fabric characterized by distinct green space and building structures. Hence, temperature differences within a single park can be greater than those between the park and adjoining built-up areas, For example, we observed a more than 0.8°C difference between the northern and the southern parts of the Friedenspark at 06:00 h in the morning while differences between the park and a major traffic junction at the southern edge of the park were marginal. The large temperature difference within the same park can be explained by complex meteorological processes in the urban environment such as air flows and heat fluxes that are strongly influenced by the overall urban building structures, materials, and terrain conditions (Moonen et al., 2012; Meili et al., 2021). Steeper temperature gradients at the very local scale may also appear, however, during the hottest period of the day between shaded and unshaded areas even within single green spaces. We found a nearly 2°C difference between adjacent open grassy and shady tree areas in the Friedenspark at 15:00 h in the afternoon, similar to previous findings in another park in Leipzig (Breuste et al., 2013).
In terms of air temperature differences between larger coherent green spaces (e.g., parks or cemeteries) and their built-up surroundings, our findings correspond to those of previous studies assessing temperature gradients, although, they are at the lower margin of the range of effect sizes. Bowler et al. (2010) and Knight et al. (2021) reported mean effect sizes of 0.94 and 0.8°C, respectively. In our study, the largest average temperature difference of approximately 1°C occurred in the morning between the large Friedenspark and the buffer zone of the Lene-Voigt-Park, i.e., not between the parks and their immediate surroundings. Hence, in our study, vegetation-related cooling, especially during the daytime, was largely spatially constrained, i.e., there were hardly any measurable cooling effects outside of larger green spaces that might be attributable to the green space itself. Cooler zones apart from the parks are rather directly linked to the presence of smaller vegetation structures such as vegetated backyards and strips of roadside greenery. We conclude that so-called “cooling distances” from green space boundaries into adjacent neighborhoods as suggested, e.g., by Jaganmohan et al. (2016), are negligible in the context of summer heat and a flat terrain, as in our case of Leipzig. Nevertheless, our study showed that the size and structure of the green space had a strong impact on spatial air temperature patterns and corresponding cooling, thus, consistent with previous findings (Jaganmohan et al., 2016; Zardo et al., 2017).
Beside public parks, allotment garden colonies appeared to be important for providing cooling at certain times of the day. These clusters of privately owned gardens typically have a diverse vegetation structure and are well maintained throughout the summer season which, in some cases, includes watering of lawn areas. Allotment garden colonies are widespread throughout the city of Leipzig as in other cities, especially in Central Europe (Cabral et al., 2017).
Strengths and Limitations
To the best of our knowledge, our study presents the first spatially explicit and detailed assessment of the air temperature regulation of urban green spaces under drought and heat conditions at the neighborhood level, and thus extending the scope compared with that of previous large-scale studies (Marando et al., 2019; Allen et al., 2021). Our study benefitted from spatially and temporally detailed in-situ data collected during an extensive field campaign that also allowed us to assess air temperature patterns during nighttime. We applied consistent measuring protocols following national and international standards (Löffler, 2012; World Meteorological Organization (WMO), 2018), facilitating reproducibility and transferability. Biased and inconsistent protocols make most studies on urban microclimate difficult to compare (Bartesaghi Koc et al., 2018), particularly when varying measurement settings were applied even within single studies (Sugawara et al., 2016; Mitterboeck and Korjenic, 2017). Considering the highly dynamic nature of air temperature, standardization of measurements is crucial.
We show that machine-learning approaches, in particular random forests, are suitable for predicting air temperatures at the fine-scale neighborhood level. However, a few of our models showed poor performance which is likely attributable to the limited amount of training data from a machine-learning perspective, since the performance of machine learning algorithms relies on the exhaustiveness of the input dataset, which usually improves with dataset size (Breiman, 2001; Willcock et al., 2018). Given the particularly high spatial heterogeneity of the urban fabric in terms of the composition and configuration of built-up and natural elements, our set of training data can in fact not be considered as exhaustively representative. First of all, our study design focused on two parks and their nearby surroundings (cf. Kabisch et al., 2021). Hence, other green spaces such as allotment gardens or backyards and larger, contiguous built-up areas are not represented in our training data and thus were modeled with higher uncertainties. Apart from our scope, we were naturally limited in two ways when running a field campaign in a public urban environment: first, by available resources (mainly personnel) that limited the spatial (and temporal) extent of our study, and second, regarding the selective distribution of measuring sites. Due to real-world circumstances, we had to install our park sensors to ensure the lowest possibility of interference from park users and their activities. Nevertheless, we accounted for the structural diversity of the parks (Kraemer and Kabisch, 2021), i.e., for park boundaries as well as densely vegetated and rather open spaces (cf. Figure 2, Supplementary Material S1).
To mount the loggers at the street sites we had to rely on the presence of suitable amenities (traffic signs or wooden planks around young street trees, cf. Kabisch et al., 2021) but also made sure to cover a variety of built-up environments, such as major and secondary streets, with and without street trees, and varying distances to buildings (cf. Supplementary Material S1). Hence, we argue that the issue of a limited number and biased distribution of measuring points in a real-world urban environment is difficult to overcome. The challenge of setting up devices in a public space might also be a reason for the lack of standardization, e.g., studies installing sensors out of range of people, i.e., not at a 2 m height, or surveys that apply mobile measurements (Jansson et al., 2007; Schwarz et al., 2012; Secerov et al., 2015).
We also had to deal with the typical behavior of random forests of underestimating extreme values as a result of reducing the variance (improving precision) in predicted values (Zhang and Lu, 2012; Wolfensberger et al., 2021). Although our models underestimated extreme values by approximately 0.5°C on average compared to measured values (cf. Table 2, Supplementary Material S2), we demonstrated that at most times of the day air temperatures showed only moderate variation between green spaces and street or built-up environments under conditions of heat and drought as compared to that in previous studies (Knight et al., 2021).
Applying a spatially dense meteorological survey involves further tradeoffs in temporal and spatial coverage. Our study was limited to an area of 250 ha and our available resources. We could run our field campaign with available resources for approximately 1 week. More extensive, district- or city-wide assessments over a longer period are desirable but would require a large number of long-term meteorological stations, ideally installed at representative locations reflecting urban spatial heterogeneity. Thus, public participation approaches such as the global networks “Weather Underground” (https://www.wunderground.com/) and “Sensor.community” (https://sensor.community) could be integrated into scientific studies such as done by Ho et al. (2014). Participatory surveys could be also aided by portable mobile sensors (e.g., Ueberham et al., 2019) that may then be combined with in-situ measurements to increase spatial coverage (Schwarz et al., 2012). However, issues regarding standardized survey methods, data quality and sufficient metadata remain for citizen-generated data and when using mobile sensors (Ho et al., 2014; Cao et al., 2020; Balázs et al., 2021). Finally, computational models and remote sensing are well-established tools for producing extensive, city-wide urban climate models that can facilitate the transferability and comparability of findings and reduce the efforts of otherwise resource-intensive field work (Bartesaghi Koc et al., 2018).
Conclusion
Providing accurate high-resolution air temperature data, our study illustrates the fine-scale air temperature dynamics that occur inside and outside urban green areas under heat and drought conditions. The spatially and temporally high-resolution data show the importance of urban park structure in providing air temperature regulation as an ecosystem service. In particular, grass-dominated green spaces are susceptible to degradation and thus reduced microclimate regulating ecosystem services under drought and heat. Under heat and drought condition, shading is the key factor for the cooling during daytime whereas open green or at least unsealed spaces remain important heat emitters during the night, suggesting a need for a balanced composition and configuration of vegetation types. We could demonstrate that the cooling effect of green spaces is spatially very limited particularly during peak time of heat during the afternoon. Thus, we emphasize the need for larger green spaces that are complemented by decentralized, well-distributed small-scale green infrastructure such as roadside greenery and vegetated backyards. Future empirical studies may also include a larger number of structurally distinct green spaces to better understand the variation of diurnal and nocturnal air temperature in urban parks depending on the vegetation structure and the potential cooling effect on adjacent neighborhoods.
With this study we exemplified how to apply standardized measurements of air temperature in an urban setting on a local neighborhood scale. However, more standardization is needed for in-situ measurements applied in urban microclimate studies to facilitate comparability across study sites and cities. Future research may also spatially extend the fine-scale assessment of the urban microclimate by further exploiting the potential of public participation, mobile exposure measurements and data science, in particular machine-learning techniques. Finally, given the recent heatwaves and the increased heat related mortality rates in Central Europe, air conditioning may be increasingly used in future urban residential development especially in countries at temperate latitudes such as Germany. The potentially increased use of air conditioning systems may impact local air temperatures and accelerate the urban heat island effect.
Data Availability Statement
All collected data used for this study are available at: Kabisch et al. (2021): Multi-disciplinary survey data for the assessment of regulating and recreational ecosystem services in urban parks under heat and drought conditions: Zenodo. Available online at https://zenodo.org/record/5148571. DOI: 10.5281/zenodo.5148570.
Author Contributions
RK and NK conceptualized and framed the study. RK organized and conducted data acquisition in the field. RK processed and analyzed the data, created the figures and wrote the first draft of the manuscript. RK and NK revised the manuscript.
Funding
This work was part of the research project “Environmental-health Interactions in Cities (GreenEquityHEALTH)—Challenges for Human Well-being under Global Changes” (2017–2022) funded by the German Federal Ministry of Education and Research (BMBF), funding code: 01LN1705A.
Conflict of Interest
The authors declare that the research was conducted in the absence of any commercial or financial relationships that could be construed as a potential conflict of interest.
Publisher’s Note
All claims expressed in this article are solely those of the authors and do not necessarily represent those of their affiliated organizations, or those of the publisher, the editors and the reviewers. Any product that may be evaluated in this article, or claim that may be made by its manufacturer, is not guaranteed or endorsed by the publisher.
Acknowledgments
We thank Jan Hemmerling (Humboldt-Universität zu Berlin), Jan Bumberger, Paul Remmler, Helko Kotas and Marco Pohle (all Helmholtz Centre of Environmental Research—UFZ, Leipzig, Department of Monitoring and Exploration Technologies) for providing technical support and advise in the air temperature measurements. We thank the City of Leipzig, Department for Urban Green and Waters, for supporting the project. We would also like to thank the three reviewers for their detailed and helpful comments to earlier versions of this paper.
Supplementary Material
The Supplementary Material for this article can be found online at: https://www.frontiersin.org/articles/10.3389/fenvs.2022.849965/full#supplementary-material
References
Allen, M. A., Roberts, D. A., and McFadden, J. P. (2021). Reduced Urban green Cover and Daytime Cooling Capacity during the 2012-2016 California Drought. Urban Clim. 36, 100768. doi:10.1016/j.uclim.2020.100768
An der Heiden, M., Buchholz, U., and Uphoff, H. (2019a). Schätzung der Zahl hitzebedingter Sterbefälle infolge der Hitzewelle 2018; Berlin und Hessen, Sommer 2018. Epidemiologisches Bull., 193–202. doi:10.25646/6178
An der Heiden, M., Muthers, S., Niemann, H., Buchholz, U., Grabenhenrich, L., and Matzarakis, A. (2019b). Schätzung hitzebedingter Todesfälle in Deutschland zwischen 2001 und 2015. Bundesgesundheitsbl 62, 571–579. doi:10.1007/s00103-019-02932-y
Balázs, B., Mooney, P., Nováková, E., Bastin, L., and Jokar Arsanjani, J. (2021). “Data Quality in Citizen Science,” in The Science of Citizen Science. Editors K. Vohland, A. Land-Zandstra, L. Ceccaroni, R. Lemmens, J. Perelló, and M. Ponti (ChamImprint: Springer International PublishingSpringer), 139–157. doi:10.1007/978-3-030-58278-4_8
Banzhaf, E., and Kollai, H. (2018). Land Use /Land Cover for Leipzig, Germany, for 2012 by an Object-Based Image Analysis (OBIA): Supplement to: Banzhaf, E.; Kollai, H.; Kindler, A. (2018): Mapping Urban Grey and green Structures for Liveable Cities Using a 3D Enhanced OBIA Approach and Vital Statistics. Geocarto Int. 35 (6). doi:10.1594/PANGAEA.89539110.1080/10106049.2018.1524514
Bartesaghi Koc, C., Osmond, P., and Peters, A. (2018). Evaluating the Cooling Effects of green Infrastructure: A Systematic Review of Methods, Indicators and Data Sources. Solar Energy 166, 486–508. doi:10.1016/j.solener.2018.03.008
Bernhofer, C., Franke, J., Fischer, S., Kirsten, L., Körner, P., Kostrowski, D., et al. (2015). Analyse der Klimaentwicklung in Sachsen.
Boergens, E., Güntner, A., Dobslaw, H., and Dahle, C. (2020). Quantifying the Central European Droughts in 2018 and 2019 with GRACE Follow‐On. Geophys. Res. Lett. 47, 1672. doi:10.1029/2020GL087285
Bosch, M., Locatelli, M., Hamel, P., Remme, R. P., Chenal, J., and Joost, S. (2021). A Spatially Explicit Approach to Simulate Urban Heat Mitigation with InVEST (v3.8.0). Geosci. Model. Dev. 14, 3521–3537. doi:10.5194/gmd-14-3521-2021
Bowler, D. E., Buyung-Ali, L., Knight, T. M., and Pullin, A. S. (2010). Urban Greening to Cool Towns and Cities: A Systematic Review of the Empirical Evidence. Landscape Urban Plann. 97, 147–155. doi:10.1016/j.landurbplan.2010.05.006
Breuste, J., Haase, D., and Elmqvist, T. (2013). “Urban Landscapes and Ecosystem Services,” in Ecosystem Services in Agricultural and Urban Landscapes. Editors S. D. Wratten, H. Sandhu, R. Cullen, and R. Costanza (Chichester: Wiley-Blackwell), 83–104. doi:10.1002/9781118506271.ch6
Cabral, I., Keim, J., Engelmann, R., Kraemer, R., Siebert, J., and Bonn, A. (2017). Ecosystem Services of Allotment and Community Gardens: A Leipzig, Germany Case Study. Urban For. Urban Green. 23, 44–53. doi:10.1016/j.ufug.2017.02.008
Cao, C., Yang, Y., Lu, Y., Schultze, N., Gu, P., Zhou, Q., et al. (2020). Performance Evaluation of a Smart Mobile Air Temperature and Humidity Sensor for Characterizing Intracity Thermal Environment. J. Atmos. Oceanic Tech. 37, 1891–1905. doi:10.1175/JTECH-D-20-0012.1
Ciscar, J. C., Ibarreta, D., Soria, A., Dosio, A. A. T., Ceglar, A., Fumagalli, D., et al. (2018). Climate Impacts in Europe: Final Report of the JRC PESETA III Project. Luxembourg: Publications Office. doi:10.2760/93257
City of Leipzig (2019b). Trockenheit setzt Leipzigs Bäumen zu. Leipzig. Available at: https://static.leipzig.de/fileadmin/extensions/pressreleases/CC0D9FAD6DBF81EDC1258440004FD083/580-fra-Trockenheit.pdf (Accessed March 2, 2022).
City of Leipzig (2019a). Trockenheit Stresst Leipzigs Teiche Auch in Diesem Jahr. Leipzig. Available at: https://static.leipzig.de/fileadmin/extensions/pressreleases/FED1865066B1E8DBC1258424003F01ED/500-quo-Trockenheit.pdf (Accessed March 2, 2022).
Dale, A. G., and Frank, S. D. (2017). Warming and Drought Combine to Increase Pest Insect Fitness on Urban Trees. PLoS ONE 12, e0173844. doi:10.1371/journal.pone.0173844
Deutscher Wetterdienst, Climate Data Center (CDC) (2021). Daily Station Measurements of Precipitation in Mm and Mean Air Temperature at 2 M Altitude in °C for Germany. Version v21.3. Available at: https://cdc.dwd.de/portal/.
European Environment Agency (EEA) (2021). Europe’s Changing Climate Hazards — an index-based Interactive EEA Report. doi:10.2800/458052
GeoSN (2018). Digital Elevation and Surface model. Survey Date: 2012. Dresden, Germany: Staatsbetrieb Geobasisinformation und Vermessung Sachsen GeoSN.
Gromke, C., Blocken, B., Janssen, W., Merema, B., van Hooff, T., and Timmermans, H. (2015). CFD Analysis of Transpirational Cooling by Vegetation: Case Study for Specific Meteorological Conditions during a Heat Wave in Arnhem, Netherlands. Building Environ. 83, 11–26. Netherlands. doi:10.1016/j.buildenv.2014.04.022
Guerreiro, S. B., Dawson, R. J., Kilsby, C., Lewis, E., and Ford, A. (2018). Future Heat-Waves, Droughts and Floods in 571 European Cities. Environ. Res. Lett. 13, 034009. doi:10.1088/1748-9326/aaaad3
Heinemann, J., Naber, M., and Schultz, A. (2019). Bevölkerungsvorausschätzung 2019: Methoden und Ergebnisse. Available at: https://static.leipzig.de/fileadmin/mediendatenbank/leipzig-de/Stadt/02.1_Dez1_Allgemeine_Verwaltung/12_Statistik_und_Wahlen/Stadtforschung/Bevolkerungsvorausschatzung_2019.pdf (Accessed March 2, 2022)
Ho, H. C., Knudby, A., Sirovyak, P., Xu, Y., Hodul, M., and Henderson, S. B. (2014). Mapping Maximum Urban Air Temperature on Hot Summer Days. Remote Sensing Environ. 154, 38–45. doi:10.1016/j.rse.2014.08.012
Iovan, C., Boldo, D., and Cord, M. (2008). Detection, Characterization, and Modeling Vegetation in Urban Areas from High-Resolution Aerial Imagery. IEEE J. Sel. Top. Appl. Earth Observations Remote Sensing 1, 206–213. doi:10.1109/JSTARS.2008.2007514
IPCC (2021). Climate Change 2021: The Physical Science Basis. Summary For Policymakers. Contribution of Working Group I to the Sixth Assessment Report of the Intergovernmental Panel on Climate Change.
Jaganmohan, M., Knapp, S., Buchmann, C. M., and Schwarz, N. (2016). The Bigger, the Better? the Influence of Urban Green Space Design on Cooling Effects for Residential Areas. J. Environ. Qual. 45, 134–145. doi:10.2134/jeq2015.01.0062
Jansson, C., Jansson, P.-E., and Gustafsson, D. (2007). Near Surface Climate in an Urban Vegetated Park and its Surroundings. Theor. Appl. Climatol. 89, 185–193. doi:10.1007/s00704-006-0259-z
Jin, L., Schubert, S., Hefny Salim, M., and Schneider, C. (2020). Impact of Air Conditioning Systems on the Outdoor Thermal Environment during Summer in Berlin, Germany. Ijerph 17, 4645. doi:10.3390/ijerph17134645
Kabisch, N., Kraemer, R., Brenck, M. E., Haase, D., Lausch, A., Luttkus, M. L., et al. (2021). A Methodological Framework for the Assessment of Regulating and Recreational Ecosystem Services in Urban parks under Heat and Drought Conditions. Ecosyst. People 17, 464–475. doi:10.1080/26395916.2021.1958062
Knight, T., Price, S., Bowler, D., Hookway, A., King, S., Konno, K., et al. (2021). How Effective Is ‘greening’ of Urban Areas in Reducing Human Exposure to Ground-Level Ozone Concentrations, UV Exposure and the ‘urban Heat Island Effect’? an Updated Systematic Review. Environ. Evid. 10, 1–38. doi:10.1186/s13750-021-00226-y
Kraemer, R., and Kabisch, N. (2021). Parks in Context: Advancing Citywide Spatial Quality Assessments of Urban green Spaces Using fine-scaled Indicators. Ecology and Society 26. doi:10.5751/ES-12485-260245
Kremer, P., Larondelle, N., Zhang, Y., Pasles, E., and Haase, D. (2018). Within-Class and Neighborhood Effects on the Relationship between Composite Urban Classes and Surface Temperature. Sustainability 10, 645. doi:10.3390/su10030645
Kumar, P. (2021). Climate Change and Cities: Challenges Ahead. Front. Sustain. Cities 3, 5. doi:10.3389/frsc.2021.645613
Lehmann, I., Mathey, J., Rößler, S., Bräuer, A., and Goldberg, V. (2014). Urban Vegetation Structure Types as a Methodological Approach for Identifying Ecosystem Services - Application to the Analysis of Micro-climatic Effects. Ecol. Indicators 42, 58–72. doi:10.1016/j.ecolind.2014.02.036
Li, J., Heap, A. D., Potter, A., and Daniell, J. J. (2011). Application of Machine Learning Methods to Spatial Interpolation of Environmental Variables. Environ. Model. Softw. 26, 1647–1659. doi:10.1016/j.envsoft.2011.07.004
Löffler, H. (2012). Meteorologische Bodenmesstechnik. Offenbach Am Main: Deutscher Wetterdienst (DWD).
Marando, F., Heris, M. P., Zulian, G., Udías, A., Mentaschi, L., Chrysoulakis, N., et al. (2022). Urban Heat Island Mitigation by green Infrastructure in European Functional Urban Areas. Sust. Cities Soc. 77, 103564. doi:10.1016/j.scs.2021.103564
Marando, F., Salvatori, E., Sebastiani, A., Fusaro, L., and Manes, F. (2019). Regulating Ecosystem Services and Green Infrastructure: Assessment of Urban Heat Island Effect Mitigation in the Municipality of Rome, Italy. Ecol. Model. 392, 92–102. doi:10.1016/j.ecolmodel.2018.11.011
McDonald, R. I., Colbert, M., Hamann, M., Simkin, R. D., and Walsh, B. J. C. (2018). Nature in the Urban century: A Global Assessment of where and How to Conserve Nature for Biodiversity and Human Wellbeing.
Meili, N., Manoli, G., Burlando, P., Carmeliet, J., Chow, W. T. L., Coutts, A. M., et al. (2021). Tree Effects on Urban Microclimate: Diurnal, Seasonal, and Climatic Temperature Differences Explained by Separating Radiation, Evapotranspiration, and Roughness Effects. Urban For. Urban Green. 58, 126970. doi:10.1016/j.ufug.2020.126970
Meinert, T., Becker, A., Bissolli, P., Daßler, J., Breidenbach, N., and Ziese, M. (2019). Ursachen und Folgen der Trockenheit in Deutschland und Europa ab Juni 2019.
Meltzer, L. (2014). Consideration of Climate Change in the Design of parks and Open Spaces: A Study within the Framework of the INTERREG IVC Project ‘Hybrid Parks’.
Mitterboeck, M., and Korjenic, A. (2017). Analysis for Improving the Passive Cooling of Building's Surroundings through the Creation of green Spaces in the Urban Built-Up Area. Energy and Buildings 148, 166–181. doi:10.1016/j.enbuild.2017.02.005
Moonen, P., Defraeye, T., Dorer, V., Blocken, B., and Carmeliet, J. (2012). Urban Physics: Effect of the Micro-climate on comfort, Health and Energy Demand. Front. Architectural Res. 1, 197–228. doi:10.1016/j.foar.2012.05.002
Nature Editorial (2021). Cities Must Protect People from Extreme Heat. Nature 595, 331–332. doi:10.1038/d41586-021-01903-1
Oke, T. R. (1982). The Energetic Basis of the Urban Heat Island. Q.J R. Met. Soc. 108, 1–24. doi:10.1002/qj.49710845502
Pace, R., De Fino, F., Rahman, S., Nowak, D. J., and Grote, R. (2021). A Single Tree Model to Consistently Simulate Cooling, Shading, and Pollution Uptake of Urban Trees. Int. J. Biometeorol. 65, 277–289. doi:10.1007/s00484-020-02030-8
Potsdam Institute for Climate Impact Research (PIK) (2021). ClimateImpactsOnline. Available at: https://www.climateimpactsonline.com/(Accessed October 04, 2021).
Rahman, M. A., Stratopoulos, L. M. F., Moser-Reischl, A., Zölch, T., Häberle, K.-H., Rötzer, T., et al. (2020). Traits of Trees for Cooling Urban Heat Islands: A Meta-Analysis. Building Environ. 170, 106606. doi:10.1016/j.buildenv.2019.106606
Raymond, C., Matthews, T., and Horton, R. M. (2020). The Emergence of Heat and Humidity Too Severe for Human Tolerance. Sci. Adv. 6, eaaw1838. doi:10.1126/sciadv.aaw1838
Robine, J.-M., Cheung, S. L. K., Le Roy, S., van Oyen, H., Griffiths, C., Michel, J.-P., et al. (2008). Death Toll Exceeded 70,000 in Europe during the Summer of 2003. Comptes Rendus Biologies 331, 171–178. doi:10.1016/j.crvi.2007.12.001
Rötzer, T., Moser-Reischl, A., Rahman, M. A., Hartmann, C., Paeth, H., Pauleit, S., et al. (2021). Urban Tree Growth and Ecosystem Services under Extreme Drought. Agric. For. Meteorology 308-309, 108532. doi:10.1016/j.agrformet.2021.108532
Schnabel, F., Purrucker, S., Schmitt, L., Engelmann, R. A., Kahl, A., Richter, R., et al. (2021). Cumulative Growth and Stress Responses to the 2018-2019 Drought in a European Floodplain forest. Glob. Change Biol. 28, 1870–1883. doi:10.1111/gcb.16028
Schwaab, J., Meier, R., Mussetti, G., Seneviratne, S., Bürgi, C., and Davin, E. L. (2021). The Role of Urban Trees in Reducing Land Surface Temperatures in European Cities. Nat. Commun. 12, 6763. doi:10.1038/s41467-021-26768-w
Schwarz, N., Schlink, U., Franck, U., and Großmann, K. (2012). Relationship of Land Surface and Air Temperatures and its Implications for Quantifying Urban Heat Island Indicators-An Application for the City of Leipzig (Germany). Ecol. Indicators 18, 693–704. doi:10.1016/j.ecolind.2012.01.001
Secerov, I., Savic, S., Milosevic, D., Markovic, V., and Bajsanski, I. (2015). Development of an Automated Urban Climate Monitoring System in Novi Sad (Serbia). Geogr. Pannon 19, 174–183. doi:10.5937/GeoPan1504174S
Sekulić, A., Kilibarda, M., Heuvelink, G. B. M., Nikolić, M., and Bajat, B. (2020). Random Forest Spatial Interpolation. Remote Sensing 12, 1687. doi:10.3390/rs12101687
Sugawara, H., Shimizu, S., Takahashi, H., Hagiwara, S., Narita, K.-I., Mikami, T., et al. (2016). Thermal Influence of a Large Green Space on a Hot Urban Environment. J. Environ. Qual. 45, 125–133. doi:10.2134/jeq2015.01.0049
UBA (2020). Gebäudeklimatisierung in Deutschland. (in German) Available at: https://www.umweltbundesamt.de/themen/klima-energie/fluorierte-treibhausgase-fckw/anwendungsbereiche-emissionsminderung/gebaeudeklimatisierung (Accessed March 02, 2022).
Ueberham, M., Schlink, U., Dijst, M., and Weiland, U. (2019). Cyclists' Multiple Environmental Urban Exposures-Comparing Subjective and Objective Measurements. Sustainability 11, 1412. doi:10.3390/su11051412
Helmholtz Centre for Environmental Research (UFZ) (2021). Dürremonitor Deutschland: Dürren seit 2014 (monatlich). Available at: https://www.ufz.de/index.php?de=40990 (Accessed October 05, 2021).
Veerkamp, C. J., Schipper, A. M., Hedlund, K., Lazarova, T., Nordin, A., and Hanson, H. I. (2021). A Review of Studies Assessing Ecosystem Services provided by Urban green and Blue Infrastructure. Ecosystem Serv. 52, 101367. doi:10.1016/j.ecoser.2021.101367
Weber, N., Haase, D., and Franck, U. (2014). Zooming into Temperature Conditions in the City of Leipzig: How Do Urban Built and green Structures Influence Earth Surface Temperatures in the City? Sci. Total Environ. 496, 289–298. doi:10.1016/j.scitotenv.2014.06.144
WHO (2021). Heat and Health in the WHO European Region: Updated Evidence for Effective Prevention. Copenhagen: WHO Regional Office for Europe Copenhagen.
Willcock, S., Martínez-López, J., Hooftman, D. A. P., Bagstad, K. J., Balbi, S., Marzo, A., et al. (2018). Machine Learning for Ecosystem Services. Ecosystem Serv. 33, 165–174. doi:10.1016/j.ecoser.2018.04.004
Willemen, L. (2020). It's about Time: Advancing Spatial Analyses of Ecosystem Services and Their Application. Ecosystem Serv. 44, 101125. doi:10.1016/j.ecoser.2020.101125
Wittig, R., Kuttler, W., and Tackenberg, O. (2012). “Urban-industrielle Lebensräume,” in Klimawandel und Biodiversität: Folgen für Deutschland. Editors V. Mosbrugger, G. Brasseur, M. Schaller, B. Stribrny, and Darmstadt (WBG (Wiss. Buchges), 290–307.
World Meteorological Organization (WMO) (2018). Guide to Instruments and Methods of Observation: Volume I – Measurement of Meteorological Variables.
Wolfensberger, D., Gabella, M., Boscacci, M., Germann, U., and Berne, A. (2021). RainForest: a Random forest Algorithm for Quantitative Precipitation Estimation over Switzerland. Atmos. Meas. Tech. 14, 3169–3193. doi:10.5194/amt-14-3169-2021
Wolff, M., Haase, A., Haase, D., and Kabisch, N. (2016). The Impact of Urban Regrowth on the Built Environment. Urban Stud. 54, 2683–2700. doi:10.1177/0042098016658231
Yu, C., and Hien, W. N. (2006). Thermal Benefits of City parks. Energy and Buildings 38, 105–120. doi:10.1016/j.enbuild.2005.04.003
Yuan, C., Norford, L., and Ng, E. (2017). A Semi-empirical Model for the Effect of Trees on the Urban Wind Environment. Landscape Urban Plann. 168, 84–93. doi:10.1016/j.landurbplan.2017.09.029
Zardo, L., Geneletti, D., Pérez-Soba, M., and van Eupen, M. (2017). Estimating the Cooling Capacity of green Infrastructures to Support Urban Planning. Ecosystem Serv. 26, 225–235. doi:10.1016/j.ecoser.2017.06.016
Zhang, G., and Lu, Y. (2012). Bias-corrected Random Forests in Regression. J. Appl. Stat. 39, 151–160. doi:10.1080/02664763.2011.578621
Zhang, R., Sun, C., Zhu, J., Zhang, R., and Li, W. (2020). Increased European Heat Waves in Recent Decades in Response to Shrinking Arctic Sea Ice and Eurasian Snow Cover. Npj Clim. Atmos. Sci. 3, 1–9. doi:10.1038/s41612-020-0110-8
Zhao, L., Oppenheimer, M., Zhu, Q., Baldwin, J. W., Ebi, K. L., Bou-Zeid, E., et al. (2018). Interactions between Urban Heat Islands and Heat Waves. Environ. Res. Lett. 13, 034003. doi:10.1088/1748-9326/aa9f73
Footnotes
1Temperature differences and changes are usually expressed in Kelvin, especially in meteorological and climatic research. However, for consistency and considering the broad, interdisciplinary audience of the journal we have chosen to use degrees Celsius (℃) throughout the article.
Keywords: urban green spaces, ecosystem services, microclimate regulation, heat, drought, urban climate, Leipzig, random forests
Citation: Kraemer R and Kabisch N (2022) Parks Under Stress: Air Temperature Regulation of Urban Green Spaces Under Conditions of Drought and Summer Heat. Front. Environ. Sci. 10:849965. doi: 10.3389/fenvs.2022.849965
Received: 06 January 2022; Accepted: 11 March 2022;
Published: 13 April 2022.
Edited by:
Francesco Orsi, Wageningen University and Research, NetherlandsReviewed by:
Jingxia Wang, Ruhr University Bochum, GermanyFederica Marando, Joint Research Centre, Italy
Junxiang Li, Shanghai Jiao Tong University, China
Copyright © 2022 Kraemer and Kabisch. This is an open-access article distributed under the terms of the Creative Commons Attribution License (CC BY). The use, distribution or reproduction in other forums is permitted, provided the original author(s) and the copyright owner(s) are credited and that the original publication in this journal is cited, in accordance with accepted academic practice. No use, distribution or reproduction is permitted which does not comply with these terms.
*Correspondence: Roland Kraemer, a3JhZW1lci5yb2xhbmRAZ21haWwuY29t