- 1Department of Economics, Gomal University, Dera Ismail Khan, Pakistan
- 2School of Economics, Bahauddin Zakariya University, Multan, Pakistan
- 3Faculty of Computer Science and Engineering, 1 Decembrie 1918 University of Alba Iulia, Alba Iulia, Romania
- 4Department of Economics, Lahore College for Women University, Lahore, Pakistan
- 5Department of Economics, COMSATS University Islamabad, Vehari Campus, Islamabad, Pakistan
- 6School of Economics and Business Administration, Danubius University, Galati, Romania
Studies investigating the interconnection of health poverty and climatic variability are rare in spatial perspectives. Given the importance of sustainable development goals 3, goal 10, and goal 13, we explored whether the geographic regions with diverse climate structure has a spatial association with health poverty; whether spatial disparities exist across districts of Pakistan. We implied the A-F methodology to estimate the MHP index using the PSLM survey, 2019–20. The climate variables were extracted from the online NASA website. We applied the spatial techniques of Moran’s I, univariate and bivariate LISA, to address the research questions. The findings revealed that the magnitude of MHP differs across districts. Punjab was found to be the better-ff whereas Baluchistan was the highest health poverty-stricken province. The spatial results indicated positive associations of MHP and climate indicators with their values in the neighbors, whereas a negative spatial association was found between the MHP and climate indicators. Also, spatial clusters and outliers of higher MHP were significant in Baluchistan and KP provinces. Government intervention and policymaker’s prioritization are needed towards health and health-related social indicators, mainly in the high poverty-stricken districts, with high temperature and low humidity and precipitation rates, especially in Baluchistan.
1 Introduction
Climate change and health poverty are the two central goals among the United Nation Sustainable Development Goals (SDGs). The first is to ensure healthy lives and promote wellbeing for all ages, and the second is to take urgent action to combat climate change and its impact (UNDP, 2015a; UNDP, 2015b). Climate change occurs in different ways, such as temperature, rainfall, humidity/precipitation, CO2, air pollution, wind/pressure, etc. Global warming, or global rise in temperature, has become an incontrovertible fact, and it had been predicted that global warming would rise in the 21st century from human activities (Hansen et al., 1981). There is growing evidence that climate change has a significant impact on human health and economic growth (Sheridan and Allen, 2015; Kjellstrom et al., 2016; Gilmont et al., 2018; Kinney, 2018; Jones, 2019; Patrick et al., 2019; Sangkhaphan and Shu, 2019; Nuţă et al., 2021; Abban et al., 2022; Dankyi et al., 2022; Li et al., 2022; Khan et al., 2023; Zhang et al., 2023). Climate change has an inverse bearing on the health sector, particularly in developing countries. By 2050 climate change will mostly aggravate the prevailing health difficulties, and the population affected by climate-related sicknesses will increase (Barros et al., 2014). Climate change influences health-related outcomes through air pollution, changing exposure to heat and cold, food safety hazards, pollen, emerging infections and functioning of health services and facilities, and other reasons such as water-borne diseases and increased exposure to Ultra-Violence radiations, changing prices of and access to nutrition (Hames and Vardoulakis, 2012; Morris et al., 2015; Kovats and Osborn, 2016).
Changes in the climate distress the social and environmental attributes of healthcare, such as safe drinking water, clean air, secure shelter, and adequate food and nutrition (WHO, 2021). Such climate-sensitive health risks are typically felt by the deprived and most vulnerable women, children, and poor societies, especially from low- and middle-income countries (WHO, 2021). Increasing access to primary healthcare services and acute hospital care mitigate the impact of temperature on mortality (Paterson et al., 2014; Cheung et al., 2020; Mullins and White, 2020). Recently, (Boyles et al., 2021), stated that environmental attributes are related to maternal health impacts such as pregnancy, infertility, breast cancer, and metabolic disorders. A bunch of previous literature has shown that climate change has a substantial impact on child health (Thompson et al., 2012; Bennett and Friel, 2014; Davenport et al., 2017; Bhandari et al., 2020; Helldén et al., 2021), or maternal health (Giulia et al., 2020; Khan et al., 2011; Kuehn and McCormick, 2017; Rylander et al., 2013; Watt and Chamberlain, 2011). Access to hospitals and family planning centers were also associated with climate change (Peters et al., 2008; Chen et al., 2020; Wang et al., 2021; Wu et al., 2022).
Nations throughout the globe face several problems in achieving good human health and accessibility to health-related indicators in general, particularly in developing countries. Lack of access to health facilities tends researchers to construct multidimensional health poverty (MHP) index to measure the extent of accessibility to health across various countries. MHP index is predicated on the most popular Alkire-Foster theoretical framework of multidimensional poverty index (MPI), which had been constructed to measure the extent of poverty in the three dimensions of health, education, and living standard (Alkire and Foster, 2011a; Alkire and Foster, 2011b; Alkire and Roche, 2013; Alkire and Santos, 2013). However, with the emergence of innovations, the health dimension has been considered a broad subject matter as it plays a pivotal role in economic development and the human wellbeing of any country. As a result, many researchers have constructed a separate MHP index (Simões et al., 2016; Weziak-Bialowolska, 2016; Clarke and Erreygers, 2020; Chi et al., 2021; Mustafa et al., 2021). Many nations have reduced health poverty in the last 3 decades, but health problems persist. Population growth and global urbanization threaten 'people’s accessibility to health-related social indicators (Klasen and Lawson, 2007; Leon, 2008; Das Gupta et al., 2011; Liddle, 2017). For example, approximately 400 million people do not have access to basic health facilities (Balakrishnan et al., 2019), and more than 15 million people with HIV have no access to medical treatment (UNDP, 2015b; WHO, 2020). Moreover, approximately 7 million people die each year due to air pollution (WHO, 2018), nearly 844 million people have no access to safe drinking water (UNDP, 2015b), and approximately 2.3 billion people lack access to sanitation facilities (UNDP, 2015b). Also, more than 3 billion people, mostly from low- and middle-income countries, use solid fuels for cooking (WHO, 2018). Assessment of health poverty in this regard captured significance because it covers the two fundamental global SDGs, the end of poverty in all its form anywhere (SDG goal 1) and ensuring healthy lives and promote wellbeing for all at all ages (SDG goal 3) (UNDP, 2015b).
MHP is dynamic that varies across geographical boundaries within the countries. Considerable variations exist in the incidence of health poverty at geographical levels (Iqbal and Nawaz, 2017; Jewczak and Korczak, 2020; Khan and Hussain, 2020; Mustafa et al., 2021; Chen et al., 2022). Inequalities in health poverty exist across various regions within the countries. In the presence of such severe inequalities, the United Nation’s SDGs Agenda of 2030 universally agreed on a need to diminish these drastic inequalities, i.e., reduce inequality within and among countries (SDG goal 10) (UNDP, 2015a; UNDP, 2015b). Thus, it is necessary to identify the disparities in MHP from spatial perspectives. The mounting acceptance of spatial analysis has played a crucial role in almost every field of research, particularly in the social sciences. The spatial analysis identifies atypical clusters and hotspots (outliers) concerning MHP, thus appearing as a new hot topic. Several studies have explored the spatial perspectives of health poverty, but they are rare in low- and middle-income countries (Weeks et al., 2012; Sharma, 2014; Jewczak and Korczak, 2020; Dong et al., 2021; Morrow et al., 2022). Most studies mainly focused on the impact of various health and social determinants of health in non-spatial perspectives. Thus, it is desirable to recognize the spatial patterns of health poverty at the regional level. On one side, health poverty is dynamic and changes across countries. On the other side, climate change is diverse and also varies across regions. As noted above, both the impact and association of climate change and health-related attributes vary across geographical locations; there is an urgent need to look at the spatial connection of climatic variability with multidimensional health poverty at the geographical boundaries of any country.
Pakistan is a developing economy, with more than half of the population living in rural areas. The country is divided into four provinces and Federally Administered Tribal Areas (FATA). These provinces and FATA regions are further administered into divisions and sub-divisions (districts). Pakistan has a very diverse climate and is as varied as its topography. Mainly, there are four different types of weather, i.e., 1) dry winter (from December to February), 2) a hot, dry spring that starts in March and ends in May, 3) the summer rainy season, also called the southwest monsoon season which occurs from June to September, and 4) retreating monsoon lasts from October to November (Climatology, 2020). There are considerable differences in the mean, minimum, and maximum temperature, precipitation, rainfall, and wind pressure across various geographies. For instance, the northern areas of Pakistan are blessed with hilly and/or cold areas such as Murree, Swat, Naran, Malam Jabba, Kalam, Shogran, Neelum Valley, Ayubia, Chitral, Quetta, Kalat. The mean, minimum, and maximum temperatures are low, and the rainfall is high in such areas. The Kharan, Thar, Cholistan, Sibbi, Hyderabad, Jacobabad, Thal, etc., are hot and dry areas of Pakistan. Similarly, the rate of humidity/precipitation and wind pressure is also different in different climatic regions of Pakistan.
The past literature suggested a strong connection between climate change and health outcomes. Several climatic and environmental attributes, such as increasing temperature, growing carbon dioxide (CO2) emissions, and air pollution, have significantly influenced health and health-related outcomes. Previous literature mainly investigated the relationship between health poverty and climate change from non-spatial perspectives. Very few efforts have been made in spatial terminologies towards these associations, particularly in developing countries. However, the association between climate change and health outcomes must be investigated further from spatial perspectives. Therefore, considering the importance of the United Nations SDGs (Goals 3, 10, and 13), this study investigates the spatial nexus between climate change and health poverty from different angles. First, it recognizes the spatial patterns of health poverty and climatic variability across districts of Pakistan. Second, it identifies the spatial clusters of hotspots/cold spots and spatial outliers both in climatic variability and health poverty. Finally, it examines the univariate and bivariate spatial associations between climatic variability and health poverty across study districts of Pakistan. This study contributes to the existing literature in two ways. First, according to our knowledge, this is the first attempt to recognize the spatial nexus between climatic variability and health poverty in Pakistan. Second, this study applies spatial econometric models instead of conventional ones to gain more robust results. This study is useful for the government in identifying locations with a strong (or weak) spatial connection between health poverty and climate change. Policy planners and government can also use these results to prioritize districts with severe health poverty and bad climate associations.
2 Literature review
A literature review is considered the backbone of any research study. This section comprehends detailed conversations on health outcomes and climate change. Human health is a broad sector, and numerous researchers are working from different perspectives and dimensions. Similarly, in many previous studies, climate change has been measured differently. Few studies demonstrate the link between health outcomes and climate variables.
Sarmiento (2023) recently analyzed the relationship between temperature, mortality, and access to healthcare in Colombia. He showed that an increase in the days of hot or cold temperatures increases mortality. Higher temperatures above the mean of 27°C boosted the mortality rates by about 0.24 deaths per 100,000 lives. Further, he also confirmed that access to healthcare services mediated between temperature and mortality. A similar study was conducted by Mullins and White (2020) in US counties in the 1960s and 1970s, in which they tested whether access to healthcare mitigates the temperature effects on mortality. Results of the study revealed that improved access to primary care services provided by community health centers moderated the association between temperature and mortality by about 14%. Besides, the findings suggested that improving access to primary care may be useful for reducing the relationship between mortality and temperature. In another study, Mullins and White (2019) examined the link between ambient temperature and mental health outcomes at the county level in the United States. Their results concluded that higher temperatures increase mental illnesses and self-rated poor mental health. The study’s findings also confirmed that cold temperatures reduce the inverse mental health outcomes.
Moreover, they concluded that the impact of temperature on mental health differs from physical health and psychological and behavioral outcomes. Grace et al. (2015) investigated the association between birth weight, precipitation, and temperature in 19 African countries. Birth weights were recorded from the Demographic and Health Survey (DHS) from 1986 to 2010, and the monthly data on precipitation and temperature was derived from satellite and ground-based weather stations. Unlike the findings of Mullins and White (2019), their empirical findings suggested that precipitation and temperature did not affect birth weight. Nawaz (2021) examined the effects of multidimensional energy poverty and climate shocks on health poverty in Pakistan. Outcomes of the empirical analysis confirmed that climate change, including temperature, flood, and rainfall, has substantially and positively influenced multidimensional health poverty. In addition, the temperature has an adverse impact on child health.
Paavola (2017) examined how social and health disparities outline the health impacts of climate change in the United Kingdom. Exposure to heat, air pollution, pollen, and floods were considered to influence health outcomes. He found that the aging population and decreasing public expenditure on social and healthcare may aggravate disparities in health outcomes linked to climate change. Epstein et al. (2023) stated that precipitation anomalies (in the form of rainfall and drought) are related to poor health outcomes. In this regard, they examined the influence of precipitation on short-term health outcomes by using demographic and health survey data from 23 sub-Saharan African countries. The empirical results concluded that precipitation anomalies were correlated with short-term mobility among women, which in turn was associated with poor health outcomes. Goldie et al. (2015) explored the relationship between humidity, temperature, and health system in the context of climate variation in Darwin, Australia. The findings of the study indicated that humidity has significantly determined hospital morbidity. They suggested that in tropical regions of Australia, there is a need for heat-health policies to accommodate the influence of humidity at varied times of the day. Gao et al. (2014) conducted a review study to understand the impact of ambient humidity on child health. They selected 37 most relevant studies out of 2,797 articles from various electronic databases, including PubMed, Web of Science, ScienceDirect, and EBSCO. They found that 78% of the studies reported that ambient humidity played a significant role in children’s prevalence and incidence of climate-related infectious diseases. Rylander et al. (2013) identified that climate change influenced human health differently, such as lack of food and safe drinking water, poor sanitation, and morbidity. Their results showed that climate change in precipitation, rainfall, and droughts will increase the risk of maternal and infant mortalities.
Bi et al. (2022) examined the relationship between wind speed in the context of air temperature and its severity on human health in the form of mortality in Shanghai, China. The study outcomes verified V-shaped associations between daily air temperature and cause-specific mortality. In addition, older ages were more sensitive to variations in air temperature. The authors suggested that air temperature is a substantial determinant of human mortality, especially for elders. Almendra et al. (2019) assessed the short-term impacts of air temperature on hospitalization for mental disorders in Portugal. The findings showed that hospital admissions significantly increased with higher air temperature. Compared to men, women were more vulnerable to changes in air temperature. However, they found no significant difference between different age groups concerning the impact of air temperature. Péres et al. (2020) measured the relationship between air temperature and health outcomes in terms of mortality in two Brazilian regions by performing a quasi-Poisson non-linear lag distributed model. The study results indicated that air temperature increasingly influenced human health, which enhanced the general and specific mortalities. They also noted the varied impact of air temperature on mortality in both regions. Other studies, including Gilmont et al. (2018); Jones (2019); Kinney (2018); Kjellstrom et al. (2016); Li et al. (2022); Patrick et al. (2019); Sangkhaphan and Shu (2019) and Sheridan and Allen (2015) have discussed the association between climate variability and health outcomes in their perspectives mainly depend on their study objectives.
Fonseca-Rodriguez et al. (2019) assessed the spatial relationship between oppressive weather types and health outcome in the context of total mortality in Sweden. Poisson regression with distributed lags model was used for time series dataset from 1991 to 2014. The study results showed that during summer, in the south, the moist tropical and dry tropical weather significantly increased the total mortality at longer lags and, in the north, dry tropical weather increased the mortality at shorter lags. During winter, in the south, dry polar and moist polar weather respectively raised mortality from lag 6 to lag 10 and from lag 19 to lag 26. In the north, no significnat effect of oppressive wather was noted. Their findings concluded varied effects of oppressive weather across regions and seasons. Huang et al. (2022) investigated the health disparities from effect of built environment on temperature-related mortality in the local geographic units in Hong Kong, China. Using Bayesian spatial models at local area level, the effects of local temperature on mortality were analyzed. The findings of the study showed that variations in local temperature did not significantly contribute towards mortality. However, green space density substantially influenced the non-accidentally and cardiovascular diseases mortalities. They also found that the spatial disparities in mortality within Hong Kong could be explained by geographical distribution of green space rathre than temperature. Their study results suggested further investigation of spatial impact of temperature on health outcome at small area boundaries. Wibawa et al. (2023) examined the impact of ambient temperature, relative humidity and preciptiation on incidence of diarrhea in five regions of Surabaya, Indonesia. Their study results showed higher incidnece rate of diarrhea in rainy season in east Surabaya. Weather condition was significantly varied across the study regions. Diarrhea risk was substantially associated with extremely high and low temperature in the eastern area. They also resulted that the extremely low humidity boosted the incidence of diarrhea with highest risk in western region. Moreover, they suggested local government an health sectors to construct weather-based early warning system to mitigate the rising risks of infectious diseases. Liu et al. (2016) conducted spatial-temporal analysis of climate change, air pollution and mortality in 120 counties of China by applying univariate and partial correlation analysis. The results showed a significant spatial clustering of Air Pollution Index (API) with highest API in northwest China and east China respectively in year 2012 and year 2013. API was inversely associated with heat index, precipitation and sunshine hours but directly correlated with air pressure. As compared to the areas with lower API concentrations, areas with high API had higher mortality rates. Their findings concluded that air pollution varied by across regions and substantially associated with mortality across the country.
In a nutshell, the above literature mainly focussed on the link between climate change and health outcomes. These studies measured climate change mainly by temperature, humidity, precipitation, rainfall, air temperature, wind position, floods and drought. Similarly, health outcomes have been measured from different angles, such as mental health, child health, maternal health, access to health infrastructure, hospitalization, and mortalities. However, all of these studies mainly assessed the association from non-spatial perspectives. Therefore, considering the climate diversity, this study tries to fill the gap between health poverty and climatic variability in spatial perspectives across districts of Pakistan.
3 Theoretical framework of multidimensional health poverty
Multidimensional health poverty is not predicated on a new method but rather built on a pre-determined methodology of the Multidimensional Poverty Index (MPI). MPI framework was introduced by Sabrina Alkire and James Foster in 2011 by criticizing unidimensional income poverty (Alkire and Foster, 2011a). Traditionally, poverty was considered a unidimensional monetary phenomenon. Nations with higher income per capita were considered to be better off economically and vice versa. Later, it was found that many countries with a higher per capita income have a lower quality of life.
In contrast, many nations with lower per capita income have enjoyed a quality standard of living, such as higher life expectancy, good education, and higher access to healthcare infrastructure. With the emergence of time, the traditional monetary approach has been strictly criticized, as Amartya Sen in 1994 pointed out that poverty is a multidimensional concept, and no single indicator is solely capturing its universal characteristics (Sen, 1994). By making Sen’s statement of multidimensionality as a foundation, Sabrina Alkire and James Foster constructed a methodology to measure poverty in this perspective (Alkire and Foster, 2011b). First, this methodology was constructed for three dimensions (each with one or more than one sub-dimensions) of health, education, and living standards. There are several advantages of this framework. First, one can add as many variables as the dataset has. Second, the most important characteristic is that one can decompose the index concerning dimensions and regions. These key characteristics allowed researchers throughout the globe to construct the MPI by including different variables which mainly depended on the dataset.
In the modern era, as innovations emerge, many researchers are of the view that MPI has narrowly explained the previously selected three domains of the composite index, and there is a need to explore these dimensions in depth. For instance, the health and energy sectors are the two important United Nations SDGs and play a pivotal role in the economic growth of all nations. There is a need to investigate separately the people’s access to healthcare services as well as to energy sources. Resultantly, researchers have constructed separate indices for health and energy poverty. Different nations have constructed these indices by including a varied number of indicators mainly depending on the data availability (Simões et al., 2016; Weziak-Bialowolska, 2016; Pelz et al., 2018; Clarke and Erreygers, 2020; Lin and Okyere, 2020; Murias et al., 2020; Sokołowski et al., 2020; Chi et al., 2021; Karpinska et al., 2021; Mustafa et al., 2021; Qurat-ul-Ann and Mirza, 2021; Awan and Bilgili, 2022). Based on the SDGs’ importance to health and wellbeing, this study has implemented the Alkire-Foster methodology to construct multidimensional health poverty. This composite index is composed of the health and health-related social indicators (mainly depending upon the data availability) to gauge the severity of districts’ health poverty. The higher the index value in a specific district is, the more the district is health deprived in terms of healthcare services and vice versa.
As this study uses the framework of multidimensional poverty index, it is necessary to initiatively define the traditional Alkire-Foster theoretical concept of MPI. According to the Alkire-Foster approach MPI can be defined as: when an individual (or a household) is deprived in one health services dimension, the individual/household is identified as health poor in at least one dimension. Further, if the individual/household is deprived in two or more than two dimensions of health or health-related services, the individual/household is considered as multidimensionally health poor. This phenomenon can be easily understood by an example described in Table 1 and Table 2.
Table 1 shows the health deprivation matrix of ten individuals/households for six dimensions. Each row represents ith person or household and each column represents jth indicator or dimension. The two elements (0 and 1) of the health deprivation matrix are explained as follow: if a person/household has access to a specific health indicator, the score is assigned as 0 (non-deprived), whereas if an individual/household has no access to that health facility, the score is assigned as 1which means the household is deprived in that indicator. Multidimensional health poverty is sensitive to the size of the deprivation cut-off (k) (Khan and Sloboda, 2022). Table 2 shows the identification of MHP at different k values. As the deprivation cut-off (k) increases the extent of multidimensional health poverty decreases. For instance, when k = 1, almost all individuals/households are considered to be multidimensionally deprived; whereas when k = 6, only 8th individual/household is multidimensionally deprived.
The extensive literature showed a significant association between health services and worldwide climate variability (temperature, rainfall, flood, drought, humidity, precipitation, and air temperature). Pakistan also has a diverse climate structure, which may or may not has a connection to health poverty. Keeping in view the importance of SDGs and previous literature, this study proposed the following hypotheses to be tested:
Hypothesis 1. There is a significant spatial agglomeration among the districts of Pakistan concerning the MHP score.
Hypothesis 2. The contiguous districts of Pakistan are significantly spatially correlated with one another regarding climate variability.
Hypothesis 3. There is a significant spatial bivariate relationship between the MHP score and climate variability at the district level.
4 Methodology
4.1 Study area
This study explores the spatial association of MHP and climatic variability across districts from four provinces and districts of the FATA region of Pakistan. There may be significant spatial differences in the extent of MHP across districts because capital districts from all provinces are relatively well-off concerning access to health and health-related social dimensions. Thus, information on health-related indicators was gathered from four provinces and FATA regions. However, Gilgit Baltistan, Azad Jammu, Kashmir, and some of the districts from four provinces were excluded. These districts were either restricted due to the law-and-order situations or sufficient information on the study variables was unavailable. The rest of the districts were considered the study areas, shown in Figure 1.
4.2 Data sources
This study used two types of data sources: one for health-related social indicators to construct the MHP index and the other for climatic variability across various geographies. Health-related indicators were extracted from the most recent Pakistan Social and Living Standard Measurement (PALM) survey, Round VII, 2019–20, conducted by the Pakistan Bureau of Statistics, Islamabad (Pakistan Bureau of Statistics,G.O.P, 2020). Though the survey was not conducted to identify the spatial clusters of health poverty, sufficient information on health-related social dimensions fulfilled our study objectives. The survey was conducted at the district level, and information on various sociodemographics, health, housing, water and sanitation, and environment were collected from households. Information on climatic variability was extracted from the most popular and well-known Online National Aeronautics and Space Administration (NASA) website established in the United States on 29 July 1958 (NASA). NASA has online information on climate-related attributes such as solar fluxes, solar cooking, mean, minimum, and maximum temperatures, earth skin temperature, humidity, precipitation, wind pressure, wind speed, and wind direction at different distances and dimensions.
4.3 Variables of the study
Before explaining the methodology of MHP in detail, choosing appropriate indicators to measure the MHP is of supreme importance. Therefore, based on the previous literature, our study has chosen seven dimensions (and 15 of their sub-dimensions) (Iqbal and Nawaz, 2017; Chi et al., 2021; Mustafa et al., 2021; Khan and Sloboda, 2022). All the study dimensions are weighted equally. A detailed picture of these dimensions (and their sub-dimensions), their deprivation cut-offs, and assigned weights are given in Table 3. Similarly, based on the data availability, six climate-related variables have been selected for this study. The first three variables were the maximum, minimum, and mean temperature of districts, all measured in degree centigrade (ºC). The humidity rate of the district was measured in grams of vapor per kilogram (g/kg), precipitation of the district was scaled in millimeters per day (mm/dd), and wind speed in the district was measured in meters per second (m/s). The selected climate-related variables influencing health poverty are described in detail in Table 4. Ten years of data (from 2011 to 2021) on these indicators were extracted, and then used their averages as the study observations.
4.4 Multidimensional health poverty
The MHP index was formulated for the districts of Pakistan through the widely used Alkire-Foster methodology (Alkire and Foster, 2011a; Alkire and Foster, 2011b; Alkire and Santos, 2013). This approach is comprised of two steps; the Identification step and the Aggregation step.
The identification step (also known as the dual cut-off) is further divided into two thresholds. The first threshold was used to choose the deprivation cut-off of indicators and assign a weight to each indicator. A household i is called to be deprived for indicator xi, if its attainment in that indicator is beneath the cut-off, where the cut-off is represented by zi, i.e., if xi < zi. Following, all study dimensions were equally weighted, i.e., (
The second threshold was used to choose the poverty cut-off to identify the poor from the non-poor. From poor to non-poor identification, a deprivation score was assigned to households according to their deprivations in component indicators. The deprivation score was designed by taking the weighted sum of household deprivations. Statistically,
where ci denotes the deprivation score of the ith household, wi is the weight assigned to ith variable, and “indi” is the ith indicator. If a household is deprived in ith indicator, i.e., if xi < zi the indi = 1, otherwise indi = 0. Next, a cut-off score was determined to identify if a household is multidimensionally health poor. The ith household is considered multidimensionally health poor if its deprivation score is higher than or equal to the poverty cut-off k, i.e., if ci ≥ k and vice versa. According to the A-F censoring approach, the ci of multidimensionally non-poor households were exchanged as 0, even if their ci is non-zero. By applying the censored deprivation score ci(k) technique, if ci ≥ k, then ci(k) = ci, and “0” otherwise.
In the second (Aggregation) step, two elements were computed. The first one was the incidence of households facing multiple deprivations denoted by
This study’s analysis unit is the district, but the MHP index has been constructed at the household level. Therefore, the MHP scores of all households were aggregated at the district level. The mean is used to recognize the spatial association of MHP and climate change at the district level. The statistical notation of mean is written as under,
Where n is the number of households i = 1, 2, 3, … , n and
4.5 Spatial analysis
Global and local univariate Moran’s I test statistics were applied to test whether MPH and climatic variability have spatial clusters and/or spatial outliers. The global univariate Moran’s I examined if a spatial association exist between the MPH and/or climate variable(s) of a specific district and the MPH and/or climate variable(s) of the surrounding/neighboring districts. Statistically, the global univariate Moran’s I is written in Eq. 3 as under,
where n is the number of districts; xi and xj are the MPH or climate indicator scores of ith and jth districts, respectively;
The local univariate Moran’s I statistic identified the districts’ significant spatial clusters and/or spatial outliers concerning MPH or climate change variables. Econometrically, the local univariate Moran’s I is written in Eq. 4 as under,
where n is the number of districts; xi and xj are the MPH or climate indicator scores of ith and jth districts, respectively;
The statistical concept of bivariate global and local Moran’s I is similar to the univariate ones. Although, in univariate analysis MPH or climate indicator(s) score of a specific district was tested with its MPH or climate indicator(s) values in the neighboring districts, while in bivariate analysis MPH scores of a district were tested with another (say climate-related) variable(s) scores in the surrounding districts. Some studies (Anselin et al., 2002; Anselin et al., 2010) proposed bivariate global and local Moran’s I tests, given in Eqs 5, 6, respectively,
where n is the number of districts; xi and yj are the MPH and climate indicator scores of ith and jth districts, respectively;
5 Results
5.1 Spatial distribution of multidimensional health poverty
Figure 2 displays the spatial distribution of MHP scores of all districts on the map. The map clearly showed varied patterns of MHP across all districts of Pakistan. MHP was higher in districts of Baluchistan, FATA, and some of the districts from Khyber Pakhtunkhwa (KP). These districts are mostly located in the country’s west-southern, western, and northern areas. The highest health poverty-stricken districts were found in Baluchistan and KP, which range from 25.68% to 51.73%. Residents of these districts were found to be health poor, which means that people in these areas have relatively less access to health and health-related social indicators.
In contrast, MHP was lower in the districts in the country’s eastern, eastern-south, central, and southern parts. These districts are mainly located in Punjab and Sindh. The lowest health poverty-stricken districts were found mainly in Punjab, which ranges from 0.15% to 1.13%. Inhabitants of these districts have relatively higher access to health and health-related social determinants.
5.2 Spatial representation of climate variables
Figure 3 displays the spatial distribution of climate variables of the study. Figures 3A–C displayed all districts’ mean, maximum, and minimum temperatures. All three panels of the figure showed almost similar patterns though slight variations were found, especially in Sindh. However, within provinces, there is a clear picture of disparities in the mean, maximum and minimum temperatures across districts. Mean, maximum and minimum temperatures were recorded to be higher in Punjab and Sindh districts, mainly located in the country’s eastern, south-eastern, and southern parts. The highest mean, maximum, and minimum temperatures ranged from 28.29°C to 29.06°C, 49.60°C to 50.77°C and 6.90°C to 10.99°C in regions of Sindh and Punjab. On the other hand, districts from Baluchistan, KP, and FATA have comparatively lower mean, maximum and minimum temperatures. These districts are mainly located in the country’s northern, west-northern, and western parts. The ranges of lowest mean, maximum, and minimum temperatures were calculated as −0.57°C to 10.63°C, 23.53°C to 32.17°C and −27.90°C to −18.32°C respectively.
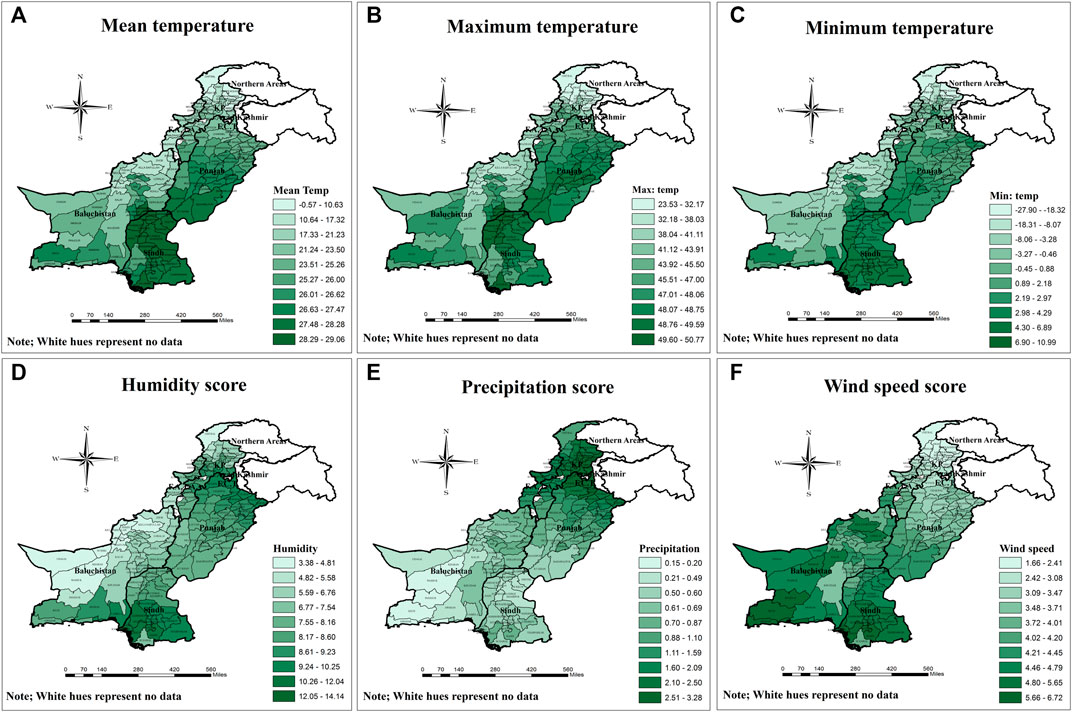
FIGURE 3. Spatial distribution of (A) Mean temperature (B) Maximum Temperature (C) Minimum temperature (D) Humidity score (E) Precipitation scores and (F) Wind speed score across districts of Pakistan.
The humidity rate of all the districts is shown in Figure 3D. There are considerable differences in the rates of humidity in all provinces. Districts from Punjab and Sindh made a spatial pattern of higher humidity compared to Baluchistan, KP, and FATA districts. Some of the districts from Baluchistan also have relatively higher humidity. Almost all regions from Baluchistan, FATA, and KP showed a spatial cluster of lower humidity rates. The highest humidity range (12.5 k/kg to 14.14 k/kg) was seen in Sindh province, whereas the lowest humidity range (3.38 k/kg to 4.81 k/kg) was found in the regions of Baluchistan. The precipitation rate displayed a similar pattern to the humidity rate, though considerable disparities were noted in the districts of KP and Sindh provinces (Figure 3E). Humidity was found to be lower in KP and higher in Sindh, whereas precipitation was the opposite. The precipitation rate was found to be higher in the districts of Punjab, KP, and FATA.
In contrast, districts from Sindh and Baluchistan showed a spatial pattern of lower precipitation rates. The higher precipitation (2.51 mm/day to 3.28 mm/day) and lower precipitation (0.15 mm/day to 0.20 mm/day) ranges were noticed in KP and Baluchistan, respectively. Finally, Figure 3F displays the wind speed across all districts of Pakistan. Like other climate variables, wind speed presented evident variations across the districts. The northern and eastern areas of the country presented a clear cluster of districts with lower wind speeds. Some of the districts from Baluchistan also have lower speed rates. In contrast, almost all districts from Sindh and Baluchistan made a spatial pattern of districts with higher wind speeds. The lowest wind speed range (1.66 m/s to 2.41 m/s) was found in the northern regions of Pakistan, whereas the highest range (5.66 m/s to 6.72 m/s) of wind speed was noticed in the areas of the southern part of Pakistan.
5.3 The univariate spatial relationship of MHP and climate indicators
Figures 4A,B, respectively, show the results of global and local Moran’s scores of MHP in Pakistan. The Moran’s I statistic score indicated that concerning MHP, the contiguous districts were significantly spatially associated by 36% with a significance level of Pseudo p-value of 0.001 (Table 5). The scatterplot of Moran’s I score of MHP is shown in Figure 4A. The univariate LISA analysis classified the spatial clusters into four quadrants (Figure 4B). A spatial cluster of H-H districts was found in the western part of the country, located in Baluchistan, which means that these districts and their surrounding districts are highly health-poverty-stricken areas. A significant spatial cluster of L-L districts was found in Punjab. In other words, districts in this cluster are relatively better off regarding health poverty. Furthermore, a spatial pattern of L-H districts was found in Baluchistan, where neighboring districts with higher health poverty scores surround the district with a lower MHP score.
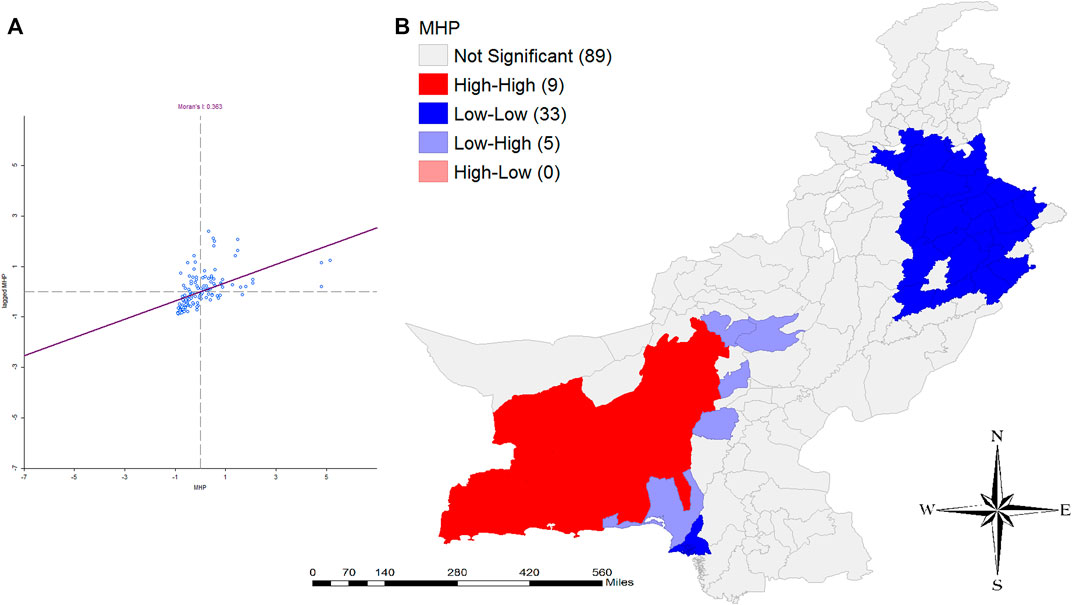
FIGURE 4. Author’s own calculations based on the Pakistan Social and Living Standard Measurement (PSLM) survey (Pakistan Bureau of Statistics,G.O.P, 2020). Spatial analysis of (A) Global Moran’s I of multidimensional health poverty and (B) Local Moran’s I of multidimensional health poverty across districts of Pakistan.
Univariate Moran’s I test scores of climate variables are shown in Table 5. The scatterplots of these indicators are provided in Appendix A, Figure A1. Table 5 indicates a solid spatial association between a specific district and its neighboring districts. All variables have a higher (than 75%) Moran’s I scores. The highest univariate spatial association was recorded for precipitation rate, and the lowest spatial relationship was calculated for minimum temperature. Figure 5 displays the univariate local Moran’s clusters of climate variables. It is evident from the figure that a spatial cluster of L-L districts concerning mean, minimum, and maximum temperatures was found mainly in KP and Baluchistan (Figures 5A–C).
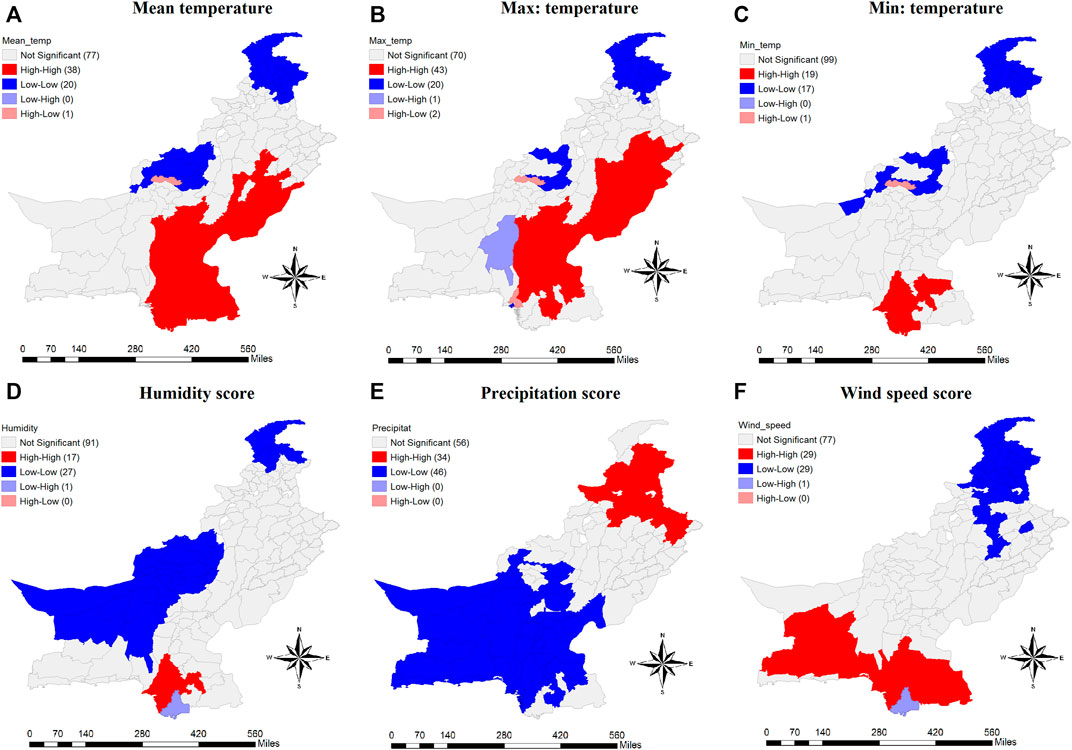
FIGURE 5. Spatial representation of univariate local Moran’I of (A) Mean temperature (B) Maximum temperature (C) Minimum temperature (D) Humidity score (E) Precipitation score and (F) Wind speed score across study districts of Pakistan.
In contrast, an H-H spatial pattern of districts for mean and maximum temperature was found in Sindh and Punjab (Figures 5A,B), whereas, for minimum temperature, it is found only in Sindh (Figure 5C). The clusters of H-L and L-H were very small, showing fewer districts where the mean, maximum and minimum temperatures are high (low) but low (high) neighbors surround them. Unlike the temperatures, humidity indicated different shapes of clusters (Figure 5D). Almost all districts from Baluchistan (and some from KP) province were spatially clustered in L-L quadrants. A spatial pattern of H-H districts was found in the province of Sindh. The precipitation rate is highly clustered across the districts of Pakistan (Figure 5E). Almost all the districts from Sindh and Baluchistan presented a spatial cluster of L-L districts. A higher precipitation rate is found in northern areas of Pakistan, significantly surrounded by districts with higher precipitation. Finally, the spatial clusters of wind speed are presented in Figure 5F. H-H spatial clustered districts were located in Sindh and Baluchistan, whereas L-L clustered districts were mainly found in KP and Punjab. Only one district in the south was clustered as L-H.
5.4 Bivariate spatial association between health poverty and climate change
Table 5 shows the bivariate global Moran’s I result of health poverty and climate attributes. MHP has a negative significant spatial association with all the climate variables of the study except wind speed which is insignificant. The highest inverse spatial association (38%) was found between the MHP score and humidity, whereas the lowest negative relationship (10%) was recorded between MHP and maximum temperature. The bivariate Moran scatterplots of multidimensional health poverty with the climate indicators are provided in Appendix A, Figure A2.
Figure 6 shows the bivariate local Moran’s I result of MHP scores and climate variables. Districts with L-L spatial clusters in the north and central part of Pakistan indicated that MHP is lower in these districts, which are surrounded by lower mean temperature districts (Figure 6A). The H-H clustered districts, mainly from Sindh, showed higher health poverty surrounded by neighbors with higher mean temperatures. A strong L-H spatial outlier was found in Punjab and Sindh provinces, which indicated that MHP is lower in these areas but is surrounded by the districts with higher mean temperatures. Also, a spatial collection of H-L districts in the north and central parts exhibited that the lower mean temperature districts surround higher health poverty. The spatial correlation of MHP and maximum temperature displayed in Figure 6B is almost similar to the behavior found in Figure 6A; however, it is different for MHP and minimum temperature presented in Figure 6C. A weak cluster of H-H districts was found in the southern part, where the higher minimum temperature of the neighbors encircled high MHP. L-H spatial cluster was also found near the H-H cluster. The H-L spatial outlier in the northern and central parts confirmed that higher MHP is encircled by the contiguous areas where the minimum temperature is low.
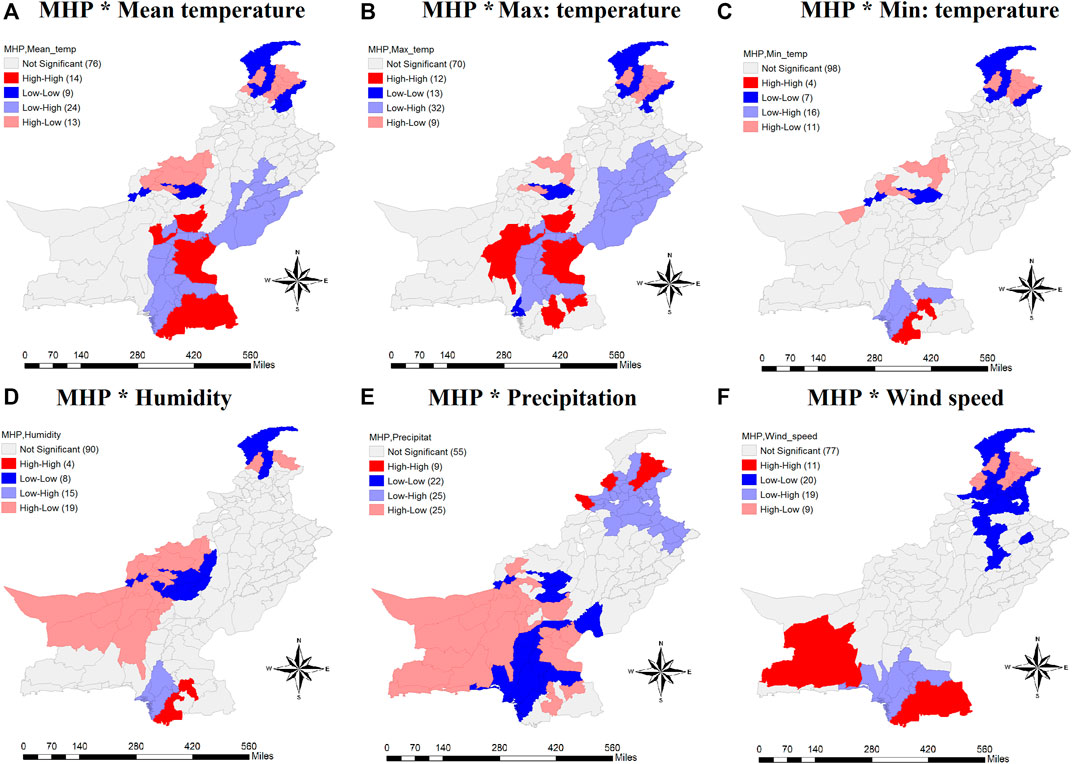
FIGURE 6. Spatial analysis of bivariate Moran’s I of (A) MHP*Mean temperature (B) MHP*Maximum temperature (C) MHP*Minimum temperature (D) MHP*Humidity rate (E) MHP*Precipitation rate and (F) MHP*Wind speed rate across all districts of Pakistan.
Figure 6D displayed the spatial bivariate local Moran’s of MHP and Humidity rate. Almost all districts from Baluchistan presented a strong spatial pattern of H-L districts, indicating that higher MHP is surrounded by the lower humidity rate in their neighboring districts. L-L clustered districts were mainly found in KP and Baluchistan. A spatial cluster of H-H regions was found in Sindh, where the higher MHP was covered with neighbors with high humidity rates. The spatial correlation between MHP and precipitation is similar to the association found in Figure 6D. However, considerable variations were found in the number of districts in each cluster except H-H (Figure 6E). A strong outlier of H-L districts in Baluchistan and Sindh exhibited that higher MHP was bounded by neighbors with lower precipitation rates. A cluster of L-L districts was also seen in these provinces, showing that lower precipitation neighbors encircle the lower health poverty. A strong spatial outlier of L-H areas was seen in the northern and east northern parts where lower MHP was covered, with the districts having higher precipitation rates. Few districts presented the cluster of H-H regions, mainly in KP and some in the FATA. Finally, a very different result of MHP and wind speed were noted in Figure 6F. H-H clusters were mainly found in Sindh and Baluchistan. The l-H outlier was also seen in Sindh, which indicated that lower MHP was shielded with districts with higher wind speeds. Districts from Punjab and KP presented a cluster of L-L districts. Also, a spatial outlier of H-L districts was seen in KP, which indicated higher health poverty walled by districts with lower wind speed.
6 Discussion
This study is the first examination of the spatial nexus between Pakistan’s MHP and climate indicators. Because this study addresses the fundamental question of whether the diverse climate across various geographies of Pakistan has spatial connectivity with the varied MHP. Another novelty is that this study identifies the regions where MHP is positively and/or negatively associated with climatic variability.
The results confirmed that people in Baluchistan and FATA have less accessibility to health and health-related indicators. In other words, inhabitants in these regions are multidimensionally health-deprived. On the other hand, residents in districts from Punjab are better off in terms of MHP scores. They have higher accessibility to health and healthcare services. Our results are consistent with the outcome of previous research (Khan and Hussain, 2020). The government of Pakistan has launched different health programs such as the “sehat sahulat program” and “family planning and primary healthcare” to improve the accessibility of all people to various healthcare services (GOP, 2015; NADRA, 2016). Despite such initiatives, significant differences exist across Pakistan districts which challenge the UNDP’s Sustainable Development Goals (SDGs). According to UNDP’s SDG 1, each nation must ensure healthy lives and promote wellbeing for all ages (UNDP, 2015a; UNDP, 2015b). Our findings suggest that Pakistan is far from the SDG that needs to be achieved. Not only the accessibility to various health-related social indicators is less, but there were disparities in such accessibility across different geographical regions. Districts from some geographical regions achieved higher access to healthcare infrastructure and got lower MHP values, whereas others still have a substantially high MHP score.
The climate in Pakistan is diverse. It changes across geographical locations. Our study results also presented varied patterns of various climatic attributes, including temperature, humidity, precipitation, and wind speed. It is confirmed that mean, maximum, and minimum temperatures are higher across the districts of Punjab and Sindh (mostly smooth areas or no hilly areas) whereas lower in most of the districts from Baluchistan, KP, and FATA, as these provinces have mostly hilly areas. Humidity and precipitation presented similar disparities across districts, whereas the wind speed provided different patterns. Districts across Baluchistan and Sindh collectively made a pattern of higher wind speed, whereas northern and northeastern districts depicted a pattern of lower wind speed.
A critical analysis component was identifying the spatial clustering in multidimensional health poverty and its connection to climate attributes. First, we conducted a univariate global and local spatial analysis to check whether significant spatial clusters and outliers exist in study indicators. According to the results, health poverty in a specific district was spatially positively associated with the MHP scores of its surrounding districts. The LISA results confirmed the location of the hot spots, cold spots, and spatial outliers. The H-H cluster in Baluchistan province showed that these districts are multidimensionally health deprived. Such findings were also found in previous studies in Pakistan (Iqbal and Nawaz, 2017; Mustafa et al., 2021). Also, the cluster of cold spots (L-L) districts in Punjab indicated that the residents of Punjab are relatively well-off in terms of MHP scores. Such spatial disparities are against one of the most important SDGs, i.e., Goal 10 of reducing inequality within and among countries (UNDP, 2015a; UNDP, 2015b).
Our findings exposed a strong spatial positive correlation between the contiguous neighbors concerning all climate-related variables. Mean, and maximum temperatures were highly clustered mainly in Sindh and Punjab, whereas minimum temperature was clustered as H-H only in Sindh. In contrast, all the temperature indicators in the northern and some central districts were clustered in L-L quadrants. The results concluded opposite outcomes for humidity and precipitation. Lower humidity and precipitation areas were found in Baluchistan and Sindh, which were surrounded by districts with lower humidity and precipitation rates. However, the case for the H-H cluster was different. Higher precipitation districts were seen in the northern and northeastern areas.
Interestingly there was no spatial outlier in terms of precipitation rate. The wind speed was also significantly spatially associated with its neighboring districts. It is confirmed that wind speed was higher in the areas of Sindh and Baluchistan whereas lower in the districts of KP and some of Punjab, which spatially clustered in L-L quadrants.
A key contribution of the study is to identify the spatial cross-correlation between multidimensional health poverty and various climate indicators. For this purpose, we conducted a bivariate global and local spatial analysis. Results of the study confirmed that all the climate-related indicators have a significant negative spatial association with MHP, except wind speed has a positive but insignificant spatial association with the MHP score. Results from earlier studies also supported our findings (Weck et al., 2008; Thompson et al., 2012; Bennett and Friel, 2014; Farooq et al., 2019; Majeed and Ozturk, 2020; Li et al., 2022). Our results suggested that the higher the temperatures, humidity, precipitation, and wind speed, the lower the MHP scores throughout Pakistan. However, separate bivariate LISA analysis of all climatic indicators with MHP provided insight into the spatial clusters and outliers.
The results show that higher MPH was clustered by neighboring areas with higher mean and maximum temperatures (Figures 6A,B). In contrast, the L-L cluster of MHP is surrounded by a lower mean and maximum temperature of the neighbors. Such districts were seen in the northern areas of Pakistan. In contrast, H-L and L-H outliers were strong because of the negative spatial association of MHP and temperature. Previous studies also supported our findings of the inverse association of health outcomes and temperature (Martiello and Giacchi, 2010; Wondmagegn et al., 2021). Most regions were clustered such that lower (higher) MHP scores were surrounded by higher (lower) mean or maximum temperature districts. The spatial clusters and outliers of MHP with minimum temperature were found to be very small. The majority of the districts were insignificant. Though, spatial outliers of lower (higher) MHP covered by the higher (lower) minimum temperature were seen in the southern and central parts of the country. Moreover, almost all districts from Baluchistan presented a spatial outlier of H-L districts which confirmed the inverse relationship between the higher MHP in specific districts surrounded by districts with lower humidity rates. Previous studies also supported the inverse association of humidity and health poverty in terms of health outcomes and health infrastructure (Barreca, 2012; Gao et al., 2014; Derby, 2017; Wolkoff, 2018). MHP with precipitation rate made a strong outlier of H-L districts, mainly in Baluchistan, which depicted a strong negative association between the two. Such inverse outcomes are consistent with previous studies (Abiona, 2017; Schramm et al., 2021). A higher MHP score lowers the precipitation rate in adjacent regions. Not only was the H-L outlier seen, but the L-H spatial outlier was also found in the country’s northern and northeastern areas, which confirmed that lower MHP is surrounded by neighboring districts with higher precipitation rates. Also, a good spatial cluster of H-H was seen in the southern regions where high MHP districts were covered by lower precipitation districts.
7 Limitations
This study has several limitations. First, there may be other health-related indicators of MHP. However, this study used only 15 health and health-related social indicators, as the available dataset did not provide sufficient information on other health-related variables. The same is true for the selection of climate-related attributes. Several other climate variables (such as CO2, air pollution, drought, and flood) also significantly determine the health services but are not included in the present study due to the unavailability of data on the NASA website. Second, the spatial analysis was performed at the district level because the shapefiles and coordinate information were unavailable at a more micro level, such as municipal or household. Third, this study only considered the districts from four provinces and the FATA region, as data on other districts were not collected due to law-and-order situations. Fourth, the spatial analysis was limited to geographical location and did not consider the time because the PSLM survey is cross-sectional.
8 Conclusion
Previous studies investigated the association between climate variability and health outcomes mostly from non-spatial perspectives. Also, health outcomes are mostly measured by a single indicator. This study assessed the relationship between climate variability and multidimensional health poverty in spatial terms by considering health poverty a multidimensional phenomenon. Therefore, we constructed a multidimensional health poverty (MHP) index to measure the overall health status of the districts. We used mean temperature, minimum temperature, maximum temperature, humidity, precipitation, and wind speed as indicators of climate variability. We applied the spatial univariate and bivariate global Moran’s I and Local Indicators of Spatial Association (LISA) tests to assess the significant spatial clusters and outliers of climate variability and MHP.
The instant results concluded that there is a considerable geographical disparity in terms of health poverty. Punjab was considered the well-off, whereas Baluchistan was considered the highly poverty-stricken province. Such a conclusion was also drawn based on the univariate spatial results. H-H cluster was found in Baluchistan, whereas L-L spatial cluster was seen in the districts of Punjab. Moreover, the study results concluded an inverse spatial association between MHP and temperatures. Bivariate spatial outliers of H-L and L-H confirmed the clusters of a significant negative relationship, especially in Baluchistan and KP. Most importantly, the results also concluded an inverse spatial association of MHP with humidity and precipitation rates. A strong spatial outlier (H-L) was seen in the districts of Baluchistan and Sindh. It is concluded that a lower humidity or precipitation rate, the higher the MHP scores.
The above results have some policy recommendations. Firstly, based on the conclusion from univariate global and local results, the government of Pakistan is recommended to set priorities to mitigate health poverty. More preferences must be given to the districts making an H-H cluster to reduce the disparities in access to health-related social indicators. Secondly, based on the significant bivariate spatial association between MHP and temperature, government and policymakers (in order to reduce health poverty) are needed to set priorities by giving more preferences to areas under H-L outliers. Thirdly, keeping the importance of bivariate relationship between MHP and precipitation and humidity rates in view, to mitigate the health poverty, policy planners are needed to adopt such steps that improve the people’s accessibility to health-related social indicators by setting priorities in Baluchistan and Sindh provinces, especially in the districts where the humidity and precipitation rates are low.
Data availability statement
Publicly available datasets were analyzed in this study. This data can be found here: https://www.pbs.gov.pk/publication/pakistan-social-and-living-standards-measurement-survey-pslm-2019-20-provincial and https://climate.nasa.gov/.
Author contributions
All authors listed have made a substantial, direct, and intellectual contribution to the work and approved it for publication.
Conflict of interest
The authors declare that the research was conducted in the absence of any commercial or financial relationships that could be construed as a potential conflict of interest.
Publisher’s note
All claims expressed in this article are solely those of the authors and do not necessarily represent those of their affiliated organizations, or those of the publisher, the editors and the reviewers. Any product that may be evaluated in this article, or claim that may be made by its manufacturer, is not guaranteed or endorsed by the publisher.
Supplementary material
The Supplementary Material for this article can be found online at: https://www.frontiersin.org/articles/10.3389/fenvs.2023.1180556/full#supplementary-material
References
Abban, O. J., Hongxing, Y., Nuta, A. C., Dankyi, A. B., Ofori, C., and Cobbinah, J. (2022). Renewable energy, economic growth, and CO2 emissions contained Co-movement in african oil-producing countries: A wavelet based analysis. Energy Strategy Rev. 44, 100977. doi:10.1016/j.esr.2022.100977
Abiona, O. (2017). Adverse effects of early life extreme precipitation shocks on short-term health and adulthood welfare outcomes. Rev. Dev. Econ. 21 (4), 1229–1254. doi:10.1111/rode.12310
Alkire, S., and Foster, J. (2011a). Counting and multidimensional poverty measurement. J. public Econ. 95 (7-8), 476–487. doi:10.1016/j.jpubeco.2010.11.006
Alkire, S., and Foster, J. (2011b). Understandings and misunderstandings of multidimensional poverty measurement. J. Econ. Inequal. 9 (2), 289–314. doi:10.1007/s10888-011-9181-4
Alkire, S., and Roche, J. M. (2013). How multidimensional poverty went down: Dynamics and comparisons. OXFORD POVERTY and HUMAN DEVELOPMENT INITIATIVE. Oxford, UK.
Alkire, S., and Santos, M. E. (2013). A multidimensional approach: Poverty measurement and beyond. Soc. Indic. Res. 112 (2), 239–257. doi:10.1007/s11205-013-0257-3
Almendra, R., Loureiro, A., Silva, G., Vasconcelos, J., and Santana, P. (2019). Short-term impacts of air temperature on hospitalizations for mental disorders in Lisbon. Sci. Total Environ. 647, 127–133. doi:10.1016/j.scitotenv.2018.07.337
Anselin, L., Syabri, I., and Smirnov, O. (2002). “Visualizing multivariate spatial correlation with dynamically linked windows,” in Paper presented at the proceedings, CSISS workshop on new tools for spatial data analysis (Santa Barbara, CA.
Anselin, L., Syabri, I., and Kho, Y. (2010). “GeoDa: An introduction to spatial data analysis,” in Handbook of applied spatial analysis (Springer), 73–89.
Anselin, L. (1995). Local indicators of spatial association—Lisa. Geogr. Anal. 27 (2), 93–115. doi:10.1111/j.1538-4632.1995.tb00338.x
Awan, A., Bilgili, F., and Rahut, D. B. (2022). Energy poverty trends and determinants in Pakistan: Empirical evidence from eight waves of HIES 1998–2019. Renew. Sustain. Energy Rev. 158, 112157. doi:10.1016/j.rser.2022.112157
Balakrishnan, K., Dey, S., Gupta, T., Dhaliwal, R., Brauer, M., Cohen, A. J., et al. (2019). The impact of air pollution on deaths, disease burden, and life expectancy across the states of India: The global burden of disease study 2017. Lancet Planet. Health 3 (1), e26–e39. doi:10.1016/s2542-5196(18)30261-4
Barreca, A. I. (2012). Climate change, humidity, and mortality in the United States. J. Environ. Econ. Manag. 63 (1), 19–34. doi:10.1016/j.jeem.2011.07.004
Barros, V. R., Field, C. B., Dokken, D. J., Mastrandrea, M. D., Mach, K. J., Bilir, T. E., et al. (2014). “Climate change 2014 impacts, adaptation, and vulnerability Part B: Regional aspects: Working group II contribution to the fifth assessment report of the intergovernmental panel on climate change,” in Climate change 2014: Impacts, adaptation and vulnerability: Part B: Regional aspects: Working group II contribution to the fifth assessment report of the intergovernmental panel on climate change (Cambridge University Press), 1–1820.
Bennett, C. M., and Friel, S. (2014). Impacts of climate change on inequities in child health. Children 1 (3), 461–473. doi:10.3390/children1030461
Bhandari, D., Bi, P., Sherchand, J. B., Dhimal, M., and Hanson-Easey, S. (2020). Assessing the effect of climate factors on childhood diarrhoea burden in Kathmandu, Nepal. Int. J. Hyg. Environ. Health 223 (1), 199–206. doi:10.1016/j.ijheh.2019.09.002
Bi, X., Wu, C., Wang, C., Wang, Y., Wang, X., Song, C., et al. (2022). Impacts of air temperature and its extremes on human mortality in Shanghai, China. Urban Clim. 41, 101072. doi:10.1016/j.uclim.2021.101072
Boyles, A. L., Beverly, B. E., Fenton, S. E., Jackson, C. L., Jukic, A. M. Z., Sutherland, V. L., et al. (2021). Environmental factors involved in maternal morbidity and mortality. J. Women's Health 30 (2), 245–252. doi:10.1089/jwh.2020.8855
Chen, T., Radke, J. D., Lang, W., and Li, X. (2020). Environment resilience and public health: Assessing healthcare's vulnerability to climate change in the San Francisco Bay Area. Growth Change 51 (2), 607–625. doi:10.1111/grow.12379
Chen, X., Zhou, T., and Wang, D. (2022). The impact of multidimensional health levels on rural poverty: Evidence from rural China. Int. J. Environ. Res. Public Health 19 (7), 4065. doi:10.3390/ijerph19074065
Cheung, C. W., He, G., and Pan, Y. (2020). Mitigating the air pollution effect? The remarkable decline in the pollution-mortality relationship in Hong Kong. J. Environ. Econ. Manag. 101, 102316. doi:10.1016/j.jeem.2020.102316
Chi, X.-X., Liu, X.-H., and Zhang, Z.-Z. (2021). Measuring multidimensional health poverty in China. Front. Public Health 9, 786325. doi:10.3389/fpubh.2021.786325
Clarke, P., and Erreygers, G. (2020). Defining and measuring health poverty. Soc. Sci. Med. 244, 112633. doi:10.1016/j.socscimed.2019.112633
Climatology (2020). Climate change knowledge portal for development practitioners and policy makers. Retrieved from: https://climateknowledgeportal.worldbank.org/country/pakistan/climate-data-historical#:∼:text=Pakistan%20lies%20in%20a%20temperate,the%20northern%20uplands%20and%20Himalayas.
Dankyi, A. B., Abban, O. J., Yusheng, K., and Coulibaly, T. P. (2022). Human capital, foreign direct investment, and economic growth: Evidence from ECOWAS in a decomposed income level panel. Environ. Challenges 9, 100602. doi:10.1016/j.envc.2022.100602
Das Gupta, M., Bongaarts, J., and Cleland, J. (2011). Population, poverty, and sustainable development: A review of the evidence. World Bank Policy Research Working Paper(5719).
Davenport, F., Grace, K., Funk, C., and Shukla, S. (2017). Child health outcomes in sub-saharan africa: A comparison of changes in climate and socio-economic factors. Glob. Environ. Change 46, 72–87. doi:10.1016/j.gloenvcha.2017.04.009
Dong, Y., Jin, G., Deng, X., and Wu, F. (2021). Multidimensional measurement of poverty and its spatio-temporal dynamics in China from the perspective of development geography. J. Geogr. Sci. 31 (1), 130–148. doi:10.1007/s11442-021-1836-x
Epstein, A., Harris, O. O., Benmarhnia, T., Camlin, C. S., and Weiser, S. D. (2023). Do precipitation anomalies influence short-term mobility in sub-saharan africa? An observational study from 23 countries. BMC Public Health 23 (1), 1–6. doi:10.1186/s12889-023-15264-z
Farooq, M. U., Shahzad, U., Sarwar, S., and ZaiJun, L. (2019). The impact of carbon emission and forest activities on health outcomes: Empirical evidence from China. Environ. Sci. Pollut. Res. 26 (13), 12894–12906. doi:10.1007/s11356-019-04779-x
Fonseca-Rodríguez, O., Lundevaller, E. H., Sheridan, S. C., and Schumann, B. (2019). Association between weather types based on the spatial synoptic classification and all-cause mortality in Sweden, 1991–2014. Int. J. Environ. Res. Public Health 16 (10), 1696. doi:10.3390/ijerph16101696
Gao, J., Sun, Y., Lu, Y., and Li, L. (2014). Impact of ambient humidity on child health: A systematic review. PloS One 9 (12), e112508. doi:10.1371/journal.pone.0112508
Gilmont, M., Hall, J. W., Grey, D., Dadson, S. J., Abele, S., and Simpson, M. (2018). Analysis of the relationship between rainfall and economic growth in Indian states. Glob. Environ. Change 49, 56–72. doi:10.1016/j.gloenvcha.2018.01.003
Giulia, S., Lea, B.-F., Carol, Z.-C., Lisa, M., Harper, S. L., and Elizabeth, C. J. (2020). The effect of climatic factors on nutrients in foods: Evidence from a systematic map. Environ. Res. Lett. 15 (11), 113002. doi:10.1088/1748-9326/abafd4
Goldie, J., Sherwood, S. C., Green, D., and Alexander, L. (2015). Temperature and humidity effects on hospital morbidity in Darwin, Australia. Ann. Glob. health 81 (3), 333–341. doi:10.1016/j.aogh.2015.07.003
GOP (2015). Sehat sahulat program. Retrieved from: https://www.pmhealthprogram.gov.pk/.
Grace, K., Davenport, F., Hanson, H., Funk, C., and Shukla, S. (2015). Linking climate change and health outcomes: Examining the relationship between temperature, precipitation and birth weight in Africa. Glob. Environ. Change 35, 125–137. doi:10.1016/j.gloenvcha.2015.06.010
Hansen, J., Johnson, D., Lacis, A., Lebedeff, S., Lee, P., Rind, D., et al. (1981). Climate impact of increasing atmospheric carbon dioxide. Science 213 (4511), 957–966. doi:10.1126/science.213.4511.957
Helldén, D., Andersson, C., Nilsson, M., Ebi, K. L., Friberg, P., and Alfvén, T. (2021). Climate change and child health: A scoping review and an expanded conceptual framework. Lancet Planet. Health 5 (3), e164–e175. doi:10.1016/s2542-5196(20)30274-6
Huang, Z., Chan, E. Y. Y., Wong, C. S., Liu, S., and Zee, B. C. Y. (2022). Health disparity resulting from the effect of built environment on temperature-related mortality in a subtropical urban setting. Int. J. Environ. Res. Public Health 19 (14), 8506. doi:10.3390/ijerph19148506
Iqbal, N., and Nawaz, S. (2017). Spatial differences and socioeconomic determinants of health poverty. Pak. Dev. Rev. 56 (3), 221–248. doi:10.30541/v56i3pp.221-248
Jewczak, M., and Korczak, K. (2020). Poverty and health state in Poland: Evidence from regional perspective. Probl. Zarzadzania 18 (3), 49–66. doi:10.7172/1644-9584.89.3
Jones, B. A. (2019). Tree shade, temperature, and human health: Evidence from invasive species-induced deforestation. Ecol. Econ. 156, 12–23. doi:10.1016/j.ecolecon.2018.09.006
Karpinska, L., Smiech, S., Gouveia, J. P., and Palma, P. (2021). Mapping regional vulnerability to energy poverty in Poland. Sustainability 13 (19), 10694. doi:10.3390/su131910694
Khan, F., and Akram, S. (2018). Sensitivity of multidimensional poverty index in Pakistan. Pak. J. Soc. Issues 9, 98–108.
Khan, S. U., and Hussain, I. (2020). Inequalities in health and health-related indicators: A spatial geographic analysis of Pakistan. BMC Public Health 20 (1), 1800–1809. doi:10.1186/s12889-020-09870-4
Khan, S. U., and Sloboda, B. W. (2022). Spatial analysis of multidimensional poverty in Pakistan: Do income and poverty score of neighboring regions matter? GeoJournal, 1–27. doi:10.1007/s10708-022-10781-7
Khan, A. E., Ireson, A., Kovats, S., Mojumder, S. K., Khusru, A., Rahman, A., et al. (2011). Drinking water salinity and maternal health in coastal Bangladesh: Implications of climate change. Environ. Health Perspect. 119 (9), 1328–1332. doi:10.1289/ehp.1002804
Khan, A. U., Saboor, A., Hussain, A., Sadiq, S., and Mohsin, A. Q. (2014). Investigating multidimensional poverty across the regions in the Sindh province of Pakistan. Soc. Indic. Res. 119 (2), 515–532. doi:10.1007/s11205-013-0511-8
Khan, A. U., Saboor, A., Hussain, A., Karim, S., and Hussain, S. (2015). Spatial and temporal investigation of multidimensional poverty in rural Pakistan. Poverty Public Policy 7 (2), 158–175. doi:10.1002/pop4.99
Khan, H., Weili, L., and Khan, I. (2023). The effect of political stability, carbon dioxide emission and economic growth on income inequality: Evidence from developing, high income and Belt Road initiative countries. Environ. Sci. Pollut. Res. 30 (3), 6758–6785. doi:10.1007/s11356-022-22675-9
Kinney, P. L. (2018). Interactions of climate change, air pollution, and human health. Curr. Environ. health Rep. 5 (1), 179–186. doi:10.1007/s40572-018-0188-x
Kjellstrom, T., Briggs, D., Freyberg, C., Lemke, B., Otto, M., and Hyatt, O. (2016). Heat, human performance, and occupational health: A key issue for the assessment of global climate change impacts. Annu. Rev. Public Health 37, 97–112. doi:10.1146/annurev-publhealth-032315-021740
Klasen, S., and Lawson, D. (2007). The impact of population growth on economic growth and poverty reduction in Uganda. Retrieved from.
Kovats, R., and Osborn, D. (2016). UK climate change risk assessment 2017: Evidence report. London: Chapter 5: People and the built environment.
Kuehn, L., and McCormick, S. (2017). Heat exposure and maternal health in the face of climate change. Int. J. Environ. Res. Public Health 14 (8), 853. doi:10.3390/ijerph14080853
Liu, L., Yang, X., Liu, H., Wang, M., Welles, S., M´rquez, S., Frank, A., and Haas, C. N. (2016). Spatial–temporal analysis of air pollution, climate change, and total mortality in 120 cities of China, 2012–2013. Frontiers in public health 4, 143
Li, Q., Sun, P., Li, B., and Mohiuddin, M. (2022). Impact of climate change on rural poverty vulnerability from an income source perspective: A study based on CHIPS2013 and county-level temperature data in China. Int. J. Environ. Res. Public Health 19 (6), 3328. doi:10.3390/ijerph19063328
Liddle, B. (2017). Urbanization and inequality/poverty. Urban Sci. 1 (4), 35. doi:10.3390/urbansci1040035
Lin, B., and Okyere, M. A. (2020). Multidimensional energy poverty and mental health: Micro-level evidence from Ghana. Int. J. Environ. Res. Public Health 17 (18), 6726. doi:10.3390/ijerph17186726
Majeed, M. T., and Ozturk, I. (2020). Environmental degradation and population health outcomes: A global panel data analysis. Environ. Sci. Pollut. Res. 27 (13), 15901–15911. doi:10.1007/s11356-020-08167-8
Martiello, M. A., and Giacchi, M. V. (2010). Review article: High temperatures and health outcomes: A review of the literature. Scand. J. Public Health 38 (8), 826–837. doi:10.1177/1403494810377685
Morris, G., Reis, S., Beck, S., Fleming, L., Adger, W., Benton, T., et al. (2015). “Climate change and health in the UK. Scoping and communicating the longer-term ""distal"" dimensions,” in Health climate change impacts summary report card, living with environmental change. Editor S Kovats.
Morrow, N., Mock, N. B., Gatto, A., LeMense, J., and Hudson, M. (2022). Protective pathways: Connecting environmental and human security at local and landscape level with NLP and geospatial analysis of a novel database of 1500 project evaluations. Land 11 (1), 123. doi:10.3390/land11010123
Mullins, J. T., and White, C. (2019). Temperature and mental health: Evidence from the spectrum of mental health outcomes. J. Health Econ. 68, 102240. doi:10.1016/j.jhealeco.2019.102240
Mullins, J. T., and White, C. (2020). Can access to health care mitigate the effects of temperature on mortality? J. public Econ. 191, 104259. doi:10.1016/j.jpubeco.2020.104259
Murias, P., Valcarcel-Aguiar, B., and Regueiro-Ferreira, R. M. (2020). A territorial estimate for household energy vulnerability: An application for Spain. Sustainability 12 (15), 5904. doi:10.3390/su12155904
Mustafa, K., Nosheen, M., and Khan, A. U. (2021). Dynamic reflections of multidimensional health poverty in Pakistan. PloS One 16 (11), e0258947. doi:10.1371/journal.pone.0258947
NADRA (2016). Prime minister national health program. Retrieved from: https://www.nadra.gov.pk/local-projects/social-protection/prime-minister-national-health-program/.
NASA (1958). Power | Data access viewer, prediction of worldwide energy and climate resources. Retrieved from: https://power.larc.nasa.gov/data-access-viewer/.
Nawaz, S. (2021). Energy poverty, climate shocks, and health deprivations. Energy Econ. 100, 105338. doi:10.1016/j.eneco.2021.105338
Nuţă, F. M., Nuţă, A. C., Zamfir, C. G., Petrea, S.-M., Munteanu, D., and Cristea, D. S. (2021). National carbon accounting—analyzing the impact of urbanization and energy-related factors upon CO2 emissions in central–eastern European countries by using machine learning algorithms and panel data analysis. Energies 14 (10), 2775. doi:10.3390/en14102775
Paavola, J. (2017). Health impacts of climate change and health and social inequalities in the UK. Environ. Health 16 (1), 113–168. doi:10.1186/s12940-017-0328-z
Pakistan Bureau of Statistics, G.O.P (2020). Pakistan Social and Living Standard Measurement (PSLM) district level survey 2019-20 microdata. Retrieved from: https://www.pbs.gov.pk/content/pslm-district-level-survey-2019-20-microdata.
Paterson, J., Berry, P., Ebi, K., and Varangu, L. (2014). Health care facilities resilient to climate change impacts. Int. J. Environ. Res. Public Health 11 (12), 13097–13116. doi:10.3390/ijerph111213097
Patrick, R., Shaw, A., Freeman, A., Henderson-Wilson, C., Lawson, J., Davison, M., et al. (2019). Human well-being and the health of the environment: Local indicators that balance the scales. Soc. Indic. Res. 146 (3), 651–667. doi:10.1007/s11205-019-02140-w
Pelz, S., Pachauri, S., and Groh, S. (2018). A critical review of modern approaches for multidimensional energy poverty measurement. Wiley Interdiscip. Rev. Energy Environ. 7 (6), e304. doi:10.1002/wene.304
Péres, W. E., Ribeiro, A. F., Russo, A., and Nunes, B. (2020). The association between air temperature and mortality in two Brazilian health regions. Climate 8 (1), 16. doi:10.3390/cli8010016
Peters, D. H., Garg, A., Bloom, G., Walker, D. G., Brieger, W. R., and Hafizur Rahman, M. (2008). Poverty and access to health care in developing countries. Ann. N. Y. Acad. Sci. 1136 (1), 161–171. doi:10.1196/annals.1425.011
Qurat-ul-Ann, A. R., and Mirza, F. M. (2021). Multidimensional energy poverty in Pakistan: Empirical evidence from household level micro data. Soc. Indic. Res. 155 (1), 211–258. doi:10.1007/s11205-020-02601-7
Rylander, C., Øyvind Odland, J., and Manning Sandanger, T. (2013). Climate change and the potential effects on maternal and pregnancy outcomes: An assessment of the most vulnerable–the mother, fetus, and newborn child. Glob. Health Action 6 (1), 19538. doi:10.3402/gha.v6i0.19538
Sangkhaphan, S., and Shu, Y. (2019). The effect of rainfall on economic growth in Thailand: A blessing for poor provinces. Economies 8 (1), 1. doi:10.3390/economies8010001
Sarmiento, J. H. (2023). Into the tropics: Temperature, mortality, and access to health care in Colombia. J. Environ. Econ. Manag. 119, 102796. doi:10.1016/j.jeem.2023.102796
Schramm, P., Brown, C., Saha, S., Conlon, K., Manangan, A., Bell, J., et al. (2021). A systematic review of the effects of temperature and precipitation on pollen concentrations and season timing, and implications for human health. Int. J. Biometeorology 65 (10), 1615–1628. doi:10.1007/s00484-021-02128-7
Sen, A. (1994). Inequality reexamined, trad. it. La diseguaglianza. Un riesame critico. Bolonga: Il Mulino.
Sharma, A. (2014). Spatial analysis of disparities in ldl-C testing for older diabetic adults: A socio-environmental framework focusing on race, poverty, and health access in Mississippi. Appl. Geogr. 55, 248–256. doi:10.1016/j.apgeog.2014.09.017
Sheridan, S. C., and Allen, M. J. (2015). Changes in the frequency and intensity of extreme temperature events and human health concerns. Curr. Clim. Change Rep. 1 (3), 155–162. doi:10.1007/s40641-015-0017-3
Simões, N., Crespo, N., Moreira, S. B., and Varum, C. A. (2016). Measurement and determinants of health poverty and richness: Evidence from Portugal. Empir. Econ. 50 (4), 1331–1358. doi:10.1007/s00181-015-0967-2
Sokołowski, J., Lewandowski, P., Kiełczewska, A., and Bouzarovski, S. (2020). A multidimensional index to measure energy poverty: The polish case. Energy Sources, Part B Econ. Plan. Policy 15 (2), 92–112. doi:10.1080/15567249.2020.1742817
Thompson, A. A., Matamale, L., and Kharidza, S. D. (2012). Impact of climate change on 'children's health in Limpopo Province, South Africa. Int. J. Environ. Res. Public Health 9 (3), 831–854. doi:10.3390/ijerph9030831
UNDP (2015a). Department of economics and social affairs. Sustainable development. Retrieved from: https://sdgs.un.org/goals.
UNDP (2015b). What are the sustainable development goals (SDGs)? Retrieved from: https://www.undp.org/content/undp/en/home/sustainable-development-goals.html.
Wang, Q.-S., Hua, Y.-F., Tao, R., and Moldovan, N.-C. (2021). Can health human capital help the sub-saharan africa out of the poverty trap? An ARDL model approach. Front. Public Health 9, 697826. doi:10.3389/fpubh.2021.697826
Watt, S., and Chamberlain, J. (2011). Water, climate change, and maternal and newborn health. Curr. Opin. Environ. Sustain. 3 (6), 491–496. doi:10.1016/j.cosust.2011.10.008
Weck, R. L., Paulose, T., and Flaws, J. A. (2008). Impact of environmental factors and poverty on pregnancy outcomes. Clin. Obstetrics Gynecol. 51 (2), 349–359. doi:10.1097/grf.0b013e31816f276e
Weeks, J. R., Getis, A., Stow, D. A., Hill, A. G., Rain, D., Engstrom, R., et al. (2012). Connecting the dots between health, poverty and place in Accra, Ghana. Ann. Assoc. Am. Geogr. 102 (5), 932–941. doi:10.1080/00045608.2012.671132
Weziak-Bialowolska, D. (2016). Spatial variation in EU poverty with respect to health, education and living standards. Soc. Indic. Res. 125 (2), 451–479. doi:10.1007/s11205-014-0848-7
WHO (2018). Household air pollution and health. WHO. Retrieved from: https://www.who.int/news-room/fact-sheets/detail/household-air-pollution-and-health.
WHO (2020). HIV/AIDS. Retrieved from: https://www.who.int/news-room/fact-sheets/detail/hiv-aids.
WHO (2021). Climate change and health. WHO. Retrieved from: https://www.who.int/news-room/fact-sheets/detail/climate-change-and-health.
Wibawa, B. S. S., Maharani, A. T., Andhikaputra, G., Putri, M. S. A., Iswara, A. P., Sapkota, A., et al. (2023). Effects of ambient temperature, relative humidity, and precipitation on diarrhea incidence in Surabaya. Int. J. Environ. Res. Public Health 20 (3), 2313. doi:10.3390/ijerph20032313
Wolkoff, P. (2018). Indoor air humidity, air quality, and health–An overview. Int. J. Hyg. Environ. Health 221 (3), 376–390. doi:10.1016/j.ijheh.2018.01.015
Wondmagegn, B. Y., Xiang, J., Dear, K., Williams, S., Hansen, A., Pisaniello, D., et al. (2021). Increasing impacts of temperature on hospital admissions, length of stay, and related healthcare costs in the context of climate change in Adelaide, South Australia. Sci. Total Environ. 773, 145656. doi:10.1016/j.scitotenv.2021.145656
Wu, Y., Xia, L., He, J., Meng, Q., Yang, D., Liu, F., et al. (2022). Investigating accessibility of hospitals in cold regions: A case study of harbin in China. HERD Health Environ. Res. Des. J. 16, 142–160. doi:10.1177/19375867221120201
Keywords: health poverty, climate change, alkire-foster methodology, univariate and bivariate LISA, inequalities, Pakistan
Citation: Khan SU, Sheikh MR, Dimen L, Batool H, Abbas A and Nuta AC (2023) Nexus between health poverty and climatic variability in Pakistan: a geospatial analysis. Front. Environ. Sci. 11:1180556. doi: 10.3389/fenvs.2023.1180556
Received: 06 March 2023; Accepted: 09 May 2023;
Published: 19 May 2023.
Edited by:
Ashfaq Ahmad Shah, Hohai University, ChinaReviewed by:
Dingde Xu, Sichuan Agricultural University, ChinaIbrahim Cutcu, Hasan Kalyoncu University, Türkiye
Copyright © 2023 Khan, Sheikh, Dimen, Batool, Abbas and Nuta. This is an open-access article distributed under the terms of the Creative Commons Attribution License (CC BY). The use, distribution or reproduction in other forums is permitted, provided the original author(s) and the copyright owner(s) are credited and that the original publication in this journal is cited, in accordance with accepted academic practice. No use, distribution or reproduction is permitted which does not comply with these terms.
*Correspondence: Levente Dimen, ZGltZW5sZXZAeWFob28uY29t; Alina Cristina Nuta, YWxpbmFudXRhQHVuaXYtZGFudWJpdXMucm8=