- 1Department of Oceanography & Coastal Sciences, Louisiana State University, Baton Rouge, LA, United States
- 2Coastal Studies Institute, Louisiana State University, Baton Rouge, LA, United States
- 3LaHouse Resource Center, Department of Biological and Agricultural Engineering, Louisiana State University Agricultural Center, Baton Rouge, LA, United States
- 4Center for Emergency Management and Homeland Security, Arizona State University, Phoenix, AZ, United States
Louisiana is one of the most hazard-prone states in the U.S., and many of its people are engaged directly or indirectly in agricultural activities that are impacted by an array of weather hazards. However, most hazard impact research on agriculture to date, for Louisiana and elsewhere, has focused on floods and hurricanes. This research develops a method of future crop loss risk assessment due to droughts, extreme low and high temperatures, hail, lightning, and tornadoes, using Louisiana as a case study. This approach improves future crop risk assessment by incorporating historical crop loss, historical and modeled future hazard intensity, cropland extent, population, consumer demand, cropping intensity, and technological development as predictors of future risk. The majority of crop activities occurred and will continue to occur in south-central and northeastern Louisiana along the river basins. Despite the fact that cropland is decreasing across most of the state, weather impacts to cropland are anticipated to increase substantially by 2050. Drought is by far the costliest among the six hazards, accounting for $56.1 million of $59.2 million (∼95%) in 2050-projected crop loss, followed by extreme cold ($1.4 million), extreme heat ($1.0 million), tornadoes ($0.4 million), hail ($0.2 million), and lightning ($0.05 million), respectively. These findings will assist decision-makers to minimize risk and enhance agricultural resilience to future weather hazards, thereby strengthening this economically-important industry in Louisiana and enhancing food security.
Introduction
Increases in population and development bring a sharp increase in the risk (i.e., the product of the probability of a hazardous event and the consequences of that event) associated with weather hazards (Bushra et al., 2021). This risk is exacerbated by policy that incentivizes development without considering the additional complications in mitigating risk to the increasing hazard exposure. The encouragement of additional development only further increases vulnerability and reduces resilience to the hazard in a positive feedback mechanism. Ultimately, environmental, social, and economic sustainability become impractical unless compensating action is taken.
While loss and risk occur in a wide range of ways, much weather hazard research focuses on property damage (e.g., Mostafiz et al., 2020a; 2021a; 2021b; 2021c; 2021d; 2022a) and casualties (e.g., Jonkman 2005). Although such research is beneficial, the risk posed to agriculture is often ignored. Moreover, the risk to agriculture posed by less-catastrophic weather hazards such as those due to lightning (e.g., Zhang et al., 2011), hail (e.g., Changnon 1972), mid-latitude wave cyclones (e.g., Mukherjee et al., 2018), and other weather hazards is also important and understudied, even as underutilized data sources, including comprehensive historical loss databases and sophisticated model output to estimate the changing hazard intensities, exist to improve such future risk assessments (Mostafiz 2022c). Assessing weather hazard risk to agriculture precisely and accurately, especially in spatially heterogeneous areas, is also often problematic due to a coarse scale of analysis. Another complication is that future population and/or land cover changes confound projection of the future risk to agriculture.
The purpose of this research is to address these gaps by developing a geospatially-based risk assessment method for census-tract-level future crop loss due to drought, extreme cold and hot temperatures, hail, lightning, and tornadoes, using Louisiana, one of the most weather-vulnerable U.S. states, as a case study.
Background
Geospatial approaches to understanding the changing weather hazard risk have proliferated in recent years. For example, Kebede and Nicholls (2012) showed that flood exposure increases are a function of the spatial distribution of socio-economic variables (i.e., economic development, urbanization, and population growth). In an analysis of socio-economic factors contributing to natural hazard exposure at the U.S. county-scale, Preston (2013) found that despite disaster risk management successes, the U.S. continues to face dire consequences of increasing economic losses due to extreme weather events. The first Intergovernmental Panel on Climate Change (IPCC) report (Cutter et al., 2012) confirmed these assertions by reporting that societal exposure (and therefore risk as defined here) is a product of development processes on hazardous landscapes and also is an anticipated key driving force contributing to future vulnerability to extreme weather events (Pielke Sr et al., 2007; Hinkel et al., 2010). There remains a paucity of risk assessment work at a scale more local than county-level, especially while also considering changing hazard intensities (Gnan et al., 2022a, 2022b; Mostafiz et al., 2022b, Mostafiz et al., 2022 R. B.; Rahim et al., 2022).
Several recent studies have focused on risk assessment and/or exposure/loss due to drought (e.g., Bushra et al., 2019). Wilhite (2000) noted that drought and agricultural losses in general stand out among weather hazards in terms of risk and exposure, and both are increasing sharply, and lamented the long-problematic lack of reliable, accurate, and accessible historic loss data. More recently, drought monitoring and crop loss prediction has improved with the Visible Infrared Imaging Radiometer Suite (VIIRS) sensor in the National Oceanic and Atmospheric Administration (NOAA) Suomi National Polar-Orbiting Partnership (S-NPP) satellite, which was launched in 2011 (Kogan et al., 2015). Numerous studies suggest that crop growing cycle exposure to drought and other hazards, including extreme temperatures and hail, is increasing with time (Potopova et al., 2016) and is likely to continue increasing, resulting in decreasing crop yields (Guo et al., 2017; Leng and Hall 2019), though benefits of CO2 fertilization and adaptations may be underestimated. Analyses at shorter time scales improve efforts to identify drought impacts on crop yields (Peña-Gallardo et al., 2019). Many of these themes are echoed in the fourth (U.S.) National Climate Assessment (NCA4) from the U.S. Global Change Research Program (USGCRP; Gowda et al., 2018).
Similarly, several recent studies have focused on projecting future risk due to extreme temperatures. Forzieri et al. (2017) concluded that European weather-related risk in 2100 due to cold, heat waves, and other hazards has increased 50-fold over the 1981–2010 period due to the increase in population exposed amid global warming. Zhang and Hu (2018) studied risk assessment of extreme cold temperature events in China using a copula distribution model based on intensity and duration of the hazard. In a crop-focused extreme temperature risk assessment, Annan and Schlenker (2015) found that in the U.S., insured soybeans and corn have 43 and 67 percent more sensitivity, respectively, to extreme heat than uninsured crops, and extreme heat is well-understood to decrease crop yields in Georgia and the Carolinas (Eck et al., 2020). Some (e.g., Lesk et al., 2016) have explained that extreme cold is less impactful than extreme heat, because cold temperatures usually occur outside of the normal growing season. In addition, if the warming temperatures occur uniformly across the seasonal cycle, extreme low temperatures will become less frequent. However, others (e.g., Gu et al., 2008) have suggested that the opposite net effect may occur—that rising temperatures may cause increased vulnerability to cold by inducing premature budding and growth before a subsequent unseasonable cold outbreak.
Overall future crop loss due to hail has not been considered comprehensively, yet improved understanding of such losses is economically important, as crop loss due to hail averages approximately 1 percent of the U.S. national annual crop output (Changnon 1972). Leigh and Kuhnel (2001) modeled loss and risk assessment associated with hail for the Sydney, Australia, region, for insurance purposes. Zhou et al. (2016) assessed the hail damage to potatoes in Washington using aerial multispectral imagery at different growth stages and seasons. Wang et al. (2016) found that hail risk increased (1950–2009) in China at different growing stages of cotton in their county-level GIS-based spatiotemporal study. Púčik et al. (2019) noted that crop loss probability increases when the hail size exceeds 2–3 cm, for central Europe.
Lightning impacts are generally considered to be decreasing vis-à-vis death rate (Mills 2020), but this trend is presumably driven by increased awareness facilitated by technological development, with the risk of injuries and crop and property loss still present. While much research has been invested in identifying lightning risk and its impact on property loss (e.g., Villamil et al., 2015; Mostafiz et al., 2020b; Brooks et al., 2020; He et al., 2020), little research focuses on the lightning-induced risk to crop loss. Kocur-Bera (2018) identified the most sensitive places in Poland to damage from lightning, in addition to drought, cold temperatures, hail, and other hazards, but the short (2010–2014) period of record limits conclusions.
Changnon et al. (2001) suggested that from 1950 to 1997, normalized tornado crop losses in the U.S. displayed no temporal trend. Subsequent work on tornado-driven risk assessments has been conducted for losses to nuclear plants (Reinhold & Ellingwood 1982) and property (Mostafiz et al., 2020b; Refan et al., 2020), and generalized tornado-induced losses have been conducted at the community (Masoomi & van de Lindt 2018), and local scales. While not tornadic in nature, the 2020 Iowa derecho (Hosseini et al., 2020) poignantly demonstrates the tremendous crop damage that can result from severe weather in general. However, a need remains for comprehensive analysis of future tornado risk focused on crop loss.
Regardless of whether the hazard examined is drought, extreme temperatures, hail, lightning, or tornadoes, increasing evidence (e.g., Rahman & Rahman 2015) suggests that a comprehensive management plan in at-risk areas, guided by both traditional and scientific considerations, is vital for assessing risk, enhancing resilience, and progressing toward sustainability. Climate change complicates efforts to improve management strategies and makes the future risk due to these hazards even more uncertain. In projecting the probable economic risk for extreme weather events at various return periods under IPCC scenarios, Franzke and Czupryna (2020) found that the risks can be increased by 3-to-5.4-fold for the U.S. by 2060. Such future risk assessments must incorporate methods for projecting population growth accurately (Wu et al., 2018).
Some attempts have been made to quantify resilience that would be useful for agriculture, such as Lam et al. (2016, 2018), who introduced the resilience inference measurement (RIM) approach. While methods such as the RIM model are useful for quantifying resilience, a similarly appropriate means of assessing the risk remains elusive. This is problematic because risk must be evaluated accurately and precisely at the planning stage for development.
In this study, it is hypothesized that, despite a temporally decreasing land cover in crops in Louisiana (Table 1), crop loss from these hazards will increase by 2050 (Gowda et al., 2018) as additional crop yield (Table 2) and production (Table 3) escalate the risk, primarily driven by the increasing population, consumer demand, and exposure to the hazards due to climate change. Enhanced data and methodological techniques are employed to improve the estimation of crop loss risk to these hazards and thereby enhance the likelihood of improved planning for mitigation, adaptation, and resilience.
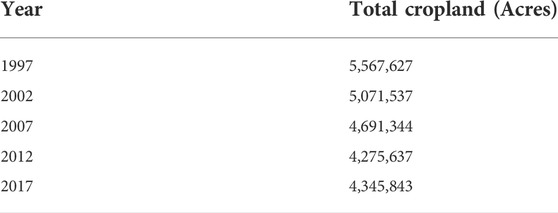
TABLE 1. Total cropland in Louisiana (Source: U.S. Department of Agriculture (USDA) National Agricultural Statistics Service (NASS) 2020).
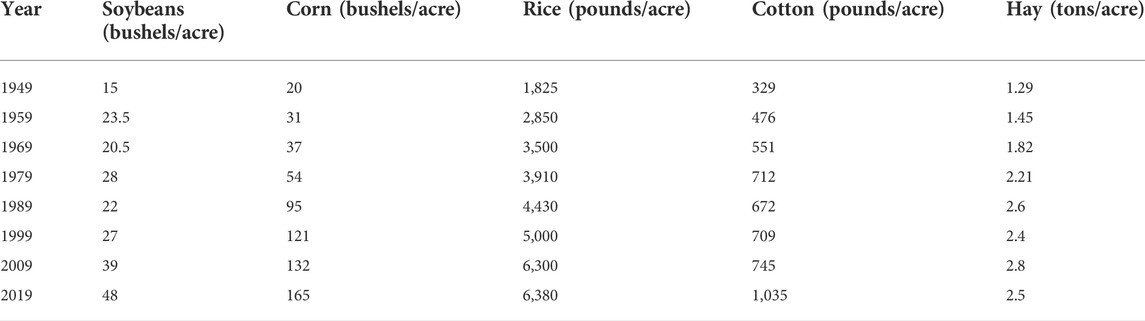
TABLE 2. Yields of the top five Louisiana crops by value (source: USDA NASS 2020).
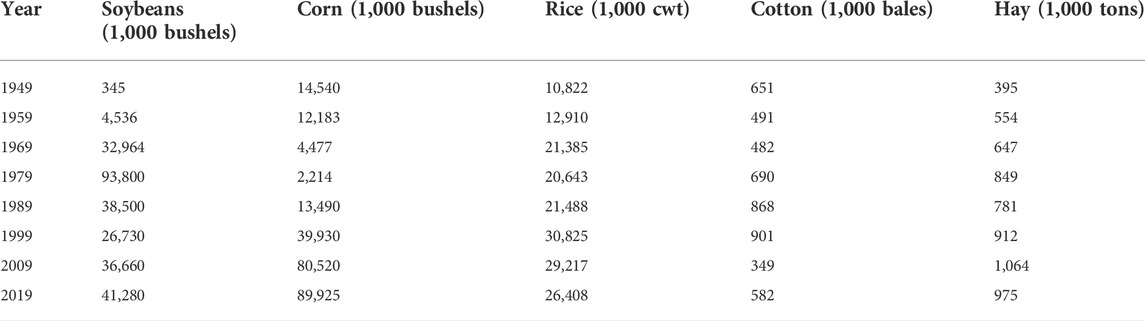
TABLE 3. As in Table 2, but for total production (source: USDA NASS 2020).
Drought, extreme cold, extreme heat, hail, lightning, and tornadoes are selected for analysis here because, with some notable exceptions (e.g., Smith and Katz 2013; Smith and Matthews, 2015) they are understudied, significant, crop-damage-producers statewide, and are important for Louisiana’s State Hazard Mitigation Plan (SHMP). Moreover, with the exception of drought, crop losses due to these hazards can be assessed relatively easily using existing data sources, in contrast to more catastrophic hazards (e.g., floods and hurricanes) in which crop damage occurs from multiple sources (i.e., wind, lightning, tornadoes, etc.) simultaneously but is not partitioned by hazard.
Study area
The U.S. state of Louisiana is highly vulnerable to extreme weather events both in terms of physical exposure as well as in terms of economic and human impacts, given the concentration of people and assets in high-risk areas, especially along the Gulf of Mexico (Mostafiz et al., 2021a). Catastrophic loss events causing more than $1 billion in damage are frequent. Since 1980 alone, Louisiana has been impacted by 25 severe storms, 19 tropical cyclones, 12 droughts, 9 floods, 7 winter storms, and 1 freeze—each causing over $1 billion (2020 Consumer Price Index (CPI) adjustment) in economic damage (NOAA National Centers for Environmental Information (NCEI, formerly known as the National Climatic Data Center (NCDC), 2020). The Southern Plains/Southwest drought and heat wave of spring-summer 2011 cost $14.2 billion (2020 CPI adjusted) and caused 95 deaths in Arizona, Kansas, Louisiana, New Mexico, Oklahoma, and Texas (NOAA NCEI 2020). This drought spanned 107 consecutive weeks and is arguably the longest to hit Louisiana, from 04/20/2010 to 05/01/2012, with approximately 65 percent of Louisiana land cover suffering from exceptional drought (D4) in late June 2011 (United States Drought Portal, 2020). The southeastern U.S. winter storm of January 2000 caused four deaths and $1.1 billion in damage over numerous states, including Louisiana. Severe weather, including high winds, hail, and tornadoes, caused $1.4 billion in damage across several southern states including Louisiana in April 2020 (NOAA NCEI 2020). With agriculture contributing over $3.1 billion, or 2.9 percent of the state’s gross domestic product (University of Arkansas Division of Agriculture 2021), the vulnerability of Louisiana agriculture to weather hazards is substantial, including to the leading crops featured in Tables 2 and 3.
Data
Crop loss data (1960–2019) at the parish level by hazard type originates from the Spatial Hazards Events and Losses Database for the United States (SHELDUS®; Center for Emergency Management and Homeland Security (CEMHS) 2020), which collects its data from the NCEI Storm Events reports. According to NOAA NCEI (2018, p. 14), “crop damage information may be obtained from reliable sources, such as the U.S. Department of Agriculture (USDA), the county (i.e., parish in Louisiana) agricultural extension agent, the state department of agriculture, crop insurance agencies, or any other reliable authority. Crop damage amounts may be obtained from the USDA or other similar agencies.” It should be noted that for Louisiana, drought-induced crop damage was only reported and available to SHELDUS beginning in 1996.
Because the intended purpose of vegetation determines whether its loss is considered as crop damage or property damage (NOAA 2018), this analysis excludes timber, as forested land cover is assumed to be unharvested and/or property rather than crop. By contrast, pasture is considered as cropland because its intended purpose is assumed to be consumed, although loss of the animals that consume the pasture are not considered as crop loss here, but would instead be considered as property loss. Moreover, because SHELDUS does not itemize losses by crop, a “bulk” analysis of all crops is undertaken here. Annual crop loss in SHELDUS are adjusted to 2019$. The indicators of historical hazard severity and their data sources are shown in Table 4.
Because risk is a product of the probability and consequence of the hazard occurrence, and the latter is a function of the cropland value, the hazard intensity data must be accompanied by data on historical and future cropland extent, demand, and population (which impacts demand). For this reason, Louisiana historical land cover data for 2001, 2003, 2006, 2008, 2011, 2013, and 2016 were downloaded from the National Land Cover Database (NLCD) archived by U.S. Geological Survey (USGS, 2016). Rasters containing the only two categories for crops in the NLCD classification system (pasture/hay (category 81) and cultivated crops (category 82)) comprise the cropland cover data, from the NCLD database. Louisiana crop market values available every 5 years from 2002 to 2017 from U.S. Department of Agriculture (USDA) National Agricultural Statistics Service (NASS, 2020) and crop export values available annually (2002─2017) from USDA Economic Research Service (2017)were used as indicator of crop demand. Historical and 2050-projected population data for the world and U.S. were acquired from the U.S. Census Bureau (2020) to assess population growth for projecting that demand to 2050. Louisiana census-tract shapefiles were downloaded from the U.S. Census Bureau (2016). The census tract is the best geographic scale to represent the crop land cover and crop loss because census blocks and block groups are too localized (e.g., <0.01 mi2), while parishes and the state level are too coarse to provide an effective representation for cropland to represent individual crops.
Methods
Because the methodology involves many steps, a flowchart (Figure 1) is used to provide guidance on each step, described in detail in the subsections below.
Historical hazard intensity
For each week, shapefiles of drought intensity from the United States Drought Monitor, (2017), coded according to the first two columns in Table 5, were rasterized using the value in the second column of Table 5, with a pixel size of 0.0005 × 0.0005 decimal degrees. The drought value for each raster cell was averaged across all weeks (2000─2017), providing the mean weekly historical drought intensity by cell.
In the extreme cold and high temperature analysis, for 139 stations within and adjacent to Louisiana (1/1/1992 to 10/14/2017), any daily data that were missing, erroneous (e.g., minimum temperature exceeds maximum temperature on that day), or spurious (following Global Historical Climate Network-Daily (NOAA, 2017a)) criteria, were discarded. Furthermore, any station with discarded temperature data exceeding 10 percent of days were removed, as were stations with less than 5 years of data. These criteria narrowed the analysis to 102 stations. The mean annual frequency of days having temperatures below 32°F, and in a separate analysis, above 95°F, were mapped using “ordinary kriging” with a spherical semivariogram, cell size of 0.0005 × 0.0005 degrees, and variable search radius of 12 points.
Methods for analyzing intensity of the other three hazards were relatively straightforward. A map of mean annual (1982─2011) frequency of days with hail of 0.75 + inches in diameter within 25 miles (NOAA National Severe Storms Laboratory 2014) was digitized. Then, a triangulated irregular network (TIN), with these hail-day contour lines generated as hard edge, was developed. This TIN was then rasterized using linear interpolation at a cell size of 0.005 × 0.005 degrees. Mean annual lightning strike data (1986─2012) were acquired in netCDF format from NOAA, (2017b). These data were rasterized to 4 km × 4 km cells and converted using lightning density in flashes mi-2 yr-1. Tornado touchdown point data (1950─2016) were acquired from the U.S. Storm Prediction Center (SPC; 2017). These data were then processed to calculate the mean annual frequency of days having a touchdown within 40 km, at a 100 m × 100 m cell size, using a spatial probability density heat map derived from kernel density estimation (Epanechnikov 1969) in QGIS®. Further details regarding the methodology employed in assessing historical hazard intensity for extreme cold, hail, lightning, and tornado are described in Mostafiz et al. (2020b).
For each hazard j, where j is 1 through 6, the mean historical hazard intensity by census tract k, where k is 1 through 1148 (Hj,k), was calculated. Hj,k is one of the key factors used for calculating projected crop loss by 2050.
Future hazard intensity
A distinctive feature of our method is the use of statewide adjustment coefficients to represent hazard intensity in future year x; this produced Hj,x of all six hazards taken individually. Because hazard frequencies and/or magnitudes may change in the future, statewide adjustment coefficients for hazard j in future year x (
It was then necessary to determine the values of Fj,x. For drought, although Louisiana precipitation is expected to change little by 2100 (Easterling et al., 2017, their Figure 7.5), enhanced evapotranspiration caused by increased temperatures may result in drying soils by 2100 over much of the continental U.S., including Louisiana, at least under the higher radiative forcing and emissions scenario (Wehner et al., 2017; their Figure 8.1). These changes will impact soil moisture availability in Louisiana. Specifically, in Louisiana, winter, spring, and summer soil moisture decreases, made with a “medium” degree of confidence, are projected to be large relative to natural variability (Wehner et al., 2017). For these reasons, an increase in drought hazard of 25 percent was assumed for the state by 2050, or
Similarly,
An analogous method of representing the severe storm (hail, lightning, and tornado) hazards as that presented in Mostafiz et al. (2020b) was employed here. Specifically, the method of assigning
However, other factors suggest increasing frequency/intensity of future hail- and lightning-producing thunderstorms and tornadoes. Thunderstorm and tornadic activity is most likely when energetic, near-surface air underlies much colder air, so the continued surface warming would destabilize the atmosphere, tending toward a net enhancement of severe storm activity. Brooks (2013) concluded that the vertical temperature gradient, or instability, as represented by a severe weather index known as convective available potential energy (CAPE, measured in J kg−1), is expected to increase. However, Brooks (2013) also noted the compensating effect of expected weakening of the vertical wind shear that spawns tornadoes. Gensini et al. (2014) suggested that atmospheric instability (as represented by frequency of days with abundant CAPE) is likely to weaken over nearly all of Louisiana, for the 2041–2065 period vs. 1981–1995. Collectively, this research guides our assignment of
For each hazard, a sensitivity analysis is run, to produce loss estimates for 2050 assuming an over- or under-estimation by 10 percentage points. For example, the 25 percent increase in the drought hazard would mean that the sensitivity analysis is run assuming values of 1.15, 1.25, and 1.35 for Fj,x.
Quantifying historical annual crop loss and projecting crop land cover change
SHELDUS-based historical, inflation-adjusted (to represent 2019$) crop loss by parish (i) was aggregated to annual total by hazard (j) and used to represent the economic impacts of past events. For each i and j, mean annual crop loss,
The crop land cover area (CLC) from NLCD (including pasture and hay) of each census tract (k) was calculated for the available years (2001, 2004, 2006, 2008, 2011, 2013, and 2016;

TABLE 6. Examples regression-based projection of crop land cover area (km2) by 2050 by census tract (
Projecting consumer demand for louisiana crops
A method of estimating future consumer demand of those crops based on trends historical and current consumption (i.e., domestic vs. international) and future population projections was necessary. Specifically, the percentage of domestic vs. international consumption was calculated from the mean historical market value (Table 7) and export value (Table 8) of Louisiana’s crop-based products. Tables 7,8 suggest that 58.5 percent of Louisiana crops is exported (i.e., consumed.
Internationally, CWorld or 0.585), and therefore, 41.5 percent is consumed domestically (CU.S. or 0.415). U.S. Census Bureau (2020) estimates that U.S. population will increase by 16.9 percent by 2050 (
Thus, assuming that the production will meet this demand, an additional 22.5 percent of crop value (2019$) will be exposed to hazards by 2050.
Projecting cropping intensity and technological development coefficient
It was assumed that technological development will increase agricultural efficiency, as for global trends (Foley et al., 2011), to meet the increasing
This approach assumes that consumer demand increase and crop land cover decrease contribute equally toward the intensity/technological development coefficient.
Projecting future crop loss
Because overall crop loss is impacted by both the presence and intensity of the hazard acting on the crop land cover in a given census tract, a method of representing each is important. For each hazard (analyzed separately), the parish-level, hazard-and-cropland-adjusted loss ratio
This method assigns the total historical parish loss based on the sum of the product of these factors.
To estimate census-tract-level crop loss by hazard in 2050 (
Results and discussion
Historical and future hazard intensity
Historical hazard intensity (Hj) for each hazard is mapped in Figures 2A–F. The northwestern part of Louisiana is the most vulnerable section of the state to drought, extreme heat, and hail (Figures 2A,C,D, respectively). Not surprisingly, extreme cold temperatures are most common throughout northern Louisiana (Figure 2B). Lightning density is concentrated inurban areas, particularly in the southeast (Figure 2E). Tornado intensity peaks prominently in south-central Louisiana, with a secondary area of maximum intensity in northwestern Louisiana (Figure 2F).
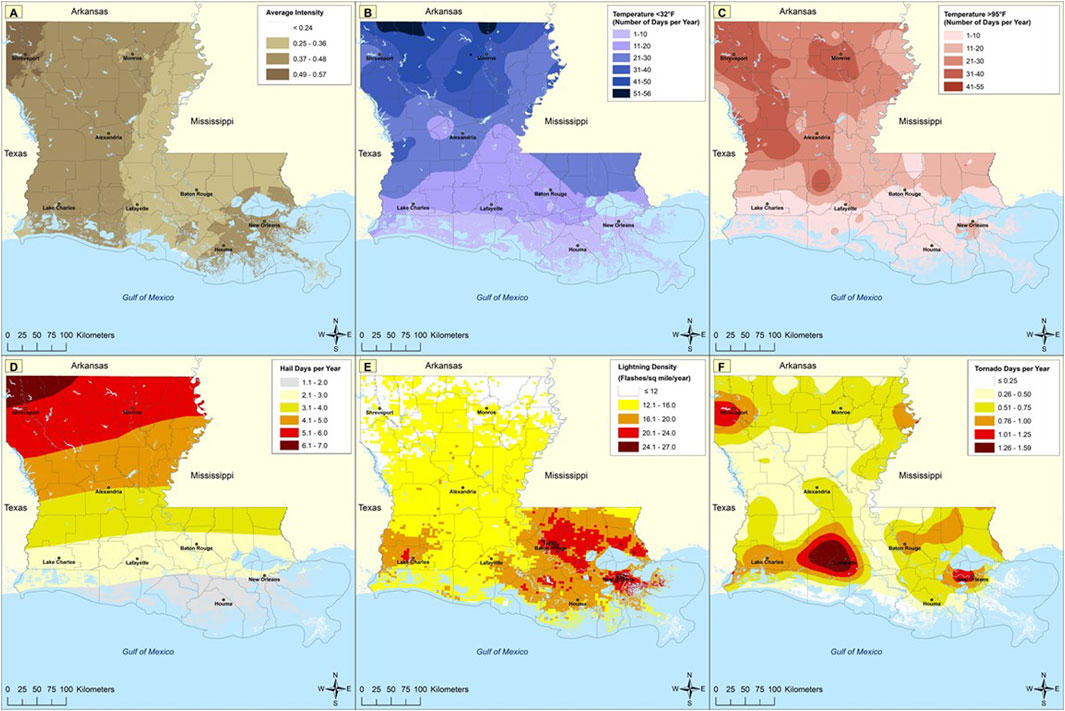
FIGURE 2. Historical mean hazard intensity (Hj) in Louisiana: (A) drought (2000─2017), (B) extreme cold temperature (1992─2017; Mostafiz et al., 2020b), (C) extreme heat (1992─2017), (D) hail (1982─2011; Mostafiz et al., 2020b), (E) lightning (1986─2012; Mostafiz et al., 2020b), and (F) tornado (1950─2016; Mostafiz et al., 2020b).
Projected changes to the hazard intensities (Hj, 2050) by 2050 are shown in Figures 3A–F. Decreases in the extreme cold temperature and hail frequencies are apparent by comparing Figures 2B to 3B and 2D to 3D, respectively. The lightning-intensity hazard is projected to increase for southeastern Louisiana (compare Figures 2E to 3E).
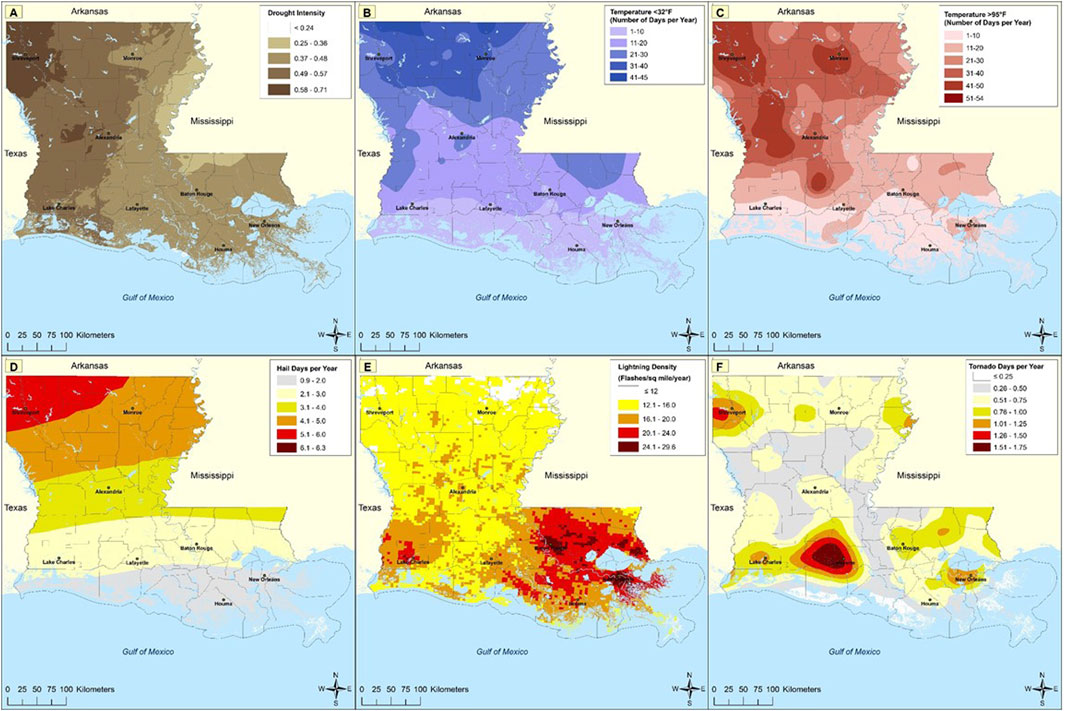
FIGURE 3. As in Figure 2, but for projected future hazard intensity in Louisiana by 2050.
Historical and future projected change in crop land cover
The majority of crop cultivation occurs in south-central and northeastern Louisiana and along the major river basins (Figure 4A). Figure 4B shows the change in crop land cover at the census tract level from 2016 to 2050 in Louisiana. Crop land cover is decreasing in the vast majority of the state, but especially in coastal, north-central, northwestern, and southeastern Louisiana (Figure 4B). Increases in crop land cover are sporadic but are mostly in the northeastern part of the state. By 2050, 320 census tracts are projected to have no crop land cover, compared to 207 census tracts in 2016. CLC is projected to increase in only 24 census tracts and decrease in 722 census tracts, with no change projected in 402 census tracts. Urban infringement and abandonment of coastal lands are major reasons for the anticipated decreases.
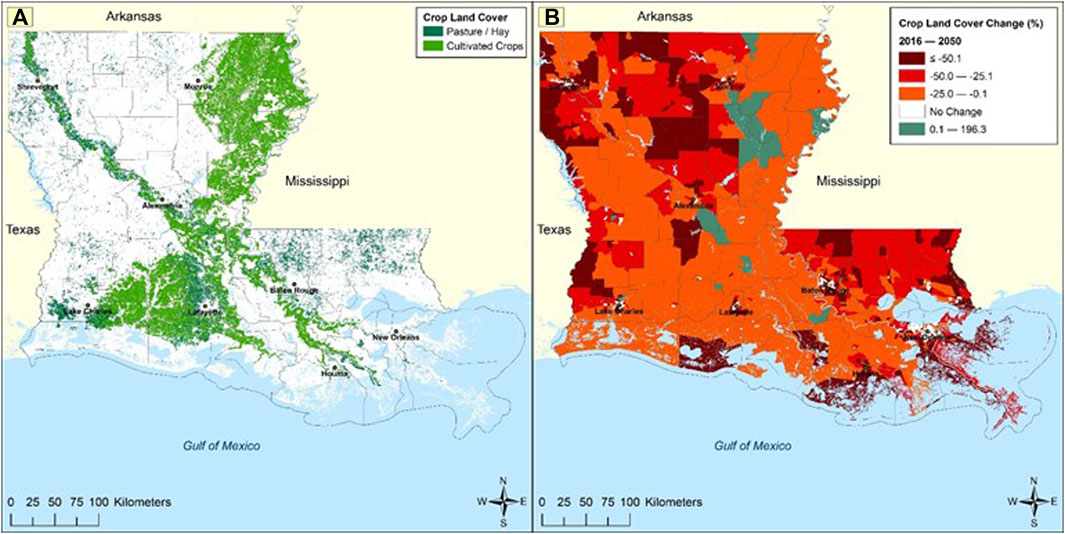
FIGURE 4. Crop land cover in 2016 (A) and change in crop land cover at the census tract level from 2016 to projection for 2050 (B), in Louisiana.
Historical and future projected annual crop loss
Historical annual crop losses due to each of the six hazards in Louisiana are shown in Supplementary Appendix S1A. On a statewide basis, drought caused 93.1 percent of historical average annual crop losses from the hazards analyzed here (Table 9). This result is largely consistent with that from Fahad et al. (2017). The relatively smaller positive impact of climate change via extreme cold rather than via extreme heat tends to support the Lesk et al. (2016) position over that of Gu et al. (2008). Caddo (northwestern Louisiana) had the greatest historical annual crop loss due to drought among the parishes ($6,397,949 or 16.3 percent of Louisiana’s total; Supplementary Appendix S1A). Likewise, Terrebonne and Lafourche (south-central Louisiana) and St. James (southeastern Louisiana) experienced the largest historical annual crop loss from extreme cold ($43,647 or 2.6 percent of the statewide total; Supplementary Appendix S1A) and hot temperatures ($14,020 or 1.9 percent of Louisiana’s total; Supplementary Appendix S1A), respectively. One caveat of this result is that since crop types are not distinguished, it is likely that losses due to high-value crops, such as the citrus orchards in Plaquemines Parish, are underestimated in this analysis. Franklin Parish (northeastern Louisiana) and Cameron Parish (southwestern Louisiana) had the greatest historical annual crop loss due to hail ($31,070 or 19.9 percent of the statewide total; Supplementary Appendix S1A) and lightning ($439 or 11.7 percent of Louisiana’s total; Supplementary Appendix S1A), respectively. Finally, St. Landry Parish in south-central Louisiana sustained the highest historical annual crop loss due to tornado ($77,107 or 24.3 percent of the total; Supplementary Appendix S1A). No historical crop losses due to tornado were reported for Beauregard, Caldwell, Cameron, De Soto, Jackson, La Salle, and Red River parishes.
By 2050, total annual crop loss (
Figures 5A–F shows the widely varying ranges for Lj,2050 by hazard, as was noted previously in Table 9. The absence of crop loss (i.e., risk) in a given area likely infers the absence of crop cultivation (e.g., urban census tracts) and/or historical crop loss for that hazard rather than absence of severe weather threat.
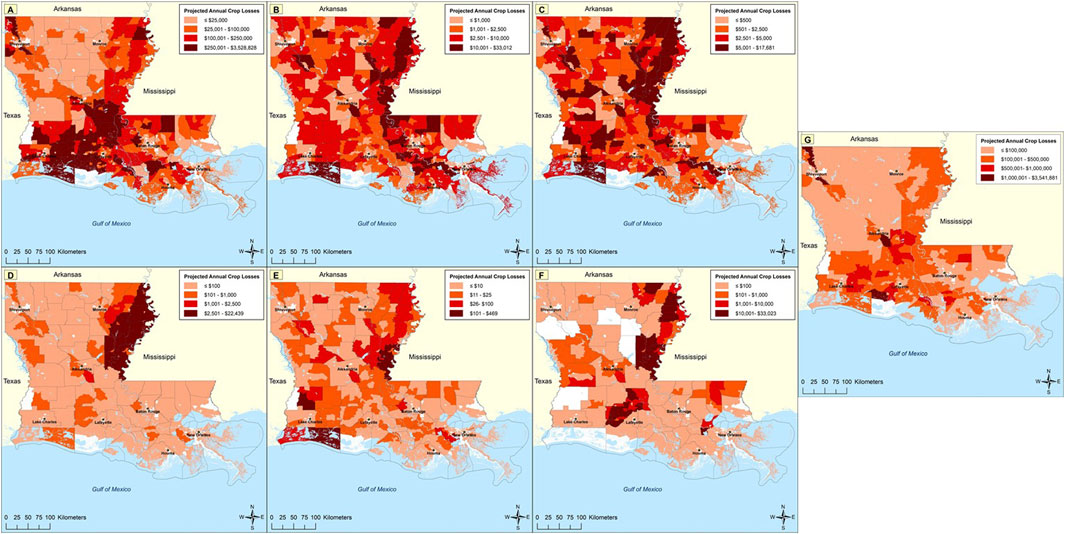
FIGURE 5. Projected annual crop loss (2019$) by Louisiana census tract, 2050: Drought (A), extreme cold temperature (B), extreme heat (C), hail (D), lightning (E), tornado (F), and total (G).
The risk due to drought by 2050 (Figure 5A) is projected to be greatest where the combination of hazard exposure (i.e., northwestern location), cropland (i.e., south-central and northeastern Louisiana), and a history of crop losses occur (i.e., Caddo, Vermilion, Avoyelles, St. Landry, Assumption, Calcasieu, and Jefferson Davis parishes). As was the case for historical data, Caddo is projected to remain the parish with the greatest annual crop loss among the parishes ($8,386,852 or 14.9 percent of Louisiana’s total; Supplementary Appendix S1B) by 2050.
Projected risk from extreme cold temperature annual crop loss in 2050 (Figure 5B) varies substantially by parish but the risk is relatively evenly distributed throughout Louisiana. Assumption Parish (south-central Louisiana) may have the highest annual crop loss ($45,734 or 3.2 percent of the statewide total) due to extreme cold temperatures in 2050 (Supplementary Appendix S1B). The greatest annual extreme cold temperature loss is projected to be in census tract 22121020300 in West Baton Rouge Parish of central Louisiana ($33,011). Extreme heat risk is also projected to be evenly distributed but is more concentrated in the northeastern part of Louisiana (Figure 5C). St. James Parish is projected to have the highest annual crop loss ($21,273) among the parishes (2.2 percent of the state’s total; Supplementary Appendix S1B). Annual crop loss peaks at $ 17,681 in census tract 22021000100 within Caldwell Parish.
Interestingly, cold temperatures pose more of a risk than hot temperatures in Louisiana, despite the projected increased temperatures. It should be noted that extreme heat and the much larger drought risk go hand-in-hand, so some of the extreme heat risk is likely to be accounted for by the drought analysis. Also, because cold and heat waves typically engulf large areas, the SHELDUS data resolve many of the historical crop losses due to extreme cold and heat at a coarse regional scale, causing the historical annual crop loss values due to extreme heat (and separately, due to extreme cold) to be assigned equally across many parishes (Supplementary Appendix S1A). The method employed here allows projected loss to vary across parishes by distributing the loss based on current and projected changes to land cover by census tract and spatial variability in hazard intensity (Supplementary Appendix S1B).
The crop risk to the hail hazard in 2050 is projected to peak in northeastern Louisiana, including Catahoula, Concordia, East Carroll, Franklin, Madison, and Tensas parishes (Figure 5D). Franklin Parish is projected to have the greatest annual crop loss among the parishes ($38,442 or 20.8 percent of Louisiana’s total; Supplementary Appendix S1B). The census tract with the highest projected annual crop loss due to hail ($22,439 in census tract 22065960200) is in Madison Parish.
Lightning risk is projected to peak in sparsely-populated southwestern and northeastern Louisiana (Figure 5E) where hazard, CLC, and historical crop loss overlap. This spatial peak in risk occurs despite a higher hazard intensity elsewhere (see again Figure 3E), as the higher hazard intensity is occurring in largely non-agricultural areas. Cameron Parish is projected to have the greatest annual crop loss ($561) among the parishes (12.2 percent of Louisiana’s total; Supplementary Appendix S1B). Annual crop loss peaks at $468 in Cameron Parish (census tract 22023970100), likely because it is one of the few tracts in Cameron with intense cultivation.
Tornado risk is anticipated to remain much higher than that of hail and lightning. Acadia Parish in southwestern Louisiana is projected to have the highest annual crop loss ($112,376 or 25.3 percent of the state total) due to tornado in 2050 (Supplementary Appendix S1B). At the census tract scale, peak losses are projected to be in the large, northeastern Louisiana census tracts in Catahoula, Concordia, Morehouse, southern East Carroll, and western Madison parishes (Figure 5F). The greatest annual tornado loss is in census tract 22095071100 in St. John the Baptist Parish ($33,022).
Sensitivity analysis
A brief sensitivity analysis to demonstrate the impact of different model assumptions regarding future conditions for each hazard, taken one at a time, is presented in Table 10. The final column in Table 10 shows the estimated change in annual crop loss if an underestimate or overestimate in modeled values of ten percentage points occurs. Interestingly, due to compensating effects of hazard intensities that are projected to increase (i.e., drought, extreme heat, lightning, and tornado) vs. those expected to decrease (i.e., extreme cold and hail), an “across-the-board” underestimation or overestimation for all six hazards leads to bulk total property losses that change by only ±7.5 percent (i.e., bottom row of Table 10).
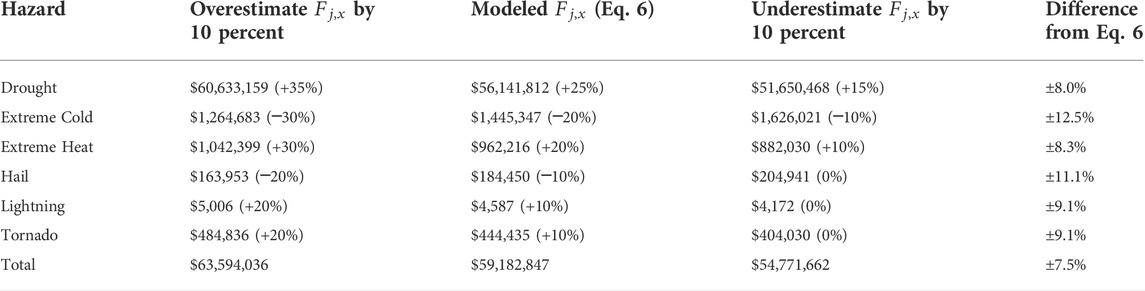
TABLE 10. Sensitivity analysis of annual crop loss (i.e., risk) estimates statewide in Louisiana for each hazard by 2050, for 10 percent overestimation or underestimation of the hazard intensity change (2019$).
Study limitations
Because of the lack of model-output data with high confidence at the sub-state scale, the geographical distribution of the hazards is assumed to be constant over space throughout Louisiana. Similarly, projected temporal changes in hazard intensity by 2050 are assumed to be consistent statewide. In reality, changing frequencies and intensities of synoptic weather patterns that produce extreme weather conditions will not be consistent across space, even at the sub-state scale.
The reliability of the crop loss as projected for the year 2050 depends on the accuracy of input data (i.e., historical hazard intensity, historic crop loss, and crop market and export values) and model estimations (i.e., future hazard conditions, future crop land cover changes, population projection, changing consumer demand, cropping intensity, and technological development). The consideration of annual values can introduce inaccuracies and uncertainties, as climate changes are assumed to occur differently by season, yet the climate model uncertainties still lag behind uncertainties of other models, such as hydrological, at the seasonal scale (Joseph et al., 2018). Human cultural and economic changes, including wealth/GDP, product preferences, adaptations (e.g., changing competition from other producers), and policy are not incorporated in the study.
This study’s source for historic crop losses (SHELDUS) has limitations. First, despite including loss estimates across all hazard types and magnitudes, gaps and biases in these estimates exist in SHELDUS. For example, because SHELDUS assumed the lower bound of the logarithmic range (e.g., $5,000 to $50,000) that NOAA’s NCEI (NCDC at the time) had been using to report loss estimates prior to 1996, losses tended to be underestimated for the earlier decades. Furthermore, indirect losses, including employment hours, health problems during evacuation, and complications caused by other storm-induced stressors, are not included (Gall et al., 2009). A known issue with loss databases and their application to projections of losses is how compound events are categorized. Zscheischler et al. (2018) acknowledged that loss underestimates could occur due to the accounting of compound events as only one hazard type. In the case of the present analysis, given that SHELDUS losses are divided equally, some errors are naturally introduced and unavoidable as the U.S. National Weather Service, the data source for SHELDUS, reports losses as compound event totals only and forgoes estimation by hazard type. A final limitation regarding the crop loss data is that because SHELDUS did not itemize crop loss by crop, the data availability necessitated a “bulk” analysis of all crops, which in fact masks the influences of different climatic extremes on the individual crops.
Data management decisions by the responsible agencies can also introduce uncertainties when used for purposes in this research. For example, although the U.S. National Weather Service strives to collect county-scale loss data, losses from some events are reported only at the multi-county scale. In such cases, SHELDUS partitions losses equally among the affected counties, regardless of differences in population, population density, or development. Because the scale of such events is so broad, the most glaring example of this type of generalization of losses is for extreme heat; nearly all parishes were assigned the same historical crop loss value (Supplementary Appendix S1A). Despite the limitations, SHELDUS data have been used successfully in similar research (e.g., Li et al., 2015; Rohli et al., 2016; Hahn et al., 2017; Paul and Sharif 2018; Mostafiz et al., 2020b) and remains the best available source for U.S. hazard-induced crop loss data.
Summary and conclusion
This study offers an approach for improving risk estimates for six important weather hazards using the example of Louisiana, one of the most weather-vulnerable U.S. states. The method avoids, where possible, aggregating data to a county-level risk. The finer-resolution spatial analysis is valuable because uneven population distribution and/or hazard exposure often makes the scale of natural hazard-induced damage, and therefore the risk, spatially heterogeneous and/or localized. This work also circumvents another perennial complication in risk assessment by incorporating estimates of future changes in both the local hazard intensity and crop land cover; one or both of these factors are often ignored in projecting risk. While our approach certainly cannot avoid making gross assumptions, the use of localized, weighted, model-based projections of crop land cover, consumer demand, and future hazard intensities to estimate census-tract-level risk of crop loss due to several severe weather hazards in Louisiana, United States, by 2050, enhances our current understanding of current and future severe weather impacts.
The major findings of this research are:
1) While the majority of cropland occurs and will continue to occur in south-central and northeastern Louisiana along the river basins, crop activity is decreasing in southeastern and northwestern Louisiana and is increasing in parts of the northeast.
2) By 2050, crop risk, measured as likelihood of economic loss, statewide is likely to continue to be dominated by drought, which is projected to account for $56 million of the $59 million (∼95%) in crop loss by 2050.
3) Extreme cold is likely to continue to produce more damage than extreme heat (although the latter often occurs in tandem with drought), despite the projected warming climate.
4) Tornadoes, hail, and lightning will remain the fourth, fifth, and sixth riskiest hazards examined, respectively.
5) The northeastern part of the state can be expected to remain impacted relatively more heavily than other parts of the state by extreme heat, hail, lightning, and tornadoes.
The findings in this study will help decision-makers to make crops more resilient to future hazards, thereby strengthening the economically-important agriculture industry in Louisiana and enhancing food security.
Work based on a similar methodology is needed to evaluate future risk from other hazards in other locations. Moreover, more sophisticated projections of cropland, consumer demand, cropping intensity, technological development, and demography will improve model projections of future losses, enhancing decision-making for allocating resources to mitigate and adapt to these natural hazards.
Data availability statement
The original contributions presented in the study are included in the article/Supplementary Material, further inquiries can be directed to the corresponding author.
Author contributions
RM developed the detailed methodology, collected and analyzed the data, and developed the initial text. RR developed the atmospheric projections and edited early and late drafts of the text. CF conceptualized the hazard quantification and census tract methodologies and revised the text. MG provided the SHELDUS data and revised the text. NB provided oversight on analysis, particularly regarding the crop land cover projections, and revised the text.
Funding
This project resulted from the 2019 Louisiana State Hazard Mitigation Plan update, for which CF and RR received funding from FEMA, via GOHSEP, grant number: 2000301135. Any opinions, findings, conclusions, and recommendations expressed in this manuscript are those of the authors and do not necessarily reflect the views of FEMA or GOHSEP.
Acknowledgments
The authors warmly appreciate the assistance of Jeffrey Giering of Louisiana’s Governor’s Office of Homeland Security and Emergency Preparedness (GOHSEP) for overall project support, David Dunaway of LSU Libraries for assistance in acquiring agricultural data, and Thanos Gentimis of LSU for statistical guidance.
Conflict of interest
The authors declare that the research was conducted in the absence of any commercial or financial relationships that could be construed as a potential conflict of interest.
Publisher’s note
All claims expressed in this article are solely those of the authors and do not necessarily represent those of their affiliated organizations, or those of the publisher, the editors and the reviewers. Any product that may be evaluated in this article, or claim that may be made by its manufacturer, is not guaranteed or endorsed by the publisher.
Supplementary material
The Supplementary Material for this article can be found online at: https://www.frontiersin.org/articles/10.3389/fenvs.2022.919782/full#supplementary-material
References
Annan, F., and Schlenker, W. (2015). Federal crop insurance and the disincentive to adapt to extreme heat. Am. Econ. Rev. 105 (5), 262–266. doi:10.1257/aer.p20151031
Brooks, H. E. (2013). Severe thunderstorms and climate change. Atmos. Res. 123, 129–138. doi:10.1016/j.atmosres.2012.04.002
Brooks, W., Barnett, D. H., Harrison, W. A., Hattz, D., Mankowski, J., Dickens, J., et al. (2020). Investigation of lightning attachment risks to small structures associated with the electrogeometric model (EGM). IEEE Trans. Plasma Sci. IEEE Nucl. Plasma Sci. Soc. 48 (6), 2163–2174. doi:10.1109/TPS.2020.2989664
Bushra, N., Mostafiz, R. B., Rohli, R. V., Friedland, C. J., and Rahim, M. A. (2021). Technical and social approaches to study shoreline change of kuakata, Bangladesh. Front. Mar. Sci., 8, Art. No. 730984. doi:10.3389/fmars.2021.730984
Bushra, N., Rohli, R. V., Lam, N. S., Zou, L., Mostafiz, R. B., and Mihunov, V. (2019). The relationship between the normalized difference vegetation index and drought indices in the South Central United States. Nat. Hazards (Dordr). 96 (2), 791–808. doi:10.1007/s11069-019-03569-5
Center for Emergency Management and Homeland Security (CEMHS) (2020). Spatial hazard events and losses database for the United States, version 19.0. Center for Emergency Management and Homeland Security, Arizona State University. Available at: https://cemhs.asu.edu/sheldus (Accessed February 11, 2021).
Changnon, S. A., Changnon, J. M., and Hewings, G. J. (2001). Losses caused by weather and climate extremes: A national index for the United States. Phys. Geogr. 22 (1), 1–27. doi:10.1080/02723646.2001.10642727
Changnon, S. A. (1972). Examples of economic losses from hail in the United States. J. Appl. Meteor. 11 (7), 1128–1137. doi:10.1175/1520-0450(1972)011<1128:eoelfh>2.0.co;2
Cutter, S., Osman-Elasha, B., Campbell, J., Cheong, S. M., McCormick, S., Pulwarty, R., et al. (2012). Managing the risks from climate extremes at the local level. Managing the risks of extreme events and disasters to advance climate change adaptation [C. B. Field, V. Barros, T. F. Stocker, D. Qin, D. J. Dokken, K. L. Ebiet al. (eds.)] In: . A special report of working groups I and II of the intergovernmental Panel on climate change (IPCC). Cambridge University Press, Cambridge, UK, and New York, NY, USA, 291–338.
Easterling, D. R., Kunkel, K. E., Arnold, J. R., Knutson, T., LeGrande, A. N., Leung, L. R., et al. (2017). “Precipitation change in the United States,” in Climate science special report: Fourth national climate assessment, volume I. Editors D. J. Wuebbles, D. W. Fahey, K. A. Hibbard, D. J. Dokken, B. C. Stewart, and T. K. Maycock (Washington, DC, USA: U.S. Global Change Research Program), 207–230. doi:10.7930/J0H993CC
Eck, M. A., Murray, A. R., Ward, A. R., and Konrad, C. E. (2020). Influence of growing season temperature and precipitation anomalies on crop yield in the southeastern United States. Agric. For. Meteorology 291, 108053. doi:10.1016/j.agrformet.2020.108053
Epanechnikov, V. A. (1969). Non-parametric estimation of a multivariate probability density. Theory Probab. Appl. 14 (1), 153–158. doi:10.1137/1114019
Fahad, S., Bajwa, A. A., Nazir, U., Anjum, S. A., Farooq, A., Zohaib, A., et al. (2017). Crop production under drought and heat stress: Plant responses and management Options. Front. Plant Sci., 8, Art. No. 1147. doi:10.3389/fpls.2017.01147
Foley, J. A., Ramankutty, N., Brauman, K. A., Cassidy, E. S., Gerber, J. S., Johnston, M., et al. (2011). Solutions for a cultivated planet. Nature 478 (7369), 337–342. doi:10.1038/nature10452
Forzieri, G., Cescatti, A., e Silva, F. B., and Feyen, L. (2017). Increasing risk over time of weather-related hazards to the European population: A data-driven prognostic study. Lancet Planet. Health 1 (5), e200–e208. doi:10.1016/S2542-5196(17)30082-7
Franzke, C. L., and Czupryna, M. (2020). Probabilistic assessment and projections of US weather and climate risks and economic damages. Clim. Change 158 (3), 503–515. doi:10.1007/s10584-019-02558-8
Gall, M., Borden, K. A., and Cutter, S. L. (2009). When do losses count? Six fallacies of natural hazards loss data. Bull. Am. Meteorol. Soc. 90 (6), 799–810. doi:10.1175/2008BAMS2721.1
Gensini, V. A., Ramseyer, C., and Mote, T. L. (2014). Future convective environments using NARCCAP. Int. J. Climatol. 34 (5), 1699–1705. doi:10.1002/joc.3769
Gnan, E., Friedland, C. J., Mostafiz, R. B., Rahim, M. A., Gentimis, T., Taghinezhad, A., et al. (2022b). Economically optimizing elevation of new, single-family residences for flood mitigation via life-cycle benefit-cost analysis. Front. Environ. Sci. 10, 889239. doi:10.3389/fenvs.2022.889239
Gnan, E., Friedland, C. J., Rahim, M. A., Mostafiz, R. B., Rohli, R. V., Orooji, F., et al. (2022a). Improved building-specific flood risk assessment and implications for depth-damage function selection. Front. Water 10, 919726. doi:10.3389/frwa.2022.919726
Gowda, P., Steiner, J. L., Olson, C., Boggess, M., Farrigan, T., and Grusak, M. A. (2018). “Agriculture and rural communities,” in Impacts, risks, and Adaptation in the United States: Fourth national climate assessment, volume II. Editors D. R. Reidmiller, C. W. Avery, D. R. Easterling, K. E. Kunkel, K. L. M. Lewis, T. K. Maycocket al. (Washington, DC, USA: U.S. Global Change Research Program), 391–437. doi:10.7930/NCA4.2018.CH10
Gu, L., Hanson, P. J., Mac Post, W., Kaiser, D. P., Yang, B., Nemani, R., et al. (2008). The 2007 eastern US spring freezes: Increased cold damage in a warming world? Bioscience 58 (3), 253–262. doi:10.1641/B580311
Guo, E., Liu, X., Zhang, J., Wang, Y., Wang, C., Wang, R., et al. (2017). Assessing spatiotemporal variation of drought and its impact on maize yield in Northeast China. J. Hydrology 553, 231–247. doi:10.1016/j.jhydrol.2017.07.060
Hahn, D. J., Emmanuelle, E., and Corotis, R. B. (2017). Multihazard mapping of the United States. ASCE-ASME J. Risk Uncertain. Eng. Syst. Part A Civ. Eng., 3(3), Art. No. 04016016. doi:10.1061/AJRUA6.0000897
He, Y., Lindbergh, S., Graves, C., and Rakas, J. (2020). Airport exposure to lightning strike hazard in the contiguous United States. Risk Anal. 41 (8), 1323–1344. doi:10.1111/risa.13630
Hinkel, J., Nicholls, R. J., Vafeidis, A. T., Tol, R. S., and Avagianou, T. (2010). Assessing risk of and adaptation to sea-level rise in the European union: An application of DIVA. Mitig. Adapt. Strateg. Glob. Chang. 15 (7), 703–719. doi:10.1007/s11027-010-9237-y
Hosseini, M., Kerner, H. R., Sahajpal, R., Puricelli, E., Lu, Y. H., Lawal, A. F., et al. (2020). Evaluating the impact of the 2020 Iowa derecho on corn and soybean fields using synthetic aperture radar. Remote Sens. 12 (23), 3878. doi:10.3390/rs12233878
Jonkman, S. N. (2005). Global perspectives on loss of human life caused by floods. Nat. Hazards (Dordr). 34 (2), 151–175. doi:10.1007/s11069-004-8891-3
Joseph, J., Ghoshab, S., Pathaka, A., and Sahaic, A. K. (2018). Hydrologic impacts of climate change: Comparisons between hydrological parameter uncertainty and climate model uncertainty. J. Hydrology 566, 1–22. doi:10.1016/j.jhydrol.2018.08.080
Kebede, A. S., and Nicholls, R. J. (2012). Exposure and vulnerability to climate extremes: Population and asset exposure to coastal flooding in dar es salaam, Tanzania. Reg. Environ. Change 12 (1), 81–94. doi:10.1007/s10113-011-0239-4
Kocur-Bera, K. (2018). A safe space of rural areas in the context of the occurrence of extreme weather events-A case study covering a part of the Euroregion Baltic. Land Use Policy 71, 518–529. doi:10.1016/j.landusepol.2017.11.013
Kogan, F., Goldberg, M., Schott, T., and Guo, W. (2015). Suomi NPP/VIIRS: Improving drought watch, crop loss prediction, and food security. Int. J. Remote Sens. 36 (21), 5373–5383. doi:10.1080/01431161.2015.1095370
Lam, N. S. N., Qiang, Y., Li, K., Cai, H., Zou, L., and Mihunov, V. (2018). Extending resilience assessment to dynamic system modeling: Perspectives on human dynamics and climate change research. J. Coast. Res. 85 (10085), 1401–1405. doi:10.2112/SI85-281.1
Lam, N. S. N., Reams, M., Li, K., Li, C., and Mata, L. P. (2016). Measuring community resilience to coastal hazards along the northern Gulf of Mexico. Nat. Hazards Rev., 17(1), Art. No. 04015013. doi:10.1061/(ASCE)NH.1527-6996.0000193
Leigh, R., and Kuhnel, I. (2001). Hailstorm loss modelling and risk assessment in the Sydney region, Australia. Nat. Hazards (Dordr). 24 (2), 171–185. doi:10.1023/A:1011855801345
Leng, G., and Hall, J. (2019). Crop yield sensitivity of global major agricultural countries to droughts and the projected changes in the future. Sci. Total Environ. 654, 811–821. doi:10.1016/j.scitotenv.2018.10.434
Lesk, C., Rowhani, P., and Ramankutty, N. (2016). Influence of extreme weather disasters on global crop production. Nature 529 (7584), 84–87. doi:10.1038/nature16467
Li, K., Lam, N. S., Qiang, Y., Zou, L., and Cai, H. (2015). A cyberinfrastructure for community resilience assessment and visualization. Cartogr. Geogr. Inf. Sci. 42 (1), 34–39. doi:10.1080/15230406.2015.1060113
Masoomi, H., and van de Lindt, J. W. (2018). Restoration and functionality assessment of a community subjected to tornado hazard. Struct. Infrastructure Eng. 14 (3), 275–291. doi:10.1080/15732479.2017.1354030
Mills, B. (2020). An updated assessment of lightning-related fatality and injury risk in Canada: 2002–2017. Nat. Hazards (Dordr). 1, 997–1009. doi:10.1007/s11069-020-03942-9
Mostafiz, R. B., Assi, A. A., Friedland, C., Rohli, R., and Rahim, M. A. (2022d). “A numerically-integrated approach for residential flood loss estimation at the community level,” in EGU general assembly 2022 (Vienna, Austria, 23–27. doi:10.5194/egusphere-egu22-10827May
Mostafiz, R. B., Bushra, N., Rohli, R. V., Friedland, C. J., and Rahim, M. A. (2021a). Present vs. future property losses from a 100-year coastal flood: A case study of grand isle, Louisiana. Front. Water, 3, Art. No. 763358. doi:10.3389/frwa.2021.763358
Mostafiz, R. B. (2022c). Estimation of economic risk from coastal natural hazards in Louisiana. LSU Doctoral Dissertations. Available at: https://digitalcommons.lsu.edu/gradschool_dissertations/5880.5880
Mostafiz, R. B., Friedland, C. J., Rahman, M. A., Rohli, R. V., Tate, E., Bushra, N., et al. (2021d). Comparison of neighborhood-scale, residential property flood-loss assessment methodologies. Front. Environ. Sci., 9, Art. No. 734294. doi:10.3389/fenvs.2021.734294
Mostafiz, R. B., Friedland, C. J., Rohli, R. V., and Bushra, N. (2022a). Estimating Future Residential Property Risk Associated with Wildfires in Louisiana, U.S.A. Climate 10 (4), 49. doi:10.3390/cli10040049
Mostafiz, R. B., Friedland, C. J., Rohli, R. V., Bushra, N., and Held, C. L. (2021c). Property risk assessment for expansive soils in Louisiana. Front. Built Environ. 7, 754761. doi:10.3389/fbuil.2021.754761
Mostafiz, R. B., Friedland, C. J., Rohli, R. V., and Bushra, N. (2021b). Property risk assessment of sinkhole hazard in Louisiana, U.S.A. Front. Environ. Sci. 9, 780870. doi:10.3389/fenvs.2021.780870
Mostafiz, R. B., Friedland, C., Rohli, R. V., and Bushra, N. (2020a). Assessing property loss in Louisiana, U.S.A., to natural hazards incorporating future projected conditions. AGU Fall Meet. Abstr. 2020, NH015–0002. Available at: https://ui.adsabs.harvard.edu/abs/2020AGUFMNH0150002M/abstract (Accessed November 23, 2021).
Mostafiz, R. B., Friedland, C., Rohli, R. V., Gall, M., Bushra, N., and Gilliland, J. M. (2020b). Census-Block-Level Property Risk Estimation Due to Extreme Cold Temperature, Hail, Lightning, and Tornadoes in Louisiana, United States. Front. Earth Sci. (Lausanne)., 8, Art. No. 601624. doi:10.3389/feart.2020.601624
Mostafiz, R. B., Rohli, R. V., Friedland, C. J., and Lee, Y. C. (2022b). Actionable Information in Flood Risk Communications and the Potential for New Web-Based Tools for Long-term Planning for Individuals and Community. Front. Earth Sci. (Lausanne). 10, 840250. doi:10.3389/feart.2022.840250
Mukherjee, S., Nateghi, R., and Hastak, M. (2018). A multi-hazard approach to assess severe weather-induced major power outage risks in the U.S. Reliab. Eng. Syst. Saf. 175, 283–305. doi:10.1016/j.ress.2018.03.015
National Oceanic and Atmospheric Administration (2017b). National Centers for Environmental Information. https://www.ncei.noaa.gov/ (Accessed February 11, 2021).
National Oceanic and Atmospheric Administration (2017a). National Centers for Environmental Information. Global Historical Climate Network Daily. Available at: https://www.ncdc.noaa.gov/ghcn-daily-description (Accessed February 11, 2021).
National Oceanic and Atmospheric Administration (2020). National Centers for Environmental Information. U.S. Billion-Dollar Weather and Climate Disasters. Available at: https://www.ncdc.noaa.gov/billions/10.25921/stkw-7w73.
National Oceanic and Atmospheric Administration (2014). National Severe Storms Laboratory. Available at: https://www.nssl.noaa.gov/ (Accessed February 11, 2021).
National Oceanic and Atmospheric AdministrationNational Centers for Environmental Information (2018). Storm Data Preparation. Operations and Services Performance, NWSPD 10-16. Available at: https://www.nws.noaa.gov/directives/sym/pd01016005curr.pdf (Accessed February 11, 2021).
Paul, S. H., and Sharif, H. O. (2018). Analysis of damage caused by hydrometeorological disasters in Texas, 1960–2016. Geosciences 8 (10), 384. doi:10.3390/geosciences8100384
Peña-Gallardo, M., Vicente-Serrano, S. M., Quiring, S., Svoboda, M., Hannaford, J., Tomas-Burguera, M., et al. (2019). Response of crop yield to different time-scales of drought in the United States: Spatio-temporal patterns and climatic and environmental drivers. Agric. For. Meteorology 264, 40–55. doi:10.1016/j.agrformet.2018.09.019
Pielke Sr, R. A., Adegoke, J., BeltraáN-Przekurat, A., Hiemstra, C. A., Lin, J., Nair, U. S., et al. (2007). An overview of regional land-use and land-cover impacts on rainfall. Tellus B Chem. Phys. Meteorology 59 (3), 587–601. doi:10.1111/j.1600-0889.2007.00251.x
Potopova, V., Boroneanţ, C., Boincean, B., and Soukup, J. (2016). Impact of agricultural drought on main crop yields in the Republic of Moldova. Int. J. Climatol. 36 (4), 2063–2082. doi:10.1002/joc.4481
Preston, B. L. (2013). Local path dependence of U.S. socioeconomic exposure to climate extremes and the vulnerability commitment. Glob. Environ. Change 23 (4), 719–732. doi:10.1016/j.gloenvcha.2013.02.009
Púčik, T., Castellano, C., Groenemeijer, P., Kühne, T., Rädler, A. T., Antonescu, B., et al. (2019). Large hail incidence and its economic and societal impacts across Europe. Mon. Weather Rev. 147 (11), 3901–3916. doi:10.1175/MWR-D-19-0204.1
Rahim, M. A., Gnan, E. S., Friedland, C. J., Mostafiz, R. B., and Rohli, R. V. (2022). “An Improved Micro Scale Average Annual Flood Loss Implementation Approach,” in EGU general assembly 2022 (Vienna, Austria, 23–27. doi:10.5194/egusphere-egu22-10940May
Rahman, M. A., and Rahman, S. (2015). Natural and traditional defense mechanisms to reduce climate risks in coastal zones of Bangladesh. Weather Clim. Extrem. 7, 84–95. doi:10.1016/j.wace.2014.12.004
Refan, M., Romanic, D., Parvu, D., and Michel, G. (2020). Tornado loss model of Oklahoma and Kansas, United States, based on the historical tornado data and Monte Carlo simulation. Int. J. Disaster Risk Reduct. 43, 101369. doi:10.1016/j.ijdrr.2019.101369
Reinhold, T. A., and Ellingwood, B. (1982). Tornado damage risk assessment (No. NUREG/CR--2944). Washington, DC (USA): National Bureau of Standards.
Rohli, R. V., Bushra, N., Lam, N., Zou, L., Mihunov, V., Reams, M. A., et al. (2016). Drought indices as drought predictors in the south-central USA. Nat. Hazards (Dordr). 83 (3), 1567–1582. doi:10.1007/s11069‒016‒2376‒z
Smith, A. B., and Katz, R. W. (2013). US billion-dollar weather and climate disasters: Data sources, trends, accuracy and biases. Nat. Hazards (Dordr). 67, 387–410. doi:10.1007/s11069-013-0566-5
Smith, A. B., and Matthews, J. L. (2015). Quantifying uncertainty and variable sensitivity within the US billion-dollar weather and climate disaster cost estimates. Nat. Hazards (Dordr). 77 (3), 1829–1851. doi:10.1007/s11069-015-1678-x
Storm Prediction Center (2017). National Oceanic and Atmospheric Administration. https://www.spc.noaa.gov/ (Accessed February 11, 2021).
United States Census Bureau (2020). Census Bureau Releases International Population Estimates and Projections, International data base web tool. https://www.census.gov/data-tools/demo/idb/#/country?YR_ANIM=2020 (Accessed February 11, 2021).
United States Census Bureau (2016). TIGER/Line Shapefiles. https://www.census.gov/geographies/mapping-files/time-series/geo/tiger-line-file.html (Accessed February 11, 2021).
United States Department of Agriculture (USDA) (2017). Economic Research Service. Available at: https://www.ers.usda.gov/data-products/state-export-data/annual-state-agricultural-exports/ (Accessed February 11, 2021).
United States Department of Agriculture (USDA) (2020). National Agricultural Statistics Service. Available at: https://www.nass.usda.gov/ (Accessed February 11, 2021).
United States Drought Monitor (2017). National Oceanic and Atmospheric Administration. Available at: https://droughtmonitor.unl.edu/ (Accessed February 11, 2021).
United States Drought Portal (2020). National Integrated Drought Information System. Available at: https://www.drought.gov/drought/states/louisiana#:∼:text=from%202000%20%2D%202020,affected%2064.94%25%20of%20Louisiana%20land (Accessed February 11, 2021).
United States Geological Survey (Usgs), (2016). Available at: https://www.usgs.gov/centers/eros/science/national-land-cover-database?qt-science_center_objects=0#qt-science_center_objects (Accessed February 11, 2021).
United States Global Change Research Program (2017). in Climate science special report: Fourth national climate assessment, volume I. Editors D. J. Wuebbles, D. W. Fahey, K. A. Hibbard, D. J. Dokken, B. C. Stewart, and T. K. U. S. Maycock (Washington, D.C., USA: Global Change Research Program), 470. doi:10.7930/J0J964J6
University of Arkansas Division of Agriculture, (2021). Economic impact of agriculture. Available at: https://economic-impact-of-ag.uark.edu/louisiana/(Accessed February 11, 2021).
Villamil, D. E., Santamaria, F., and Diaz, W. (2015). “Lightning disaster risk assessment method in Colombia,” in 2015 International Symposium on Lightning Protection (XIII SIPDA) (IEEE), 146–152.
Vose, R. S., Easterling, D. R., Kunkel, K. E., LeGrande, A. N., and Wehner, M. F. (2017). “Temperature changes in the United States,” in Climate science special report: Fourth national climate assessment, volume I. Editors D. J. Wuebbles, D. W. Fahey, K. A. Hibbard, D. J. Dokken, B. C. Stewart, and T. K. Maycock (Washington D.C.: U.S. Global Change Research Program), 185–206. doi:10.7930/J0N29V45
Wang, L., Hu, G., Yue, Y., Ye, X., Li, M., Zhao, J., et al. (2016). GIS-based risk assessment of hail disasters affecting cotton and its spatiotemporal evolution in China. Sustainability 8 (3), 218. doi:10.3390/su8030218
Wehner, M. F., Arnold, J. R., Knutson, T., Kunkel, K. E., and LeGrande, A. N. (2017). “Droughts, floods, and wildfires,” in Climate science special report: Fourth national climate assessment, volume I [wuebbles. Editors D. W. Fahey, K. A. Hibbard, D. J. Dokken, B. C. Stewart, and T. K. Maycock (Washington, DC, USA: U.S. Global Change Research Program), 231–256. doi:10.7930/JOCJ8BNN
Wilhite, D. A. (2000). Chapter 1 Drought as a Natural Hazard: Concepts and Defnitions, 69. Drought Mitigation Center Faculty Publications. Available at: https://digitalcommons.unl.edu/droughtfacpub/69/?utm_source=digitalcommons.unl.edu%2Fdroughtfacpub%2F69&utm_medium=PDF&utm_campaign=PDFCoverPages (Accessed November 23, 2021).
Wu, J. D., Li, Y., Li, N., and Shi, P. J. (2018). Development of an asset value map for disaster risk assessment in China by spatial disaggregation using ancillary remote sensing data. Risk Anal. 38 (1), 17–30. doi:10.1111/risa.12806
Zhang, W., Meng, Q., Ma, M., and Zhang, Y. (2011). Lightning casualties and damages in China from 1997 to 2009. Nat. Hazards (Dordr). 57 (2), 465–476. doi:10.1007/s11069-010-9628-0
Zhang, X., and Hu, H. (2018). Copula-based hazard risk assessment of winter extreme cold events in Beijing. Atmosphere 9 (7), 263. doi:10.3390/atmos9070263
Zhou, J., Pavek, M. J., Shelton, S. C., Holden, Z. J., and Sankaran, S. (2016). Aerial multispectral imaging for crop hail damage assessment in potato. Comput. Electron. Agric. 127, 406–412. doi:10.1016/j.compag.2016.06.019
Keywords: weather impacts, natural hazards, crop loss, resilience, Louisiana, risk assessment
Citation: Mostafiz RB, Rohli RV, Friedland CJ, Gall M and Bushra N (2022) Future crop risk estimation due to drought, extreme temperature, hail, lightning, and tornado at the census tract level in Louisiana. Front. Environ. Sci. 10:919782. doi: 10.3389/fenvs.2022.919782
Received: 13 April 2022; Accepted: 21 July 2022;
Published: 23 August 2022.
Edited by:
Teng Wu, University at Buffalo, United StatesReviewed by:
Meiry Sayuri Sakamoto, Fundação Cearense de Meteorologia e Recursos Hídricos, BrazilSina Nabaei, Science and Research Branch, Islamic Azad University, Iran
Copyright © 2022 Mostafiz, Rohli, Friedland, Gall and Bushra. This is an open-access article distributed under the terms of the Creative Commons Attribution License (CC BY). The use, distribution or reproduction in other forums is permitted, provided the original author(s) and the copyright owner(s) are credited and that the original publication in this journal is cited, in accordance with accepted academic practice. No use, distribution or reproduction is permitted which does not comply with these terms.
*Correspondence: Rubayet Bin Mostafiz, cmJpbm1vMUBsc3UuZWR1