- 1School of Renewable Natural Resources, Louisiana State University and Agricultural Center, Baton Rouge, LA, United States
- 2Department of Biological Sciences, California State Polytechnic University, Pomona, Pomona, CA, United States
- 3Department of Biology, Austin Peay State University, Clarksville, TN, United States
The 2010 Deepwater Horizon (DWH) disaster led to extensive oil deposition in Louisiana’s coastal wetlands, followed two years later by Hurricane Isaac. These disturbances led to changes in saltmarsh invertebrate communities, potentially affecting higher-level predators and the saltmarsh food web. Seaside Sparrows (Ammospiza maritima) are ubiquitous, year-round residents of the coastal saltmarsh affected by the DWH spill, where they consume diverse invertebrates and may be considered an indicator species for the ecosystem’s integrity. We used DNA metabarcoding to evaluate prey consumed by Seaside Sparrows to understand how sparrows responded to residual contamination from the DWH oil spill and ecosystem disturbance caused by Hurricane Isaac. To do so, we evaluated metrics of diet (prey richness, diversity, overall diet composition) and resource use (total niche width, individual specialization) from 2011 to 2017 on oiled, unoiled, and reference sites. We found that while diet composition varied across years and site type, Hurricane Isaac had an even greater effect on the richness and diversity of prey consumed. Resource use—as measured by the total niche width of the populations and degree of individual specialization—was most stable on unoiled sites compared to unoiled and reference sites. Finally, we analyzed resource use for each combination of site type and year (i.e.: “2014 oiled sites”), which indicated a strong correlation between individual specialization and total niche width: as total niche width increased, individuals became more specialized, following the predictions of the Niche Variation Hypothesis.
1 Introduction
In an effort to understand the resource use of populations, Van Valen (1965) proposed the niche variation hypothesis (NVH), suggesting that in the absence of interspecific competition, the overall suite of resources used by a population—or the total niche width (TNW)—will broaden as individuals take advantage of available resources. The NVH posits that unique phenotypes of individuals (or behavioral differences; Werner and Sherry 1987; Toscano et al., 2016) will drive them to consume unique prey—that is, individuals will specialize on resources suited to their unique morphology or cognitive ability and the overall diversity of prey consumed by the population will increase, leading to a broader TNW (Van Valen 1965). Since the width of a trophic niche is driven by the number of resources used, specialist species are often assumed to have narrow TNW, while generalist species have broader TNW (though see Peers et al., 2012).
Dietary niche is one important component in determining how species cope with ecosystem disturbance (Graham et al., 2011; Cloyed et al., 2021). Trophic generalists consume a variety of prey and can adapt to changing prey communities through foraging plasticity, with individuals switching between food sources when resources are scarce. This flexibility can allow generalist species to persist despite changes in their environment (Swihart et al., 2003). Trophic specialists, on the other hand, may struggle to survive if their preferred resources become unavailable (Clavel et al., 2011). The evolution of these trophic strategies may be driven by a variety of pressures, including intra- and interspecific competition, resource availability, and environmental factors. Ecosystem heterogeneity is one such factor, with spatially and temporally stable ecosystems favoring the evolution of specialists and more heterogenous or unpredictable environments leading to the evolution of generalists (Kassen, 2002). Disturbance can create environmental heterogeneity by altering habitat and the structure of prey communities through time and space, ultimately influencing diets of higher predators (Waide, 1991; Alves et al., 2016; Trevelline et al., 2018). In ecosystems where disturbances are frequent, selection should favor generalist strategies over specialist strategies (Waide, 1991).
Traditionally, ecological studies assumed all individuals in a generalist population sample the full suite of resources used by the population (Colwell and Futuyma, 1971; Pielou, 1972). However, it has become increasingly appreciated that individual variation and specialization within generalist populations may be more common—and important—than previously recognized (Durell, 2000; DeSantis et al., 2022). Within a generalist population, individuals may specialize to take advantage of distinct resources (type A generalists, as predicted by the NVH); individuals may share the same, broad set of resources (type B generalists); or the population may be composed of individuals that fall somewhere in between these two extremes (Bolnick et al., 2003).
Here, we present a case study on the response of the Seaside Sparrow (Ammospiza maritima) to anthropogenic and natural disturbances, for which we used DNA metabarcoding to identify prey consumed and evaluate resource use. Seaside Sparrows are year-round residents of the coastal saltmarshes of southeast Louisiana, an area that experiences frequent large-scale storms (Keim et al., 2007). These saltmarshes were also affected by the 2010 Deepwater Horizon (DWH) oil spill disaster, which led to the oiling of 1,105 km of wetlands, with the majority (1,055 km) in Louisiana (Nixon et al., 2016). This created justifiable concern for the coastal ecosystem, as Louisiana is an important stopover site for migratory birds and home to many resident species, including approximately 3.5 million non-migratory Seaside Sparrows, comprising ∼55% of their global population (Remsen et al., 2019). Only two years later, in August 2012, Hurricane Isaac made landfall. The storm inundated coastal Louisiana for several days and was later found to have redistributed oil that had come ashore from the DWH disaster (Turner et al., 2014; Zengel et al., 2015). Invertebrate communities changed following both saltmarsh oiling and hurricane disturbance (McCall and Pennings, 2012; Zengel et al., 2015; Bam et al., 2018). Accordingly, resources used by Seaside Sparrows may have changed as a result: for example, sparrows may have had access to fewer species of prey, or the reduced availability of prey may have led to an increase in competition. That is, competition might be more intense on sites with reduced prey (i.e. poorer quality sites, like oiled sites) compared to sites with more robust invertebrate communities (i.e. higher quality sites, like unoiled sites).
To assess the effect of oil contamination and hurricane disturbance on the coastal saltmarsh food web, we quantified the diets of Seaside Sparrows from 2011 to 2017 on sites with varying degrees of oil contamination by evaluating dietary diversity, richness, and overall diet composition. We compared the degrees of individual specialization to the total niche width (TNW) of Seaside Sparrows on sites throughout the study period to determine if sparrow resource use follows the predictions of NVH, that is, whether individuals specialize when competition is reduced.
2 Methods
2.1 Study sites
Sites were chosen as part of a larger project studying the effects of the Deepwater Horizon oil spill and were based on the degree of shoreline oiling as determined by the National Oceanic and Atmospheric Administration (NOAA, 2013) Shoreline Cleanup Assessment Technique (SCAT; NOAA 2013; Michel et al., 2013). SCAT maps from 3 August 2010 were used to identify oiled (n = 5) and unoiled (n = 6) sites in Bays Batiste and Sansbois, LA (both located within the larger Barataria Bay). After Hurricane Isaac (2012), additional sites (n = 3) were added near Pointe à la Hache, LA on the northeast side of the Mississippi River to serve as an outgroup in the event that the hurricane redistributed oil in Barataria Bay (Figure 1).
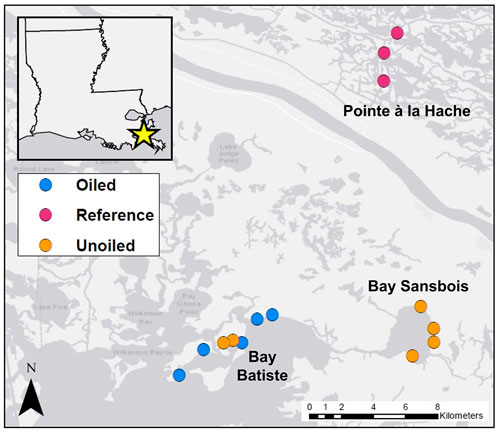
FIGURE 1. Study sites in southeastern Louisiana. Main study sites are located in Bay Batiste and nearby Bay Sansbois. Additional sites were added on the northeastern side of the Mississippi River in 2013, after Hurricane Isaac affected the entire area in 2012.
Seaside Sparrows exhibit high site fidelity, with some birds even returning to their original capture location after Hurricane Isaac (Stouffer et al., 2013). To date, no research has been conducted on the individual breeding territory or home range size of Seaside Sparrows in Louisiana; throughout their range, territory size varies from 1,203 m2 (Oak Beach, New York; Post and Greenlaw, 1975) to 36,000 m2 (Ochopee, Florida; Werner and Woolfenden, 1983), with variation between these sizes at different locations (Baker, 1973; Werner, 1975; Post et al., 1983; DeRagon, 1988; Marshall and Reinert, 1990). Additionally, Seaside Sparrows will forage outside of their territory during nesting periods to provision for their young, but the distance travelled was rarely over 100 m from the center of their territory in New York (Post, 1974). Given this, sites in this study were selected to minimize the possibility of individuals traveling between locations; our closest sites were approximately 1 km apart.
2.2 Sample collection
Adult Seaside Sparrows were collected in August 2011 and June 2012–2017. Birds were euthanized and dissected to collect tissues for multiple studies (Olin et al., 2017; Perez-Umphrey et al., 2018; Bonisoli-Alquati et al., 2020; Moyo et al., 2021). In all years except 2013, stomach contents were immediately removed from the stomach in the field before being flash frozen and stored in liquid nitrogen, then transferred to long-term storage at -80°C. In 2013, stomachs were flash frozen whole, with the contents inside. For samples collected in 2013, the contents were removed in the lab using clean scalpels and forceps. A total of 168 stomach content samples were collected throughout the study period (2011: n = 9, 2012: n = 19, 2013: n = 25, 2014: n = 27, 2015: n = 22, 2016: n = 33, 2017: n = 33).
2.3 DNA extraction and amplification
DNA extraction followed Vo & Jedlicka (2014); a detailed description can be found in Snider et al. (2022). The entire sample was extracted to avoid subsampling, which can lead to reduced prey detection and incomplete description of the diet (Oehm et al., 2011; Jedlicka et al., 2013). In brief, stomach contents were first weighed, and samples over 50 mg were divided into multiple extractions. For samples split into multiple extractions, the final DNA elutions were combined. All extractions included a negative control. DNA concentration was quantified with the Denovix Fluorescence dsDNA High Sensitivity Assay kit (Denovix Inc. Wilmington, DE) and samples below 0.2 ng/μL were concentrated in a vacuufuge (Savant DNA 120 SpeedVac Concentrator, Thermo Scientific) for 20 min on medium heat.
PCRs targeted two markers: COI, an amplicon from the mitochondrial genome, and 18s, an amplicon from nuclear DNA that codes for 18s rRNA. These markers were selected to complement each other, because together they detect prey across a wide range of taxa (da Silva et al., 2019). Generally, COI is useful for high-resolution prey identification (Clarke et al., 2017) but is limited in the breadth of prey it can detect. 18s is a more conserved marker that is less suited for high-resolution prey identification (Tang et al., 2012) but is able to identify a wider breadth of prey. Seaside Sparrows are a generalist species, and despite consuming both plants and animals they are thought to primarily feed on insects and other invertebrates during the summer, when samples were collected (Martin et al., 1961; Post, 1974; Post and Greenlaw, 2006). With this a priori knowledge of the diet, the Zeale et al. (2011) arthropod-specific primer set was chosen to identify arthropod prey, coupled with the Jarman et al. (2013) 18s primer set to identify non-arthropod organisms (i.e., da Silva et al., 2019).
PCR reactions and thermocycler conditions for COI followed Jedlicka et al. (2016). PCR reactions consisted of 1X Phusion GC Buffer, 200 µM dNTPs, 5% DMSO, 0.5 µM of each primer modified with Illumina overhangs (ZBJ-ArtF1c and ZBJ-ArtR2c; Zeale et al., 2011), 1 U Phusion High-Fidelity DNA Polymerase (New England Biolabs, Ipswich, MA, United States), 0.004–4.25 ng DNA, and nH2O to 20 µl. COI thermocycler conditions followed an initial denaturation step of 98°C for 2 min; 35 cycles of 98°C for 8 s, 48.5°C for 20 s, 72°C for 30 s; and a final extension step of 72°C for 7 min. PCR reactions for 18s consisted of 1X NEBNext Ultra II Q5 master mix, 0.5 µM of each primer modified with Illumina overhangs (SSU3′F and SSU3′R; Jarman et al., 2013), 0.01–8.5 ng DNA, and nH2O to 10 µl. Thermocycler conditions for 18s followed an initial denaturation step of 98°C for 30 s; 35 cycles of 98°C for 15 s, 45°C for 30 s, 72°C for 30 s; and a final extension step of 72°C for 2 min. Both COI and 18s primers were modified to include Illumina-specific overhangs to anneal sample-specific indexes during library preparation (Illumina, 2013). PCRs were performed in triplicate, with resulting PCR products pooled before remaining library preparation steps in an effort to increase prey detection (Jedlicka et al., 2016; Alberdi et al., 2019). Negative controls were included with each PCR.
2.4 Library preparation and sequencing
Libraries were prepared following a two-step PCR protocol (Illumina, 2013). In the first PCR, detailed above, DNA was amplified by primers modified with Illumina-specific overhangs. In the second PCR, unique combinations of Illumina Nextera XT indexes (v2) (Illumina, San Diego, CA, United States) were annealed to each sample using an enrichment PCR (detailed in: Illumina 2013). For additional details on library preparation, see Snider et al. (2022). Since 18s amplicon size differed from COI, the SPRI cleanups were 2.1x and 1.5x SPRI:product concentrations, respectively. The samples used in this study were split across two sequencing lanes: the first included all COI amplicons, while the second contained all 18s amplicons. Both pooled libraries were sequenced at the Genomics Core Facility at the Pennington Biomedical Research Center (Baton Rouge, LA, United States) and quantified on an Agilent Bioanalyzer. Both libraries were spiked with 15% PhiX before being sequenced (2 × 250 bp) on an Illumina MiSeq platform with v2 reagent kit (Illumina). Reads were de-multiplexed by the sequencing facility and raw reads are available on the NCBI Sequence Read Archive (https://www.ncbi.nlm.nih.gov/sra).
2.5 Bioinformatics and statistical analysis
De-multiplexed reads were imported into QIIME2 version 2021.2 (Bolyen et al., 2019) and processed. QIIME2 is a wrapper and the following programs were used within the QIIME2 pipeline: first, primer sequences were trimmed and any reads not containing these sequences were removed using Cutadapt (Martin, 2011). Next, trimmed reads were quality filtered with DADA2 (Callahan et al., 2016): chimeras were removed, Phred score quality cutoffs were implemented (COI: 30, 18s: 25), paired end reads were merged, and identical reads were collapsed into amplicon sequence variants (ASVs). The Phred cutoffs differed by marker because read quality was lower with the 18s primer set, likely because this marker is intended to cover a broader range of organisms. Finally, singletons—or ASVs that appeared only once in the dataset—were removed before taxonomy was assigned (Burgar et al., 2014).
To assign taxonomy to ASVs, two naive Bayes classifiers were trained (Bokulich et al., 2018). The 18s classifier was based on a custom 18s dataset of plant, algae, and metazoan sequences and the COI classifier was comprised of arthropod orders previously found to make up Seaside Sparrow diet (Snider et al., 2022). Both training datasets were retrieved from NCBI’s GenBank using RESCRIPt (Robeson II et al., 2021); training datasets were then trimmed before each classifier was trained in order to improve ASV identification, and function feature-classifier fit-classifier-naive-bayes was used to train the classifiers. Once ASVs were assigned taxonomic IDs with their respective classifiers, unassigned reads were removed, as were ASVs that matched to Aves and Mammalia. Next, taxonomic ranks were edited in the following way: for COI, identifications were edited to follow Zeale et al. (2011) so that species-level IDs were made when they matched a reference at 99.4–100%, genus at 95.0–99.3%, family at 91.1–94.9%, and order at 86.0–91.0%; any taxonomic assignment with less than 86% confidence was removed. For 18s, reads were edited to follow Holovachov et al. (2017), so that species-level IDs were made when they matched a reference at 99.0–100%, genus at 96.5–98.9%, family at 90.0–96.4%, and order at 84.0–89.9%; any taxonomic assignment with less than 86% confidence was removed. Next, ASVs identified in negative controls were removed, duplicate ASVs were collapsed, and resulting read tables were imported into R (R Core Team, 2019), using R Studio (version 2022.02.3; RStudio Team 2020) for further analysis. To create the combined dataset, duplicate ASVs that were detected by both markers were collapsed within each sample.
Datasets were first visualized with an NMDS plot, accompanied by the permutational ANOVA, Adonis, to test for significance. Both the NMDS and Adonis were performed on a presence-absence matrix of all ASVs in the diet using Bray-Curtis dissimilarity in vegan (Oksanen et al., 2019). In addition to evaluating year and site type, the NMDS and Adonis were also performed to evaluate “bay” (that is, the bay each site was located in: Batiste, Sansbois, or Pointe à la Hache) to assess any possible role that location may play in similarities between diets. The function pairwise.adonis (Martinez Arbizu, 2017) was used as a post-hoc test to evaluate which group, if any, was significantly different from the others. For each year and site type, diversity indexes were calculated: Shannon-Wiener diversity index (H) and Simpson’s diversity index (D) were calculated using the vegan package (Oksanen et al., 2019). For both Shannon and Simpson’s diversity indexes, means were compared across years using a Kruskal–Wallis rank sum test, as the data were not normally distributed (Shapiro-Wilk, Shannon: W = 0.95, p-value = 3.18 × 10−5; Simpson: W = 0.93, p-value = 6.45 × 10−7). The mean diversity metrics were then compared across year and site type using a pairwise Wilcoxon rank sum test with the Benjamini–Hochberg (BH) p-value adjustment.
Next the RInSp package (Zaccarelli et al., 2013) was used to calculate dietary richness (function pop.diet, “average” method) and total niche width (TNW) (WTdMC function). TNW refers to the entire suite of resources used by a population (Roughgarden, 1972); TNW values were calculated for each combination of site type and year, then the values were compared with a two-way ANOVA, with site type and year as factors (TNW ∼ Site Type + Year). A Tukey’s HSD test was used to evaluate the factors. TNW can be broken down into two components: the between individual component (BIC) and within individual component (WIC), such that BIC + WIC = TNW (Roughgarden, 1972). BIC refers to the variation in diet between individuals, while WIC refers to the variation in diet within individuals (Bolnick et al., 2003). WIC/TNW, or the ratio of the individual/population’s resource use, is one way to measure individual specialization (Roughgarden, 1972); the metric produced by RInSp uses the Roughgarden (1979) formula, which incorporates the Shannon diversity index (Zaccarelli et al., 2013). Another measure of individual specialization (IS), based on Bolnick et al. (2002), was calculated (function PSicalc). IS describes individual specialization as the “mean proportional similarity (PSi) between individuals and population” (Bolnick et al., 2002). Both metrics of individual specialization (WIC/TNW and IS) range from zero to one, where a value close to zero represents very little overlap between individuals and the population, and a value close to one represents complete overlap (Bolnick et al., 2002).
For both WIC/TNW and IS, the RInSp package (Zaccarelli et al., 2013) produces a single value for each combination of year and site type. To assess the significance of these values compared to the null hypothesis that individuals are sampling equally from the full suite of resources, a Monte Carlo resampling procedure was run with 9,999 permutations (Zaccarelli et al., 2013; Lunghi et al., 2020). WIC/TNW and IS were calculated for each combination of year and site type (Table 1). A linear regression model was used to test whether there was any relationship between TNW and IS or TNW and WIC/TNW.
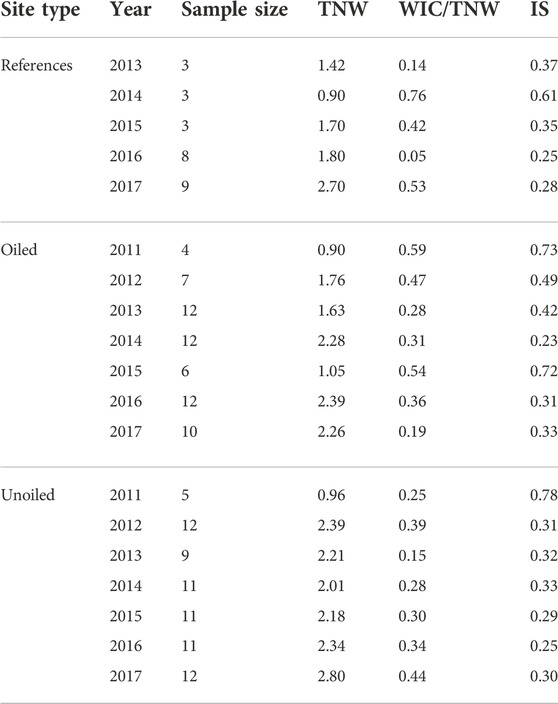
TABLE 1. Sample size, TNW, and both metrics of individual specialization (WIC/TNW and IS) of Seaside Sparrows, broken down by year and site type. Sparrows that did not produce metabarcoding results are not included in the sample size. The average measure of total niche width (TNW) and individual specialization were calculated by WIC/TNW and through the PSicalc function (IS). All values of WIC/TNW and IS have p < 0.05. Note that reference sites were not established until 2013.
3 Results
3.1 Seaside Sparrow diet
Overall, 126 ASVs were detected in Seaside Sparrow gut contents. 121 ASVs were identified as Metazoa, four ASVs as Viridiplantae (green plants), and one ASV as Fungi. A majority of the metazoan ASVs were Arthropoda (100/121), while the remainder were Platyhelminthes (7/121), Mollusca (7/121), Nematoda (2/121), Annelida (1/121), Acanthocephala (1/121), Cnidaria (1/121), and Chordata (1/121). Of the 100 ASVs that were Arthropoda, 73 were identified as Insecta, 15 as Arachnida, and 10 as Malacostraca.
The most common ASV was Insecta, with a 70.81% frequency of occurrence (FOO), meaning that Insecta occurred in 70.81% of samples (Table 2). Of the 18 ASVs detected in at least 10% of samples, eight were insects, including the orders Diptera (FOO = 51.55%), Hemiptera (FOO = 39.75%), and Lepidoptera (FOO = 31.68%). Additional insect ASVs were identified to the family-level as Chironomidae (FOO = 34.78%), Crambidae (FOO = 19.88%), Dolichopodidae (FOO = 16.15%), and Tabanidae (FOO = 11.80%). Four types of Arachnids (including the families Salticidae and Lycosidae), two types of Malacostraca (including the family Ocypodidae), and one gastropod were also detected. Only two of the most common ASVs (defined as >10% frequency of occurrence) were identified as plants.
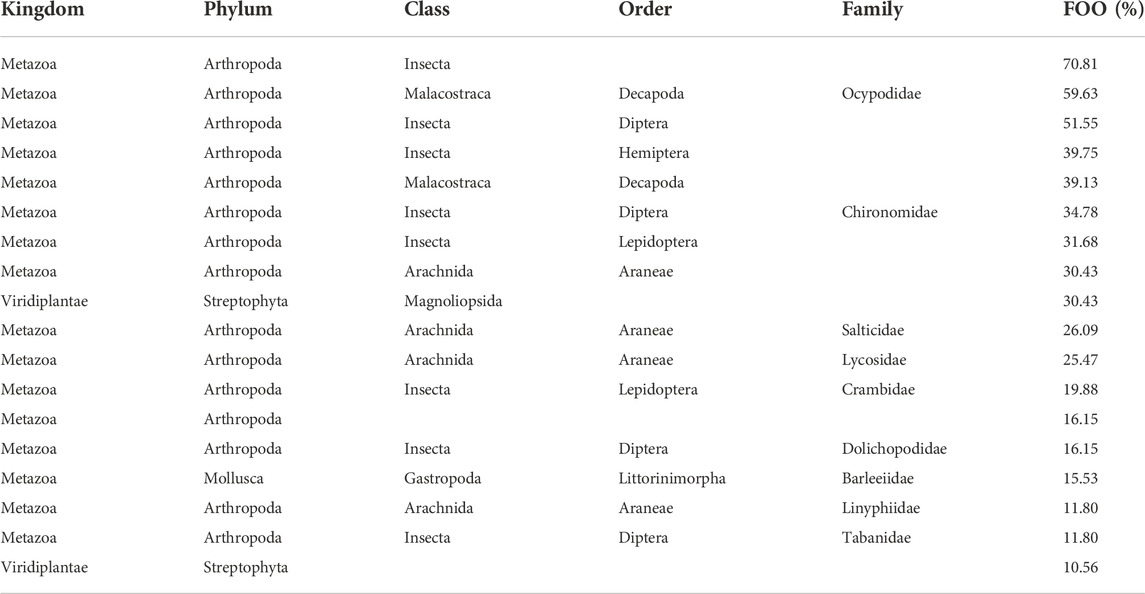
TABLE 2. The most commonly occurring (>10% frequency of occurrence, or “FOO”) ASVs detected in Seaside Sparrow diet. Note that each row is one ASV, independent of the other rows.
3.2 Resource use
A total of 160 birds (of the 168 collected) passed all filtering and quality control steps for at least one marker and were included in the combined dataset (Table 1). Adonis indicated both year and site type (oiled sites) to be significant drivers of diet based on the presence/absence of ASVs (year: pseudo F = 2.79; R2 = 0.12; df = 6, 153; p = 0.001; site type: pseudo F = 5.40, R2 = 0.06, df = 2, 157 p = 0.001). Bay also appeared to be a significant driver of diet composition based on Adonis (pseudo F = 5.75, R2 = 0.07, df = 2, 157, p = 0.001). The NMDS plots, which illustrate each individual bird’s diet composition as a single point based on the ordination of all birds, show high overlap among years, with differences in the spread of points so that 2011 and 2012 are nested within later years (Figure 2A). Post-hoc tests show that diet composition in 2012 was significantly different from all other years (all adjusted p-values = 0.02, Supplementary Table S1), 2013 was significantly different from 2014, 2016, and 2017 (adjusted p-value = 2014: 0.04; 2016, 2017: 0.02; Supplementary Table S1), and 2014 was significantly different from 2017 (adjusted p-value = 0.04, Supplementary Table S1). The NMDS plot of site type shows reference points are clustered separately from both oiled and unoiled points (Figure 2B) and looks very similar to the NMDS plot organized by bay (Figure 2C). Post-hoc tests indicate that diet composition is significantly different among all treatments (all adjusted p-values = oiled vs. unoiled: 0.02, oiled vs. reference: 0.003, unoiled vs. reference: 0.003; Supplementary Table S2), as well as all bays (all adjusted p-values = 0.003, Supplementary Table S3). Kruskal–Wallis rank sum tests indicated a significant difference in both Shannon and Simpson diversity between years (Shannon: chi-squared = 22.68, df = 6, p-value = 0.0009; Simpson: chi-squared = 19.92, df = 6, p-value = 0.003). There were no differences in either index among site type (chi-squared = 4.20, df = 2, p-value = 0.12; chi-squared = 3.49, df = 2, p-value = 0.17). Pairwise Wilcoxon rank sum tests showed similar trends for both Shannon and Simpson diversity, with low diversity in 2011, an increase in 2012, followed by a drop in 2013 and little change until diversity increased in 2017 (Tables 3 and 4, Figures 3A,B). Richness shows a similar trend, with a drop in the number of ASVs detected in 2013, despite the addition of reference sites that year (Figure 3C).
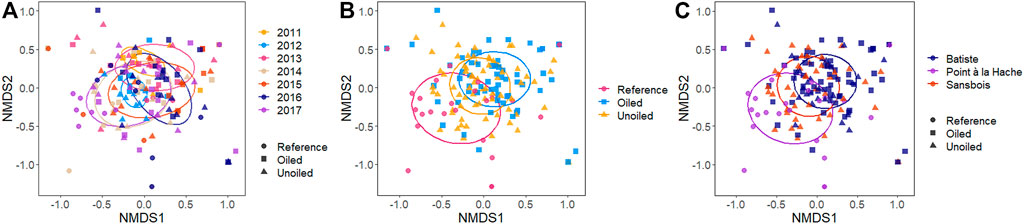
FIGURE 2. NMDS plot of all diet points coded to show the spread of diet between years (A), site type (B), and bay (C). Samples that are more similar in composition are closer together. Three outlier points have been removed for the sake of visualization. Stress = 0.17. Ellipses show 95% confidence level.
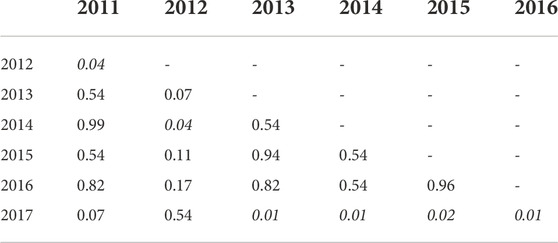
TABLE 3. Mean Shannon diversity indexes for each year were compared with a pairwise Wilcoxon rank sum test with the Benjamini–Hochberg (BH) p-value adjustment. In this table, all site types (unoiled, oiled, and reference) are pooled within the year they were collected. Significant values are in italics and indicate a statistically significant difference in the mean diversity between two years.
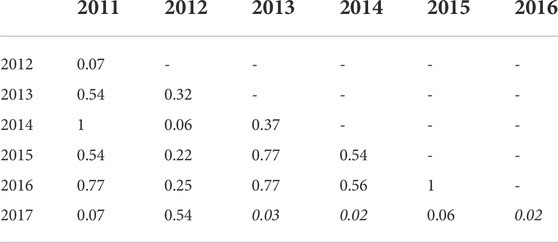
TABLE 4. Mean Simpson diversity indexes for each year were compared with a pairwise Wilcoxon rank sum test with the Benjamini–Hochberg (BH) p-value adjustment. In this table, all site types (unoiled, oiled, and reference) are pooled within the year they were collected. Significant values are in italics and indicate a statistically significant difference in the mean diversity between two years.
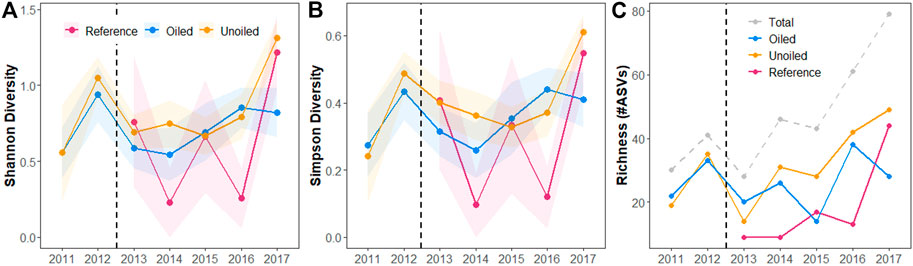
FIGURE 3. Diversity and richness trends in Seaside Sparrow diet over time. In figures (A,B), points represent population means and shading represent ±1 standard error. In figure (C), the total number of ASVs detected per year is represented by the gray dashed line, but note that duplicate ASVs are only counted once in the overall total richness. For example, an ASV detected in both oiled and unoiled sites would only be counted once toward the total ASVs in a year. The dashed black line in all figures represents the approximate time of Hurricane Isaac making landfall.
While there was no statistical difference in TNW between site type (ANOVA; TNW: df = 2; F = 2.21; p = 0.16), year was a significant factor in determining TNW (ANOVA; TNW: df = 6; F = 4.56; p = 0.018). Tukey’s HSD indicated that 2011 was significantly different from 2016 (p = 0.046) and 2017 (p = 0.009). Across oiling status, TNW was significantly negatively correlated with individual specialization, when individual specialization was calculated with PSicalc (IS: Multiple R2 = 0.74, Adjusted R2 = 0.73, F1,17 = 48.89, p = 2.17 × 10−6; Figure 4D), meaning that, as TNW increases, individuals become more specialized. When calculated as WIC/TNW, there was no significant correlation with TNW (Multiple R2 = 0.07, Adjusted R2 = 0.02, F1,17 = 1.30, p = 0.27; Figure 4E).
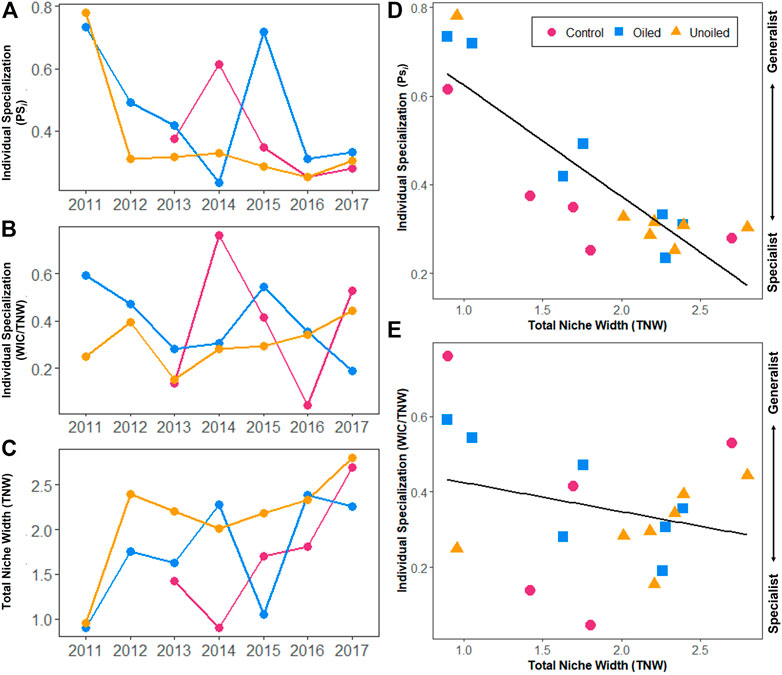
FIGURE 4. Individual specialization (IS), calculated by PSicalc (A) and WIC/TNW (B), was most stable on unoiled sites throughout time, as was total niche width (TNW), (C). There is a strong correlation between TNW and IS (when is calculated by PSicalc), meaning as a sample group’s TNW increases, individuals become more specialized (D). As IS values approach one, the more individual diet overlaps with the population, where one represents complete overlap. As the values approach zero, the less an individual’s diet overlaps with the population and the more specialized individuals are. However, when IS calculated by WIC/TNW, there is no significant correlation with TNW (E).
4 Discussion
We used DNA metabarcoding to evaluate resource use by Seaside Sparrows from 2011 to 2017 on oiled, unoiled, and reference sites, with the goal of understanding how sparrows responded to the residual contamination left from the Deepwater Horizon oil spill and from ecosystem disturbance caused by Hurricane Isaac. Our DNA metabarcoding study is the most detailed account of the diet of any animal following the Deepwater Horizon spill, and the most comprehensive assessment of Seaside Sparrow diet to date. Previous studies on Seaside Sparrow diet and resource use have used traditional methods, manually observing foraging or stomach contents (Post, 1974; Post and Greenlaw, 2006; Post and Greenlaw, 2020) or isotopic approaches (Johnson, 2017; Olin et al., 2017; Johnson et al., 2019; Moyo et al., 2021). Our use of metabarcoding allows for a more detailed evaluation of diet compared to these approaches, providing a more nuanced evaluation of resource use than previously attained.
4.1 Seaside Sparrow diet
While we detected three of the prey groups identified previously via morphology on nearby Grand Isle, Louisiana [Orthoptera (33%), moth larvae (26%), and spiders (18%) by volume (cited without attribution in Post and Greenlaw 2020)], Orthoptera were less common than expected; we only found Orthoptera in six (out of 160) samples. We detected three Lepidoptera families (Crambidae, Eriebidae, and Tortricidae) and while metabarcoding cannot determine if these moths were consumed as adults or larvae, we expect both life stages were consumed based on previous work (Post 1974) and field observations (A. Snider, personal observation). Finally, we detected five spider families; the two families most commonly consumed (Lycosidae and Salticidae) are ground-dwelling spiders, while web-building spiders (i.e., Linyphiidae and Araneidae) (Döbel et al., 1990) were consumed less frequently.
Interestingly, we found some families of prey that could represent secondary ingestion, i.e., prey ingested by the sparrow’s prey, or unintentional ingestion through consuming a parasitized prey item (da Silva et al., 2019). For example, we detected mites that could be parasites of both insect (Arrenuridae) or plant resources (Eriophyidae). We also detected several families that are presumed to be parasites of sparrows, including feather mites (Proctophyllodidae) and internal parasites such as Archiacanthocephala, Microphallidae, Acuariidae, and several Trematodes. Although we did not detect some prey taxa reported previously (including amphipods), or only detected them minimally (isopods) (Post et al., 1983; Post and Greenlaw, 2006), our findings were broadly similar to prior Seaside Sparrow diet studies throughout their range (Post 1974; Post and Greenlaw 2020). Additionally, due to primer bias, some taxa may have been present, but were poorly amplified. For instance, ants (Formicidae) were not detected in our study, but this taxon does not amplify well with the Zeale primer set (da Silva et al., 2019), and may be consumed more frequently than we were able to determine. This could have implications for our estimates of resource use if ants are a regular component of Seaside Sparrow diet, as ant abundance was significantly reduced in 2013 (Bam et al., 2018).
4.2 Resource use
The overall alpha diversity (both for Shannon and Simpson diversity metrics) dropped sharply in 2013, corresponding with the first field season after Hurricane Isaac. We interpret this as mortality of non-aquatic prey across all sites caused by storm surge that left the marsh completely underwater for several days. Alpha diversity then stayed low and remained relatively stable from 2013 to 2016, with a sharp increase in 2017. A similar trend was seen with the overall richness of diet, with the richness declining in 2013 and increasing—possibly due to ecosystem recovery or stabilization—by the end of the sampling period in 2017. Assuming that Seaside Sparrow diet is a reflection of the available prey, both alpha diversity and richness corroborate previous findings that Hurricane Isaac altered the invertebrate community in the saltmarsh (Bam et al., 2018; Chen et al., 2020). Additionally, although TNW was not significantly different in 2013, other studies conducted post-DWH in this region have found various indicators of disturbance to the ecosystem in 2013. These include increased alkanes and polycyclic aromatic hydrocarbons in marsh sediment (Turner et al., 2014), corresponding signs of this increased contamination in different species (Paruk et al., 2016; Perez-Umphrey et al., 2018), altered invertebrate communities (Bam et al., 2018), and altered patterns of resource use (Olin et al., 2017).
We did, however, find that TNW, WIC/TNW, and IS were less consistent over time on oiled and reference sites compared to unoiled sites. Oiled and reference sites appear to have greater fluctuations across time, but these fluctuations were not synchronized. For example, there was a steep reduction in TNW on oiled sites in 2015 but not at unoiled sites; previous work has highlighted similar anomalies in vertebrate diet on these study sites in 2015 (Moyo et al., 2021). However, with no major storms or weather incidents, it is unclear what might have driven these changes.
In evaluating overall diet composition, we found that diets from oiled, unoiled, and reference sites were all significantly different from each other. In NMDS plots, the clustering of sparrows on reference sites was further from birds on both oiled and unoiled sites, likely due to the underlying ecosystem differences on either side of the Mississippi River. Reference sites were located in marshes that are slightly less brackish than those on the southwest side of the river (oiled and unoiled sites); these differences in salinity influence the plant communities on these sites which likely leads to differences in the invertebrate community and thus, sparrow diet. This could potentially explain the tighter clustering of diet in 2011 and 2012, as reference sites had not yet been established and no samples were collected from these sites in these years.
Based on data from Louisiana State University’s Coastal Emergency Risks Assessment (CERA, 2022: https://cera.coastalrisk.live/), all study sites were flooded for approximately the same length of time (2–3 days) as Hurricane Isaac passed through the region (for more details on the flooding of sites west of the Mississippi river, see Stouffer et al. (2013)). Due to the counterclockwise rotation of Hurricane Isaac, sites on the east side of the Mississippi River (reference sites) experienced a greater degree of flooding compared to the oiled and unoiled sites on the west side: at peak flood depth, reference sites were inundated with over 4 m of water, compared to a peak depth of 2–3 m at oiled and unoiled sites. Since the marsh was completely submerged at all study sites for approximately the same length of time (thus affecting prey communities in the same way), we do not expect that this difference in flood depth is a contributing factor to any differences detected between site type in this study.
The difference in diet composition between bays makes it difficult to determine the underlying cause of what is driving the difference in diet composition. Sites were selected because of their degree of oiling, but the oil each site received is directly related to their location within the larger Barataria Bay system. Untangling whether diet is driven by hydrologic processes within each bay or residual contamination is an area of research that would likely benefit a host of ongoing studies taking place in this system and provide context to previous work that took place in Barataria Bay (Olin et al., 2017; Moyo et al., 2021). While Seaside Sparrows were physiologically impacted by contamination on oiled sites (Bonisoli-Alquati et al., 2016; Perez-Umphrey et al., 2018; Bonisoli-Alquati et al., 2020), diet composition might be impacted for years beyond the initial disturbance as the saltmarsh community—and prey species—recover. To know whether prey communities are inherently different between locations, or if differences are due to the various recovery processes of different species (Zengel et al., 2016a; Zengel et al., 2016b; Zengel et al., 2017; Bam et al., 2018), would require long-term community monitoring data that is not currently available. If a sparrow foraged on one site, then was captured on another during this study, this would probably cause the two sites to appear more similar in overall diet composition; since the birds in this study were not banded, there would be no way to know whether or not this occurred. In a concurrent banding study at nearby sites from 2012 to 2017, we recorded 199 recapture events representing 137 unique individuals. Over the 6 years of banding data, only 25 of those recaptures were a bird that had moved between sites, but a bird never was recaptured on the opposite side of the Mississippi River (Snider et al., unpublished data). As the banding study was conducted over the course of at least 4 months each year (March-June, as well as October 2012), with low movement among sites, we expect the likelihood of birds moving among sites does not pose a significant issue in the data presented here. Finally, we found evidence for a relationship between IS and TNW that appears to support the NVH. Assuming that oiled sites are poor quality and low in resources compared to unoiled sites, our findings indicate that individuals broaden the scope of their diets when resources are scarce: when individual specialization is measured by PSicalc, individuals were more generalized when TNW was low. Further, individuals on unoiled sites remained specialized and stable after 2011, in conjunction with high, stable TNW. This suggests that in higher quality areas, presumably due to reduced competition or a wider variety of resources available, individuals can specialize on unique resources. This increase in individual specialization broadens the TNW of the population, as predicted by the NVH (Bolnick et al., 2007).
Both oiled and unoiled sites showed more generalized individuals (when measured by PSicalc) in 2011. If oiling was the driving factor behind reduced TNW in 2011, we would expect unoiled and oiled sites to be different. This small TNW for both site types in 2011 could be influenced by a low sample size, or perhaps additional abiotic factors that were not considered when setting up the experimental design. Overall, if we assume that unoiled sites represent higher quality habitat, and that the years post-2013 represent more stable environmental conditions on these sites, it appears that Seaside Sparrows are type B generalists (sensu Bolnick et al., 2007): a generalist population made up of relatively specialized individuals when environmental conditions are stable.
Empirically, populations have responded to reduced resources with mixed results (niche width increase: Manlick and Pauli, 2020, Jesmer et al., 2020, Rahman et al., 2021; niche width decrease after disturbance: di Lascio et al., 2013). Our findings, however, agree with previous work from the same geographic area showing that Seaside Sparrow total niche width was smaller on oiled sites compared to unoiled sites in 2015 and 2017 (Moyo et al., 2021); the unoiled and oiled sites presented in this study are located in the same bays (Sansbois and Batiste) as those presented in Moyo et al. (2021). This is interesting given the different temporal scales represented by our studies. DNA metabarcoding of stomach contents provides a snapshot of sparrow diet, likely representing diet on the scale of hours or even single foraging bouts (Snider et al., 2022), whereas the blood stable isotope approach used by Moyo et al. (2021) summarizes diet over a scale of days (Podlesak et al., 2005). While DNA metabarcoding showed Seaside Sparrows had roughly the same TNW on oiled and unoiled sites in 2016, Moyo et al. (2021) showed that sparrows on unoiled sites had a wider TNW than those on oiled sites in 2016. This underscores the need for further work to assess how different approaches to studying diet may provide inconsistent results, as well as how these methods can be used in conjunction to provide comprehensive interpretations (Hoenig et al., 2022).
As pointed out by Bolnick et al. (2003), these measures of resource use (TNW, WIC/TNW, IS) need to be carefully interpreted in the context of temporal variation: while these measures can indeed be driven by behavioral or phenotypic variation between individuals, they can also be influenced by stochastic events, like patchy resource distribution (e.g., insect hatches, Hansen et al., 2020). Using blood plasma for a stable isotope study, Woo et al. (2008) found that these tissue samples—which describe diet on the scale of weeks—can suffer from temporal biases, so the stomach contents used here for DNA metabarcoding (which likely reflect diet on the scale of hours) almost certainly contain these same biases. When possible, long-term studies should be used to evaluate the diet from the same individuals repeatedly to account for this (Bolnick et al., 2003), which could easily be accomplished by collecting fecal samples from individuals throughout time. Future work in this system will benefit from the integration of multiple approaches, such as using both stable isotope and DNA-based methods together, allowing for a more holistic approach of resource use across multiple time scales.
Our findings on the resource use of Seaside Sparrows fit into the larger context of previous work on the resource use by generalists, such as that of Bolnick et al. (2003), who found support across several populations and species that generalist populations tend to be comprised of heterogenous individuals (i.e., type B generalists). Our description of Seaside Sparrow resource use could also be influenced by some key factors, including high rates of ecosystem disturbance, low interspecific competition, and low resource diversity. First, areas of frequent disturbance should favor generalists (Kassen, 2002) and it is likely that this is an important factor in the Seaside Sparrow’s southern range. On average, southeast Louisiana experiences a tropical storm or hurricane every 3 years (Keim et al., 2007). Second, Seaside Sparrows are often abundant in the Louisiana saltmarshes, with few other terrestrial bird species present. Under the NVH, Seaside Sparrow individuals should be able to take advantage of the available resources through individuals specializing on unique resources (Van Valen, 1965). Finally, saltmarshes are highly productive habitats; however, they are surprisingly low in species diversity (Odum, 1988; Silliman, 2014). In low diversity environments, like saltmarshes, individuals in generalist populations are more specialized (Tinker et al., 2008; Robinson and Strauss 2020). Given these factors, Seaside Sparrows may have evolved a generalist dietary strategy, where individuals are able to take advantage of unique resources in less disturbed areas, using dietary plasticity as an adaptation to periodic ecosystem disturbance.
Data availability statement
Data are publicly available through NCBI Sequence Read Archive at https://www.ncbi.nlm.nih.gov/sra, BioProject: PRJNA629391, Accession Numbers: SAMN14775163-SAMN14775290 and SAMN31697890-SAMN31698083. Further inquiries can be directed to the corresponding author.
Ethics statement
Seaside Sparrows were handled and collected under USFWS permits 22648 and MB095918-0, LDWF permits LNHP-12-023 (2012), LNHP-13-059 (2013), LNHP-14-051 (2014), LNHP-15-033 (2015), LNHP-16-048 (2016), and LNHP-17-039 (2017). Methods were approved by the Institutional Animal Care and Use Committee of the Louisiana State University AgCenter, permits AE2011-04 (2011-2014), A2012-05 (2012-2014), and A2015-04 (2015-2018). 2011 Seaside Sparrows were collected under collection permits USFWS MB679782 and LDWF LNHP11-068.
Author contributions
Conceived the research idea and design: PS, ST, and AS. Collected data and performed field work: AP-U, AB-A, SW, AS. Wrote first draft of manuscript: AS. Provided substantial input and editing to manuscript: ST, PS, AB-A, SW, and AP-U. Developed and designed methods: AS. Analyzed the data: AS. Contributed substantial materials, resources, or funding: PS and ST.
Funding
This research was made possible in part by grants from The Gulf of Mexico Research Initiative, the American Ornithological Society, and in part by the National Institute of Food and Agriculture, U.S. Department of Agriculture, McIntire Stennis program. This work used Genomics core facilities that are supported in part by COBRE (NIH8 1P30GM118430-02) and NORC (NIH 2P30DK072476) center grants from the National Institutes of Health.
Acknowledgments
We wish to thank the many collaborators who provided valuable feedback on earlier versions of this manuscript, including Thomas W. Sherry, Michael J. Polito, S. Tyler Williams, Kelcee L. Smith, Amie E. Settlecowski, and Alessandra M. Bresnan. We thank Eliza D. Stein for providing the map of our study sites. We also wish to thank the communities of Happy Jack and Port Sulphur, Louisiana. An early version of this manuscript appeared in the dissertation, “Molecular Analysis of Seaside Sparrow (Ammospiza maritima) Diet: Methodological Insights and Ecological Applications” by AS. Finally, we thank Fred Sheldon at the LSU Museum of Natural Science, who collected the 2011 Seaside Sparrows.
Conflict of interest
The authors declare that the research was conducted in the absence of any commercial or financial relationships that could be construed as a potential conflict of interest.
The handling editor PL declared a past co-authorship with the authors.
Publisher’s note
All claims expressed in this article are solely those of the authors and do not necessarily represent those of their affiliated organizations, or those of the publisher, the editors and the reviewers. Any product that may be evaluated in this article, or claim that may be made by its manufacturer, is not guaranteed or endorsed by the publisher.
Supplementary material
The Supplementary Material for this article can be found online at: https://www.frontiersin.org/articles/10.3389/fenvs.2022.978325/full#supplementary-material
References
Alberdi, A., Aizpurua, O., Bohmann, K., Gopalakrishnan, S., Lynggaard, C., Nielsen, M., et al. (2019). Promises and pitfalls of using high-throughput sequencing for diet analysis. Mol. Ecol. Resour. 19, 327–348. doi:10.1111/1755-0998.12960
Alves, V. E. N., Patrício, J., Dolbeth, M., Pessanha, A., Palma, A. R. T., Dantas, E. W., et al. (2016). Do different degrees of human activity affect the diet of Brazilian silverside Atherinella brasiliensis? J. Fish Biol. 89, 1239–1257. doi:10.1111/jfb.13023
Baker, J. L. (1973). Preliminary studies of the Dusky Seaside Sparrow on the st. Johns national wildlife refuge. Southeastern Association of Fish and Wildlife Agencies. Available at: https://seafwa.org/journal/1973/preliminary-studies-dusky-seaside-sparrow-st-johns-national-wildlife-refuge (Accessed September 1, 2022).
Bam, W., Hooper-Bui, L. M., Strecker, R. M., Adhikari, P. L., and Overton, E. B. (2018). Coupled effects of oil spill and hurricane on saltmarsh terrestrial arthropods. PLOS ONE 13, e0194941. doi:10.1371/journal.pone.0194941
Bokulich, N. A., Kaehler, B. D., Rideout, J. R., Dillon, M., Bolyen, E., Knight, R., et al. (2018). Optimizing taxonomic classification of marker-gene amplicon sequences with QIIME 2’s q2-feature-classifier plugin. Microbiome 6, 90. doi:10.1186/s40168-018-0470-z
Bolnick, D. I., Svanbäck, R., Araújo, M. S., and Persson, L. (2007). Comparative support for the niche variation hypothesis that more generalized populations also are more heterogeneous. Proc. Natl. Acad. Sci. U. S. A. 104, 10075–10079. doi:10.1073/pnas.0703743104
Bolnick, D. I., Svanbäck, R., Fordyce, J. A., Yang, L. H., Davis, J. M., Hulsey, C. D., et al. (2003). The ecology of individuals: Incidence and implications of individual specialization. Am. Nat. 161, 1–28. doi:10.1086/343878
Bolnick, D. I., Yang, L. H., Fordyce, J. A., Davis, J. M., and Svanbäck, R. (2002). Measuring individual-level resource specialization. Ecology 83, 2936–2941. doi:10.1890/0012-9658(2002)083[2936:MILRS]2.0.CO;2
Bolyen, E., Rideout, J. R., Dillon, M. R., Bokulich, N. A., Abnet, C. C., Al-Ghalith, G. A., et al. (2019). Reproducible, interactive, scalable and extensible microbiome data science using QIIME 2. Nat. Biotechnol. 37, 852–857. doi:10.1038/s41587-019-0209-9
Bonisoli-Alquati, A., Stouffer, P. C., Turner, R. E., Woltmann, S., and Taylor, S. S. (2016). Incorporation of Deepwater Horizon oil in a terrestrial bird. Environ. Res. Lett. 11, 114023. doi:10.1088/1748-9326/11/11/114023
Bonisoli-Alquati, A., Xu, W., Stouffer, P. C., and Taylor, S. S. (2020). Transcriptome analysis indicates a broad range of toxic effects of Deepwater Horizon oil on Seaside Sparrows. Sci. Total Environ. 720, 137583. doi:10.1016/j.scitotenv.2020.137583
Burgar, J. M., Murray, D. C., Craig, M. D., Haile, J., Houston, J., Stokes, V., et al. (2014). Who’s for dinner? High-throughput sequencing reveals bat dietary differentiation in a biodiversity hotspot where prey taxonomy is largely undescribed. Mol. Ecol. 23, 3605–3617. doi:10.1111/mec.12531
Callahan, B. J., McMurdie, P. J., Rosen, M. J., Han, A. W., Johnson, A. J. A., and Holmes, S. P. (2016). DADA2: High-resolution sample inference from Illumina amplicon data. Nat. Methods 13, 581–583. doi:10.1038/nmeth.3869
CERA (2022). CERA - coastal emergency Risks assessment. Available at: https://cera.coastalrisk.live/(Accessed November 17, 2022).
Chen, X., Adams, B. J., Platt, W. J., and Hooper-Bùi, L. M. (2020). Effects of a tropical cyclone on salt marsh insect communities and post-cyclone reassembly processes. Ecography 43, 834–847. doi:10.1111/ecog.04932
Clarke, L. J., Beard, J. M., Swadling, K. M., and Deagle, B. E. (2017). Effect of marker choice and thermal cycling protocol on zooplankton DNA metabarcoding studies. Ecol. Evol. 7, 873–883. doi:10.1002/ece3.2667
Clavel, J., Julliard, R., and Devictor, V. (2011). Worldwide decline of specialist species: Toward a global functional homogenization? Front. Ecol. Environ. 9, 222–228. doi:10.1890/080216
Cloyed, C. S., Balmer, B. C., Schwacke, L. H., Wells, R. S., Berens McCabe, E. J., Barleycorn, A. A., et al. (2021). Interaction between dietary and habitat niche breadth influences cetacean vulnerability to environmental disturbance. Ecosphere 12, e03759. doi:10.1002/ecs2.3759
Colwell, R. K., and Futuyma, D. J. (1971). On the measurement of niche breadth and overlap. Ecology 52, 567–576. doi:10.2307/1934144
da Silva, L. P., Mata, V. A., Lopes, P. B., Pereira, P., Jarman, S. N., Lopes, R. J., et al. (2019). Advancing the integration of multi-marker metabarcoding data in dietary analysis of trophic generalists. Mol. Ecol. Resour. 19, 1420–1432. doi:10.1111/1755-0998.13060
DeRagon, W. R. (1988). Breeding ecology of Seaside and Sharp-tailed sparrows in Rhode Island salt marshes. MS thesis. Kingston, RI: University of Rhode Island.
DeSantis, L. R. G., Pardi, M. I., Du, A., Greshko, M. A., Yann, L. T., Hulbert, R. C., et al. (2022). Global long-term stability of individual dietary specialization in herbivorous mammals. Proc. R. Soc. B 289, 20211839. doi:10.1098/rspb.2021.1839
di Lascio, A., Rossi, L., Carlino, P., Calizza, E., Rossi, D., and Costantini, M. L. (2013). Stable isotope variation in macroinvertebrates indicates anthropogenic disturbance along an urban stretch of the river Tiber (Rome, Italy). Ecol. Indic. 28, 107–114. doi:10.1016/j.ecolind.2012.04.006
Döbel, H. G., Denno, R. F., and Coddington, J. A. (1990). Spider (Araneae) community structure in an intertidal salt marsh: Effects of vegetation structure and tidal flooding. Environ. Entomol. 19, 1356–1370. doi:10.1093/ee/19.5.1356
Durell, S. E. (2000). Individual feeding specialisation in shorebirds: Population consequences and conservation implications. Biol. Rev. 75, 503–518. doi:10.1111/j.1469-185X.2000.tb00053.x
Graham, N. A. J., Chabanet, P., Evans, R. D., Jennings, S., Letourneur, Y., Aaron MacNeil, M., et al. (2011). Extinction vulnerability of coral reef fishes. Ecol. Lett. 14, 341–348. doi:10.1111/j.1461-0248.2011.01592.x
Hansen, H. H., Pegg, M., Van Den Broeke, M., Watkinson, D., and Enders, E. C. (2020). An unseen synchrony or recurrent resource pulse opportunity? Linking fisheries with aeroecology. Remote Sens. Ecol. Conserv. 6, 366–380. doi:10.1002/rse2.147
Hoenig, B. D., Snider, A. M., Forsman, A. M., Hobson, K. A., Latta, S. C., Miller, E. T., et al. (2022). Current methods and future directions in avian diet analysis. Ornithology 139, ukab077. doi:10.1093/ornithology/ukab077
Holovachov, O., Haenel, Q., Bourlat, S. J., and Jondelius, U. (2017). Taxonomy assignment approach determines the efficiency of identification of OTUs in marine nematodes. R. Soc. open Sci. 4, 170315. doi:10.1098/rsos.170315
Illumina (2013). 16S metagenomic sequencing library preparation. Available at: https://www.illumina.com/content/dam/illumina-support/documents/documentation/chemistry_documentation/16s/16s-metagenomic-library-prep-guide-15044223-b.pdf.
Jarman, S. N., McInnes, J. C., Faux, C., Polanowski, A. M., Marthick, J., Deagle, B. E., et al. (2013). Adélie penguin population diet monitoring by analysis of food DNA in scats. PLOS ONE 8, e82227. doi:10.1371/journal.pone.0082227
Jedlicka, J. A., Sharma, A. M., and Almeida, R. P. P. (2013). Molecular tools reveal diets of insectivorous birds from predator fecal matter. Conserv. Genet. Resour. 5, 879–885. doi:10.1007/s12686-013-9900-1
Jedlicka, J. A., Vo, A.-T. E., and Almeida, R. P. P. (2016). Molecular scatology and high-throughput sequencing reveal predominately herbivorous insects in the diets of adult and nestling Western Bluebirds (Sialia mexicana) in California vineyards. Auk 134, 116–127. doi:10.1642/AUK-16-103.1
Jesmer, B. R., Kauffman, M. J., Murphy, M. A., and Goheen, J. R. (2020). A test of the Niche Variation Hypothesis in a ruminant herbivore. J. Anim. Ecol. 89, 2825–2839. doi:10.1111/1365-2656.13351
Johnson, J. (2017). Characterization of carbon flow in a Barataria Bay, Louisiana, salt marsh using trophic biomarkers. LSU Master’s Theses. Available at: https://digitalcommons.lsu.edu/gradschool_theses/4409.
Johnson, J. J., Olin, J. A., and Polito, M. J. (2019). A multi-biomarker approach supports the use of compound-specific stable isotope analysis of amino acids to quantify basal carbon source use in a salt marsh consumer. Rapid Commun. Mass Spectrom. 33, 1781–1791. doi:10.1002/rcm.8538
Kassen, R. (2002). The experimental evolution of specialists, generalists, and the maintenance of diversity. J. Evol. Biol. 15, 173–190. doi:10.1046/j.1420-9101.2002.00377.x
Keim, B. D., Muller, R. A., and Stone, G. W. (2007). Spatiotemporal patterns and return periods of tropical storm and hurricane strikes from Texas to Maine. J. Clim. 20, 3498–3509. doi:10.1175/JCLI4187.1
Lunghi, E., Cianferoni, F., Ceccolini, F., Zhao, Y., Manenti, R., Corti, C., et al. (2020). Same diet, different strategies: Variability of individual feeding habits across three populations of Ambrosi’s cave salamander (Hydromantes ambrosii). Diversity 12, 180. doi:10.3390/d12050180
Manlick, P. J., and Pauli, J. N. (2020). Human disturbance increases trophic niche overlap in terrestrial carnivore communities. Proc. Natl. Acad. Sci. U. S. A. 117, 26842–26848. doi:10.1073/pnas.2012774117
Marshall, R. M., and Reinert, S. E. (1990). Breeding ecology of Seaside Sparrows in a Massachusetts salt marsh. Wilson Bull. 102, 501–513.
Martin, A. C., Zim, H. S., and Nelson, A. L. (1961). American wildlife and plants: A guide to wildlife food habits. New York, NY: Dover Publications.
Martin, M. (2011). Cutadapt removes adapter sequences from high-throughput sequencing reads. EMBnet. J. 17, 10–12. doi:10.14806/ej.17.1.200
Martinez Arbizu, P. (2017). PairwiseAdonis: Pairwise multilevel comparison using adonis. R package version 0.4. Available at: https://github.com/pmartinezarbizu/pairwiseAdonis.
McCall, B. D., and Pennings, S. C. (2012). Disturbance and recovery of salt marsh arthropod communities following BP deepwater Horizon oil spill. PLOS ONE 7, e32735. doi:10.1371/journal.pone.0032735
Michel, J., Owens, E. H., Zengel, S., Graham, A., Nixon, Z., Allard, T., et al. (2013). Extent and degree of shoreline oiling: Deepwater Horizon oil spill, Gulf of Mexico, USA. PLOS ONE 8, e65087. doi:10.1371/journal.pone.0065087
Moyo, S., Bennadji, H., Laguaite, D., Pérez-Umphrey, A. A., Snider, A. M., Bonisoli-Alquati, A., et al. (2021). Stable isotope analyses identify trophic niche partitioning between sympatric terrestrial vertebrates in coastal saltmarshes with differing oiling histories. PeerJ 9, e11392. doi:10.7717/peerj.11392
Nixon, Z., Zengel, S., Baker, M., Steinhoff, M., Fricano, G., Rouhani, S., et al. (2016). Shoreline oiling from the Deepwater Horizon oil spill. Mar. Pollut. Bull. 107, 170–178. doi:10.1016/j.marpolbul.2016.04.003
NOAA (2013). Shoreline assessment manual. 4th edition. Seattle, Washington: Emergency Response Division, Office of Response and Restoration. National Oceanic and Atmospheric Administration.
Odum, W. E. (1988). Comparative ecology of tidal freshwater and salt marshes. Annu. Rev. Ecol. Syst. 19, 147–176. doi:10.1146/annurev.es.19.110188.001051
Oehm, J., Juen, A., Nagiller, K., Neuhauser, S., and Traugott, M. (2011). Molecular scatology: How to improve prey DNA detection success in avian faeces? Mol. Ecol. Resour. 11, 620–628. doi:10.1111/j.1755-0998.2011.03001.x
Oksanen, J., Blanchet, F. G., Friendly, M., Kindt, R., Legendre, P., McGlinn, D., et al. (2019). vegan: Community ecology package. Available at: https://CRAN.R-project.org/package=vegan.
Olin, J. A., Bergeon Burns, C. M., Woltmann, S., Taylor, S. S., Stouffer, P. C., Bam, W., et al. (2017). Seaside Sparrows reveal contrasting food web responses to large-scale stressors in coastal Louisiana saltmarshes. Ecosphere 8, e1878. doi:10.1002/ecs2.1878
Paruk, J. D., Adams, E. M., Uher-Koch, H., Kovach, K. A., Long, D., Perkins, C., et al. (2016). Polycyclic aromatic hydrocarbons in blood related to lower body mass in Common Loons. Sci. Total Environ. 565, 360–368. doi:10.1016/j.scitotenv.2016.04.150
Peers, M. J. L., Thornton, D. H., and Murray, D. L. (2012). Reconsidering the specialist-generalist paradigm in niche breadth dynamics: Resource gradient selection by Canada lynx and bobcat. PLOS ONE 7, e51488. doi:10.1371/journal.pone.0051488
Perez-Umphrey, A. A., Bergeon Burns, C. M., Stouffer, P. C., Woltmann, S., and Taylor, S. S. (2018). Polycyclic aromatic hydrocarbon exposure in Seaside Sparrows (Ammodramus maritimus) following the 2010 Deepwater Horizon oil spill. Sci. Total Environ. 630, 1086–1094. doi:10.1016/j.scitotenv.2018.02.281
Pielou, E. C. (1972). Niche width and niche overlap: A method for measuring them. Ecology 53, 687–692. doi:10.2307/1934784
Podlesak, D. W., McWilliams, S. R., and Hatch, K. A. (2005). Stable isotopes in breath, blood, feces and feathers can indicate intra-individual changes in the diet of migratory songbirds. Oecologia 142, 501–510. doi:10.1007/s00442-004-1737-6
Post, W. (1974). Functional analysis of space-related behavior in the Seaside Sparrow. Ecology 55, 564–575. doi:10.2307/1935147
Post, W., Greenlaw, J. S., Merriam, T. L., and Wood, L. A. (1983). “Comparative ecology of northern and southern populations of the Seaside Sparrow,” in The Seaside Sparrow, its biology and management (Raleigh, North Carolina: Occasional Papers of the North Carolina Biological Survey), 123–136.
Post, W., and Greenlaw, J. S. (2006). Nestling diets of coexisting salt marsh sparrows: Opportunism in a food-rich environment. Estuaries Coasts 29, 765–775. doi:10.1007/BF02786527
Post, W., and Greenlaw, J. S. (2020). Seaside Sparrow (Ammospiza maritima). Birds of the world. doi:10.2173/bow.seaspa.01
Post, W., and Greenlaw, J. S. (1975). Seaside Sparrow displays: Their function in social organization and habitat. Auk 92, 461–492. doi:10.2307/4084602
R Core Team (2019). R: A language and environment for statistical computing. Available at: https://www.R-project.org/.
Rahman, A. U., Jones, H. P., Hosler, S. C., Geddes, S., Nelson, M., and Barber, N. A. (2021). Disturbance-induced trophic niche shifts in ground beetles (Coleoptera: Carabidae) in restored grasslands. Environ. Entomol. 50, 1075–1087. doi:10.1093/ee/nvab065
Remsen, J. V., Wallace, B. P., Seymour, M. A., O’Malley, D. A., and Johnson, E. I. (2019). The regional, national, and international importance of Louisiana’s coastal avifauna. Wilson J. Ornithol. 131, 221. doi:10.1676/18-111
Robeson, M. S. R., O’Rourke, D. R., Kaehler, B. D., Ziemski, M., Dillon, M. R., Foster, J. T., et al. (2021). RESCRIPt: Reproducible sequence taxonomy reference database management. PLoS Comput. Biol. 17, e1009581. doi:10.1371/journal.pcbi.1009581
Robinson, M. L., and Strauss, S. Y. (2020). Generalists are more specialized in low-resource habitats, increasing stability of ecological network structure. Proc. Natl. Acad. Sci. U. S. A. 117, 2043–2048. doi:10.1073/pnas.1820143117
Roughgarden, J. (1979). Theory of population genetics and evolutionary ecology: An introduction. New York, NY: Macmillan.
RStudio Team (2020). RStudio: Integrated development environment for R. Available at: http://www.rstudio.com/.
Snider, A. M., Bonisoli-Alquati, A., Pérez-Umphrey, A. A., Stouffer, P. C., and Taylor, S. S. (2022). Metabarcoding of stomach contents and fecal samples provide similar insights about Seaside Sparrow diet. Ornithol. Appl. 124, duab060. doi:10.1093/ornithapp/duab060
Stouffer, P. C., Taylor, S., Woltmann, S., Burns, C. M. B., Shupe, T. F., and Bowen, M. S. (2013). “Staying alive on the edge of the Earth: Response of seaside sparrows (Ammodramus maritumus) to salt marsh inundation, with implications for storms, spills, and climate change,” in Proceedings of the 4th Louisiana Natural Resources Symposium. Editors T. F. Shupe, and M. S. Bowen (Baton Rouge, LA), 82–93.
Swihart, R. K., Gehring, T. M., Kolozsvary, M. B., and Nupp, T. E. (2003). Responses of ‘resistant’ vertebrates to habitat loss and fragmentation: The importance of niche breadth and range boundaries. Divers. Distrib. 9, 1–18. doi:10.1046/j.1472-4642.2003.00158.x
Tang, C. Q., Leasi, F., Obertegger, U., Kieneke, A., Barraclough, T. G., and Fontaneto, D. (2012). The widely used small subunit 18S rDNA molecule greatly underestimates true diversity in biodiversity surveys of the meiofauna. Proc. Natl. Acad. Sci. U. S. A. 109, 16208–16212. doi:10.1073/pnas.1209160109
Tinker, M. T., Bentall, G., and Estes, J. A. (2008). Food limitation leads to behavioral diversification and dietary specialization in sea otters. Proc. Natl. Acad. Sci. U. S. A. 105, 560–565. doi:10.1073/pnas.0709263105
Toscano, B. J., Gownaris, N. J., Heerhartz, S. M., and Monaco, C. J. (2016). Personality, foraging behavior and specialization: Integrating behavioral and food web ecology at the individual level. Oecologia 182, 55–69. doi:10.1007/s00442-016-3648-8
Trevelline, B. K., Nuttle, T., Porter, B. A., Brouwer, N. L., Hoenig, B. D., Steffensmeier, Z. D., et al. (2018). Stream acidification and reduced aquatic prey availability are associated with dietary shifts in an obligate riparian Neotropical migratory songbird. PeerJ 6, e5141. doi:10.7717/peerj.5141
Turner, R. E., Overton, E. B., Meyer, B. M., Miles, M. S., McClenachan, G., Hooper-Bui, L., et al. (2014). Distribution and recovery trajectory of Macondo (Mississippi Canyon 252) oil in Louisiana coastal wetlands. Mar. Pollut. Bull. 87, 57–67. doi:10.1016/j.marpolbul.2014.08.011
Van Valen, L. (1965). Morphological variation and width of ecological niche. Am. Nat. 99, 377–390. doi:10.1086/282379
Vo, A.-T., and Jedlicka, J. A. (2014). Protocols for metagenomic DNA extraction and Illumina amplicon library preparation for faecal and swab samples. Mol. Ecol. Resour. 14, 1183–1197. doi:10.1111/1755-0998.12269
Waide, R. B. (1991). The effect of hurricane hugo on bird populations in the Luquillo Experimental Forest, Puerto Rico. Biotropica 23, 475–480. doi:10.2307/2388269
Werner, H. W. (1975). The biology of the Cape Sable Seaside Sparrow. Florida: Everglades National Park.
Werner, H. W., and Woolfenden, G. E. (1983). “The Cape Sable Sparrow: Its habitat, habits, and history,” in The Seaside Sparrow: Its biology and management (Raleigh, North Carolina: Occasional Papers of the North Carolina Biological Survey).
Werner, T. K., and Sherry, T. W. (1987). Behavioral feeding specialization in Pinaroloxias inornata, the “Darwin’s finch” of Cocos Island, Costa Rica. Proc. Natl. Acad. Sci. U. S. A. 84, 5506–5510. doi:10.1073/pnas.84.15.5506
Woo, K. J., Elliott, K. H., Davidson, M., Gaston, A. J., and Davoren, G. K. (2008). Individual specialization in diet by a generalist marine predator reflects specialization in foraging behaviour. J. Anim. Ecol. 77, 1082–1091. doi:10.1111/j.1365-2656.2008.01429.x
Zaccarelli, N., Bolnick, D. I., and Mancinelli, G. (2013). RInSp: An r package for the analysis of individual specialization in resource use. Methods Ecol. Evol. 4, 1018–1023. doi:10.1111/2041-210X.12079
Zeale, M. R., Butlin, R. K., Barker, G. L., Lees, D. C., and Jones, G. (2011). Taxon-specific PCR for DNA barcoding arthropod prey in bat faeces. Mol. Ecol. Resour. 11, 236–244. doi:10.1111/j.1755-0998.2010.02920.x
Zengel, S., Bernik, B. M., Rutherford, N., Nixon, Z., and Michel, J. (2015). Heavily oiled salt marsh following the deepwater Horizon oil spill, ecological comparisons of shoreline cleanup treatments and recovery. PLOS ONE 10, e0132324. doi:10.1371/journal.pone.0132324
Zengel, S., Montague, C. L., Pennings, S. C., Powers, S. P., Steinhoff, M., Fricano, G., et al. (2016a). Impacts of the Deepwater Horizon oil spill on salt marsh periwinkles (Littoraria irrorata). Environ. Sci. Technol. 50, 643–652. doi:10.1021/acs.est.5b04371
Zengel, S., Pennings, S. C., Silliman, B., Montague, C., Weaver, J., Deis, D. R., et al. (2016b). Deepwater Horizon oil spill impacts on salt marsh fiddler crabs (Uca spp.). Estuaries Coasts 39, 1154–1163. doi:10.1007/s12237-016-0072-6
Keywords: Seaside Sparrow, DNA metabarcoding, niche variation hypothesis, diet, hurricane, disturbance, Deepwater Horizon (DWH)
Citation: Snider AM, Bonisoli-Alquati A, Pérez-Umphrey AA, Woltmann S, Stouffer PC and Taylor SS (2022) Diet and disturbance: Seaside Sparrow resource use driven by oiling and Hurricane Isaac. Front. Environ. Sci. 10:978325. doi: 10.3389/fenvs.2022.978325
Received: 25 June 2022; Accepted: 14 November 2022;
Published: 06 December 2022.
Edited by:
Paola Lopez-Duarte, University of North Carolina at Charlotte, United StatesReviewed by:
Scott Rush, Mississippi State University, United StatesCharles William Martin, University of Florida, United States
Copyright © 2022 Snider, Bonisoli-Alquati, Pérez-Umphrey, Woltmann, Stouffer and Taylor. This is an open-access article distributed under the terms of the Creative Commons Attribution License (CC BY). The use, distribution or reproduction in other forums is permitted, provided the original author(s) and the copyright owner(s) are credited and that the original publication in this journal is cited, in accordance with accepted academic practice. No use, distribution or reproduction is permitted which does not comply with these terms.
*Correspondence: Allison M. Snider, YWxsaXNvbm1zbmlkZXJAZ21haWwuY29t