- 1Research Institute of Forestry, Chinese Academy of Forestry, Beijing, China
- 2Department of Biology, Brigham Young University, Provo, UT, United States
- 3Urumqi Seedling Farm, Urumqi, Xinjiang, China
Little is known about the effects of grassland degradation on the carbohydrate-active enzyme (CAZYme) genes responsible for C cycling. Here we used a metagenomic approach to reveal variation in abundance and composition of CAZyme genes in grassland experiencing a range of degradation severity (i.e., non-, light, moderately, and severely degraded) in two soil layers (0–10 cm, 10–20 cm) in a steppe grassland in northern China. We observed a higher CAZyme abundance in severely degraded grassland compared with the other three degradation severities. Glycoside hydrolase (GH) and glycosyltransferase (GT) were identified as the most abundant gene families. The Mantel test and variation partitioning suggested an interactive effect of degradation severity and soil depth with respect to CAZyme gene composition. Structural equation modeling indicated that total soil carbon, microbial biomass carbon and organic carbon were the three soil characteristics most important to CAZyme abundance, which suggests an interaction between degradation and soil carbon fractions in determining CAZyme gene composition. Both above- and below-ground factors linked to soil organic matter play a central role in determining the abundance of CAZyme gene families.
Introduction
Grassland soils store huge carbon stocks and thus play a vital role in global carbon sequestration (Bai and Cotrufo, 2022). The degradation of grasslands due to overgrazing and climate change (Akiyama and Kawamura, 2007; Li et al., 2016), has reduced carbon sequestration capacity and enhanced soil carbon and nitrogen losses (Zhang et al., 2011; Wang et al., 2014; An et al., 2019). The structure and function of soil microbial communities are key indicators of both carbon cycling and grassland degradation (Singh, 2015; Khan et al., 2019; Liang et al., 2019; Yu et al., 2021) because they change throughout grassland degradation, according to soil pH (Che et al., 2019), soil organic carbon concentration (SOC) (Yu et al., 2021) and soil total nitrogen concentration (TN). Grassland degradation could additionally affect soil microbial communities via a decline in the quantity or quality of litter input to the soil (Zhang et al., 2011; He and Richards, 2015). Soil microbes may also exert feedback effects on soil degradation. For example, as an important indicator of fertility, soil microorganisms can aid in the restoration of degraded grasslands by converting exogenous C into stable soil organic C, or by enhancing grassland degradation by intensifying the decomposition of soil organic carbon (Liang et al., 2017; Zhu et al., 2020). Therefore, studying the relationship between grassland degradation and soil microbial activity can help us more effectively remediate degraded grasslands.
Soil microbial communities are usually described either by their taxonomic composition or functional gene composition. Due to limited understanding of the relationship between taxonomy and function, assumptions of functional coherence are usually made for the various taxonomic groups of microorganisms (Philippot et al., 2010; Fierer et al., 2012). However, recent advances in shotgun DNA sequencing methods provide an increased potential for characterizing environmental metagenomes, enhancing the resolution of function down to the responsible genes contained within soil microbial communities. Previous studies on grassland degradation have mainly focused on the taxonomic composition of soil microbial communities (Cai et al., 2014; Che et al., 2019; Zhou et al., 2019), so the effect of grassland degradation on soil microbial function remains unclear.
Metagenomics provides a powerful molecular approach to characterize microbial genes encoding the enzymes taking part in functions such as C cycling. The carbohydrate-active enzymes (CAZymes) are the enzymes involved in the hydrolysis and biosynthesis of complex carbohydrates. CAZymes include glycoside hydrolases (GHs), polysaccharide lyases (PLs), carbohydrate esterases (CEs), glycosyltransferases (GTs), proteins with non-catalytic carbohydrate-binding modules (CBMs), and enzymes with auxiliary activities (AA). The classification of CAZymesis based on the similarity of amino acid sequences in protein domains, which may reflect their biological functions (Cantarel et al., 2008). The GHs cleave the glycosidic bonds within carbohydrates or between a carbohydrate and a non-carbohydrate moiety, and are important in degradation of cellulose, hemicellulose, chitin and glucans. The GTs participate in the formation of glycosidic bonds. The AAs are redox enzymes that act in conjunction with CAZymes, and several AA enzymes play roles in the degradation of cellulose and lignin. The CEs participate in the decomposition of hemicelluloses. The PLs cleave uronic acid-containing polysaccharide chains via a β-Elimination mechanism to generate an unsaturated hexenuronic acid residue and a new reducing end. Catalytically active CAZymes may contain carbohydrate-binding modules (CBMs), which are essential for effective hydrolysis because they mediate binding to cellulose, xylan, chitin or other carbohydrates (Donohoe and Resch, 2015). The CAZyme gene abundances may reflect the C cycling potential of a microbial community. Therefore, in this study annotation with the CAZy database was used to reveal the functional shifts in soil microbial communities following vegetation changes such as been done previously for the conversion of cropland to plantation (Zhang and Lv 2020), reclamation of saline-alkali soil (Yin and Zhang 2022) and afforestation of farmland (Ren et al., 2021). Previous studies have provided CAZy annotations of soil metagenomes in grasslands (Howe et al., 2016; Noronha et al., 2017; Yeager et al., 2017). However, the interactions between soil CAZyme abundances and microbial community structure during grassland degradation is still unclear. While soil microbes mainly function as decomposers in degrading grassland, debate still exists as to whether there are other microbial functional responses to the decreased availability of litter caused by grassland degradation (Liang et al., 2017; Liang et al., 2019). Low litter input has been shown to reduce microbial CAZymes (ˇZifˇc´akov´a et al., 2016; Ren et al., 2017; Zhong et al., 2020), but one may easily envision the case in which microorganisms invest more energy in CAZymes to maximize energy and nutrient capture from the limited supply of litter (Liang et al., 2017; Luo et al., 2017). Thus, understanding the overall status of the C cycle functional genes would be helpful in recognizing the interactions between soil microbial communities and degrading grassland.
A recent study investigated the role of CAZymes as microbial functional responses to C turnover (Cantarel et al., 2008). Presently, there are few papers on specific glycoside hydrolases (GHs) or enzymes with auxiliary activities (AA) that drives C dynamics > These have been related to polysaccharides and lignin decomposition, respectively (Llado et al., 2019; Lopez-Mondejar et al., 2020). Previous reports indicated that plant biomass was mainly degraded by cellulases or hemicellulases (GHs), while lysozymes and chitinases (GHs) were responsible for degrading bacterial and fungal biomass (Lombard et al., 2014; ˇZifˇc´akov´a et al., 2016). It was also reported that soil substrates and environmental characteristics were important controls of the activities of microbial CAZymes, as they can impact microbial communities in soils. Therefore, soil C sources have been suggested as the drivers of the transcription of gene that encode specific CAZymes (Hu et al., 2020; Lopez-Mondejar et al., 2020). Additionally, it should also be noted that season may affect CAZyme activity, leading to changes in C cycling (ˇZifˇc´akov´a et al., 2016).
In the present study, we collected soil samples from various portions of the Xilingol Grassland in Inner Mongolia, China, experiencing different degrees of degradation. Xilingol Grassland is a true steppe and one of the three largest natural grasslands in China. It is located in the middle of the Inner Mongolia Autonomous Region and has an area of 1,79,600 square kilometers. It is an important ecological barrier in northern China. The Xilingol Grassland transitions from meadow grassland to desert grassland as one moves from the northeast to the southwest. The most typical grassland type is distributed in the middle of the Xiling League and accounts for more than half of the Xilingol Grassland area. Since the 1970s, the Xilingol grassland has suffered from various forms of degradation, including desertification. By 2010, the area of degraded, sandy and salinized grassland reached 75.14% of the total grassland area (Bai et al., 2017). Therefore, the objectives of this study were to 1) investigate abundance of CAZyme genesin soils of the Xilingol grassland along a gradient of degradation severity, 2) document the community structure of CAZyme gene along the degradation gradient, and 3) determine the contribution of various environmental variables to variation in CAZyme genes. We hypothesized that 1) grassland degradation causes significant variation in CAZyme gene abundance and composition; 2) grassland degradation increases the abundance of functional genes involved in C decomposition; and 3) plant community type and soil carbon fractions interact to affect CAZyme gene composition.
Materials and methods
Soil sampling
We sampled soils in the Xilingol Grassland, Inner Mongolia, China (45°50′7.74 ″N, 116°33′21.19 ″E). The average annual rainfall and temperature are ∼300 mm and 1.6°C. Past overgrazing resulted in severe grassland degradation in this region. Based on the grassland degradation index (Dong et al., 2019), non-degraded (ungrazed for the last 10 years, GDI = 0.93), lightly degraded (GDI = 0.75), moderately degraded (GDI = 0.63) and severely degraded (GDI = 0.52) sites were chosen for study. All sites occurred within a 5 km × 5 km area to minimize differences in climate and original vegetation composition among them. Vegetation characteristics including plant species, biomass, abundance, vegetation cover, and soil properties including total nitrogen, total carbon, total phosphorus, total potassium, pH and NO3− -N, were also determined and listed in Supplementary Table S1.
At each site we established three plots (1 × 1 m), and from each plot we randomly sampled five soil cores (diameter 4 cm) after removing the humus layer. Two soil depths (0–10 cm and 10–20-cm) were sampled separately but for each depth the five soil cores were pooled to form a single independent sample per plot during the growing season (August) of 2021. Twenty-four samples were obtained (4 sites × 2 depths × 3 replicate plots). Soil samples (200 g) were sieved using a 2-mm mesh to remove debris and stones. Sieved samples were stored in a −80°C freezer prior to DNA extraction and at 4°C prior to chemical analyses. We also recorded the richness and abundance of each plant species from the 1 × 1 m sampling plots.
Metagenomic analysis
A FastDNA SPIN Kit (MP Biomedicals, Santa Ana, CA, United States) was used to obtain soil genomic DNA from 500 mg soil samples based on the manufacturer’s protocol. We diluted the extracted DNA 10 ngμL−1 using a spectrophotometer (NanoDrop ND-1000, NanoDrop Technologies), which was then fragmented to ∼350 bp using an ultrasonicator (Covaris M220, Gene Company Limited, China) to construct paired-end libraries using a TruSeq DNA Sample Prep Kit (Illumina, San Diego, CA, United States). Then, blunt-end fragments were linked with adapters possessing the full complement of sequencing primer hybridization sites. The Illumina HiSeq 4000 PE150 platform was employed for pair-end sequencing (Illumina Inc., San Diego, CA, United States) based on the manufacturer instructions of the HiSeq 3000/4000 PE Cluster Kit and HiSeq 3000/4000 SBS Kits.
Trimmomatic 0.36, a read-trimming tool, was used to process the raw data (Bolger et al., 2014) to remove adapters and reads of moderate quality. Sequences with Q values < 20 were excluded. Short reads were assembled with De bruijn-graph-based assembler Megahit (v1.0.6) (Li et al., 2015), using a 19.7%–40.1% mapped ratio across all the samples. The open reading frames (ORFs) of each metagenomic sample were estimated with Prodigal (v2.60) (Hyatt et al., 2012), and those of lengths >100 bp were translated into amino acid sequences according to the NCBI translation table (https://www.ncbi.nlm.nih.gov/Taxonomy/taxonomyhome.html/index.cgi?chapter = tgencodes#SG1). CD-HIT (http://www.bioinformatics.org/cd-hit/) was used to cluster sequences from gene sets with a 95% sequence identity (90% coverage) into a non-redundant gene catalog, which were then mapped with Bowtie 1.1.2. Next, sam2counts 0.91 was employed to convert the mapped results to reference sequence counts to generate a gene table for functional annotation.
CAZymes in the metagenome contigs were annotated following gene calling with pipeline dbCAN2 and non-redundant protein (Yin et al., 2012), according to the manual curation of sequence alignments from the CAZy database (July 2020). Non-redundant protein taxonomical annotations were determined using the easy-taxonomy mode and nr database of MMseqs2 (Steinegger and S¨oding, 2017). Next, we mapped the high-quality sequences of the samples on the predicted gene sequences to assess the abundance of these genes using Salmon (Patro et al., 2017). We then used the transcripts per kilobase million (TPM) mapped reads to normalize the abundance values in the metagenomes, and normalized the total sequencing number to one million reads per sample to avoid the sequencing depth bias in the comparative analysis.
Soil properties
We determine soil total nitrogen (TN), total carbon (TC), total potassium, total phosphorus, extractable phosphorus, pH, water content, NH4+-N, NO3−-N, organic carbon, microbial biomass carbon (MBC), microbial biomass nitrogen (MBN), and microbial biomass phosphorus (MBP). Soil TC and TN were determined by performing elemental analyses (Elementar Vario MACRO, Germany). An automated discrete analysis was used to quantify ammonium nitrogen (NH4+-N) and nitrate nitrogen (NO3−-N) (CleverChem 380, Germany). Total K was determined by flame photometry (Lu, 1999). Total and extractable P concentrations were determined using a spectrophotometer (UV-1600 spectrophotometer, Beijing) (Lu, 1999). The standard Walkley-Black potassium dichromate oxidation method (Nelson and Sommers, 1982) was used for organic carbon. A 1:1 ratio of soil to water was used to measure soil pH (HANNA, Padova, Italy). Additionally, we gravimetrically measured soil water content (SWC) by oven drying to constant mass at 105°C, and MBC, MBN, and MBP were determined using the chloroform fumigation extraction method.
Statistical analysis
The effect of grassland degradation severity and soil depth on CAZyme gene family read abundances were determined using two-way analyses of variance (ANOVAs). If ANOVA indicated significant effects, then the means of treatments were compared using Tukey’s honestly significant difference test (p < 0.05). Two-way ANOVA and multiple comparisons were also performed on each of the glycoside hydrolase (GH), glycosyltransferase (GT), polysaccharide lyases (PL), carbohydrate esterase (CE), carbohydrate-binding module (CBM), and auxiliary activity (AA) families.
Non-metric multidimensional scaling (NMDS) analysis was performed using Bray-Curtis distances to assess variation in the CAZyme gene community structure. Significant differences in CAZyme gene communities among different degradation severities and soil depths were determined using the PerMANOVA test (Vegan package in R, Oksanen et al., 2013).
After confirming that the structure of a CAZyme gene family was significantly affected by treatment, indicator species analyses were conducted to investigate genes with significantly different abundances using the ‘signassoc’ function in the ‘indicspecies’ package (DeCáceres and Legendre 2009) according to each gene’s abundance in a sample. The mode zero (site-based) was used and p-values were obtained following Sidak’s correction for multiple testing.
We assessed the association of CAZyme gene composition with plant community composition or soil characteristics using the Mantel tests. Partial mantel tests were performed between CAZyme gene composition and plant community composition or soil characteristics after controlling for other factors.
Variance partitioning of Bray-Curtis distances of CAZyme gene compositions was performed using a multiple regression for distance matrices (Swenson, 2014) to assess the significance of plant community compositions and soil characteristics in determining CAZyme gene composition.
We built structural equation models (SEM) with Mantel R values as inputs in AMOS 20.0 (Arbuckle, 2011) to determine the direct and indirect effects of plant communities on soil characteristics of the CAZyme gene families. Basing on the Mantel test and variance partitioning, which showed a significant interactive effect between plant communities and soil characteristics, a conceptual model was built whereby plant communities affected soil characteristics and both types of variables jointly affected CAZyme gene composition. SEM models with observations were compared using a maximum likelihood estimation method. Based on the methodology of Hu and Bentler (1999), we assessed the goodness of fit using a root mean squared error of approximation (RMSEA) <0.05 and TLI (Tucker-Lewis Index) >0.95.
Results
Variation in CAZyme gene abundance across grassland degradation severity and soil depth
There were a total of 1,676,180 CAZyme gene sequences (minimum 67,091; maximum 72,168; mean 69,840 per sample). GT and GH were identified as the two dominant families of genes, representing an average of 32.29% and 25.33%, respectively.
Degradation severity and soil depth, and their interaction, were significant with respect to CAZyme gene sequence abundance (p < 0.05; Figure 1; Supplementary Table S2). CAZyme gene abundance was significantly different between the two soil depths (p < 0.05). For the lightly and moderately degraded grassland, the abundance of the CAZyme genes were significantly higher in the 10–20 cm depth soil than in 0–10 cm soil depth. For the non- and severely degraded grassland, CAZyme gene abundances were not different between the two soil layers. Among the different degradation severities, CAZyme gene abundance significantly differed between non-degraded and severely degraded, between lightly and severely degraded, and between moderately and severely degraded, but not among non-degraded, lightly degraded and moderately degraded grassland (Figure 1; Supplementary Table S3).
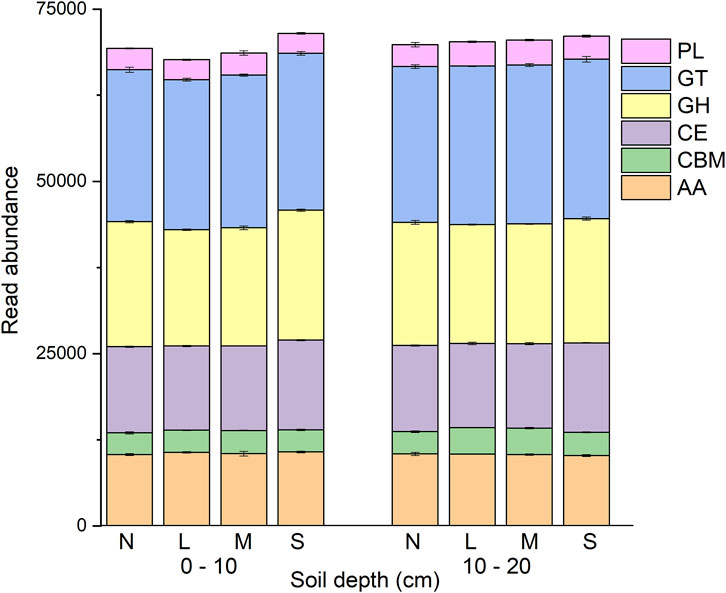
FIGURE 1. Read abundance of CAZyme families in grassland soil under various degradation severities and soil depth. N: non-degraded; L: lightly degraded; M: moderately degraded; S: severely degraded.
Structures of CAZyme gene composition across grassland degradation severity and soil depth
The NMDS together with the two-way PerMANOVA (p < 0.05 for degradation severity, soil depth, and their interaction) indicated a significant difference in the structure of CAZyme gene families across degradation severity and soil depth (Figure 2; Table 1).
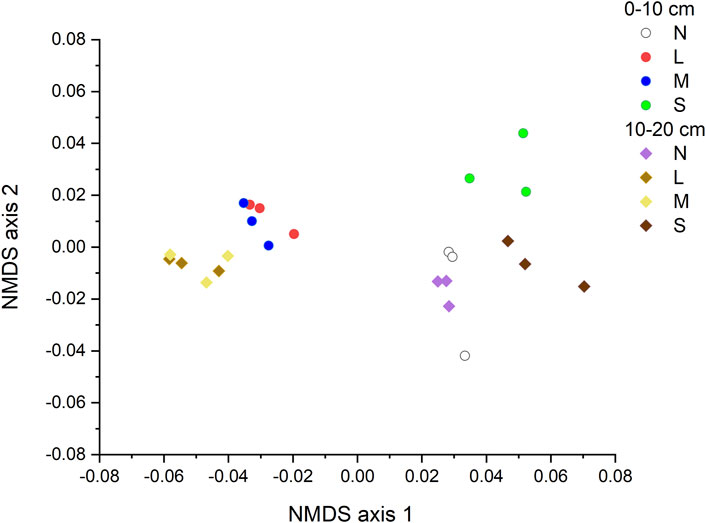
FIGURE 2. NMDS showing communities structures of CAZyme families under various degradation severities and soil depth. N: non-degraded; L: lightly degraded; M: moderately degraded; S: severely degraded.
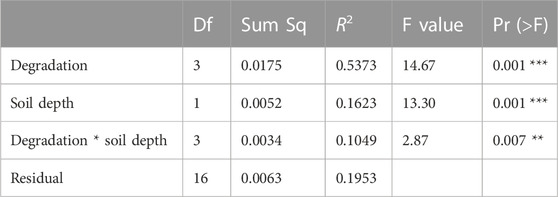
TABLE 1. Two-way PerMANOVA of degradation severity and soil depth on composition of CAZyme gene families. Df: degree of freedom; Sum Sq: sum of squares.
Indicator species analysis identified 201 gene families that differed significantly in abundance across treatments (Figure 3). Of these, 100 gene families were significantly higher in severely degradation grassland, of which 52 had a soil depth exceeding 10–20 cm and 48 exceeding 0–10 cm (Figure 3). Further, 49 out of the 100 gene families enriched in severely degraded sites were GHs, of which 20 had a soil depth exceeding 10–20 cm, and 29 exceeded 0–10 cm (Figure 3).
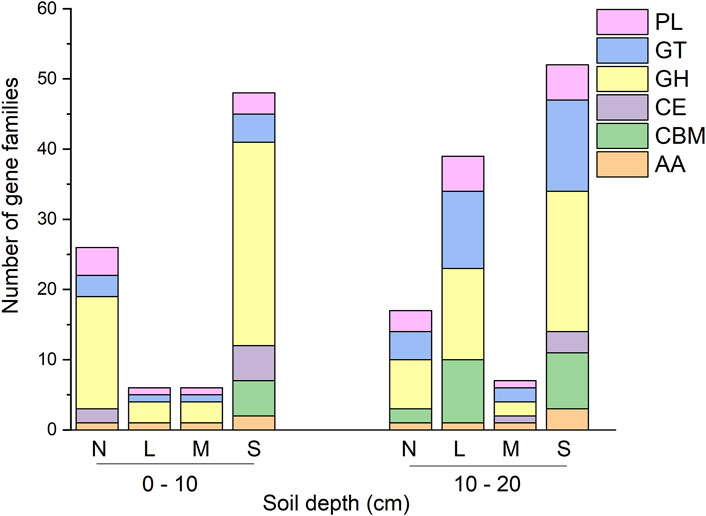
FIGURE 3. Richness of CAZyme indicator gene families that were found to be enriched in each degradation severity and soil depth. N: non-degraded; L: lightly degraded; M: moderately degraded; S: severely degraded.
Relationship between structures of CAZyme gene families and plant community and soil characteristics
Mantel tests showed a significant relationship between the structure of CAZyme gene families and plant communities (p < 0.05; Table 2) and soil characteristics (p < 0.05; Table 2), at both of the soil depths. In the partial Mantel test, after controlling for soil characteristics, plant communities showed a significant relationship with structure of CAZyme gene families (p < 0.05; Table 2). However, soil characteristics were not significantly related to CAZymes after controlling for plant community structure (p > 0.05; Table 2).
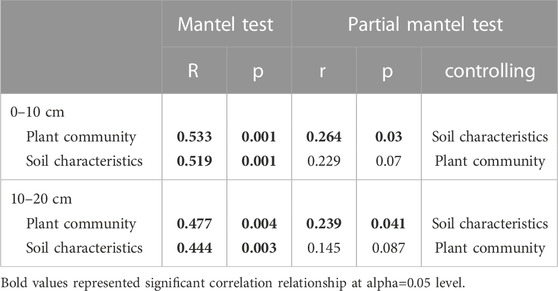
TABLE 2. Mantel test and partial mantel test between CAZyme gene composition and plant community or soil characteristics.
Using the distance matrix multiple regression, plant communities and soil characteristics variance partitioning was used to examine their relative influence on CAZyme gene structure. These two factors together explained 30% and 22% for CAZyme composition at soil depths of 0–10 cm and 10–20 cm, respectively (Figure 4). The interpretation strength is mainly due to the interaction of plant communities and soil characteristics, with 23% and 18% for CAZyme structure at soil depths of 0–10 cm and 10–20 cm.
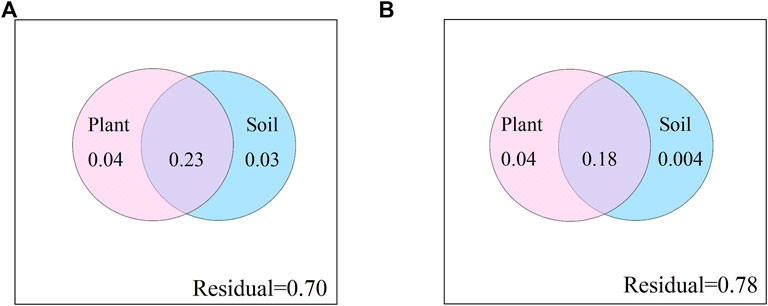
FIGURE 4. Variance partitioning of structure of CAZyme gene composition among plant community composition and soil characteristics. (A):0–10 cm; (B):10–20 cm.
We found that plant community structure and soil characteristics explained much of CAZyme structure in the SEM model for both of the soil layers (for 0–10 cm, Chi-square = 0.375, df = 3, probability level = 0.945, RSMEA = 0.001, TLI = 0.999; for 10–20 cm, Chi-square = 0.004, df = 2, probability level = 0.998, RSMEA = 0.001, TLI = 0.999) (Figure 5). The final model explained 33% and 37% of the variation in structure of CAZyme gene families for soil depths of 0–10 cm and 10–20 cm, respectively. Total carbon, organic carbon and MBC explained significant variation in the structure of CAZyme composition. Furthermore, the SEM model also identified the interactive effect of plant community structure and soil characteristics. Plant community structure had an indirect effect on CAZyme composition by influencing soil total carbon or soil organic carbon. Soil total carbon and organic carbon also exerted an indirect effect on CAZyme composition by influencing MBC.
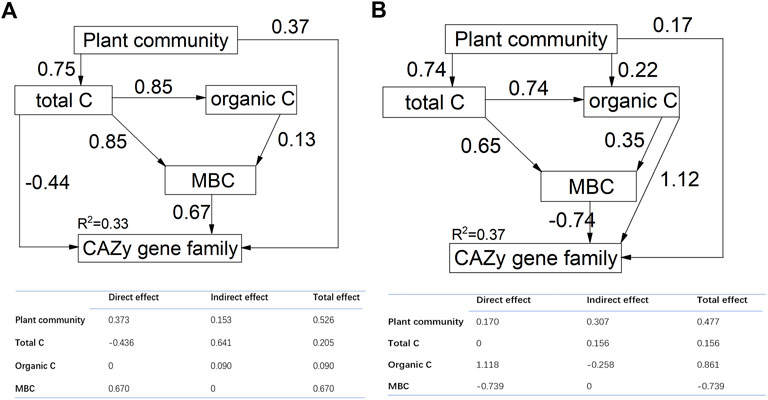
FIGURE 5. Structural equation models (SEM) showing the direct effects of plant community and soil characteristics and their indirect effects on CAZyme families. The numbers above the arrows indicate path coefficients. R2 values represent the proportion of variance explained by each variable. Adequate model fits are indicated by root square mean errors of approximation (RSMEA <0.06) and TLI (Tucker-Lewis Index >0.95). Each path in this model is significant with p < 0.05. The table shows the standardized direct, indirect and total effects. (A):0–10 cm; (B):10–20 cm.
Discussion
Grasslands sequester large amounts of organic C and are thus important for climate regulation (Bai and Cotrufo, 2022). As grasslands become degraded due to human activity and climate change, their ability to store carbon may be compromised. We therefore set out to determine the impact of grassland degradation on enzyme families responsible for much of C cycling in ecosystems. Our study showed that the abundance of soil microbial CAZyme gene families significantly increased in the severely degraded grassland compared to the grasslands of the other three degradation severities. Therefore, the ability to sequester C may be reduced in severely degraded grassland.
Severe degradation in grassland is accompanied by a decrease in litter input to soil and a decrease in plant-derived substrates for soil microorganisms. In our study, plant biomass decreased with increased degradation; plant biomass in severely degraded grassland decreased by 76% compared to the non-degraded grassland (Supplementary Table S1). Previous studies showed that nutrient limitations due to low litter input could restrict the growth of microbes and decrease CAZymes abundance (ˇZifˇc´akov´a et al., 2016; Ren et al., 2017; Zhong et al., 2020). However, some studies show that microorganisms secrete more extracellular enzymes with decreased litter input (Liang et al., 2017; Liang et al., 2019), which is consistent with our results For example, several abundant AAs and GHs encoding for decomposition of litter components were observed to be enriched in the severely degraded grassland (Supplementary Table S4). This suggests that enhanced microorganism enzyme activity might be achieved at the expense of metabolic reserves (ˇZifˇc´akov´a et al., 2016) in the severely degraded grassland.
In addition, GTs were enriched in the severely degraded grassland (Supplementary Table S4). GTs are related to peptidoglycan hydrolases and synthetases of bacterial cell walls. In some circumstances, bacteria may remodel their cell walls according to environmental changes (Horcajo et al., 2012; Cava and de Pedro, 2014; Bernal-Cabas et al., 2015). Severely degraded grassland experienced a significant increase in genes associated with bacterial outer membrane and cell wall biogenesis (COG0399 and COG2982) (data were not shown here) according to the eggNOG database (Huerta-Cepas et al., 2019). As degradation increases, grasslands experience larger environmental fluctuations as a consequence of reduced vegetation cover and poorer soil nutrient status. These changes in enzyme abundance in the severely degraded grassland may function to maintain cell wall homeostasis and enable adaptation to environmental disturbance.
Our results showed that structure of CAZyme genes varied significantly across a gradient of grassland degradation (Figure 2; Table 1), which was supported by Luo et al. (2020). The variation across the degradation gradient was associated with variation in plant community structure and soil characteristics and their interaction. Further, SEM suggests that CAZyme variation was primarily driven by interaction between plant community structure and soil total carbon, organic carbon and MBC. It was also demonstrated that organic matter and energy flux had pivotal roles in ecosystems’ responses to degradation above- and below-ground (Hagedorn et al., 2009; Breidenbach et al., 2022). In this study, the NMDS showed that non-degraded grassland and the severe degraded grassland are grouped for the CAZyme gene composition. In grassland, severe degradation may result from overgrazing and subsequent plant species replacement, reduced plant production, root death and decomposition. These changes could also affect the structures and functions of microbial communities for adapting to reduced SOC contents. Thus, the results of NMDS might originate from a stimulation of microorganism enzyme activity by low substrate input in the severely degraded grassland, making it similar to that in the non-degraded grassland (ˇZifˇc´akov´a et al., 2016). On the other hand, these phenomena may further promote the loss of fine-textured soil containing SOC.
Implications, limitations, and future directions
This study provided empirical evidence that abundance and composition structure of the soil C cycling functional genes, CAZyme gene families, varied across a gradient of grassland degradation. Some CAZyme families were overrepresented in the severely degraded grassland. The result of high CAZyme abundance and low litter input may lead to reduced C sequestration. The decrease in SOC in the severely degraded grassland suggests that unstabilized organic matter of plant origin (plant litter and root exudates) is rapidly mineralized. Moreover, a recent study reported that microbial functional changes result in an irreversible degradation of Tibetan Plateau pastures (Breidenbach et al., 2022). Thus, conventional strategies excluding grazing to mitigate severe degradation is unlikely to lead to ecosystem recovery without intervention. We urgently need to seek for ecological restoration approaches for severely degraded grassland. Reseeding may be one approach to restoring severely degraded grassland, but one must be aware that conventional N fertilization often carries the risk of N leaching and pollution (Mejias et al., 2021). For the non-degraded, lightly degraded and moderately degraded grasslands, more reasonable levels of grazing (e.g., rotational grazing; Dong et al., 2022) compared to the current grazing pattern could be explored to prevent further ecosystem degradation.
An important limitation associated with this present study was that we only performed sampling on a single occasion with only three replicate of each degradation severity. Thus, it is difficult for us to generalize our findings to other grassland systems or to our grassland at different times of year. Thus, more studies comprising larger temporal and spatial scales across various degraded and restored grassland ecosystems are needed. Furthermore, future studies might fruitfully consider microbial activity with respect to C sequestration using meta-transcriptome and metaproteome approaches in the prediction of C accumulation.
Data availability statement
The data presented in the study are deposited in the CNGBdb repository, accession number CNP0003968.
Author contributions
Conceived the ideas: QZ and JC. Field investigation and sampling: QZ, XX, JD, and LX. Analysis and interpretation of data: QZ and JC. Drafting of the manuscript: QZ. Critical revision of the manuscript for important intellectual content: QZ, XX, JD, RK, LX, and JC.
Funding
This work was supported by the Strategic Priority Research Program of Chinese Academy of Sciences (Grant No. XDA26020102), the Central Public-interest Scientific Institution Basal Research Fund (CAFYBB2020ZB001) and the Natural Science Foundation of China (No. 31870099).
Conflict of interest
The authors declare that the research was conducted in the absence of any commercial or financial relationships that could be construed as a potential conflict of interest.
Publisher’s note
All claims expressed in this article are solely those of the authors and do not necessarily represent those of their affiliated organizations, or those of the publisher, the editors and the reviewers. Any product that may be evaluated in this article, or claim that may be made by its manufacturer, is not guaranteed or endorsed by the publisher.
Supplementary material
The Supplementary Material for this article can be found online at: https://www.frontiersin.org/articles/10.3389/fenvs.2023.1080505/full#supplementary-material
References
Akiyama, T., and Kawamura, K. (2007). Grassland degradation in China: Methods of monitoring, management and restoration. Grassl. Sci. 53, 1–17. doi:10.1111/j.1744-697X.2007.00073.x
An, H., Tang, Z. S., Keesstra, S., and Shangguan, Z. P. (2019). Impact of desertification on soil and plant nutrient stoichiometry in a desert grassland. Sci. Rep. 9, 9422. doi:10.1038/s41598-019-45927-0
Arbuckle, J. L. (2011). IBMVR SPSSVR AmosTM 20 user’s guide. Armonk, New York, USA: IBM Corporation.
Bai, Y. F., and Cotrufo, M. F. (2022). Grassland soil carbon sequestration: Current understanding, challenges, and solutions. Science 377, 603–608. doi:10.1126/science.abo2380
Bai, Y. F., Pan, Q. M., and Xing, Q. (2017). Fundamental theories and technologies for optimizing the production functions and ecological functions in grassland ecosystems. Chin. Sci. Bull. 12, 201–212. doi:10.1360/N972015-00768
Bernal-Cabas, M., Ayala, J. A., and Raivio, T. L. (2015). The Cpx envelope stress response modifies peptidoglycan cross-linking via the L,D-transpeptidase LdtD and the novel protein YgaU. J. Bacteriol. 197 (3), 603–614. doi:10.1128/JB.02449-14
Bolger, A. M., Lohse, M., and Usadel, B. (2014). Trimmomatic: A flexible trimmer for illumina sequence data. Bioinformatics 30, 2114–2120. doi:10.1093/bioinformatics/btu170
Breidenbach, A., Schleuss, P. M., Liu, S., Schneider, D., Dippold, M. A., de la Haye, T., et al. (2022). Microbial functional changes mark irreversible course of Tibetan grassland degradation. Nat. Commun. 13, 2681. doi:10.1038/s41467-022-30047-7
Cai, X. B., Peng, Y. L., Yang, M. N., Zhang, T., and Zhang, Q. (2014). Grassland degradation decrease the diversity of arbuscular mycorrhizal fungi species in tibet plateau. Not. Bot. Horti Agrobot. Cluj-Napoca 42, 333–339. doi:10.15835/nbha.42.2.9458
Cantarel, B. L. I., Coutinho, P. M., Rancurel, C., Bernard, T., Lombard, V., and Henrissat, B. (2008). The Carbohydrate-Active EnZymes database (CAZy): An expert resource for Glycogenomics. Nucleic Acids Res. 37 (1), D233–D238. doi:10.1093/nar/gkn663
Cava, F., and de Pedro, M. A. (2014). Peptidoglycan plasticity in bacteria: Emerging variability of the murein sacculus and their associated biological functions. Curr. Opin. Microbiol. 18 (1), 46–53. doi:10.1016/j.mib.2014.01.004
Che, R. X., Wang, Y. F., Li, K. X., Xu, Z. H., Hu, J. M., Wang, F., et al. (2019). Degraded patch formation significantly changed microbial community composition in alpine meadow soils. Soil till. Res. 195, 104426. doi:10.1016/j.still.2019.104426
De Cáceres, M., and Legendre, P. (2009). Associations between species and groups of sites: Indices and statistical inference. Ecology 90, 3566–3574. doi:10.1890/08-1823.1
Dong, C., Wang, W., Liu, H., Xu, X., and Zeng, H. (2019). Temperate grassland shifted from nitrogen to phosphorus limitation induced by degradation and nitrogen deposition: Evidence from soil extracellular enzyme stoichiometry. Ecol. Indic. 101, 453–464. doi:10.1016/j.ecolind.2019.01.046
Dong, L., Zheng, Y., Martinsen, V., Liang, C., and Mulder, J. (2022). Effect of grazing exclusion and rotational grazing on soil aggregate stability in typical grasslands in Inner Mongolia, China. Front. Environ. Sci. 10, 844151. doi:10.3389/fenvs.2022.844151
Donohoe, B. S., and Resch, M. G. (2015). Mechanisms employed by cellulase systems to gain access through the complex architecture of lignocellulosic substrates. Curr. Opin. Chem. Biol. 29, 100–107. doi:10.1016/j.cbpa.2015.08.014
Fierer, N., Leff, J. W., Adams, B. J., Nielsen, U. N., Bates, S. T., Lauber, C. L., et al. (2012). Cross-biome metagenomic analyses of soil microbial communities and their functional attributes. P. Natl. Acad. Sci. U. S. A. 109, 21390–21395. doi:10.1073/pnas.1215210110
Hagedorn, F., Gavazov, K., and Alexander, J. M. (2009). Above- and belowground linkages shape responses of mountain vegetation to climate change. Science 365, 1119–1123. doi:10.1126/science.aax4737
He, S., and Richards, K. (2015). Impact of meadow degradation on soil water status and pasture management—a case-study in tibet. Land Degrad. Dev. 26, 468–479. doi:10.1002/ldr.2358
Horcajo, P., De Pedro, M. A., and Cava, F. (2012). Peptidoglycan plasticity in bacteria: Stress-induced peptidoglycan editing by noncanonical D-amino acids. Microb.Drug Resist. 18 (3), 306–313. doi:10.1089/mdr.2012.0009
Howe, A., Yang, F., Williams, R. J., Meyer, F., and Hofmockel, K. S. (2016). Identification of the core set of carbon-associated genes in a bioenergy grassland soil. PLoS ONE 11 (11), e0166578. doi:10.1371/journal.pone.0166578
Hu, L., and Bentler, P. M. (1999). Cutoff criteria for fit indexes in covariance structure analysis: Conventional criteria versus new alternatives. Struct. Equ. Model. 6, 1–55. doi:10.1080/10705519909540118
Hu, Y. T., Zheng, Q., Noll, L., Zhang, S. S., and Wanek, W. (2020). Direct measurement of the in situ decomposition of microbial-derived soil organic matter. Soil Biol. biochem. 141, 107660. doi:10.1016/j.soilbio.2019.107660
Huerta-Cepas, J., Szklarczyk, D., Heller, D., Hernández-Plaza, A., Forslund, S. K., Cook, H., et al. (2019). eggNOG 5.0: a hierarchical, functionally and phylogenetically annotated orthology resource based on 5090 organisms and 2502 viruses. Nucleic Acids Res. 47 (D1), D309–D314. doi:10.1093/nar/gky1085
Hyatt, D., Locascio, P. F., Hauser, L. J., and Uberbacher, E. C. (2012). Gene and translation initiation site prediction in metagenomic sequences. Bioinformatics 28, 2223–2230. doi:10.1093/bioinformatics/bts429
Khan, A., Kong, W., Muhammad, S., Wang, F., Zhang, G., and Kang, S. (2019). Contrasting environmental factors drive bacterial and eukaryotic community successions in freshly deglaciated soils. FEMS Microbiol. Lett. 366, fnz229. doi:10.1093/femsle/fnz229
Li, D. H., Liu, C. M., Luo, R. B., Sadakane, K., and Lam, T. W. (2015). Megahit: An ultra-fast single-node solution for large and complex metagenomics assembly via succinct de Bruijn graph. Bioinformatics 31, 1674–1676. doi:10.1093/bioinformatics/btv033
Li, Y. M., Wang, S. P., Jiang, L. L., Zhang, L. R., Cui, S. J., Meng, F. D., et al. (2016). Changes of soil microbial community under different degraded gradients of alpine meadow. Agr. Ecosyst. Environ. 222, 213–222. doi:10.1016/j.agee.2016.02.020
Liang, C., Amelung, W., Lehmann, J., and Kästner, M. (2019). Quantitative assessment of microbial necromass contribution to soil organic matter. Glob. Chang. Biol. 25, 3578–3590. doi:10.1111/gcb.14781
Liang, C., Schimel, J. P., and Jastrow, J. D. (2017). The importance of anabolism in microbial control over soil carbon storage. Nat. Microbiol. 2, 17105. doi:10.1038/nmicrobiol.2017.105
Llado, S., Vetrovsky, T., and Baldrian, P. (2019). Tracking of the activity of individual bacteria in temperate forest soils shows guild-specific responses to seasonality. Soil Biol. biochem. 135, 275–282. doi:10.1016/j.soilbio.2019.05.010
Lombard, V., Ramulu, H. G., Drula, E., Coutinho, P. M., and Henrissat, B. (2014). The carbohydrate-active enzymes database (CAZy) in 2013. Nucleic Acids Res. 42, D490–D495. doi:10.1093/nar/gkt1178
Lopez-Mondejar, R., Tlaskal, V., Vetrovsky, T., Stursova, M., Toscan, R., da Rocha, U. N., et al. (2020). Metagenomics and stable isotope probing reveal the complementary contribution of fungal and bacterial communities in the recycling of dead biomass in forest soil. Soil Biol. biochem. 148, 107875. doi:10.1016/j.soilbio.2020.107875
Luo, L., Meng, H., and Gu, J. D. (2017). Microbial extracellular enzymes in biogeochemical cycling of ecosystems. J. Environ. Manage. 197, 539–549. doi:10.1016/j.jenvman.2017.04.023
Luo, Z. M., Liu, J. X., Jia, T., Chai, B. F., and Wu, T. H. (2020). Soil bacterial community response and nitrogen cycling variations associated with subalpine meadow degradation on the Loess Plateau, China. Appl. Environ. Microb. 86, e00180-20. doi:10.1128/AEM.00180-20
Mejias, J. H., Salazar, F., Pérez Amaro, L., Hube, S., Rodriguez, M., and Alfaro, M. (2021). Nanofertilizers: A cutting-edge approach to increase nitrogen use efficiency in grasslands. Front. Environ. Sci. 9, 635114. doi:10.3389/fenvs.2021.635114
Noronha, M. F., Lacerda, G. V., Gilbert, J. A., and de Oliveira, V. M. (2017). Taxonomic and functional patterns across soil microbial communities of global biomes. Sci. Total Environ. 609, 1064–1074. doi:10.1016/j.scitotenv.2017.07.159
Oksanen, J., Blanchet, F. G., Kindt, R., Legendre, P., Minchin, P. R., O’Hara, R. B., et al. (2013). Vegan: Community ecology package. R package version 2.0–10. http://CRAN.R-project.org/package=vegan.
Patro, R., Duggal, G., Love, M. I., Irizarry, R. A., and Kingsford, C. (2017). Salmon provides fast and bias-aware quantification of transcript expression. Nat. Methods 14, 417–419. doi:10.1038/nmeth.4197
Philippot, L., Andersson, S. G., Battin, T. J., Prosser, J. I., Schimel, J. P., Whitman, W. B., et al. (2010). The ecological coherence of high bacterial taxonomic ranks. Nat. Rev. Microbiol. 8, 523–529. doi:10.1038/nrmicro2367
Ren, C. J., Chen, J., Deng, J., Zhao, F. Z., Han, X. H., Yang, G. H., et al. (2017). Response of microbial diversity to C: N:P stoichiometry in fine root and microbial biomass following afforestation. Bio. Fert. Soils 53, 457–468. doi:10.1007/s00374-017-1197-x
Ren, C., Zhang, X., Zhang, S., Wang, J., Xu, M., Guo, Y., et al. (2021). Altered microbial cazyme families indicated dead biomass decomposition following afforestation. Soil Biol. biochem. 160, 108362. doi:10.1016/j.soilbio.2021.108362
Singh, J. S. (2015). Microbes: The chief ecological engineers in reinstating equilibrium in degraded ecosystems. Agr. Ecosyst. Environ. 203, 80–82. doi:10.1016/j.agee.2015.01.026
Steinegger, M., and S¨oding, J. (2017). MMseqs2 enables sensitive protein sequence searching for the analysis of massive data sets. Nat. Biotechnol. 35, 1026–1028. doi:10.1038/nbt.3988
Wang, X., Dong, S., Yang, B., Li, Y., and Su, X. (2014). The effects of grassland degradation on plant diversity, primary productivity, and soil fertility in the alpine region of Asia's headwaters. Environ. Monit. Assess. 186, 6903–6917. doi:10.1007/s10661-014-3898-z
Yeager, C. M., Gallegos-Graves, L., Dunbar, J., Hesse, C. N., Daligault, H., and Kuske, C. R. (2017). Polysaccharide degradation capability of Actinomycetales soil isolates from a semiarid grassland of the Colorado Plateau. Appl. Environ. Microbiol. 83, e03020-16. doi:10.1128/AEM.03020-16
Yin, F., and Zhang, F. (2022). Reclamation of abandoned saline-alkali soil increased soil microbial diversity and degradation potential. Plant Soil 477, 521–538. doi:10.1007/s11104-022-05451-z
Yin, Y., Mao, X., Yang, J., Chen, X., Mao, F., and Xu, Y. (2012). dbCAN: a web resource for automated carbohydrate-active enzyme annotation. Nucleic Acids Res. 40, W445–W451. doi:10.1093/nar/gks479
Yu, Y., Zheng, L., Zhou, Y. J., Sang, W. G., Zhao, J. N., Liu, L., et al. (2021). Changes in soil microbial community structure and function following degradation in a temperate grassland. J. Plant Ecol. 14, 384–397. doi:10.1093/jpe/rtaa102
Zhang, G. G., Kang, Y. M., Han, G. D., Mei, H., and Sakurai, K. (2011). Grassland degradation reduces the carbon sequestration capacity of the vegetation and enhances the soil carbon and nitrogen loss. Acta Agr. Scand. B-S.P. 4, 356–364. doi:10.1080/09064710.2010.495079
Zhang, L., and Lv, J. P. (2020). Metagenomic analysis of microbial community and function reveals the response of soil respiration to the conversion of cropland to plantations in the Loess Plateau of China. Glob. Ecol. Conserv. 23, e01067. doi:10.1016/j.gecco.2020.e01067
Zhong, Z. K., Li, W. J., Lu, X. Q., Gu, Y. Q., Wu, S. J., Shen, Z. Y., et al. (2020). Adaptive pathways of soil microorganisms to stoichiometric imbalances regulate microbial respiration following afforestation in the Loess Plateau, China. Soil Biol. biochem. 151, 108048. doi:10.1016/j.soilbio.2020.108048
Zhou, H., Zhang, D. G., Jiang, Z. H., Sun, P., Xiao, H. L., Wu, Y. X., et al. (2019). Changes in the soil microbial communities of alpinesteppe at Qinghai-Tibetan Plateau under different degradation levels. Sci. Total Environ. 651, 2281–2291. doi:10.1016/j.scitotenv.2018.09.336
Zhu, X., Jackson, R. D., DeLucia, E. H., Tiedje, J. M., and Liang, C. (2020). The soil microbial carbon pump: From conceptual insights to empirical assessments. Glob. Change Biol. 26, 6032–6039. doi:10.1111/gcb.15319
Keywords: grassland degradation, CAZyme, soil organic carbon, plant community, metagenomics
Citation: Zhang Q, Xu X, Duan J, Koide RT, Xu L and Chu J (2023) Variation in microbial CAZyme families across degradation severity in a steppe grassland in northern China. Front. Environ. Sci. 11:1080505. doi: 10.3389/fenvs.2023.1080505
Received: 26 October 2022; Accepted: 12 January 2023;
Published: 21 February 2023.
Edited by:
Katerina Dontsova, University of Arizona, United StatesReviewed by:
Shaokun Wang, Northwest Institute of Eco-Environment and Resources (CAS), ChinaYuntao Hu, Berkeley Lab (DOE), United States
Copyright © 2023 Zhang, Xu, Duan, Koide, Xu and Chu. This is an open-access article distributed under the terms of the Creative Commons Attribution License (CC BY). The use, distribution or reproduction in other forums is permitted, provided the original author(s) and the copyright owner(s) are credited and that the original publication in this journal is cited, in accordance with accepted academic practice. No use, distribution or reproduction is permitted which does not comply with these terms.
*Correspondence: Jianmin Chu, Y2ptY2FmQDE2My5jb20=