- 1Volcani Center, Institute of Soil, Water and Environmental Sciences, Agricultural Research Organization (ARO), Bet-Dagan, Israel
- 2Department of Geography, The Hebrew University of Jerusalem, Jerusalem, Israel
- 3Department of Biology and Environment, University of Haifa at Oranim, Kiryat Tivon, Israel
- 4Remote Sensing Research Centre, School of the Environment, University of Queensland, Brisbane, QLD, Australia
The Wildland-Urban Interface (WUI) is the area where houses and natural vegetation meet or intermingle. WUI areas are exposed to an increased hazard of wildfires and have significantly expanded worldwide in the past few decades. In this study, we developed a new empirical approach for mapping the WUI by generating a WUI index based on the juxtaposition among buildings, vegetation, and the fire history of the study area. We first calculated the percentage coverage of buildings and three different fuel typologies within circular moving windows with radii of 100, 250, and 500 m, and then acquired the fire history data between 2012 and 2021 for Israel and the West Bank (Palestinian Authority) from the VIIRS active fires remote sensing product. We defined the WUI as cells where the combination of vegetation cover and building cover had more VIIRS fire detections than expected by chance. To assess the effects of using broad vs. local scale parameterizations on resulting WUI maps, we repeated this process twice, first using national-scale data, and then separately in four distinct geographic regions. We assessed the congruence in the amounts and patterns of WUI in regions as mapped by information from these two analysis scales. We found that the WUI in Israel and the West Bank ranged from 0.5% to 1.7%, depending on fuel type and moving window radius. The scale of parameterization (national vs. regional) affected the WUI patterns only in one of the regions, whose characteristics differed markedly than the rest of the country. Our new method differs from existing WUI mapping methods as it is empirical and geographically flexible. These two traits allow it to robustly map the WUI in other countries with different settlement, fuel, climate and wildfire characteristics.
1 Introduction
The Wildland-Urban Interface (WUI) is the area where human settlements meet or intermingle with natural and semi-natural ecosystems (Radeloff et al., 2005). Within those areas a variety of human-environment conflicts take place (Bar-Massada et al., 2014), with wildfire being chief among them given the high hazard to human lives and property due to the proximity of flammable vegetation (Radeloff et al., 2018). The wildfire problem in the WUI is twofold, as on one hand the WUI is an area with greater fire hazard for humans, and on the other hand it is a source of fire hazard to surrounding natural habitats by the spread of wildfires due to human-caused ignitions (Radeloff et al., 2005).
There are many operative definitions to the WUI (Bento-Gonçalves and Vieira, 2020), and different countries delineate the WUI according to various parameters (Table 1). Some define the WUI according to the area (forest and urban) exposed to wildfire hazards, while others define it according to the density of houses near flammable vegetation (Intini et al., 2020). Nevertheless, all WUI definitions share three components: the presence of human property, the presence of wildland vegetation, and the degree of spatial juxtaposition between the human component and the surrounding vegetative component.
The presence of human property refers to structures (e.g., houses, infrastructures, industries) or population (Theobald and Romme, 2007), while the presence of wildland vegetation usually focuses on hazardous fuels (Modugno et al., 2016). The spatial juxtaposition, in turn, is often determined by the distance between the anthropogenic and vegetated parts of the landscape and represents the potential likelihood of wildfire spread among different landcover types, given the two main types of spread: direct exposure to the fire front (i.e., short-distance exposure), and showering embers (long distance exposure) (Beverly et al., 2010). Previous studies described the former according to an estimated “home ignition zone”, i.e., the distance in which heat transfer can ignite a structure (Cohen, 2008), while the latter reflects the distance a firebrand can fly ahead of a fire front (Radeloff et al., 2005) or, more figuratively, as “the distance a golf ball will fly off the porch” (Stewart et al., 2007). The considerable variation among WUI typologies stems from the different approaches to defining or quantifying these distances.
In general, WUI typologies may be qualitative or quantitative. For example, the European Commission’s WARM project (Wildland-Urban Area Fire Risk Management Project) has a qualitative approach to the WUI definition, based on multiple combinations of housing and vegetation patterns. The WARM project classifies WUI regions into five categories based on their spatial pattern: sparse intermix, clustered intermix, urban, clustered urban interface, and occluded urban (González-Cabán, 2008). In contrast, the French Forest Orientation Law of 9 July 2002, utilizes a quantitative approach for mapping the WUI, defining it as an area within a fixed distance threshold of 100 m around each house which is located at a distance of fewer than 200 m from forests or scrublands (Lampin-Maillet et al., 2010). The US Federal Register approach distinguishes between two primary sources of fire exposure to buildings: direct exposure to flame fronts (Intermix WUI) and exposure to flaming embers from distant fuel sources (Interface WUI). In the former, homes are scattered among vegetation, whereas in the latter, homes are grouped and adjacent to areas with dense vegetation. Quantitatively, the Intermix WUI is any area which contains more than 6.17 housing units/km2 in an area dominated by (more than 50%) wildland vegetation; The Interface WUI is an area that has the same housing density as intermix but has less than 50% wildland vegetation, yet is within 1.5 miles (2.4 km) of an area that is densely vegetated (more than 75% flammable vegetation) and is larger than 5 km2. These parameters and thresholds were set according to US wildfire conditions, and it is possible to alter them elsewhere. Typically, WUI mapping methods which are based on a priori threshold conditions are adjusted for each country’s unique landscape characteristics of settlement patterns, vegetation characteristics, and the interfaces between them. In recent study the ember distance threshold was reduced from 2.4 km to 600 m to better reflect management considerations related to building exposure assessments in the EU (Bar-Massada et al., 2023).
However, the fixed threshold conditions limit the application of the mapping methods for areas with different pyrogeographic and settlement characteristics. Where land cover patterns and fire regimes differ, flexible or adaptive parameterizations might be more suited than consistent parameterizations (Bar-Massada et al., 2023), as they may account for geographic differences in wildfire exposure to human settlements. Moreover, the choice of mapping parameters and threshold conditions typically relies on theoretical knowledge and is often not based on empirical knowledge of wildfire exposure. This is problematic, as it may limit the utility of WUI maps for robust fire exposure assessments because their estimation of danger may be inaccurate if parameter choices do not align with the actual drivers of fire occurrence and spread (Caggiano et al., 2020).
In order to reduce the uncertainty introduced into WUI mapping by fixed parameterizations and spatially uniform threshold conditions, we developed and tested a novel WUI mapping approach. Our aims were 1. To develop a new, empirical, and geographically variable WUI mapping method. 2. To assess and demonstrate the importance of using different WUI parameterizations across regions with different settlement and fire characteristics. Our method is based on building locations (Bar Massada et al., 2013) and relies on the juxtaposition among buildings, flammable vegetation, and the actual fire history obtained from remotely sensed sources. Analyzing the actual fire history is a novel contribution that distinguishes our approach from most previous approaches for mapping the WUI. Therefore, our method does not rely on a priori fixed threshold choices, which enables its effective application in areas with different patterns of settlements and vegetative fuels as well as varying fire regimes.
2 Materials and methods
2.1 Study area
Our study area, covering the State of Israel and the West Bank (Palestinian Authority), extends over 28,000 km2 of diverse land in terms of precipitation amount (20–1,200 mm per year) and vegetation types (Misgav, 2000). Israel’s natural vegetation covers a wide range, from desert plants, through Mediterranean herbaceous vegetation, “batha” of semi-shrubs with no trees, maquis woodlands (at varying height and density levels, as a function of grazing and wildfire disturbances), and planted (mostly coniferous) forests (Danin, 1988).
As a consequence of a centralized historical planning policy (Shachar, 1998; Alterman, 2001; Orenstein and Hamburg, 2009; Feitelson, 2018), multiple types of interfaces have evolved between settlements and wildland ecosystems, differing from region to region. Settlements, industries, infrastructure, and agricultural lands meet or overlap with planted coniferous forests, Mediterranean forests, woodlands, scrub, and desert. In this study we divided the study area into eight regions, roughly based on their dominant natural vegetation formations: Golan, Galilee-Carmel-Hermon (GCH), Valleys, West Bank (WB), Coastal, Jerusalem Hills (JH), Southern Coastal Plain (SCP), and Desert (Figure 1). Conceptually, most of the WUI in the study area is of the Interface WUI type due to the historical tendency in the region, based on land ownership patterns, to develop entire settlements in wildland areas, and the relative scarcity of isolated buildings. However, in areas where villages and towns were not centrally planned (often in older settlements), and consequently tend to be characterized by irregular spatial patterns, intermix WUI may also be found.
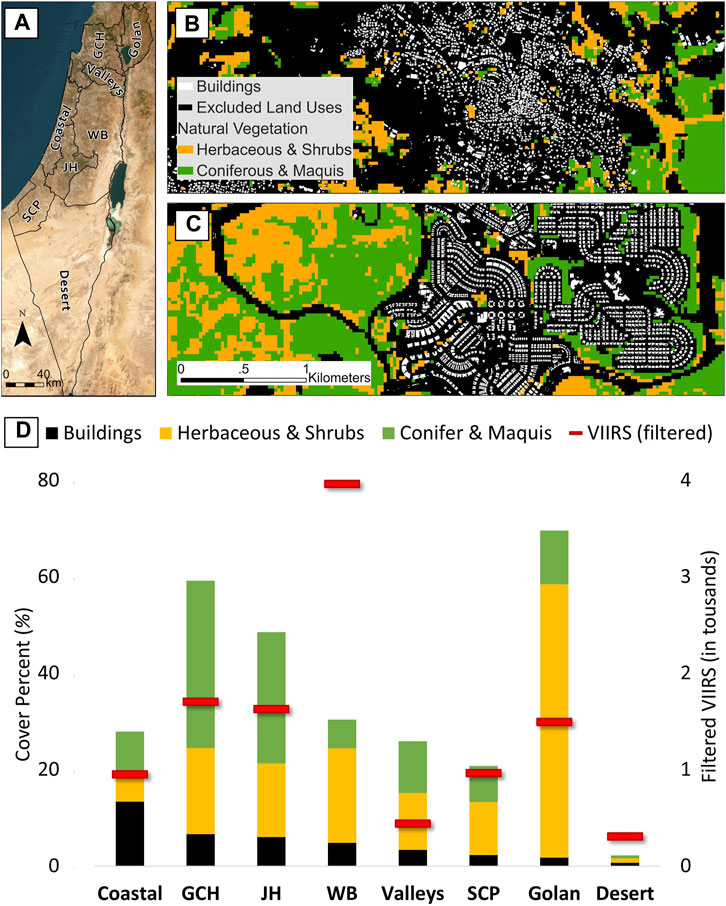
FIGURE 1. (A) The study area, divided by regions. (B) Buildings and vegetation around the town of I’billin, Galil-Carmel-Hermon (GCH) region*. (C) Buildings and natural vegetation around the city of Modi’in-Maccabim-Re’ut, Jerusalem Hills (JH) region*. (D) Land cover composition of the study area. The right-hand Y-axis represents the number of VIIRS active fires (after filtering; depicted by red lines). *The area in black in (B,C) represents excluded land cover types: built-up (we used building locations instead), agriculture, disturbed, quarries, and low vegetation cover.
The wildfire season in the study area coincides with the dry season from May to November. The peak months for large wildfires are typically in transition seasons (spring and autumn), when hot and dry meteorological conditions are more common (Levin and Saaroni, 1999; Kutiel, 2012). The overwhelming majority of fires in the study area are caused by human activities, intentionally or accidentally. The largest forest and maquis wildfire incidents in Israel’s history were the September 1989 Carmel Park (400 ha), the July 1995 Sha’ar Ha’Guy (1,300 ha), the December 2010 Carmel Park (2,200 ha) which also led to the death of 44 people (Levin and Heimowitz, 2012), and the August 2021 Jerusalem Hills wildfire which consumed about 11 km2. The November 2016 “Wildfire Wave”, namely, multiple wildfires that took place over a few days in different regions, consumed more than 41 km2 overall (The State Comptroller Report, 2018), and the May 2019 fire of Mevo Modi’in was the first fire in Israel’s history to burn an entire village.
2.2 Datasets
The mapping of WUI areas based on the juxtaposition between buildings and flammable vegetation, while accounting for the fire history of the study area, required datasets from multiple different sources as listed below (and summarized in Table 2 and Figure 2).
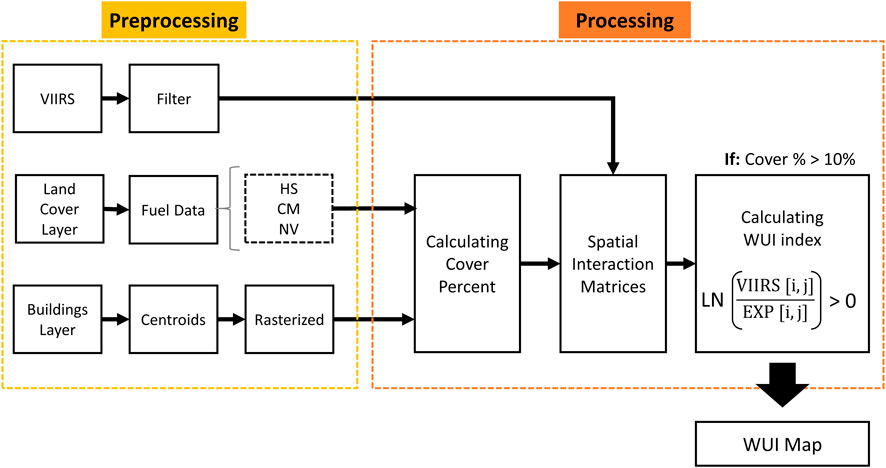
FIGURE 2. The mapping method workflow. We repeated this workflow nine times: for three types of fuel [Herbaceous and Shrubs (HS), Conifer and Maquis (CM), and all Natural Vegetation (NV)] at three radii: 100, 250, and 500 m.
2.2.1 Vegetation types
Land cover information was derived from a thematic raster layer, updated to 2018, with a minimum mapping unit of 25 m, created by Hama’arag (The National Program for Assessing the State of Nature in Israel). The layer defines several types of vegetation which may serve as fuel during a wildfire (Figures 1B, C). We created two categories of fuel types, which differ from each other mostly based on their height: Herbaceous and Shrubs (HS) and Coniferous and Maquis (CM). We also united HS and CM to form a layer of all fuels (Natural Vegetation; NV). Bare soil, quarries, and agriculture areas were not included in the analysis and in our subsequent WUI map since the first two are less flammable, and vegetation at agricultural lands is well-managed in most of Israel.
2.2.2 Building footprints
The buildings dataset was a vector polygonal layer, created by The Survey of Israel, consisting of around 1.9 million individual buildings, and updated to 2021. The layer represents an anthropogenic presence and includes all reported structures in the study area (besides some military and security facilities), from individual buildings to sheds in parking lots (Figures 1B, C). Since the minimum mapping unit of the vegetation layer we had was 25 m, and to minimize information loss, we created a layer of building centroids and then rasterized them to a 25-m resolution where each cell contains “1” if it contained a building centroid and “0” otherwise.
2.2.3 Fire activity
Israel has a new unified wildfire database which contains all fire incidents greater than 10 ha (for the years 2015–2022; Guk et al., 2023). Yet in order to allow the replication of our approach elsewhere (where such data do not exist), we used remote sensing data to reconstruct the fire history of our study area. The Visible Infrared Imaging Radiometer Suite (VIIRS) for active fires and thermal anomalies, onboard Soumi-NPP and NOAA-20, was suitable for this purpose. The VIIRS database (Csiszar et al., 2014; Schroeder et al., 2014) was created by NASA’s Fire Information for Resource Management System (FIRMS) and is distributed as a point layer representing a pixel center. The VIIRS database was chosen over the alternative MODIS active fires database because of the landscape complexity of the study area, and since its spatial resolution could be downscaled to 375 m depending on the scan angle. The dataset was collected by the Soumi-NPP satellite since January 2012, and since mid-2018 the NOAA-20 satellite became operational, collecting information in addition (50 min later) to the Soumi-NPP satellite.
The VIIRS database, from January 2012 to December 2021, consisted of 21,966 pixels (at a spatial resolution of 25 m) of active fires and thermal anomalies within our study area. We used three GIS layers to remove erroneous fire detections (false positives) from the VIIRS database, which mostly referred to quarries, industrial areas, and solar farms. The information about quarries was obtained from the National Master Plan (NOP #14/B) for mining and quarrying. The NOP covers the State of Israel and does not include information from the West Bank. The source of industrial areas is the Ministry of Economy and Industry. The layer was obtained from the “Hatzav” project, for land transportation planning, of the Ministry of Transportation (https://geo.mot.gov.il/; accessed on 25/07/2023). The information about solar farms was obtained from Hama’arag thematic layer (2018).
We also detected specific areas with a human source emitting high heat, characterized by consistently high temporal frequency of VIIRS active fire detections over months and years. For this purpose, we created a grid with one square kilometer cells and counted for how many months each cell “burned” over a 10-year period (120 months). We identified cells containing false positive fire detections if they 'burned' in more than 12 months. We then removed all VIIRS pixels located in these grid cells (Figure 3).
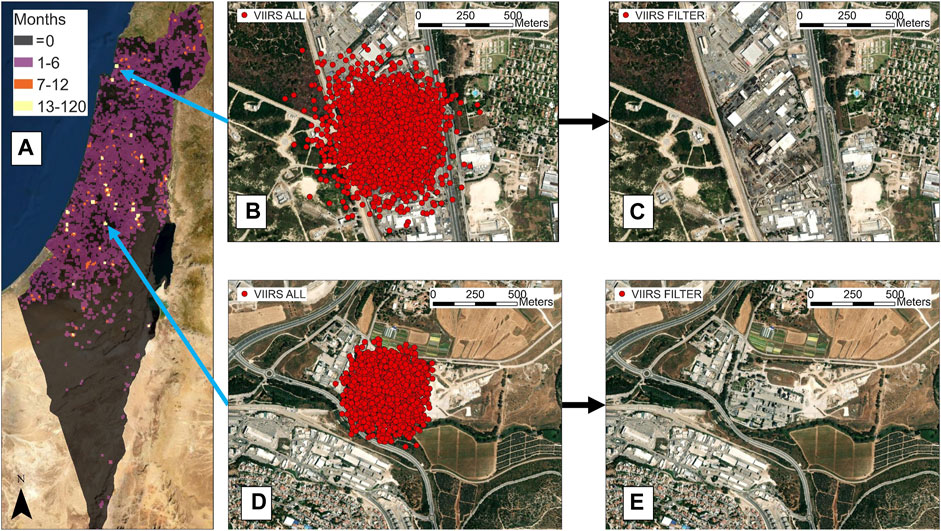
FIGURE 3. (A) National map of VIIRS active fires pixel concentrations (pre-filtering), counted in months. The yellow locations are defined as areas of false detections (B) Masarik industrial area before filtering (C) Masarik industrial area after filtering (D) Bet-Shemesh industrial area before filtering (E) Bet-Shemesh after filtering.
The filtered dataset comprised 11,585 pixels, 47% of the original (21,966). The original dataset had a concentration of false positive identification of fires in Built-Up and Agriculture land cover classes, where about 70% of all pixels were found. After filtering, we found most of the VIIRS detections in Agriculture and HS land cover classes, representing about 68% of the filtered VIIRS database. The filtering process did not affect the CM class significantly, with 1,613 identifications before the filtering and 1,383 identifications after (Supplementary Figure S1A). The filtering process at Built-Up and Disturbed classes corresponded with industrial areas, photovoltaic farms, and quarries. These land uses produce heat due to human activity, i.e., their detection by VIIRS implies a false positive identification and not actual fires.
The filtered database exhibited a similar temporal trend to the original database (Supplementary Figure S1B), with most fires taking place during the dry season (May to August with 61% of the dataset and October to November with 18% of the dataset). This trend is also known from the literature and was found in different fire databases, from different periods, from 1987 to date (Levin and Saaroni, 1999; Levin and Heimowitz, 2012; Turco et al., 2017; Guk et al., 2023), which confirms that the removal of those active fires was mainly of false positive detections.
2.3 The WUI mapping process
We started by calculating the percent cover of buildings and fuel (HS, CN, and NV classes) within circular moving windows of three different radii (100, 250, and 500 m) around each 25 m pixel in our study area. We then created a regular grid of points for sampling purposes. To eliminate spatial overlap among adjacent sampling areas, we created a different grid for each moving window radius, where the distance between adjacent sampling points was set to twice the size of the radius. For example, for a 100 m radius, we used a grid with a 200 m distance between sampling points.
Using the sampling grids, we extracted the percent cover of buildings and flammable vegetation from the pixel that intersected each point, and the presence of VIIRS active fires inside it. Our sampling database therefore included, for each sampling location, the percent cover of buildings and of flammable vegetation, and the existence or nonexistence of VIIRS fire detections (binary value). We reclassified the percent cover values according to percentiles of ten (values at range 0%–10% reclassified as 0, 10%–20% reclassified as 1, 20%–30% reclassified as 2, etc.). From these cover classes, we created spatial interaction matrices with building classes as rows (i) and fuel classes as columns (j). In one matrix we counted the frequency of VIIRS active fires across all years (the observed matrix), and in the second matrix (the expected matrix) we calculated the expected by-chance value, assuming a uniform distribution.
Finally, we generated a Wildland-Urban Interface (WUI) index to evaluate the relationship among fires, buildings, and fuels. This index was calculated by dividing each cell in the observed matrix by its corresponding cell in the expected matrix and applying a natural logarithm to this ratio, as follows:
Where the WUI index stands for the Wildland-Urban Interface index, the VIIRS[] matrix represents the filtered VIIRS frequency across all years for a given combination of the i-th building density class and the j-th fuel density class, and the EXP[] matrix represents the expected by-chance value for the corresponding combination of i and j derived from the expected matrix. We applied a logarithmic transformation to the ratio to reduce the impact of extreme values on the resulting distribution of the WUI index. Positive values represent areas where the hazard of fires is greater than expected by chance (with higher values denoting more extreme potential exposure), while negative values represent areas with low fire exposure. We omitted cells where the percentage cover of buildings and fuels were greater than 10% (to eliminate mostly uninhabited wildlands from the WUI map).
We repeated this process nine times using all possible combinations of buildings and fuel types (vegetation types of HS, CM, NV), at three moving window radii (100, 250, and 500 m). Then, we quantified the aerial extent of the WUI across our study area and in different regions and the number of buildings in it, by converting the resulting WUI raster to polygons and intersecting the (buildings) centroids with the WUI polygons in each region.
2.4 Analyzing the effect of parametrization on the resulting WUI map
We used the inherent variation in environmental conditions between the regions in our study area (Figure 1D) to assess the importance of different empirical parameterizations on the resulting WUI maps.
For this purpose, we examined, in each region, the spatial correspondence between a WUI map created using national parameters (i.e., matrices of percentage buildings and fuels were derived from the entire study area) and a map created using regional parameters only. We conducted this comparison in four regions which had high WUI cover. For the sake of simplicity, we converted the continuous WUI maps to binary form based on WUI index > 0 and used only the maps that were based on the NV fuel type and a 100 m moving window radius. Finally, we compared the spatial agreement between the WUI map based on national parameters and WUI maps based on regional parameterizations using a confusion matrix and Cohen’s Kappa index of agreement (Cohen, 1960; Congalton, 1983).
3 Results
3.1 WUI extent and number of buildings
We found that WUI extent and the number of buildings in the WUI varied considerably among regions, moving-window radii, and fuel type classifications. Generally, we detected larger WUI areas and more buildings in the WUI when using the combined fuel category (NV, a unification of HS and CM) and the largest analysis radius, 500 m (Figures 4, 5). For example, using NV and a 100 m radius, the WUI constituted about 1% (264 km2) of the study area, though its amount varied considerably across regions from 0.2% in the Desert region to 6.6% in the GCH region. Increasing the calculation radius to 500 m increased the WUI extent to 1.7% (474.3 km2) with a much wider regional distribution from 0.2% in the Desert to 12.4% in GCH (Table 3). Most buildings located within WUI areas were located in the WB and GCH regions, and a 500 m mapping radius identified more buildings in the WUI than the 100 m radius (Table 4). In contrast to the positive effect of calculation radius on the number of WUI buildings in regions with high WUI cover, the Desert region, mainly characterized by small settlements and bare soil, demonstrated an opposite pattern. The increase of the calculation radius reduced the number of buildings but did not affect the amount of WUI area in the desert (Table 3).
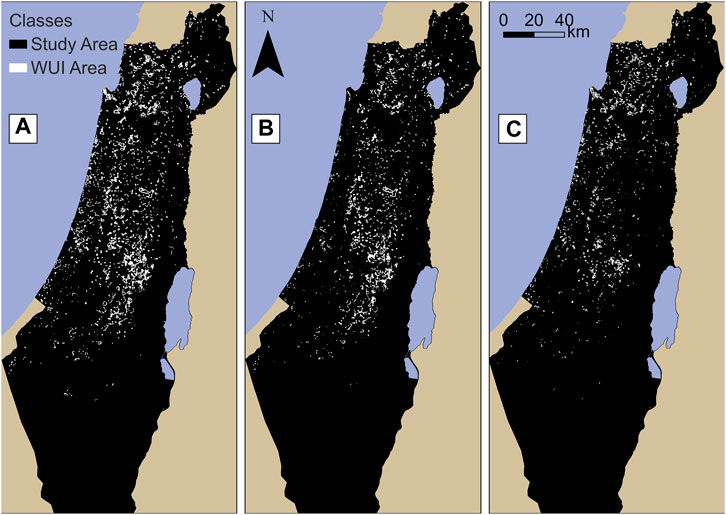
FIGURE 4. Binary WUI maps (WUI index > 0) of the study area based on a 500 m analysis radius for three vegetation types: (A) all Natural Vegetation (B) Herbaceous and Shrubs (C) Conifer and Maquis.
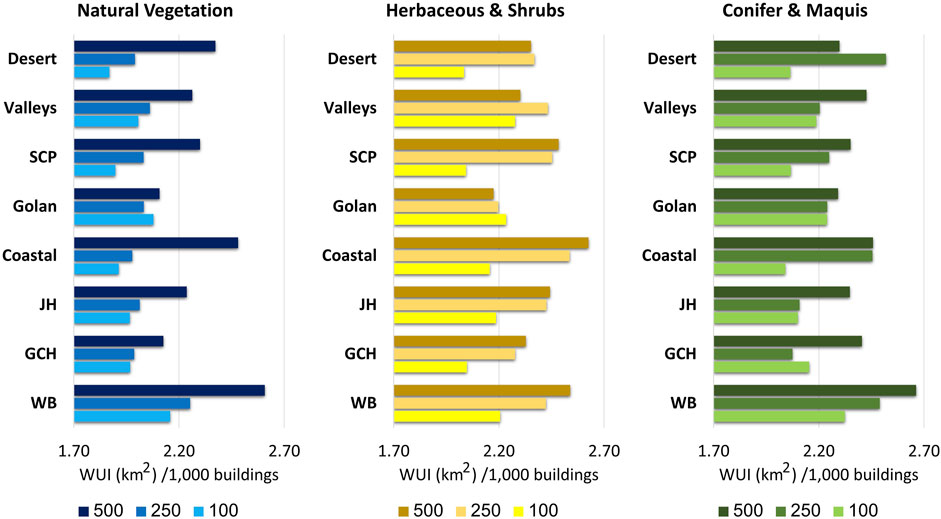
FIGURE 5. WUI area (in km2) relative to the number of buildings (in thousands), according to three types of vegetation (all natural vegetation, Herbaceous and Shrubs, Conifer and Maquis) at three radii.
3.2 WUI development patterns and fire exposure
From a local perspective, we found that the radius size of the analysis window had considerable effects on the mapping results and that in different regions of the study area, a different radius was found to be more appropriate for mapping the WUI. For example, the city of Ramat-Gan is located in the center of the Tel Aviv Metropolitan area and is characterized by dense built-up areas. We found that using a 100 m radius resulted in the inclusion of small in-town urban parks in the WUI, while increasing the radius length to 500 m does not decrease building density, so only city edges were defined as WUI (Figures 6A, B).
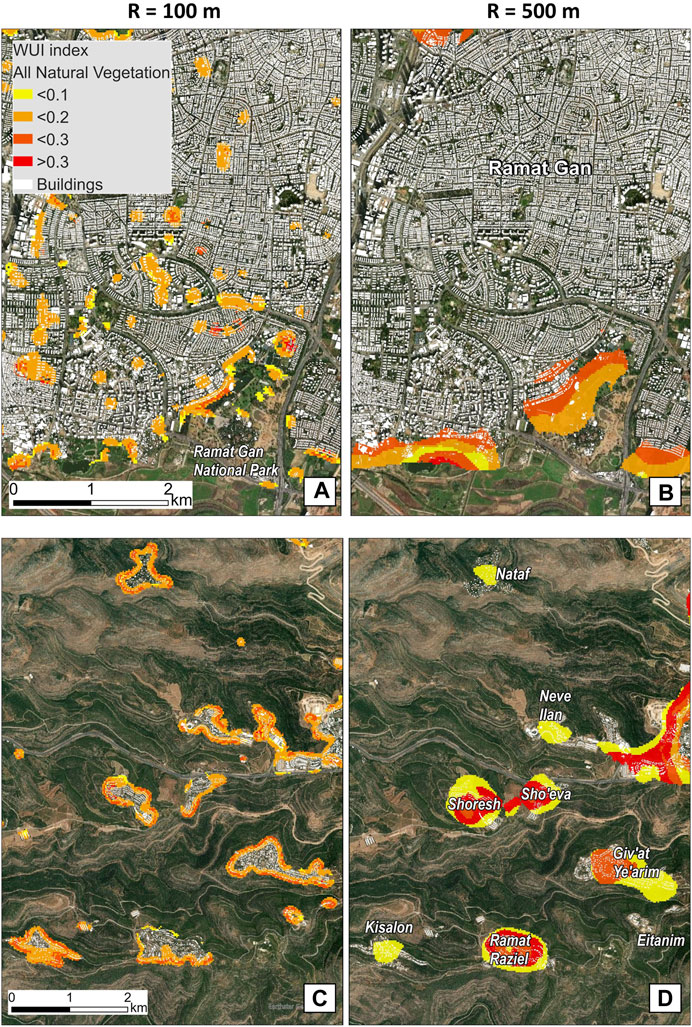
FIGURE 6. The effect of radius size on the spatial patterns of the WUI index and its values* in two areas with different landscapes characteristics. (A,B) depict the WUI index at the city of Ramat Gan. A 100 m radius (left) defined urban parks as WUI, while a 500 m radius (right) defined only the city edges as WUI. (C,D) depict the WUI index at part of the Jerusalem Hills region. A 100 m radius (left) identified settlement edges, while a 500 m radius (right) marked entire settlements as WUI and often missed their edges. *WUI index values, derived from the equation ln(VIIRS[i,j]/EXP[i,j]), represent normalized fire exposure in any combination of buildings (i) and natural vegetation (j).
In contrast, in the mountainous JH region, which is characterized by scattered settlement patterns nestled within natural vegetation, a 100 m radius identified only settlement edges as WUI, whereas a 500 m radius often defined entire settlements as WUI but excluded parts of the edges of larger settlements, and even omitted entire small settlements. For example, Eitanim hospital, which is situated on a mountaintop in the Jerusalem Forest, and which was exposed to a wildfire recently, comprises a sparse building cluster surrounded by natural vegetation. Increasing the radius from 100 to 500 m caused building cover to drop below 10%, and subsequently to the exclusion of this settlement from the WUI despite its proven exposure to wildfire (Figures 6C, D).
In general, our mapping approach was able to distinguish between fire exposure levels since the values of the WUI index are continuous, with higher values reflecting a larger deviation in the occurrence of fires compared to the null expectation. We found that the analysis radius affected the distribution of WUI index, where increasing the radius produced more WUI areas with extreme values (larger than 0.3, i.e., ∼35% more fires than expected by chance). Considering vegetation types, we found that most of CM-WUI areas tended to be characterized by relatively lower index values (less than 0.1), i.e., low exposure levels. HS-WUI areas demonstrated the opposite trend, with more areas characterized by relatively medium and high index values (larger than 0.2), which correspond with relatively high exposure levels compared to other areas (Figure 7).
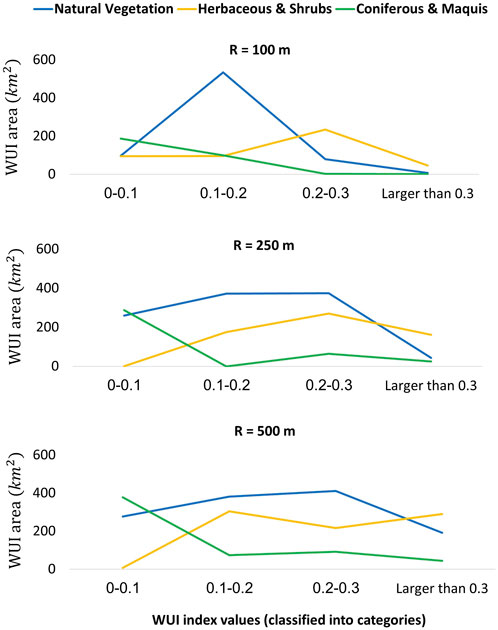
FIGURE 7. The distribution of the WUI index (calculated with national parameters) by area (km2), according to three vegetation types in three different analysis radii.
3.3 Effects of parametrization in WUI mapping
We found that the regions WB, GCH, JH, Coastal, and Golan had the largest WUI areas, both numerically and percentage wise. We omitted the Coastal region from the subsequent analysis since we used the 100 m radius, which added many urban parks and small gardens into the WUI (see previous section). In other regions, we found that a mapping process based on a national-scale parameterization identified a little more WUI area in each region compared to the mapping process that used regional parameterization. The GCH and JH demonstrated an increase of 0.1% between regional and national parameters (6.7%–6.8% and 4.7%–4.8%, respectively). The WB demonstrated an increase of 0.8% between regional and national parameters (4%–4.8%), while the Golan demonstrated an increase of 1.4% (Figure 8; Supplementary Table S1). At the same time, we found that the regions WB, GCH, and JH had relatively similar spatial patterns of WUI in both parameterizations (national and regional), as their Kappa coefficients were greater than 0.9 (Figure 8). In contrast, the Golan region, characterized by sparsely scattered small settlements nestled within natural vegetation dominated by grasslands, had a large spatial difference in WUI patterns between the national and regional WUI maps (Kappa = 0.51, indicating a weak level of agreement). This difference corresponded with a high ratio of fuel cover to building cover, which was much higher in the sparsely populated Golan than in the other, more populous regions (Figure 8).
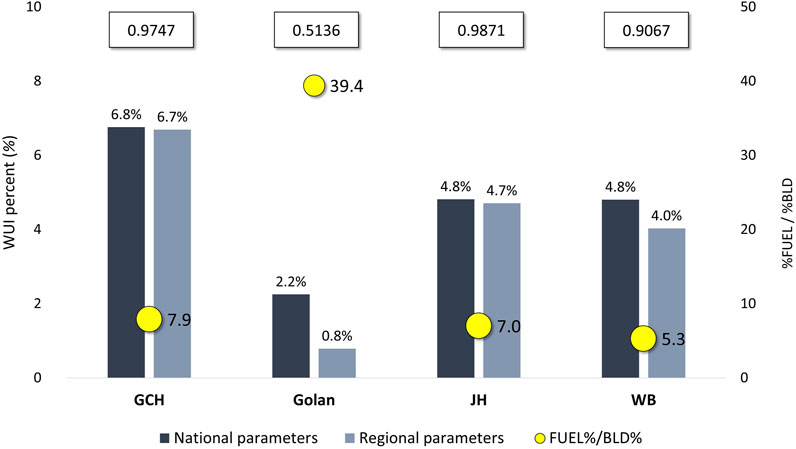
FIGURE 8. Comparison between WUI maps at four regions, using national vs. regional parameters. The bars represent the percent of WUI according to the national or regional parameters. The yellow points depict the ratio between the cover percent of natural vegetation and buildings (%Fuel/%Bld; e.g., the cover percent of natural vegetation in the Golan is 39.4 greater than the cover percent of buildings). The numbers at the top of the figure represent the Kappa agreement between the national WUI map and the regional WUI map considering all natural vegetation, based on a 100 m analysis radius.
4 Discussion
The WUI mapping method we developed was based on the juxtaposition of buildings, flammable vegetation, and the fire history of the study area. Using fire history is novel compared to most existing WUI mapping approaches, which rely on a priori thresholds and parameters which do not necessarily reflect variations in WUI patterns or the exposure of WUI areas to actual fire events (Radeloff et al., 2005; Lampin-Maillet et al., 2010; Modugno et al., 2016). We also analyzed, for the first time, the role of geographical variations in WUI parameterizations and found that they can have considerable effects on resulting WUI maps.
Our results indicated that both landscape and settlement patterns, which vary across regions, affected the extent and spatial pattern of the WUI across Israel and the WB (Tables 3, 4; Figure 5). This variation may not be accurately reflected in WUI patterns when the parameterization of the national WUI map does not correspond with the characteristics of unique regions, such as the Golan in our study area. In contrast, in regions whose characteristics correspond well with the average conditions across the entire study area, the WUI obtained with regional parameters is similar in most cases to the WUI obtained from national parameters (Figure 8). This finding emphasizes the importance of mapping the WUI in heterogeneous regions using geographically varying parameters rather than consistent parameterizations, as these may lead to an inconsistent representation of fire exposure in the WUI (Bar-Massada et al., 2023).
A specific novelty of our WUI mapping approach is that it is modular and its parameterization may be easily changed by the users for different regions: the buffer radius, the threshold value of the WUI index, the source of the wildfire history data, and the type of flammable vegetation to be included. Our mapping approach, therefore, may serve as a decision-making tool for policymakers and land managers in operations needed for reducing wildfire hazards.
In this study, we also devised a way to improve the estimation of wildfire exposure in the WUI by accounting for the study area’s fire history (derived from remote sensing). In a recent study which mapped WUI in California, the history of wildfires was used to determine the threshold of vegetation cover percent (Li et al., 2022). Our approach was different, as it included both vegetation and building cover percentages within different radii. We used the information on fire activity from the VIIRS active fire archive to identify combinations of building and vegetation cover that tend to be associated with extreme fire exposure. To qualitatively demonstrate the validity of our approach, we highlight the case of Mevo Modi’in, a settlement which was completely burnt in a wildfire in May 2019 (the only such case in the history of the study area). As shown in Figure 9, the entire settlement was identified as located within a WUI area, with many parts of it having index values above 0.2, hence it was highly exposed to wildfire hazard. Yet this specific case also highlights the importance of selecting the proper analysis parameters: the entire village was identified as WUI only when a 100 m buffer radius was used, whereas a 500 m radius failed to identify it as WUI.
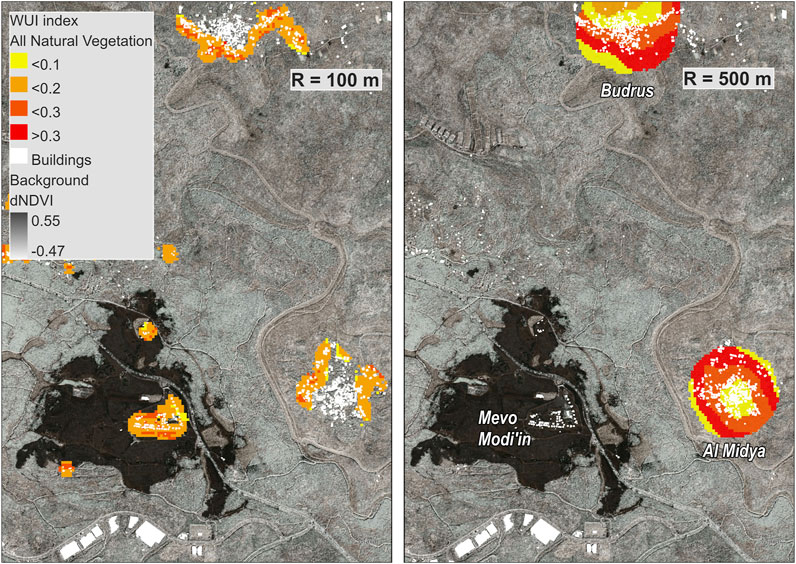
FIGURE 9. On 23 May 2019, a wildfire consumed all houses in the village of Mevo Modi’in at the Jerusalem Hills (JH) region. The background (dNDVI) represents the difference in NDVI values between 22 May 2019, and 28 May 2019 (based on PlanetScope satellite images), where dark areas indicate a decrease in photosynthetic activity, i.e., the burn scar from 23 May 2019. The WUI index is displayed as a color overlay, based on a calculation radius of 100 m (left) and 500 m (right).
We found that the regional heterogeneity of landscapes in the study area affected the extent and spatial distribution of the WUI in them. The WB, GCH, and JH regions are characterized by mostly scattered, low-density settlement patterns (Orenstein and Hamburg, 2009) with intermediate-to-high cover of natural vegetation (Figure 1D). Those regions had more WUI areas and buildings at fire hazard (Tables 3, 4) than regions with different landscape characteristics. This result is consistent with previous studies indicating those areas (dispersed buildings in a forested landscape), characterized with WUI zones in direct contact with forest continuity, had more fires and larger burned areas (Badia et al., 2019). In contrast, though the Desert, SCP and Valleys were also characterized by scattered settlement patterns, the amount of WUI areas in them was low, due to their somewhat less-flammable dominant vegetation types and the low overall cover of flammable vegetation. The SCP and Valleys regions are mostly agricultural, and there is not enough vegetation in the Desert to support fire spread.
From a local perspective, we found that the cover percent of buildings interacts with the analysis radius to affect the WUI pattern, and this interaction manifests differently across landscapes with different urbanization levels. An urban landscape requires a larger analysis radius to identify the WUI in a correct manner (Carlson et al., 2022) compared to landscapes with scattered settlements dominated by natural vegetation (Figures 6, 9). In the former, there is need to use larger radii to exclude urban parks and isolated vegetated patches that are unlikely to support considerable fire activity (e.g., the case of Ramat Gan city in the results section), whereas in the latter smaller radii are needed to correctly identify small settlements engulfed in flammable vegetation which are highly exposed to wildfire spread from surrounding wildlands (the example of Mevo Modi’in, above).
The mapping method presented in this study builds on empirical active fire data rather than a priori threshold conditions. Nevertheless, alternative sources of fires can be used for this purpose: ignition points, burned areas, or fire-spread simulation models (Bar Massada et al., 2009). Using ignition points as the fire data will produce WUI maps that are biased toward areas exposed to small fires, as most ignition events result in small burned areas. Additionally, precise data on ignition locations is unavailable in many countries, or, collected inconsistently by different land management agencies (Guk et al., 2023). A different approach would be to use data on burned areas (or fire perimeters) from available remote sensing products, e.g., MODIS (Roy et al., 2008). We expect that such data will yield generally similar WUI maps to those created using our approach, with differences stemming from the tendency of active fire datasets to mostly consist of larger fires (in terms of intensity, size, and duration), compared to burned area products that are typically able to retrospectively detect much smaller fires based on their burn scars. Therefore, an approach using burned areas as the source of fire history might be more comprehensive than using active fires. Either way, using remote sensing products of fire history will produce WUI maps biased towards areas subjected to larger and more intense fires, compared to maps generated using ignition points. Finally, a completely different approach for obtaining a fire history for WUI mapping would be to recreate it using fire simulation models such as FlamMap or FARSITE (Bar Massada et al., 2009). This approach offers considerable flexibility in accounting for different ignition locations and weather conditions, and can generate synthetic fire regimes even in regions with little fire activity under current conditions, but which is expected to worsen under climate change (Chuvieco et al., 2023). The limitations of this approach include the uncertainties associated with simulation modeling when predicting a large set of plausible wildfire footprints in vast landscapes under different weather and suppression scenarios, as well as the complexity of its application relative to the simplicity and availability of recent wildfire data (Finney et al., 2011; Salis et al., 2016). Using fire models to generate WUI maps may therefore highlight areas with very little contemporary wildfire exposure as suitable WUI locations.
In general, the inherent limitation of empirical WUI mapping approaches like ours is their reliance on the contemporary or near-past fire history of the study area. This is suitable under the implicit assumption that the fire regime will not change in the near future. However, this assumption may not hold due to climate change (Turco et al., 2017; Lestienne et al., 2022) or local anthropogenic activities such as fuel management, reforestation, or improved fire prevention and suppression capabilities.
As with all other WUI mapping methods, our new method is also subject to several limitations stemming from the particularities of its formative datasets. The land cover layer we used was a static thematic layer that does not reflect structure and fuel load. Since wildfires in the study area tend to occur under certain weather conditions in certain seasons, the hazard of wildfires in the WUI is also influenced by dead and live fuel moisture conditions, which affects fire intensity (Yebra et al., 2013; Gale and Cary, 2022). Also, Classification errors in the initial dataset may propagate into the resulting WUI maps (Ramat-Gan national park as an example, Figures 6A, B). Lastly, our estimate of building exposure in the WUI does not reflect the “home ignition zone” (Cohen, 2008), as our landcover dataset does not contain information on urban vegetation and building materials. This results in a general overestimation of fire exposure to buildings, as the presence of fire-proof materials cannot be accounted for. Finally, our method (like most previous ones) focuses on fire exposure but ignores fire behavior. To make the map more informative for fire risk assessment at the local scale, one would need to quantify vegetation density (Safford et al., 2012), height (Gale and Cary, 2022), and moisture content (Yebra et al., 2013) in WUI areas, in order to identify specific WUI areas exposed to high wildfire danger.
Data availability statement
Publicly available datasets were analyzed in this study. This data can be found here: VIIRS active fire: https://firms.modaps.eosdis.nasa.gov/download/ Land Cover Map: https://hamaarag.org.il/. The building database is accessible (financial cost) via emailing the Survey of Israel at this email: Y3VzdG9tZXJzZXJ2aWNlQG1hcGkuZ292Lmls.
Author contributions
IT, AB-M, and NL contributed to the conception and design of the study. IT created and organized the database. IT, AB-M, and NL performed statistical analyses. IT wrote the first draft of the manuscript and created the illustrations. AB-M and NL reviewed and edited. All authors contributed to the article and approved the submitted version.
Funding
This study was funded by the European Union’s Horizon 2020 Research and Innovation Programme under the Grant Agreement no. 101003890, FirEUrisk.
Acknowledgments
IT thanks the David Amiran Scholarship for academic excellence by the Hebrew University of Jerusalem, Israel.
Conflict of interest
The authors declare that the research was conducted in the absence of any commercial or financial relationships that could be construed as a potential conflict of interest.
The handling editor FA has declared past co-authorship with the author AB-M.
Publisher’s note
All claims expressed in this article are solely those of the authors and do not necessarily represent those of their affiliated organizations, or those of the publisher, the editors and the reviewers. Any product that may be evaluated in this article, or claim that may be made by its manufacturer, is not guaranteed or endorsed by the publisher.
Supplementary material
The Supplementary Material for this article can be found online at: https://www.frontiersin.org/articles/10.3389/fenvs.2023.1231490/full#supplementary-material
SUPPLEMENTARY TABLE S1 | WUI index according to national and regional parameters.
SUPPLEMENTARY FIGURE S1 | The VIIRS dataset characteristics, before (in blue) and after (in red) the filtering process. (A) Distribution by land cover classes. The black dots represent the percent reduction in active fires following the filtering at each land cover class. (B) Distribution by months. The number of pixels displayed as median because in 2018 the satellite NOAA-20 became operational, collecting data in addition to the Soumi-NPP satellite. The grey area represents the reducing percent at each month.
SUPPLEMENTARY FIGURE S2 | Demonstration of the WUI index calculation. The expected values are based on the actual distribution of buildings and fuel.
References
Alterman, R. (2001). National-level planning in democratic countries: An international comparison of city and regional policy-making. Liverpool University Press.
Badia, A., Pallares-Barbera, M., Valldeperas, N., and Gisbert, M. (2019). Wildfires in the wildland-urban interface in Catalonia: Vulnerability analysis based on land use and land cover change. Sci. Total Environ. 673, 184–196. doi:10.1016/j.scitotenv.2019.04.012
Bar Massada, A., Radeloff, V. C., Stewart, S. I., and Hawbaker, T. J. (2009). Wildfire risk in the wildland–urban interface: A simulation study in northwestern Wisconsin. For. Ecol. Manag. 258, 1990–1999. doi:10.1016/j.foreco.2009.07.051
Bar Massada, A., Syphard, A. D., Stewart, S. I., and Radeloff, V. C. (2013). Wildfire ignition-distribution modelling: A comparative study in the Huron?Manistee national forest, Michigan, USA. Int. J. Wildland Fire 22, 174. doi:10.1071/WF11178
Bar-Massada, A., Alcasena, F., Schug, F., and Radeloff, V. C. (2023). The wildland – urban interface in Europe: Spatial patterns and associations with socioeconomic and demographic variables. Landsc. Urban Plan. 235, 104759. doi:10.1016/j.landurbplan.2023.104759
Bar-Massada, A., Radeloff, V. C., and Stewart, S. I. (2014). Biotic and abiotic effects of human settlements in the wildland–urban interface. BioScience 64, 429–437. doi:10.1093/biosci/biu039
Bento-Gonçalves, A., and Vieira, A. (2020). Wildfires in the wildland-urban interface: Key concepts and evaluation methodologies. Sci. Total Environ. 707, 135592. doi:10.1016/j.scitotenv.2019.135592
Beverly, J. L., Bothwell, P., Conner, J. C. R., and Herd, E. P. K. (2010). Assessing the exposure of the built environment to potential ignition sources generated from vegetative fuel. Int. J. Wildland Fire 19, 299. doi:10.1071/WF09071
Caggiano, M. D., Hawbaker, T. J., Gannon, B. M., and Hoffman, C. M. (2020). Building loss in WUI disasters: Evaluating the core components of the wildland–urban interface definition. Fire 3, 73. doi:10.3390/fire3040073
Carlson, A. R., Helmers, D. P., Hawbaker, T. J., Mockrin, M. H., and Radeloff, V. C. (2022). The wildland–urban interface in the United States based on 125 million building locations. Ecol. Appl. 32, e2597. doi:10.1002/eap.2597
Chuvieco, E., Yebra, M., Martino, S., Thonicke, K., Gómez-Giménez, M., San-Miguel, J., et al. (2023). Towards an integrated approach to wildfire risk assessment: When, where, what and how may the landscapes burn. Fire 6, 215. doi:10.3390/fire6050215
Cohen, J. (1960). A coefficient of agreement for nominal scales. [WWW Document]. URL https://journals.sagepub.com/doi/10.1177/001316446002000104 (Accessed August 28, 2023).
Cohen, J. D. (2008). The wildland-urban interface fire problem: A consequence of the fire exclusion paradigm. For. Hist. Today Fall, 20–26.
Congalton, R. G. (1983). A quantitative method to test for consistency and correctness in photointerpretation. Photogramm. Eng.
Csiszar, I., Schroeder, W., Giglio, L., Ellicott, E., Vadrevu, K. P., Justice, C. O., et al. (2014). Active fires from the suomi NPP visible infrared imaging radiometer suite: Product status and first evaluation results. J. Geophys. Res. Atmos. 119, 803–816. doi:10.1002/2013JD020453
Feitelson, E. (2018). Shifting sands of planning in Israel. Land Use Policy 79, 695–706. doi:10.1016/j.landusepol.2018.09.017
Finney, M. A., McHugh, C. W., Grenfell, I. C., Riley, K. L., and Short, K. C. (2011). A simulation of probabilistic wildfire risk components for the continental United States. Stoch. Environ. Res. Risk Assess. 25, 973–1000. doi:10.1007/s00477-011-0462-z
Gale, M. G., and Cary, G. J. (2022). What determines variation in remotely sensed fire severity? Consideration of remote sensing limitations and confounding factors. Int. J. Wildland Fire 31, 291–305. doi:10.1071/WF21131
González-Cabán, A. (2008). Proceedings of the second international symposium on fire economics, planning, and policy: A global view (No. PSW-GTR-208). Albany, CA: U.S. Department of Agriculture, Forest Service, Pacific Southwest Research Station. doi:10.2737/PSW-GTR-208
Guk, E., Bar-Massada, A., and Levin, N. (2023). Constructing a comprehensive national wildfire database from incomplete sources: Israel as a case study. Fire 6, 131. doi:10.3390/fire6040131
Intini, P., Ronchi, E., Gwynne, S., and Bénichou, N. (2020). Guidance on design and construction of the built environment against wildland urban interface fire hazard: A review. Fire Technol. 56, 1853–1883. doi:10.1007/s10694-019-00902-z
Kutiel, H. (2012). Weather conditions and forest fire propagation—the case of the Carmel fire, december 2010. Isr. J. Ecol. Evol. 58, 113–122. doi:10.1560/IJEE.58.2-3.113
Lampin-Maillet, C., Jappiot, M., Long, M., Bouillon, C., Morge, D., and Ferrier, J.-P. (2010). Mapping wildland-urban interfaces at large scales integrating housing density and vegetation aggregation for fire prevention in the South of France. J. Environ. Manage. 91, 732–741. doi:10.1016/j.jenvman.2009.10.001
Lestienne, M., Vannière, B., Curt, T., Jouffroy-Bapicot, I., and Hély, C. (2022). Climate-driven Mediterranean fire hazard assessments for 2020–2100 on the light of past millennial variability. Clim. Change 170, 14. doi:10.1007/s10584-021-03258-y
Levin, N., and Heimowitz, A. (2012). Mapping spatial and temporal patterns of Mediterranean wildfires from MODIS. Remote Sens. Environ. 126, 12–26. doi:10.1016/j.rse.2012.08.003
Levin, N., and Saaroni, H. (1999). Fire weather in Israel — synoptic climatological analysis. GeoJournal 47, 523–538. doi:10.1023/a:1007087217249
Li, S., Dao, V., Kumar, M., Nguyen, P., and Banerjee, T. (2022). Mapping the wildland-urban interface in California using remote sensing data. Sci. Rep. 12, 5789. doi:10.1038/s41598-022-09707-7
Misgav, A. (2000). Visual preference of the public for vegetation groups in Israel. Landsc. Urban Plan. 48, 143–159. doi:10.1016/S0169-2046(00)00038-4
Modugno, S., Balzter, H., Cole, B., and Borrelli, P. (2016). Mapping regional patterns of large forest fires in Wildland–Urban Interface areas in Europe. J. Environ. Manage. 172, 112–126. doi:10.1016/j.jenvman.2016.02.013
Orenstein, D. E., and Hamburg, S. P. (2009). To populate or preserve? Evolving political-demographic and environmental paradigms in Israeli land-use policy. Land Use Policy 26, 984–1000. doi:10.1016/j.landusepol.2008.12.003
Radeloff, V. C., Hammer, R. B., Stewart, S. I., Fried, J. S., Holcomb, S. S., and McKeefry, J. F. (2005). The wildland–urban interface in the United States. Ecol. Appl. 15, 799–805. doi:10.1890/04-1413
Radeloff, V. C., Helmers, D. P., Kramer, H. A., Mockrin, M. H., Alexandre, P. M., Bar-Massada, A., et al. (2018). Rapid growth of the US wildland-urban interface raises wildfire risk. Proc. Natl. Acad. Sci. 115, 3314–3319. doi:10.1073/pnas.1718850115
Roy, D. P., Boschetti, L., Justice, C. O., and Ju, J. (2008). The collection 5 MODIS burned area product — global evaluation by comparison with the MODIS active fire product. Remote Sens. Environ. 112, 3690–3707. doi:10.1016/j.rse.2008.05.013
Safford, H. D., Stevens, J. T., Merriam, K., Meyer, M. D., and Latimer, A. M. (2012). Fuel treatment effectiveness in California yellow pine and mixed conifer forests. For. Ecol. Manag. 274, 17–28. doi:10.1016/j.foreco.2012.02.013
Salis, M., Arca, B., Alcasena, F., Arianoutsou, M., Bacciu, V., Duce, P., et al. (2016). Predicting wildfire spread and behaviour in Mediterranean landscapes. Int. J. Wildland Fire 25, 1015–1032. doi:10.1071/WF15081
Schroeder, W., Oliva, P., Giglio, L., and Csiszar, I. A. (2014). The New VIIRS 375m active fire detection data product: Algorithm description and initial assessment. Remote Sens. Environ. 143, 85–96. doi:10.1016/j.rse.2013.12.008
Shachar, A. (1998). Reshaping the map of Israel: A new national planning doctrine. Ann. Am. Acad. Pol. Soc. Sci. 555, 209–218. doi:10.1177/0002716298555001014
Stewart, S. I., Radeloff, V. C., Hammer, R. B., and Hawbaker, T. J. (2007). Defining the wildland–urban interface. J. For. 105, 201–207. doi:10.1093/jof/105.4.201
The State Comptroller Report (2018). Available at: https://www.mevaker.gov.il/he/Reports/Pages/645.aspx#tab-details (Accessed September 9, 2023).
Theobald, D. M., and Romme, W. H. (2007). Expansion of the US wildland–urban interface. Landsc. Urban Plan. 83, 340–354. doi:10.1016/j.landurbplan.2007.06.002
Turco, M., Levin, N., Tessler, N., and Saaroni, H. (2017). Recent changes and relations among drought, vegetation and wildfires in the Eastern Mediterranean: The case of Israel. Glob. Planet. Change, Clim. Var. Change Mediterr. Region 151, 28–35. doi:10.1016/j.gloplacha.2016.09.002
Keywords: Wildland-Urban Interface (WUI), VIIRS active fire, remote sensing, wildfires, risk mapping, natural hazards
Citation: Tikotzki I, Bar-Massada A and Levin N (2023) A geographically flexible approach for mapping the Wildland-Urban Interface integrating fire activity data. Front. Environ. Sci. 11:1231490. doi: 10.3389/fenvs.2023.1231490
Received: 30 May 2023; Accepted: 07 September 2023;
Published: 25 September 2023.
Edited by:
Juan Ramón Molina, University of Cordoba, SpainReviewed by:
Valentina Bacciu, National Research Council (CNR), ItalyFermín Alcasena, Universitat Politecnica de Catalunya, Spain
Copyright © 2023 Tikotzki, Bar-Massada and Levin. This is an open-access article distributed under the terms of the Creative Commons Attribution License (CC BY). The use, distribution or reproduction in other forums is permitted, provided the original author(s) and the copyright owner(s) are credited and that the original publication in this journal is cited, in accordance with accepted academic practice. No use, distribution or reproduction is permitted which does not comply with these terms.
*Correspondence: Idit Tikotzki, aWRpdC50aWtvdHpraUBtYWlsLmh1amkuYWMuaWw=