- 1School of Public Administration, Xi’an University of Architecture and Technology, Xi’an, China
- 2School of Public Administration, Central China Normal University, Wuhan, China
With China’s rapid urbanization and rising living standards, the household sector has become the second largest contributor to urban carbon emissions and important pathway to achieve China’s carbon reduction targets. Based on data of Chinese Residential Energy Consumption Survey in 2014, this paper establishes standard households in 54 cities to identify the carbon emission characteristics and explores the emission reduction paths of urban households. We present evidence that rich households tend to increase their use of clean energy, low-income households will not completely abandon inferior energy because of their usage habits and costs and they always live in suburban areas and urban villages. Cities with high household carbon emissions are almost located in north of Huai River/Qinling Mountains line, while cities with low carbon emissions are mostly located around the line and which are mainly on the south side. Monocentric urban development patterns and frigid climates are easy to increase higher household carbon emissions. In this regard, policy makers should help low-income households to escape from inferior energy dependence, encourage the use of new energy vehicles and green housing technologies, introduce a two-part pricing system for central heating services and maintain relative balance of residential and public resources in urban planning.
1 Introduction
As urbanization advances and population climbs, cities have become centers of economic, cultural and political power. However, the uncontrolled expansion of urbanization has also led to high energy consumption and exponential growth of greenhouse gas emissions, which poses huge environmental and climate risks to society (Ding and Li, 2017; Sun H. et al., 2020). In recent years, the household sector has become a new focus for carbon emissions with the improvement of industrialization and energy efficiency in China (Cui et al., 2016). In developed countries such as Europe and the United States, carbon emissions generated by the household sector account for 70%–80% of total domestic carbon emissions (Bin and Dowlatabadi, 2005; Baiocchi et al., 2010), but households only contribute 40% of total carbon emissions in China, making them the second largest source of emissions after the industrial sector (Liu et al., 2011; Dong and Zhao, 2017). As the structure of residential energy consumption is gradually being transformed, driven by the process of urbanization, the energy consumption and carbon emissions generated by households will further increase (Du, 2016). At the same time, the household sector has 25% of all energy saving opportunities (The McKinsey Global Institute, 2007), so households are not only the main contributors to urban carbon emissions, but also an important path to achieve energy conservation and emission reduction in China. In the context of carbon peak and carbon neutrality goals, it is of great significance practical to develop low-carbon economy and construct green cities, through reducing household carbon emissions with clarifying urban households’ energy consumption habits and changing their energy consumption behavior and motivation.
In general, ecological degradation affects urbanization through the exclusion of the resident population (Lu et al., 2018; Wang et al., 2021). In order to exclude systematic differences in population distribution between cities, Glaeser and Kahn (2010) use the “standardized household” concept to explore the “pure” effect of urban characteristics on the carbon emissions of residents. This is a household with a fixed household size, income level and age of household members. On this basis, Zheng S. et al. (2011) rank the carbon footprints of households in 74 major Chinese cities based on China Urban Household Survey in 2006. They assess the impact of regional development plans on CO2 emissions in China, suggesting that regional economic development policies that promote urban development in the Northeast may bring about an increase in household carbon emissions. Using data from the China Household Income Survey in 2002, Li et al. (2013) estimate residential carbon emissions and inter-city migration costs in 56 major cities in China and analyze the reasons for differences in inter-city carbon emission. They find that low-density urban development patterns and lower winter temperatures both can increase residential carbon emissions. However, the above studies cannot fully reflect the specific status of household carbon emission in detail due to the limitation of early micro-survey data in China.
This paper uses data from the Chinese Residential Energy Consumption Survey (CRECS) of 2014 to empirically analyze the characteristics and emission reduction paths of Chinese urban household carbon emissions, reflecting a more realistic picture of urban household carbon emissions in China and providing a theoretical reference for urban carbon emission reduction. We also make the following contributions: 1) we use micro-data to comprehensively measure the complete carbon emissions of Chinese households for the first time, including transportation, honeycomb briquet, straw and firewood, which have been always neglected in previous studies. 2) Compared with previous studies, our results better reflect the characteristics of China’s urban household carbon emissions in the new era, including cost calculations of household migration from low-carbon cities to high-carbon cities. 3) In contrast to using the urban density, we use the polycentric spatial structure to better capture the impact of urban characteristics on household carbon emissions.
The remainder of this paper is organized as follows: we review relevant literatures in Section 2; Section 3 presents the econometric model and associated data; Section 4 gives a detailed analysis of the empirical results. Section 5 introduces the ranking of urban household carbon emissions and the explanation of the difference, and these are discussed in Section 6; Section 7 presents conclusions and policy recommendations.
2 Literature Review
With increasingly serious environmental problems, the energy consumption from the household sector and its carbon emissions have attracted widespread attention from the academic community (Han and Wei, 2021). Several scholars have explored and discussed the influencing factors and regional differences in household carbon emissions, based on research methods such as carbon emission coefficient, input-output, and life-cycle evaluation methods. In the analysis of impact factors influencing household carbon emissions, household characteristics and urban form are a hot topic of research. In terms of household characteristics, Wu and Zheng (2022) find that an increase in household income would contribute to the energy transition of households, but rural residents would not completely abandon “inferior” energy sources due to energy prices and supply. Tong and Zhou (2020) believe that the characteristics of family aging help reduce household carbon emissions, and family structures in which elderly and young members live together are more “energy-saving and environmentally friendly.” Yu et al. (2018) show that the smaller household size and family aging increases household energy consumption and carbon emissions. Hu et al. (2018) argue that household size has a significant inhibitory effect on average household carbon emissions. It is undesirable to control carbon emissions by reducing household size, and it is necessary to further strengthen of low-carbon environmental knowledge dissemination as well as to implement energy-efficient appliances. The economic level and urban scale of cities also have a significant impact on household carbon emissions. Shen and Shi (2018) find a “U” shaped relationship between urban population and per capita domestic energy consumption in the early stage of urbanization. The per capita domestic energy consumption tends to decline due to the scale effect generated by urban population gathering. With the spread of urban expansion and the change of residents’ lifestyles, the impact on domestic energy consumption turns positive in the middle and late stages of urbanization. Hu et al. (2018) argue that city size is an important contributing factor to urban household carbon emissions, while the level of urban economy significantly drives the upgrading of household energy structure.
Urban spatial form is also a key influencing factor of household carbon emissions. Liu et al. (2016) show that urban sprawl has a significant positive effect on household carbon emissions in southern Chinese cities and call for a compact and rational urban spatial structure in the urbanization process. Sun B. et al. (2020) find that compared with the “monocentric” spatial structure, the “polycentric” urban spatial structure is more conducive to shortening commuting time, thereby reducing commuting carbon emissions. However, households have larger housing space in “polycentric” cities, thus also increasing household energy consumption and carbon emissions. Li et al. (2020) emphasize the impact of physical environmental factors around households on household energy consumption. They find that household energy consumption and carbon emissions are mutually substituted between the two spatial domains of residence and transportation. This substitution phenomenon between different energy sources in different spatial domains is attributed to the different characteristics of influencing factors. Li et al. (2017) find that the farther the distance between housing and public services, the higher the tendency of residents to choose high-carbon travel modes. Martins et al. (2019) show that an increase in building height dispersion in a building complex can significantly reduce the heating energy demand of a building, reducing energy consumption by nearly 40 KWh per cubic meter per year.
Climatic conditions can also have a significant impact on residential carbon emissions. China established centralized urban heating system at the beginning of the country based on the Soviet model. Due to the relatively backward economic level and limited coal production capacity at that time, the Huai River/Qinling Mountains line was created to artificially divide the northern compulsory centralized heating area due to the cost of energy saving and the colder north. Central heating is provided in the north but not in the south (Almond et al., 2009). Zheng et al. (2017) argue that there are significant north-south differences in the energy consumption characteristics of Chinese households. Per capita energy consumptions and carbon emissions are significantly higher in the north than in the south due to differences in winter heating practices. Zheng S Q. et al. (2011) show that the per capita residential carbon emissions in cities on both sides of the Huai River/Qinling Mountains line have significantly discontinuity due to China’s heating policy. The per capita residential carbon emissions of northern cities are 1.7 times higher than southern cities. Comparing the differences in residential energy consumption and energy use behavior under different heating modes, Jing et al. (2020) find that centralized heating produces higher energy consumption and emissions compared to self-heating but is also relatively more energy efficient. If centralized heating is adjusted by charging heating consumption, the heating expenditure of residents can be reduced by more than 35%.
In summary, research on household carbon emissions has developed rapidly in recent years, and the number of quantitative studies related to the evaluation of urban household carbon emission patterns and policies has increased. While city-level studies tend to analyze the overall carbon emission characteristics of urban households, household-level studies focus more on the energy consumption in communities and households itself. The former mainly provides a quantitative basis for the government to formulate energy conservation and environmental protection policies for macro regulation, while the latter identifies factors driving the differences in lifestyles from the perspective of consumers themselves and then proposes specific operation suggestions. Domestic research in China has gradually evolved from the earlier analysis of macro statistical data to the use of comprehensive social survey data. Because of the lack of detailed data on household energy consumption at the national level, most studies focused on the a few conventional energy sources or certain type of factors affecting energy consumption. Therefore, we used data from the 2014 Chinese Residential Energy Consumption Survey to capture the use of non-conventional energy sources, such as coal, firewood and straw, while also estimating the energy consumption of sample households due to central heating and private car travel based on Zheng et al. (2017), with the aim that the results would be more consistent with the actual situation of energy consumption and carbon emissions in urban households. Specifically, this paper firstly establishes behavioral equations for different energy types in each cities as a way to study the relationship between household energy consumption and specific household characteristics. Secondly, it estimates and ranks total household carbon emissions in each city. Finally, it analyzes the differences in standardized household carbon emissions between cities and discusses the impact of polycentric urban spatial structure on residents’ transportation trips.
3 Data and Estimation Strategy
3.1 Data Sources and Variables Selection
This study uses data from the Chinese Residential Energy Consumption Survey (CRECS) of 2014, which was initiated by the School of Applied Economics, Renmin University of China, with the aim of monitoring the overall pattern of household energy consumption in China under a dynamic socio-economic background. Similar to the US Household Energy Consumption Survey (RECS) conducted by the US Energy Information Administration (EIA), the CRECS is divided into six modules: household demographic characteristics, residential characteristics, kitchen and household appliances, residential heating and cooling, private and public transportation and household energy consumption. In addition, the CRECS also collects detailed information on appliances (power, year of purchase, energy efficiency label) and consumption behavior of these residential devices (frequency and duration of device use, maintenance, etc.), allowing us to estimation of the energy consumption of devices. Compared with other comprehensive Chinese micro household surveys, the CRECS not only records the use of conventional energy such as electricity, natural gas and coal gas, but it also records the consumption of coal, gasoline, firewood and straw, thus reflecting the energy consumption of Chinese households in a more comprehensive, systematic and accurate way (Zheng et al., 2014). The CRECS 2014 covered a total of 3,863 urban and rural households in 28 provinces and 85 cities. Only urban residents were selected in this study sample. In order to ensure that each city has enough sample observations, 1,610 households in 54 cities (including municipalities directly under the central government, provincial capitals, prefecture-level cities and some county-level cities) were finally selected.
City-level data are mainly from the China City Statistical Yearbook. Climate data are drawn from the China Meteorological Network, and the nighttime light brightness data are drawn from the global DMSP/OLS nighttime light remote sensing data provided by the website of the National Oceanic and Atmospheric Administration (NOAA) of the United States. Carbon emission coefficients of household electricity consumption in different regions were obtained from the 2014 China Regional Grid Baseline Emission Coefficients Bulletin announced by the National Development and Reform Commission (NDRC). It is only necessary to match the city where the household is located with the coverage area of each power grid to obtain the carbon emission coefficient generated per unit of electricity, and then estimate their electricity carbon emissions from domestic electricity consumption with their electricity consumption. And the carbon coefficients for the household fuels such as coal, liquefied petroleum gas (LPG), natural gas and firewood are obtained from the Provincial Greenhouse Gas Inventory Weaving Guide. Tables 1, 2 present definitions and the statistics descriptions of the key variables, A “standardized household” is a household with an annual income of 96,146 yuan, three household members and a household head with an average age of 50.8. The values of these three indicators are obtained by averaging the entire samples. Detailed use of various types of energy by sample households in various cities is shown in (Supplementary Table S1).
3.2 Framework for Estimating Household Carbon Emissions
In order to exclude systematic differences in population distribution among cities and thus examine more precisely the impact of urban factors on household carbon emissions, we construct standardized households in 54 cities by referring to Glaeser and Kahn (2010), which can answer the question of whether total carbon emissions will rise or fall if a household moves from a low-carbon emission city to a high-carbon emissions city. In this paper, we focus on four main sources of household carbon emissions: residential electricity, residential heating, domestic fuels and travel. Based on this, we construct a framework to estimate the CO2 emissions of a standardized Chinese urban household, as shown in Eq. 1.
4 Household Energy Consumption Model Selection and Empirical Results
According to Eq. 1, we analyze household energy consumption in each city by regressing the energy consumption of each 54 sample cities, which can estimate the carbon emissions of energy consumption of one standardized household in the sample cities. We first estimate the standardized household electricity consumption, and the model of household electricity consumption is shown to the following form:
where i and j denote a household i living in city j. ELE is the standardized household electricity consumption, Inc indicates the total household income, Age means the age of the household head, Hsize is the household size,
Considering the area of each household, residential construction year, use of insulation materials and other factors, we estimate the central heating energy consumption with methods proposed by Zheng et al. (2017). As for household electricity consumption, we regress the central heating energy consumption for a standardized household based on household income and demographic data.
Many households in the sample cities have zero consumption of a particular energy type due to differences in household energy use habits. For example, the ownership rate of private cars in Shanghai is 9.7%, which means that 90.3% of all households have zero transportation energy consumption. The same problem exists for other types of energy consumption, such as coal and LPG, etc. In order to correct for the bias in the regression estimation due to sample selection, we use the Heckman two-step estimation for the other types of energy consumption (Heckman, 1979).
In the first step, we calculate the probabilities of using various energy types with a probit model:
In the second step, we estimate:
Because of the smaller sample sizes of the different types of energy consumption, the effects of age of household head and household size on energy consumption cannot be accurately estimated, and we exclude these variables from the second step of the Heckman method. We use pooled regressions for the households in 54 cities and add city fixed effects for the electricity and heating equations in Table 3 to control for differences in marginal effects on energy consumption across cities.
Table 3 shows that, the income elasticity of electricity consumption is 0.119, which indicates that household energy demand also rises with improving living standard. However, the popularity of energy-efficient appliances and environmental protection policies promote the efficient use of energy, so the electricity consumption does not show a larger increase with the rise of the residents’ income. In addition, household size also has a significant positive effect on electricity consumption. The effect of the age of the household head on household electricity consumption is negative and insignificant. We believe that the difficulties in the use of new technologies may constrain the use of new energy-using products by the elderly. In addition, older people are more conscious of saving and more sensitive to energy prices than younger people (Hu et al., 2020; Wu and Zheng, 2022). The coefficient of family size for central heating energy consumption is 0.048, which is due to the fact that larger households tend to choose larger houses, resulting in more heating energy consumption. The income elasticity of private car use is 0.669, indicating that an increase in resident’ income significantly enhances the willingness to purchase and use private cars. Unlike the results of previous studies, the income elasticity of private car energy consumption is −0.041, but it is not statistically significant. It indicates that there is still a more complex relationship between residents’ income and carbon emissions from transportation energy consumption, which will be one of the focuses of future research.
LPG is generally used more intensively in urban villages and peri-urban areas, where a large number of rural migrant workers live. As their income increases, the workers may still use LPG in order to save money and the consumption has been reduced. Both the willingness to use natural gas and its consumption are positively correlated with family income, this is due to the fact that it is cleaner than LPG and piped directly to homes, which making it more convenient to use. Similar to the use of LPG, households in urban villages and peri-urban areas tend to use more inferior energy sources such as honeycomb, briquettes, firewood and straw, which can produce a lot of pollution and carbon emissions. As their incomes rise, they are significantly less willing to use such type of energy, and more willing to use clean energy. This suggests that income growth will contribute the energy transition of households, but the replacement of inferior energy by clear energy will not happen overnight. The rise in the energy ladder will not see a complete substitution of clear energy for inferior energy, and residents will not completely abandon the use of inferior energy due to energy use habits and costs. As in Wu and Zheng (2022), we find that the energy ladder process is also coeval with the energy stack process in urban areas.
5 The Difference Analysis of Urban Household Carbon Emissions
5.1 Estimation of Urban Household Carbon Emissions
In order to further assess whether the migration of a household from the city of residence to the target city leads to an increase in carbon emissions from household energy consumption, we use Eqs 2, 3 to approximate the regression analysis for a standardized household and we also use the microdata to estimate the regression model for household’ electricity and central heating consumption across all 54 cities, each with the same equation.
For example, the electricity regression estimation based on the data from the sample of 191 urban households in Shanghai is as follows:
Next, using Eq. 6, we estimate the annual electricity consumption of a standardized household in Shanghai. The annual electricity consumption of this standardized household is 2,463.53 kWh. This electricity consumption is then multiplied by the grid emission coefficient (0.8095 tCO2/mWh), which is
For transportation and other fuel, we use the Heckman two-step model to predict the energy consumption of a standardized household. Using Shanghai as an example, the equations are shown below.
Based on the first regression step, we predict the probability of car ownership in a standardized households to be 9.7%. Using the second regression step, we estimate the energy consumption of private car use in a standardized households to be 307.94 kg of coal equivalent (kgce)/year. The energy consumption is then converted into carbon emissions based on the CO2 emission coefficient of standard coal. The results show that a standardized household in Shanghai produces about 0.76 tons of CO2 emissions per year from the use of private cars (Table 4). The same method are be used to estimate the other fuel carbon emissions (such as honeycomb briquette, coal, LPG, natural gas, gas, straw and firewood). The total household carbon emissions are presented in Table 4. As there are a few observation samples of private cars in CRECS urban household data, we follow the practice of Zheng S. et al. (2011) to use the statistic yearbook data to replace some cities with insufficient observation samples (the same situation also exists in liquefied gas, natural gas and gas).
5.2 China’s Cities Ranking Based on the Household Carbon Emissions
Based on Eq. 1, we rank the total carbon emissions of a standardized household in 54 sample cities. The results are presented in Table 4. The 10 cities with lowest carbon emissions are Shangrao, Fuyang, Zhangzhou, Zunyi, Xiangyang, Leshan, Huaihua, Nanjing, Luzhou, and Meishan, and the 10 cities with the highest carbon emissions are Qiqihar, Handan, Harbin, Changzhi, Xining, Shenyang, Beijing, Wuhan, Qitaihe and Taian. In terms of location, cities with high carbon emissions are mostly concentrated in the northeastern of China (such as Harbin and Qitaihe). The temperatures in these areas are extremely low in winter and thus there are higher demands for heating services. On the other hand, cities with lower carbon emission are mostly on both sides of the Huai River/Qinling Mountains line, especially on the south side (such as Fuyang and Xiangyang). Compared with other southern cities, the temperature differences between the northern and the southern side of such cities are smaller and thus there is no high demand central heating in winter or electricity for cooling in summer. In terms of city size, large cities with developed economies have more carbon emissions (such as Wuhan and Guangzhou) compared to small and medium-sized cities, which have higher demand with electricity consumption and private car. In terms of resource endowments, resource-based cities such as Qiqihar, Changzhi, and Handan have rich mineral resources, low coal prices and higher emission. Energy structure reform can be implemented in resource-based cities to develop renewable energy sources such as wind power, photovoltaics and hydropower according to local conditions, promoting the exploitation and utilization of clean fuels such as coalbed methane (CBM), natural gas and unconventional gas (shale gas), and which can further promote long-term policy support for the systematic construction of total consumption and coal control in such cities (Feng et al., 2017; Sun et al., 2021).
Under the condition of zero carbon tax, Glaeser and Kahn (2010) regard the social cost of standardized household carbon emissions in different regions as an optimal location tax. For example, the difference in emissions costs of 2,154 yuan between Qiqihar and Shangrao means families should pay 2,154 yuan in migrating taxes each year moving from Shangrao to Qiqihar. If the migrating tax is high, the residents will reduce their willingness to move, and conversely, it will increase the residents’ willingness to move. But the study also shows that a direct carbon tax would improve social welfare more than any local tax. Therefore, the significance of calculating the migrating cost is more to show the environmental externalities of the residents from the low emission cities to the high emission cities. The external cost of carbon emissions from households located in place A rather than in place B is equal to the increase in carbon emissions in place A minus the decrease in carbon emissions in place B, multiplied by the social cost minus the current level of carbon tax. The marginal social cost per ton of carbon emissions is 35 dollars (Metcalf, 2009), the environmental cost of a standardized household moving from the most “green” city to the most “brown” city would be 2,154.14 yuan (converted from 2014 average exchange rates), which represents about 2.24% of a standardized household’s annual income. We believe this gap would be greatly alleviated if the government imposes an appropriate carbon tax on areas and households with high carbon emissions. However, the factors to be considered are very complicated in the process of formulating carbon tax policy. It may be a focus of future research with how to accurately transfer the negative externalities of carbon emissions to consumers while ensuring the stability of energy prices.
5.3 Analysis of Inter-City Differences in Household Carbon Emissions
Table 5 presents regression between standardized household carbon emissions and urban characteristics (urban income level, population size, average temperatures and urban spatial structure). Using the method of Liu et al. (2017), we calculate polycentric index reflecting the degree of monocentric/polycentric of the city to analyze the spatial distribution of the population in the city area:
Based on corrected nighttime light data, we extract the total value of nighttime light brightness DN for all counties, county-level cities and municipal districts within each sample city. The proportion of the total light brightness value of municipal districts to the entire city total light brightness value reflect the spatial structure of the city. The value of the index is between 0 and 1: the closer to 1, the more monocentric the spatial organization of the city.
We find that household electricity consumption is positively correlated to the urban population size and income level, which means the larger and the more developed the city, the higher household electricity emissions. There is negative relationship between summer temperature and household electricity emissions, but it is not significant. There is also no significant relationship between centralized heating emissions and urban income and population size, which may be due to China’s centralized heating policy, based on the climatic conditions of different regions while not relying on the economic level and development of the cities. Winter temperatures significantly affect household heating carbon emissions and even overall household carbon emissions. The regression results indicate that the demand for heating services is stronger in regions with colder climates, if the temperature rises by 1°C in January, the carbon dioxide emissions generated by central heating for a standardized household will decrease by 0.077 tons. Figure 1 gives the relationship between the total carbon emissions of a standardized household and the average winter temperature in 54 cities, and we can see that the cities with higher carbon emissions include Qiqihar, Harbin and Qitaihe. These cities are located in the northeast of China and the average winter temperature can reach below −15°C, the cold temperature and longer heating period also make residential heating becomes the main source of household carbon emissions. Cities like Fuzhou and Shenzhen, which have high winter temperatures, do not have excessive heating demand. Even though the standardized household in these cities has higher electricity emissions, the total carbon emissions are relatively low. China has set up pilot areas for clean energy heating such as nuclear and solar power (Kong and Liang, 2021; Zhao, 2021). If centralized heating system can be upgraded and such new heating technologies can be further promoted, then carbon emissions in northeast China and even the whole northern region can be significantly reduced, thus effectively relieving the pressure of carbon emission reduction in China.
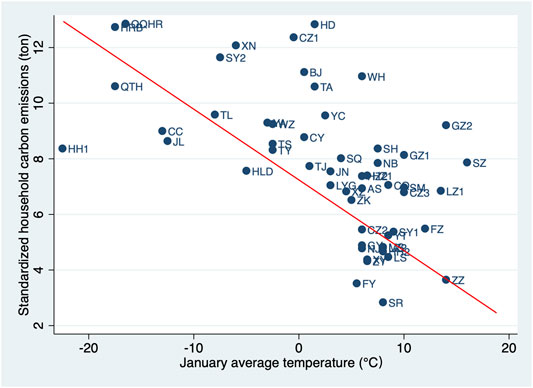
FIGURE 1. The cross-city relationship between winter temperature and household carbon emissions. Note: The corresponding abbreviations and full names of each city are as follows: AS, Anshun; BJ, Beijing; CC, Changchun; CZ1, Changzhi; CZ2, ChangZhou; CY, Chaoyang; CZ3, Chenzhou; CQ, Chongqing; FY, Fuyang; FZ, Fuzhou; GY, Guiyang; GZ1, Ganzhou; GZ2, Guangzhou; HD, Handan; HZ1, Hangzhou; HRB, Harbin; HH1, Heihe; HH2, Huaihua; HLD, Huludao; HZ2, Huzhou; JL, Jilin; JN, Jining; LS, Leshan; LYG, Lianyungang; LZ1, Liuzhou; LZ2, Luzhou; MS, Meishan; NJ, Nanjing; NB, Ningbo; QQHR, Qiqihar; QTH, Qitaihe; SM, Sanming; SH, Shanghai; SQ, Shangqiu; SY1, Shaoyang; SY2, Shenyang; SY3, Shangrao; SZ, ShenZhen; TA, Taian; TY, Taiyuan; TS, Tangshan; TJ, TianJin; TL, Tieling; WH, Wuhan; WZ, Wuzhong; XY, Xiangyang; XN, Xining; XZ, Xuzhou; YA, Yan’an; YT, Yingtan; YC, Yuncheng; ZZ, Zhangzhou; ZK, Zhoukou; ZY, Zunyi.
After controlling for urban income and population size, the regression results show that there is a significant negative relationship between the polycentricity urban spatial structure with transportation carbon emissions. It may be due to the fact that a higher degree of polycentricity is often associated with a rational urban spatial layout, where businesses and residents can periodically adjust their residential locations to achieve a local balance of residence and employment, thus achieving shorter commuting distances and lower transportation emissions (Gordon and Wong, 1985; Giuliano and Small, 1993; Yan and Sun, 2015). Some scholars also believe that public transportation is better built in polycentric areas, and residents will choose public transportation more often, thus reducing emissions from private car (Veneri and Burgalassi, 2012). While promoting urban polycentric development, policy makers should also prevent urban sprawl in the urban planning and build a relatively balanced spatial distribution system for employment, housing and public resources. In addition, the government should build a more convenient and reasonable urban public transportation system and encourage residents to take public transportation such as subways and buses to alleviate the current excessive demand for private cars among urban households.
6 Discussion
Compared to developed countries such as Europe and the United States, China is a late starter in field of household energy consumption survey, and the existing data stock is less able to meet the needs of increasingly complex studies. According to the type of data used, domestic studies in China can be basically divided into two categories. The first type of research is mainly based on macro statistics (Wu et al., 2016). In addition to the existence of various types of energy statistics by species, by region and by sector in the energy balance sheet, the statistical yearbook of each city also includes the consumption of three types of energy for urban residents: electricity, gas and heating. Although macro data can be better combined with other macroeconomic indicators and housing conditions in China, it is difficult to reveal the differences and causes of carbon emissions caused by different household consumption patterns, which makes it difficult for relevant research to find out the driving factors of lifestyle differences from the perspective of consumers themselves and put forward specific management suggestions. Therefore, Glaeser and Kahn (2010) proposed the “standardized household,” which combined macro urban data with micro household data and partly made up for the lack of macro data indicators. The second type of research is based on comprehensive survey data. There are a few large-scale special survey on household consumption in China, and most comprehensive survey data only roughly record the expenditure or consumption of individual types of energy used by the households interviewed, making it difficult for researchers to fully grasp all energy types and their consumption characteristics for a long time. Small-scale survey data can make up for the lack of energy types, but the survey area is limited to a specific area such as a city. As can be seen above, previous studies have been constrained by comprehensive micro-survey data, which cannot comprehensively examine the characteristics of energy consumption and carbon emissions of different types households. The CRECS data can not only better integrate with macro data, but also record more complete energy types and consumption, making our research get more comprehensive statistics results of household carbon emissions.
In terms of research methods, our study basically follows the research methods of Glaeser and Kahn (2010) and Zheng S.Q. et al. (2011). Carbon coefficient method is mainly used to measure carbon emissions from household energy consumption, Heckman two-step method is used to explore the relationship between household characteristics and household energy consumption and carbon emissions. For the measurement indicators, we estimate energy consumption for central heating and private car based on CRECS data and using the methods of Zheng et al. (2017), replacing the residential area and city-level transportation data, which are substitute indicators for central heating and transportation energy consumption in previous studies due to the lack of relevant household consumption data. In particular, we take into account the age of the residence, insulation technology, heating area and heating duration in the estimation of energy consumption for central heating. In the estimation of private car energy consumption, we take into account the actual fuel consumption, mileage and fuel type of private cars, which makes our estimation results more reasonable and accurate.
Our research conclusions are basically consistent with the findings of Pfaff et al. (2004), Huo et al. (2010), Glaeser and Kahn (2010), Zheng S. et al. (2011), and Li et al. (2013). With the improvement of income levels, urban residents’ willingness to consumption more energy to meet service demands thereby increasing household energy consumption and carbon emissions. The household carbon emission in Northeast China and resource-based cities are higher, and the same applies to large cities compared with small and medium-sized cities. The migration of urban residents from “green” cities to “brown” cities will generate certain environmental externalities. Urban economic level, population size, temperature and urban form all exert influences on household carbon emissions. Unlike previous studies, our study also find the following: Firstly, households living in suburban or urban villages use inferior energy sources such as coal, firewood and straw, while use clear energy sources such as electricity and natural gas, creating an energy stack of multiple energy sources at the same time (Masera et al., 2000). This is due to the fact that most of the families are rural migrant workers. On the one hand, they maintain their traditional energy use habits, and on the other hand, their income is relatively low, so the cost of continuously using high-powered heating equipment such as air conditioners or high-quality fuels such as natural gas will beyond the family’s ability to pay. In order to save the cost of living, these families have to continue to use coal, straw and firewood, which are inexpensive but polluting energy sources. Secondly, compared with Zheng S. Q. et al. (2011) and Li et al. (2013), the carbon dioxide emissions of standardized household’s central heating and private car calculated in this study are significantly higher in the green city ranking. In addition to the differences in estimation methods, the reason for this change may also be that the residential area and private car ownership of urban residents have increased significantly. Thirdly, compared with previous studies, we find that the environmental externalities caused by households moving from low emission cities to high emission cities have increased. Finally, we find that the polycentric urban spatial structure is beneficial in reducing carbon emissions from private car. However, there is still debate on this issue. Opponents of polycentric development argue that polycentric urban development will further exacerbate the spatial separation of jobs and residences, increase the tendency to cross-commute, and make it easier for residents to choose private cars as a mode of travel (Ha et al., 2021). Therefore, a more detailed and meticulous analysis of this issue will be an important direction for future research.
7 Conclusion and Policy Recommendations
With micro data from the CRECS 2014, this paper has examined the impact of rapid urbanization on the carbon emissions of standardized household in different cities, based on a more comprehensive understanding of the energy consumption of Chinese urban households. The study has found that low-income households living in suburban and urban villages always use coal, firewood, straw and other inferior energy sources, and they are the key objects to realize energy saving and carbon emission reduction of urban households in the future. Second, we find that cities with cold winter climates and resource-based cities have higher carbon emissions after analyzing the city rankings, and cities with lower carbon emission are mostly on both sides of the Huai River/Qinling Mountains line, especially on the south side. Compared with small and medium-sized cities, large and economically developed cities have higher carbon emissions. Third, factors such as urban income, population size and temperatures all have effects on household carbon emissions, the polycentric development of urban spatial structure is conducive to the reduction of carbon emissions from private car.
Based on these empirical results, we propose several suggestions for policymakers. Firstly, the government should formulate differentiated fiscal and energy policies to help low-income households get rid of their dependence on inferior energy sources. While implementing energy upgrading policies such as those for the changes of “coal to electricity” and “coal to gas” in suburban areas, low-income families should be given energy subsidies, realizing financial funds to low-income people and backward areas, so as to make up for the shortcomings of transformation of household energy in urban areas. For high-income households, the relevant authorities should guide them to form low-carbon and reasonable consumption habits through policy promotion. Appropriate carbon taxes should be imposed on high emission areas and “high carbon” consumption behaviors.
Secondly, government should actively consider the application of alternative heating technologies and the adjustment of heating service prices in the face of high carbon emissions from central heating in northern cities. On the one hand, the heating department should speed up the upgrading of urban heating systems and promote the application of new heating technologies such as solar and nuclear energy. On the other hand, two pricing methods that combine basic charging and heat charging should be implemented on a trial basis in other northern heating areas except the northeast. At the same time, the installation of temperature regulation equipment in home can enable residents to control the heating energy consumption of households more flexibly according to their own conditions, thus help to relieve the pressure of carbon emission reduction in northern cities.
Meanwhile, policy makers could encourage developers to build green houses with energy-saving technologies through tax breaks, financial subsidies and green credits. Insulation technology can be chosen for new housing construction (Such as solar systems, ground source heat pump systems, appliances for natural ventilation and Low-E insulation windows), thus reducing energy loss due to indoor and outdoor air exchange. In response to growing transportation carbon emissions, the authorities should impose stricter emission standards for fuel vehicles and tighten licensing control policies to limit the growth in demand for fuel vehicles while encouraging consumers to purchase new energy vehicles and hybrid energy vehicles. Provide “supportive” financial subsidies and tax incentives to new energy vehicle enterprises to reduce the cost of technological innovation, enhance their enthusiasm and thus effectively cultivate the new energy vehicle market. While promoting urban polycentric development, the government should prevent the disorderly expansion of cities and build a balanced spatial distribution system for residence, employment and public resources. At the same time, the government should also build a more convenient and reasonable urban public transportation system and encourage residents to take public transportation such as subways and buses, so as to alleviate the current excessive demand for private cars.
Finally, decision makers should attach importance to green publicity, and guide the public to form a low-carbon and reasonable living habit ideologically. National energy departments should provide more information and guidance on household energy use and promote residents’ understanding of low-carbon lifestyles through activities and programs with educational and popular science content. At the same time, mass media and the communities should inform the public about the hazards of greenhouse gas emissions and make them aware of the urgency and importance of low-carbon energy conservation. When promoting green energy-saving products, the government and enterprises should pay attention to the combination of green energy-saving labels and advertising claims, so as to increase the public’s awareness and trust in energy-saving products and technologies.
However, there are still several limitations of the study. We cannot consider the carbon emissions of firewood and straw in the city rankings due to the small sample size of CRECS, for cities and energy types with insufficient observational samples, we can only supplement and revise them based on yearbook data. The CRECS data only includes the energy consumption of private car, so that we cannot observe the carbon emissions produced by other transportation. We only discuss the effect of urban polycentric development on private vehicle emissions and does not conduct further empirical analysis on its mechanism in more detail. The emergence of various new technologies and policies in recent years has also led to significant changes in residents’ energy use behavior, we can make policy recommendations that are more in line with the reality if we can use the updated CRECS data and contain a larger sample size.
Data Availability Statement
Publicly available datasets were analyzed in this study. This data can be found here: China Household Energy Consumption Survey, http://crecs.ruc.edu.cn/category/uncategorized/; DMSP/OLS nighttime light remote sensing data, https://www.noaa.gov; The city level datasets, http://www.stats.gov.cn. The China Regional Grid Baseline Carbon Emission Coefficient: https://www.mee.gov.cn/ywgz/ydqhbh/wsqtkz/201812/W020181220585203933181.pdf.
Author Contributions
ZL: Conceptualization, methodology, data curation, project administration: YL: Writing-original draft, formal analysis, resources, software; ZZ: Visualization, writing-review and editing, supervision, validation.
Funding
This research has received funding by the National Natural Science Foundation of China (Nos. 72074175, 72174071 and 71774066); General Project for Humanities and Social Sciences Research of Ministry of Education of China (No. 19YJCZH094); Key project of Shaanxi Provincial Department of Education of China (No. 19JZ042).
Conflict of Interest
The authors declare that the research was conducted in the absence of any commercial or financial relationships that could be construed as a potential conflict of interest.
Publisher’s Note
All claims expressed in this article are solely those of the authors and do not necessarily represent those of their affiliated organizations, or those of the publisher, the editors and the reviewers. Any product that may be evaluated in this article, or claim that may be made by its manufacturer, is not guaranteed or endorsed by the publisher.
Supplementary Material
The Supplementary Material for this article can be found online at: https://www.frontiersin.org/articles/10.3389/fenvs.2022.896765/full#supplementary-material
References
Almond, D., Chen, Y., Greenstone, M., and Li, H. (2009). Winter Heating or Clean Air? Unintended Impacts of China's Huai River Policy. Am. Econ. Rev. 99 (2), 184–190. doi:10.1257/aer.99.2.184
Baiocchi, G., Minx, J., and Hubacek, K. (2010). The Impact of Social Factors and Consumer Behavior on Carbon Dioxide Emissions in the United Kingdom. J. Ind. Ecol. 14 (1), 50–72. doi:10.1111/j.1530-9290.2009.00216.x
Bin, S., and Dowlatabadi, H. (2005). Consumer Lifestyle Approach to US Energy Use and the Related CO2 Emissions. Energy Policy 33 (2), 197–208. doi:10.1016/s0301-4215(03)00210-6
Cui, Y., Liu, Y., and Zhuge, C. (2016). Review of Studies on Energy Consumption of Urban Households. China J. Popul. Res. Environ. 26 (12), 117–124. doi:10.3969/j.issn.1002-2104.2016.12.016
Ding, Y., and Li, F. (2017). Examining the Effects of Urbanization and Industrialization on Carbon Dioxide Emission: Evidence from China's Provincial Regions. Energy 125, 533–542. doi:10.1016/j.energy.2017.02.156
Dong, Y., and Zhao, T. (2017). Difference Analysis of the Relationship between Household Per Capita Income, Per Capita Expenditure and Per Capita CO2 Emissions in China: 1997-2014. Atmos. Pollut. Res. 8 (2), 310–319. doi:10.1016/j.apr.2016.09.006
Du, W. (2016). Analysis of Characteristics of Chinese Residents’ Indirect Carbon Emissions in Daily Life Based on LMDI Decomposition Model. China J. Popul. Res. Environ. 26 (S2), 5–9.
Feng, X. Z., Cai, B. F., and Wang, M. (2017). A Comparative Study on Co_2 Emission of Resource-Based Cities in China. China J. Popul. Res. Environ. 27 (02), 5–9. doi:10.3969/j.issn.1002-2104.2017.02.002
Giuliano, G., and Small, K. A. (1993). Is the Journey to Work Explained by Urban Structure? Urban Stud. 30 (9), 1485–1500. doi:10.1080/00420989320081461
Glaeser, E. L., and Kahn, M. E. (2010). The Greenness of Cities: Carbon Dioxide Emissions and Urban Development. J. Urban Econ. 67 (3), 404–418. doi:10.1016/j.jue.2009.11.006
Gordon, P., and Wong, H. L. (1985). The Costs of Urban Sprawl: Some New Evidence. Environ. Plan. A 17 (5), 661–666. doi:10.1068/a170661
Ha, J., Lee, S., and Kwon, S. M. (2021). Revisiting the Relationship between Urban Form and Excess Commuting in US Metropolitan Areas. J. Plan. Educ. Res. 41 (3), 294–311. doi:10.1177/0739456x18787886
Han, X., and Wei, C. (2021). Household Energy Consumption: State of the Art, Research Gaps, and Future Prospects. Environ. Dev. Sustain. 23 (8), 12479–12504. doi:10.1007/s10668-020-01179-x
Heckman, J. J. (1979). Sample Selection Bias as a Specification Error. Econometrica 47, 153–161. doi:10.2307/1912352
Hu, Z., He, J., and Wang, Y. (2018). Factor Analysis and Enlightenment of Household Carbon Emissions in Japan Based on IPAT-LMDI Extension Model. Resour. Sci. 40 (9), 1831–1842. doi:10.18402/resci.2018.09.13
Hu, Z., Wang, M., Cheng, Z., and Yang, Z. (2020). Impact of Marginal and Intergenerational Effects on Carbon Emissions from Household Energy Consumption in China. J. Clean. Prod. 273, 123022. doi:10.1016/j.jclepro.2020.123022
Huo, Y., Zheng, S. Q., and Yang, Z. (2010). Low-carbon Lifestyle and its Determinants: an Empirical Analysis Based on Survey of "household Energy Consumption and Community Environment in Beijing. J. Urban Regional Plan.
Jing, J., Wang, Y. C., and Zheng, X. Y. (2020). Should District Heating Cross the Huai River? Estimation Based on Chinese Residential Energy Consumption Survey. China Econ. Q. 19 (02), 685–708. doi:10.13821/j.cnki.ceq.2020.01.14
Kong, D., and Liang, J. (2021). Analysis of the Status Quo of solar heating under the background of carbon neutrality. E3S Web Conf. 271, 02020. doi:10.1051/E3SCONF/202127102020
Li, S. C., Xiao, W. D., and Wang, Z. B. (2020). Study on Factors Affecting Energy Consumption and. CO2 Emissions Structure in Household Sector——An Empirical Analysis Based on Mode of PLS-SEM. Soft Sci. 34 (02), 117–123. doi:10.13956/j.ss.1001-8409.2020.02.19
Li, Z., Li, G. P., and Hu, Z. (2017). Xi’an household carbon emission characteristics. Resour. Sci. 39 (07), 1394–1405. doi:10.18402/resci.2017.07.16
Li, Z., Li, P., and Guo, J. E. (2013). Impact Factors Estimation and Research on the Differences Across Cities of Residential CO2 Emissions in Chinese Major Cities. China J. Popul. Res. Environ. 23 (10), 87–94. doi:10.3969/j.issn.1002-2104.2013.10.013
Liu, L.-C., Wu, G., Wang, J.-N., and Wei, Y.-M. (2011). China's carbon emissions from urban and rural households during 1992-2007. J. Clean. Prod. 19 (15), 1754–1762. doi:10.1016/j.jclepro.2011.06.011
Liu, X. Y., Li, S. L., and Qin, M. (2017). Urban Spatial Structure and Regional Economic Efficiency: On the Selection of China’s Urbanization Development Patterns. Beijing, China: Management World, 51–64. doi:10.19744/j.cnki.11-1235/f.2017.01.00601
Liu, X. Y., Wang, L. M., and Zhu, S. W. (2016). Urban sprawl and residential carbon emission:panel data on southern cities in China. J. Southeast Univ. (Philosophy Soc. Sci. 18 (05), 101–108+148. doi:10.13916/j.cnki.issn1671-511x.2016.05.014
Lu, H., Yue, A., Chen, H., and Long, R. (2018). Could smog pollution lead to the migration of local skilled workers? Evidence from the Jing-Jin-Ji region in China. Resour. Conservation Recycl. 130, 177–187. doi:10.1016/j.resconrec.2017.11.024
Martins, T. A. d. L., Faraut, S., and Adolphe, L. (2019). Influence of context-sensitive urban and architectural design factors on the energy demand of buildings in Toulouse, France. Energy Build. 190, 262–278. doi:10.1016/j.enbuild.2019.02.019
Masera, O. R., Saatkamp, B. D., and Kammen, D. M. (2000). From Linear Fuel Switching to Multiple Cooking Strategies: A Critique and Alternative to the Energy Ladder Model. World Dev. 28 (12), 2083–2103. doi:10.1016/S0305750X(00)00076-0
Metcalf, G. E. (2009). Designing a Carbon Tax to Reduce U.S. Greenhouse Gas Emissions. Rev. Environ. Econ. Policy 3 (1), 63–83. doi:10.1093/reep/ren015
Pfaff, A., Chaudhuri, S., and Nye, H. (2004). Household Production and Environmental Kuznets Curves-Examining the Desirability and Feasibility of Substitution. Environ. Resour. Econ. 27 (2), 187–200. doi:10.1023/B:EARE.0000017279.79445.72
Shen, K., and Shi, Q. (2018). The impacts of population structure and household size on residential energy consumption: evidences based on provincial-level panel data. Popul. Res. 42 (06), 100–110. CNKI:SUN:RKYZ.0.2018-06-009.
Sun, B., Han, S., and Li, W. (2020). Effects of the polycentric spatial structures of Chinese city regions on CO2 concentrations. Transp. Res. Part D Transp. Environ. 82, 102333. doi:10.1016/j.trd.2020.102333
Sun, H., Edziah, B. K., Sun, C., and Kporsu, A. K. (2021). Institutional quality and its spatial spillover effects on energy efficiency. Socio-Economic Plan. Sci. 2021, 101023. doi:10.1016/j.seps.2021.101023
Sun, H., Samuel, C. A., Kofi Amissah, J. C., Taghizadeh-Hesary, F., and Mensah, I. A. (2020). Non-linear nexus between CO2 emissions and economic growth: A comparison of OECD and B&R countries. Energy 212, 118637. doi:10.1016/j.energy.2020.118637
The McKinsey Global Institute (2007). Leapfrogging to Higher Energy Productivity in China. Shanghai, China. Available at: https://www.mckinsey.com/business-functions/sustainability/our-insights/leapfrogging-to-higher-energy-productivity-in-china (Accessed March 9, 2022).
Tong, Y. F., and Zhou, W. (2020). The Impact of Family Population Aging on Carbon Emissions——An Empirical Study Based on the Micro Perspective of the Family. Popul. J. 42 (03), 78–88. doi:10.16405/j.cnki.1004-129X.2020.03.007
Veneri, P., and Burgalassi, D. (2012). Questioning Polycentric Development and its Effects. Issues of Definition and Measurement for the Italian NUTS-2 Regions. Eur. Plan. Stud. 20 (6), 1017–1037. doi:10.1080/09654313.2012.673566
Wang, Z. H., Ma, J. H., and Zhang, B. (2021). Air Pollution and Residential Migration: Empirical Evidence from Smart Meter Data. Manag. World 37 (03), 19–33+3. doi:10.19744/j.cnki.11-1235/f.2021.0033
Wu, J., Wu, Y., Guo, X., and Cheong, T. S. (2016). Convergence of carbon dioxide emissions in Chinese cities: A continuous dynamic distribution approach. Energy Policy 91, 207–219. doi:10.1016/j.enpol.2015.12.028
Wu, S. M., and Zheng, X. Y. (2022). Revisit of Household Energy Ladder-Empirical Evidence from Household survey in Rural China. China Econ. Q. (01), 45–66. doi:10.13821/j.cnki.ceq.2022.01.03
Yan, H., and Sun, B. D. (2015). The impact of polycentric urban spatial structure on energy consumption: empirical study on the prefecture-level and above cities in China. Urban Dev. Stud. 22 (12), 13–19. CNKI:SUN:CSFY.0.2015-12-012.
Yu, B., Wei, Y.-M., Kei, G., and Matsuoka, Y. (2018). Future scenarios for energy consumption and carbon emissions due to demographic transitions in Chinese households. Nat. Energy. 3 (2), 109–118. doi:10.1038/s41560-017-0053-4
Zhao, Z. Y. (2021). Nuclear energy heating ushered in the development of “window period”. CHINA. ENERGY news. 2021 (012). 11-01. doi:10.28693/n.cnki.nshca.2021.002481
Zheng, S. Q., Huo, Y., and Cao, J. (2011). Elasticity estimation and inter-city variability of residential carbon emissions in Chinese cities. Inq. Into Econ. Issues (09), 124–130. doi:10.3969/j.issn.1006-2912.2011.09.026
Zheng, S., Wang, R., Glaeser, E. L., and Kahn, M. E. (2011). The greenness of China: household carbon dioxide emissions and urban development. J. Econ. Geogr. 11 (5), 761–792. doi:10.1093/jeg/lbq031
Zheng, X., Wei, C., Qin, P., Guo, J., Yu, Y., Song, F., et al. (2014). Characteristics of residential energy consumption in China: Findings from a household survey. Energy Policy 75, 126–135. doi:10.1016/j.enpol.2014.07.016
Keywords: household carbon emissions, standardized household, migration costs, city ranking, reduction pathways
Citation: Li Z, Liu Y and Zhang Z (2022) Carbon Emission Characteristics and Reduction Pathways of Urban Household in China. Front. Environ. Sci. 10:896765. doi: 10.3389/fenvs.2022.896765
Received: 15 March 2022; Accepted: 13 May 2022;
Published: 21 June 2022.
Edited by:
Huaping Sun, Jiangsu University, ChinaReviewed by:
Qingsong He, Huazhong University of Science and Technology, ChinaJean-Michel Guldmann, The Ohio State University, United States
Haoran Zhang, Guangdong University of Finance and Economics, China
Copyright © 2022 Li, Liu and Zhang. This is an open-access article distributed under the terms of the Creative Commons Attribution License (CC BY). The use, distribution or reproduction in other forums is permitted, provided the original author(s) and the copyright owner(s) are credited and that the original publication in this journal is cited, in accordance with accepted academic practice. No use, distribution or reproduction is permitted which does not comply with these terms.
*Correspondence: Yuze Liu, bGl1eXV6ZWZvcm1hbEAxNjMuY29t