- College of Economics, Shandong University of Technology, Zibo, China
The accumulation of rural human capital is one of the important factors to realize the sustainable development of the rural economy. Digital inclusive finance eases the budget constraints of farmers and affects their willingness and ability to invest in education which improves human capital accumulation. The impact of digital inclusive finance on rural human capital accumulation is strong but complex to determine. Based on the data from 31 provinces of China from 2011 to 2020, this study uses the systematic Generalized Moment Method (GMM) to build an analytical framework to explore the impact mechanism and differences of digital inclusive finance over the rural human capital accumulation regional levels. The study found that with the development of digital inclusive finance and the expansion of the breadth of coverage, and usage of digital inclusive finance can significantly enhance the accumulation of rural human capital. In terms of regional differences, the boosting effect of digital inclusive finance on rural human capital accumulation is greater in the Northeast than that in the Central, Western and Eastern regions of China. The impact of the extent of digital inclusive finance coverage on rural human capital accumulation is significantly greater in Northeast and Eastern regions than in Central and Western regions; the impact of the depth of digital inclusive finance usage on rural human capital accumulation is limited in Central China. Its findings and results can help to further develop digital inclusive finance, particularly for human capital accumulation in rural areas.
Introduction
In literature, human capital accumulation has captured considerable attention from scholars. Human capital is considered a pivotal element of economic growth, efficiency, and productivity. The process of accumulation of this capital has become a competitive advantage for modern economies. Here, the infusion of digitalization for human capital accumulation has boosted economic efficiency and development in such a contemporary and competitive environment. On the other hand, as the world is progressing in terms of digitalization, it has become a crucial element of human life to accept this change and live accordingly (Prasetyo, 2019a; Prasetyo, 2019b; Grigorescu et al., 2021; Grigorescu et al., 2021). Principally, human capital accumulation is directly linked with technological advancement (Ganeva, 2010). It is theoretically proven that human capital incurs a positive influence on economic growth and sustainability (Ahmad et al., 2021a). Although in various empirical investigations, this association is often not recognized because of other exogenous factors, but still, the importance of human capital accumulation in economic progress cannot be ignored (Prasetyo, 2020).
In the digital trend, financial innovation and the development of information and communication technology can promote sustainable economic growth in China (Shehzad et al., 2021). Digital Inclusive Finance, as a combination of the two, makes it easier for the people who were not enjoying the financial services before and were deprived of these. It can affect the lifestyle of rural inhabitants in different ways. Firstly, it will offer the availability of credit lines to them. It means that farmers will have easy access to loans and other banking facilities to improve their businesses. Secondly, it may help to develop the rural human capital and its accumulation by offering easy financial assistance to promote higher and technical education. Thirdly, it may affect the accumulation of rural human capital by giving more job opportunities and other sources of income to rural residents (Wang et al., 2022). An increase in income level will raise the propensity of the people to spend income on education and technical learning. In order to provide a clearer picture of the relationship between digital inclusion and rural human capital accumulation, below is given the linkages of them with each other (Figure 1).
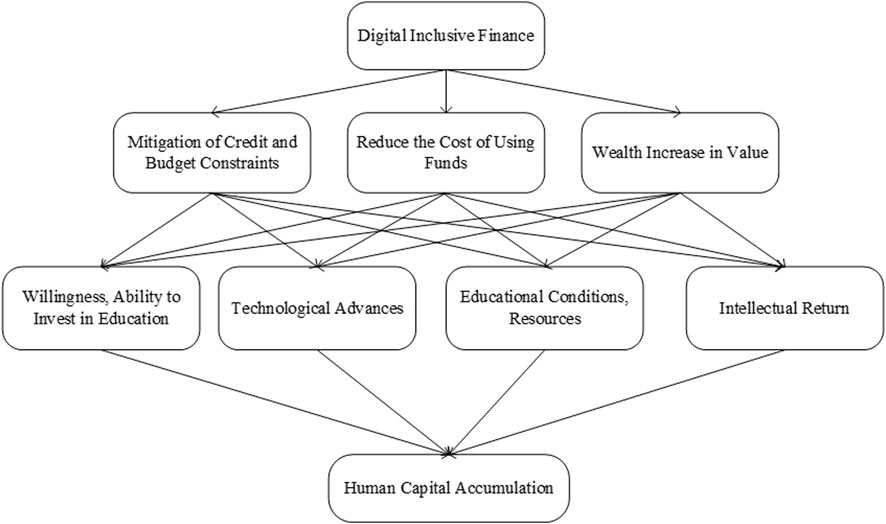
FIGURE 1. Diagram of the pathway of digital inclusive finance impacting rural human capital accumulation. Source: Drawn by the author.
Digital finance inclusion has signified rapid economic and business expansion in China over the past few years which is highly remarkable. Despite this, empirical evidence regarding digital financial inclusion’s contribution to the determination of economic growth is moderately thin (Ahmad et al., 2021b). Additionally, none of the past research has initialized the role of human capital accumulation in this consideration. Therefore, this study tries to examine the digital financial inclusion in the accumulation of China’s rural human capital. New proxies have been introduced for measuring digital finance inclusion containing a breadth of coverage, degree of digitalization, and depth of use.
By taking into account key findings of subsequent detailed discussion, this research focuses on the impact of digital finance inclusive on human capital accumulation specifically in rural areas of China. Additionally, each component of Digital Financial Inclusion (DFI) will be examined individually to affirm the robustness of estimates obtained from the digital financial inclusion index reliability. In addition, the role of urbanization, inflation, degree of openness, and disposable income have also been taken into account for the accumulation of human capital in the rural areas of China. The central focus was asserted on the socially disadvantaged group of Chinese living in rural areas. This study uses panel data from 31 rural regions of China for the period 2011–2020. For statistical estimation, a GMM dynamic panel model is used for empirical analysis, with the core explanatory variable being the Digital Inclusive Finance Index, the explanatory variable being rural human capital accumulation (using average years of schooling instead), and the control variables being the urbanisation rate, inflation rate, per capita disposable income, state fiscal spending on education, and openness to the outside world.
In this research following questions are to be answered:
1. Does Digital Financial Inclusion has an impact on rural human capital accumulation, particularly in the case of China?
2. What is the impact of the different dimensions encompassed by digital financial inclusion on rural human capital accumulation in China?
3. What is the impact of digital financial inclusion on rural human capital accumulation in Eastern, Central, Western, and North-Eastern China?
For the last 2 decades, human capital accumulation is one of the major economic topics the researchers to work on to determine its impact on the sustainability and development of economies. Various studies illustrated that there are various external and internal variables or factors that better explain and determine human capital accumulation. Although, there is a need to integrate the attention of researchers towards the role of digital financial inclusion. In this regard, plenty of research have been conducted about digital financial inclusion and its role in changing and developing modern economies. However, there is a need to highlight the role of digital financial inclusion in accelerating human capital accumulation. Here, this study tries to incorporate digital financial inclusion and human capital accumulation.
The remainder of the study is structured as follows: Literature review provides a review of the relevant field literature, Research data and methods defines the data and methodological framework, Results presents the results of the study and Conclusion and policy implications summarise the conclusions and policy recommendations.
Literature review
Literarily, human capital accumulation is to gather and develop a strong value of the human capital in the economy. However, in economic terminology, it is to develop, train and gather a strong human capital force that is directed to achieve high productivity, efficiency, technological advancement, and economic activities (Jorgenson and Pachon, 1983). Different economic theories have used this broader term within the spectrum of various core variables. For instance, (Dalton et al., 2005; Henderson and Russell, 2005; Prskawetz et al., 2008; Lucas, 2015) used this term for getting high productivity; (Cinnirella and Streb, 2017; Munjal and Kundu, 2017; Van Uden et al., 2017) used it for high innovation and technological advancement; (Stijns, 2006; Becker, 2009) used it for cost efficiency, especially in labor-intensive countries; (Wright and McMahan, 2011; Ployhart et al., 2014) used it to resource management. In fact, this has a pivotal role in economic stability and development in multiple dimensions.
In this era of globalization and digitalization, the global formal financial system has witnessed considerable growth with digital inclusive finance. This is due to the fact that it has helped the economies in a better way to manage human and capital resources which resulted in the eradication of poverty, involvement of the wider population in the economic activities, and creation of competitive advantage. There is no doubt that in modern economies the digitalization of the financial system has included even those segments of the societies which were out of the economic activities due to nonavailability of the financial services (Naumenkova et al., 2019). This financial inclusion is not only limited to dealing with day-to-day transactions but also provides financial resources to the human capital to upgrade their knowledge, skills, and role to meet the contemporary business and economic challenges (Ozili, 2021).
Modern economic theories highlight the relationship between financial inclusion with the human capital accumulation for economic optimization and expansion. Similarly, (Hong Vo et al., 2021) conducted an empirical investigation on Southeast Asian countries and illustrated that digital inclusive finance has a significant role in making human capital accumulation, especially in labor intensive countries.
There are various empirical studies which have highlighted the importance of human capital accumulation in the economic growth and development, especially in labor-intensive economies like China. Digital inclusive finance in rural areas is also provoked because of the positive and significant impact of human capital accumulation on the economic growth (Pelinescu, 2015). According to (Prasetyo, 2019b), economic growth is an outcome of technological changes which is obtained from human capital accumulation. Human capital accumulation will lead to economic effectiveness, efficiency, and productivity that encourage competitiveness in the international market. In sum, human capital provokes economic growth at both micro and macro levels.
In the classical general economic theory, the role of human capital is considered an exogenous factor without considering the role of productivity, education, and training in economic activities. On the other side, the market value theory considers the significant role of education and creativity in developing human capital which, in long run, leads to economic development and sustainable growth (Hong Vo et al., 2021). In fact, various theories have highlighted the different impacts and importance of human capital accumulation for economic growth.
Li and Li, (2022) studied the impact of digital inclusive finance in the case of China. The Plan for Promoting the Development of Inclusive Finance (2016–2020) and the G20 High-Level Principles on Inclusive digital Finance in 2016 were used to investigate the impact of these plans on the innovation and economic activities in urban areas of China. It was empirically witnessed that digital inclusive finance promoted urban innovation by supporting the effective allocation of credit resources, expenditures, and technological advancement.
After the success of digital inclusive finance in urban areas of China, this financial model is going to influence the rural areas of China which was backward and financially deprived. The financial disparity has promoted urbanization and affected the human capital accumulation in rural areas. Liu et al. (2021a) elaborated on the digital inclusive finance and its impact on the agricultural development and increase in the lifestyle of the people in the rural areas of China. These digital financial services make it possible to provide credit facilities to rural people which has increased their lifestyles and economic activities. This has attracted human capital accumulation in rural areas of China.
Digital inclusive finance has offered financial support for education, farming, technological advancement, pesticides, imported machinery, and even access to the international markets (Liu et al., 2021b). According to (Gomber et al., 2017), digital financial business functions including digital financing, digital investment, and digital payment, digital financial advice have transformed traditional inclusive finance into inclusive digital finance. Furthermore, (Ozili, 2018) elaborated on the role of government, financial institutions, and financial technology to promote financial services in remote areas. Arora and Ratnasiri, (2011) elaborated on the relationship between financial inclusion and the development of human capital in the case of India. This result is also witnessed in case of Italy in which the evolution of educational institutions resulted into high-quality human resource accumulation and national growth (Bertola and Sestito, 2011). Aziz and Naima, (2021) highlighted the increase in education, eradication of poverty, development of human capital, and progress in economic growth after the digital inclusive finance in the case of Bangladesh.
Economies are focusing on inclusive digital finance to deploy financial resources in underdeveloped and emerging markets. Private and public organizations are working jointly to promote inclusive digital finance in different deprived areas. According to the World Bank Report (2018), China is one of the largest economies which showed rapid growth in promoting inclusive digital finance to improve the development of the deprived markets. One of the significant links between digital inclusive finance and economic growth in the case of China is human capital accumulation. This has a significant role in the upgradation of technological advancement, education, and prosperity in the rural areas of China.
In fact, various theoretical and empirical studies illustrate the positive impact of digital Inclusive Finance on urbanization, technological progress, high productivity, human capital development, and economic growth. However, most of the research areas are urban areas, and the research on describing this positive relationship in rural areas is limited. As the infrastructure, economic development, and cultural level in rural areas are generally lower than those in urban areas, digital inclusive finance plays a significant role in promoting economic development in backward areas and helping vulnerable groups. It is worth discussing whether digital Inclusive Finance has promoted the accumulation of human capital in rural areas. According to the above literature review, the literature on analyzing the impact of digital Inclusive Finance on rural human capital accumulation is still missing. Therefore, this study fills this gap and discusses the impact of digital Inclusive Finance on rural human capital accumulation in provinces of China. Based on this, we propose the following four Hypotheses:
1) Hypothesis-1
Ho1 = Digital inclusive finance can alleviate financial exclusion in rural areas and has a positive impact on rural human capital accumulation.
2) Hypothesis-2
Ho2 = Breadth of digital inclusive financial coverage can contribute to rural human capital accumulation and there is regional heterogeneity.
3) Hypothesis-3
Ho3 = Degree of digitization of inclusion finance can promote rural human capital accumulation and there is regional heterogeneity.
4) Hypothesis-4
Ho4 = Depth of use of digital inclusive finance can contribute to rural human capital accumulation and there is regional heterogeneity.
The study adopts a dynamic GMM research method to conduct an empirical study, which is novel in that it: combines digital inclusive finance with rural human capital accumulation, and empirically analyses the impact of digital inclusive finance on rural human capital accumulation at a provincial level in China, and explores the development of rural human capital accumulation under the development of digital inclusive finance. This study can enrich the research field of digital inclusive finance and rural human capital accumulation by paying more attention to the impact of digital inclusive finance on less developed regions, especially to provide empirical references for developing countries to promote rural human capital accumulation.
Research data and methods
Data
In this study, the researcher utilizes a panel dataset for the 31 provinces of China over the period from 2011 to 2020. Geographically, these regions are further divided into the Eastern region, Central region, Western region, and Northeast region to distinguish regional differences.
The explanatory variables in this study are the digital inclusive financial index and three dimensions which are, namely, coverage breadth, depth of use, and degree of digitization. In this study, data will represent these three dimensions. Here, the proxy used for Digital Inclusive Finance is the Digital Inclusive Finance Index as published by Peking University’s Digital Finance Research Centre, which measures nine aspects of digital inclusive finance: coverage, depth of use, payments, insurance, money funds, investment, credit, credit research, and digitalization. This index is the most suitable representative indicator of the extent of digital inclusive finance development specifically in the case of China. Data from 31 Chinese provinces are taken for analysis. Due to the unavailability of the data, three regions of China named Macau, Hong Kong, and Taiwan are excluded from the sample. The examined digital inclusive finance index has been developed by employing three components that are: breadth of coverage (BRC), degree of digitalization (DDIG), and depth of use (DUSE) (Figure 2). In this study, all variables are used in logarithmic form to ensure the accuracy of the results.
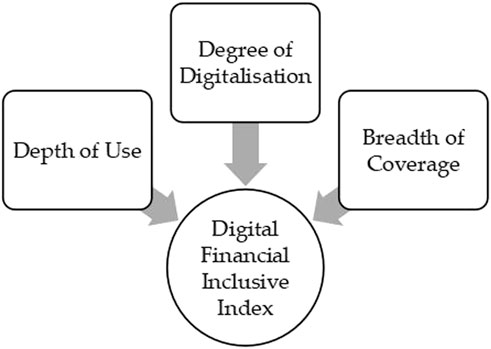
FIGURE 2. Digital Inclusion Index and its Dimensions. Source: Published by the Peking University Institute of Digital Finance.
The current statistics only disclose a rough age structure, so we cannot control other forms of human capital such as agency work experience. The health status of the labor force cannot be characterized due to the lack of data, so these variables cannot be controlled in the empirical test. Although omitting them may lead to biased estimates and unreliable results, the higher the level of education of the labor force, the more conducive it is to obtain other forms of human capital, such as on-the-job training, learning by doing, and other knowledge beyond formal education (Topel, 1999). In this sense, the variable of average education also captures some other unobservable human capital to some extent in the model.
Theoretically, the development of education is a variable that is very relevant to the level of economic development. At the same time, there is a very strong relationship between education and economic development. Therefore, referring to the practices of other literatures, we considered the average number of years of education in rural areas as the explanatory variable. It is more in line with the increasing trend of education and economic development in different regions of China (Zhao et al., 2004; Yao and zhanghai, 2008; yongmin, 2014). The data of this variable is collected through the statistical yearbook of China’s population and employment and then obtained through the calculation method as given in the below table. The accumulation of human capital in rural areas (expressed as RHMC) refers mainly to the long-term accumulation of education. Therefore, this study measures the level of human capital accumulation in rural areas of China in terms of the average number of years of education in selected areas. In the specific measurement: illiterate or seldom literate, primary school, junior high school, senior high school, university, or post-secondary education are expressed as 3, 6, 9, 12, and 16, respectively. Rural human capital accumulation is multiplied by RHMC, taking into account the reality, the years of education for illiterate or seldom literate people are expressed as the median value of three in the primary school system, and post-secondary education includes undergraduate, master’s and doctoral degrees, so the 15 years of post-secondary education is added by 1 year to the weighting and calculate it as 16 years of education. The data is collected from the statistical yearbook of China’s population and employment.
In the aforedisplayed table, the calculation process of rural human capital accumulation has been described (Table 1).
In order to control the impact of other provincial characteristics on rural human capital accumulation, some control variables are used in this study which is also used by various researchers to conduct such types of studies (Gong and Wang, 2021; Pan et al., 2021). These control variables include: Urbanization Rate (URB), Per Capita Disposable Income (LDI), National Financial Expenditure on Education (LFINEX), Inflation Rate (INF-Expressed by Consumer Price Index), and Regional Openness (LDOP). All variables affected by prices have been deflated based on 2010. The data relating to these variables are collected from the National Bureau of Statistics. The calculations of these control variables are given below (Table 2):
Methods
In order to integrate the role of digital inclusive finance in the determination of rural human capital accumulation, the sample is taken from the people who belong to financially disadvantaged rural areas of China. In this era of globalization, technology, and urbanization, a large share of individuals who mainly belong to rural areas remains deprived, unaware, and less facilitated from the services enjoyed by the urban people. Hence, there is a need to introduce methods that may incur the state of the Virtuous Cycle in rural China. The data selected for this study is short panel data, and there is an endogenous problem between the major research variables i.e. digital Inclusive Finance and rural human capital accumulation; Here, Generalized Moment (GMM) estimation is the suitable estimation method that can be used when the dataset pertains to the short panel and has the issue of endogeneity. It mainly includes system moment estimation and difference moment estimation. The system moment estimation has the characteristics of more stringent requirements and more accurate results (Su et al., 2018). Considering these factors, this study uses the System GMM method to study the model. For checking the accuracy of statistical results, robustness regression is examined. Principally, this study has been constructed on the following econometric model:
The prime focus of this model is on the affiliation between DFI and Rural Human Capital (RHMC) accumulation. The examined digital inclusive finance index has been constructed by utilizing three major components that are BRC, DDIG, and DUSE. Hence, it becomes necessary to integrate the influence of these components separately.
In the second model of this study, the researcher examined the LBRC effect on RHMC. See the second model below;
Yet, there is still a need to evaluate the influence of some control variables that are empirically acquainted as an influencing factor for rural human capital and digital inclusive finance. In this regard, INF, LFINEX, URB, LDI, and DOP have been employed.
In the third model of this study, the researcher examined the LDDIG effect on RHMC.
Furthermore, in the fourth econometric model, the researcher considered the effect of LDDIG on RHMC. The fourth model of this research is as follows;
The terms abbreviated in the above section replicates;
RHMC = rural human capital accumulation.
LDFI = Log of digital inclusive finance index.
LBRC = Log of breadth of coverage.
LDDIG = Log of degree of digitalization.
LDUSE = Log of depth of use.
INF = Inflation.
LFINEX = Log of state financial expenditures on education.
URB = Urbanization.
LDI = Log of disposable income.
LDOP = Log of degree of regional openness
ε = Error term.
In addition, β0 reflects constant or intercept term. However, β1 β2, β3, β4, β5 β6, β7, and β8 indicate coefficients which are examined in the next section. In addition, “t” imitates the time period from 2011–2020, and the “i” term exhibits examined 31 provinces.
Results
In order to represent huge data series in an organized, well-displayed, summarized, and arranged manner, Descriptive statistics are usually employed by the researchers. With this approach, the salient features of the considered data set are highlighted. Mainly, Descriptive statistics estimation contains average mean and median tendencies, maximum and minimum values, observation number, standard deviation, and the sum value (Fisher and Marshall, 2009). In this study, Eviews-9 is used for statistical estimation and results from the dataset.
For better understanding see the following Table 3 calculations;
In the afore-exhibited table, descriptive statistics for selected variables are displayed. It is shown in the subsequent table that the mean and median values of inflation are the highest which is approximately 102 whereas the lowest values are of urbanization. Overall, the maximum value of the whole dataset is 106 which is of inflation while the minimum value of the complete data series is 0.0075 which is of DOP. Furthermore, the highest standard deviation in the data is of INF. Particularly, the dataset of all variables are normally distributed. Though, RHMC, LDFI, LBRC, LDDIG, and LDUSE are negatively skewed while INF, URB, DOP, and DI are positively skewed. Additionally, all variables of this study are leptokurtic. In the last column, the total number of observations are displayed which is 310.
In the next step of data estimation, the researcher considered the correlation matrix. Mainly, the correlation matrix shows the inter-relationship between two factors. It is important to determine whether the examined variables are correlated with each other or not. Hence, in the following section with the help of the correlation matrix, not only the correlation between examined factors is presented but also its nature (either positive or negative) of association (Steiger, 1980). In the following table correlation matrix is presented;
In the subsequent table, the correlation between dependent and independent variables of this research is demonstrated. The correlation matrix shows the correlation between variables which range from 0 to 1. Here, RHMC, LFINEX, URB, and LDI have confirmed positive association with all variables except in the case of INF which is negative. DOP has a positive correlation with all variables of this study. While INF has negative correlation with all variables of this study except for DOP which is positive. Furthermore, LDFI, LBRC, LDDIG, and LDUSE have a positive correlation with all variables except for the case of INF which has a negative correlation.
After the correlation matrix, it is to show the cause and effect association between the examined variables of this research. Granger causality test shows either the association between examined two factors is linear or binominal. In simple terms, it shows either “x” is causing “y” or “y” is causing “x” or not. If only “x” is causing “y” and “y” is not causing “x” or vice versa then it is a linear relationship. However, if “x” is causing “y” and also “y” is causing “x” then it is a binominal relationship. Here, to estimate the cause and effect or bidirectional relationship, the Granger causality test is applied using Eviews-10. For better understanding see the following displayed table;
In the Table 4 explained, cause and effect association between selected variables is exhibited. In the case of INF, the null hypothesis is rejected which is to infer that INF does not cause RHMC, and also RHMC does not cause INF. However, LFINEX does not cause RHMC, but RHMC causes LFINEX. Hence, there exists a linear relationship between these two variables. In addition, URB does cause RHMC, but RHMC does not cause an effect on URB. Nonetheless, LDI does cause the RHMC, but in turn, RHMC does not cause LDI. In addition, DOP does not granger causes RHMC, but RHMC does cause an effect on DOP. Although LDDIG is estimated to have a two-way causality, it can be inferred that LDDIG does cause RHMC, and RHMC also causes an effect on LDDIG. Furthermore, LDFI is estimated to have a binominal association with RHMC. However, LDUSE has a linear association as it has an effect on RHMC, but, in turn, RHMC does not cause LDUSE. Similarly, LBRC has linear association as it causes an effect on RHMC, but, in turn, RHMC does not cause LBRC.
After developing the cause and effect relationship between research variables, the GMM estimation of the dataset is conducted. In order to verify whether the tool variables and estimated results of the System GMM are valid, this study adopts two test methods as proposed by Arellano and Bover (1990). The first is the second-order sequence correlation test AR (2), whose main function is to test whether the residual of the System GMM estimation has sequence correlation. When the two-stage autoregression has no sequence correlation, the estimated result of the equation is valid; second is the over-identification constraint test, which is mainly used to verify whether the instrumental variables used in the System GMM estimation are generally effective. In this empirical study, the Sargan test is used for identification. In the following statistical estimates obtained from GMM/dynamic panel modeling, estimated results of all four models of the study are presented.
The estimated results of the systematic GMM are given in Table 5. Models I to IV consider the impact of the total digital inclusion index, the breadth of digital inclusion coverage, the depth of digital inclusion use, and the digitization of digital inclusion on rural human capital accumulation, respectively. As is given in Table 5, the overall regression results of the model are good with the total digital inclusive finance index, the breadth of digital inclusive finance coverage, the depth of digital inclusive finance usage, and the degree of digital inclusive finance digitization. All of the variables have a significant positive contribution to human capital accumulation. A one-period lag in rural human capital accumulation also has a significant positive impact on human capital accumulation in the current period. Among the control variables, the degree of regional openness has a positive effect on rural human capital accumulation. The higher the degree of regional openness, the more comprehensive the access to various capital elements in rural areas, which promotes rural residents’ investment in education, which is consistent with reality.
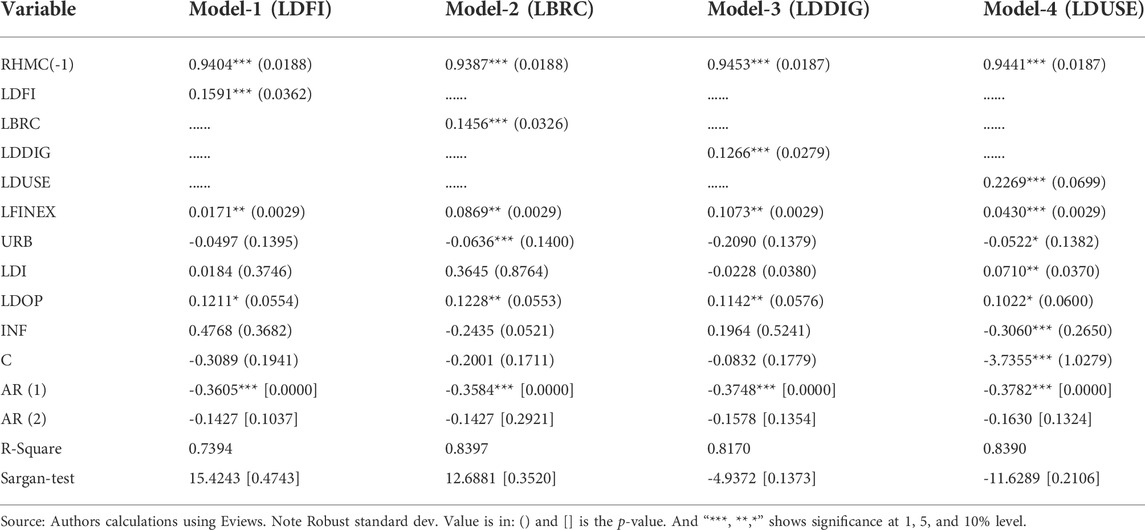
TABLE 5. Results of systematic GMM estimation of the impact of digital inclusion finance on rural human capital accumulation.
The results of the autocorrelation tests of the four models show that the first-order perturbation terms are autocorrelated, but none of the second-order perturbation terms are autocorrelated. At the same time, the p-values of the Sargan test for each model are not significant, indicating that the instrumental variables of the models are selected nationally.
Further analysis of the above results may have the following reasons. First of all, the development of digital inclusive finance in rural areas of China can improve the availability and access of financial services to these people. Through advanced digital technology, digital inclusive finance breaks the limitations of traditional financial services in space and time, so that more farmers have the opportunity to enjoy rural financial services, alleviates the budget constraints caused by lack of funds for education investment, and promotes the accumulation of rural human capital. Secondly, the interest rate of digital inclusive finance is more favorable than that of traditional inclusive finance, which means that digital inclusive finance can reduce the cost of capital use, attract farmers with a high level of human capital to return to China for entrepreneurship, and improve the level of rural human capital through spill-over effect. In addition, the development of digital inclusive finance can make farmers easy access to funds, bonds, stocks, investment opportunities, and core financial services, facilitate farmers’ financial management, maintain or increase wealth, and increase farmers’ willingness and ability to invest in upgrading the skill and education of human capital. Therefore, it can be concluded that DFI has a positive effect on rural human capital accumulation (Wang, He and Le, 2022) (Wang et al., 2022). This positive relationship becomes more significant and progressive with the development and application of digital inclusive finance in these areas. Based on this, Ho1 is valid which is that digital inclusive finance can promote the accumulation of rural human capital.
Here, LDFI has confirmed a 15% change in rural human capital accumulation in rural China. But it does not show which component of the DFI index contributes the most. Hence, the second, third, and fourth models of this table highlight role of each complement separately. LBRC causes a 14% variation in RHMC as shown in the above table. Furthermore, LDDIG causes a 12% change in the accumulation of rural human capital. Model-4 shows that the depth of use accumulates around 22% of human capital in rural China. Therefore, on the basis of these statistics, it can be concluded that depth of use contributes largely to human capital accumulation in rural China. He degree of digitalization is the second utmost provoking factor of RHMC.
LFINEX in all four models gives statistically significant results, also its influence on RHMC is positive. However, URB in the second and fourth models gives statistically significant results while with model-1 and model-3 are insignificant. The influence of URB on RHMC is negative. LDOP gives affirmative and statistically significant results in all four models. On the contrary, INF has caused a negative influence on RHMC. In sum, statistical estimates of all four models as given in Table 5, shows that LDFI is significantly determined by human capital accumulation in rural China.
Though, the Sargan test estimation confirmed that the selection of instrumental variables is reasonable as the p-value is insignificant. Based on the estimation as given in Table 5, Ho1 cannot be rejected and it is inferred that digital inclusive finance significantly contributes to rural human capital accumulation and can alleviate financial inclusion in rural areas. In addition, Ho2, Ho3, and Ho4 cannot be rejected which shows that breadth of coverage, degree of digitalization, and depth of usage significantly contribute to rural human capital accumulation in the case of China. The estimation proved that urbanization has a negative impact on the rural human capital accumulation. Additionally, inflation has a positive effect on rural human capital accumulation. Therefore, it can be concluded that inflation has a negative impact on the RHMC in rural China.
In Table 6, the long-run relationship between the digital inclusive finance index and the rural human capital is presented by employing Robustness Regression Analysis. The central objective of this analysis is just to confirm the accuracy of statistical estimates obtained in Table 5 from GMM analysis. With the Robustness checking, it is confirmed that the statistical results obtained in Table 5 are much similar to that of Table 6, which shows that examined models and results are accurate and statistically stable. Here, LDFI causes an effect of around 17% on RHMC which is higher than Table 5 (which is 15%). Also, LBRC reflects an 18% variation in RHMC which is 14% according to Table 7 estimates. On the contrary, LDDIG and LDUSE showed 9 and 26% cointegration respectively with RHMC according to robustness checking. It is also estimated that all control variables have a statistically significant influence on RHMC.
Exploring the impact of digital Inclusive Finance on rural human capital accumulation in different regions will help the government formulate targeted strategies, so as to enhance the effect of digital Inclusive Finance on rural human capital. Due to China’s vast territory, there are significant differences in the level of economic development in different regions. In order to specifically analyze the heterogeneous impact of digital Inclusive Finance on the accumulation of rural human capital in different regions, this study divides the samples into four regions, namely, the East, the middle, the West and the northeast, according to the Economic Zoning of the National Bureau of statistics, and uses systematic GMM to conduct regression respectively.
It can be seen from Table 8 that the impact of digital Inclusive Finance on rural human capital accumulation in different regions is heterogeneous. From the estimation coefficient of variable LDFI, the impact of digital Inclusive Finance on rural human capital accumulation in Northeast China is greater than that in the other three regions. First of all, from the perspective of Northeast China, Northeast China, as China’s old industrial base, has experienced a trend from development to decline. At present, it is one of the least developed regions. The most significant impact of digital Inclusive Finance on rural human capital accumulation in Northeast China may be that in order to realize the transformation of the old heavy industry base, the state strongly supports the development of Northeast China, whether in terms of infrastructure or talent training. The upgrading and improvement of digital infrastructure have promoted the development of digital Inclusive Finance, as well as the development of Internet employment and entrepreneurship platforms. Benefiting from the national strategic decision to revitalize the northeast, the northeast region has seized the opportunity to pay attention to and attract talents. At this time, the development of digital Inclusive Finance is conducive to better education investment. At the same time, convenient credit services, preferential interest rates, and wealth management are conducive to attracting high-level human capital workers to settle down or return home, promoting the rapid improvement of human capital.
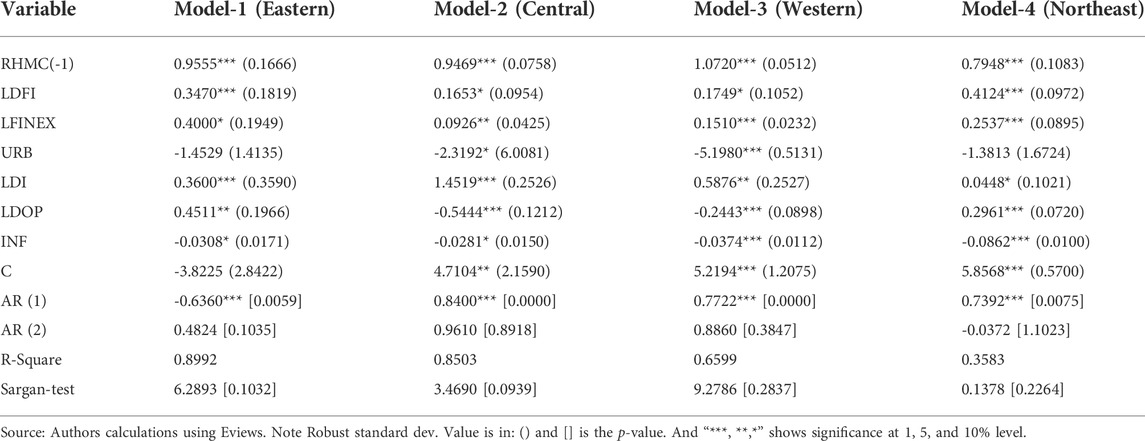
TABLE 8. Results of systematic GMM estimation of the Total Digital Inclusion Index on rural human capital accumulation in different regions.
Secondly, the role of digital Inclusive Finance in promoting the accumulation of rural human capital in the eastern region is second only to that in the northeast region and significantly higher than that in the central and western regions. As the most developed and economically dynamic region in China, the eastern region is at the forefront of the country in terms of infrastructure construction and digital level. The promotion of digital Inclusive Finance has inherent advantages, and farmers are more likely to have access to information products. At this time, digital inclusive finance can ease credit and budget constraints, increase farmers’ willingness and ability to invest in education, and promote the accumulation and improvement of human capital. In addition, digital Inclusive Finance also has a significant impact on the accumulation of rural human capital in the central and western regions, but the impact is smaller than that in the other two regions, which may be subject to farmers’ financial literacy and digital infrastructure. In the future, we should focus on the two regions to avoid the “digital divide” and increase the development gap between regions.
In the end, it can be concluded that LDFI contributes greatly to the accumulation of human capital in rural China. From Table 8, it can be inferred that because of LFINEX, there are 40, 09, 15, and 25% changes in RHMC of Eastern, Central, Western, and Northeast region of China, respectively. High financial expenses of a state on education will cause a better and improved educational environment which, in turn, results in the form of enhanced human capital (Ahmad et al., 2021b).
In contrast, URB signified negative and statistically significant cointegration with RHMC in Central and Western regions. A high or increased level of urbanization will lower the level of human capital accumulation in rural areas of China. That is why its affiliation with RHMC is negative. In the case of the Eastern and Northeast regions, URB is a negative but insignificant affiliation with RHMC. The reason behind this impact is the increase in the urbanization in these regions because of the lack of opportunities; as a result, Eastern and Northeast regions are facing challenges in RHMC. Additionally, LDI causes cause 36, 45, 58, and 4% changes in RHMC in Eastern, Central, Western, and Northeast rural areas of China, respectively.
An increase in disposable income of rural people shows that a big share of income will be spent on education which, in turn, provokes high RHMC (Wang and Guan, 2017; Zhao et al., 2022). Except for Eastern and Northeast regions, LDOP has a negative impact on RHMC in the case of Central and Western regions (54 and 24% respectively). In the Eastern and Northeast regions, LDOP has a positive relationship which is 45 and 29% respectively. This means that the increase in LDOP is increasing RHMC in these regions. In the case of INF, it is shown that there is negative cointegration with RHMC. It is estimated that the increase in INF is reducing the RHMC by 3, 2, 3, and 8% in Eastern, Central, Western, and Northeast regions respectively (Heylen and Pozzi, 2007). Overall, the creditability and accuracy of this table are grounded accordingly and the overall model is statistically stable and significant.
The autocorrelation test results in all four models show that AR (1) is significant to varying degrees, but AR (2) is always not significant. The first-order disturbance term has autocorrelation, but the second-order disturbance term has no autocorrelation, indicating that the random terms of all models do not have autocorrelation. The estimation results are accurate and reliable.
According to the calculation in Table 8, HO1 cannot be rejected, that is, digital inclusive finance reduces financial exclusion and significantly accumulates rural human capital accumulation. In addition, with the presence of regional heterogeneity, Ho2, Ho3, and Ho4 cannot be rejected which state that breadth of coverage, degree of digitalization, and depth of usage significantly contribute to rural human capital accumulation in the case of China.
In order to test the effectiveness of model 1 in Table 5, we use the three components of LDFI to test the accumulation of rural human capital respectively. Table 9 shows the estimated results of LBRC. Here, LBRC confirmed the 57% change in rural human capital accumulation in Northeast China. At the same time, the accumulation of rural human capital in the eastern region has changed by about 35%, which is also quite remarkable. However, the regression results show that the changes in rural human capital accumulation caused by LBRC in the central and western regions are relatively small, which is similar to the results of the total index of digital Inclusive Finance in Table 8.
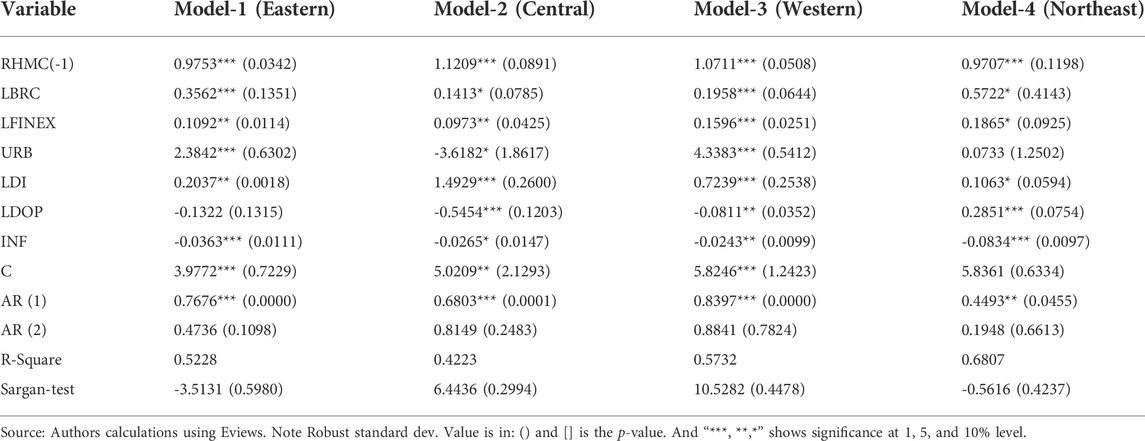
TABLE 9. Results of systematic GMM estimation of the effect of coverage breadth on rural human capital accumulation in different regions.
The autocorrelation test results in all four models show that AR (1) is significant to varying degrees, but AR (2) is always not significant. The first-order disturbance term has autocorrelation, but the second-order disturbance term has no autocorrelation, indicating that the random terms of all models do not have autocorrelation. The estimation results are accurate and reliable.
Through Sargan test estimation, it is estimated that selection of instrumental variables is rational. Based on the calculation of Table 8, Ho2 cannot be rejected which states that LBRC is positively linked with rural human capital accumulation (Ahmad et al., 2021b). It is also revealed that LBRC will cause a much stronger effect in the utmost backward region of China (Northeast region). This may be because of the reason that coverage refers to the account coverage. With the implementation of the Revitalization Strategy in Northeast China, the strengthening of digital infrastructure has provided farmers with more opportunities to access digital financial services. The direct manifestation is the increase of registered users. However, the Eastern region itself has a higher degree of development. Therefore, the growth rate is not as fast as that observed in Northeast China. More accounts means that people have more opportunities to improve their human capital. Therefore, the coverage of digital inclusive finance has improved the accumulation of rural human capital, specifically, in the case of rural areas of China.
In the above table, the systematic GMM Estimation Test between LDDIG and RHMC was conducted in rural areas of China. At the same time, LFINEX, URB, LDI, LDOP, and LINF are also used as control variables for all four regions. Here, rural human capital (RHMC) is a dependent variable. Here, LDDIG has confirmed that there is a 23% difference in the accumulation of rural human capital in Northeast China, while the accumulation of rural human capital in Western China. At the same time, in the eastern and central regions, the changes in rural human capital accumulation are about 15 and 14% respectively. The changes in relatively developed regions are smaller than those in relatively backward regions, which fully confirms the characteristics that digital Inclusive Finance has a more obvious effect on vulnerable groups and economically underdeveloped regions.
The autocorrelation test results in all four models show that AR (1) is significant to varying degrees, but AR (2) is always not significant. The first-order disturbance term has autocorrelation, but the second-order disturbance term has no autocorrelation, indicating that the random terms of all models do not have autocorrelation. Meanwhile, the Sargan test also proved that the selection of tool variables was reasonable. The estimation results are accurate and reliable.
The digitalization degree of digital Inclusive Finance includes four aspects: mobility, affordability, credit, and convenience. From the changes in the four regions, it can be found that the change range is about 14–23%, and the change range is small. Although the digitalization degree has a significant positive impact on the changes in rural human capital accumulation in various regions of China, its impact is relatively balanced. The northeast and western regions of China, which belong to the less developed regions of China, have a relatively large range of changes. Considering the profitability and other issues, the coverage rate of traditional financial institutions is relatively low compared with that in developed regions. At this time, digital inclusive finance can make up for the vacancy of traditional financial services and promote the improvement of rural human capital in underdeveloped regions through mobility, affordability, credit, and convenience. Therefore, according to the calculation in Table 10, we cannot reject the original assumption H03, that is, the digitalization degree of digital inclusive finance can promote the accumulation of rural human capital (Wang and Guan, 2017; Zhao et al., 2022).
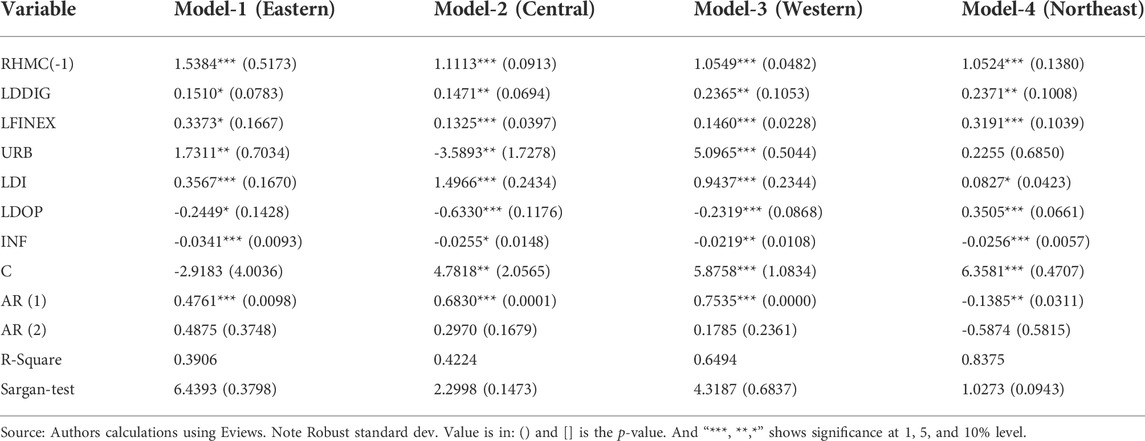
TABLE 10. Results of systematic GMM estimation of the effect of depth of use on rural human capital accumulation in different regions.
In Table 11, LDUSE and RHMC conducted systematic GMM Estimation tests in rural areas of China. At the same time, LFINEX, URB, LDI, LDOP, and LINF are also used as control variables for all four areas. Among the four regions, the estimated coefficient of LDUSE is significantly positive. In terms of the estimated regression coefficient of variable LDUSE, the large changes are in the Northeast (44.6%), the eastern region (42.1%), the western region (41.4%), and the central region only have a difference of 22.3%. The empirical results show that the depth of the use of digital inclusive finance can promote the accumulation of rural human capital in different regions, but there is regional heterogeneity.
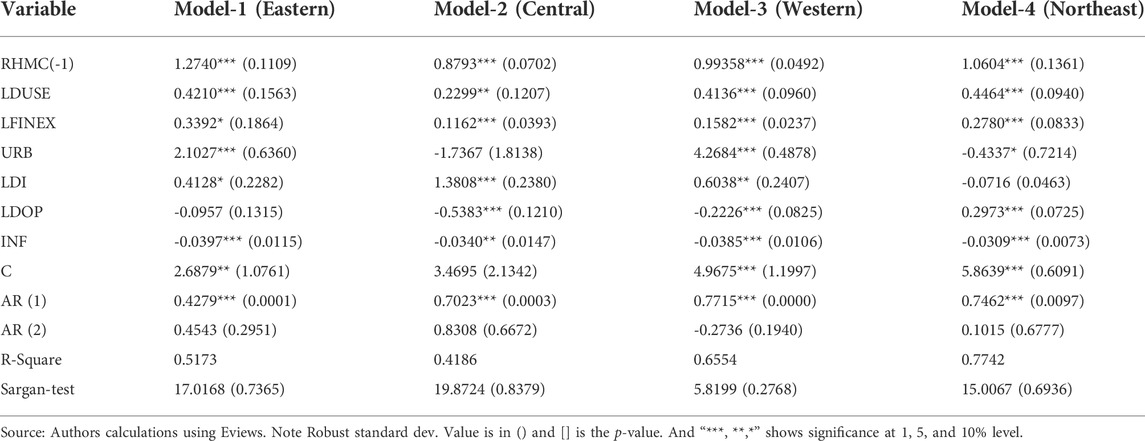
TABLE 11. Results of systematic GMM estimation of the impact of digitization on rural human capital accumulation in different regions.
For a long time, the overall development of financial services in rural areas has been inferior to that in urban areas, and the status of farmers’ assets cannot reach the service threshold of most traditional financial institutions. Therefore, all aspects of development in rural areas are vulnerable to restrictions, especially the development of education needs a lot of investment (Tinghui Li, 2021) (Li and Ma, 2021). The emergence of digital Inclusive Finance has well solved this problem. It is worth emphasizing that the depth of the use of digital Inclusive Finance includes four aspects: credit business, insurance business, investment business, and credit business. In both underdeveloped and relatively developed regions, farmers can obtain funds through the credit business of digital Inclusive Finance to ease credit constraints, especially when education investment is constrained by the budget, This has greatly enhanced the accumulation of rural human capital. In addition, insurance businesses and investment businesses have realized the preservation and appreciation of assets, and improved farmers’ willingness and ability to participate in education investment.
The autocorrelation test results of the four models show that AR (1) has different degrees of significance, but AR (2) is always not significant. The first-order perturbation term has autocorrelation, while the second-order perturbation term has no autocorrelation, indicating that the random terms of all models have no autocorrelation. At the same time, the Sargan test also proved that the choice of tool variables is reasonable. It can be concluded that the estimation results are accurate and reliable. Based on the calculation of Table 11, Ho4 can be rejected which replicates that the LDUSE does influence the rural human capital accumulation.
Conclusion and policy implications
Based on the panel data of 31 provinces in the Chinese Mainland from 2011 to 2020, the study empirically tested the impact of digital Inclusive Finance on rural human capital accumulation and its regional heterogeneity by using the systematic GMM method. At the same time, it also used the three dimensions contained in digital Inclusive Finance to analyze respectively, so as to more accurately understand which dimension can promote rural human capital accumulation, so as to formulate targeted strategies.
Digital inclusive finance can significantly promote the accumulation of rural human capital. The higher the index of digital Inclusive Finance, the more conducive it is to promote the accumulation of rural human capital. However, there are different degrees of influence in different regions. The study found that: from the national perspective, both the total index of digital Inclusive Finance and its three dimensions, namely, coverage, digitalization, and depth of use, have significantly promoted the accumulation of rural human capital, and the depth of use of digital Inclusive Finance has the most obvious impact on the accumulation of rural human capital.
From the perspective of regional differences, digital Inclusive Finance has different effects on the accumulation of rural human capital in different regions. First of all, digital inclusive finance plays an important role in the accumulation of rural human capital in the northeast, but it is relatively less obvious in the central and western regions. This indicates that there is regional heterogeneity in the effect of digital Inclusive Finance on rural human capital accumulation. Secondly, from the perspective of the segmentation dimension, the coverage of digital Inclusive Finance is the prerequisite, and the depth of use is considered to be the closest to the actual use. These two dimensions reflect the popularity of digital Inclusive Finance. The degree of digitalization can be regarded as a potential condition, which reflects the benefits of digital Inclusive Finance. The impact of the coverage and depth of digital Inclusive Finance on the accumulation of rural human capital is the most important in the northeast, followed by the eastern region, while the impact on the central region is relatively weak. The impact of digitalization on the accumulation of rural human capital is the highest in Northeast China, followed by Western China, and the weakest in Central China.
Based on the above research conclusions, this study puts forward the following four enlightenments and suggestions: First, we will continue to promote the development of rural digital Inclusive Finance, focus on relatively underdeveloped regions and central China, and strengthen the construction of rural Internet and other infrastructure, lay a good foundation for the development of rural digital Inclusive Finance in China, solve the development imbalance caused by backward geographical infrastructure, avoid the “digital divide”, and allow more rural residents to access digital inclusive financial services, To promote the accumulation of rural human capital.
Second, innovate and develop digital inclusive financial products and services with different focuses in different regions, so as to provide richer choices for the diversified needs of rural residents. For example, develop a fully upgraded digital inclusive financial promotion strategy for the central region, and provide more targeted insurance, investment, and financial products, and credit services for the western region, so as to enhance the accumulation of rural human capital. Through preferential credit interest rates and green channels, farmers with a high level of human capital will be attracted to return home for entrepreneurship and employment, so as to realize a virtuous cycle of rural human capital accumulation through the spillover effect.
Third, the digitalization degree of digital Inclusive Finance is the aspect that has the smallest impact on the accumulation of rural human capital among the three dimensions. The digitalization degree reflects the potential use of digital Inclusive Finance. Therefore, it can improve the financial literacy of rural residents, increase the dissemination of knowledge related to digital Inclusive Finance, expand publicity and education, cultivate farmers’ ability to identify risks, promote the acceptance of new investment methods and investment ideas, enhance the willingness and ability to invest in education, and promote the accumulation of rural human capital.
Although this study has made considerable efforts in analyzing the impact of DFI on rural human capital accumulation. This study also has some limitations. Firstly, the DFI data is for the period 2011 to 2020, and the short-term data has some limitations. Secondly, the findings are limited to one country (China), which is a country with a well-developed digital economy, and therefore cannot be generalized to all developing countries. Thirdly, the Digital Inclusion Index is measured by the Digital Finance Centre of Peking University, and although hundreds of millions of micro-data were used to measure it. It does not fully cover the state of digital inclusion development in China. However, it can be extended in several ways in the future. Firstly, data from cities under provincial breakdowns could be used to validate and analyze the detailed development situation. Secondly, other proxies for digital inclusive finance could be used to validate the results of this examination. Thirdly, a comparative analysis of relevant areas in other countries should be conducted to summarise strengths and weaknesses and better provide lessons learned. Finally, the specific processes by which digital inclusive finance affects rural human capital accumulation could be further considered, and this is the next stage of the authors’ research.
Data availability statement
The original contributions presented in the study are included in the article/Supplementary Material, further inquiries can be directed to the corresponding author.
Author contributions
HL was responsible for the creation of the overall idea and the writing of the article. RZ is responsible for collecting data on indicators related to digital inclusive finance and making an analysis. JH was responsible for the construction of the total factor productivity indicator system for agriculture as well as the collection and processing of raw data and the calculation of the results. PZ was responsible for the analysis using Eviews software to produce the results. All authors have read and agreed to the published version of the manuscript. MG updated the measurement model in the manuscript according to the reviewers' suggestions, it helped us modify the literature review and empirical result analysis, and finally, checked the grammar of our revised manuscript.
Funding
This study is sponsored by the National Social Science Foundation Project of China (CN) (Grant number 20BJY118), and the Natural Science Foundation of Shandong Province (Grant number ZR2020MG045).
Conflict of interest
The authors declare that the research was conducted in the absence of any commercial or financial relationships that could be construed as a potential conflict of interest.
Publisher’s note
All claims expressed in this article are solely those of the authors and do not necessarily represent those of their affiliated organizations, or those of the publisher, the editors, and the reviewers. Any product that may be evaluated in this article, or claim that may be made by its manufacturer, is not guaranteed or endorsed by the publisher.
Supplementary material
The Supplementary Material for this article can be found online at: https://www.frontiersin.org/articles/10.3389/fenvs.2022.936648/full#supplementary-material
References
Ahmad, M., Majeed, A., Khan, M. A., Sohaib, M., and Shehzad, K. (2021). Digital financial inclusion and economic growth: Provincial data analysis of China. China Econ. J. 14, 291–310. doi:10.1080/17538963.2021.1882064
Ahmad, M., Majeed, A., Khan, M. A., Sohaib, M., and Shehzad, K. (2021). Digital financial inclusion and economic growth: Provincial data analysis of China. China Econ. J. 14 (3), 291–310. doi:10.1080/17538963.2021.1882064
Arellano, M., and Bover, O. (1990). Another look at the instrumental variable estimation of error-components models. J. Econom. 68 (1), 29–51. doi:10.1016/0304-4076(94)01642-d
Arora, R. U., and Ratnasiri, S. (2011). Financial development, financial inclusion and human capital: How close is the link? A study of India. Indian economy update. Canberra: Australian National University.
Aziz, A., and Naima, U. (2021). Rethinking digital financial inclusion: Evidence from Bangladesh. Technol. Soc. 64, 101509. doi:10.1016/j.techsoc.2020.101509
Becker, G. S. (2009). Human capital: A theoretical and empirical analysis, with special reference to education. University of Chicago press.
Bertola, G., and Sestito, P. (2011). A comparative perspective on Italy’s human capital accumulation. Italy: SSRN Electronic Journal. doi:10.2139/ssrn.2233837
Cinnirella, F., and Streb, J. (2017). The role of human capital and innovation in economic development: Evidence from post-malthusian prussia. J. Econ. GrowthBost. 22 (2), 193–227. doi:10.1007/s10887-017-9141-3
Dalton, T. J., Lilja, N., Johnson, N. L., and Howeler, R. H. (2005). Human capital accumulation and productivity improvements in asian cassava systems: Are participatory research approaches beneficial?
Fisher, M. J., and Marshall, A. P. (2009). Understanding descriptive statistics. Aust. Crit. care 22 (2), 93–97. doi:10.1016/j.aucc.2008.11.003
Ganeva, R. S. (2010). Human capital in economic growth: A review of theory and empirics. Econ. Thought 7 (1), 131–149.
Gomber, P., Koch, J.-A., and Siering, M. (2017). Digital finance and FinTech: Current research and future research directions. J. Bus. Econ. 87 (5), 537–580. doi:10.1007/s11573-017-0852-x
Gong, M. G., and Wang, R. (2021). Research on the impact of rural land property right system reform on rural human capital accumulation -- an empirical analysis based on panel data. CMP method Bus. Res. (02), 65–73. doi:10.13902/j.cnki.syyj.2021.02.008
Grigorescu, A., Pelinescu, E., Ion, A. E., and Dutcas, M. F. (2021). Human capital in digital economy: An empirical analysis of central and eastern European countries from the European Union. Sustainability 13 (4), 2020. doi:10.3390/su13042020
Henderson, D. J., and Russell, R. R. (2005). Human capital and convergence: A production‐frontier approach. Int. Econ. Rev. Phila. 46 (4), 1167–1205. doi:10.1111/j.1468-2354.2005.00364.x
Heylen, F., and Pozzi, L. (2007). Crises and human capital accumulation. Can. J. Economics/Revue Can. d'economique. 40 (4), 1261–1285. doi:10.1111/j.1365-2966.2007.00451.x
Hong Vo, D., Tran, N. P., and Nguyen, H. M. (2021). Does financial development improve human capital accumulation in the Southeast Asian countries? Cogent Bus. Manag. 8 (1), 1932245. doi:10.1080/23311975.2021.1932245
Jorgenson, D. W., and Pachon, A. (1983). “The accumulation of human and non-human capital,” in The determinants of national saving and wealth (Springer), 302–350.
Li, J., and Li, B. (2022). Digital inclusive finance and urban innovation: Evidence from China. Rev. Dev. Econ. 26 (2), 1010–1034. doi:10.1111/rode.12846
Li, T., and Ma, J. (2021). Does digital finance benefit the income of rural residents? A case study on China. Quantitative Finance Econ. 5 (4), 664–688. doi:10.3934/QFE.2021030
Liu, G., Huang, Y., and Huang, Z. (2021). Determinants and mechanisms of digital financial inclusion development: Based on urban-rural differences. Agronomy 11 (9), 1833. doi:10.3390/agronomy11091833
Liu, Y., Liu, C., and Zhou, M. (2021). Does digital inclusive finance promote agricultural production for rural households in China? Research based on the Chinese family database (CFD). China Agricul. Econ. Rev. online ahead of print. doi:10.1108/CAER-06-2020-0141
Lucas, R. E. (2015). Human capital and growth. Am. Econ. Rev. 105 (5), 85–88. doi:10.1257/aer.p20151065
Munjal, S., and Kundu, S. (2017). “Exploring the connection between human capital and innovation in the globalising world,” in Human capital and innovation (Springer), 1–11.
Naumenkova, S., Mishchenko, S., and Dorofeiev, D. (2019). Digital financial inclusion: Evidence from Ukraine. Invest. Manag. Financial Innovations 16 (3), 194–205. doi:10.21511/imfi.16(3).2019.18
Ozili, P. K. (2021). Financial inclusion research around the world: A review. Forum Soc. Econ. 50 (4), 457–479. doi:10.1080/07360932.2020.1715238
Ozili, P. K. (2018). Impact of digital finance on financial inclusion and stability. Borsa Istanb. Rev. 18 (4), 329–340. doi:10.1016/j.bir.2017.12.003
Pan, Y., Ma, L., and Wang, Y. (2021). How and what kind of cities benefit from the development of digital inclusive finance? Evidence from the upgrading of export in Chinese cities. Croatia: Economic Research-Ekonomska Istraživanja, 1–29. doi:10.1080/1331677X.2021.2007414
Pelinescu, E. (2015). The impact of human capital on economic growth. Procedia Econ. Finance 22, 184–190. doi:10.1016/s2212-5671(15)00258-0
Ployhart, R. E., Nyberg, A. J., Reilly, G., and Maltarich, M. A. (2014). Human capital is dead; long live human capital resources. J. Manag. 40 (2), 371–398. doi:10.1177/0149206313512152
Prasetyo, P. E. (2020). Human capital as the main determinant of regional economic growth. Int. J. Adv. Sci. Technol. 29 (03), 6261–6267.
Prasetyo, P. E. (2019). The reliability of entrepreneurial productivity as driver of economic growth and employment. Int. J. Entrepreneursh. 23 (4), 1–15.
Prasetyo, P. E. (2019). The reliability of entrepreneurial productivity as driver of economic growth and employment. Int. J. Entrepreneursh. 23 (4), 1–15.
Prskawetz, A., Bloom, D. E., and Lutz, W. (2008). Book review of population aging, human capital accumulation, and productivity growth.. United States: Population and Development Review, 34. doi:10.25336/P6B62X
Shehzad, K., Zaman, U., Jose, A. E., Kocak, E., and Ferreira, P. (2021). An officious impact of financial innovations and ICT on economic evolution in China: Revealing the substantial role of BRI. Sustainability 1316, 8962. doi:10.3390/su13168962
Steiger, J. H. (1980). Tests for comparing elements of a correlation matrix. Psychol. Bull. 87 (2), 245–251. doi:10.1037/0033-2909.87.2.245
Stijns, J.-P. (2006). Natural resource abundance and human capital accumulation. World Dev. 34 (6), 1060–1083. doi:10.1016/j.worlddev.2005.11.005
Su, A., Pab, C., and Gz, D. (2018). Dealing with endogeneity bias: The generalized method of moments (GMM) for panel data. Ind. Mark. Manag. 71, 69–78. doi:10.1016/j.indmarman.2017.11.010
Van Uden, A., Knoben, J., and Vermeulen, P. (2017). Human capital and innovation in sub-saharan countries: A firm-level study. Innovation 19 (2), 103–124. doi:10.1080/14479338.2016.1237303
Wang, W., He, T., and Li, Z. (2022). Digital inclusive finance, economic growth and innovative development. Kybernetes.
Wang, X., and Guan, J. (2017). Financial inclusion: Measurement, spatial effects and influencing factors. Appl. Econ. 49, 1751–1762. doi:10.1080/00036846.2016.1226488
Wright, P. M., and McMahan, G. C. (2011). Exploring human capital: Putting ‘human’back into strategic human resource management. Hum. Resour. Manag. J. 21 (2), 93–104. doi:10.1111/j.1748-8583.2010.00165.x
Yao, X., and zhanghai, F. (2008). Education, human capital and regional economic differences Economic Research, 47–57.05
yongmin, L. (2014). The spatial characteristics of the income increasing effect of rural human capital in China. Manage world 09, 58–76. doi:10.19744/j.cnki.11-1235/f.2014.09.007
Zhao, C., Lu, M., and Jin, Y. (2004). Regional differences in human capital and education development in China: Estimation of panel data world economy, 25–31+77.12
Keywords: digital inclusive finance, China, rural human capital accumulation, inflation, urbanization, disposable income, degree of openness, GMM
Citation: Li H, Zhuge R, Han J, Zhao P and Gong M (2022) Research on the impact of digital inclusive finance on rural human capital accumulation: A case study of China. Front. Environ. Sci. 10:936648. doi: 10.3389/fenvs.2022.936648
Received: 10 May 2022; Accepted: 08 July 2022;
Published: 25 August 2022.
Edited by:
Faik Bilgili, Erciyes University, TurkeyCopyright © 2022 Li, Zhuge, Han, Zhao and Gong. This is an open-access article distributed under the terms of the Creative Commons Attribution License (CC BY). The use, distribution or reproduction in other forums is permitted, provided the original author(s) and the copyright owner(s) are credited and that the original publication in this journal is cited, in accordance with accepted academic practice. No use, distribution or reproduction is permitted which does not comply with these terms.
*Correspondence: Maogang Gong, R09OR01BT0dBTkcxOTgyQG91dGxvb2suY29t