- 1School for Environment and Sustainability, University of Michigan, Ann Arbor, MI, United States
- 2Landscape Architecture and Environmental Planning, Utah State University, Logan, UT, United States
Globally, geodiversity and the associated ecosystem services it provides, are both under threat from anthropogenic pressures and environmental changes. The UNESCO Global Geoparks Programme was established to create protected areas that not only preserve geodiversity but enhance human wellbeing and cultural heritage. Understanding the role geodiversity plays in providing such ecosystem services is necessary for providing holistic conservation priorities that ensure their sustainable use. Studies have extensively used social media data to assess cultural ecosystem services, however, the photographic viewable area, which demonstrates scenic or areas of interest, has been scarcely evaluated. Studies often take crude measurements of viewsheds rather than isolating viewed content. Here, we gather georeferenced images taken inside geopark boundaries from the social media website Flickr as a proxy for aesthetically pleasing viewpoints. To understand what landscape characteristics are visible from these viewpoints we calculate viewshed analysis following the novel visual magnitude approach. The use of magnitude viewshed provides a metric for visual relevancy compared to traditional viewshed approaches that only provide a binary metric representing visible or non-visible. Here, we find that the use of the visual magnitude methodology provides richer information about what is visible than from traditional viewshed analysis. We find that aesthetically appreciated views as inferred by social media within the Troodos Geopark, Cyprus, do not only include natural features such as forest and water but are often related to geodiversity and anthropogenically altered landscapes such as mineral extraction sites and vineyards, for which the geopark is known. These results can help to inform geoconservation practices with the goal of sustainable access and enjoyment of aesthetic services.
Introduction
Ecosystem services (ES), the material and non-material benefits we gain from nature are threatened by a wide range of environmental changes and anthropogenic pressures (Díaz et al., 2018). Following the Common International Classification of Ecosystem Services (CICES) (Haines-Young and Potschin-Young, 2018), ES can be classified into three main categories of services: provisioning, regulating and cultural, while a fourth category of regulating or supporting services facilitate their production. ES are produced through our interactions with biodiversity (biotic nature) and geodiversity (abiotic nature) (Gray, 2012; Fischer and Eastwood, 2016; Fox et al., 2020b). Furthermore, geosystem services (GS) are services that geodiversity generates in the absence of interaction with biodiversity (Gray, 2012; Fox et al., 2020b). For example, provisioning services such as rare earth metals, construction materials and ornate materials; regulating services such as thermal flows, geochemical cycles, and carbon regulation (Van Ree and Van Beukering 2016). Furthermore, geodiversity contributes to the non-material benefits associated with cultural ecosystem services (CES). For example, natural features like caves and mountains that add to the perceived scenic or beauty of nature (Kubalíková et al., 2021), and materials derived from geodiversity used as a building material promoting a sense of place, e.g., liós, a distinct whitish limestone used in the palaces and cathedrals of Lisbon, Portugal (da Silva 2019). Geodiversity and GS also contribute to knowledge acquisition from features such as sediments and ice cores (Gray 2012). However, research and management practices tend to focus on biodiversity rather than geodiversity. To target conservation efforts to areas of high scenic appreciation, a better understanding of the aesthetic appreciation of geodiversity is needed. This will enable a more complete understanding of the aesthetic relationship between biodiversity, geodiversity, and human features, and lead to more holistic and management and conservation methods.
Several definitions of geodiversity appear in the literature often flexible for and tailored to the study approach (Fox et al., 2020b). Definitions have been inclusive of the diversity of all abiotic nature (e.g., Benito-Calvo et al., 2009) as well as focused on specific geological features (e.g., Ruban, 2010). Our definition is inclusive of a broad array of abiotic nature including “features and processes of geology, geomorphology, sediments and soils, and hydrology” (Gray, 2013; Fox et al., 2020b). The variety in definitions is also reflected in the different methods used to map and measure geodiversity. Some studies use qualitative approaches such as expert opinion or descriptive documentation to measure geodiversity while others use quantitative approaches such as geodiversity indices or landscape metrics (Zwoliński et al., 2018). These methodology approaches both have benefits and disadvantages, for instance, qualitative studies are more easily repeated than qualitative studies but are limited by the availability of suitable datasets (Zwoliński et al., 2018).
As well as its values in contributing to ES and GS, geodiversity has conservation value. Geodiversity and the ES it produces are at risk of loss or destruction from environmental and anthropogenic pressures, including urbanisation and climate change (Hjort et al., 2015). While geodiversity conservation, or geoconservation, is still not a widely known or accepted concept, often not a priority in the nature conservation agenda (Crofts, 2022), in 2022 UNESCO formally acknowledged it by establishing an annual International Geodiversity Day (6th of October). The new annual International Geodiversity Day complements previous efforts achieved through the UNESCO Global Geoparks Programme, which aims to raise the profile of geodiversity for geoconservation. Global Geoparks have an expansive conservation mandate that not only includes preserving geodiversity but also aims to benefit local peoples, particularly through promoting the sustainable use of cultural ecosystem services through geotourism (a form of tourism analogous to ecotourism) and educational opportunities (Prosser, 2013). Furthermore, involving Indigenous peoples can benefit geoconservation efforts as their knowledge of the land can help to provide meaningful input into conservation plans and maintain Indigenous cultural values. For example, the Stonehammer UNESCO Global Geopark, New Brunswick promotes sustainable geotourism and education while excluding members of the public from Indigenous sacred sites (Slaymaker et al., 2020).
Recreational and geotourism activities may contribute to the destruction and degradation of geodiversity features and processes (Hjort et al., 2015), resulting in a decline in an ecosystem’s capacity to generate ES (Fox et al., 2020b). For example, increased footfall from tourism can exacerbate erosion, non-compliance of geotourists leaving designated pathways to touch geological formations can cause degradation, while other geotourists may remove specimens such as rocks and fossils from the site to keep as a “souvenir” (Gray, 2008; Newsome and Dowling, 2018). Cases of geotourist based destruction have been widely documented. In the Swabian Alb UNESCO Global Geopark, Germany, trampling has caused damage to fragile calcerous tufa rocks (Megerle, 2021), while in the Bohemian Paradise UNESCO Global Geopark, Czech Republic, sandstone rocks are often damaged by visitors engraving into the rocks (Drápela, 2021). Furthermore, mismanagement of geodiversity features such as modifying specimens or destruction through infrastructure creation can diminish the geoheritage value of the geodiversity features and processes. For example, in the Wudalianchi UNESCO Global Geopark, China, the installation of lighting and ice-carvings has degraded the natural condition of the valuable permafrost lava tube that originally attracted geotourists (Newsome and Dowling, 2018). Examples of geodiversity destruction are often due to poor geoconservation strategies, however, even processes perceived as destructive such as mining can be beneficial to the production of geoheritage values if carried out in a responsible manner (Németh et al., 2021a). Therefore, to ensure sustainable use, geodiversity should be conserved in relation to natural processes, not just preserved, requiring monitoring and active management (Burek and Prosser, 2008).
As the aesthetic benefits of geodiverse landscapes are at risk of degradation through a range of different actions there is a need to better understand the pathways from which aesthetic services arise so we can best manage landscapes (Schirpke et al., 2016). Aesthetic services are personal and unique to everyone and are based on an individual’s emotional responses to their surroundings (Figueroa-Alfaro and Tang, 2017). Due to the personal aspect of aesthetic services, previous studies often relied on surveys or interviews to untangle human-nature relationships. However, obtaining biodiversity, geodiversity, and social datasets useful for understanding these relationships are often constrained by the high financial costs and time-intensive methods associated with traditional data collection techniques like intercept surveys (Wood et al., 2013). Furthermore, biases may be introduced to social datasets through the choice of collection methods, data processing and analytical methods, for example, filtering the data based on an author’s preconceived assumptions (Olteanu et al., 2019). Here, social media datasets, such as those from Flickr, Twitter, and Reddit, are an invaluable resource, due to their high spatio-temporal resolution and the relative ease with which they can be obtained (Fox et al., 2020a). In particular, social media datasets are effective at assessing a range of cultural ecosystem services including aesthetic appeal (Figueroa-Alfaro and Tang, 2017; Van Berkel et al., 2018; Johnson et al., 2019); recreational activities (Mancini et al., 2018; Graham and Eigenbrod, 2019; Fox et al., 2021a); spiritual and religious beliefs (Thiagarajah et al., 2015; Fox et al., 2021b); and identifying trends in geoeducation and geoconservation at tourist attractions (Németh et al., 2021b). However, not all social media posts are related to CES, for example, posts may not pertain to interactions with nature (e.g., photographs of food, text posts about a film), and where posts are about interactions with nature, they may not reflect a positive relationship (e.g., a picture of a fallen tree accompanied text post about damage from a flood). Social media datasets may therefore need classifying to better represent these positive interactions between people and nature, with previous studies filtering posts based on the content of images (Gosal et al., 2019; Zhao and Han, 2021), or sentiment expressed in textual metadata (Fox et al., 2021a). Here, we address sentiment in terms of where the social media post reflects a positive attitude towards nature (Johnson et al., 2021).
While Flickr data offer a geolocated photo and some form of semantic description, these data do not establish the context within which the observer is viewing. One method to establish context and identify what that person sees around them is by producing a viewshed analysis - a computational method that determines what areas are visible from a given location. A viewshed analysis provides a spatial representation of all the areas an individual can see on the landscape by calculating the 360° area that is theoretically visible from a discrete location based on the line-of-sight of a viewer and excluding points that are obstructed by the terrain and other features (e.g., buildings, trees). Innovative studies using social media data have assessed aesthetic services using viewshed analysis (Figueroa-Alfaro and Tang, 2017; Van Berkel et al., 2018). Traditional methods of viewshed analysis, however, are limited as they assume equal visibility for near and far features as a binary (visible or not visible) map, not accounting for the variability in an observer’s view based on the surrounding landscape (Chamberlain and Cook, 2019). To rectify this, visual magnitude enhances viewshed analysis by calculating the amount of space that a visible area occupies within the view of the observer by quantifying the effect of slope, aspect, and distance of an area relative to the observer (Chamberlain and Meitner, 2013). Visual magnitude provides an absolute continuous value ranging from 0 to 1. A pixel with a value of 0 represents a pixel that is not visible, while a pixel with a value of 1 would represent an area that occupies 100% of the viewer’s perspective and therefore be the only pixel visible. Values falling between 0 and 1 are representative of the degree of visibility (Chamberlain and Meitner, 2013; Chamberlain et al., 2015).
Data from Flickr has previously been used to assess the relationship between geodiversity and cultural ecosystem services (Fox et al., 2022), geoconservation (Németh et al., 2021b), as well as aesthetic services (Figueroa-Alfaro and Tang, 2017; Van Berkel et al., 2018). However, to date, no such study exists that uses Flickr data to assess aesthetic services where geodiversity or geoconservation are intersected with the visual context the user engages with. Therefore, the aim of this paper is to assess geodiversity’s role in aesthetic appreciation using novel datasets and innovative methodologies. We evaluate these methods for capturing these complex spatial-visual interactions assessing both the validity of using social media data and the information gained from magnitude viewshed analysis. Our main objectives include:
• Objective 1: assesses whether the filtering of social media data into different sentiments (pro-nature/irrelevant) impacts on the representation of visible land cover
• Objective 2: understand whether the characterization of land cover types and geodiversity is fundamentally different between the traditional and visual magnitude viewshed analysis
• Objective 3: evaluate which landscape characteristics contribute to visually appealing views within the Troodos Geopark, Cyprus.
Methods
We use social media posts from the website Flickr as a proxy to identify aesthetically pleasing viewpoints within the Troodos Geopark, Cyprus. To test the added value of using the novel magnitude viewshed analysis, we calculate and compare two viewshed metrics; visual magnitude values (hence referred to as visual magnitude viewshed) and the number of visible pixels within each land cover (hence referred to as traditional viewshed). Furthermore, we assess the impact of filtering social media posts on the results drawn from viewshed assessments. Using these results, we can infer which landscape characteristics are providing aesthetically pleasing views.
Study Site
The Troodos Geopark, designated in 2015, is a 137,000-ha protected area situated in central Cyprus (Figure 1). Predominantly dominated by mountainous terrain with an elevation range of 1652 m, its highest peak is the famed Mount Olympus at 1,952 m (Bukała et al., 2016) (Figure 2). The geopark is characterised by its ophiolite complex where the oceanic crust and the underlying upper mantle has been uplifted and exposed above sea level (Bukała et al., 2016). Its designation as a geopark is related to how this unique geology has shaped the area’s landscapes. As part of its protective status, park management is tasked with promoting sustainability and education, focusing on the local geodiversity, flora, fauna and mining practices from the region, while also providing a range of tourism and recreational opportunities. For example, the visitor centre has exhibitions on geological history and the abandoned Asbestos and Chromite mines (Figure 2), while the wider park has several hiking “geo-trails,” such as the Teichia tis Madaris Geo-Trail and Artemis Geo-Trail, which highlight the biodiversity and geodiversity of the region. There are also a number of villages and hamlets within the park boundaries, including Troodos, Argos and Ikos.
Social Media Data
Geotagged posts on social media websites are a good proxy for aesthetically pleasing viewpoints (Figueroa-Alfaro and Tang, 2017; Zhao and Han, 2021). Therefore, to assess the aesthetic benefits of the geopark, we mined photographs from the photo-sharing site Flickr. Flickr is a popular photograph and video hosting website, which has an Application Programming Interface (API) through which metadata for images including their georeferenced location can be gathered (flickr.com/services/api). Images taken within the park between 1 January 2000 and 1 January 2022 were gathered using the park boundaries (UNEP-WCMC IUCN, 2021). Data collection was carried out in the R environment (R Core Team, 2021) using the “photosearcher package” (Fox et al., 2020a). See Supplementary Material S1 for a code snippet for Flickr data collection.
Social media data is often messy and requires some pre-processing (Ghermandi and Sinclair, 2019). Biases in the distribution of photographs may be influenced by single users taking multiple photographs at a single point, or from busier urban areas. Biases by busy areas such as urban districts or single users taking lots of photographs. Some authors have tried to normalize the skewed distribution of Flickr data by transforming visitation rates. (Németh et al., 2021b). While other studies clean social media data using the “Photo User Days” (PUD) metric introduced by Wood et al. (2013), filters social media data to represent a single photograph per user per day. However, applying the PUD metric across the whole study site means that if a user travels around the park and takes photographs from different areas on a single day these photographs would be aggregated to only a single point. We, therefore, filtered the photographs to represent a single photograph from each user at each unique latitude and longitude. This did not add a minimum distance between points as even slight changes in elevation or location could drastically alter the potential view. The final filtered dataset consisted of 2,227 unique georeferenced images.
Textual sentiment analysis is often carried out using a predefined dictionary, applying an emotional scale to a word. For example, the AFIN dictionary (Nielsen 2011) rates words on a scale of –5 to +5 while the NRC dictionary (Mohammad & Turney 2013) attributes an emotion such as “fear” or “trust” to different words. While this “word association” approach offers a simple and transparent method for assessing textual sentiment, the choice of dictionary can influence results and the polarity of sentences. To solve these well-known issues, the “classecol” R package (Johnson et al., 2021) applies 11 different dictionaries to a piece of text to better calculate the expressed sentiment. Furthermore, the sophisticated model of polarity better captures the complexities of expressed sentiment by assessing and accounting for the valence of the text including negators (“e.g., “I am not happy”), amplifiers (e.g., “I am very happy”), de-amplifiers (e.g., “I am not that happy”), and adversative conjunction terms (e.g., “I am happy, but sad”). Furthermore, leveraging the classecol package provides functionality to classify whether a piece of text discusses nature in tandem with the sentiment of the text. The text can therefore be classified as: irrelevant (prose not about nature); positive-nature (positive phrasing); positive-nature (negative phrasing e.g., concern for nature); and against-nature. Here we applied the “classecol” classifier to the text associated with Flickr images assessing the title, tag and description contained in the metadata. When a Flickr user posts an image, they simultaneously add descriptors as part of the title, text, or tags. The final classified photographs belonged to three of the four “classecol” classes: irrelevant (n = 1605); pro-nature (positive phrasing) (n = 611); pro-nature (negative phrasing) (n = 11); and against-nature (n = 0). Subsequent analysis was carried out on the pro-nature (positive phrasing) (hence referred to as positive-nature) and irrelevant images, due to the low instances of pro-nature (negative phrasing) and against-nature images.
Environmental Data
To assess how landscape geodiversity contributes to the aesthetic benefits of the park, we calculated the viewsheds for these photographic points, then intersected these visible areas with land cover maps. We assume that the land cover visible from the topographically diverse area contributes both to the aesthetic appreciation and overall experience of the landscape for the photographer. Previous research suggests that geodiversity generates biodiversity through habitat diversity, resource availability and niche variety (Parks and Mulligan, 2010; Bailey et al., 2017), which influences the functional trait diversity and phylogenetic diversity of the area (Pepper et al., 2013; Cheesman et al., 2018). While geodiveristy underpins habitat diversity across a range of scales from landscapes to smaller isolated systems such as caves (Hjort et al., 2015), it is not always plainly visible. Furthermore, both the magnitude and direction of geodiversity-biodiversity relationships are highly context and spatially dependent, and it is therefore possible to have higher biodiversity without high geodiversity (Ren et al., 2021). Previous studies used land cover to calculate aspects of geodiversity in spatial assessments (Jankowski et al., 2020), as well as a proxy for aesthetic values (Van Berkel et al., 2018). We, therefore, use landcover as a measure of aesthetic appealing landscape characteristics generated by geodiversity features. For our calculations, we used the Corine Land Cover (CLC) 2018 European land cover dataset (European Union, Copernicus Land Monitoring Service, 2021), which has a minimum mapping unit (MMU) of 25 ha, to understand which landscape characteristics may be providing aesthetic values to geopark visitors (Supplementary Material S2).
Visual Magnitude Viewshed Analysis
Viewsheds were calculated based on the image coordinates from the metadata and a 25 m digital surface model (DSM) (European Union, Copernicus Land Monitoring Service, 2021) (Supplementary Material S3). We limited our analysis to the park boundary clipping the DSM to this area. While this may have omitted distant landscape covers captured in photographs and experienced by the photographer, a pilot test of visual magnitude shows exceedingly minimal impact for these features outside the park. The pilot test used a random subset of 500 photographs and the full DSM of Cyprus. However, using the full extent of the DSM greatly increased the time taken to calculate the viewshed for each point without providing additional information on aesthetics within the Geopark. Our viewsheds analysis uses a novel technique that better describes the visual magnitude of a location as described above. Magnitude viewshed was carried out in ArcPro using the “magnitude viewshed” plugin as described in Chamberlain and Cook (2019). The magnitude viewshed plugin allows for two types of outputs to be written: the visual magnitude viewshed for each individual point and an aggregated mean visual magnitude viewshed for a group of points.
This method is often computationally intensive, especially at high resolutions. Research suggests that there are few accuracy trade-offs in calculating viewshed at a lower resolution and decreased computational time using visual magnitude (Hoffman et al., 2021). To ensure that the resolution of the DSM did not impact the results we varied the resolution analysis, resampling the 25 m raster to a 100 m cell using the nearest neighbour assignment. We compared the value of overlapping pixels at each location using the ratio of the covariance between the two layers divided by the product of their standard deviations to test for spatial correlation. Correlation of +1 represents a positive relationship (as the value in a pixel at one location increases the corresponding pixel in the other raster increases), 0 no relationship, and −1 a negative relationship (as the value in a pixel at one location increases the corresponding pixel in the other raster decreases) (Taylor, 1990). Though this comparison showed virtually no variation between the two rasters (correlation of 0.99962) and using the aggregated raster would have had minimal impact on the viewshed analysis, we chose to carry out the rest of the analysis using the original 25 m raster.
Objective 1: Impact of Filtering Social Media by Sentiment
To test the association between different sentiments we calculated the visual magnitude for positive-nature and irrelevant photographs. For both classes of sentiment, we calculated both the visual magnitude for each individual photograph and the aggregated mean visual magnitude value for all photographs that express that sentiment. We then calculated a spatially explicit correlation between the two aggregated mean visual magnitudes. The correlation was calculated using the ratio of the covariance between the positive-nature and irrelevant photographs’ visual magnitude outputs divided by the product of their SDs, with the result potentially ranging from −1 to +1. To map these relationships between the two layers, we classified correlation classes: <0.35 as weak correlation; 0.36–0.67 as a modest correlation; 0.68–0.89 a high correlation and >0.9 a very high correlation (Taylor, 1990). We did not test for statistical significance as it will likely show as significant regardless of the correlation coefficient due to the large sample size associated with the number of pixels (Taylor, 1990).
Areas of the highest visual magnitude across the entire site were highlighted by classifying the mean visual magnitude into two categories using the geometric intervals method (highest and lowest magnitude values). This resulted into two maps, one showing areas of high visual magnitude for positive-nature photographs and one showing areas of high visual magnitude for irrelevant photographs. We mapped the difference in distribution of these highest magnitude value maps.
Objective 2: Effect of Viewshed Method Land Cover Visibility
The calculated percentage of visible land cover could be influenced by two factors, the method of calculating viewshed (sum of visual magnitude versus pixel count) and the use of differently classified points (positive-nature versus irrelevant). Therefore, to address how land cover characterisation is impacted by choice of viewshed method, as well the classification of textual sentiment we, calculated a two-way ANOVA for each of the 26 individual land cover classes. The response variable for the ANOVA was the percentage visibility of that landcover class from each viewpoint given the independent variables of viewshed method and sentiment classification. As ANOVA tests are robust to the deviation of normality (Blanca Mena et al., 2017; Schmider et al., 2010), they have been a useful method of assessing non-normal data in cultural service and landscape studies (e.g., Schlesinger et al., 2020). We, therefore, analysed all data including those that have non-normal distributions. Statistical significance alone does not explain the size of the effect and therefore we calculated effect size using the partial eta squared (ηp2). Partial eta squared has previously been used in landscape preference research and allows for comparison across studies (Lakens, 2013; Lindquist et al., 2016).
The characterization of land cover types when assessing aesthetic services are often calculated or assessed in terms of landscape diversity (Hermes et al., 2018; Albert et al., 2019). It is therefore important to understand whether the method of calculating visibility impacts diversity matrices. Here, we used the positive-nature photographs and calculated land cover visibility as a percentage (both the magnitude sum and pixel count) for each individual viewpoint. We calculated diversity using Simpson’s diversity index as it is a suitable method for calculating land cover diversity using percentage cover (Nagendra, 2002). Simpson’s diversity ranges from 0 to 1, with scores closer to 1 indicating a higher diversity. The diversity values calculated from the two methods (magnitude sum and pixel count) were compared using a two-tailed t-test.
Objective 3: Landscape Aesthetics in the Troodos Geopark
To assess which land cover types may be providing aesthetic appreciation we assess visible land cover using social media photograph locations in comparison to those available across the park. Using the results of the two previous objectives, we can make an informed decision that using positive-nature images and magnitude viewshed analysis will provide a robust interpretation of aesthetic qualities. Therefore, we calculated the visual magnitude for each individual positive-nature image, and using the land cover data, summed the visual magnitude values inside each land cover type. Using a two-tailed t-test, the percentage of land cover visible from these viewsheds was then compared against the true percentage that land cover type accounts for across the DSM extent. As the results of a two-tailed t-test for most land cover types indicated a significant difference, we further investigated the direction of the effect - whether the significant difference was due to the land cover percentage visible from social media posts being less than or more than the percentage of land cover for the entire area. For each land cover we calculated two one-tailed t-tests: 1) a one-tailed less than t-test to assess whether the percentage of land cover visible is less than the known actual land cover coverage for the area and 2) a one-tailed more than t-test to assess whether the percentage of land cover visible is more than the known actual land cover coverage for the area. Where the one-tailed less than test shows a significant difference, these land covers are underrepresented by social media posts, and in those where the one-tailed more than test was significant, these land covers are overrepresented by social media posts. Our assumption is that uploading images to Flickr reveals locations and features that people perceived to be attractive and scenic. Comparing the viewsheds at these locations with all other locations throughout the geopark using a t-test indicates the reveals preferences for landscape characteristics when given the opportunity to photograph any landcover type. Furthermore, we extracted and mapped the land covers associated with areas of high visual magnitude for the positive-nature images (categorized into highest and lowest values using geometric intervals).
Results
Social Media Data
The relatively high number of positive-nature images and absence of against-nature classes of images highlight a generally positive sentiment in regard to the park’s landscape aesthetics. The spatial distribution of the points is similar across the irrelevant and positive-nature images, indicating a possible spatial autocorrelation (Figure 3). A high number of photographs were found in the surroundings of Mount Olympus, as well as the visitor centre and its mines. There were also a high number of images near villages and hamlets.
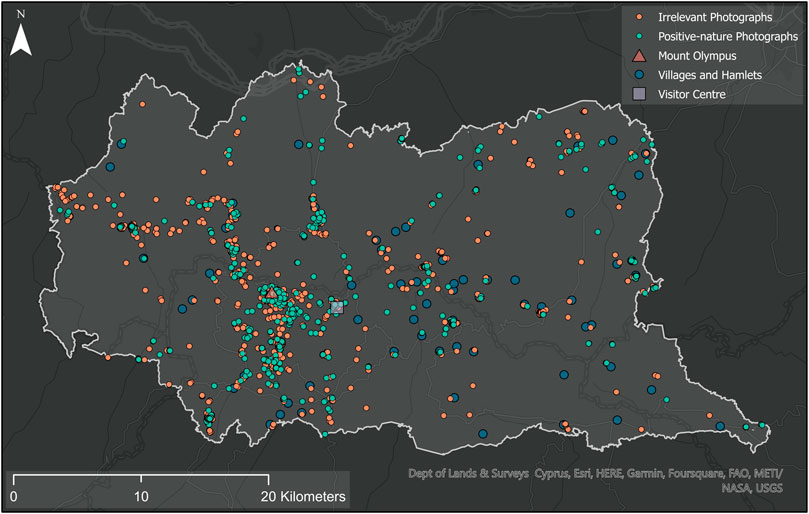
FIGURE 3. Distribution of Flickr images captured within the Troodos Geopark boundaries, taken and uploaded between 1 January 2000 and 1 January 2022.
Visual Magnitude Viewshed Analysis
Graphical comparison of the two viewshed analyses (traditional and visual magnitude) indicates equal areas that are visible but demonstrate substantially different contexts. In Figure 4, the traditional viewshed analysis shows areas that are quantitatively equally visible across the entire range, with some visible areas up to 6.7 km away. Whereas the visual magnitude indicates a gradient of visibility across the range. The value of 0.36, shown as the darkest green, indicates that a 25 m cell occupies roughly 3.6% of the viewer’s field of view. The value of 1.34e−9, shown as the lightest green, indicates that a 25 m cell occupies a near-incomprehensible percentage of the viewer’s field of view. At first glance, the visual magnitude appears as though values decrease in relation to distance. However, closer inspection reveals some areas further away from the viewpoint are a darker green than areas closer. These areas indicate that topography plays an important role because the slope and direction of that cell are more apparent to the viewer. In summary, the traditional viewshed is giving a substantially higher weight to the landscape at a distance that may be imperceivable.
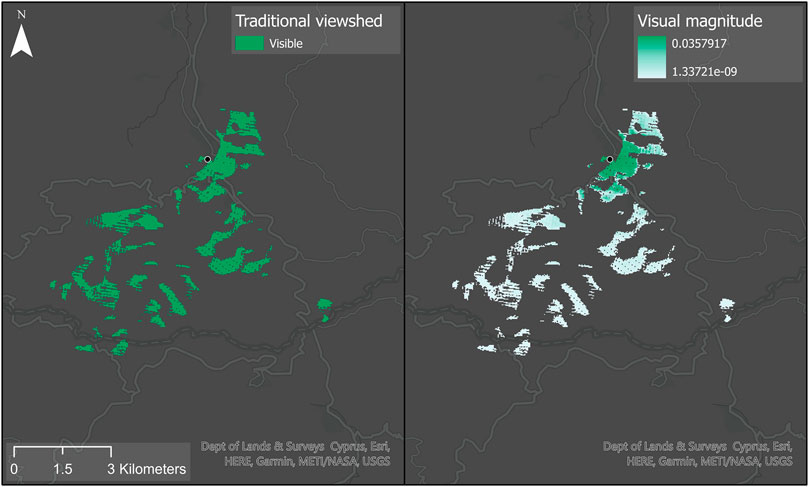
FIGURE 4. Example comparison of visual magnitude and traditional viewshed. Map A is the output of the traditional viewshed for a single point. Map B is the output of visual magnitude for a single point.
Objective 1: Impact of Filtering Social Media by Sentiment
From the full viewshed average rasters (Supplementary Material S4) there is a moderate/strong positive correlation (0.68), suggesting that filtering photographs based on textual sentiment can produce differences in the calculated visual magnitude results. While aggregated visual magnitude outputs for positive-nature and irrelevant photographs share many areas of high visual magnitude, there are specific differences between these classed values (Figure 5). For example, both classifications of photographs have a high visual magnitude in the southwest and east of the park, while sporadic patches of high visual magnitude from the positive-nature images can be found throughout the centre of the park, particularly surrounding Mount Olympus.
Objective 2: Effect of Viewshed Method Land Cover Visibility
Results of the 26 individual ANOVAs indicate a significant difference between methods for calculating viewsheds (traditional versus visual magnitude), as well as between the image classes (positive-nature versus irrelevant) (Table 1 and Figure 6). Methods of viewshed analyses resulted in more significant differences than text classification type, with 20 out of 26 land cover types being significantly influenced by method and only five out of 26 by photograph classification. Dump sites (e.g. commercial or industrial waste sites, including waste rock sites), mineral extraction sites, non-irrigated arable land and vineyards were statistically different across classification and viewshed methods, while natural grasslands, olive groves, pastures, and permanently irrigated land were not significantly different in either. For land covers that have a significant value in the method, this represents land covers whose visibility has been calculated significantly differently when using either visual magnitude or traditional viewshed. Where land cover types are significantly different across sentiment classification this suggests that the visual magnitude of viewsheds of the positive-nature images have different visibility photographs than those classed as irrelevant. As shown by the partial-eta values for some of the significantly different land cover findings there was a medium effect size introduced by the choice of method. For example, for discontinuous urban fabric, 12% of the variance is explained by the choice of the viewshed method. There was however no large, medium or small effect size introduced by the classification of sentiment.
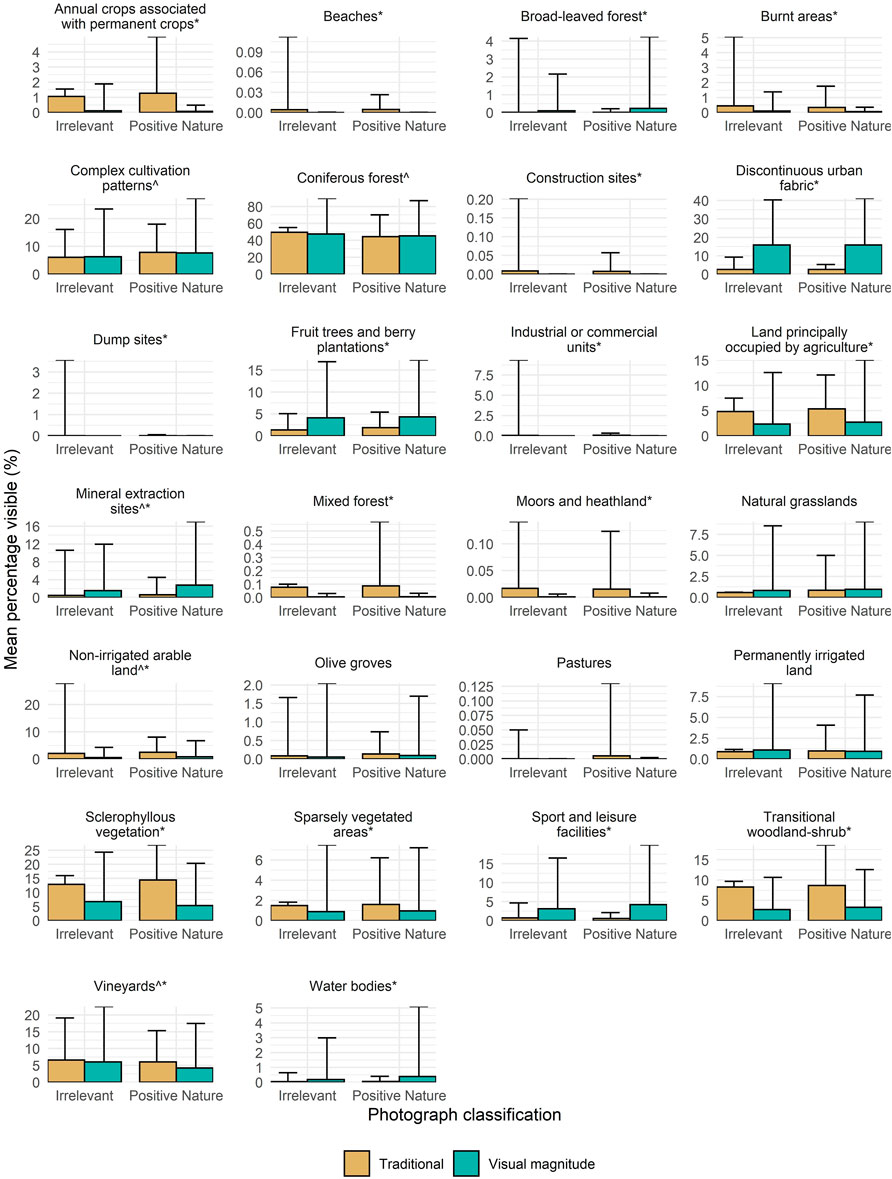
FIGURE 6. Average land cover percentage aggregated across all viewpoints (mean + 1 SD). ^Textual sentiment class has a significant effect, *method of viewshed has a significant effect.
The characterization of land cover diversity calculated by the two methods (visual magnitude and traditional) show different patterns. The two-sample t-test showed a significant difference in the calculated Simpson diversity index depending on whether the percentage of visible land cover was calculated using the sum of the magnitude viewshed or purely the number of visible pixels (t = 24.53, df = 1200.7, p < 0.05). A test for skewness shows that using the count of pixels results in left skew (−1.37) while the sum of magnitude viewshed has almost no skewness (−0.08) (Figure 7). Therefore, when using binary, visible or not pixel counts, there is a higher calculated Simpsons diversity for land cover than when using the sum of magnitude viewshed values.
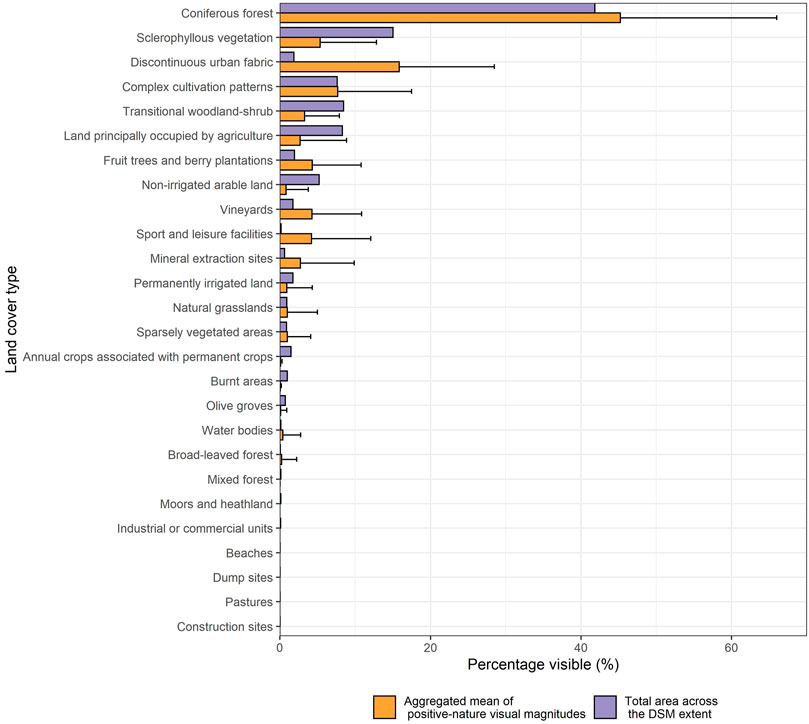
FIGURE 7. Land cover (mean + 1 SD) from positive-nature photograph viewpoints and actual percentage land cover across the study site.
Objective 3: Landscape Aesthetics in the Troodos Geopark
The most dominant land cover classes across the extent of the DSM were coniferous forests (41.85%), sclerophyllous vegetation (15.02%), transitional woodland-shrub (8.47%), land principally occupied by agriculture (8.29%), and complex cultivation patterns (7.61%) (Figures 8, 9). The results of the averaged viewsheds of the positive-nature and irrelevant images using visual magnitude show different land cover viewing compared to those dominant in the park. The land cover types captured from positive-nature images were coniferous forests (x̄ = 45.22%), discontinuous urban fabric (x̄ = 15.85%), complex cultivation patterns (x̄ = 7.66%), sclerophyllous vegetation (x̄ = 5.33%) and vineyards (x̄ = 4.25%) (Figure 7). Comparing these to the land cover present across the DSM we find that discontinuous urban fabric (x̄ = 15.85) and mineral extraction sites (x̄ = 2.73) are more prominent, while mixed forest (x̄ = 0.005) and Sclerophyllous vegetation (x̄ = 5.33) less prominent (Table 2). Land covers such as natural grassland and sparsely vegetated areas had no significant difference compared to the total land cover.
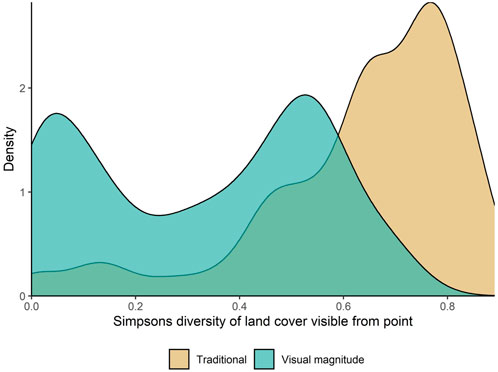
FIGURE 8. Density plot of Simpsons diversity indices across the sum of visual magnitude values and pixel count.
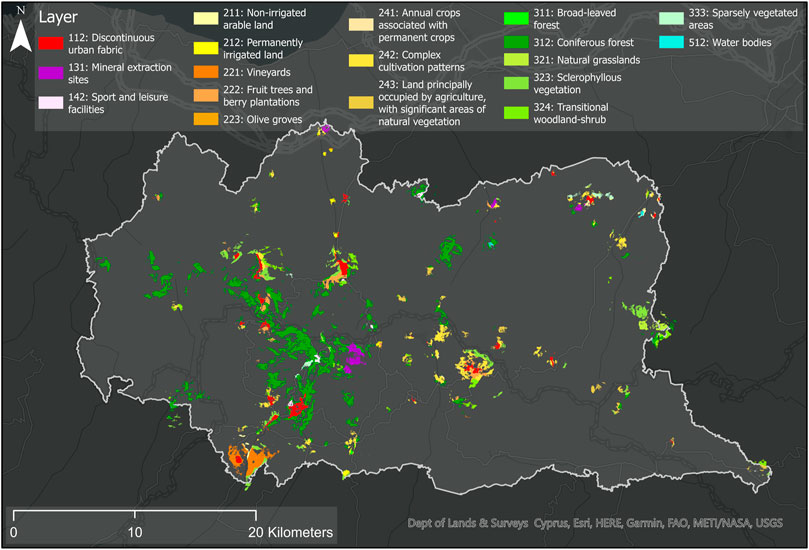
FIGURE 9. Land cover associated with the areas of highest visual magnitude for the positive-nature images.
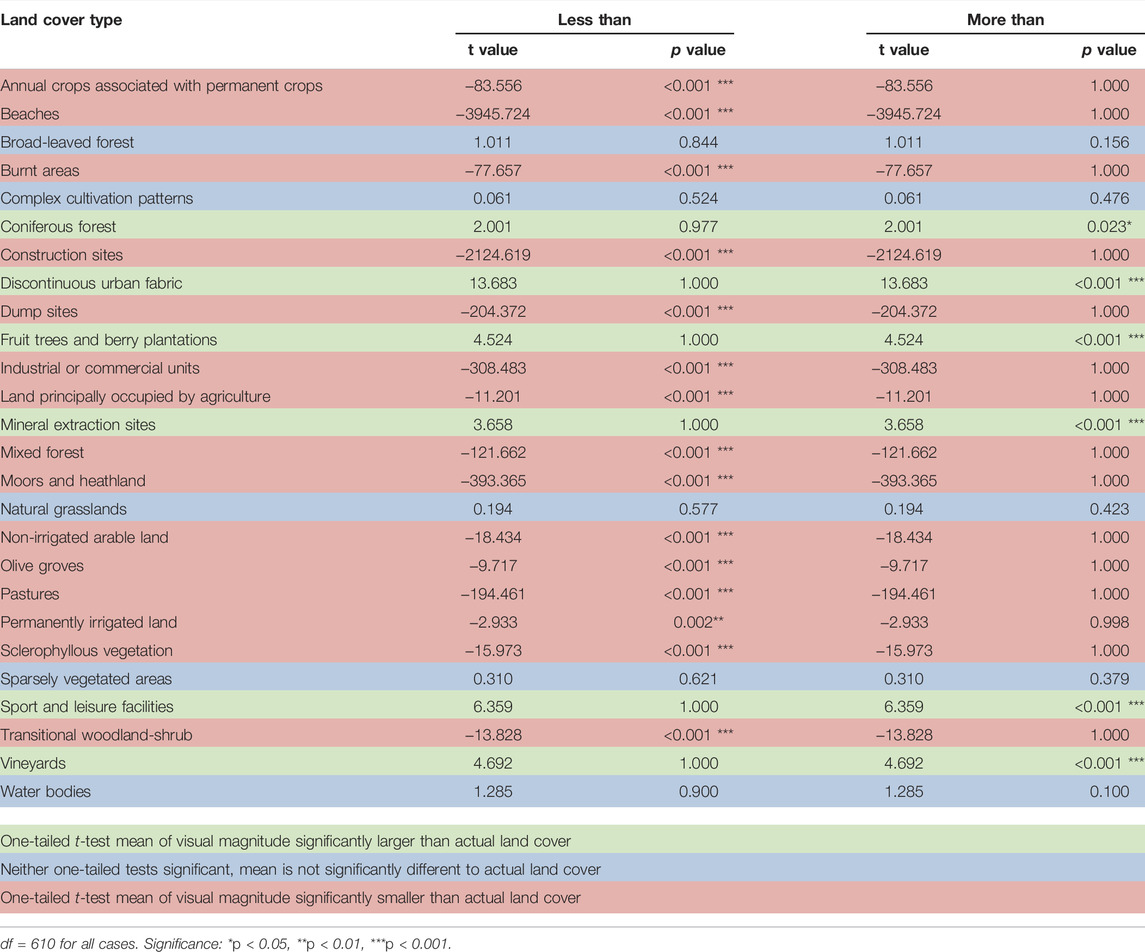
TABLE 2. One sample t-test of land cover percentages from the visual magnitude viewsheds of positive-nature Flickr images and the percent land cover visible across the DSM.
Discussion
Big data from social media websites offer a unique way to study the complex interactions between geodiversity, biodiversity and people generated in Geoparks CES (Fox et al., 2021a). Here we combined social media data from Flickr with the novel visual magnitude approach to viewshed analysis in order to better understand aesthetic CES in a prime example of a UNESCO Global Geopark. By understanding which areas of the Troodos Geopark have the highest associated scenic beauty, park decision-makers can utilise these results to better inform targeted geoconservation. For example, by understanding areas of high associated aesthetic appeal, management plans can be developed to ensure that these areas are not overused, damaging the integrity of the area’s geodiversity and biodiversity and associated aesthetic views (Clemente et al., 2019). The method presented here is not only applicable for understanding scenic areas from social media users’ photographic locations. It can also be used to visualise the visual impact of proposed park management, such as forestry or road construction, and therefore help guide the mitigation of significant visual impacts (Chamberlain et al., 2015).
Objective 3: Landscape Aesthetics in the Troodos Geopark
By using visual magnitude, we have shown non-natural land cover types such as mineral extraction sites and discontinuous urban fabric are often visible from the locations social media pictures are taken, particularly compared to the total land cover across the study site. As with other studies, these results demonstrate that urban areas may not negatively impact aesthetic qualities, but instead may play a role in their provision (Langemeyer et al., 2018; Zhao and Han, 2021), offering a unique story or important history of development within a geodiverse landscape. CES, including visual aesthetics, are therefore not only produced by nature but may also be co-produced by people, including through built infrastructure (Fischer and Eastwood, 2016). These results suggest that the people are not only visiting these areas for natural scenic beauty but also are engaging with the social-cultural aspects of the parks.
A major attraction of the Troodos Geopark is the number of historic mines that showcase the unique geology of the area. As a result of a potentially negative practice, these anthropogenically altered landscapes in fact enhance aesthetic appreciation by revealing the unique geodiversity features such as exposed rock walls and water bodies (Bétard, 2013). Though these mines do not represent a large percentage of the wider landscapes’ total land cover, they are relatively highly represented in the viewshed of photographs from social media websites. Furthermore, the Troodos Geopark is known for local wine production (Pijet-Migoń and Migoń, 2021), and the overrepresentation of vineyards in social media views demonstrate that they serve as CES benefit, e.g., aesthetic views of vineyards coupled with experiencing cultural heritage. Promoting sustainability in geoparks will not only require paying attention to geodiversity but also providing economic stability for local people, and these interactions of visitors and the social-cultural park elements reinforce that local communities and the local economy can benefit from geotourism and recreational visitors (Sagala et al., 2018).
Not all overrepresented land cover types were non-natural. For example, coniferous forests had proportionally higher visibility compared to the area’s total land cover. As with previous studies, these results demonstrate that forested areas can have a vital role in providing aesthetically pleasing landscapes (van Berkel and Verburg, 2014; van Zanten et al., 2014). We also found that water bodies had a relatively high representation thus highlighting their importance in providing aesthetically pleasing landscapes (Schirpke et al., 2016; Van Berkel et al., 2018). This difference in natural and non-natural land cover representation aligns with previous studies that found geopark visitors can be classified into two groups, “soft visitors” those who visit geoparks for attractions such as visitor centres and “hard visitors” those who visit geoparks to explore areas of intrinsic beauty (Cheung et al., 2014).
Objective 2: Effect of Viewshed Method Land Cover Visibility
Though the distribution of what is visible does not vary between the visual magnitude and traditional viewshed metrics, the additional data associated with visual magnitude can provide an enriched understanding of what people are looking at. The visual magnitude plug-in allows for analysis of a single point (e.g., a viewing platform) or multiple points along a line (e.g., along a trail), or multiple points across a given space (such as here). By weighting visibility based on visual magnitude, decision-makers can more accurately and precisely determine areas of greater aesthetical importance compared to the traditional viewshed, enabling them to make more informed decisions regarding potential alterations to aesthetic quality. For example, decision-makers may reject proposed landscape management when using the traditional viewshed for fears of it degrading the view, but in reality, the area of proposed management may only account for an infinitesimally small proportion of the viewer’s field of view. This difference is likely to be introduced by pixels closer to the viewpoint often being assigned a greater visual magnitude weighting as land cover closer to the user will often take up a greater proportion of the field of view (Chamberlain and Meitner, 2013; Chamberlain et al., 2015).
Objective 1: Impact of Filtering Social Media by Sentiment
The potential of using semantic analysis as a scalable method for understanding CES is increasingly being explored (Fox et al., 2021a). While the method seems promising, its efficacy for understanding actual preference has been scarcely investigated. Though others have found little spatial autocorrelation between positive and negative images at large scales (Fox et al., 2021a), our results indicate that this may not be the case for all datasets derived from Flickr. To better understand how social media data can elicit information on CES, it is therefore imperative that the relationship between positive and negative posts be further explored.
Our tests that groups images into positive-nature and irrelevant from the associated text show that sentiment differences explain some differences in landscape appreciation. Comparing the calculated percentage of land cover visible from these different points in the ANOVA tests indicated a significant difference between mineral extraction sites and non-irrigated arable land. This was also found when comparing land cover diversity between positive-nature and irrelevant images. However, a significant difference was not found for other land cover types e.g., beaches and broad-leaved forests. Furthermore, there was no large effect size when using positive-nature images compared to irrelevant images. The importance of classifying social media posts to better represent positive human-nature interactions may therefore be context-dependent. As classification methods can often be time-consuming or expensive (e.g., image content classification through machine learning algorithms) (Fox et al., 2021a) the use of these methods needs to be more carefully considered when assessing social media data.
Finally, the importance of land cover diversity in aesthetic appreciation calculated by previous studies (Hermes et al., 2018; Albert et al., 2019) may have overestimated the importance of diversity due to the simplistic measure of “visible” or “not visible.” By filtering social media images to represent positive human-nature interactions only, our results have demonstrated that geopark visitors can still experience aesthetic services in low diversity landscapes. Individual landscape characteristics (e.g., sites of cultural heritage or water bodies) may therefore be just as important in determining aesthetic value as landscape diversity (van Berkel and Verburg, 2014).
Limitations and Future Direction
Previous studies have incorporated broad spatial data, such as geomorphological, geological and soil diversity, to assess geodiversity-ES relationships (Alahuhta et al., 2018). Though here land cover helped demonstrate border scale landscape interactions, the use of land cover as a proxy may not always be suitable for assessing ES as it often does not untangle finer scale human-nature relationships (Eigenbrod et al., 2010). To better understand the human-geodiversity relationships in producing ES, future studies should apply magnitude viewshed analysis to more explicit spatial measures of geodiversity such as landform diversity as demonstrated in Bailey et al. (2017). Furthermore, higher resolution datasets could be used to better understand smaller spatial scale interactions, including the use of national or regional landcover maps. Understanding the finer scale and more explicit relationship between geodiversity and ES can help to inform more holistic approaches to geoconservation (Alahuhta et al., 2018; Fox et al., 2020b).
The use of social media data may be subject to biases introduced by user groups (Langemeyer et al., 2018). Users of different social media sites have different motivations for uploading posts (Fox et al., 2021b), while education, gender, age and access to technology can also influence who is posting geotagged images (Clemente et al., 2019). Though different sites have different user groups and motivations for use, this study, as with the many studies that also only use one website, only reflect the opinions of one group of people (Wilkins et al., 2021). While previous studies have drawn parallels between social media posts and other datasets such as visitation rates and national statistics (Wood et al., 2013; Graham and Eigenbrod, 2019), future research should continue to assess the validity of social media data for CES research. Results of this work should therefore not be used to generalize to a population level but should be used to complement other approaches to assessing aesthetic services. Furthermore, these results may be influenced by the accessibility of viewpoints. For example, studies assessing the distributions of social media data in relation to CES studies found that points may be influenced by the distance to facilities and transport such as visitor centres and roads (Walden-Schreiner et al., 2018; Muñoz et al., 2020; Arslan and Örücü, 2021). There is concern that declining use of certain sites or changes to the access of social media data may impact future research. For example, there is an indication that Flickr use may be declining; Instagram started preventing access to data in 2018; and Panoramio was discontinued in 2016 (Wilkins et al., 2021).
Though the methods used here can only highlight areas of aesthetic beauty based on the availability of social media images, moving forward these results could be further implemented into predictive models that can be used to forecast areas of possible high scenic beauty that are not yet actualised by people. For example, as social media data is presence-only many studies have used maximum entropy modelling (MaxEnt) to plot the potential distributions of CES from Flickr photographs (Richards and Tunçer, 2018; Walden-Schreiner et al., 2018; Arslan and Örücü, 2021). These studies have used a range of predictor variables such as vegetation cover and distance from attractions. Moving forward, the outputs of visual magnitude analysis could be used to train models to identify areas of potential aesthetic services that incorporate geodiversity variables (Fox et al., 2022). In doing so park managers could create accessible routes to new areas that could alleviate pressures of over-usage from other areas of the park (Clemente et al., 2019).
Conclusion
The results of visual magnitude assessments can provide insight into both where people visit for scenic beauty and what they are viewing. Magnitude viewshed is advantageous over traditional viewshed methods and can help provide a more proportional measure of which landscape characteristics are contributing to an individual’s aesthetic appreciation. Here, we found that non-natural land cover classes such as mineral extraction and vineyards sites were highly represented in the viewshed of locations extracted from social media data, but likely because of the geodiverse context within which they reside. CES provided by geodiversity can therefore have high social, cultural and economic value, and the promotion of their sustainable use through geoconservation initiatives such as the UNESCO Global Geopark Programme is helping to ensure local communities benefit from geotourism and recreational CES consumption. Improving our understanding of the role of geodiversity in these services could help to further promote future geoconservation efforts. These insights can therefore be used to inform geoconservation priorities within geopark boundaries. Though this work has been applied to the specific case of a UNESCO Geopark, the methodologies used here are applicable to a range of contexts across different spatial scales.
Data Availability Statement
The datasets presented in this article are not readily available because of the Flickr API terms of services and all relevant ethical guidelines. Requests to access the datasets should be directed to Zm94bmF0QHVtaWNoLmVkdQ==.
Author Contributions
The ideas for the manuscript were conceived by all authors. NF led the data collection, analysis and manuscript preparation. BC designed the visual magnitude analysis for this study. ML and DV assisted in data analysis. All authors critically contributed to the writing of the final manuscript.
Funding
For this project was provided by the US National Science Foundation (NSF) through grant #2033320, “Designing high performing novel landscapes to tackle climate change using gamification & crowdsourcing” (NF, ML, and DV) and the Utah Agricultural Experiment Station UTA01440 (BC).
Conflict of Interest
The authors declare that the research was conducted in the absence of any commercial or financial relationships that could be construed as a potential conflict of interest.
Publisher’s Note
All claims expressed in this article are solely those of the authors and do not necessarily represent those of their affiliated organizations, or those of the publisher, the editors and the reviewers. Any product that may be evaluated in this article, or claim that may be made by its manufacturer, is not guaranteed or endorsed by the publisher.
Supplementary Material
The Supplementary Material for this article can be found online at: https://www.frontiersin.org/articles/10.3389/fenvs.2022.884115/full#supplementary-material
References
Alahuhta, J., Ala-Hulkko, T., Tukiainen, H., Purola, L., Akujärvi, A., Lampinen, R., et al. (2018). The Role of Geodiversity in Providing Ecosystem Services at Broad Scales. Ecol. Indic. 91, 47–56. doi:10.1016/j.ecolind.2018.03.068
Albert, C., Boll, T., Haus, P., Hermes, J., and von Haaren, C. (2019). “Measures for Landscape Aesthetics and Recreational Quality,” in Landscape Planning with Ecosystem Services: Theories and Methods for Application in Europe, Landscape Series. Editors C. von Haaren, A. A. Lovett, and C. Albert (Dordrecht: Springer Netherlands), 381–387. doi:10.1007/978-94-024-1681-7_24
Arslan, E. S., and Örücü, Ö. K. (2021). MaxEnt Modelling of the Potential Distribution Areas of Cultural Ecosystem Services Using Social Media Data and GIS. Environ. Dev. Sustain. 23, 2655–2667. doi:10.1007/s10668-020-00692-3
Bailey, J. J., Boyd, D. S., Hjort, J., Lavers, C. P., and Field, R. (2017). Modelling Native and Alien Vascular Plant Species Richness: At Which Scales Is Geodiversity Most Relevant? Glob. Ecol. Biogeogr. 26, 763–776. doi:10.1111/geb.12574
Benito-Calvo, A., Pérez-González, A., Magri, O., and Meza, P. (2009) Assessing Regional Geodiversity: the Iberian Peninsula. Earth Surf. Process. Landforms 34:1433–1445. doi:10.1002/esp.1840
Bétard, F. (2013). Patch-Scale Relationships between Geodiversity and Biodiversity in Hard Rock Quarries: Case Study from a Disused Quartzite Quarry in NW France. Geoheritage 5, 59–71. doi:10.1007/s12371-013-0078-4
Blanca Mena, M. J., Alarcón Postigo, R., Arnau Gras, J., Bono Cabré, R., and Bendayan, R. (2017). Non-normal Data: Is ANOVA Still a Valid Option? Psicothema.
Bukała, M., Zboińska, K., and Szadkowski, M. (2016). Troodos Ophiolite Mantle Section Exposed along Atalante Geo-Trail, Troodos Geopark, Cyprus. Geosci. Rec. 3, 1–6. doi:10.1515/georec-2016-0005
Burek, C. V., and Prosser, C. D. (2008). The History of Geoconservation: an Introduction. Geol. Soc. Lond. Spec. Publ. 300, 1–5. doi:10.1144/SP300.1
Chamberlain, B. C., and Meitner, M. J. (2013). A Route-Based Visibility Analysis for Landscape Management. Landsc. Urban Plan. 111, 13–24. doi:10.1016/j.landurbplan.2012.12.004
Chamberlain, B. C., Meitner, M. J., and Ballinger, R. (2015). Applications of Visual Magnitude in Forest Planning: A Case Study. For. Chron. 91, 417–425. doi:10.5558/tfc2015-071
Chamberlain, B., and Cook, F. (2019). An Empirical Assessment of the Arcpro Visual Magnitude Viewshed Plugin. Vis. Resour. Steward. Conf.
Cheesman, A. W., Preece, N. D., Oosterzee, P., Erskine, P. D., and Cernusak, L. A. (2018). The Role of Topography and Plant Functional Traits in Determining Tropical Reforestation Success. J. Appl. Ecol. 55, 1029–1039. doi:10.1111/1365-2664.12980
Cheung, L. T. O., Fok, L., and Fang, W. (2014). Understanding Geopark Visitors' Preferences and Willingness to Pay for Global Geopark Management and Conservation. J. Ecotourism 13, 35–51. doi:10.1080/14724049.2014.941848
Clemente, P., Calvache, M., Antunes, P., Santos, R., Cerdeira, J. O., and Martins, M. J. (2019). Combining Social Media Photographs and Species Distribution Models to Map Cultural Ecosystem Services: The Case of a Natural Park in Portugal. Ecol. Indic. 96, 59–68. doi:10.1016/j.ecolind.2018.08.043
Crofts, R. (2022). Progress and Future Challenges for Geoconservation in Protected and Conserved Areas. Parks Steward. Forum 38, 156110. doi:10.5070/P538156110
Díaz, S., Pascual, U., Stenseke, M., Martín-López, B., Watson, R. T., Molnár, Z., et al. (2018). Assessing Nature's Contributions to People. Science 359, 270–272. doi:10.1126/science.aap8826
Drápela, E. (2021). Prevention of Damage to Sandstone Rocks in Protected Areas of Nature in Northern Bohemia. AIMS Geosci. 7, 56–73. doi:10.3934/geosci.2021003
Eigenbrod, F., Armsworth, P. R., Anderson, B. J., Heinemeyer, A., Gillings, S., Roy, D. B., et al. (2010). The Impact of Proxy-Based Methods on Mapping the Distribution of Ecosystem Services. J. Appl. Ecol. 47, 377–385. doi:10.1111/j.1365-2664.2010.01777.x
European Union, Copernicus Land Monitoring Service (2021). “European Environment Agency (EEA)", f.Ex,” in 2018: “© European Union, Copernicus Land Monitoring Service 2018 (European Environment Agency (EEA).
Figueroa-Alfaro, R. W., and Tang, Z. (2017). Evaluating the Aesthetic Value of Cultural Ecosystem Services by Mapping Geo-Tagged Photographs from Social Media Data on Panoramio and Flickr. J. Environ. Plan. Manag. 60, 266–281. doi:10.1080/09640568.2016.1151772
Fischer, A., and Eastwood, A. (2016). Coproduction of Ecosystem Services as Human-Nature Interactions-An Analytical Framework. Land Use Policy 52, 41–50. doi:10.1016/j.landusepol.2015.12.004
Fox, N., August, T., Mancini, F., Parks, K. E., Eigenbrod, F., Bullock, J. M., et al. (2020a). "photosearcher" Package in R: An Accessible and Reproducible Method for Harvesting Large Datasets from Flickr. SoftwareX 12, 100624. doi:10.1016/j.softx.2020.100624
Fox, N., Graham, L. J., Eigenbrod, F., Bullock, J. M., and Parks, K. E. (2021a). Enriching Social Media Data Allows a More Robust Representation of Cultural Ecosystem Services. Ecosyst. Serv. 50, 101328. doi:10.1016/j.ecoser.2021.101328
Fox, N., Graham, L. J., Eigenbrod, F., Bullock, J. M., and Parks, K. E. (2022). Geodiversity Supports Cultural Ecosystem Services: an Assessment Using Social Media. Geoheritage 14, 27. doi:10.1007/s12371-022-00665-0
Fox, N., Graham, L. J., Eigenbrod, F., Bullock, J. M., and Parks, K. E. (2020b). Incorporating Geodiversity in Ecosystem Service Decisions. Ecosyst. People 16, 151–159. doi:10.1080/26395916.2020.1758214
Fox, N., Graham, L. J., Eigenbrod, F., Bullock, J. M., and Parks, K. E. (2021b). Reddit: A Novel Data Source for Cultural Ecosystem Service Studies. Ecosyst. Serv. 50, 101331. doi:10.1016/j.ecoser.2021.101331
Ghermandi, A., and Sinclair, M. (2019). Passive Crowdsourcing of Social Media in Environmental Research: A Systematic Map. Glob. Environ. Change 55, 36–47. doi:10.1016/j.gloenvcha.2019.02.003
Gosal, A. S., Geijzendorffer, I. R., Václavík, T., Poulin, B., and Ziv, G. (2019). Using Social Media, Machine Learning and Natural Language Processing to Map Multiple Recreational Beneficiaries. Ecosyst. Serv. 38, 100958. doi:10.1016/j.ecoser.2019.100958
Graham, L. J., and Eigenbrod, F. (2019). Scale Dependency in Drivers of Outdoor Recreation in England. People Nat. 1, 406–416. doi:10.1002/pan3.10042
Gray, M. (2008). Geodiversity: Developing the Paradigm. Proc. Geologists' Assoc. 119, 287–298. doi:10.1016/S0016-7878(08)80307-0
Gray, M. (2013). Geodiversity: Valuing and Conserving Abiotic Nature. Chichester: John Wiley & Sons.
Gray, M. (2012). Valuing Geodiversity in an 'Ecosystem Services' Context. Scott. Geogr. J. 128, 177–194. doi:10.1080/14702541.2012.725858
Haines-Young, R., and Potschin-Young, M. (2018). Revision of the Common International Classification for Ecosystem Services (CICES V5.1): A Policy Brief. Oe 3, e27108–157. doi:10.3897/oneeco.3.e27108
Hermes, J., Albert, C., and von Haaren, C. (2018). Assessing the Aesthetic Quality of Landscapes in Germany. Ecosyst. Serv. 31, 296–307. doi:10.1016/j.ecoser.2018.02.015
Hjort, J., Gordon, J. E., Gray, M., and Hunter, M. L. M. L. (2015). Why Geodiversity Matters in Valuing Nature's Stage. Conserv. Biol. 29, 630–639. doi:10.1111/cobi.12510
Hoffman, R., Chamberlain, B., and Smardon, R. (2021). Optimizing Viewpoint Selection for Route Based Experiences: Finding a Threshold between Sampling Rate and Model Accuracy. Proc. Vis. Resour. Steward. 2021, 12.
Jankowski, P., Najwer, A., Zwoliński, Z., and Niesterowicz, J. (2020). Geodiversity Assessment with Crowdsourced Data and Spatial Multicriteria Analysis. Ijgi 9, 716. doi:10.3390/ijgi9120716
Johnson, M., Campbell, L., Svendsen, E., and McMillen, H. (2019). Mapping Urban Park Cultural Ecosystem Services: A Comparison of Twitter and Semi-structured Interview Methods. Sustainability 11, 6137. doi:10.3390/su11216137
Johnson, T. F., Kent, H., Hill, B. M., Dunn, G., Dommett, L., Penwill, N., et al. (2021). Classecol: Classifiers to Understand Public Opinions of Nature. Methods Ecol. Evol. 12, 1329–1334. doi:10.1111/2041-210X.13596
Kubalíková, L., Bajer, A., and Balková, M. (2021). Brief Notes on Geodiversity and Geoheritage Perception by the Lay Public. Geosciences 11, 54. doi:10.3390/geosciences11020054
Lakens, D. (2013). Calculating and Reporting Effect Sizes to Facilitate Cumulative Science: a Practical Primer for T-Tests and ANOVAs. Front. Psychol. 4, 863. doi:10.3389/fpsyg.2013.00863
Langemeyer, J., Calcagni, F., and Baró, F. (2018). Mapping the Intangible: Using Geolocated Social Media Data to Examine Landscape Aesthetics. Land Use Policy 77, 542–552. doi:10.1016/j.landusepol.2018.05.049
Lindquist, M., Lange, E., and Kang, J. (2016). From 3D Landscape Visualization to Environmental Simulation: The Contribution of Sound to the Perception of Virtual Environments. Landsc. Urban Plan. 148, 216–231. doi:10.1016/j.landurbplan.2015.12.017
Mancini, F., Coghill, G. M., and Lusseau, D. (2018). Using Social Media to Quantify Spatial and Temporal Dynamics of Nature-Based Recreational Activities. PLOS ONE 13, e0200565. doi:10.1371/journal.pone.0200565
Megerle, H. E. (2021). Calcerous Tufa as Invaluable Geotopes Endangered by (Over-)Tourism: A Case Study in the UNESCO Global Geopark Swabian Alb, Germany. Geosciences 11, 198. doi:10.3390/geosciences11050198
Mohammad, S. M., and Turney, P. D. (2013). Nrc Emotion Lexicon. Canada: National Research Council, 2.
Muñoz, L., Hausner, V. H., Runge, C., Brown, G., and Daigle, R. (2020). Using Crowdsourced Spatial Data from Flickr vs. PPGIS for Understanding Nature's Contribution to People in Southern Norway. People Nat. 2, 437–449. doi:10.1002/pan3.10083
Nagendra, H. (2002). Opposite Trends in Response for the Shannon and Simpson Indices of Landscape Diversity. Appl. Geogr. 22, 175–186. doi:10.1016/S0143-6228(02)00002-4
Németh, B., Németh, K., Procter, J. N., and Farrelly, T. (2021a). Geoheritage Conservation: Systematic Mapping Study for Conceptual Synthesis. Geoheritage 13 (2), 1–21. doi:10.1007/s12371-021-00561-z
Németh, B., Németh, K., and Procter, J. N. (2021b). Visitation Rate Analysis of Geoheritage Features from Earth Science Education Perspective Using Automated Landform Classification and Crowdsourcing: A Geoeducation Capacity Map of the Auckland Volcanic Field, New Zealand. Geosciences 11 (11), 480. doi:10.3390/geosciences11110480
Newsome, D., and Dowling, R. (2018). “Geoheritage and Geotourism,” in Geoheritage. Editors E. Reynard, and J. Brilha (Elsevier), 305–321. doi:10.1016/B978-0-12-809531-7.00017-4
Nielsen, F. Å. (2011). “A New ANEW: Evaluation of a Word List for Sentiment Analysis in Microblogs,” in Proceedings of the ESWC2011 Workshop on ‘Making Sense of Microposts’: Big Things Come in Small Packages. CEUR Workshop Proceedings No. 718, 93–98.
Olteanu, A., Castillo, C., Diaz, F., and Kıcıman, E. (2019). Social Data: Biases, Methodological Pitfalls, and Ethical Boundaries. Front. Big Data 2, 13. doi:10.3389/fdata.2019.00013
Parks, K. E., and Mulligan, M. (2010). On the Relationship between a Resource Based Measure of Geodiversity and Broad Scale Biodiversity Patterns. Biodivers. Conserv. 19, 2751–2766. doi:10.1007/s10531-010-9876-z
Pepper, M., Doughty, P., and Keogh, J. S. (2013). Geodiversity and Endemism in the Iconic Australian Pilbara Region: a Review of Landscape Evolution and Biotic Response in an Ancient Refugium. J. Biogeogr. 40, 1225–1239. doi:10.1111/jbi.12080
Pijet-Migoń, E., and Migoń, P. (2021). Linking Wine Culture and Geoheritage-Missing Opportunities at European UNESCO World Heritage Sites and in UNESCO Global Geoparks? A Survey of Web-Based Resources. Geoheritage 13, 71. doi:10.1007/s12371-021-00594-4
Prosser, C. D. (2013). Our Rich and Varied Geoconservation Portfolio: the Foundation for the Future. Proc. Geologists' Assoc. 124, 568–580. doi:10.1016/j.pgeola.2012.06.001
R Core Team (2021). R: A Language and Environment for Statistical Computing. Vienna, Austria: R Foundation for Statistical Computing. URL https://www.R-project.org/.
Ren, Y., Lü, Y., Hu, J., and Yin, L. (2021). Geodiversity Underpins Biodiversity but the Relations Can Be Complex: Implications from Two Biodiversity Proxies. Glob. Ecol. Conservation 31, e01830. doi:10.1016/j.gecco.2021.e01830
Richards, D. R., and Tunçer, B. (2018). Using Image Recognition to Automate Assessment of Cultural Ecosystem Services from Social Media Photographs. Ecosyst. Serv. 31, 318–325. doi:10.1016/j.ecoser.2017.09.004
Ruban, D. A. (2010). Quantification of Geodiversity and its Loss. Proc. Geologists' Assoc. 121, 326–333. doi:10.1016/j.pgeola.2010.07.002
Sagala, S., Rosyidie, A., Sasongko, M. A., and Syahbid, M. M. (2018). Who Gets the Benefits of Geopark Establishment? A Study of Batur Geopark Area, Bali Province, Indonesia. IOP Conf. Ser. Earth Environ. Sci. 158, 012034. doi:10.1088/1755-1315/158/1/012034
Schirpke, U., Timmermann, F., Tappeiner, U., and Tasser, E. (2016). Cultural Ecosystem Services of Mountain Regions: Modelling the Aesthetic Value. Ecol. Indic. 69, 78–90. doi:10.1016/j.ecolind.2016.04.001
Schlesinger, W., Cervera-Taulet, A., and Pérez-Cabañero, C. (2020). Exploring the Links between Destination Attributes, Quality of Service Experience and Loyalty in Emerging Mediterranean Destinations. Tour. Manag. Perspect. 35, 100699. doi:10.1016/j.tmp.2020.100699
Schmider, E., Ziegler, M., Danay, E., Beyer, L., and Bühner, M. (2010). Is it Really robust? Reinvestigating the Robustness of ANOVA Against Violations of the Normal Distribution Assumption Methodol.: Eur. J. Res. Methods Behav. Soc. Sci. 6 (4), 147.
Slaymaker, O., Catto, N., and Kovanen, D. J. (2020). “Protecting Geodiversity in Eastern Canada,” in Landscapes and Landforms of Eastern Canada (Cham: Springer), 557–582. doi:10.1007/978-3-030-35137-3_25
Taylor, R. (1990). Interpretation of the Correlation Coefficient: A Basic Review. J. Diagnostic Med. Sonogr. 6, 35–39. doi:10.1177/875647939000600106
Thiagarajah, J., Wong, S. K. M., Richards, D. R., and Friess, D. A. (2015). Historical and Contemporary Cultural Ecosystem Service Values in the Rapidly Urbanizing City State of Singapore. Ambio 44, 666–677. doi:10.1007/s13280-015-0647-7
UNEP-WCMC, IUCN (2021). Protected Planet: [Troodos Unesco Global Geopark; the World Database on Protected Areas (WDPA)/The World Database on Other Effective Area-Based Conservation Measures (WD-OECM)/The Global Database on Protected Areas Management Effectiveness. Cambridge, UK: UNEP-WCMC and IUCN. (GD-PAME)] [On-line], [2021]Available at: www.protectedplanet.net.
Van Berkel, D. B., Tabrizian, P., Dorning, M. A., Smart, L., Newcomb, D., Mehaffey, M., et al. (2018). Quantifying the Visual-Sensory Landscape Qualities that Contribute to Cultural Ecosystem Services Using Social Media and LiDAR. Ecosyst. Serv. 31, 326–335. doi:10.1016/j.ecoser.2018.03.022
van Berkel, D. B., and Verburg, P. H. (2014). Spatial Quantification and Valuation of Cultural Ecosystem Services in an Agricultural Landscape. Ecol. Indic. 37, 163–174. doi:10.1016/j.ecolind.2012.06.025
Van Ree, C. C. D. F., and Van Beukering, P. J. H. (2016). Geosystem Services: A Concept in Support of Sustainable Development of the Subsurface. Ecosyst. Serv. 20, 30–36. doi:10.1016/j.ecoser.2016.06.004
van Zanten, B. T., Verburg, P. H., Koetse, M. J., and van Beukering, P. J. H. (2014). Preferences for European Agrarian Landscapes: A Meta-Analysis of Case Studies. Landsc. Urban Plan. 132, 89–101. doi:10.1016/j.landurbplan.2014.08.012
Walden-Schreiner, C., Rossi, S. D., Barros, A., Pickering, C., and Leung, Y.-F. (2018). Using Crowd-Sourced Photos to Assess Seasonal Patterns of Visitor Use in Mountain-Protected Areas. Ambio 47, 781–793. doi:10.1007/s13280-018-1020-4
Wilkins, E. J., Wood, S. A., and Smith, J. W. (2021). Uses and Limitations of Social Media to Inform Visitor Use Management in Parks and Protected Areas: A Systematic Review. Environ. Manage. 67, 120–132. doi:10.1007/s00267-020-01373-7
Wood, S. A., Guerry, A. D., Silver, J. M., and Lacayo, M. (2013). Using Social Media to Quantify Nature-Based Tourism and Recreation. Sci. Rep. 3, 2976. doi:10.1038/srep02976
Zhao, W., and Han, W. (2021). Examination of Urban Landscape Aesthetics through Mapping Using Geotagged Social Media Data from Flickr. Cus 09, 66–82. doi:10.4236/cus.2021.91005
Keywords: geodiversity, cultural ecosystem services, geoparks, aesthetics, viewshed analysis, visual magnitude
Citation: Fox N, Chamberlain B, Lindquist M and Van Berkel D (2022) Understanding Landscape Aesthetics Using a Novel Viewshed Assessment of Social Media Locations Within the Troodos UNESCO Global Geopark, Cyprus. Front. Environ. Sci. 10:884115. doi: 10.3389/fenvs.2022.884115
Received: 25 February 2022; Accepted: 23 June 2022;
Published: 25 July 2022.
Edited by:
Janne Alahuhta, University of Oulu, FinlandReviewed by:
Thomas Campagnaro, University of Padua, ItalyKaroly Nemeth, Massey University, New Zealand
Copyright © 2022 Fox, Chamberlain, Lindquist and Van Berkel. This is an open-access article distributed under the terms of the Creative Commons Attribution License (CC BY). The use, distribution or reproduction in other forums is permitted, provided the original author(s) and the copyright owner(s) are credited and that the original publication in this journal is cited, in accordance with accepted academic practice. No use, distribution or reproduction is permitted which does not comply with these terms.
*Correspondence: Nathan Fox, Zm94bmF0QHVtaWNoLmVkdQ==