- 1Department of Aquatic Sciences and Assessment, Swedish University of Agricultural Sciences, Uppsala, Sweden
- 2Department of Soil and Environment, Swedish University of Agricultural Sciences, Uppsala, Sweden
- 3Department of Civil and Environmental Engineering, Trinity College Dublin, Dublin, Ireland
Erosion, soil loss and consequent nutrient fluxes impair water quality and can degrade arable soils. Erosion rates in Sweden are generally low but episodic losses of suspended solids (SS) can affect water quality. Identifying critical source areas (CSAs) and “hot moments” is essential to reduce erosive losses from arable land. Here we use a distributed, dynamic high-resolution erosion model that simulates the sum of all transported material, i.e., erosion within the soil profile, on the soil surface and transport through drainage systems. We simulate monthly SS transport in six small agricultural catchments with varying soil texture over 8 years. Kling-Gupta Efficiency (KGE) was used as model performance statistics, and calibration (KGE = 0.45–0.78) and validation (KGE = 0.64–0.83) showed acceptable model performance for all catchments, with mean annual SS losses between 2.1 and 31.5 t km-2yr-1. Equifinality could be minimised by using more precise initial parameter values. We suggest that the model can be applied to comparable unmonitored catchments to identify erosion-sensitive periods and CSAs.
1 Introduction
Soil loss from arable land is a major problem globally, resulting in reduced crop production and impaired water quality associated with transport of nutrients and pesticides from fields to receiving surface waters (Boardman and Poesen, 2006). In Europe, water-induced erosion is the most important erosive process, whereas, e.g., wind-induced erosion is more important in other parts of the world (Panagos et al., 2015). Landscape-scale hydrology and hydrological conductivity need to be considered when describing the process of water-induced erosion. There are multiple pathways for water to move through the landscape, e.g., streams, surface runoff, subsurface flow, macropore (quick) flow and drainage systems. When implementing measures to control erosion, there is also a need to identify where, when and under what circumstances it occurs. Mean soil erosion losses in Europe are 10–880 t km−2 year−1 (Verheijen et al., 2012). Sweden is at the lower end of this range, with mean losses of 1–175 t km-2 year−1 (Ulén et al., 2012), but inputs of suspended solids (SS) and associated solutes to receiving surface waters can still cause problems.
Different soil types are more or less vulnerable to erosion, e.g., finer soil types are more vulnerable than coarser soils (Ulén and Jakobsson, 2005; Villa Solís, 2014). Erosion can occur within the soil, through permanent cracks and channelized pathways (Øygarden et al., 1997), and on the soil surface. Eroded material can be transported through, e.g., surface runoff, via surface inlet wells and through drainage systems. The infiltration capacity of soils in humid temperate areas, including Sweden, is generally high relative to rainfall intensity and rarely leads to infiltration-excess surface runoff (Grip and Rodhe, 2000). However, downslope flow of water may locally exceed soil storage capacity and result in flow over the surface (Beven and Kirkby, 1979). In such cases, topography exerts first-order control on spatial variations in hydrological conditions (Sörensen et al., 2006), which can be captured by high-resolution digital elevation models (DEM). There are different intensities of surface erosion, with sheet erosion being the most common form in Sweden (Villa et al., 2014). Sheet erosion is the uniform loss of a thin layer of topsoil, which mainly mobilises and transports smaller particles with a large surface area. These particles can carry nutrients, especially phosphorus (P). Other forms of erosion are rill erosion, where small channels are formed by small, intermittent water courses, and gully erosion, where small channels develop into deeper channels. Gully erosion is not very common in Sweden, but can occur during extreme rainfall events. Almost half (47%) of Swedish agricultural fields are artificially drained (Statistics Sweden, 2017), so particles can be transported through drainage pipes and out to receiving waters. Inlets, drainage wells and even macropores can intercept overland flow and act as entry points for particles and associated nutrients to drainage systems (Djodjic, 2001; Djodjic and Markensten, 2019).
Impacts of SS input to surface waters include, e.g., reduced penetration of light and changes in temperature (Bilotta and Brazier, 2008). Suspended solids can also carry other pollutants, e.g., pesticides, heavy metals (Kronvang et al., 2003) and nutrients, mainly P (Haygarth et al., 2006; Sandström et al., 2020). Since mitigation measures are costly, time-consuming and cannot be applied everywhere, modelling can be used to identify target areas particularly sensitive to soil erosion, referred to as critical source areas (CSAs) (Pionke et al., 2000; Sharpley et al., 2015). Identification of CSAs is crucial for catchment management, i.e., in targeting areas where measures for controlling erosion and particle losses will have the greatest effect. Numerous large-scale erosion models have been developed, e.g., Panagos et al. (2015) modelled European Union (EU)-scale soil erosion at 25 × 25 m2 grid scale using a modified version of the Revised universal Soil Loss Equation (RUSLE) and found that soil loss rates were highest for cropland. Sediment transport have been modelled for small Danish catchments using the Water and Tillage Erosion Model (WaTEM), where they found that 6% of all farmland had a high erosion risk (Onnen et al., 2019). Attempts have been made in Sweden (Djodjic and Villa, 2015) and Ireland (Thomas et al., 2016) to model soil losses using high-resolution data, and specifically to identify CSAs. Djodjic and Markensten (2019) modelled CSAs for the entire south of Sweden, covering more than 90% of arable land, using a “worst case scenario” approach that gave good agreement between measured and observed erosion-prone areas. They used the modified Unit Stream Power Erosion Deposition (USPED) model (Mitasova et al., 2001) incorporated within the PCRaster framework to simulate SS transport, but also SS as a proxy for total P transport. Thomas et al. (2016) identified CSAs using a hydrologically sensitive area (HSA) index and mobile soil P concentrations (water-extractable P, WEP) to predict the risk of dissolved P losses in runoff from legacy soil P. They included landscape-scale hydrology, taking into account hydrological connectivity between the modelled CSAs. They found a strong relationship between total CSA index value within the HSA and total reactive P load in runoff, allowing them to identify areas within the studied catchments at high risk of P losses that should be targeted by mitigation measures.
However, there is a lack of studies on CSAs and associated SS transport over both space and time. An ability to identify environmental conditions and periods of the year when CSAs are most active would enable better targeting of mitigation measures. Using the modified USPED model, it is possible to simulate SS export at any location within a catchment. Modelling this transport over time and identifying the types of catchments for which this approach works would provide valuable information for farmers and stakeholders. Assessment of model sensitivity through calibration and identification of optimal, catchment-specific parameter values for k (soil erodibility), p (soil permeability) and c (vegetation cover-land use) would further improve the model and provide a deeper understanding of SS transport in the landscape. In addition, the possibility to test model results against measured SS values during calibration and validation would allow for evaluation of model robustness.
The static version of the unit stream power erosion deposition model was primarily developed for catchments dominated by clay soils. The objectives in the present study were to develop a new dynamic version of the model covering a broader range of soil texture; to assess whether this dynamic model can be applied to catchments with varying soil texture, climate conditions and land use distribution; and to quantify variations in model parameters between catchments. The possibility to use simulated SS transport as a proxy for particulate P (PP) transport was also explored. Ultimately, a model calibrated on a range of catchments could be used on other similar, but unmonitored, catchments. The overall aim in this study was thus to extend the revised USPED model to support quantitative, distributed, dynamic high-resolution modelling of erosion and SS transport. This was performed by calibration and validation of the updated model for a range of small agricultural catchments in Sweden (Figure 1), using long time series of high-quality monitoring data. The hypothesis is that monthly erosion rates in small agricultural catchments may be properly quantified using high-resolution distributed models by accounting for catchments’ hydrology, topography, and land use and soil distribution.
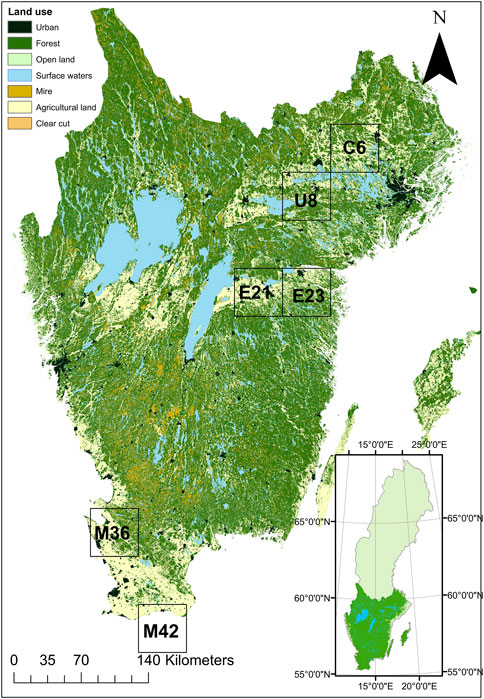
FIGURE 1. Approximate location of the six selected catchments in southern Sweden, with land use as a background map. The catchments are located somewhere within the 50 × 50 km2 square, exact location cannot be provided due to the conditions in the monitoring programme.
2 Material and methods
2.1 Catchment descriptions
All catchments used in this study are part of the Swedish agricultural catchment monitoring programme, which focuses on nutrient losses under various climate, geohydrological and agricultural production conditions (Kyllmar et al., 2014) (Table 1). Water discharge at the catchment stream outlet is measured continuously and aggregated to daily values. Flow-proportional water quality sampling is performed fortnightly and water quality parameters are analysed at the certified laboratory at the Department of Aquatic Sciences and Assessment, following standard methods. In the present study, data on daily water discharge (m3 s−1) calculated to monthly specific runoff (mm month−1) and calculated monthly SS loads (kg km−2 month−1) (Linefur et al., 2019) were used. In observation fields (21E and 2M) within two of the catchments (E21 and M42), water quality is sampled directly from the drainage pipe outlet. Data from these observation fields were also used in model evaluation, to test performance at field scale. The catchments were chosen to capture a range of soil textures and climate conditions, in order to test the model over a range of conditions (Figure 2). Although all catchments are dominated by agriculture, the proportion of arable land ranges from 58% to 95% (Table 1).
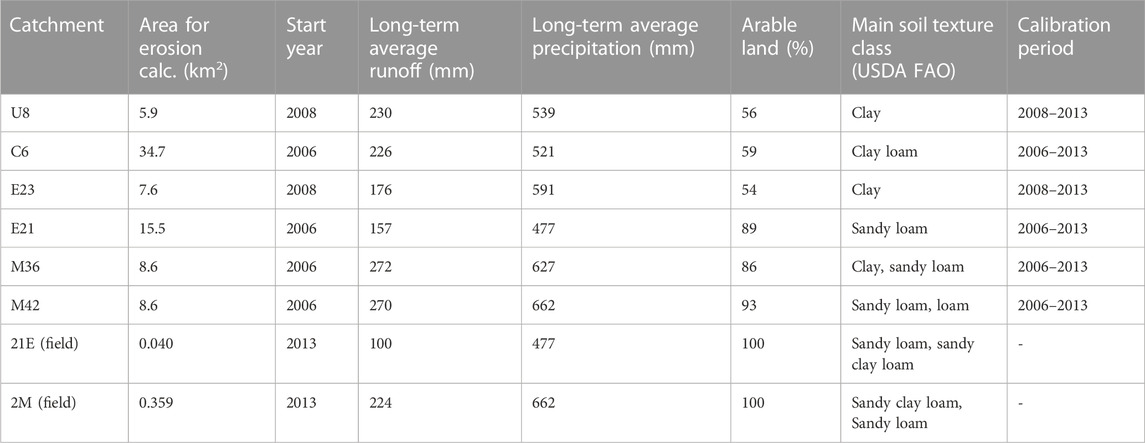
TABLE 1. Catchment characteristics. Long-term yearly average values of runoff (2005/2006–2016/2017) and precipitation (1961–1990) from Linefur et al. (2019) for catchments and Norberg et al. (2021) for observation fields. Average runoff for observation fields represents the period 2006/2007–2019/2020. Calibration periods start by year of available data. Validation period for all catchments was 2013–2021.
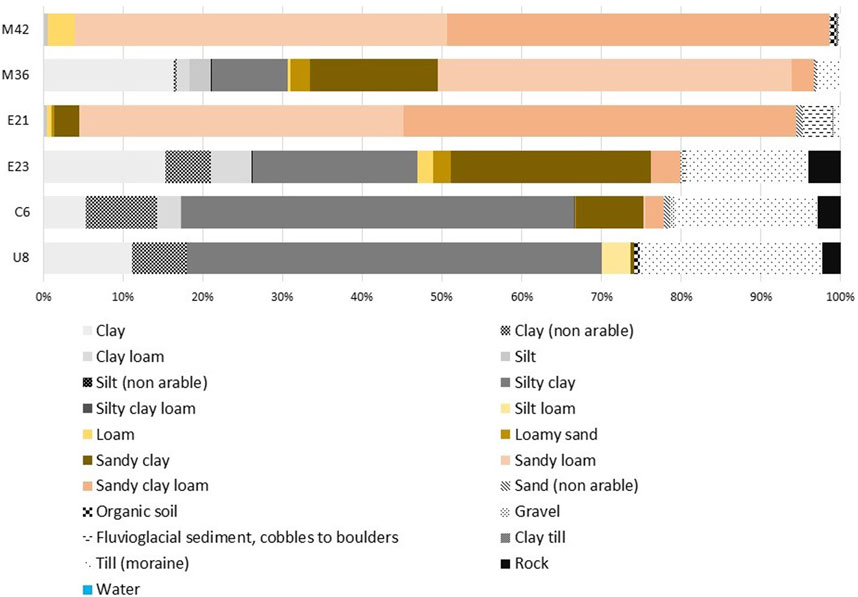
FIGURE 2. Soil distribution in the six catchments, based on the digital soil map for all arable land in Sweden (FAO) (Söderström and Piikki, 2016) and a map from the Swedish Geological Survey (SGU) showing all non-arable land (SGU, 2016). The x-axis indicates percentage of different soil textures, which are indicated by different colours. Yellow, orange and grey scales represent arable land according to the FAO classes, while blue and black patterned scales represent water and non-arable land, respectively.
2.2 Model description
A modified version of the USPED model was used in this study. The original USPED model is a distributed model based on the universal Soil Loss Equation (USLE) (Wischmeier and Smith, 1978) and RUSLE (Renard et al., 1991) models. The modified USPED model predicts the spatial distribution of erosion and deposition patterns based on change in overland flow depth and local terrain geometry, with consideration given to the influence on erosion/deposition patterns of flow convergence or divergence (Mitasova et al., 1996; Rieke-Zapp and Nearing, 2005). We made the model dynamic by applying a monthly time step of specific runoff (mm month−1) to each cell and simulated either net erosion or net deposition depending on cell properties (slope, soil texture class, land cover, etc.). In the modified USPED model, the slope length factor (LS) in the RUSLE equation is replaced with upslope contributing area (Moore and Burch, 1986), calculated as:
where A is upslope contributing area (m2) and b is the slope angle in degrees. Here the exponent value of 1.6 was used as in Mitasova et al. (2001), and 22.13 is a unit conversion factor. The LS value for the catchments in this study ranged from 0 to 0.742. Parameter p represents soil permeability and varies for different soil texture classes (Supplementary Table S1).
To account for the effect of slope shape (concave or convex) on erosion and deposition patterns, maps of slope profile (ProfCurv) and tangential curvature (TanCurv) based on catchment DEMs were created, using profcurv and plancurv (PCRaster, 2013) from the PCRaster package in Python (Karssenberg et al., 2010). ProfCurv describes the shape of the slope profile, where a positive value indicates a concave shape and a negative value a convex shape. The value of ProfCurv for the six selected catchments ranged from −3.25 to 6.09. For TanCurv, describing the tangential curvature, a positive value represents convex diversion of flow and a negative value represents concave flow concentration. The value of TanCurv ranged from −3.81 to 3.76. Erosion/deposition (ED) patterns were then calculated as:
where R is monthly runoff (mm month-1) (range 0–104), k is soil erodibility factor, c is a vegetation cover factor and 4 is a scaling factor (determined by the map resolution, 2 × 2 m2). R varies with a monthly time step, as does the c value for arable land (values representative for autumn wheat were used here) (Supplementary Table S2). Both k and p vary with soil texture classes (Supplementary Table SA1). The model was calibrated against measured suspended solids (SS) loads (kg km−2 month−1) at the outlet of each catchment, which is a sum of all hydrological pathways in the catchment (i.e., surface runoff, internal erosion, drainage wells and tile drains). The values of k and p were assumed to include all these pathways, without explicitly specifying them in the simplified model. A concave slope (positive ProfCurv) will result in a negative value of the term, indicating net deposition in that cell while a convex shape (negative ProfCurv) will result in a positive value, indicating net erosion in that cell. Likewise for the tangential curvature, diversion of flow (positive TanCurv) will result in a negative value of the term, meaning net deposition and concentration of flow (negative ProfCurv) will result in a positive value, meaning net erosion. Finally, each cell will have a net erosion or net deposition value.
High-resolution (2 × 2 m2) DEMs of each catchment were used (Supplementary Figure S1) (Lantmäteriet, 2014). From the DEM, maps of flow direction (local drainage direction), slope and slope length were created using the tools ldd, slope and slopelength (PCRaster, 2013). To account for land use in the catchments, the Swedish land cover data map was used (Naturvårdsverket, 2019). Soil texture maps of the catchments were based on the digital soil map for arable land (Söderström and Piikki, 2016) and the Swedish Geological Survey (SGU) map (SGU, 2016) for all non-arable land. All maps were cut and re-sampled to the same cell size and extent as in the DEM, using the ArcMap tools Clip and Resample. The model itself was created and run in Python 3.6.6, using the PCRaster package. The outputs of modelling were a map of erosion/deposition patterns (where particles are mobilised) and erosion accumulation (where particles accumulate), and a time series of exported SS at the catchment outlet. Accumulated erosion was calculated using the accuflux tool (PCRaster, 2013).
2.3 Model calibration
The SPOTPY optimisation tool was used for model calibration (Houska et al., 2015). The calibration algorithm chosen was Monte Carlo (MC) (see Houska (2021) for algorithm description). The objective function chosen was Kling-Gupta efficiency (KGE). In recent years, KGE has been frequently used for model evaluation within hydrological modelling, since it better describes the variability in data than, e.g., Nash-Sutcliffe efficiency (NSE) (Gupta et al., 2009). KGE combines the correlation between simulated and observed data (r), the ratio of standard deviation between simulated data and standard deviation of observed data (α) and the ratio of the mean value of simulated data and mean value of observed values (β) (Gupta et al., 2009):
The value of KGE ranges between–Inf and 1, where 1 represents perfect model fit.
The time series datasets available for each catchment were split into two, with one-half used for model calibration and the other half for model validation (Table 1). The validation period was chosen to be the same length for all catchments (2013–2021) and 1 year was defined as the agro-hydrological year (July-June). For calibration and validation, monthly specific runoff (mm month-1) and monthly SS transport (kg km−2 month−1) were used. The three calibration parameters were p, k and c (Eqs 1, 2). As the values of k and p differ for different soil types and the value of c differs for different land use categories, a percentage change was imposed on the initial values of k, p and c (k0, p0 and c0) during the calibration, as shown in Eq. 4, shifting the baseline of the parameters using pseudo parameters (kpar, ppar and cpar). See Supplementary Tables S1, S2 for initial values for all parameters.
The same structure was used for p and c. For the two catchments that contained observation fields, the calibration was performed against the catchment outlet and the validation against both catchment outlet and observation field outlet. Each catchment was calibrated separately, using the following steps.
1 Initial calibration where all parameters allowing to vary with a starting parameter range (Supplementary Tables S1, S2) with 100 repetitions to explore the parameter space.
2 One parameter at a time was locked, and the other two were varied freely within the starting parameter range, with 100 repetitions for each combination, to assess how sensitive the different parameters were and whether the pattern of variation (in relation to KGE values) changed.
3 All variables were varied freely within the starting parameter range, with 1000 repetitions.
4 An initial evaluation of the parameter values and their corresponding KGE values was performed using dotty plots. Based on this, parameter ranges for ppar and cpar were expanded (Supplementary Tables S1, S2).
5 All parameters were varied freely within the new parameter range, with 1000 repetitions.
6 The 50 best parameter combinations for each catchment were chosen, and the model was run using these parameter combinations on the validation period for all six catchments and for the two observation fields. Since a degree of equifinality (similar model performance for several parameter combinations) was found, a range of parameter combinations was chosen for evaluation. Since the range of good KGE values (>0.5) varied between catchments, the top 10 best runs were plotted against observed values, and KGE values for the validation period were calculated.
The same parameter setup was used for validation of the results for the two observation fields as the bigger catchments they were located within. However, measured water discharge (specific runoff) for the fields were used. After evaluation of the validation results, the model was also run for another ‘test point’ located slightly upstream in one of the observation fields (21E), which was more representative for transport from the field in general. This was done to explore how errors in the DEM can affect the results, especially when zooming in on small areas.
Final values for parameters k and p were calculated based on the best parameter combinations of kpar, ppar and cpar for each catchment, and then multiplied by the initial parameter value for each texture class, using Eq. 4.
To explore possible parameter set equifinality, average area-weighted c, p and k values (caw, paw, kaw) were calculated for each catchment. For k and p, the area-weighted average was calculated as the sum of the initial k and p values for each soil type multiplied by the proportion of area of that specific soil type. These average area-weighted parameter values were multiplied by the kpar and ppar values for the 10 runs with the highest KGE values, to evaluate equifinality in the modelling results. For c, the area-weighted average was calculated as the sum of the initial c values for each land use category multiplied by the proportion of area of that specific land use. As initial c values for arable land vary on a monthly basis, the annual average c factor was used. As for k and p factors, the average area-weighted value of parameter c was multiplied by the cpar value of the 10 runs with the highest KGE values.
2.4 Statistical analysis
To test whether the simulated SS values could be used as a proxy for particulate P (PP), linear regressions were performed between simulated SS (kg km−2 month−1) and measured and calculated transport of particulate P (kg km-2) from the selected catchments. This was also done for measured SS and measured PP. Factor of variation (FV) value (highest value divided by lowest value) was calculated for both observed and simulated loads of SS. All statistical analyses were performed in R 4.0.3 using the lm function in the stats package (R Core Team, 2020).
3 Results
3.1 Measured annual SS transport and simulated values
There was a wide range of measured annual SS transport (t km−2 yr−1) for the different catchments, with C6 and U8 having the highest mean annual SS transport in both the calibration and validation periods (>30 t km−2 yr−1), and E21 the lowest (2.2 and 2.1 t km−2 yr−1, respectively) (Table 2). There was also high temporal variation in both measured and simulated annual SS transport. The years 2010–2011 and 2016–2017 had particularly low SS transport in all catchments, while high SS transport was recorded in 2019–2020 in all catchments (Table 2).
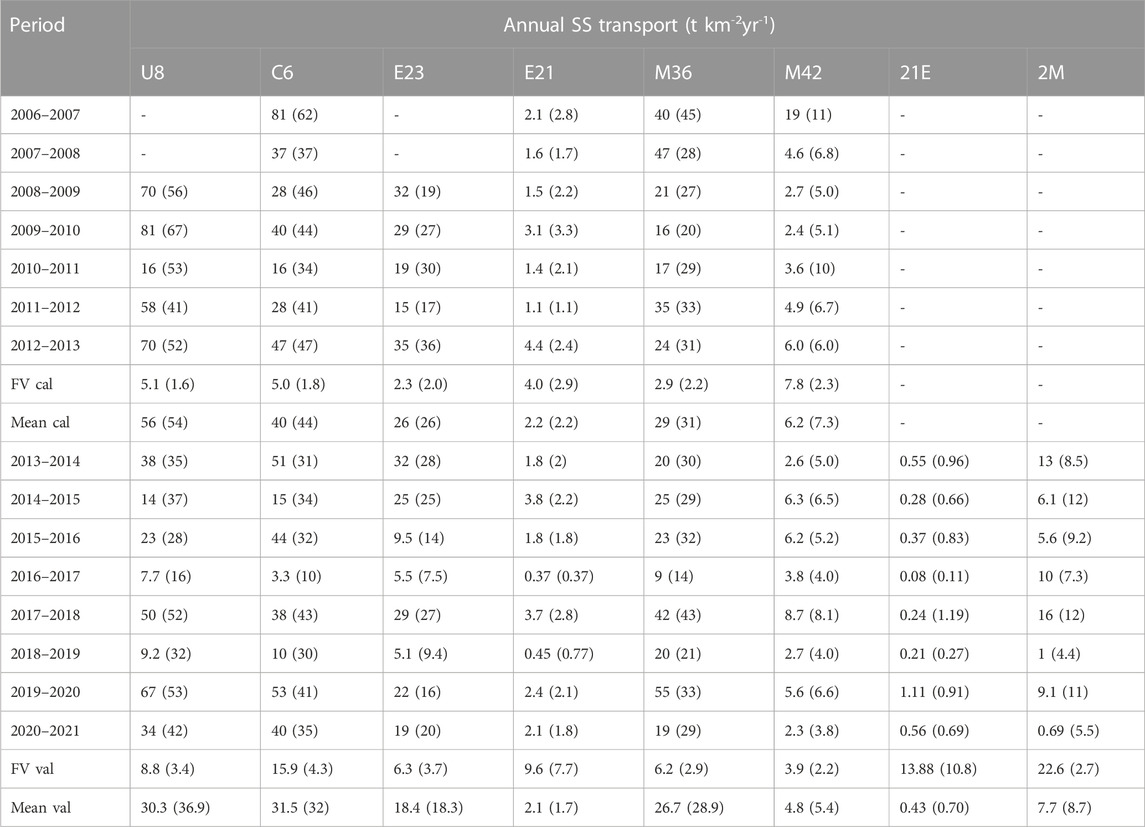
TABLE 2. Measured annual suspended solids (SS) transport (t km-2 yr-1) in the calibration and validation periods. Factor of variation (FV) value, calculated as the ratio between maximum and minimum, is shown for all measured values. Total mean value for both periods is also shown, with corresponding simulated values in brackets. For field 21E, simulated values for the ‘test point’ are shown. Fields 21E and 2M were calibrated against their catchment outlets (E21 and M42), so no values for the calibration period are shown.
There was good agreement (KGE>0.6) between simulated and observed values for the validation period for all catchments (Figure 3; Tables 2, 3). Some months were overestimated by the model, but in general temporal erosion patterns were successfully captured. The model was also capable of reasonably accurate quantification of SS transport for the two catchments with sandy loam soils (E21 and M42), which had quite low SS export compared with the other catchments (Tables 2, 3; Figure 3). There was a clear seasonal pattern in SS transport, with higher losses during winter-spring and very low losses during summer-autumn (Figure 3). In four of the six catchments (U8, C6, E23, M36), the largest peak was observed in winter 2020, where the model failed to capture peak magnitude (Figure 3). This was also the year in which all catchments had the highest annual SS losses (Table 2).
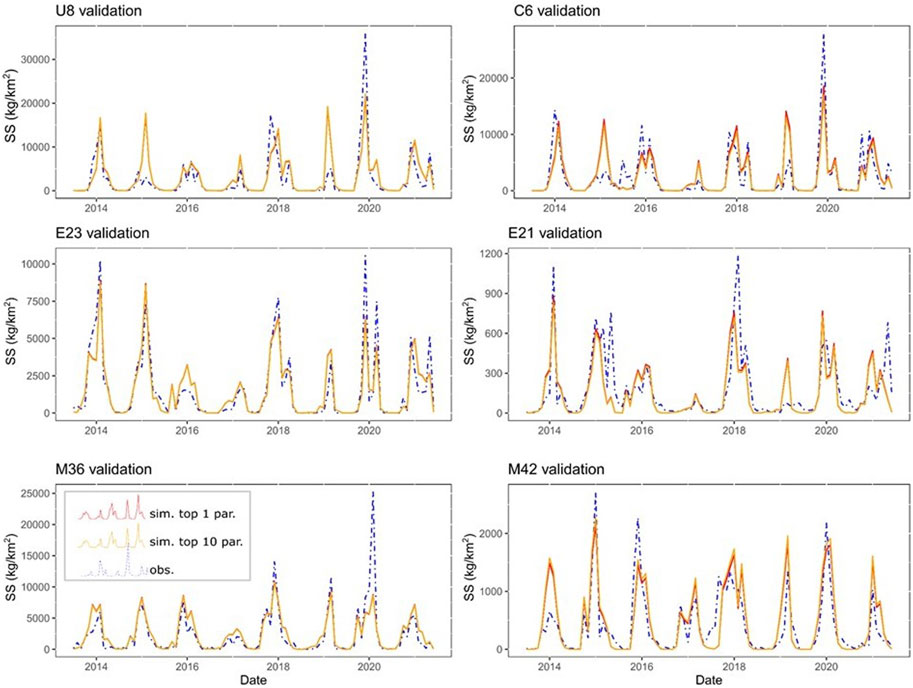
FIGURE 3. Simulated monthly suspended solids (SS) transport from the six catchments in the validation period. Solid lines represent simulated values, orange the best parameter combination and red the 10th best parameter combination. Dashed blue lines represent monthly observed values. Please note the difference in scale of the y-axis.
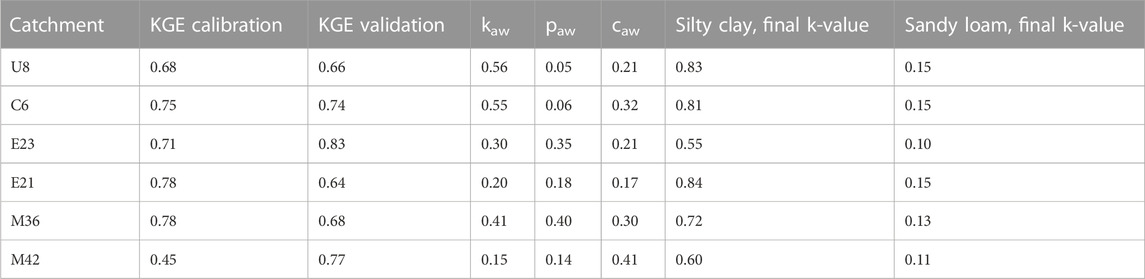
TABLE 3. Kling-Gupta efficiency (KGE) value of the best parameter combination for each catchments in the calibration and validation periods and corresponding re-calculated area-weighted parameter values. Final values for soil erodibility parameter k for silty clay and sandy loam soils, based on the best pseudo parameter kpar from the calibration, are also shown.
Total mean SS transport from all catchments during the calibration period was simulated accurately, especially for catchments U8, E23, E21, and M36 (Table 2). During the validation period, the simulated mean values for C6, E23 and M36 were very similar to observed values (Table 2). During the calibration period, KGE values were generally high (>0.67) except for M42 (0.45) (Table 3), but the FV value was higher (7.8) for observed SS transport values than for the simulated values (2.3) (Table 2). In the validation period, however, the KGE value for M42 increased markedly compared with the calibration period (Table 3). The low KGE value for that catchment during the calibration period was probably due to one large peak at the start of the period being completely missed by the model (data not shown), also resulting in the high FV value.
On zooming in on the two observation fields, the model fit was worse than for the catchments. For field 2M, located within catchment M42, the dynamics of SS transport were captured by the model to some extent (Figure 4), although the model underestimated some high peaks (winter 2014 and 2018) and overestimated SS transport on other occasions (2019 and 2021). However, the total sum of SS transport was in good agreement with measured values (Table 2). At the modelled outlet of observation field 21E, erosion transport was greatly overestimated by the model. Model fit was better for the ‘test point’ in field 21E, but was still an overestimate. For both observation fields, the observed values of SS were low.
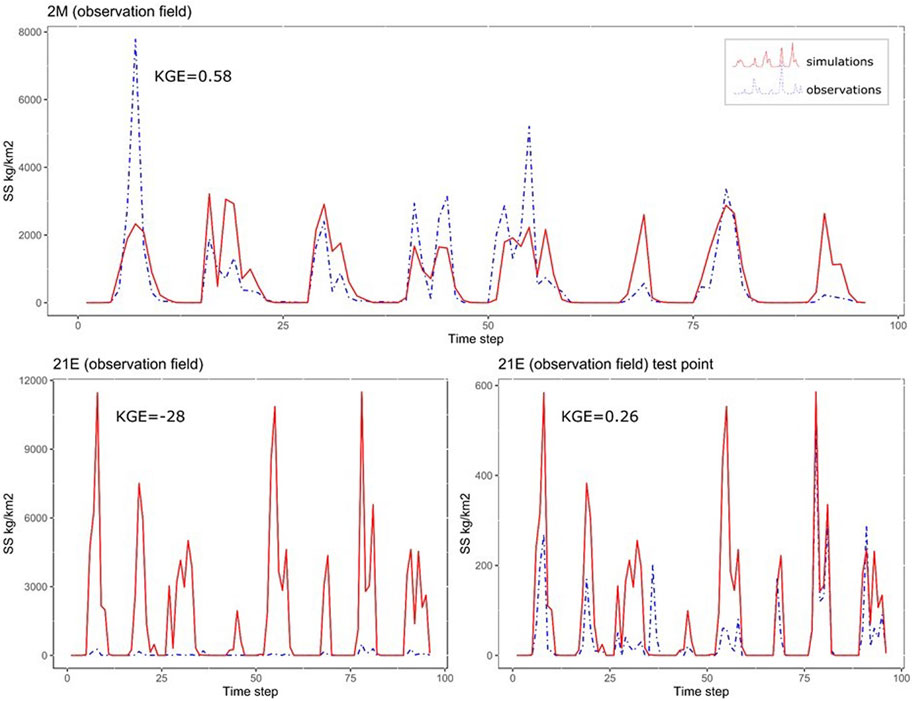
FIGURE 4. Simulated and observed monthly suspended solids (SS) transport from the two observation fields. Solid red lines are simulated values and dotted blue lines are observed values. The top panel displays the simulated and observed values over time for field 2M, while the bottom panels display simulated and observed values for (left) the outlet of field 21E outlet and (right) a test point located upstream in field 21E that was more representative for the field in general. Kling-Gupta efficiency (KGE) values for the simulations are shown within the diagrams.
For the two common and contrasting soil texture classes (silty clay (initial k value: 0.82) and sandy loam (initial k value: 0.15); Figure 2), the final k values, representing soil erodibility, for the best model fit were similar in catchments U8, C6, E21, and M36 (Table 3). For instance U8 (0.83) and C6 (0.81), where silty clay dominates where close to the initial value, but also E21 and M36 had similar values (0.81 and 0.72, respectively). Catchments E23 and M42, with several soil textures dominating (Figure 2), had a lower final k value for silty clay (0.55 and 0.60, respectively) (Table 3). A similar pattern was found for the final k value for sandy loam, where catchments E23 and M42 had a lower value than the other catchments (Table 3).
The simulated SS values covered 62% of the variation in measured PP transport overall (Supplementary Figure S4), while measured SS values explained 88% of the variation in measured PP transport during the validation period (see Supplementary Material S1 for more details).
3.2 Parameter sensitivity and model evaluation
After the first 1000 MC runs, the patterns of the parameter combinations were evaluated (Figure 5). For ppar and cpar in two of the catchments (E21, E23), there seemed to be a pattern of better KGE values with expansion of the parameter range. To determine whether better model performance could be achieved, the model was run again using an expanded parameter range for all catchments and re-evaluated (Supplementary Tables S1, S2; Supplementary Figure S3). All dotty plots revealed clear equifinality, with similar KGE values achieved for different combinations of the three pseudo parameters (Figure 5; Supplementary Figure S3). Hence, equifinality was further explored and evaluated, as mentioned previously.
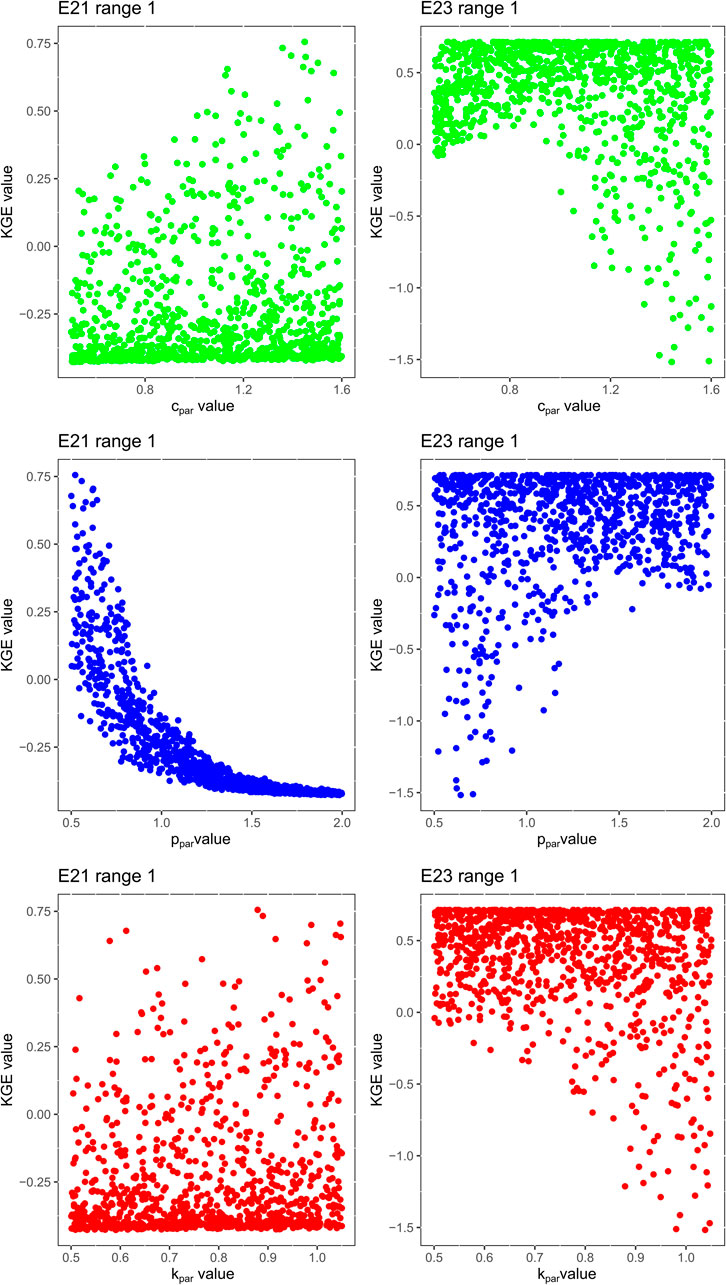
FIGURE 5. Dotty plots for the first parameter range for the three pseudo parameters cpar, ppar and kpar (1000 Monte Carlo runs) in catchments E21 and E23. Parameter value on the x-axis and corresponding Kling-Gupta efficiency (KGE) value on the y-axis.
Examination of area-weighted average values for parameters c, k and p (caw, kaw and paw) for the top 10 runs showed that the range of variation depended on the soil texture distribution within the catchments (Figure 2; Supplementary Figure S5). For instance, in catchment M36, containing sandy soils (sandy loam) and soils with higher clay content (silty clay and clay) (Figure 2), the parameter range was wider than in more uniform catchments dominated by either clay soils (C6, U8) or sandy soils (E21, M42).
3.3 Changes in spatial erosion deposition patterns
The CSAs identified in the different catchments were quite consistent over time, but more or less prominent during certain periods of the year, with some CSAs not active at all in certain time steps (Figure 6). It was also evident that erosion mobilization patterns were more active around steeper and wetter areas in the catchments (Figure 6). Simulated accumulated erosion lines followed existing ditches and the stream through the catchments, with higher amounts of accumulated erosion closer to the outlet. Based on the peak in E23 (Figure 6), it is clear that, as expected, the stream transported the largest amount of SS through the catchment.
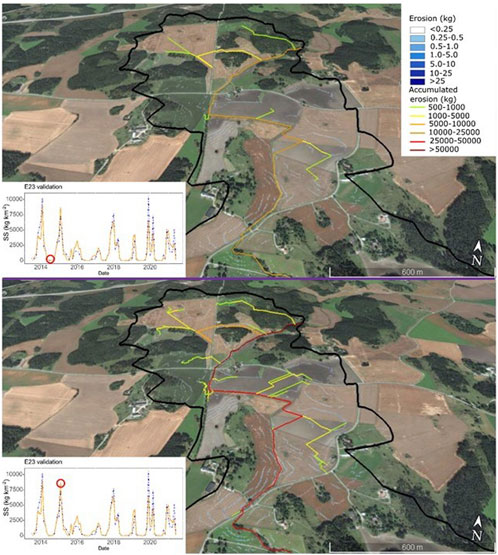
FIGURE 6. Examples of simulated erosion mobilization/deposition patterns (kg) and accumulated erosion (kg) by the outlet of catchment E23. The solid black lines represent the catchment outline, green to dark red lines represent accumulated erosion and light blue lines represent areas where particles are mobilised (especially visible in the bottom map, next to the red line representing accumulated erosion). The maps are separated by a solid purple line. Time steps are (upper panel) December 2014 and (lower panel) February 2015. Note that the underlying satellite layer is from the same time point in both diagrams and that mobilised erosion <0.25 kg is not visible in the maps.
4 Discussion
4.1 Temporal and spatial erosion transport
Measured annual SS transport values showed a wide range in the study catchments, from very low (E21) to considerably higher values (C6, U8) (Table 2). However, in comparison with erosion losses in other European countries, these values are still low. For example, in a study simulating erosion losses using the RUSLE-2015 model on European scale, the mean value for Swedish arable land was 112 t km−2 year−1, whereas arable land in other countries had mean values of up to 1500 t km−2 year−1 (Malta), but more commonly around 200–400 t km−2 year−1 (Panagos et al., 2015). All these values are higher than in the six selected catchments in this study. Actual erosion rates in Europe from sheet and rill erosion average 10–880 t km−2 year−1 (Verheijen et al., 2012), and our study catchments were at the lower end of that range. Among the Nordic countries, erosion rates are higher in Norway than in Sweden and Denmark (Bechmann et al., 2008), possibly due to differences in topography. Risk erosion classes for standard autumn ploughing in Norway range from low (<50 t km−2 year−1), to medium (50–200 t km−2 year−1), high (200–800 t km−2 year−1) and very high (>800 t km−2 year−1), with two sites in Norway and one in Finland classed as high risk in one study and two selected Swedish sites classed as medium and low risk, respectively (Ulén et al., 2012). The catchments in this study were in the low or medium risk classes (Table 2). However, even though erosion losses per se are not high in comparison to other countries, critical events where a lot of soil material is lost during short episodes can still have large impacts on receiving waters. The connection between erosion of particles and losses of P is well-known, and especially for these catchments where a power-law relationship between PP and SS concentrations in the streams has been previously established (Sandström et al., 2020). These large amounts of SS losses also lead to high P losses, which in turn impact the water quality. Simulated SS losses were in general a good proxy for PP losses (Supplementary Figure S4) and the slope is similar to previous findings (Sandström et al., 2020).
Identified CSAs in the catchments seem to be stable over time, but more or less prominent and active during certain time periods with higher losses (Figure 6). In comparison to previous studies where worst case scenarios were modelled (Djodjic and Villa, 2015; Djodjic and Markensten, 2019), the shape of the CSAs follows the topography lines more distinctly than previously, where soil texture is more prominent. Since actual measured monthly runoff values are used here, and not the sum of three high flow months representing “worst case scenario,” the SS exported was lower, resulting in lower amounts exported in each time step and hence less clear CSAs. For identification of CSAs in the catchments, the steady state model might be more suitable. However, use of a dynamic model made it possible to test model robustness by calibration against measured SS data, which was not possible previously. In addition, by using the results for parameter values in the steady state model, more robust results can be achieved.
4.2 Model fit and calibration strategy
For all catchments, good model fit (KGE values > 0.5) was obtained for both the calibration and validation periods (Figure 3; Table 3). In all catchments, the KGE values remained at approximately the same level during the calibration and validation periods, indicating stable model results. For two catchments (U8, E23), the calibration period was shorter than for the others due to later start of flow-proportional sampling (1 year later), but this did not seem to affect the results. We opted to have the same length of validation period for all catchments, in order to test all catchments on an equal basis. During calibration, the choice was made to run the simulations for each catchment for 1000 MC repetitions, as a compromise between a fair amount of parameter combinations and reasonable computing time (the model processes several high-resolution maps in each time-step, so each calibration run may take up to several minutes, or even several days for larger catchments). It is important to consider the time aspect when calibrating these types of models. By using our results, better initial values and reasonable ranges for the different parameters can be determined, hopefully leading to better model fits with fewer runs. The MC approach was used to decrease uncertainties connected to modelling results. First, 1000 MC simulations were run for each catchment and the results were evaluated in the form of dotty plots (Figure 5). After this initial evaluation, the parameter range was extended and ran and evaluated another 1000 MC simulations (Supplementary Figure S3). These multiple MC simulations decreased the number of parameter combinations giving equally good model fit and thus decreased the uncertainties. The equifinality issue is discussed further in Section 4.3.
The dynamic model simulates the sum of all transported particles and pathways through the catchment, rather than distinguishing between different pathways and processes, and is calibrated against measured data at the catchment outlet. The main purpose is to describe where mobilization occurs in the catchment, where sediment is transported and how much material is transported. Simulated accumulation lines therefore do not necessarily represent exclusively surface runoff, but rather lumped water and solute transport (i.e., including subsurface flow and flow through drainage systems) at a given point.
Since the data on SS transport showed high variability, KGE was chosen for model evaluation. Based on the results, KGE values described model fit fairly well. The model generally captured both the level of erosion in the catchments (Table 2) and temporal patterns (Figure 3), which was the objective of the study. Based on the KGE values, the model seemed to perform equally well for catchments with different soil textures (clay-dominated and sand-dominated). However, catchments dominated by sandy loam (E21 and M42) generally showed lower levels of erosion than clay-dominated catchments, which may introduce more uncertainties in the simulations. In addition, coarser-texture soils are less erosive than finer-textured soils and may have different pathways dominating P transport. It might be more important to consider other pathways, processes and factors for P losses, such as leaching through the soil profile, nutrient content in the soil and fertilisation regime, in these cases (Djodjic et al., 2018).
The agro-hydrological year 2019–2020 had the greatest observed SS transport for all catchments (Table 2), with one or 2 months (November 2019-February 2020) making a large contribution to total annual transport. The model failed to capture the magnitude of these winter peaks for four of the six catchments (U8, C6, E23, M36) in which these events represented the highest observed transport of SS during the validation period. In particular, the model was not able to adequately simulate this high SS transport for catchment M36, which had no observed peaks of the same magnitude previously. These observed peaks were the result of unusually high winter temperatures and above-normal precipitation (SMHI, 2020), possibly leading to higher runoff and precipitation on semi-frozen or thawing soil and resulting in high transport of SS. Frozen soil may be almost impermeable to water (Zuzel and Pikul, 1987) resulting in surface runoff and intensified erosion (Øygarden, 2003). At the same time, freeze-thaw cycles can increase soil erodibility, by reducing shear strength (Formanek et al., 1984). To capture more extreme events, the model would need temporally varying k and p values, coupled to air and/or soil temperature. With climate change leading to higher temperatures, milder winters and more extreme weather events (Madsen et al., 2014), a calibration period containing more extreme events could be tested to see whether the model can simulate these types more accurately. In addition, during years of low precipitation and runoff, sediment will accumulate in the streambed, to be flushed out later during these extreme events, resulting in high SS transport. The possibility to simulate SS transport over time provided by the dynamic model also opens the way for future projections and simulations under different climate change scenarios. For instance, Grusson et al. (2021) concluded that the predicted increase in heavy precipitation events in Sweden will produce more runoff than soil infiltration. Ongoing climate change is predicted to result in milder winters with higher-intensity rains and less snow accumulation in Sweden (Xu, 2000), which will have an effect on SS transport and ultimately also on P transport. A close connection between SS and P transport has been shown in previous studies modelling possible effects on P transport under different climate change scenarios. For example, a modelling study on three headwater catchments in Great Britain testing two types of models where an increase in winter precipitation led to increased P load found that the majority of the annual P load occurred during winter (under current and future climate conditions) and during the highest discharge events (Ockenden et al., 2017).
When evaluating the large model overestimation for the outlet of field 21E, we noted that a ditch running along the southern edge of the field was included in simulated SS transport at the outlet, due to inaccuracy in the DEM. This inaccuracy resulted in major overestimation of SS by the model, since in reality any SS transport in this ditch does not pass through the outlet. Therefore, the model was run for an upstream ‘test point’ where export from the ditch was not included in the output and achieved much better simulation of observed SS transport, although the model fit was weaker than for the catchment (Figure 4). These results demonstrate how small errors can have a large impact on the results, especially when zooming in to field level (21E has an area of 4 ha). Both observation fields studied are artificially drained and SS transport is measured directly from the drainage pipes, where the water consists of a mixture of surface runoff intercepted by inlet wells and/or macropores and water percolating through the soil profile. The simplified model does not take drainage into account explicitly, but previous studies have shown that modelled erosion accumulation lines generally run above the main subsurface drains and, where present, surface water inlets in the drainage system (Djodjic and Villa, 2015). The results indicate that the model simulated these losses fairly well. However, for applications at individual field level, these processes would need to be accounted for.
In previous studies, simulated values were compared with observed spatial patterns of surface runoff and erosion (Djodjic and Villa, 2015; Djodjic et al., 2018; Djodjic and Markensten, 2019) or a snapshot worst-case scenario illustrated by the 90th percentile of measured monthly loads of SS (Djodjic and Markensten, 2019). Despite the above-mentioned uncertainties and discrepancies, the dynamic modelling results presented here, with comparison to measured monthly SS loads over long periods, are a great step forward in terms of improved quantification of SS losses in small (<50 km2) catchments in Sweden. It is worth mentioning that the model input variables used in our study are derived from freely available national data sets, so similar modelling could be scaled up to any Swedish catchment.
4.3 Equifinality
In spite of using only three parameters for calibration, some equifinality issues arose, with equally good model performance achieved with different combinations of the three pseudo parameters (cpar, kpar, ppar). There was a consistent pattern for all catchments where the same parameter settings resulted in the highest KGE values in both the calibration and validation period (e.g., Supplementary Figure S2). However, some of the area-weighted parameters varied over quite a wide range for some catchments (Supplementary Figure S5). There are several possible explanations for this. First, there are possibly correlations between the three parameters themselves. In fact, on plotting parameters for the 10 runs with the highest KGE values for each catchment, we found strongly significant correlations in some cases (Supplementary Table SA3). For instance, in catchments E21 and M36 there was a strong correlation between p (soil permeability) and c (land use cover), which means that these two parameters can compensate for each other, resulting in a wider range of values of both parameters but similar KGE values. The same was true for some other catchments (Supplementary Table SA3). Second, there was a tendency for wider parameter ranges in catchments with a more diverse soil texture distribution.
The values of kaw and paw varied within a narrower range in clay-dominated catchments (C6, U8) or predominantly sandy catchments (E21), whereas in the catchments with both sandy and clay soils (E23 and M36) the parameter values tended to vary over a broader range (Figure 2, Supplementary Figure S5). It should be noted that the initial soil-specific values for k and p were used to centre the range of variation explored for these parameter values in the calibration (Eq. 3). This means that in catchments with contrasting soil texture, special attention should be paid to the initial parameter values, since overestimation of those for sandy soils and underestimation of corresponding values for clay soils, or vice versa, may result in high equifinality. Securing more representative initial parameter values by modelling more homogenous sandy/clay catchments to establish appropriate initial values might be a way forward to decrease equifinality in contrasting catchments. Finally, paw had the highest total FV values of the three parameters tested, while slope length factor LS, calculated based on p and slope conditions (eq. 1), was the least sensitive factor during sensitivity analysis of the steady state model (Djodjic and Markensten, 2019). Therefore, if constant slope values arise during model calibration, it is reasonable to assume that the least sensitive factor will need the largest range to achieve a certain change in calculated erosion.
4.4 Model limitations and future developments
There are some limitations to the model presented here. For example, further testing of the land cover parameter c is necessary to determine how it varies on arable land. In this study, a value for autumn wheat was used on all catchments, with no consideration given to crop rotation or other crops actually grown within each catchment, which is a simplification of reality. Since c can have a large impact on modelled runoff, this could improve the model results and provide a better representation of actual SS transport, particularly when zooming in on field level. Further development of remote sensing or agricultural databases, such as Agriculture Land Parcel Identification System (LPIS) (European Court of Auditors, 2016), could help in spatial identification and precise location of different crops.
The soil texture classes used as input maps to describe parameters k and p (soil erodibility and permeability) are categorical variables. In reality, each texture class represents a range of clay, silt and sand content values, with some internal heterogeneity. Specifically, two catchments with the same percentage of silty clay soil could still have different amounts of clay/silt/sand within that texture class. This could be one explanation for the lower final k value for catchment E23 (Table 3) for both silty clay and sandy loam in comparison to the other catchments. Comparing soil texture samples from E23 and C6, the E23 samples represented a smaller range and lower percentage of silt than the C6 samples and had a higher organic matter content (data not shown). These differences can explain the lower final k value for silty clay in E23 (0.55) than in C6 (0.81), as a lower percentage of silt and higher percentage of organic matter would lead to lower erodibility (Römkens et al., 1997). Except for catchment E23 and partly M42, the final k-values are very similar between catchments for both silty clay and sandy loam, indicating a robustness of the model for applications in different parts of Sweden. Replacement of soil texture classes as descriptors of k and p with, e.g., percentage clay or silt soil in the catchment could be tested to see if this discrepancy could be avoided.
The time step is another aspect of the model that could be refined. In this study a monthly time step was used, mainly because measurements and corresponding load calculations used to calibrate the model were available in monthly time steps. It would be possible to decrease this to, e.g., a daily time step, but the question is whether the model would benefit from having this finer temporal resolution considering the extra computational time required. It is known that SS and P dynamics are episodic and variable, so a single storm event could result in high SS and P losses that are missed by the model with a monthly time step. If the intention is for the model to capture these peaks, it could be beneficial to decrease the time step and try to calibrate against high-frequency measurements. If the intention is to simulate erosion patterns and amount of SS transported from the catchment over a certain period, the monthly time step is sufficient.
In this study, small catchments with agriculture as the dominant land use and with no large surface water body (lake or pond) that could retain particles and affect SS load at the outlet were modelled. Although surface erosion and associated SS and P losses are mainly an issue for arable land in Sweden, before applying the model to larger catchments it is important to determine how to handle lakes and also, e.g., urban areas. Urban areas could probably be handled by adjusting the parameter values, especially land cover (c) and p to account for impermeable areas. The model would need to be tested on catchments containing lakes and larger urban areas to determine the impact on the results. At present, we recommend restricting use of the model to headwater catchments smaller than 50 km2 and without large ponds/lakes.
The uncertainty in modelling results can also be expected to increase when applying the model outside the range of calibration conditions. Even within the calibration range, there are uncertainties associated with the model results, such as the equifinality issues discussed in Section 4.3 and lack of explicit description of subsurface transport of SS through macropores or drainage wells. As mentioned, infiltration-exceeding overland flow occurs seldom in Sweden and is usually a consequence of soil compaction (Djodjic and Villa, 2015). Satisfactory model fit was achieved under prevailing saturation-excess overland flow, but further applications and studies are needed to test model performance in conditions where infiltration-exceeding overland flow is the main cause of erosion and SS transport.
A further improvement of the model would be to model P losses directly. Earlier studies have used the USPED model (static version) to model P losses indirectly, using SS as a proxy (Djodjic and Markensten, 2019), as done here. However, previously identified relationships between SS and PP (Sandström et al., 2020) could be used directly in future model versions to enable both calibration against measured p values and simulation of P losses.
4.5 Importance for stakeholders and management
In this study, we made a dynamic version of an existing static model in order to model losses of SS over time and obtained good agreement with measured values on a small catchment scale. Our dynamic approach can be used to model similar unmonitored catchments. Authorities, advisors and farmers can then use modelling results to identify CSAs and periods of the year when the highest losses occur and target mitigation measures for nutrient and SS losses accordingly. Optimal placement of such mitigation measures is needed to ensure that they are cost-effective (Sidemo-Holm et al., 2018; Djodjic et al., 2022). The high-resolution maps generated during modelling can be used to support decision making on efficient placement of catchment mitigation measures, considering weather, land use and soil conditions where SS losses occur. Such information can also be helpful for planning farm operations, e.g., tillage, fertilisation and crop rotations. Knowledge of flow and erosion accumulation pathways can also help to evaluate hydrological connectivity (Thomas et al., 2016) and the need to improve the function of open ditches. This is especially relevant when water from upstream forest areas flows over downstream arable fields, causing mobilization of soil particles, erosion and particle-bound nutrient (mostly P) losses.
5 Conclusion
Using a dynamic USPED model within the PCRaster framework in Python, we successfully simulated SS transport at monthly time steps in six small agricultural catchments and two arable fields. Model calibration resulted in acceptable fit for all catchments, across soil texture classes. The dynamic modelling approach also made it possible to test and confirm model robustness by calibration against long-term measured SS data, thereby increasing the reliability of the model for possible future applications, as the wide range of input variables and parameter settings were tested against measured data. Some equifinality issues arose during calibration of the model, but these could be minimised by using more precise initial parameter values for the different soil texture classes and land use categories. Based on our results, the model can be applied to identify times of the year and locations sensitive to SS losses from similar unmonitored catchments.
Data availability statement
Publicly available datasets were analyzed in this study. This data can be found here: https://www.slu.se/en/departments/soil-environment/research/agricultural-water-management-/monitoring-nutrients.
Author contributions
All authors contributed to conceptualization and planning of the study. SS set up the model in Python with input from FD and HM, and SS did all model runs and calibration runs. HM helped with main calibration programming. SS did all statistical analysis. SS wrote the first draft of the manuscript. All authors contributed to the article and approved the submitted version.
Funding
This study was funded by FORMAS (Grant Number: 2017-00017) as part of a joint EU project funded by Water JPI. Four of the selected catchments (C6, E21, M36, M42) are included in the national part of the programme funded by the Swedish EPA and conducted at the Swedish University of Agricultural Sciences while the remaining two (U8, E23) are included in the regional part of the programme funded by County Administration Boards and the Swedish Agency for Marine and Water Management. MF acknowledges project PuddleJump (Grant Number: 2022-02138).
Conflict of interest
The authors declare that the research was conducted in the absence of any commercial or financial relationships that could be construed as a potential conflict of interest.
Publisher’s note
All claims expressed in this article are solely those of the authors and do not necessarily represent those of their affiliated organizations, or those of the publisher, the editors and the reviewers. Any product that may be evaluated in this article, or claim that may be made by its manufacturer, is not guaranteed or endorsed by the publisher.
Supplementary material
The Supplementary Material for this article can be found online at: https://www.frontiersin.org/articles/10.3389/fenvs.2023.1196048/full#supplementary-material
References
Bechmann, M., Deelstra, J., Stalnacke, P., Eggestad, H. O., Oygarden, L., and Pengerud, A. (2008). Monitoring catchment scale agricultural pollution in Norway: Policy instruments, implementation of mitigation methods and trends in nutrient and sediment losses. Environ. Sci. Policy 11, 102–114. doi:10.1016/j.envsci.2007.10.005
Beven, K. J., and Kirkby, M. J. (1979). A physically based, variable contributing area model of basin hydrology/un modèle À base physique de zone D'appel variable de L'hydrologie du bassin versant. Hydrological Sci. J. 24, 43–69. doi:10.1080/02626667909491834
Bilotta, G. S., and Brazier, R. E. (2008). Understanding the influence of suspended solids on water quality and aquatic biota. Water Res. 42, 2849–2861. doi:10.1016/j.watres.2008.03.018
Djodjic, F. (2001). Displacement of phosphorus in structured soils. PhD Doctoral thesis. Uppsala, Sweden: Swedish university of agricultural sciences.
Djodjic, F., Elmquist, H., and Collentine, D. (2018). Targeting critical source areas for phosphorus losses: Evaluation with soil testing, farmers' assessment and modelling. Ambio 47, 45–56. doi:10.1007/s13280-017-0935-5
Djodjic, F., Geranmayeh, P., Collentine, D., Markensten, H., and Futter, M. (2022). Cost effectiveness of nutrient retention in constructed wetlands at a landscape level. J. Environ. Manag. 324, 116325. doi:10.1016/j.jenvman.2022.116325
Djodjic, F., and Markensten, H. (2019). From single fields to river basins: Identification of critical source areas for erosion and phosphorus losses at high resolution. Ambio 48, 1129–1142. doi:10.1007/s13280-018-1134-8
Djodjic, F., and Villa, A. (2015). Distributed, high-resolution modelling of critical source areas for erosion and phosphorus losses. Ambio 44, S241–S251. doi:10.1007/s13280-014-0618-4
European Court of Auditors (2016). The land Parcel identification system: A useful tool to determine the eligibility of agricultural land—but its management could Be further improved. Luxembourg: Publications office of the European Union.
Formanek, G. E., McCool, D. K., and Papendick, R. I. (1984). Freeze-thaw and consolidation effects on strength of a wet silt loam. Trans. ASAE 27, 1749–1752. doi:10.13031/2013.33040
Grusson, Y., Wesström, I., Svedberg, E., and Joel, A. (2021). Influence of climate change on water partitioning in agricultural watersheds: Examples from Sweden. Agric. Water Manag. 249, 106766. doi:10.1016/j.agwat.2021.106766
Gupta, H. V., Kling, H., Yilmaz, K. K., and Martinez, G. F. (2009). Decomposition of the mean squared error and nse performance criteria: Implications for improving hydrological modelling. J. hydrology 377, 80–91. doi:10.1016/j.jhydrol.2009.08.003
Haygarth, P., Bilotta, G., Bol, R., Brazier, R., Butler, P., Freer, J., et al. (2006). Processes affecting transfer of sediment and colloids, with associated phosphorus, from intensively farmed grasslands: An overview of key issues. Hydrol. Process. 20, 4407–4413. doi:10.1002/hyp.6598
Houska, T. (2021). Algorithm guide. Avaliable At: https://github.com/thouska/spotpy/blob/master/docs/Algorithm_guide.md.
Houska, T., Kraft, P., Chamorro-Chavez, A., and Breuer, L. (2015). Spotting model parameters using a ready-made Python package. PloS one 10, e0145180. doi:10.1371/journal.pone.0145180
Karssenberg, D., Schmitz, O., Salamon, P., De Jong, K., and Bierkens, M. F. (2010). A software framework for construction of process-based stochastic spatio-temporal models and data assimilation. Environ. Model. Softw. 25, 489–502. doi:10.1016/j.envsoft.2009.10.004
Kronvang, B., Laubel, A., Larsen, S. E., and Friberg, N. (2003). Pesticides and heavy metals in Danish streambed sediment. Hydrobiologia 494, 93–101. doi:10.1023/a:1025441610434
Kyllmar, K., Forsberg, L. S., Andersson, S., and Martensson, K. (2014). Small agricultural monitoring catchments in Sweden representing environmental impact. Agric. Ecosyst. Environ. 198, 25–35. doi:10.1016/j.agee.2014.05.016
Lantmäteriet (2014). Produktbeskrivning: GSD-höjddata, grid 2+ lantmäteriet. Gävle, Sweden: GSD Geografiska Sverige Data.
Linefur, H., Norberg, L., Kyllmar, K., Andersson, S., and Blomberg, M. (2019). Växtnäringsförluster I små jordbruksdominerade avrinningsområden 2017/2018. Sveriges lantbruksuniversitet. Uppsala: Ekohydrologi, 161.
Madsen, H., Lawrence, D., Lang, M., Martinkova, M., and Kjeldsen, T. R. (2014). Review of trend analysis and climate change projections of extreme precipitation and floods in Europe. J. Hydrology 519, 3634–3650. doi:10.1016/j.jhydrol.2014.11.003
Mitasova, H., Hofierka, J., Zlocha, M., and Iverson, L. R. (1996). Modelling topographic potential for erosion and deposition using gis. Int. J. Geogr. Inf. Syst. 10, 629–641. doi:10.1080/02693799608902101
Mitasova, H., Mitas, L., and Brown, W. M. (2001). “Multiscale simulation of land use impact on soil erosion and deposition patterns,” in Selected papers from the 10th International Soil Conservation Organization Meeting held May 24–29, 1999, Purdue University and the USDA-ARS National Soil Erosion Research Laboratory.
Moore, I. D., and Burch, G. J. (1986). Physical basis of the length-slope factor in the universal soil loss equation. Soil Sci. Soc. Am. J. 50, 1294–1298. doi:10.2136/sssaj1986.03615995005000050042x
Norberg, L., Linefur, H., Andersson, S., and Blomberg, M. (2021). Växtnäringsförluster från åkermark 2019/2020. Uppsala: Sveriges lantbruksuniversitet Ekohydrologi, 172.
Ockenden, M. C., Hollaway, M. J., Beven, K. J., Collins, A., Evans, R., Falloon, P., et al. (2017). Major agricultural changes required to mitigate phosphorus losses under climate change. Nat. Commun. 8, 161–169. doi:10.1038/s41467-017-00232-0
Onnen, N., Heckrath, G., Stevens, A., Olsen, P., Greve, M. B., Pullens, J. W., et al. (2019). Distributed water erosion modelling at fine spatial resolution across Denmark. Geomorphology 342, 150–162. doi:10.1016/j.geomorph.2019.06.011
Øygarden, L., Kværner, J., and Jenssen, P. (1997). Soil erosion via preferential flow to drainage systems in clay soils. Geoderma 76, 65–86. doi:10.1016/s0016-7061(96)00099-7
Øygarden, L. (2003). Rill and gully development during an extreme winter runoff event in Norway. Catena 50, 217–242. doi:10.1016/s0341-8162(02)00138-8
Panagos, P., Borrelli, P., Poesen, J., Ballabio, C., Lugato, E., Meusburger, K., et al. (2015). The new assessment of soil loss by water erosion in Europe. Environ. Sci. policy 54, 438–447. doi:10.1016/j.envsci.2015.08.012
PCRaster (2013). PCRaster documentation. Avaliable At: https://svn.oss.deltares.nl/repos/openearthtools/trunk/programs/DRIHM/HBV/bin/hbv/openstreams_2013/software/pcraster/pcraster-4.0.0-beta-20131030_x86-64_lsbcc-4/doc/manual/operations.html.
Pionke, H. B., Gburek, W. J., and Sharpley, A. N. (2000). Critical source area controls on water quality in an agricultural watershed located in the chesapeake basin. Ecol. Eng. 14, 325–335. doi:10.1016/s0925-8574(99)00059-2
R Core Team (2020). R: A language and environment for statistical computing. Vienna, Austria: R Foundation for Statistical Computing. Avaliable At: https://www.R-project.org/.
Renard, K. G., Foster, G. R., Weesies, G. A., and Porter, J. P. (1991). Rusle: Revised universal soil loss equation. J. soil Water Conservation 46, 30–33.
Rieke-Zapp, D. H., and Nearing, M. A. (2005). Slope shape effects on erosion: A laboratory study. Soil Sci. Soc. Am. J. 69, 1463–1471. doi:10.2136/sssaj2005.0015
Römkens, M., Young, R., Poesen, J., McCool, D., El-Swaify, S., and Bradford, J. (1997). “Soil erodibility factor (K). Compilers),” in Predicting soil erosion by water: A guide to conservation planning with the revised universal soil loss equation (RUSLE). Editors K. G. Renard, G. R. Foster, G. A. Weesies, D. K. McCool, and D. C. Yoder (Washington, DC, USA: Agric. HB), 65–99.
Sandström, S., Futter, M. N., Kyllmar, K., Bishop, K., O'Connell, D. W., and Djodjic, F. (2020). Particulate phosphorus and suspended solids losses from small agricultural catchments: Links to stream and catchment characteristics. Sci. Total Environ. 711, 134616. doi:10.1016/j.scitotenv.2019.134616
Sharpley, A. N., Bergström, L., Aronsson, H., Bechmann, M., Bolster, C. H., Börling, K., et al. (2015). Future agriculture with minimized phosphorus losses to waters: Research needs and direction. AMBIO 44, 163–179. doi:10.1007/s13280-014-0612-x
Sidemo-Holm, W., Smith, H. G., and Brady, M. V. (2018). Improving agricultural pollution abatement through result-based payment schemes. Land Use Policy 77, 209–219. doi:10.1016/j.landusepol.2018.05.017
SMHI (2020). Vintern 2020 – ovanligt rekordrik vinter. Avaliable At: https://www.smhi.se/klimat/klimatet-da-och-nu/arets-vader/vintern-2020-ovanligt-rekordrik-vinter-1.155690.
Söderström, M., and Piikki, K. (2016). “Digital soil map - detailed mapping of soil texture in the topsoil of the arable land,” in Swedish University of Agricultural Sciences, Department of Soil and Environment 37, 342. Available at: file://storage.slu.se/Home$/sasm0007/Downloads/postek37_dsms.pdf.
Sörensen, R., Zinko, U., and Seibert, J. (2006). On the calculation of the topographic wetness index: Evaluation of different methods based on field observations. Hydrology Earth Syst. Sci. 10, 101–112. doi:10.5194/hess-10-101-2006
Statistics Sweden (2017). “Dränering av jordbruksmark 2016, slutlig statistik. Swedish board of Agriculture,” in Jordbruk, skogsbruk och fiske 41, 1701. Available at: https://jordbruksverket.se/download/18.1baa822517cd5f669364208f/1635761809412/JO41SM1701.pdf.
Thomas, I. A., Mellander, P. E., Murphy, P., Fenton, O., Shine, O., Djodjic, F., et al. (2016). A sub-field scale critical source area index for legacy phosphorus management using high resolution data. Agric. Ecosyst. Environ. 233, 238–252. doi:10.1016/j.agee.2016.09.012
Ulén, B., Bechmann, M., Øygarden, L., and Kyllmar, K. (2012). Soil erosion in nordic countries – future challenges and research needs. Acta Agric. Scand. Sect. B — Soil & Plant Sci. 62, 176–184. doi:10.1080/09064710.2012.712862
Ulén, B., and Jakobsson, C. (2005). Critical evaluation of measures to mitigate phosphorus losses from agricultural land to surface waters in Sweden. Sci. Total Environ. 344, 37–50. doi:10.1016/j.scitotenv.2005.02.004
Verheijen, F. G. A., Jones, R. J. A., Rickson, R. J., Smith, C. J., Bastos, A. C., Nunes, J. P., et al. (2012). Concise overview of European soil erosion research and evaluation. Acta Agric. Scand. Sect. B — Soil & Plant Sci. 62, 185–190. doi:10.1080/09064710.2012.697573
Villa, A., Djodjic, F., and Bergstrom, L. (2014). Soil dispersion tests combined with topographical information can describe field-scale sediment and phosphorus losses. Soil Use Manag. 30, 342–350. doi:10.1111/sum.12121
Villa Solís, A. (2014). Risk assessment of erosion and losses of particulate phosphorus. Uppsala: Swedish University of Agricultural Sciences.
Wischmeier, W. H., and Smith, D. D. (1978). Predicting rainfall erosion losses: A guide to conservation planning. Washington, DC: United States Department of Agriculture.
Xu, C. Y. (2000). Modelling the effects of climate change on water resources in Central Sweden. Water Resour. Manag. 14, 177–189. doi:10.1023/A:1026502114663
Keywords: high-resolution modelling, suspended solids, erosion, critical source areas (CSA), USPED, dynamic modelling
Citation: Sandström S, Markensten H, Futter MN, Kyllmar K, O’Connell DW, Bishop K and Djodjic F (2023) Distributed dynamic modelling of suspended sediment mobilization and transport from small agricultural catchments. Front. Environ. Sci. 11:1196048. doi: 10.3389/fenvs.2023.1196048
Received: 29 March 2023; Accepted: 31 July 2023;
Published: 11 August 2023.
Edited by:
Krishnaswamy Jayachandran, Florida International University, United StatesReviewed by:
Yang Yu, Beijing Forestry University, ChinaNazirul Mubin Zahari, Universiti Tenaga Nasional, Malaysia
Copyright © 2023 Sandström, Markensten, Futter, Kyllmar, O’Connell, Bishop and Djodjic. This is an open-access article distributed under the terms of the Creative Commons Attribution License (CC BY). The use, distribution or reproduction in other forums is permitted, provided the original author(s) and the copyright owner(s) are credited and that the original publication in this journal is cited, in accordance with accepted academic practice. No use, distribution or reproduction is permitted which does not comply with these terms.
*Correspondence: Sara Sandström, c2FyYS5zYW5kc3Ryb21Ac2x1LnNl