- Radboud Institute for Biological and Environmental Sciences (RIBES), Radboud University, Nijmegen, Netherlands
Plastic pollution in the ocean occurs mainly via riverine transport. In rivers, plastic is pervasive in sediments and in the water column. Monitoring of floating plastics in rivers is time consuming as it is usually collected using nets and classified by hand, or counted and classified visually. To make plastic detection in the water column more time- and cost-efficient, there is a need to explore remote sensing options. Here we present the results of two semi-controlled pilot tests in standing water using two imaging sonar technologies: an Adaptive Resolution Imaging Sonar (ARIS) sonar and a low-cost side-scan sonar (SSS). Additionally, the ARIS sonar was tested in flowing water at a sheltered shore channel behind a longitudinal training dam in the river Waal, Netherlands. Both technologies were able to detect 100% of the macroplastics tested in standing water. The ARIS sonar provided higher resolution images of the targets tested due to its high operation frequency detecting macroplastics down to a size of 1 cm2. The ARIS sonar detected macroplastics in the field, however, the detection decreased to 67% in flowing water. This sonar was limited to the 2D horizontal position of targets. The SSS is a low-cost option for monitoring of plastics and is integrated with CHIRP sonar technology that combines side and down imaging providing the 3D position of targets. For future monitoring, an ARIS sonar in motion or two ARIS sonars used simultaneously may provide the necessary 3D spatial information of plastic targets.
1 Introduction
Globally, plastic pollution is a major problem with adverse effects for freshwater and marine ecosystems (Thompson et al., 2004; Cole et al., 2013; Free et al., 2014; Lechner et al., 2014; Schmidt et al., 2017; Lau et al., 2020; Van Emmerik et al., 2022a). Once plastic is produced and discarded on land, a large amount is retained in riverine sinks while only a fraction ends up in marine ecosystems via riverine transport (Moore et al., 2011; Lebreton et al., 2017; Schmidt et al., 2017; D’Hont et al., 2021; Meijer et al., 2021; Van Emmerik et al., 2022b). In rivers, plastics have been observed accumulating in sediments and vegetation of banks and floodplains, transported in the riverbed and floating or suspended in the water column (Schwarz et al., 2019; Van Emmerik and Schwarz, 2019; Van Emmerik et al., 2022b). However, there is a data deficiency for all of the plastic sources and sinks in rivers due to differing or lacking long-term monitoring strategies (Schöneich-Argent et al., 2020; Van Emmerik et al., 2022a). Previous findings have suggested that plastics persist throughout riverine ecosystems with a large fraction found suspended in the water column (Hohenblum et al., 2015; Broere et al., 2021). Presently, plastic monitoring in the water column of rivers is time consuming as plastics are mainly collected with nets, then sorted and counted manually, or visually identified in situ (Cheshire et al., 2009; OSPAR Commission, 2010; Morritt et al., 2014; Schöneich-Argent et al., 2020; Schreyers et al., 2021). Riverine plastic pollution categorization involves large volumes of waste, thus time- and cost-effective techniques for long-term monitoring are needed (Van Emmerik et al., 2020b; Schöneich-Argent et al., 2020; Meijer et al., 2021; Van Emmerik et al., 2022a). Consequently, there is an increasing need for less labor intensive plastic monitoring in the water column of freshwater ecosystems. Sonar technology offers an opportunity for off-site monitoring of plastics through the recording of data or remote monitoring via internet connection. Several remote sensing technologies have been tested for plastic detection in aquatic ecosystems including Compressed High Intensity Radiated Pulse (CHIRP) and multibeam echosounders (MBES; Garaba et al., 2018; Topouzelis et al., 2019; Weiland, 2019; Sappé, 2020; Broere et al., 2021). Additionally, automation of sonar image identification is possible with segmentation algorithms (Shahrestani et al., 2017; Broere et al., 2021).
Sonar transducers emit sound waves that reflect off the surfaces in their paths and then are received back by the transducers. The two-way travel time and the speed of sound in the travel medium are used to determine the distance, or range to the surfaces or targets. Each target that the waves reflect from has a different sound wave reflection based on its unique physical attributes (i.e. hardness, density, etc.,) which may be used in mathematical models to map or image the targets (Klein and Edgeton, 1968; De Moustier and Matsumoto, 1993; Chandrashekar, 2014).
Adaptive Resolution Imaging Sonars (ARIS) are imaging multibeam sonars that emit a horizontal fan of high frequency (Megahertz) beams into the water column (Sound Metrics, 2020). Traditional MBES systems operate at lower frequencies (Kilohertz) resulting in lower resolution imaging (De Moustier and Matsumoto, 1993). The higher frequencies allow continuous reflections to be received by the transducer and imaged in high resolution, capturing the shape, size, orientation and direction of travel of passing targets in turbid or low light settings (Sound Metrics, 2020). ARIS sonars have been commonly used in the biological science for the identification, behavioral observation and length measurement estimation of fish species from sonar images (Shahrestani et al., 2017; Cook et al., 2019; Jones et al., 2021). ARIS sonar technology is also promising for macroplastics detection because it has successfully been used in the identification of marine debris (i.e., hooks, tires, plastic bottle, etc.,) at the bottom of a tank in a laboratory setting for which the images were analyzed using Deep Neural Networks (Valdenegro-Toro, 2019). Nevertheless, for plastic monitoring in rivers, the detection of moving targets in the water column is necessary.
A second promising imaging sonar technology for macroplastic detection is side-scan sonar (SSS). This consists of an oblique (>50⁰) transducer that emits and receives sound at an angle allowing for the imaging of the bottom in 2D along the horizontal plane (De Moustier and Matsumoto, 1993). Because of this, the technique has been previously used to map riverbeds (Anima et al., 2007; Kaeser et al., 2013; Richter et al., 2016). Compared to the ARIS sonar, some SSS are very low-cost (€2,000–3,000) as they are designed for sport fishing often combining SSS with CHIRP technology. CHIRP technology has already been confirmed to detect macroplastics in the water column of rivers providing the depth (z-axis coordinate) to the target (Broere et al., 2021). The combination of CHIRP with SSS gives an ideal opportunity to find the 3D position (x, y and z-axes coordinates) of a target in the water column. This since SSS provides the x and y-axes coordinates to the target.
With this study we aim to expand the knowledge on water column plastics detection using sonars by testing two previously untested sonar technologies being a very high frequency multibeam sonar and a side-scan sonar. In doing so this work expands on the work by Broere et al. (2021) who tested CHIRP technology for underwater plastic detection. We collected data on the area of the sonar reflections of suspended macroplastics and expand the analysis performed by Broere et al. (2021) by also adding the mean intensity of the target reflections. The aim of this pilot study is to determine whether 1) an ARIS sonar and a low-cost SSS with CHIRP could detect macroplastics in the water column, and 2) the mean target reflections differ between various types and sizes of macroplastics. Macroplastics are defined as relatively large particles of plastic, typically >0.5 cm (Moore, 2008; Koelmans et al., 2017).
2 Materials and Methods
2.1 ARIS Sonar
An ARIS EXPLORER 3000 (Sound Metrics Corp.) was used for data collection using the “detection frequency” of 1.8 MHz which allowed for a range up to 15 m. The sonar provided continuous high definition images of the objects placed in the fan composed by 128 beams with 0.25° width. ARIS technology enables the collection of data at rates of up to 15 frames per second (Sound Metrics, 2020). The raw data was recorded in ARIS (.aris) format using ARIScope data collection software. Data were replayed and exported using ARISFish software, a complete data replaying and visualization software package with functionalities such as signal filtering, target measuring tools and multiple options for signal visualization.
2.1.1 ARIS Test in Standing Water
A semi-controlled test in standing water (flow velocity ∼0 m/s) was completed as a first assessment of the reflections obtained by placing plastics within the beams fan of the ARIS sonar. On 16 April 2021, data were collected by submerging the ARIS sonar next to a dock in a floodplain lake at the Rijkswaterstaat (RWS; The Directorate-General for Public Works and Water Management) Rosandepolder facility near Arnhem, Netherlands (N51.979289, E5.868003). The sonar was mounted on a square metal frame that maintained the sonar off of the bottom while allowing to change the angle of the sensor as needed. For the test 16 plastic pieces (See Supplementary Figure S1 and Supplementary Table S1) of different sizes were cut from recycled polyethylene terephthalate (rPET) clear-plastic cups (H = 10.5 cm and ø = 6 cm) and polypropylene (PP) square white-plastic food containers (L = 17 cm, W = 12 cm and H = 8 cm). Detection of individual macroplastics using the ARIS sonar was completed at ∼0.5–1 m water depth by attaching objects to a fishing line weighted with a stainless steel pin or a bow shackle. ARIScope acquisition software was used to visually assess the data in real-time and data files were recorded. After collection, the playbacks of the data were visually interpreted using ARISFish software (Figure 1). The area (pixels2) and mean intensity (mean gray value) of the target reflections for five frames were measured using open-source ImageJ2 software (Rueden et al., 2017). The still images were selected based on having relatively empty backgrounds, less or no interference from the fishing line, similar ranges (0.9–1.3 m) and orientation of the objects. During the testing the beams fan had a blind zone of 0.7 m in front of the sonar unit where the target could not be detected.
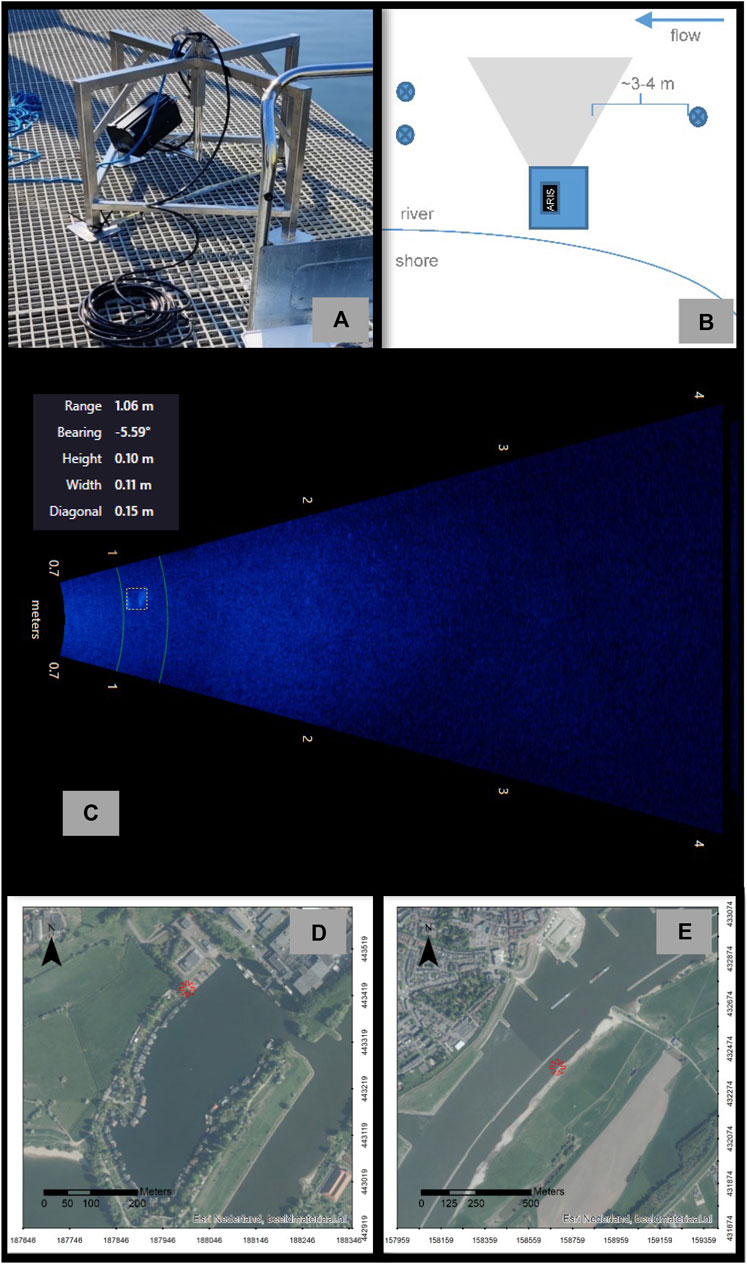
FIGURE 1. (A) The ARIS sonar installed on the mount as used for both the standing water and flowing water tests, (B) schematic drawing of the set-up for the flowing water test (the circles with crosses indicate one wading person standing upstream and two standing downstream of the sonar to release and catch the macroplastics, respectively, (C) the beams fan of the ARIS sonar as seen in ARISFish software near the centre at 1.06 m range a macroplastic (11 × 10 cm) recorded during data collection performed in April, 2021, (D) map of the Rosandepolder RWS facility used for the ARIS standing water test, and (E) map of the sheltered shore channel site used for the ARIS flowing water test. The exact locations are denoted by the red asterisk. Photo by N.Y. Flores. Aerial images source: Environmental Systems Research Institute (ESRI) Nederland, beeldmateriaal.nl.
2.1.2 ARIS Test in Flowing Water
On 19 April 2021, the flowing water test data (flow velocities ∼0.8 m/s) were collected using the ARIS sonar mounted in the square metal frame deployed in the littoral zone of a sheltered shore channel behind a longitudinal training dam in the river Waal near Dreumel, Netherlands (Flores et al., 2022; Figure 1; N51.880995, E5.441262). This location was selected since it is void of the influence of passing inland navigation resulting in a more laminar flow pattern (Collas et al., 2018). This to increase the likelihood that the released macroplastics would pass in front of the sonar and not be moved away from the beams fan by the current. The macroplastics were retrieved from the river Waal during previous plastic monitoring campaigns (Collas et al., 2021). Objects were composed of different polymers and included a plastic bag, glove, wire, band-aid and foil, among others. Some macroplastics, a plastic cup and a jug, were tested while attached to a fishing line in order to avoid losing them. The rest of the macroplastics were manually released one at a time from a location ∼3–4 m upstream from the sonar. A total of 27 macroplastics were tested once each. Released macroplastics were allowed to be carried by the current through the sonar beams fan. When possible the macroplastics were recaptured downstream using handheld nets. During data collection real-time visual verification of the objects within the beams fan of the sonar was done when possible. If an object was observed by the individuals in the water to not pass in front of the sonar the test was repeated. The data was replayed in ARISFish and the echograms were visually interpreted for any reflections that differed from the background noise, each macroplastic was classified as either detected or not detected.
2.2 Lowrance Side-Scan Sonar
A Lowrance Elite-12 Ti2 unit with a pole mounted Active ImagingTM 3-in-1 transducer was used. The pole mount had a clamp that allowed to fix the transducer to any solid platform. The transducer was operated at 455 kHz with a 20 m range for the side image and 200 kHz and a 10 m range for the down image. Data were replayed, saved and exported using the screen display and software of the Lowrance unit. Sonar backscatter data was collected in .sl2 format.
2.2.1 Side-Scan Sonar Test in Standing Water
A semi-controlled test in standing water was completed on 19 November 2020 in the dock (water depth ∼3.9 m) of the Rosandepolder RWS facility, Netherlands (N51.979405, E5.870228), using the Lowrance unit. The transducer was pole mounted to a dock 20 cm below the water surface. Four macroplastics were used consisting of a polyethylene terephthalate (PET) 0.5 L water bottle, a polypropylene (PP) 150 g lunch meat packet, a low density polyethylene (LDPE) sanitary napkin wrapper and a small polypropylene (PP) cup (Naik et al., 2021; See Supplementary Figure S2 and Supplementary Table S2). These macroplastics were retrieved from the river Waal during previous plastic monitoring campaigns (Collas et al., 2021). Each macroplastics was prepared by attaching each to 1.2 m of weighted fishing line then leaving 1 m of fishing line between the object and a float (piece of wood) connected to a rope for towing. The objects were introduced into the water at ∼0.5–1 m water depth and towed from a 5 m mark in front of the transducer to the starboard side at ranges equal to 0.2–1.2 m (Figure 2). This was repeated 4 times per object. The raw sonar data was imported into ReefMaster Software Ltd. (version 2.0) and the side view (side-scan) data was exported as still images for the best identifiable instances of each target, leading to four images per macroplastic. The images were selected based on having less or no interference from the fishing line. The area (pixels2) and mean intensity (mean gray value) of the target reflections for each macroplastic were measured using open-source ImageJ2 software (Rueden et al., 2017).
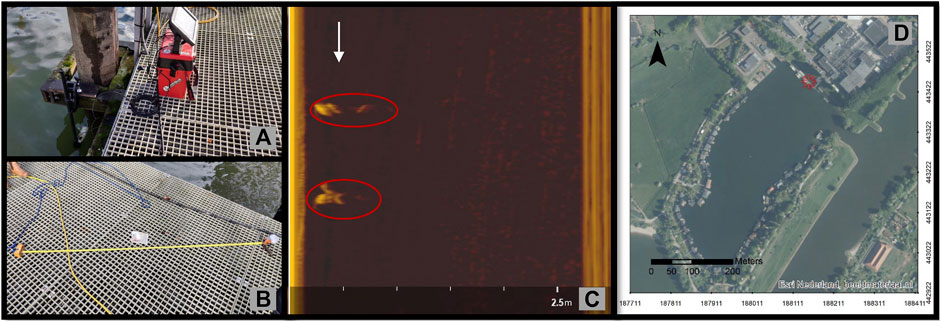
FIGURE 2. (A) The set-up of the SSS in the dock of the Rosandepolder RWS facility for the semi-controlled experiment in standing water, (B) a plastic object (lunch meat packet) and the wood float and towing rope, (C) a view of the plastic bottle as it appeared twice in the sonar image when moved on the starboard side of the transducer (white arrow indicates the direction of towing), and (D) map of the Rosandepolder RWS facility used for the SSS standing water test. The exact location is denoted by the red asterisk. Photos by N.Y. Flores. Aerial images source: Environmental Systems Research Institute (ESRI) Nederland, beeldmateriaal.nl.
2.3 Statistical Analysis
The mean intensity and area of the target reflections were checked statistically in order to find any differences between the macroplastics tested. The data for each standing water experiment was checked for normality using a Shapiro test in R statistics version 4.0.4. For not normally distributed data a Kruskal–Wallis test was completed to check if the area and mean intensity of the target reflections were significantly different between macroplastics. Post hoc pairwise-comparisons were performed with the Wilcoxon Rank Sum test with p-values adjusted using the Benjamini and Hochberg (1995) method to identify which macroplastics were significantly different from each other (R Core Team, 2021). Normally distributed data were checked using a one-way ANOVA. Post hoc pairwise-comparisons were performed with the Tukey’s honest significance test to identify which macroplastics were significantly different from each other (R Core Team, 2021). Statistical significance were tested at p-values < 0.05.
3 Results
3.1 ARIS Test in Standing Water
During the test, the water in the dock was clear and the bottom (∼2 m depth) was fully visible. Thus the orientation of the sonar and the shapes, sizes and orientations of the targets within the beams fan were visually confirmed real-time in the water and in ARIScope. The bottom at the test location was clearly visible in the sonar images, including tree branches and rocks. These objects were distinguishable from the tested macroplastics and even a distinctive reflection was sometimes appreciable for the fishing line, albeit it depended on its orientation. The ARIS sonar successfully detected both types of polymers (i.e., opaque and transparent macroplastics). The area and mean intensity of the target reflections differed between macroplastics (Figure 3). The mean area of the macroplastic reflections ranged from 627 to 12948 pixels2. The mean intensity of the target reflections ranged from 40 to 84. In standing water, there was 100% detection of the plastic pieces tested down to a 1 cm2 piece of a food container.
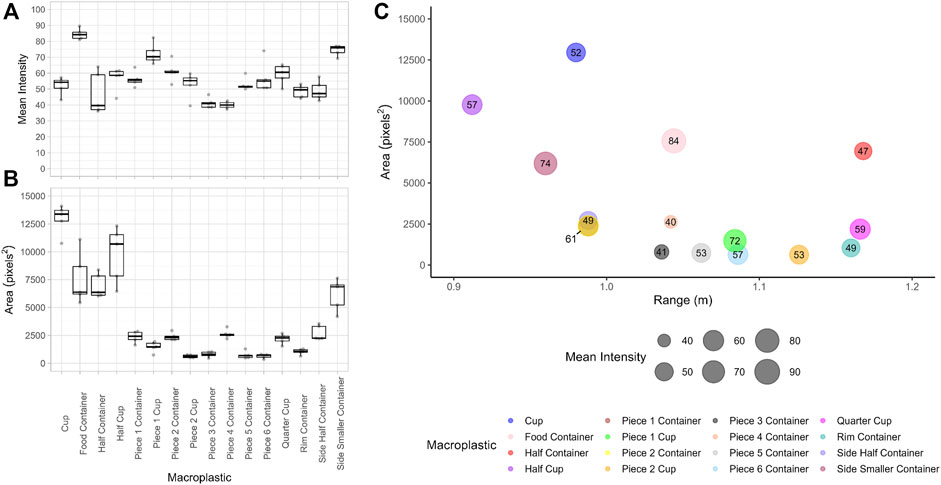
FIGURE 3. Box plots of (A) the mean intensity and (B) the area of the macroplastic reflections (n = 5) from the ARIS sonar images as detected in standing water. The center lines represent the median values, the edges of the boxes the first and third quartiles, the whiskers the minimum and maximum, and the underlying data points appear in grey. (C) Unique mean reflections for each type of macroplastic given by the mean area (y-axis) and mean intensity (bubble sizes/values) at mean range (x-axis) for the ARIS sonar images as detected in standing water.
The areas of the target reflections were not normally distributed (p-value < 0.001), neither were the mean intensities (p-value < 0.05). The Kruskal–Wallis tests showed that the areas of the target reflections were significantly different between macroplastics (χ2 = 73.34, df = 15, p-value < 0.001). Post hoc pairwise-comparisons showed significant differences (p-value < 0.05) between the areas of the target reflections of several macroplastics with some larger objects not showing significant differences between each other. The same was the case for some of the smaller objects. The area of the reflection of the cup was significantly different from all the other macroplastics tested (p-value < 0.05; See Supplementary Table S3). Similarly, the mean intensities of the reflections were significantly different between macroplastics (χ2 = 57.071, df = 15, p-value < 0.001). Post hoc pairwise-comparisons showed significant differences (p-value < 0.05) between the mean intensity of the target reflections of several macroplastics, yet some objects made of the different polymers (i.e. opaque and transparent) did not show significant differences from each other (See Supplementary Table S4).
3.2 ARIS Test in Flowing Water
During the test in the shore channel near Dreumel, the riverbed was visible including algae in motion that was attached to rocks. There were also more artifacts in the sonar images from what seem to be water bubbles and particles suspended in the water column. These artifacts and the high flow velocities, which decreased exposure time to the beams of the sonar, often resulted in poor quality images that made the targets hard to visually interpret. In one data recording (i.e., small wires test) a foreign object was observed passing through the beams fan. Both transparent and opaque plastics were detected. However, only 67% of the macroplastics (n = 27) tested were interpreted as detected in the sonar images. Detected objects included a bag, cup, jug, glove, bottle cap, vegetable container, wires, coffee cup half, cottage cheese container, band-aid, Capri-Sun container, wrapper, coffee cup lid, foil, straw, macroplastics pieces, macroplastics pieces (smaller) and the side of a food container. Not detected were a fork, golf ball, shore protection textile, small cup, sanitary napkin, firework tube and small wires. Unclear detection was assigned to a noodle seasoning packet (See Supplementary Table S5).
3.3 Side-Scan Sonar Test in Standing Water
In the SSS backscatter images reflections were seen for all of the macroplastics (i.e., opaque and transparent) tested. Raw SSS data recorded showed weak reflections while testing the fishing line and the zip tie used to attach the objects. However, during the macroplastics test the fishing line was often not in the sonar images as it remained close to the water surface, below the object and/or out of the vertical reach of the side imaging of the sonar. The area and mean intensity of the target reflections differed between macroplastics (Figure 4). The mean area of the macroplastic reflections ranged from 335 to 963 pixels2. The mean intensity of the target reflections ranged from 47 to 79. This standing water test resulted in 100% detection of the macroplastics tested.
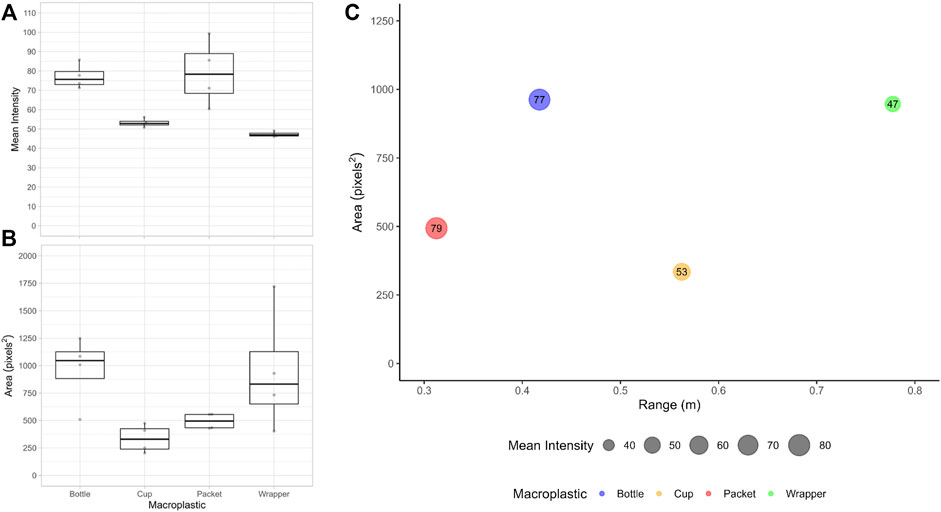
FIGURE 4. Box plots of (A) the mean intensity and (B) the area of the macroplastic (n = 4) reflections from the SSS images as detected in standing water. The center lines represent the median values, the edges of the boxes the first and third quartiles, the whiskers the minimum and maximum, and the underlying data points appear in grey. (C) Unique mean reflections for each type of macroplastic given by the mean area (y-axis) and mean intensity (bubble sizes/values) at mean range (x-axis) for the SSS images as detected in standing water.
The areas of the target reflections were not normally distributed (p-value < 0.05), whereas the mean intensities of the target reflection were normally distributed (p = 0.07283). The Kruskal–Wallis test showed that the areas of the target reflections were significantly different between macroplastics (χ2 = 8.1176, df = 3, p-value < 0.05). However, post hoc pairwise-comparisons did not show significant differences between the areas of the reflections of the macroplastics (See Supplementary Table S6). Similarly, the one-way ANOVA test showed that the mean intensities of the target reflections were significantly different between macroplastics (df = 3, Sum Sq = 3,188, Mean Sq = 1,062.6, F-value = 12.63, p-value < 0.001). Post hoc pairwise-comparisons showed significant differences between the mean intensity of the target reflections of some macroplastics, yet the transparent plastic lunch meat packet and water bottle were not significantly different from each other. The opaque plastic sanitary napkin wrapper and cup were also not significantly different from each other (See Supplementary Table S7).
4 Discussion
Our pilot study showed that an ARIS sonar as well as a SSS 1) were able to detect macroplastics in the water column while tested in standing water, and 2) the resulting mean target reflections differed between the macroplastics tested. The ARIS sonar was also able to detect macroplastics in the water column of a river channel under flowing conditions.
4.1 ARIS Test in Standing Water
The areas of the target reflections generally increased with the size of macroplastics (Figure 3). This was also observed by Broere et al. (2021), using a CHIRP echosounder for macroplastic detection. Mean intensities of the reflections differed between the macroplastics. This was expected since the two polymers used in the test had different densities (PPρ = 0.85–0.92 and PETρ = 1.38–1.41; Morét-Ferguson et al., 2010), yet there was also some overlap between the results. Weiland (2019) found similar reflection coefficients for different types of plastics that were close to those of substrate such as sand. These coefficients could indicate the intensity of a reflection for a material as they are calculated based on the density of materials and depend on the angle of incidence, or the angle at which sound waves hit the object. Hence, some of the overlap in the results could be due to the different angles of incidence. This most likely resulted from changes in position and orientation of the targets with respect to the sonar during the test. This possibly led to over- or underestimations of the reflection parameters as the objects were more or less exposed to the beams (Chandrashekar, 2014). However, it is expected that objects will change position and orientation during monitoring efforts. Moreover, the distance to targets will likely be larger during monitoring. Therefore, the test in flowing water is a more realistic approach for testing the usefulness of this technology for macroplastic monitoring purposes. In our approach detection efficiency may be subjective as it is based on the user doing the visual interpretation, however, we did not notice a difference between the different types and sizes of macroplastics.
4.2 ARIS Test in Flowing Water
The flowing water changed the initial position and orientation of the macroplastics. Moreover, it could have deformed, moved objects away from the beams fan, or into the blind zone of the sonar leading to poor quality images, unclear detection or undetected objects. This since some relatively large objects were not detected while smaller ones were. Overall, the flow resulted in noise and distortion in the sonar images which made visual interpretation of the targets difficult. Consequently, detection decreased from 100% in standing water to 67% in flowing water. Nonetheless, a similar detection percentage for fish in a small river (62.6%) was reported, using an ARIS sonar and checking against fish catches with a net (Egg et al., 2018). The ARIS sonar seems to be adequate for macroplastic detection from a static position at locations with low flow velocities (<0.8 m/s).
A limitation of the ARIS sonar in plastic detection is the horizontality of the beams fan. The target position was only resolved horizontally in 2D, meaning that it was not possible to determine the depth of the target in the water column. Nevertheless, the high resolution of the resulting images, make it possible to not only detect macroplastics in the water column, but also to often collect data on the general shape, size, orientation and direction of travel of targets (Figure 1C). Since our study aimed at testing the detection of macroplastics, we used the low frequencies of the imaging sonars. It should be noted that higher frequencies lead to sonar images with higher spatial resolution, but also reduce the range of detection (Klein and Edgeton, 1968; Sound Metrics, 2020).
More data should be collected to fully assess and model the potential of this technology for plastic detection in the field using plastics collected by monitoring programs. For future research, higher frequencies could be useful to improve the detection efficiency of this approach. This could also allow for the identification of macroplastics in the water column as the ARIS sonar is able to operate at 3 MHz. Jing et al. (2018) recommended the use of ARIS sonar images collected from different positions in order to obtain 3D images and positions of targets. This was accomplished by using an ARIS sonar in motion (Jing et al., 2018). Two ARIS sonars used simultaneously could also be used to test the potential of this technique for the 3D imaging and tracking of plastics objects. This sonar technology may be useful to obtain more complete spatial data on plastic pollution in riverine ecosystems. Further, the ARIS sonar also shows potential to assess the bed transport of macroplastics. Hereafter future work should also focus on testing the sensitivity of this technology in flowing water using larger distances to plastic targets. Additional data could then be used to train models in order to enhance the plastic detection efficiency and accuracy by using machine learning algorithms as in Broere et al. (2021) and Valdenegro-Toro (2019). Additionally, the selection of an appropriate monitoring location with low flow velocities (<0.8 m/s), more laminar flow and spatially limited to the range of the sonar may help to further improve the detection efficiency. This since noise was evident in the images and would likely increase in more turbulent flow conditions. Testing in locations with higher flow velocities might be feasible if the sonar can be secured enough to avoid vibration and any related noise. Moreover, Martí et al. (2020) found that only 47% of plastic pollution in the ocean is composed of white and transparent plastics, thus testing more plastics of different colors remains important when aiming to further elucidate any differences or similarities between the reflection intensities of opaque and transparent plastics. Other characteristics of plastics such as their structure may also impact the reflections (Sappé, 2020). Finally, Lacerda et al. (2022) found highly biodiverse biofilms accumulating on plastics as they persist in the environment, thus the effects of biofilm on target reflections should also be assessed in future research.
4.3 Side-Scan Sonar Test in Standing Water
The SSS data showed that the areas of reflection generally increased with the size of macroplastics (Figure 4). This correlates with findings by Broere et al. (2021) using CHIRP technology. Two of the polymers used in the experiment had similar densities (PPρ = 0.85–0.92 and LDPEρ = 0.89–0.93; Morét-Ferguson et al., 2010) while the third was denser (PETρ = 1.38–1.41; Morét-Ferguson et al., 2010). Denser materials should result in more intense reflections and less dense materials in less intense ones (Klein and Edgeton, 1968). The mean intensity of the target reflections were different between the macroplastics tested, yet the bottle which was made of the densest polymer was not significantly different from the lunch meat packet which was less dense. The same was observed for the cup and the wrapper, which were composed of less dense opaque plastics. This was possibly partially due to other physical characteristics of the materials, such as transparency and texture. Sappé (2020) suggests that the structure of plastics when detected by an MBES also appears to have an impact on the target reflections. Just like in SSS substrate mapping, detection efficiency may be subjective and dependent on the user that is visually interpreting the objects in the sonar images (Kaeser et al., 2013; Litts and Kaeser, 2016).
The macroplastics were often placed close to the transducer. The towing of objects could have caused noise and distortion of the plastic, especially the soft wrapper, leading to an over- or underestimation of the reflection parameters (Chandrashekar, 2014; Broere et al., 2021). This differs from the way plastics would move towards the transducer in flowing water. However, the combination with CHIRP provides the 3D position of targets due to a combination of down and side imaging. Moreover, this technology is very low-cost. These advantages give it the potential to be used not only for macroplastic detection in the water column but also for monitoring bed transport of macroplastics. Additionally, SSS can be used mounted on a boat or on a static surface. This sonar operated at lower frequencies than the ARIS sonar, resulting in images with lower resolution and thus less detail.
Future testing should use the higher frequency setting of the Lowrance SSS (800 kHz) in order to produce higher resolution images. Another future research aim should be to assess the maximum range that could be used to detect the targets. More plastic types and colors should be used when retesting SSS technology for suspended plastic detection. Investigating any effects that biofilm accumulation on plastics may have on their reflections is also recommended. The classification of the images should also be automated by using machine learning for more efficient and accurate detection.
5 Conclusion
The ARIS sonar and the SSS were able to detect 100% of the macroplastics tested in standing water. In the field under flowing conditions up to ∼0.8 m/s, the ARIS sonar was able to detect 67% of the macroplastic tested, which consisted of plastic litter collected during plastic monitoring campaigns. The ARIS sonar was more effective at detecting objects in standing water as it was able to detect transparent and opaque plastics, with one target as small as 1 cm2 in size. This sonar is promising for the detection, counting and identification of macroplastics including their shape, size, orientation, direction of travel and 2D horizontal position in the water column. For future monitoring stations, the use of an ARIS sonar in motion or two ARIS sonars operating simultaneously could help to provide 3D spatial information and images of suspended macroplastics. The SSS with CHIRP sonar is a low-cost option for plastic detection and monitoring which may be mounted on a boat or used from a static position. This sonar is also promising for suspended plastics detection as it provided the 3D position of targets, but in lower resolution images than the ARIS sonar.
Data Availability Statement
The datasets presented in this study were stored in the DANS EASY repository of the Radboud University, Nijmegen (https://doi.org/10.17026/dans-zq4-p7dz).
Author Contributions
Acquiring financial support and supervision: FPLC and RSEWL. Conceptualization: NYF and FPLC. Methodology: NYF and FPLC. Data collection: NYF, FPLC, and SBO. Data analysis and visualization: NYF. First draft: NYF. Reviewing and editing: NYF, SBO, RSEWL, and FPLC.
Funding
Rijkswaterstaat Oost Nederland provided the funds to rent the ARIS sonar and to construct the mount for testing. No formal RWS projects are linked to this study.
Conflict of Interest
The authors declare that the research was conducted in the absence of any commercial or financial relationships that could be construed as a potential conflict of interest.
Publisher’s Note
All claims expressed in this article are solely those of the authors and do not necessarily represent those of their affiliated organizations, or those of the publisher, the editors and the reviewers. Any product that may be evaluated in this article, or claim that may be made by its manufacturer, is not guaranteed or endorsed by the publisher.
Acknowledgments
We are very grateful to Margriet Schoor (Rijkswaterstaat Oost Nederland) for renting and facilitating the use of the ARIS sonar and test locations. Further, we would like to thank Jan-Willem Mol (Rijkswaterstaat Centrale Informatievoorziening) for designing and commissioning the construction of the ARIS sonar mount and for his help during the fieldwork and throughout the process of organizing the data collection. We also would like to thank Demi van Klink and Sophie Broere for their help during the ARIS test in the Dreumel shore channel and side-scan sonar experiments, respectively. We are also grateful to András Szöllősi-Nagy and two reviewers for their valuable recommendations for improving this article.
Supplementary Material
The Supplementary Material for this article can be found online at: https://www.frontiersin.org/articles/10.3389/fenvs.2022.875917/full#supplementary-material
References
Anima, R., Wong, F. L., Hogg, D., and Galanis, P. (2007). Side-scan Sonar Imaging of the Colorado River. Open-File Report 2007–1216. Grand Canyon: U.S. Geological Survey Virginia, 4.
Benjamini, Y., and Hochberg, Y. (1995). Controlling the False Discovery Rate: a Practical and Powerful Approach to Multiple Testing. J. R. Stat. Soc. Ser. B Methodol. 57, 289–300. doi:10.1111/j.2517-6161.1995.tb02031.x
Broere, S., Van Emmerik, T., González-Fernández, D., Luxemburg, W., De Schipper, M., Cózar, A., et al. (2021). Towards Underwater Macroplastic Monitoring Using Echo Sounding. Front. Earth Sci. 9, 598. doi:10.3389/feart.2021.628704
Chandrashekar, L. (2014). Characteristics of Sonar Sensors for Short Range Measurement. Int. J. Eng. Res. Technol. 3 (11), 340–344.
Cheshire, A. C., Adler, E., Barbière, J., Cohen, Y., Evans, S., Jarayabhand, S., et al. (2009). UNEP/IOC Guidelines on Survey and Monitoring of Marine Litter. UNEP Regional Seas Reports and Studies No. 186. Paris: United Nations Environment Programme, 120. IOC Technical Series No. 83.
Cole, M., Lindeque, P., Fileman, E., Halsband, C., Goodhead, R., Moger, J., et al. (2013). Microplastic Ingestion by Zooplankton. Environ. Sci. Technol. 47 (12), 6646–6655. doi:10.1021/es400663f
Collas, F. P. L., Buijse, A. D., Van den Heuvel, L., Van Kessel, N., Schoor, M. M., Eerden, H., et al. (2018). Longitudinal Training Dams Mitigate Effects of Shipping on Environmental Conditions and Fish Density in the Littoral Zones of the River Rhine. Sci. Total Environ. 619-620, 1183–1193. doi:10.1016/j.scitotenv.2017.10.299
Collas, F. P. L., Oswald, S. B., and Verberk, W. C. E. P. (2021). Plastic in de waterkolom van de Boven-Rijn. Waal en IJssel, Series of Reports on Animal Ecology and Physiology 2021-4. Nijmegen: Radboud University, 17.
Cook, D., Middlemiss, K., Jaksons, P., Davison, W., and Jerrett, A. (2019). Validation of Fish Length Estimations from a High Frequency Multi-Beam Sonar (ARIS) and its Utilisation as a Field-Based Measurement Technique. Fish. Res. 218, 59–68. doi:10.1016/j.fishres.2019.05.004
D'Hont, A., Gittenberger, A., Leuven, R. S. E. W., and Hendriks, A. J. (2021). Dropping the Microbead: Source and Sink Related Microplastic Distribution in the Black Sea and Caspian Sea Basins. Mar. Pollut. Bull. 173, 112982. doi:10.1016/j.marpolbul.2021.112982
De Moustier, C., and Matsumoto, H. (1993). Seafloor Acoustic Remote Sensing with Multibeam Echo-Sounders and Bathymetric Sidescan Sonar Systems. Mar. Geophys Res. 15 (1), 27–42. doi:10.1007/BF01204150
Egg, L., Pander, J., Mueller, M., and Geist, J. (2018). Comparison of Sonar-, Camera- and Net-Based Methods in Detecting Riverine Fish-Movement Patterns. Mar. Freshw. Res. 69, 1905. doi:10.1071/MF18068
Emmerik, T., and Schwarz, A. (2019). Plastic Debris in Rivers. WIREs Water 7, e1398. doi:10.1002/wat2.1398
Flores, N. Y., Collas, F. P. L., Mehler, K., Schoor, M. M., Feld, C. K., and Leuven, R. S. E. W. (2022). Assessing Habitat Suitability for Native and Alien Freshwater Mussels in the River Waal (the Netherlands), Using Hydroacoustics and Species Sensitivity Distributions. Environ. Model. Assess. 27, 187–204. doi:10.1007/s10666-021-09776-4
Free, C. M., Jensen, O. P., Mason, S. A., Eriksen, M., Williamson, N. J., and Boldgiv, B. (2014). High-levels of Microplastic Pollution in a Large, Remote, Mountain Lake. Mar. Pollut. Bull. 85 (1), 156–163. doi:10.1016/j.marpolbul.2014.06.001
Garaba, S. P., Aitken, J., Slat, B., DierssenLebreton, H. M. L., Lebreton, L., Zielinski, O., et al. (2018). Sensing Ocean Plastics with an Airborne Hyperspectral Shortwave Infrared Imager. Environ. Sci. Technol. 52 (20), 11699–11707. doi:10.1021/acs.est.8b02855
Hohenblum, P., Frischenschlager, H., Reisinger, H., Konecny, R., Uhl, M., Mühlegger, S., et al. (2015). Plastik in der Donau-Untersuchung zum Vorkommen von Kunststoffen in der Donau in Österreich. REP-0547. Vienna: Umweltbundesamt-BOKU Report REP.
Jing, D., Han, J., and Zhang, J. (2018). A Method to Track Targets in Three-Dimensional Space Using an Imaging Sonar. Sensors 18, 1992. doi:10.3390/s18071992
Jones, R. E., Griffin, R. A., and Unsworth, R. K. F. (2021). Adaptive Resolution Imaging Sonar (ARIS) as a Tool for Marine Fish Identification. Fish. Res. 243, 106092. doi:10.1016/j.fishres.2021.106092
Kaeser, A. J., Litts, T. L., and Tracy, T. W. (2013). Using Low-Cost Side-Scan Sonar for Benthic Mapping throughout the Lower Flint River, Georgia, USA. River Res. applic. 29, 634–644. doi:10.1002/rra.2556
Klein, M., and Edgerton, H. (1968). Sonarߝ a Modern Technique for Ocean Exploitation. IEEE Spectr. 5 (6), 40–46. doi:10.1109/mspec.1968.5214684
Koelmans, A. A., Kooi, M., Law, K. L., and Van Sebille, E. (2017). All Is Not Lost: Deriving a Top-Down Mass Budget of Plastic at Sea. Environ. Res. Lett. 12, 114028. doi:10.1088/1748-9326/aa9500
Lacerda, A. L. D. F., Taylor, J. D., Rodrigues, L. D. S., Kessler, F., Secchi, E., and Proietti, M. C. (2022). Floating Plastics and Their Associated Biota in the Western South Atlantic. Sci. Total Environ. 805, 150186. doi:10.1016/j.scitotenv.2021.150186
Lau, W. W. Y., Shiran, Y., Bailey, R. M., Cook, E., Stuchtey, M. R., Koskella, J., et al. (2020). Evaluating Scenarios toward Zero Plastic Pollution. Science 369 (6510), 1455–1461. doi:10.1126/science.aba9475
Lebreton, L. C. M., van der Zwet, J., Damsteeg, J.-W., Slat, B., Andrady, A., and Reisser, J. (2017). River Plastic Emissions to the World's Oceans. Nat. Commun. 8, 15611. doi:10.1038/ncomms15611
Lechner, A., Keckeis, H., Lumesberger-Loisl, F., Zens, B., Krusch, R., Tritthart, M., et al. (2014). The Danube So Colourful: A Potpourri of Plastic Litter Outnumbers Fish Larvae in Europe's Second Largest River. Environ. Pollut. 188, 177–181. doi:10.1016/j.envpol.2014.02.006
Litts, T. L., and Kaeser, A. J. (2016). Mapping Potential Spawning Substrate for Shortnose and Atlantic Sturgeon in Coastal Plain Rivers of Georgia Using Low-Cost Side-Scan Sonar. J. Southeast. Assoc. Fish Wildl. Agencies 3, 80–88.
Martí, E., Martin, C., Galli, M., Echevarría, F., Duarte, C. M., and Cózar, A. (2020). The Colors of the Ocean Plastics. Environ. Sci. Technol. 54 (11), 6594–6601. doi:10.1021/acs.est.9b06400
Meijer, L. J. J., Van Emmerik, T., Van der Ent, R., Schmidt, C., and Lebreton, L. (2021). More Than 1000 Rivers Account for 80% of Global Riverine Plastic Emissions into the Ocean. Sci. Adv. 7 (18), aaz5803. doi:10.1126/sciadv.aaz5803
Moore, C. J., Lattin, G. L., and Zellers, A. F. (2011). Quantity and Type of Plastic Debris Flowing from Two Urban Rivers to Coastal Waters and Beaches of Southern California. Rgci 11, 65–73. doi:10.5894/rgci194
Moore, C. J. (2008). Synthetic Polymers in the Marine Environment: A Rapidly Increasing, Long-Term Threat. Environ. Res. 108 (2), 131–139. doi:10.1016/j.envres.2008.07.025
Morét-Ferguson, S., Law, K. L., Proskurowski, G., Murphy, E. K., Peacock, E. E., and Reddy, C. M. (2010). The Size, Mass, and Composition of Plastic Debris in the Western North Atlantic Ocean. Mar. Pollut. Bull. 60 (10), 1873–1878. doi:10.1016/j.marpolbul.2010.07.020
Morritt, D., Stefanoudis, P. V., Pearce, D., Crimmen, O. A., and Clark, P. F. (2014). Plastic in the Thames: a River Runs through it. Mar. Pollut. Bull. 78, 196–200. doi:10.1016/j.marpolbul.2013.10.035
Naik, M. S., Supnekar, S. P., and Pawar, P. R. (2021). Assessment of Marine Debris and Plastic Polymer Types along the Panvel Creek, Navi Mumbai, West Coast of India. Ijzi 7 (1), 278–293. doi:10.33745/ijzi.2021.v07i01.023
OSPAR Commission (2010). Guideline for Monitoring Marine Litter on the Beaches in the OSPAR Maritime Area. AvalaibleAt: https://www.ospar.org/ospar-data/10-02e_beachlitter%20 guideline_ english%20only.pdf (Accessed June 11, 2021).
R Core Team (2021). R: A Language and Environment for Statistical Computing. Vienna, Austria: R Foundation for Statistical Computing. AvalaibleAt: https://www.R-project.org/(Accessed April 20, 2021).
Richter, J. T., Sloss, B. L., and Isermann, D. A. (2016). Validation of a Side-Scan Sonar Method for Quantifying Walleye Spawning Habitat Availability in the Littoral Zone of Northern Wisconsin Lakes. North Am. J. Fish. Manag. 36 (4), 942–950. doi:10.1080/02755947.2016.1173141
Rueden, C. T., Schindelin, J., Hiner, M. C., DeZonia, B. E., Walter, A. E., Arena, E. T., et al. (2017). ImageJ2: ImageJ for the Next Generation of Scientific Image Data. BMC Bioinforma. 18, 529. doi:10.1186/s12859-017-1934-z
Sappé, N. (2020). How to Find Plastic with a Multibeam. Leeuwarden: Braveheart Survey B.V NHL Stenden University of Applied Sciences, 41. Bachelor thesis.
Schmidt, C., Krauth, T., and Wagner, S. (2017). Export of Plastic Debris by Rivers into the Sea. Environ. Sci. Technol. 51 (21), 12246–12253. doi:10.1021/acs.est.7b02368
Schöneich-Argent, R. I., Dau, K., and Freund, H. (2020). Wasting the North Sea? - A Field-Based Assessment of Anthropogenic Macrolitter Loads and Emission Rates of Three German Tributaries. Environ. Pollut. 263, 114367. doi:10.1016/j.envpol.2020.114367
Schreyers, L., Van Emmerik, T., Nguyen, T. L., Phung, N.-A., Kieu-Le, T.-C., Castrop, E., et al. (2021). A Field Guide for Monitoring Riverine Macroplastic Entrapment in Water Hyacinths. Front. Environ. Sci. 9, 716516. doi:10.3389/fenvs.2021.716516
Schwarz, A. E., Ligthart, T. N., Boukris, E., and Van Harmelen, T. (2019). Sources, Transport, and Accumulation of Different Types of Plastic Litter in Aquatic Environments: a Review Study. Mar. Pollut. Bull. 143, 92–100. doi:10.1016/j.marpolbul.2019.04.029
Shahrestani, S., Bi, H., Lyubchich, V., and Boswell, K. M. (2017). Detecting a Nearshore Fish Parade Using the Adaptive Resolution Imaging Sonar (ARIS): An Automated Procedure for Data Analysis. Fish. Res. 191, 190–199. doi:10.1016/j.fishres.2017.03.013
Sound Metrics (2020). ARIS Explorer 3000. AvalaibleAt: http://www.soundmetrics.com/Products/ARIS-Sonars/ARIS-Explorer-3000/015335_RevD_ARIS-Explorer-3000_Brochure (Accessed April 10, 2020).
Thompson, R. C., Olsen, Y., Mitchell, R. P., Davis, A., Rowland, S. J., John, A. W. G., et al. (2004). Lost at Sea: where Is All the Plastic? Science 304 (5672), 838. doi:10.1126/science.1094559
Topouzelis, K., Papakonstantinou, A., and Garaba, S. P. (2019). Detection of Floating Plastics from Satellite and Unmanned Aerial Systems (Plastic Litter Project 2018). Int. J. Appl. Earth Observation Geoinformation 79, 175–183. doi:10.1016/j.jag.2019.03.011
Valdenegro-Toro, M. (2019). Deep Neural Networks for Marine Debris Detection in Sonar Images. Edinburgh: Heriot-Watt University, 28–106. PhD thesis.
Van Emmerik, T., Mellink, Y., Hauk, R., Waldschläger, K., and Schreyers, L. (2022b). Rivers as Plastic Reservoirs. Front. Water 3, 786936. doi:10.3389/frwa.2021.786936
Van Emmerik, T., Vriend, P., and Copius Peereboom, E. (2022a). Roadmap for Long-Term Macroplastic Monitoring in Rivers. Front. Environ. Sci. 9, 802245. doi:10.3389/fenvs.2021.802245
Keywords: ARIS sonar, mesoplastic, plastic pollution, rivers, side-scan sonar
Citation: Flores NY, Oswald SB, Leuven RSEW and Collas FPL (2022) Underwater Macroplastic Detection Using Imaging Sonars. Front. Environ. Sci. 10:875917. doi: 10.3389/fenvs.2022.875917
Received: 14 February 2022; Accepted: 24 May 2022;
Published: 24 June 2022.
Edited by:
Anik Bhaduri, Griffith University, AustraliaReviewed by:
Tim van Emmerik, Wageningen University and Research, NetherlandsJoão P. G. L. Frias, Galway-Mayo Institute of Technology, Ireland
Copyright © 2022 Flores, Oswald, Leuven and Collas. This is an open-access article distributed under the terms of the Creative Commons Attribution License (CC BY). The use, distribution or reproduction in other forums is permitted, provided the original author(s) and the copyright owner(s) are credited and that the original publication in this journal is cited, in accordance with accepted academic practice. No use, distribution or reproduction is permitted which does not comply with these terms.
*Correspondence: Natasha Y. Flores, bi5mbG9yZXNAc2NpZW5jZS5ydS5ubA==