- 1Department of Entomology, Louisiana State University Agricultural Center, Baton Rouge, LA, United States
- 2Molecular, Cellular, and Biomedical Sciences, University of New Hampshire, Durham, NH, United States
Tidal marshes are among the most valuable, productive, and vulnerable ecosystems with high biodiversity. Louisiana’s saltmarshes are endangered by natural and man-made stressors, including oil pollution, saltwater intrusion, and land loss due to sea level rise and erosion. Freshwater diversions have been planned to restore sediment input from the Mississippi River to rebuild marsh habitats in South Louisiana. These proposed diversions will undoubtedly change salinity levels, which is a major controlling factor in the distribution of marsh organisms, including those in soil; however, detailed pre-event inventories are lacking. This study describes the diversity of metazoan meiofauna (organisms between 45 and 500 μm) and environmental DNA in marsh soil collected in 2018 from Barataria and Caillou Bay, Louisiana, across three salinity zones and four distances from the marsh edge. Diversity analyses using 18S rRNA gene metabarcodes identified salinity as a factor impacting soil metazoan composition. Nematoda and Mollusca were equally distributed across salinity zones. Gastrotricha, Bryozoa, Rotifera, and Platyhelminthes were more prevalent in low salinity while Kinorhyncha were not detected in low salinity. Annelida and insects were equally common in low and high salinity but less in mid salinity. Five nematodes (Eumonhystera filiformis, two Prismatolaimus spp., Anoplostoma sp., and Prodorylaimus sp.), two annelids (Marionina southerni and Dendronereis aestuarina), two platyhelminthes (Rhynchoscolex simplex and Olisthanella truncula), the gastrotrich Chaetonotus novenarius and four collembola and ostracods appear to be low salinity bioindicators and are expected to expand range with freshwater diversions. No frequently detected organisms were unique to mid or high salinity zones, but four Nematoda (Meleidogyne spartinae, Prochaetosoma sp., Halalaimus sp., and Dichromadora sp.), two Annelida (Alitta succinea and Namalycastis jaya), two Platyhelminthes (Macrostomum kepneri and Mesorhynchus terminostylis), and one Kinorhyncha (Echinoderes sp.) were never detected in low salinity zones. None of the frequently detected taxa were unique for a particular distance from the marsh edge or bay. This dataset will be useful as baseline for assessing how soil communities will change in response to salinity changes caused by freshwater diversions and saltwater intrusion as well as measuring the environmental impact of pollution and other stressors.
Introduction
Tidal saltmarshes provide a number of ecosystem services which are valuable to humans, including coastal protection from storms, carbon sequestration, and denitrification (Barbier et al., 2011). These habitats also act as nurseries for commercially and recreationally important species (Boesch and Turner, 1984; Zimmerman et al., 2002; Mackenzie and Dionne, 2008). However, salt marshes across the globe are in decline due to a variety of factors (MEA, 2005; UNEP, 2006).
The marshes of Louisiana were initially built by yearly flooding of the Mississippi River over thousands of years (Fisk and McFarlan, 1955) but have lost an area the size of the state of Delaware since 1932 (Couvillion et al., 2017) due to flood control structures preventing these floods and sediment accretion. River sediment compacts readily causing subsidence, which together with sea-level rise leads to land loss and salt water intrusion (Delaune and Pezeshki, 1994; Yuill et al., 2009). In some areas of Louisiana marsh, subsidence may exceed 1 cm per year, which is among the highest rates of subsidence along the Gulf Coast of the United States (Penland and Ramsey, 1990). In addition, marshland is lost due to erosion exacerbated by hurricanes and oil spills (Palaseanu-Lovejoy et al., 2013; Rabalais and Turner, 2016). For example, oiling after the 2010 Deepwater Horizon oil spill of Louisiana’s salt marshes, which are dominated by Spartina alterniflora (Loisel.) and Juncus roemerianus (Scheele), led in some areas to complete loss of vegetation, which resulted in shearing of the marsh edge and accelerated land loss after hurricanes (Lin and Mendelssohn, 2012; Mendelssohn et al., 2012; Silliman et al., 2012; Zengel et al., 2015; Khanna et al., 2017).
A comprehensive Louisiana Coastal Master Plan pledging $50 billion to 79 coastal restoration and protection projects through the next half century has been compiled by the Coastal Resource Management Agency to counteract the alarming rate of loss of Louisiana marshes (Coastal Protection and Restoration Authority of Louisiana, 2007; Coastal Protection and Restoration Authority of Louisiana, 2012; Peyronnin et al., 2013; Coastal Protection and Restoration Authority of Louisiana, 2017). Included in these projects are several sediment and freshwater diversions planned to divert flow from the Mississippi River into the marshes to promote land accretion and reduce saltwater intrusion into historically freshwater marshes (Elsey-Quirk et al., 2019). The first of these major sediment diversions is the Mid-Barataria Sediment Diversion, which was slated to begin construction in 2022 but is now delayed. Environmental impact studies of these diversions will require the establishment of baselines of the composition and distribution of organisms in the marsh. Because salinity is one of the dominant environmental variables in salt marshes (Chapman, 1977; Holland et al., 1990), detailed inventories of different salinity zones are vital to measuring effects of freshwater diversions, saltwater intrusion and other environmental disturbances on the ecology of the marsh community.
Invertebrate organisms are often used for ecological monitoring because they have a high diversity of species, life histories and niches and are responsive to environmental change such as salinity shifts. Therefore, Aker (Aker, 2020) established detailed year-long insect inventories across different salinity zones in Louisiana marshes and identified bioindicator taxa for salinity zones. In addition, sediment-dwelling infauna acts as a linkage between microbe and macrofauna food webs and between benthic and pelagic food webs (Coull, 1999; Posey et al., 2005; Rozas and Minello, 2011; Schratzberger and Ingels, 2018). Meiofauna are infauna typically ranging from 45 to 500 μm (Giere, 2009). The intergenerational time of many groups of meiofauna is quite short, with multiple generations per year, allowing rapid response in both diversity and abundance of major taxa to environmental changes (Warwick, 1981; Kennedy and Jacoby, 1999; Montagna et al., 2002; Cai et al., 2012; Ngo et al., 2013; Zeppilli et al., 2015). Therefore, meiofauna are often used as bioindicators of marsh health, especially with regards to pollution responses (Fleeger et al., 1982; Fleeger and Chandler, 1983; Fleeger, 1985; Fleeger et al., 2007; Fleeger et al., 2015; Semprucci et al., 2015; Bam et al., 2018). However, soil infauna is also likely to be affected by salinity changes. Meiofauna abundance has been shown to increase in salt marshes as salinity increases due to the influx of marine species (Montagna et al., 2002). Salinity was also a major factor impacting benthic infauna across marsh zones from tidal flats to high marsh of the Atlantic coast (MacKenzie et al., 2015; van Regteren et al., 2020).
While meiofauna have been studied in both Gulf and Atlantic coastal marshes, these studies tend to identify meiofauna to major taxonomic groups such as nematodes, harpacticoid copepods, and oligochaetes (Bell, 1979; Coull and Bell, 1979; Fleeger, 1985; Kennedy and Jacoby, 1999; MacKenzie et al., 2015; van Regteren et al., 2020) or a limited number of well described species (Fleeger et al., 2015; Fleeger et al., 2018; Fleeger et al., 2019). Meiofauna diversity in general remains poorly known at the species level (Snelgrove et al., 1997; Gielings et al., 2021). This is largely due to the effort and technical experience required to identify meiofauna to the species level with classical morphological methods. Traditional studies of meiofauna also are limited by capture of certain groups, especially the smaller, soft bodied organisms such as the Platyhelminthes, Rotifera, and Gastrotricha, due to sampling bias and preservation issues (Kennedy and Jacoby, 1999; Carugati et al., 2015; Leasi et al., 2018). To overcome these limitations, studies on meiofauna are increasingly using DNA metabarcoding methods for rapid, cost-effective identification of meiofauna over traditional methods (Bik et al., 2012; Brannock et al., 2014; Brannock and Halanych, 2015; Creer et al., 2016; Husseneder et al., 2022, reviewed in Gielings et al., 2021). Metabarcoding is used to assess diversity via high-throughput sequencing of amplified DNA markers and assign taxonomic information by comparison to sequences of identified organisms in reference databases (Taberlet et al., 2012). Metabarcoding captures and analyzes DNA from all living organisms in a sample without the need of isolating them, but also from genetic material shed into the environment (environmental DNA, Zaiko et al., 2018). The use of DNA sequencing methods detects even rare taxa and cryptic life stages and allows diversity comparisons even when only a fraction of the species is described and, thus, often exceeds conventional diversity assessments in terms of taxonomic resolution, precision and sensitivity (Zaiko et al., 2018). Thus, metabarcoding is an excellent tool in ecological monitoring (Chariton et al., 2015; DiBattista et al., 2020).
The purpose of this study was to describe metazoan community diversity consisting of meiofauna and environmental DNA at different salinity zones in Louisiana estuaries to detect changes in these communities caused by the planned freshwater diversion projects or other unforeseen environmental disturbances including saltwater intrusion and pollution. Communities were described from three salinity zones in two Louisiana bays, Barataria Bay and Caillou Bay, which previously were selected for the collection of baseline insect community data across the salinity zones (Aker, 2020). Samples were collected from four distances from the marsh edge to capture diversity and test whether there would be distance-dependent differences in metazoan composition. The timing of this study was critical for the collection of a baseline taxa and diversity inventory since Barataria Bay likely will be immediately affected by the Mid-Barataria Sediment Diversion project when it is initiated (Coastal Protection and Restoration Authority of Louisiana, 2007; Mccall and Pennings, 2012; Minowa and Garaffoni, 2017).
Materials and methods
Site determination and sample collection
Sampling sites were selected from two major Louisiana estuaries: Caillou Bay and Barataria Bay. Caillou Bay is to the southwest of the mouth of the Atchafalaya River, and Barataria Bay is to the East of the mouth of the Mississippi River. Monthly recordings by six Coastal Reference Monitoring Stations from 2014 to 2017 (CPRA, 2018, http://cims.coastal.louisiana.gov) were analyzed to choose sample sites in three salinity zones (low (∼4.8 ppt), mid (∼8.5 ppt), and high salinity (∼13.9 ppt) for each bay (Supplementary Figure S1). Salinity differed significantly among the three zones but no differences were detected between corresponding zones in both bays (Aker, 2020). Sample sites were not exposed to oiling by the Deepwater Horizon oil spill in April 2010 as determined by landing data from the Environmental Response Management Application (NOAA, 2018) (https://erma.noaa.gov/gulfofmexico/). Within each zone in each bay, three replicate sites were selected by accessibility and proximity to the Coastal Reference Monitoring Station used to determine local salinity. The sites in Barataria Bay were also selected for proximity to the proposed Mid-Barataria Sediment Diversion project. Four soil samples were collected at each site along a transect at 0, 1, 5, and 10 m from the water edge. The starting point of transects (0 m into the marsh) was defined as the edge of the marsh, where the outermost plants begin to appear. Soil samples were collected using a coring device with removable 10.2 cm diameter × 30.5 cm long cylindrical acrylic cores (Rayle, 2021). Once the soil sample was collected, it was immediately capped and placed into a cooler with ice for transport. Soil cores were stored in a −20°C freezer until processing. Overall, 72 soil samples were collected (two bays * three salinity zones * four distances from the marsh edge * three replicates at each site).
Soil chemistry and analysis
Samples collected 5 and 10 m from the marsh edge (n = 36) were processed at the LSU Agcenter Soil Testing and Plant Analysis lab for chemistry analysis using routine methods described at http://www.lsuagcenter.com/portals/our_offices/departments/spess/servicelabs/soil_testing_lab. Parameters measured were Carbon (%), Nitrogen (%), carbon/nitrogen (C/N) ratios, Percent Organic Matter (%), Aluminum (ppm), Boron (ppm), Calcium (ppm), Chloride (ppm), Conductivity (dS/m), Copper (ppm), Iron (ppm), Magnesium (ppm), Manganese (ppm), pH, Phosphorus (ppm), Potassium (ppm), Salts (ppm), Sodium (ppm), Sodium Adsorption Ratio (SAR), Sulfur (ppm), Zinc (ppm). All soil chemistry data were tested for significant differences across the salinity zones, the different bays, and the different distances from the marsh edge using a three-way ANOVA including all 2- and 3-way interactions in the aov function in the stats package in R (Chambers et al., 1992). The Tukey HSD method in R (Yandell, 1997), was used to perform post hoc testing on all analyses. Spearman correlations were performed to test for correlation of sediment chemistry parameters and metazoan alpha-diversity.
Sample processing
Frozen soil cores were removed from the acrylic cores and an approximately 5 g section was cut from the top one-centimeter section of each core staying well above the anoxic zone using a handsaw disinfected with 10% bleach. The cut sections were thawed in 95% ethanol at 4°C, then rinsed through a 500 μm sieve onto a 45 μm sieve. The portion of the sample retained on the 500 μm sieve was stored in 95% ethanol at −20°C as voucher material. The portion of the sample retained on the 45 μm sieve was mixed with Ludox (Sigma-Aldrich, Munich, Germany) for 1 h and centrifuged at 45,000 rpm for 15 min at 25°C. The supernatants containing the organic portion including the meiofauna were collected onto a 45 μm sieve, thoroughly rinsed and stored in 95% ethanol at 4°C until DNA extraction (Rayle, 2021). Since the sediment samples were frozen and then thawed and agitated, fragments of macrofauna were captured along with meiofauna.
DNA extraction, polymerase chain amplification, and sequencing
Total DNA extractions were performed on three 0.25 g portions of the organic portion of each sample using DNeasy Powersoil kits (Qiagen, Hilden, Germany). All aliquots were checked for DNA concentration using the Invitrogen Qubit 4 Fluorometer (Thermo Fisher Scientific, Wilmington, DE) with the Qubit dsDNA BR Assay Kit. The NF1/18Sr2b primer set (Porazinska et al., 2009; Porazinska et al., 2010), with NextEra Illumina adapter sequences was used to amplify a portion of the 18S small ribosomal subunit gene, which is a universal marker for comparatively unbiased identification of nearly all eukaryote taxa (Creer et al., 2016; Jacquiod et al., 2016). The PCR mix consisted of 10.5 μl Taq polymerase master mix (New England BioLabs), 0.5 μl (at 5 μM concentration) of each of the forward and reverse primer construct, 1 μl extracted sample DNA (at 10 ng/μl), and 12.5 μl DNA-ase free water for a total of 25 μl for each reaction. All PCR products were sequenced at the University of New Hampshire Hubbard Center for Genome Studies on the Illumina Hiseq 2500 platform (Caporaso et al., 2012), using NextEra DNA Flex Library Prep kits (Illumina, San Diego, California). For all DNA aliquots, 2 × 250 base pair forward and reverse FASTQ files were produced. Sequence files and metadata are available in NCBI GenBank via the BioProject accession number PRJNA706429.
Bioinformatics
Initial bioinformatics steps were performed on the University of New Hampshire Hubbard Genome Center server using QIIME2 version 2020-2 (Bolyen et al., 2019). Sequences were subjected to rigorous quality control and ends with low Phred quality scores were trimmed using the DADA2 algorithm (Callahan et al., 2016). Due to the low quality of the reverse sequences, only forward sequences were used for further analyses. The resulting amplicon sequence variants (ASVs), which are unique representative sequences, were taxonomically classified by comparing them to the SILVA 132 database (Quast et al., 2013) using the BLAST algorithm (Camacho et al., 2009) with a <97% cutoff for unassigned sequences. This procedure resulted in 11,620 ASVs represented by approximately 15 million reads with 3,813 ASVs represented by approximately eight million reads assigned to eukaryote taxa. The separate entries in the ASV table for each DNA aliquot were merged into a single entry per sample using the q2-feature-table plugin. All ASVs were filtered to remove non-metazoa taxa, vertebrate environmental DNAs, and all taxa which might be contaminants using the filter-table method of the q2-taxa plugin.
These filtered ASVs were clustered at 97% using the cluster-features-de-novo method in the q2-vsearch plugin to account for the intraspecific variation present in metazoans and to avoid artificially inflating biodiversity (Bucklin et al., 2011; Phillips et al., 2019; Brandt et al., 2020). Clustering produced a table of operational taxonomic units (OTUs) by sample. The sequences were aligned using the mafft method (Katoh et al., 2002) and masked (Lane, 1991) to create a phylogenetic tree with the q2-fasttree plugin (Price et al., 2010). The OTU table was exported from the qiime format to the biom format for use in R with the biomformat package (McMurdie and Paulson, 2020). Read counts were manually converted into incidence per sample. Throughout this manuscript the term “incidence” refers to the presence of an OTU in a sample. If only one OTU is considered, incidence represents the number of samples in which the OTU was detected. For a group of OTUs incidence is the sum of the number of times the OTUs were detected in the dataset. Incidences were preferred over read counts because read counts are only weakly correlated to biomass or the number of individuals in multicellular metazoa (Egge et al., 2013).
The method rarecurve in the R package vegan (Oksanen et al., 2018) was used to generate alpha rarefaction curves featuring OTU richness plotted against sequencing depth for each sample. Additional sample and coverage-based rarefaction curves were generated in the R package iNEXT (Hsieh et al., 2016) using three metrics to measure effective diversity, i.e., richness, the exponential of Shannon’s entropy index, and the inverse of Simpson’s index. Effective diversity is the number of equally abundant OTUs required to reach the value of the metric at the number of samples or estimated coverage.
Diversity profiles were calculated with the R package SpadeR (Chao and Jost 2012; Chao et al., 2015) using the OTU incidence table. The diversity profile consisted of observed total incidences, total richness, coverage estimate, estimated coefficient of variation, incidences, and total OTUs for both the frequent (in >10 samples) and infrequent groups (in ≤10 samples) of OTUs. The coverage estimate is a measure of sampling completeness, which estimates the percentage of the actual community present in the environment that the sampling captured. The coefficient of variation is the measure of heterogeneity in the dataset. Richness values for the actual community were estimated using the homogenous model, the Chao2 model, the iChao2 model, and the incidence-based coverage estimator (ICE) model. The homogenous model estimates richness as though all OTUs have an equal chance of being detected. The Chao2 model uses OTUs detected in only one or two samples to estimate actual richness. The iChao2 model uses the OTUs detected in four or less samples to estimate actual richness and is less biased than the Chao2 model for more heterogeneous datasets. The ICE model uses the infrequent group of OTUs to estimate OTUs, and is an incidence based version of the abundance-based coverage estimator (Chao and Lee, 1992).
Taxonomically assigned OTUs were manually checked using the BLAST + algorithm against the NCBI GenBank database (Benson et al., 2011). All OTUs were given additional assignments based on the top BLAST hit, sorted by E-value and taxonomic strings were checked for accuracy against the World Register of Marine Species database (Horton et al., 2022). Taxa bar plots for each salinity zone were created using the OTU incidence table in the R package ggplot2.
Alpha-diversity, i.e., diversity within samples, was calculated using the incidence-based OTU richness and Faith’s Phylogenetic Diversity (PD) measures in the q2-alpha plugin. The OTU richness is the number of OTUs in a sample, while the Faith’s PD value is the total branch length of the phylogenetic tree of all OTUs within a sample (Faith, 1992). Both values were used to create boxplots in the ggplot2 package in R and tested for differences across the salinity zones, bays, and distances from the marsh edge using a Kruskal-Wallis procedure in the q2-diversity plugin (Kruskal and Wallis, 1952).
For calculating Beta-diversity, i.e., diversity among groups of samples, a distance matrix of Sørensen index values for each pair of samples in the dataset was created using the q2-diversity plugin (Sørensen, 1948). This index was chosen because it uses the composition of samples, rather than read counts, which avoids the issue of individual numbers not corresponding to read counts in metazoan taxa. The distance matrix was then tested using the Adonis procedure in the q2-diversity plugin, with the formula “salinity zone * bay * distance from marsh edge” and 10,000 permutations. The Adonis procedure is a multifactorial PERMANOVA test, which uses the sum of squares of the distances between centroids of groups of samples belonging to each factor and the overall centroid to calculate F-ratios and permutations of observations to determine significant differences. An assumption of the PERMANOVA test is that all groups have similar spread in the multivariate space. A PERMDISP test was used to determine the spread of these groups. Following all tests, p-values were corrected to account for the false discovery rate using the p.adjust function in the stats package in R (Benjamini and Hochberg, 1995). Beta-diversity, i.e., dispersion and differentiation of the metazoan composition of the samples from the different salinity zones, bays, and the distances from marsh edge, was visualized using Non-metric MultiDimensional Scaling (NMDS) ordination using the Sørensen index in the metaMDS method in the R package vegan (Oksanen et al., 2018). The ordination was plotted using the R package ggordiplot, available at https://github.com/jfq3/ggordiplots (Quensen, 2020).
Results
Sequence depth-, sample-, and coverage-based rarefaction of metazoan communities
Only the groups Metazoa, Fungi, and Archaeplastida were present in all samples with the majority of reads in most samples belonging to the Metazoa (Supplementary Figure S1). Metazoa also had the highest rate of clear taxonomic assignments (Supplementary Table S1). Therefore, the dataset was filtered for non-vertebrate Metazoa (893 ASVs with approximately four million reads). After clustering of ASVs, which were more than 97% similar, to control for intraspecific variation, the final total number of OTUs was 312.
Rarefaction curves of OTU richness versus sequencing depth for all samples leveled off, indicating that sufficient sampling depth was achieved for all samples to collect all metazoan OTUs present (Figure 1). In addition, though a wide range of both richness and sampling depth was detected, higher sequencing depth did not lead to higher OTU richness.
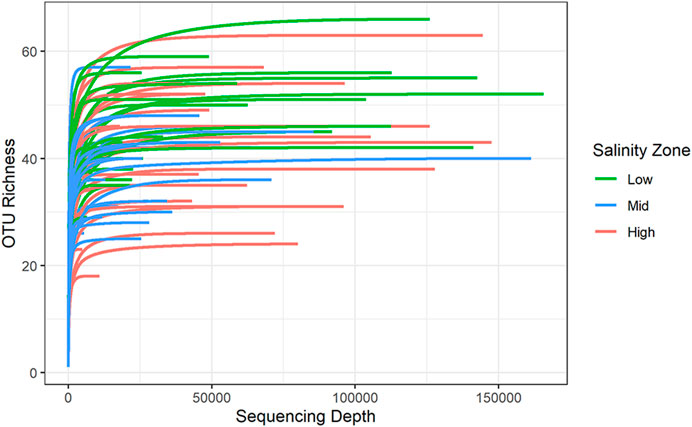
FIGURE 1. Alpha rarefaction curves show the number of OTUs detected in each sample when randomly sampling sequences at each given sequencing depth.
Sample-based rarefaction curves for groups of samples from each salinity zone began to level off at actual sample sizes, but the extrapolated portions of the richness curves continued to increase with additional samples (Figure 2). The curves for the effective diversity of the Shannon and Simpson Inverse indices barely increased, which indicates that all common OTUs within the actual metazoan community were collected (Figure 2). Sample-based rarefaction curves for the different bays and distances from the marsh edge also showed sufficient capture of common OTUs (Supplementary Figure S3).
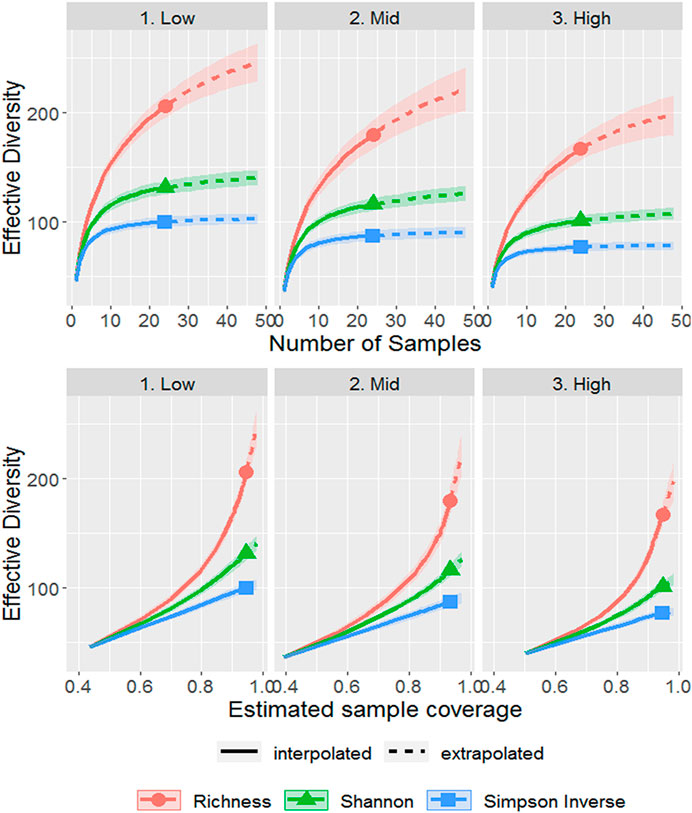
FIGURE 2. Sample-(top) and coverage-based (bottom) rarefaction curves for the metazoan community from marsh soil of three salinity zones (low, mid, and high salinity) calculated with three metrics of effective diversity (Richness, Shannon, and Simpson inverse indices). Extrapolation extended out to twice the number of samples. The shaded area around each curve represents the 95% confidence interval.
Coverage-based rarefaction curves confirmed that the current number of samples collected represented the majority of OTU richness in the actual metazoan community for each salinity zone (92%–94% coverage, Figure 2), bay (94%, Supplementary Figure S3) and distance from the marsh edge (90%, Supplementary Figure S3), which is consistent with a high Coverage Estimate for the entire data set (96.7%, Supplementary Table S2). However, the dataset was heterogeneous with a high Coefficient of Variation (1.4, Supplementary Table S2). Only 88 of the 312 OTUs were present in more than 10 samples but they represented the majority of the incidences (2,345 of the 2,993 incidences, Supplementary Table S2). The observed OTU richness (312) represented at least 58% (535 OTUs at the upper bound of the Chao2 model) to 92% (339 OTUs at the lower bound of the Homogenous Model, Supplementary Figure S4) of the total estimated metazoan OTU richness.
Alpha-diversity of the saltmarsh metazoan community
The OTU richness was significantly higher in samples from the low salinity zone than in those from the mid salinity zone [Kruskal-Wallis pairwise test, χ2(2) = 9.16, Benjamini Hochberg corrected p < 0.01], but samples from the high salinity zone did not differ in terms of metazoan richness from the other salinity zones (Figure 3). No significant differences in OTU richness were detected for samples collected from different bays or distances from the marsh edge or interaction of the three factors. Values for the Faith’s Phylogenetic Diversity metric showed no significant differences for any factor or interaction of factors. Since Faith’s PD was not significantly different across the three salinity zones (Kruskal-Wallis test, p = 0.35) the elevated richness in low salinity samples was not caused by the addition of widely phylogenetically different taxa.
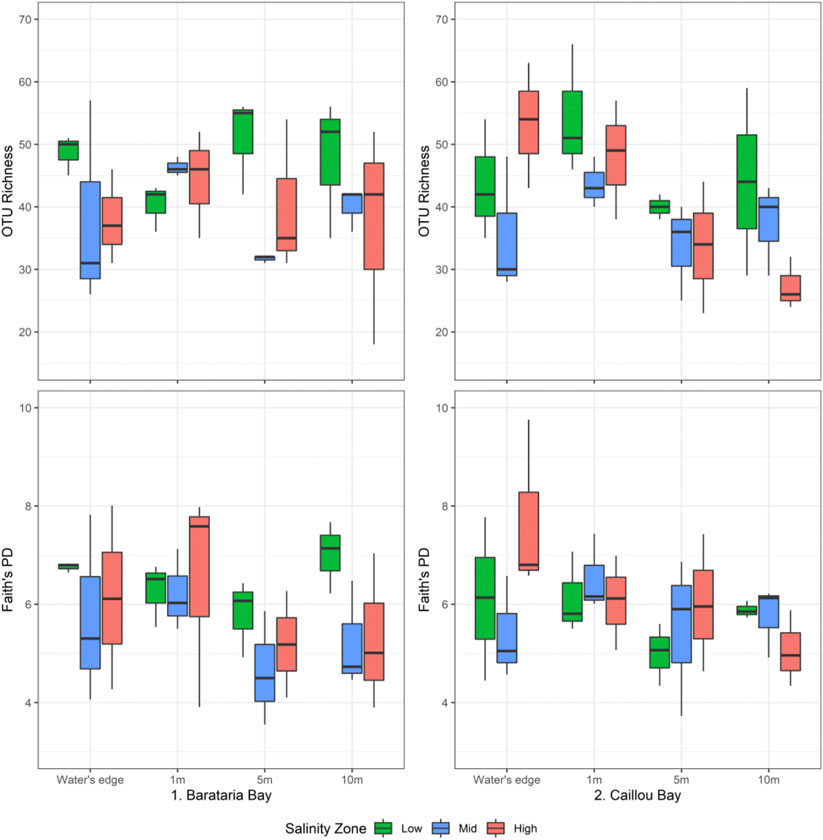
FIGURE 3. Boxplots of metazoan OTU richness (top) and Faith’s PD values (bottom), divided by bay, salinity zone, and distance from marsh edge.
Correlation between 21 soil chemistry variables (Supplementary Table S3) and OTU richness only produced significant results for carbon and nitrogen percentage, which were both positively correlated with metazoan richness (p = 0.015 and 0.011, Spearman rank). Faith’s PD was not significantly correlated with any of the soil chemistry variables (p > 0.05).
Beta-diversity of metazoans from different bays, salinity zones, and distance from the marsh edge
Similarity among metazoan communities measured by the number of shared OTUs and Sørensen index values was lowest among salinity zones, followed by similarity between bays and among distances from marsh edge (Supplementary Table S4). Pairwise Sørensen index values indicated that the low and high salinity zone communities had the lowest similarity of any pair of the salinity zone communities because they shared the lowest number of OTUs (Supplementary Table S4).
All factors (salinity zone, bay, and distance from marsh edge) and all factor interactions had significant effects on the differentiation of metazoan composition of samples in the dataset (Adonis test with Benjamini-Hochberg correction, Table 1). Salinity accounted for more variation in the dataset than any other factor or interaction (20%, Table 1). Distance from the marsh edge and bay explained only 7% and 6% of variation, respectively. Nevertheless, the significant interactions indicate that the effect of salinity also depend on those minor factors.
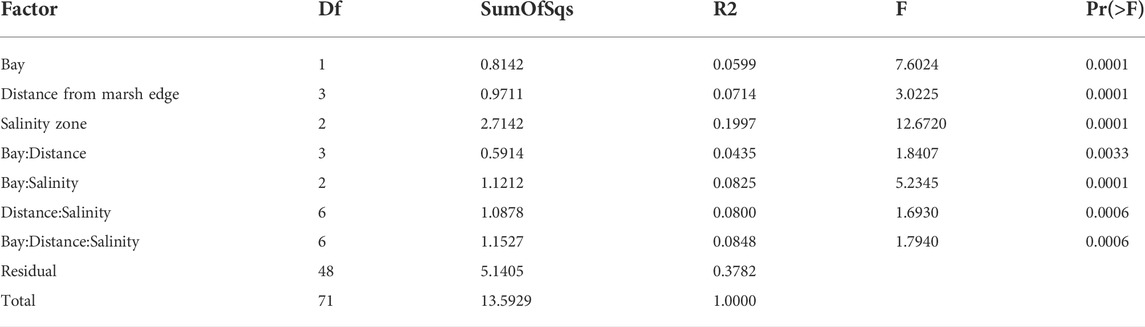
TABLE 1. Adonis test results for differentiation of metazoan communities among samples from different bays (Caillou and Barataria), distances from the marsh edge (0, 1, 5, and 10 m) and salinity zones (low, mid, and high). All p-values remained significant after the Benjamini-Hochberg correction.
Since significant differences in dispersion had been observed for salinity and bay (PERMDISP, Benjamini-Hochberg corrected p < 0.05), differences detected by Adonis within these factors may be a combination of differences in the multivariate spread of the groups and differences in the location of the groups in the multivariate space. To visualize contributions of the three factors in terms of spread and differentiation between groups in the multivariate space, we employed NMDS scaling (Figure 4). Plotting the Beta-diversity among samples from different salinity zones showed a distinct separation of samples from the low and high salinity zones, with samples from the mid salinity zone falling roughly between them (Figure 4 top). Samples from the mid salinity zone showed larger dispersion than the samples from the high and low salinity zones (pairwise PERMDISP, Benjamini Hochberg corrected p < 0.05). The large spread of samples in the mid salinity zone was mainly caused by samples from Caillou Bay which had significantly different multivariate spread from all other groups (pairwise PERMDISP, p < 0.05, Figure 4 bottom). Distance from the marsh edge showed no particular pattern (Rayle 2021).
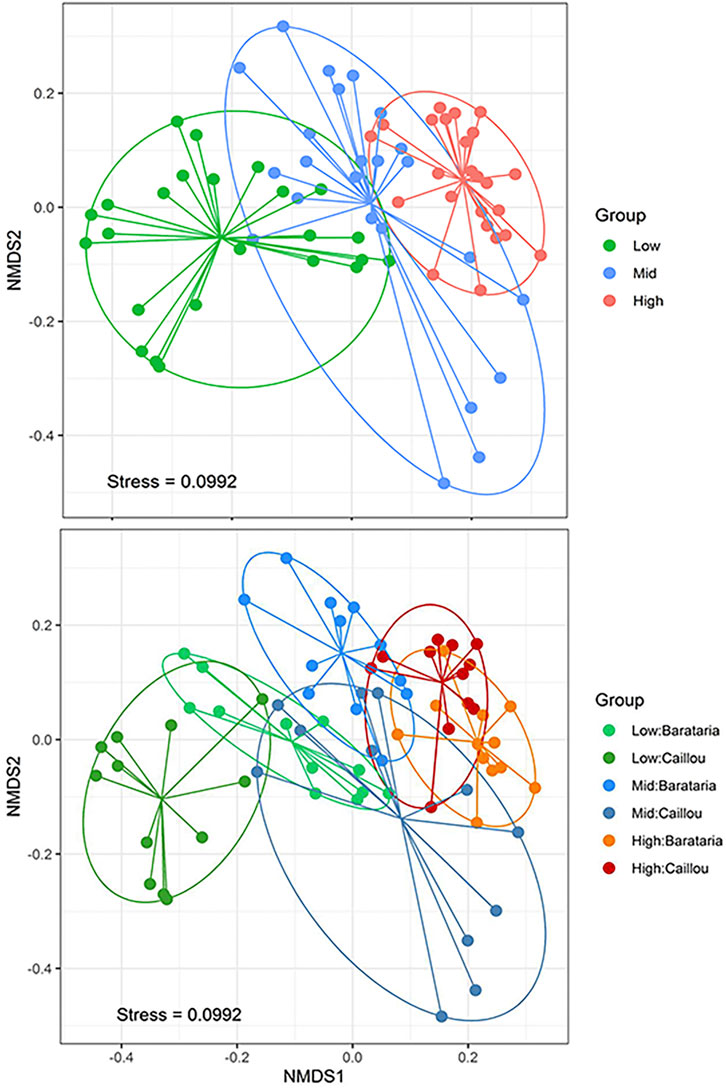
FIGURE 4. Plot of the first two dimensions of the NMDS ordination of the Sørensen distance matrix, with salinity zones (top) and from each bay (bottom). Dots represent the metazoan composition of individual samples. Solid ellipses represent the elliptical hulls of the groups. Solid, straight lines connect a sample to the group centroid. Stress denotes the goodness of fit of the regression of the original distance matrix values against the ordination distances.
Taxonomic assignment of metazoan infauna in Louisiana marshes
Each sample contained between 5 and 10 phyla. Nematodes, arthropods, and annelids were present in all samples (Figure 5). The phylum Nematoda made up the largest percentage of the 312 OTUs and the 2,993 total incidences (Supplementary Figure S5), followed by Arthropoda, Annelida, and Platyhelminthes. Platyhelminthes, rotifers, and mollusks were present in nearly all samples. Members of the phyla Nemertea, Tardigrada, Xenacoelomorpha, and Calcinea were rarely detected in samples (Figure 5; Supplementary Table S5).
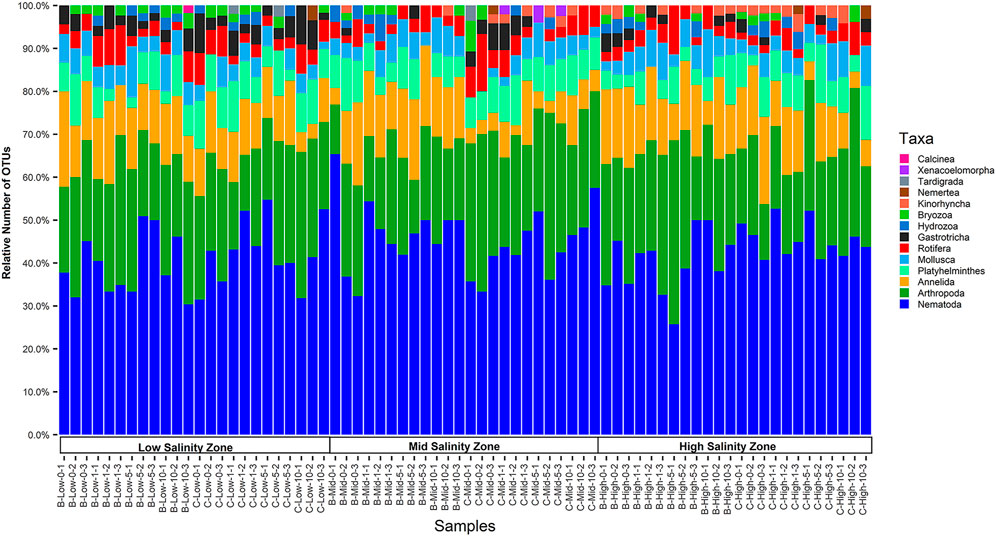
FIGURE 5. Taxa bar plots of the 72 samples collected from the three salinity zones of Barataria and Caillou Bays during July 2018. Each colored section represents the relative proportion of OTU incidences belonging to different phyla within that sample. Phyla are sorted within each bar by the overall highest to lowest relative percentage (from top to bottom). For the x axis, samples are labelled as B (Barataria) or C (Caillou), and Low, Mid, and High for the salinity zones. The number following the salinity zone designation indicates the distance from the edge of the marsh, designated as 0, 1, 5, or 10 m, followed by the transect replicate number.
The most common OTU in the dataset was assigned to the rotifer Octotrocha speciosa (detected in 99% of samples), followed by an OTU assigned to the arthropod Chrysops flavidus (deer fly in 96% of samples) and one assigned to the nematode Spirinia parasitifera (94% of samples, Supplementary Table S5). The frequent group (in >10 samples) consisted of 88 OTUs. The frequent group included 38 nematode OTUs of the orders Enoplida (9 OTUs), Monhysterida (8 OTUs), Tylenchida (7 OTUs), Chromadorida (4 OTUs) and Dorylamida (4 OTUs), Desmodorida (2 OTUs), Plectida (2 OTUs), Areolamida (1 OTU), and Rhabditida (1 OTU, Supplementary Table S5). The frequent group also contained 19 Arthropoda OTUs of which nine OTUs belonged to the crustacean orders Podocopida, Harpacticoida, Decapoda, and Tanaidacea, five OTUs belonged to the insect order Diptera, four to the Collembola, and one OTU belonged to the Acari (Supplementary Table S5). Of the 12 frequently detected annelid OTUs, half were assigned as the oligochaete order Haplotaxida the other half were polychaetes. Three Haplotaxida OTUs belonged to the family Naididae, including the most commonly detected annelid OTU in the dataset assigned to the species Monopylephorus rubroniveus (Naididae) and detected in 88% of samples. The remaining Haplotaxida OTUs were all assigned to members of the genus Marionina. Three polychaete OTUs of the order Spionida each were assigned to members of various genera in the family Spionidae, while the three Phyllodocida OTUs were assigned to the family Nereididae. The seven Platyhelminthes OTUs of the frequent group were mostly assigned to the order Rhabdocoela (5 OTUs), with one OTU assigned to the order Macrostomida and one assigned to the class Catenulida. In addition, top GenBank matches identified an ambiguous OTU as another member of the Macrostomida, Macrostomum lignano, which appeared in 63% of samples. Both OTUs of the Gastrotricha frequent group were assigned to the order Chaetonotida. The three frequently detected mollusk OTUs were members of the sea snails (orders Littorinimorpha and Caenogastropoda) and bivalves (Veneroida), the two Bryozoa OTUs were assigned to the orders Ctenostomatida and Plumatellida and the two Rotifera OTUs were assigned to the orders Flosculariaceae and Ploimida. The only hydrozoan in the frequent group was assigned to the order Limnomedusae, while the only kinorhynch OTU was assigned to the order Cyclorhagida (Echinoderes sp.). Assignments of OTUs from the infrequent group are also listed in Supplementary Table S5.
Taxa unique to salinity zones
Overall, metazoan DNA samples showed higher taxa richness and incidence in low salinity compared to mid salinity zones; however, samples from high salinity zones had high values similar to low salinity (Supplementary Table S5). The Nematoda and Mollusca were fairly equally distributed across salinity zones (Supplementary Table S5). Gastrotricha, Bryozoa, Platyhelminthes, and Rotifera were more prevalent in the low salinity zone (61%, 48%, 41%, and 41% of incidences, respectively) than in the other two salinity zones. Kinorhyncha (Echinoderes sp.) were never detected in the low salinity zone and were more commonly detected in the high (68% of incidences) than in the mid salinity zone (32%).
While most Arthropoda were detected in low salinity, insect diversity was U-shaped across the salinity zones with lower detection in mid salinity (97 incidences of 20 OTUs in 12 families) when compared to the low (106 incidences of 25 OTUs in 16 families) and high salinity zones (106 incidences of 24 OTUs in 15 families, Supplementary Table S5). Fifty-five percent of the insect OTUs detected were unique to a single salinity zone with most of them being unique in the low salinity zone. However, there were five Diptera OTUs of gall midges (Cecidomyiidae) and tabanids (Chrysops and Tabanus spp.) in the frequent group that were common among all salinity zones. These and several other OTUs were assigned to species known from Louisiana marshes, including Cedusa obscura (Derbidae) and biting midges (Culicoides, Cerapogonidae) (Supplementary Table S5). Similarly, the frequency of detection of the Annelida, across salinity zones was U-shaped with lower numbers of annelid incidence (26%) in the mid salinity zone compared to low and high salinity zones (∼37% of total annelid incidence).
Many of the OTUs (113) were either unique to one of the salinity zones or were absent from one of the salinity zones (Supplementary Table S5). Twenty-three of the unique OTUs belonged to the frequent group (in >10 samples) and included nematodes, platyhelminths, annelids, arthropods, gastrotrichs, and kinorhynchs (Table 2). Two nematodes (assigned as E. filiformis and Prismatolaimus sp.) and one platyhelminth OTU (assigned as R. simplex) were unique to the low salinity zone. One gastrotrich (C. novenarius) was detected 15 times in the low salinity zone samples but was absent in mid salinity and only detected once in high salinity (Supplementary Table S5). A second Prismatolaimus sp. OTU, Anoplostoma sp., and Prodorylaimus sp. of the Nematoda, two annelids (assigned as M. southerni and D. aestuarina), four arthropods (Collembola assigned as ambiguous taxa and Cryptopygus sverdrupi as well as ostracods of the Cyprididae and Cypria sp.) and the platyhelminth assigned as O. truncula were present in low and mid but absent from high salinity (Table 2). In contrast, two frequent group members of the annelids (A. succinea and N. jaya), a kinorhynch (assigned as an ambiguous Cyclorhagida), four nematodes (Meloidogyne spartinae, Prochaetosoma sp., Halalaimus sp., and Dichromadora sp.), and two platyhelminths (assigned as M. kepneri and M. terminostylis) were present in both the mid and high but not low salinity zones. Rare OTUs unique to salinity zones, including the polychaete Manayunkia aestuarina, are listed in Supplementary Table S6.
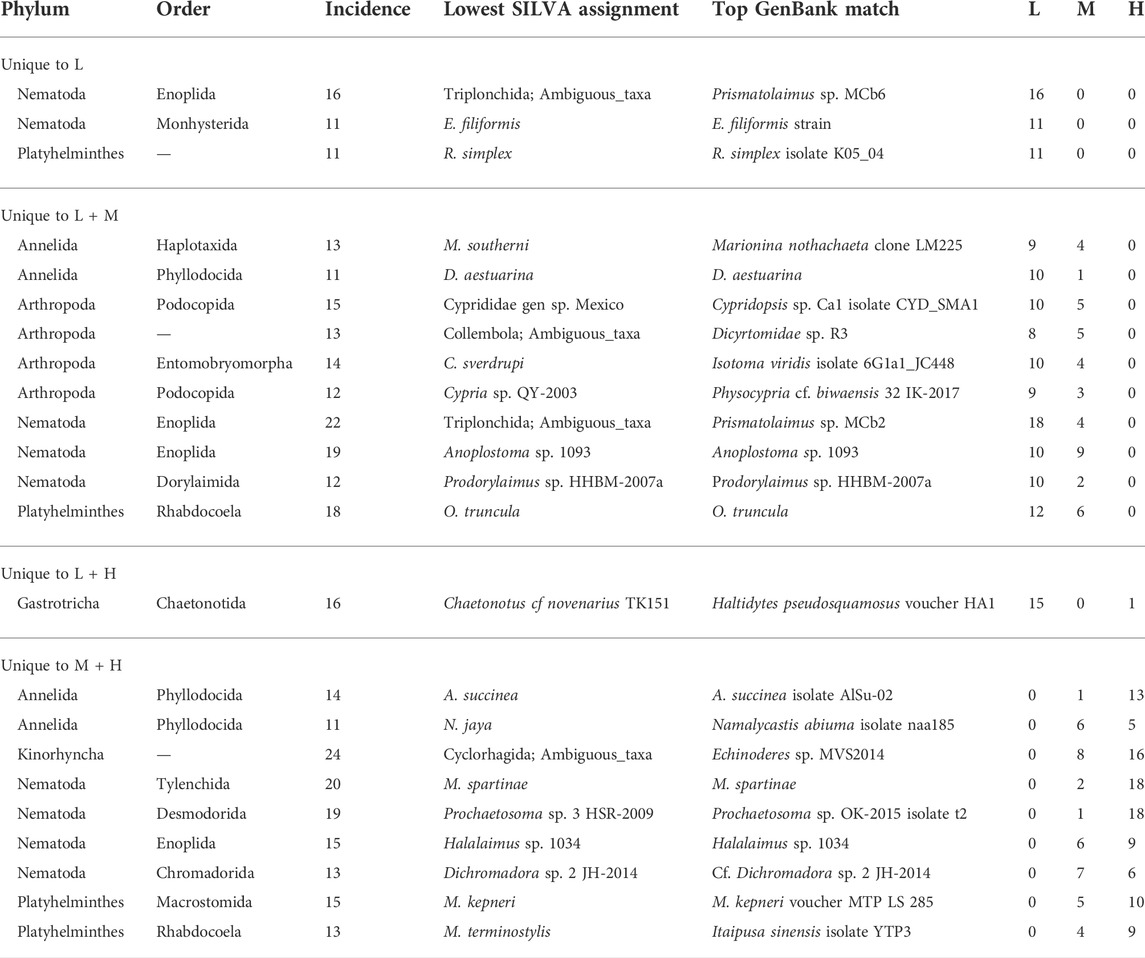
TABLE 2. Frequent OTUs with unique incidences in samples from different salinity zones. OTUs listed were not detected in at least one of the salinity zones (L = Low, M = Mid, H = High), and were detected at least twice in one or two salinity zones. No frequent OTUs were unique for mid or high salinity. Infrequent OTUs (in ≤10 samples) are listed in Supplementary Table S6.
Annelid (e.g. Marionina spp.) and mollusk OTUs were much more commonly detected in samples from Barataria Bay, while gastrotrichs, platyhelminths and kinorhynchs (e.g., Echinoderes sp.) were more commonly detected in the Caillou Bay samples (Supplementary Table S5). Annelids, gastrotrichs, and hydrozoans more frequently detected at the marsh edge and at 1 m from the marsh edge while mollusks and nematodes were more common in the samples 1 m from marsh edge than further inland (Supplementary Table S5). None of the frequent group OTUs were uniquely found at a certain bay or distance from the marsh edge. Incidences of frequent and rare OTUs for each bay and distance in addition to salinity zone, are listed in Supplementary Tables S5, S7.
Discussion
Coastal marsh ecosystems, especially within Louisiana, are at risk due to numerous natural and anthropogenic environmental stressors (Yuill et al., 2009; Wong et al., 2010; Lin and Mendelssohn 2012; Kirwan et al., 2016). Assessment of the impact of these stressors on marsh communities and habitat remediation via restoration projects must take changes in salinity levels and resulting shifts in marsh communities into account. Therefore, detailed inventories of organisms relative to salinity zones are needed as baselines to measure impacts of environmental changes on marsh community health.
Soil meiofauna and macrofauna (collectively, infauna) have been studied for decades in Louisiana’s coastal marshes and inventories based on morphological classification are relatively complete for major taxa (Fleeger, 1985; Fleeger et al., 2015; Fleeger et al., 2018; Fleeger et al., 2019). However, morphological identification is likely to underestimate meiofaunal diversity (Gielings et al., 2021) and only few studies included eukaryote metabarcoding to describe infauna diversity in Louisiana’s tidal marshes (Husseneder et al., 2022). Moreover, species inventories of Louisiana’s tidal marshes lack detailed metadata concerning salinity, which is expected to be one of the major factors changing due to sea level rise and freshwater diversions (Das et al., 2012). This project was designed to provide baselines of metazoan biodiversity based on meiofauna and environmental DNA across salinity zones to monitor changes in marsh habitat primarily due to salinity changes with saltwater intrusion or freshwater influx when the planned Mid-Barataria Sediment Diversion commences (Coastal Protection and Restoration Authority of Louisiana, 2007; Coastal Protection and Restoration Authority of Louisiana, 2012; Coastal Protection and Restoration Authority of Louisiana, 2017; Peyronnin et al., 2013). Freshwater flow has been shown to drastically change meiofauna populations (Montagna and Kalke 1992; Montagna et al., 2002).
In summary, our study showed that salinity, but not bay or distance from the marsh edge significantly impacted metazoan Alpha-diversity within samples. Salinity was also a major factor accounting for 20% of the variation in community composition among samples, with many frequent OTUs being uniquely present or absent in salinity zones. Bay and distance from the marsh edge as well as interactions among the factors only had minor impacts on community differentiation (all <8%).
Differences in metazoan communities among bays might be driven by differences in the plant communities between the bays. For example, Marionina spp. (Annelida), which are likely associated with Spartina stems (Healy and Walters, 1994), were more common in the Barataria Bay, which had more Spartina ground cover than Caillou Bay (Aker, 2020). Long-term impacts of the Deepwater Horizon oil spill might also explain some differences between bays. Taxa with slow recovery due to poor dispersal activity including the kinorynchs (Fleeger et al., 2018; Fleeger et al., 2019), might still endure reduced abundance in Barataria Bay, which had been more heavily impacted by the Deepwater Horizon oil spill than Caillou Bay (Michel et al., 2013). Kinorhyncha detected by metabarcoding made up less than 1% of the total OTUs and were assigned as Echinoderes sp. Echinoderes were an indicator for oil pollution, since they were absent from the heavily oiled marsh sites following the Deepwater Horizon oil spill (Fleeger et al., 2015; Fleeger et al., 2018). Even though our sites were not oiled, area wide population reduction for some species has been observed even at sites not directly oiled (Husseneder et al., 2022).
Previous studies reported distance effects with higher invertebrate densities near the marsh-water interface due to larval recruitment and food deposited by inundation (Kneib, 1984; MacKenzie et al., 2015) and clear differentiation of benthos communities across marsh zones from the tidal flats landward to the pioneer zone, low marsh and high marsh (MacKenzie et al., 2015; van Regteren et al., 2020). Dominance of annelids and mollusks in the pioneer zone and tidal flats reported by van Regentren et al. (2020) supports the higher frequency of detection of these phyla at our sample sites close to the marsh edge. In contrast, MacKenzie et al. (2015) found higher species richness in the high marsh compared to low marsh but this was due to sampling across larger distances and elevations compared to our study. Overall, distance effects were much less pronounced in our metabarcoding study and none of the frequently detected OTUs were uniquely present or absent at a certain distance. Our study used much shorter transects, which explains why less community differentiation was detected compared to other studies across marsh zones. Moreover, with the low elevation gain of Louisiana marshes even our sites furthest from the marsh edge (10 m) received frequent inundation.
Similar to our study, salinity was a major explanatory variable of benthic macro- and meiofauna community variation in previous studies. Generally, macro- and meiofauna diversity increases with higher salinity along marine salinity gradients (Broman et al., 2019). Studies in saltmarshes of the East coast and estuaries of the Texas Gulf coast found weak correlations of salinity to observed soil invertebrate communities (MacKenzie et al., 2015; Van Diggelen and Montagna, 2016). However, in our metabarcoding study, the relationship of overall metazoan diversity and salinity was U-shaped with the lowest values in mid salinity zones. In particular, insects and annelids had the lowest detection frequency in mid salinity. This pattern has been observed frequently in estuarine systems (Deaton and Greenberg, 1986).The mid salinity zone in our study averaged approximately 8.5 ppt, which is close to the “Artenminimum” zone between 5 and 8 ppt where overall species numbers are lowest in estuaries and bodies of brackish water but numbers of brackish water species are highest (Remane, 1931; Deaton and Greenberg, 1986). The low species diversity in the mid salinity zone has been hypothesized to occur because speciation rates in dilute brackish environments are lower than for freshwater and marine species and few animals acquire successful physiological adaptations to tolerate the range of conditions in this habitat (Deaton and Greenberg, 1986).
In traditional studies of tidal marsh fauna, nematodes are typically among the most abundant non-insect invertebrate group (Bell, 1979; Fleeger, 1985; Alves et al., 2013; MacKenzie et al., 2015), which was consistent with nematodes being the most frequently detected group in our study. Nematodes are known for their ability to tolerate extreme environmental conditions (Zeppilli et al., 2018). Previous studies showed a positive correlation of nematode diversity or abundance with salinity gradients of intertidal rivers and the Baltic Sea (Tran et al., 2018; Broman et al., 2019). However, no such relationship was detected with our incidence data across similar salinity ranges. The most frequently detected nematode, S. parasitifera a marine microbivore in the family Desmodoridae also commonly found in European estuaries (Soetaert et al., 1995; Hodda, 2011), was detected across all three salinity zones. Five nematode OTUs of the frequent group were never detected in samples from the high salinity zone: E. filiformis, two Prismatolaimus sp. OTUs, Anoplostoma sp., and Prodorylaimus sp. We predict that salinity reduction following the opening of the Mid-Barataria freshwater diversion will lead to range expansion of these OTUs into formerly high salinity areas. The opposite is expected for taxa predominantly found in high salinity, such as the plant-parasitic root-knot nematode M. spartinae (Plantard et al., 2007) and an OTU of the genus Prochaetosoma, which contains nematode species known from sub-tidal zones (Rho et al., 2010). Notably, some nematodes can survive in hypersaline zones with salinities higher than seawater (Zeppilli et al., 2018).
Arthropoda were the second most abundant group in the dataset after the nematodes. Similar to the nematode distribution, arthropod incidences were approximately evenly distributed across the different bays and distances from marsh edges, but Arthropods were more commonly detected in the low salinity zone than in the mid and high salinity zone. This was mainly caused by non-insect arthropods. Frequently detected non-insect arthropods that were possible bioindicators for low salinity included Collembola assigned as ambiguous taxa and C. sverdrupi as well as ostracods of the Cyprididae and Cypria sp.
Insects are typically among the most common organisms in the low and high marsh (MacKenzie et al., 2015; van Regteren et al., 2020). To confirm taxonomic assignments of the insect OTUs and compare incidences to organismal abundance, we compared our data to those of Aker (2020), who conducted a year-long sampling of insects (2018-2019) using sweep net techniques at the same sites and salinity zones as sampled in this study. While insect abundance cannot be directly compared between metabarcoding and sweep net collection studies due to differences in sampling strategy and data representation, broader insect community comparisons can be made. Aker’s studies found that insect abundance increased dramatically with salinity while insect family-level biodiversity decreased as salinity increased, which mirrored the decrease in plant species. In contrast, the DNA-based incidences and number of insect OTUs and families was U-shaped across salinity zones with slightly lower numbers detected at the mid salinity zone and high salinity zones.
Over half of the insect OTUs detected were unique to a single salinity zone. Being unique for a salinity zone alone could not unequivocally identify these insect OTUs as salinity bioindicators due to their low detection rate; however, closely related species have been confirmed independently as salinity bioindicators (Aker, 2020). Ischnura ramburii (Coenagrionidae, Odonata) and C. obscura (Derbidae, Hemiptera) were identified as bioindicators of low salinity by Aker (2020). Therefore, the metabarcoded OTUs from the families Derbidae (C. obscura) and Coenagrionidae (ambiguous taxa) likely represent bioindicators of low-salinity insect communities because they were detected at the low salinity sites within a month of their peak abundances described by Aker (2020). Biting midges of the genus Culicoides (Ceratopogonidae) are abundant in marshes along the Atlantic (Kneib, 1984; MacKenzie et al., 2015) and Gulf coast (Aker, 2020). The Culicoides OTU detected predominantly in mid and high salinity zones is likely the highly abundant high salinity bioindicator identified in Aker’s studies, Culicoides mississippiensis.
Fly larvae are common in saltmarsh macrofaunal samples but are typically rare in meiofauna assemblages (Kneib, 1984). In our study, five Diptera OTUs belonging to gall midges (Cecidomyiidae) and tabanids (Chrysops and Tabanus spp.) were common among all salinity zones. Gall midges of salt marsh succulents have been described from Australia (Veenstra-Quah et al., 2007), but were not found in Aker’s sweep net surveys because larvae are found in plant tissue and dispersing adults might only be present for a limited time. The detection rate of tabanids across samples and their wide distribution across salinity zones is remarkable and supports the prevalence of tabanid larvae in salt marsh sediment where they are top invertebrate predators (Kneib, 1984; Husseneder et al., 2022). All tabanid OTUs belonged to the known estuarine species of Tabanidae (Tabanus nigrovittatus, T. acutus, T. hinellus, and C. flavidus) collected from the same coastal sites by Aker (2020) with sweep nets and Davis (2022) using tabanid targeted trap techniques (Hribar et al., 1991). Tabanus nigrovittatus is the saltmarsh greenhead horse fly that showed severe population crashes after the Deepwater Horizon oil spill and is thus regarded as a bioindicator of oil pollution (Husseneder et al., 2016; Husseneder et al., 2018; Husseneder et al., 2022). Although direct comparison of the different detection methods is difficult, the sweep net and metabarcoding inventories represent complementary aspects of the marsh community. The advantage of the use of metabarcoding over traditional insect surveys by collection is that insect taxa can be monitored without the need for catching and identifying intact organisms. This makes metabarcoding especially useful for high throughput detection of insects including those living in inaccessible niches or outside of the daily or annual activity period.
Similar to the insects, the frequency of detection of the third most numerous group, i.e., the Annelida, across salinity zones was also U-shaped with lower numbers of annelid incidence in the mid salinity zone compared to low and high salinity zones. The annelid species M. southerni and D. aestuarina were never detected in the high salinity zone samples and are likely low salinity bioindicators. The organisms that these OTUs represent may experience range expansions associated with decreased salinity resulting from the future Mid Barataria freshwater diversion. The polychaete M. aestuarina, which is commonly represented among both macrofauna and meiofauna in marshes of the Southeastern U.S. (Bell, 1979; Kneib, 1984) and is a well-known bioindicator species for the impact of oil spills (Fleeger et al., 2015; Fleeger et al., 2018) was detected in the high salinity zone but in rare incidences. Polychaetes typically show the highest abundance in the tidal flats with higher soil salinity and moisture than the low marsh where oligochaetes have their peak abundance (MacKenzie et al., 2015; van Regteren et al., 2020). The limited detection of Manayunkia is likely due to the processing of the samples, since this species was among the most frequent OTUs in unprocessed soil at three marsh islands in Barataria Bay surrounding Bay Jimmy while in samples sieved for meiofauna it belonged to the infrequent group (Rayle, 2021).
The Platyhelminthes were the fourth most commonly detected and numerous group of OTUs. The Platyhelminthes are not commonly detected or reported in traditional studies of meiofauna (Fleeger et al., 2018), presumably since traditional sampling and extraction methodology destroys the soft bodied members of the meiofauna such as the Platyhelminthes (Carugati et al., 2015). However, they are abundantly represented in environmental DNA studies (Fonseca et al., 2010). Detection frequency of platyhelminths decreased with increasing salinity. Two Platyhelminthes (O. truncula and R. simplex) were never detected in the high salinity zone and, thus, are likely indicators of reduced salinity, which might be able to expand into the areas currently designated as high salinity when the Mid-Barataria freshwater diversion opens. In contrast, two members of the genus Macrostomum were never detected in the low salinity zone and are, thus, potential candidates for indicators of saltwater intrusion. The remarkable ability of saline acclimation of intertidal infauna has been studied in the model M. lignano, which was the most abundant flatworm OTU in our study. Macrostomum was able to survive in high salinity through upregulation of antioxidant enzymes to combat oxidative stress from increased respiration rates to meet the energy demand for osmoregulation (Rivera-Ingraham et al., 2016). In low salinity M. lignano decreased respiration and activity in a hyposmotically induced metabolic shutdown similar to that in other intertidal invertebrates like the marsh periwinkle snail.
The Gastrotricha were the fifth most numerous but the seventh most commonly detected group and were more prevalent in the low salinity zone than in the other two salinity zones. Specifically, the OTU assigned as C. novenarius can be considered as a low salinity bioindicator which might experience a range expansion with freshwater diversions. The Mollusca were the sixth most numerous group of OTUs and the fifth most commonly detected. Most of the conspicuous mollusk species in southeastern US saltmarshes are large and are only represented by traces of their environmental DNA in our data (Kneib, 1984). Most mollusk OTUs were roughly evenly distributed across salinity zones. Kinorhyncha (Echinoderes sp.) were never detected in the low salinity zone and were most prevalent in the high salinity zone. Kinorhynchs of the genus Echinoderes are believed to have special osmoregulatory adaptations provided by an enlarged nephridial sieve plate that enables them to tolerate salinity fluctuations and hypersaline conditions (Zeppilli et al., 2018). Therefore, increasing kinorhynch populations may be an early indicator of high salinity through saltwater intrusion.
This metabarcoding study provided a comparison of compositional inventories and diversity of soil organisms among salinity zones in Louisiana tidal marshes based on sequence data from metazoan meiofauna and environmental DNA. The advantage of the use of metabarcoding over traditional surveys by collection is that taxa can be monitored based on their DNA without the need for having intact organisms, keys and expertise for time-consuming morphologically identification. This makes metabarcoding especially useful for high throughput detection of diversity including taxa that are not well described or difficult to collect because they are small, fragile, cryptic or inactive during the collection period (Gielings et al., 2021). However, the disadvantage is that it is unknown how long environmental DNA can be detected in the absence of living organisms (Harrison et al., 2019; Sakata et al., 2020), which might create background noise for monitoring current state and functional changes in ecosystems. In addition, number of sequence reads correlates only weakly with organism numbers or biomass (Egge et al., 2013) in multicellular organisms, so population abundance is more accurately determined by collections of organisms via traditional methods. Ideally, metabarcoding would not replace traditional sampling methods but rather add a complementary view of the marsh soil community with increased sampling depth and diversity detection. In summary, using environmental DNA we found numerous meiofaunal taxa in Louisiana salt marshes that are potentially limited in distribution by specific salinity conditions. These data are not only useful by providing potential indicator taxa for salinity changes from future events such as freshwater releases or sea-level rise, but also for predicting what meiofaunal communities may look like before these changes occur.
Data availability statement
The datasets presented in this study can be found in online repositories. The names of the repository/repositories and accession number(s) can be found below: https://www.ncbi.nlm.nih.gov/bioproject/PRJNA706429.
Author contributions
LF and CH designed the study and obtained funding. LF supervised the field work, CH supervised the lab work and bioinformatics. BA and PR conducted the soil collection. PR performed DNA extractions and analyses. WT supervised next-generation sequencing and provided expertise in bioinformatics and meiofauna composition. CH, PR, BA, and LF wrote the manuscript.
Funding
This study was funded by a grant from the Gulf of Mexico Research Initiative Research Board (Grant Agreement No. 231613-00) to LF and CH.
Acknowledgments
We thank the team from the University of New Hampshire Hubbard Genome Center, for bioinformatics training during the 2017 BITMaB (Benthic Invertebrates Taxonomy, Metagenomics, and Bioinformatics Workshop) and for granting us access to their server and bioinformatics support staff. We thank Joseph Sevigny for assistance with bioinformatics. We also thank Mike Becker, Darrius Davis, Erin Stevens, and Julian Lucero for assistance in soil collections.
Conflict of interest
The authors declare that the research was conducted in the absence of any commercial or financial relationships that could be construed as a potential conflict of interest.
Publisher’s note
All claims expressed in this article are solely those of the authors and do not necessarily represent those of their affiliated organizations, or those of the publisher, the editors and the reviewers. Any product that may be evaluated in this article, or claim that may be made by its manufacturer, is not guaranteed or endorsed by the publisher.
Supplementary material
The Supplementary Material for this article can be found online at: https://www.frontiersin.org/articles/10.3389/fenvs.2022.950527/full#supplementary-material
References
Aker, B. G. (2020). The collection of baseline data on insect and plant communities across multiple salinity zones within Louisiana’s tidal marshes. LSU Master’s thesis. Baton Rouge, LA: Louisiana State University, 5203. Available at: https://digitalcommons.lsu.edu/gradschool_theses/5203.
Alves, A. S., Adão, H., Ferrero, T. J., Marques, J. C., Costa, M. J., and Patrício, J. (2013). Benthic meiofauna as indicator of ecological changes in estuarine ecosystems: The use of nematodes in ecological quality assessment. Ecol. Indic. 24, 462–475. doi:10.1016/j.ecolind.2012.07.013
Bam, W., Hooper-Bùi, L. M., Strecker, R. M., Adhikari, P. L., and Overton, B. (2018). Coupled effects of oil spill and hurricane on saltmarsh terrestrial arthropods. PLoS One 13, e0194941–16. doi:10.1371/journal.pone.0194941
Barbier, E. B., Hacker, S. D., Kennedy, C., Koch, E. W., Stier, A. C., and Silliman, B. R. (2011). The value of estuarine and coastal ecosystem services. Ecol. Monogr. 81, 169–193. doi:10.1890/10-1510.1
Bell, S. S. (1979). Short- and long-term variation in a high marsh meiofauna community. Estuar. Coast. Mar. Sci. 9, 331–350. doi:10.1016/0302-3524(79)90045-8
Benjamini, Y., and Hochberg, Y. (1995). Controlling the false discovery rate: A practical and powerful approach to multiple testing. J. R. Stat. Soc. Ser. B 57, 289–300. doi:10.1111/j.2517-6161.1995.tb02031.x
Benson, D. A., Karsch-Mizrachi, I., Lipman, D. J., Ostell, J., and Sayers, E. W. (2011). GenBank. GenBank. Nucleic Acids Res. 39, D32–D37. doi:10.1093/nar/gkq1079
Bik, H. M., Halanych, K. M., Sharma, J., and Thomas, W. K. (2012). Dramatic shifts in benthic microbial eukaryote communities following the deepwater horizon oil spill. PLoS One 7, e38550–6. doi:10.1371/journal.pone.0038550
Boesch, D., and Turner, R. (1984). Dependence of fishery species on salt marshes: The role of food and refuge. Estuaries 7, 460–468. doi:10.2307/1351627
Bolyen, E., Dillon, M., Bokulich, N., Abnet, C., Al-Ghalith, G., Alexander, H., et al. (2019). Qiime 2: Reproducible, interactive, scalable, and extensible microbiome data science. Nat. Biotechnol. 1, 852. doi:10.7287/peerj.preprints.27295
Brandt, M. I., Trouche, B., Quintric, L., Wincker, P., Poulain, J., and Arnaud-Haond, S. (2020). A flexible pipeline combining bioinformatic correction tools for prokaryotic and eukaryotic metabarcoding. bioRxiv 4, 717355. doi:10.1101/717355
Brannock, P. M., and Halanych, K. M. (2015). Meiofaunal community analysis by high-throughput sequencing: Comparison of extraction, quality filtering, and clustering methods. Mar. Genomics 23, 67–75. doi:10.1016/J.MARGEN.2015.05.007
Brannock, P. M., Waits, D. S., Sharma, J., and Halanych, K. M. (2014). High-throughput sequencing characterizes intertidal meiofaunal communities in northern gulf of Mexico (dauphin island and mobile bay, Alabama). Biol. Bull. 227, 161–174. doi:10.1086/BBLv227n2p161
Broman, E., Raymond, C., Sommer, C., Gunnarson, J. S., Creer, S., and Nascimiento, F. J. A. (2019). Salinity drives meiofaunal community structure dynamics across the Baltic ecosystem. Mol. Ecol. 28, 3813–3829. doi:10.1111/mec.15179
Bucklin, A., Steinke, D., and Blanco-Bercial, L. (2011). DNA barcoding of marine metazoa. Ann. Rev. Mar. Sci. 3, 471–508. doi:10.1146/annurev-marine-120308-080950
Cai, L., Fu, S., Yang, J., and Zhou, X. (2012). Distribution of meiofaunal abundance in relation to environmental factors in Beibu Gulf, South China Sea. Acta Oceanol. Sin. 31, 92–103. doi:10.1007/s13131-012-0256-2
Callahan, B. J., McMurdie, P. J., Rosen, M. J., Han, A. W., Johnson, A. J. A., and Holmes, S. P. (2016). DADA2: High-resolution sample inference from Illumina amplicon data. Nat. Methods 13, 581–583. doi:10.1038/nmeth.3869
Camacho, C., Coulouris, G., Avagyan, V., Ma, N., Papadopoulos, J., Bealer, K., et al. (2009). BLAST+: Architecture and applications. BMC Bioinforma. 10, 421. doi:10.1186/1471-2105-10-421
Caporaso, J. G., Lauber, C. L., Walters, W. A., Berg-Lyons, D., Huntley, J., Fierer, N., et al. (2012). Ultra-high-throughput microbial community analysis on the Illumina HiSeq and MiSeq platforms. Isme J. 6, 1621–1624. doi:10.1038/ismej.2012.8
Carugati, L., Corinaldesi, C., Dell’Anno, A., and Danovaro, R. (2015). Metagenetic tools for the census of marine meiofaunal biodiversity: An overview. Mar. Genomics 24, 11–20. doi:10.1016/J.MARGEN.2015.04.010
Chambers, J. M., Freeny, A., and Heiberger, R. M. (1992). “Analysis of variance; designed experiments,” in Statistical models in S (New York, NY: Routledge), 145–193.
Chao, A., and Jost, L. (2012). Coverage-based rarefaction and extrapolation: Standardizing samples by completeness rather than size. Ecology 93, 2533–2547. doi:10.1890/11-1952.1
Chao, A., and Lee, S. M. (1992). Estimating the number of classes via sample coverage. J. Am. Stat. Assoc. 87, 210–217. doi:10.1080/01621459.1992.10475194
Chao, A., Ma, K. H., Hsieh, T. C., and Chiu, C. H. (2015). Online program SpadeR (Species-richness prediction and diversity estimation in R). Program and user’s guide. Available at: http://chao.stat.nthu.edu.tw/wordpress/software_download/.
Chapman, V. J. (1977). Wet coastal ecosystems. Amsterdam: Elsevier Scientific Pub. Co. Available at: https://books.google.com/books?id=3kASAAAAYAAJ.
Chariton, A. A., Stephenson, S., Morgan, M. J., Steven, A. D., Colloff, M. J., Court, L. N., et al. (2015). Metabarcoding of benthic eukaryote communities predicts the ecological condition of estuaries. Environ. Pollut. 203, 165–174. doi:10.1016/j.envpol.2015.03.047
Coastal ProtectionRestoration Authority of Louisiana (2007). Integrated ecosystem restoration and hurricane protection: Louisiana’s comprehensive masterplan for a sustainable coast. LA: Baton Rouge.
Coastal ProtectionRestoration Authority of Louisiana (2012). Louisiana’s comprehensive master plan for a sustainable coast. LA: Baton Rouge.
Coastal ProtectionRestoration Authority of Louisiana (2017). Louisiana’s comprehensive master plan for a sustainable coast. LA: Baton Rouge.
Coull, B. C., and Bell, S. S. (1979). “Perspectives of marine meiofaunal ecology,” in Ecological processes in coastal and marine systems. Editor R. J. Livingston (Boston, MA: Springer), 189–216. doi:10.5860/crl_08_01_37
Coull, B. C. (1999). Role of meiofauna in estuarine soft-bottom habitats. Austral Ecol. 24, 327–343. doi:10.1046/j.1442-9993.1999.00979.x
Couvillion, B. R., Beck, H., Schoolmaster, D., and Fischer, M. (2017). Land area change in coastal Louisiana 1932 to 2016: U.S. Geological Survey Scientific Investigations Map 3381. Pamphlet, 16. doi:10.3133/sim3381
CPRA, (2018). Coastwide reference monitoring system-wetlands monitoring data. Available at: http://cims.coastal.louisiana.gov.
Creer, S., Deiner, K., Frey, S., Porazinska, D., Taberlet, P., Thomas, W. K., et al. (2016). The ecologist’s field guide to sequence-based identification of biodiversity. Methods Ecol. Evol. 7, 1008–1018. doi:10.1111/2041-210X.12574
Das, A., Justic, D., Inoue, M., Hoda, A., Huang, H., and Park, D. (2012). Impacts of Mississippi River diversions on salinity gradients in a deltaic Louisiana estuary: Ecological and management implications. Estuar. Coast. Shelf Sci. 111, 17–26. doi:10.1016/j.ecss.2012.06.005
Davis, D. M. (2022). Understanding the horse fly species and species relations of the Tabanus nigrovittatus complex along coastal Louisiana. LSU Master's thesis. Baton Rouge, LA: Louisiana State University, 5639. Available at: https://digitalcommons.lsu.edu/gradschool_theses/5639.
Deaton, L. E., and Greenberg, M. J. (1986). There is no horohalinicum. Estuaries 9, 20–30. doi:10.2307/1352189
Delaune, R. D., and Pezeshki, S. R. (1994). The Influence of Subsidence and Saltwater Intrusion on Coastal Marsh Stability:Louisiana Gulf Coast, U.S.A. Coast. hazards., 77–89.
DiBattista, J. D., Reimer, J. D., Stat, M., Masucci, G. D., Biondi, P., De Brauwer, M., et al. (2020). Environmental DNA can act as a biodiversity barometer of anthropogenic pressures in coastal ecosystems. Sci. Rep. 10, 8365. doi:10.1038/s41598-020-64858-9
Egge, E., Bittner, L., Andersen, T., Audic, S., de Vargas, C., and Edvardsen, B. (2013). 454 Pyrosequencing to Describe Microbial Eukaryotic Community Composition, Diversity and Relative Abundance: A Test for Marine Haptophytes. PLOS ONE 8, e74371. doi:10.1371/journal.pone.0074371
Elsey-Quirk, T., Graham, S. A., Mendelssohn, I. A., Snedden, G., Day, J. W., Twilley, R. R., et al. (2019). Mississippi river sediment diversions and coastal wetland sustainability : Synthesis of responses to freshwater , sediment , and nutrient inputs. Estuar. Coast. Shelf Sci. 221, 170–183. doi:10.1016/j.ecss.2019.03.002
Faith, D. P. (1992). Conservation evaluation and phylogenetic diversity. Biol. Conserv. 61, 1–10. doi:10.1016/0006-3207(92)91201-3
Fisk, H. N., and McFarlan, E. J. (1955). Late Quaternary deltaic deposits of the Mississippi River. Geol. Soc. Am. Spec. Pap. 62, 279–302. doi:10.1130/SPE62-p279
Fleeger, J., and Chandler, G. (1983). Meiofauna responses to an experimental oil spill in a Louisiana salt marsh. Mar. Ecol. Prog. Ser. 11, 257–264. doi:10.3354/meps011257
Fleeger, J. W., Carman, K. R., Riggio, M. R., Mendelssohn, I. A., Lin, Q. X., Hou, A., et al. (2015). Recovery of salt marsh benthic microalgae and meiofauna following the Deepwater Horizon oil spill linked to recovery of Spartina alterniflora. Mar. Ecol. Prog. Ser. 536, 39–54. doi:10.3354/meps11451
Fleeger, J. W., Gust, K. A., Marlborough, S. J., and Tita, G. (2007). Mixtures of metals and polynuclear aromatic hydrocarbons elicit complex, nonadditive toxicological interactions in meiobenthic copepods. Environ. Toxicol. Chem. 26, 1677–1685. doi:10.1897/06-397R.1
Fleeger, J. W. (1985). Meiofaunal densities and copepod species composition in a Louisiana, U.S.A., estuary. Trans. Am. Microsc. Soc. 104, 321–332. doi:10.2307/3226485
Fleeger, J. W., Riggio, M. R., Mendelssohn, I. A., Lin, Q., Deis, D. R., Johnson, D. S., et al. (2019). What promotes the recovery of salt marsh infauna after oil spills? Estuaries Coasts 42, 204–217. doi:10.1007/s12237-018-0443-2
Fleeger, J. W., Riggio, M. R., Mendelssohn, I. A., Lin, Q., Hou, A., and Deis, D. R. (2018). Recovery of saltmarsh meiofauna six years after the Deepwater Horizon oil spill. J. Exp. Mar. Biol. Ecol. 502, 182–190. doi:10.1016/j.jembe.2017.03.001
Fleeger, J. W., Whipple, S. A., and Cook, L. L. (1982). Field manipulations of tidal flushing, light exposure and natant macrofauna in a Louisiana salt marsh: Effects on the meiofauna. J. Exp. Mar. Biol. Ecol. 56, 87–100. doi:10.1016/0022-0981(81)90009-5
Fonseca, V. G., Cavalho, G. R., Sung, W., Johnson, H. F., Power, D. M., Neill, S. P., et al. (2010). Second-generation environmental sequencing unmasks marine metazoan biodiversity. Nat. Commun. 1, 98. doi:10.1038/ncomms1095
Gielings, R., Fais, M., Fontaneto, D., Creer, S., Costa, F. O., Renema, W., et al. (2021). DNA metabarcoding methods for the study of marine benthic meiofauna: A review. Front. Mar. Sci. 8, 730063. doi:10.3389/fmars.2021730063
Giere, O. (2009). Meiobenthology: The microscopic motile fauna of aquatic sediments. Berlin: Springer-Verlag.
Harrison, J. B., Sunday, J. M., and Rogers, S. M. (2019). Predicting the fate of eDNA in the environment and implications for studying biodiversity. Proc. R. Soc. B 286, 20191409–20191780. doi:10.1098/rspb.2019.1409
Healy, B., and Walters, K. (1994). Oligochaeta in Spartina stems: the microdistribution of Enchytraeidae and Tubificidae in a salt marsh, Sapelo Island, USA. Hydrobiologia 278, 111–123. doi:10.1007/BF00142318
Hodda, M. (2011). Phylum Nematoda cobb,” in Animal biodiversity: An outline of higher-level classification and survey of taxonomic richness, 63–95.Zootaxa)
Holland, M. M., Whigham, D. F., and Gopal, B. (1990). “The characteristics of wetland ecotones,” in The ecology and management of aquatic-terrestrial ecotones. Editors R. J. Naiman, and H. Décamps (Park Ridge, NJ: The Pantheon Publishing Group Inc), 171–198.
Horton, T., Kroh, A., Ahyong, S., Bailly, N., Bieler, R., Boyko, C. B., et al. (2022). World Register of Marine Species (WoRMS). Available at: https://www.marinespecies.org.
Hribar, L. J., Leprince, D. J., and Foil, L. D. (1991). Design for a canopy trap for collecting horse flies (Diptera: Tabanidae). J. Am. Mosq. Control Assoc. 7. Available at: http://europepmc.org/abstract/MED/1787413. 657.
Hsieh, T. C., Ma, K. H., and Chao, A. (2016). iNEXT: an R package for rarefaction and extrapolation of species diversity (Hill numbers). Methods Ecol. Evol. 7, 1451–1456. doi:10.1111/2041-210X.12613
Husseneder, C., Bhalerao, D. R., and Foil, L. D. (2022). Was the decline of saltmarsh tabanid populations after the 2010 oil spill associated with change in the larval food web? Ecosphere 13. in press. doi:10.1002/ecs2.4157
Husseneder, C., Donaldson, J. R., and Foil, L. D. (2016). Impact of the 2010 Deepwater Horizon oil spill on population size and genetic structure of horse flies in Louisiana marshes. Sci. Rep. 6, 18968. doi:10.1038/srep18968
Husseneder, C., Park, J. S., and Foil, L. D. (2018). Recovery of horse fly populations in Louisiana marshes following the Deepwater Horizon oil spill. Sci. Rep. 8 (1), 13777. doi:10.1038/s41598-018-31442-1
Jacquiod, S., Stenbæk, J., Santos, S. S., Winding, A., Sørensen, S. J., and Priemé, A. (2016). Metagenomes provide valuable comparative information on soil microeukaryotes. Res. Microbiol. 167, 436–450. doi:10.1016/j.resmic.2016.03.003
Katoh, K., Misawa, K., Kuma, K., and Miyata, T. (2002). Mafft: a novel method for rapid multiple sequence alignment based on fast Fourier transform. Nucleic Acids Res. 30, 3059–3066. doi:10.1093/nar/gkf436
Kennedy, A. D., and Jacoby, C. A. (1999). Biological Indicators of Marine Environmental Health: Meiofauna – A Neglected Benthic Component? Environ. Monit. Assess. 54, 47–68. doi:10.1023/A:1005854731889
Khanna, S., Santos, M. J., Koltunov, A., Shapiro, K. D., Lay, M., and Ustin, S. L. (2017). Marsh loss due to cumulative impacts of hurricane isaac and the DeepWater Horizon oil spill in Louisiana. Remote Sens. 9 (2), 169. doi:10.3390/rs9020169
Kirwan, M. L., Temmerman, S., Skeehan, E. E., Guntenspergen, G. R., and Fagherazzi, S. (2016). Overestimation of marsh vulnerability to sea level rise. Nat. Clim. Chang. 6, 253–260. doi:10.1038/nclimate2909
Kneib, R. T. (1984). Patterns of invertebrate distribution and abundance in the intertidal salt marsh: Causes and questions. Estuaries 7, 392–412. doi:10.2307/1351621
Kruskal, W. H., and Wallis, W. A. (1952). Use of Ranks in One-Criterion Variance Analysis. J. Am. Stat. Assoc. 47, 583–621. doi:10.1080/01621459.1952.10483441
Lane, D. J. (1991). “16S/23S rRNA Sequencing,” in Nucleic acid techniques in bacterial systematics (New York, NY, USA: John Wiley & Sons), 115–175.
Leasi, F., Sevigny, J. L., Laflamme, E. M., Artois, T., Curini-Galletti, M., de Jesus Navarrete, A., et al. (2018). Biodiversity estimates and ecological interpretations of meiofaunal communities are biased by the taxonomic approach. Commun. Biol. 1, 112–12. doi:10.1038/s42003-018-0119-2
Lin, Q., and Mendelssohn, I. A. (2012). Impacts and recovery of the deepwater horizon oil spill on vegetation structure and function of coastal salt marshes in the northern gulf of Mexico. Environ. Sci. Technol. 46, 3737–3743. doi:10.1021/es203552p
MacKenzie, R. A., and Dionne, M. (2008). Habitat heterogeneity : importance of salt marsh pools and high marsh surfaces to fish production in two Gulf of Maine salt marshes. Mar. Ecol. Prog. Ser. 368, 217–230. doi:10.3354/meps07560
MacKenzie, R. A., Dionne, M., MillerHaas, J. M., and Morgan, P. A. (2015). Community structure and abundance of benthic infaunal invertebrates in Maine fringing marsh ecosystems, 38, 1317. doi:10.1007/s12237-015-9977-8Estuaries Coasts
McCall, B. D., and Pennings, S. C. (2012). Disturbance and recovery of salt marsh arthropod communities following BP Deepwater Horizon oil spill. PLOS ONE 7, e32735. doi:10.1371/journal.pone.0032735
McMurdie, P. J., and Paulson, J. N. (2020). biomformat: An interface package for the BIOM file format. https://github.com/joey711/biomformat/.
MEA (2005). Ecosystem and human well-being: Current state and trends. Washington, DC, USA: Coastal Systems.
Mendelssohn, I. A., Andersen, G. L., Baltz, D. M., Caffey, R. H., Carman, K. R., Fleeger, J. W., et al. (2012). Oil impacts on coastal wetlands: Implications for the Mississippi River Delta ecosystem after the Deepwater Horizon Oil Spill. Bioscience 62, 562–574. doi:10.1525/bio.2012.62.6.7
Michel, J., Owens, E. H., Zengel, S., Graham, A., Nixon, Z., Allard, T., et al. (2013). Extent and Degree of Shoreline Oiling: Deepwater Horizon Oil Spill, Gulf of Mexico, USA. PLoS One 8, e65087–9. doi:10.1371/journal.pone.0065087
Minello, T. J. (2000). Temporal development of salt marsh value for nekton and epifauna: Utilization of dredged material marshes in Galveston Bay, Texas, USA. Wetl. Ecol. Manag. 8, 327–342. doi:10.1023/A:1008487303992
Minello, T. J., and Webb, J. W. (1997). Use of natural and created Spartina alterniflora salt marshes by fishery species and other aquatic fauna in Galveston Bay, Texas, USA. Mar. Ecol. Prog. Ser. 151, 165–179. doi:10.3354/meps151165
Minowa, A. K., and Garraffoni, A. R. S. (2017). A new species of Haltidytes Remane, 1936 (Gastrotricha: Chaetonotida: Dasydytidae) from an urban lagoon in Brazil with a phylogenetic reconstruction of the genus based on morphological data. Zool. Anz. 269, 100–109. doi:10.1016/j.jcz.2017.08.003
Montagna, P. A., Kalke, R. D., and Ritter, C. (2002). Effect of restored freshwater inflow on macrofauna and meiofauna in upper Rincon Bayou, Texas, USA. Estuaries 25, 1436–1447. doi:10.1007/bf02692237
Montagna, P. A., and Kalke, R. D. (1992). The effect of freshwater inflow on meiofaunal and macrofaunal populations in the Guadalupe and Nueces Estuaries, Texas. Estuaries 15, 307–326. doi:10.2307/1352779
Ngo, X. Q., Smol, N., and Vanreusel, A. (2013). The meiofauna distribution in correlation with environmental characteristics in 5 Mekong estuaries, Vietnam. Cah. Biol. Mar. 54, 71–83. doi:10.21411/cbm.a.ec530b25
NOAA (2018). Environmental Response Management Application, Gulf of Mexico. Available at: https://erma.noaa.gov/gulfofmexico/erma.html%0A.
Oksanen, J., Blanchet, F. G., Friendly, M., Kindt, R., Legendre, P., McGlinn, D., et al. (2018). Vegan: Community ecology. Available at: https://cran.r-project.org/package=vegan.
Palaseanu-Lovejoy, M., Kranenburg, C., Barras, J. A., and Brock, J. C. (2013). Land Loss Due to Recent Hurricanes in Coastal Louisiana, U.S.A. J. Coast. Res. 63, 97–109. doi:10.2112/si63-009.1
Penland, S., and Ramsey, K. E. (1990). Relative Sea-Level Rise in Louisiana and the Gulf of Mexico : 1908-1988. J. Coast. Res. 6, 323–342.
Peyronnin, N., Green, M., Richards, C. P., Owens, A., Reed, D., Chamberlain, J., et al. (2013). Louisiana’s 2012 Coastal Master Plan: Overview of a science-based and publicly informed decision-making process. J. Coast. Res. 67, 1–15. doi:10.2112/si_67_1.1
Phillips, J. D., Gillis, D. J., and Hanner, R. H. (2019). Incomplete estimates of genetic diversity within species: Implications for DNA barcoding. Ecol. Evol. 9, 2996–3010. doi:10.1002/ece3.4757
Plantard, O., Valette, S., and Gross, M. F. (2007). The root-knot nematode producing galls on Spartina alterniflora belongs to the genus Meloidogyne: Rejection of Hypsoperine and Spartonema spp. J. Nematol. 39, 127–132.
Porazinska, D. L., Giblin-Davis, R. M., Faller, L., Farmerie, W., Kanzaki, N., Morris, K., et al. (2009). Evaluating high-throughput sequencing as a method for metagenomic analysis of nematode diversity. Mol. Ecol. Resour. 9, 1439–1450. doi:10.1111/j.1755-0998.2009.02611.x
Porazinska, D. L., Sung, W., Giblin-Davis, R. M., and Thomas, W. K. (2010). Reproducibility of read numbers in high-throughput sequencing analysis of nematode community composition and structure. Mol. Ecol. Resour. 10, 666–676. doi:10.1111/j.1755-0998.2009.02819.x
Posey, M. H., Alphin, T. D., Harwell, H., and Allen, B. (2005). Importance of low salinity areas for juvenile blue crabs, Callinectes sapidus Rathbun, in river-dominated estuaries of southeastern United States. J. Exp. Mar. Biol. Ecol. 319, 81–100. doi:10.1016/j.jembe.2004.04.021
Price, M. N., Dehal, P. S., and Arkin, A. P. (2010). FastTree 2 - Approximately maximum-likelihood trees for large alignments. PLoS One 5, e9490. doi:10.1371/journal.pone.0009490
Quast, C., Pruesse, E., Yilmaz, P., Gerken, J., Schweer, T., Yarza, P., et al. (2013). The SILVA ribosomal RNA gene database project: improved data processing and web-based tools. Nucleic Acids Res. 41, D590–D596. doi:10.1093/nar/gks1219
Quensen, J. (2020). ggordiplots: Make ggplot versions of Vegan’s ordiplots. Available at: http://github.com/jfq3/ggordiplots.
Rabalais, N. N., and Turner, R. E. (2016). Effects of the Deepwater Horizon Oil Spill on Coastal Marshes and Associated Organisms. Oceanogr. Wash. D. C). 29, 150–159. doi:10.5670/oceanog.2016.79
Rayle, P. M. (2021). A comparison of intertidal metazoan biodiversity between previously oiled sheared and intact marsh margins and between multiple salinity zones in the coastal marshes of Louisiana. LSU Master's thesis. Baton Rouge, LA: Louisiana State University, 5287. Available at: https://digitalcommons.lsu.edu/gradschool_theses/5287.
Rho, H. S., Min, W. G., Decraemer, W., and Kim, D. S. (2010). Prochaetosoma dokdoense sp. nov. (Nematoda: Draconematidae) from Dokdo, Korea: First record of the genus Prochaetosoma from a shallow subtidal zone in the northwest Pacific Ocean. Mar. Biol. Res. 6, 172–188. doi:10.1080/17451000903078622
Rivera-Ingraham, G. A., Nommick, A., Blondeau-Bidet, E., Ladurner, P., and Lignot, J. H. (2016). Salinity stress from the perspective of the energy-redox axis: lessons from a marine intertidal flatworm. Redox Biol. 10, 53–64. doi:10.1016/j.redox.2016.09.012
Roberts, H. H., and Coleman, J. M. (1996). Holocene evolution of the deltaic plain: A perspective - From Fisk to present. Eng. Geol. 45, 113–138. doi:10.1016/s0013-7952(96)00010-5
Rozas, L. P., and Minello, T. J. (2011). Variation in penaeid shrimp growth rates along an estuarine salinity gradient: Implications for managing river diversions. J. Exp. Mar. Biol. Ecol. 397, 196–207. doi:10.1016/j.jembe.2010.12.003
Sakata, M. K., Yamamoto, S., Gotoh, R. O., Miya, M., Yamanaka, H., and Minamoto, T. (2020). Sedimentary eDNA provides different information on timescale and fish species composition compared with aqueous eDNA. Environ. DNA 2, 505–518. doi:10.1002/edn3.75
Schratzberger, M., and Ingels, J. (2018). Meiofauna matters: The roles of meiofauna in benthic ecosystems. J. Exp. Mar. Biol. Ecol. 502, 12–25. doi:10.1016/j.jembe.2017.01.007
Semprucci, F., Sbrocca, C., Rocchi, M., and Balsamo, M. (2015). Temporal changes of the meiofaunal assemblage as a tool for the assessment of the ecological quality status. J. Mar. Biol. Assoc. U. K. 95, 247–254. doi:10.1017/S0025315414001271
Silliman, B. R., Van De Koppel, J., McCoy, M. W., Diller, J., Kasozi, G. N., Earl, K., et al. (2012). Degradation and resilience in Louisiana salt marshes after the BP-Deepwater Horizon oil spill. Proc. Natl. Acad. Sci. U. S. A. 109, 11234–11239. doi:10.1073/pnas.1204922109
Snelgrove, P. V. R., Henry Blackburn, T., Hutchings, P. A., Alongi, D. M., Frederick Grassle, J., Hummel, H., et al. (1997). The importance of marine sediment biodiversity in ecosystem processes. Ambio 26, 578–583.
Soetaert, K., Vincx, M., Wittoeck, J., and Tulkens, M. (1995). Meiobenthic distribution and nematode community structure in five European estuaries. Hydrobiologia 311, 185–206. doi:10.1007/BF00008580
Sørensen, T. (1948). A method of establishing groups of equal amplitude in plant sociology based on similarity of species content. Bull. Soc. Plant Ecol. 5, 1–34. doi:10.18960/bse.1.1_56_1
Taberlet, P., Coissac, E., Pompanon, F., Brochmann, C., and Willerslev, E. (2012). Towards next-generation biodiversity assessment using DNA metabarcoding. Mol. Ecol. 21, 2045–2050. doi:10.1111/j.1365-294x.2012.05470.x
Tran, T. T., Nguyen Le, Q. L., Le, H. D., Nguyen, T. M. Y., and Ngo, X. Q. (2018). Intertidal meiofaunal communities in relation to salinity gradients in the Ba Lai river, Vietnam. J. Viet. Env. 10, 138–150. doi:10.13141/jve.vol10.no2.pp138-150
UNEP (2006). Marine and coastal ecosystems and human well-being: A synthesis report based on the findings of the millenium ecosystem Assessment. Nairobi, Kenya.
Van Diggelen, A. D., and Montagna, P. A. (2016). Is salinity variability a benthic disturbance in Estuaries?. Estuar. Coasts 39, 967–980. doi:10.1007/s12237-015-0058-9
Van Regteren, M., Amptmeijer, D., de Groot, A. V., Baptist, M. J., and Elschot, K. (2020). Where does the salt marsh start? Field-based evidence for the lack of a transitional area between a gradually sloping intertidal flat and salt marsh. Estuar. Estuar. Coast. Shelf Sci. 243, 106909. doi:10.1016/j.ecss.2020.106909
Veenstra-Quah, A. A., Milne, J., and Kolesik, P. (2007). Taxonomy and biology of two new species of gall midge (Diptera: Cecidomyiidae) infesting Sarcocornia quinqueflora (Chenopodiaceae) in Australian salt marshes. Aust. J. Entomol. 46, 198–206. doi:10.1111/j.1440-6055.2007.00603.x
Warwick, R. M. (1981). “Survival Strategies of Meiofauna,” in Feeding and survival srategies of estuarine organisms (Boston, MA: Springer US), 39–52. doi:10.1007/978-1-4613-3318-0_4
Wong, W. H., Rabalais, N. N., and Turner, R. E. (2010). Abundance and ecological significance of the clam Rangia cuneata (Sowerby, 1831) in the upper Barataria Estuary (Louisiana, USA). Hydrobiologia 651, 305–315. doi:10.1007/s10750-010-0310-z
Yuill, B., Lavoie, D., and Reed, D. J. (2009). Understanding subsidence processes in coastal Louisiana. J. Coast. Res. 10054, 23–36. doi:10.2112/SI54-012.1
Zaiko, A., Pochon, X., Garcia-Vazquez, E., Olenin, S., and Wood, S. A. (2018). Advantages and Limitations of Environmental DNA/RNA Tools for Marine Biosecurity: Management and Surveillance of Non-indigenous Species. Front. Mar. Sci. 5, 322. doi:10.3389/fmars.2018.00322
Zengel, S., Bernik, B. M., Rutherford, N., Nixon, Z., and Michel, J. (2015). Heavily oiled salt marsh following the deepwater horizon oil spill, ecological comparisons of shoreline cleanup treatments and recovery. PLoS One 10, e0132324–27. doi:10.1371/journal.pone.0132324
Zeppilli, D., Leduc, D., Fontanier, C., Fontaneto, D., Fuchs, S., Gooday, A. J., et al. (2018). Characteristics of meiofauna in extreme marine ecosystems: a review. Mar. Biodiv. 48, 35–71. doi:10.1007/s12526-017-0815-z
Zeppilli, D., Sarrazin, J., Leduc, D., Arbizu, P. M., Fontaneto, D., Fontanier, C., et al. (2015). Is the meiofauna a good indicator for climate change and anthropogenic impacts? Mar. Biodivers. 45, 505–535. doi:10.1007/s12526-015-0359-z
Keywords: 18S metabarcoding, next-generation sequencing, environmental DNA, meiofauna, soil organisms
Citation: Husseneder C, Rayle P, Aker BG, Thomas WK and Foil LD (2022) A comparison of intertidal metazoan biodiversity among different salinity zones of Louisiana coastal marshes. Front. Environ. Sci. 10:950527. doi: 10.3389/fenvs.2022.950527
Received: 23 May 2022; Accepted: 22 August 2022;
Published: 12 September 2022.
Edited by:
Jill A. Olin, Michigan Technological University, United StatesReviewed by:
Travis William Washburn, Geological Survey of Japan (AIST), JapanLi Wen, NSW Department of Planning, Industry, and Environment, Australia
Copyright © 2022 Husseneder, Rayle, Aker, Thomas and Foil. This is an open-access article distributed under the terms of the Creative Commons Attribution License (CC BY). The use, distribution or reproduction in other forums is permitted, provided the original author(s) and the copyright owner(s) are credited and that the original publication in this journal is cited, in accordance with accepted academic practice. No use, distribution or reproduction is permitted which does not comply with these terms.
*Correspondence: Claudia Husseneder, Y2h1c3NlbmVkZXJAYWdjZW50ZXIubHN1LmVkdQ==