- 1Department of Zoology and Entomology, University of the Free State, Phuthaditjhaba, South Africa
- 2Endangered Wildlife Trust, Johannesburg, South Africa
Roads impact wildlife through a range of mechanisms from habitat loss and decreased landscape connectivity to direct mortality through wildlife-vehicle collisions (roadkill). These collisions have been rated amongst the highest modern risks to wildlife. With the development of “citizen science” projects, in which members of the public participate in data collection, it is now possible to monitor the impacts of roads over scales far beyond the limit of traditional studies. However, the reliability of data provided by citizen scientists for roadkill studies remains largely untested. This study used a dataset of 2,666 roadkill reports on national and regional roads in South Africa (total length ~170,000 km) over 3 years. We first compared roadkill data collected from trained road patrols operating on a major highway with data submitted by citizen scientists on the same road section (431 km). We found that despite minor differences, the broad spatial and taxonomic patterns were similar between trained reporters and untrained citizen scientists. We then compared data provided by two groups of citizen scientists across South Africa: (1) those working in the zoology/conservation sector (that we have termed “regular observers,” whose reports were considered to be more accurate due to their knowledge and experience), and (2) occasional observers, whose reports required verification by an expert. Again, there were few differences between the type of roadkill report provided by regular and occasional reporters; both types identified the same area (or cluster) where roadkill was reported most frequently. However, occasional observers tended to report charismatic and easily identifiable species more often than road patrols or regular observers. We conclude that citizen scientists can provide reliable data for roadkill studies when it comes to identifying general patterns and high-risk areas. Thus, citizen science has the potential to be a valuable tool for identifying potential roadkill hotspots and at-risk species across large spatial and temporal scales that are otherwise impractical and expensive when using standard data collection methodologies. This tool allows researchers to extract data and focus their efforts on potential areas and species of concern, with the ultimate goal of implementing effective roadkill-reduction measures.
Introduction
Roads (and their associated users) affect wildlife through a wide range of mechanisms. They are responsible for habitat loss, degradation (Trombulak and Frissell, 2000), and decreased landscape connectivity resulting in a barrier effect and road avoidance behavior (D'Amico et al., 2015a). Furthermore, these fragmented habitats act as filters, allowing some species to cross while others are killed (e.g., snake species that cross roads at low speed are more at risk of mortality than others that cross rapidly, Andrews and Gibbons, 2005). The most conspicuous and studied effect of roads on wildlife is the direct casualties resulting from collisions with vehicles (i.e., roadkill). Wildlife mortality due to roadkill often exceeds natural rates (Forman et al., 2003) and has the potential to affect all individuals in a population equally, unlike predation (Jaarsma et al., 2006). For some species, such as the Florida panther (Felis concolor coryi), vehicle collisions are the main cause of mortality (Harris and Scheck, 1991). In certain cases, it can even be the cause of population decline, for example, causing a decrease of 30% in hedgehog (Erinaceus europaeus) density in The Netherlands (Huijser and Bergers, 2000).
In recent decades, the number of studies focusing on the impacts of roads on biodiversity has increased considerably, leading to the rise of an applied scientific discipline called “road ecology” (Forman et al., 2003; Coffin, 2007). Early road ecology research used roadkill to monitor changes in population (e.g., Baker et al., 2004), focused on emblematic species (e.g., Hobday and Minstrell, 2008) or examined spatial and temporal patterns in the distribution of roadkill (e.g., Taylor and Goldingay, 2004; Ramp et al., 2005). Many of these studies relied on road surveys conducted at regular intervals by trained observers (Barthelmess and Brooks, 2010; D'Amico et al., 2015b). Despite providing high quality data, these methods are costly in terms of both time and logistics and can thus only be applied to relatively small areas (Caro et al., 2000; Barthelmess and Brooks, 2010).
Citizen science—a new form of data acquisition involving public participation - potentially provides a large pool of enthusiastic contributors that could enhance data collection at scales far beyond the limit of traditional field (Wilson et al., 2013). Globally, dozens of web-based systems for reporting roadkill exist (Shilling et al., 2015). Examples include Project Splatter in the UK (https://projectsplatter.co.uk), the National Wildlife Accident Council in Sweden (http://www.viltolycka.se/) or the California Roadkill Observation System in the USA (CROS - http://www.wildlifecrossing.net/california). These systems vary greatly in purpose and taxonomic focus. While some citizen science projects have a standardized methodology for data collection, most systems allow for the submission of opportunistic or ad hoc observations, even though these are perceived to be of lower quality (Bird et al., 2014). Such non-standardized methods of data collection can bias the information and conclusions, as scientists cannot control for research effort, accurate species identification, or an observational bias toward more charismatic species. In the design of roadkill mitigation, it is therefore vital to understand whether informally collected data are sufficiently biased to potentially direct conservationists' attention to the incorrect areas or even species.
Several studies have tried to identify and quantify bias in roadkill data collection (e.g., Slater, 2002; Santos et al., 2011) or develop standardized recording methods (e.g., Collinson et al., 2014), but to our knowledge none has tested the capacity of data from citizen science surveys to provide reliable roadkill data. In this study, we assessed the potential value of citizen science data for roadkill studies by comparing ad hoc data provided by citizen scientists (termed “occasional”) to that of (1) road patrols by trained personnel (termed “road patrol”) and (2) regular, informed observers working in the conservation field (termed “regular”). These data were collated in the first national database on mammalian mortality on South African roads, by the Endangered Wildlife Trust (EWT), from records collected from 2010 to 2015. We compared both the species reported as well as the spatial clustering of roadkill reports between the three reporter types (occasional, regular and road patrol), to assess differences and potential sources of bias. If bias exists in the citizen science data, we expected to find higher frequencies of larger, more common and charismatic species in the citizen science roadkill data (Caro et al., 2000).
Methods
Data Collection
Following the launch of a national roadkill awareness campaign in 2013, the EWT gathered roadkill data using four different approaches: (i) developing a form-based reporting system on a website (ii) soliciting historical records of roadkill incidents (iii) developing a smartphone-application called “EWT Road Watch” and (iv) using social media such as LinkedIn, Twitter and Facebook to attract interest. All of these methods relied on data collected by lay people, satisfying the criteria for citizen science. From these four methods, two main types of data collection strategies emerged: occasional/random observations (187 individuals), and data from regular observers (nine individuals) that each provided >50 roadkill reports. Regular observers were not trained in data collection but were working in the Zoology/Conservation sector and thus their data were considered to be accurate. Road patrol staff received annual training (conducted by the EWT) in species identification, collection of roadkill data, and the taking of photographs. Data could only be verified if a photograph was submitted with the report.
In parallel to citizen scientist data collection, road patrol agencies were trained by EWT staff to conduct regular road transects on set routes. Transects were conducted on the N3 highway from Johannesburg to Durban along a total length of 431 km, driven four times a day every day (twice in each direction); teams were allocated to six shorter sections to ensure the whole distance was covered effectively. Once discovered, carcasses were removed from the road to avoid recounts (Collinson et al., 2014; Guinard et al., 2015). Road patrols took place from July 2011 to November 2014.
Due to the small number of reports pre-2011 and in 2015, we conducted the following analyses on the data from 2011 to 2014 only.
Identification of Repeated Sampling
Within each group of reporters (regular vs. occasional and road patrol vs. citizen scientists), we defined “repeated sampling” as a roadkill that was reported for the same species within a 2-day period, <150 m from one another. This resulted in two reports from the occasional reporter's dataset and 22 from the regular reporters' datasets being removed from the final dataset. There were no repeated samples by citizen scientists on the N3 since the road patrol removed carcasses from the road once detected.
The final dataset comprised a total of 2,642 roadkill reports, 1,647 from regular reporters, 786 from occasional reporters and 209 from the N3 road patrols (see Figure 1 for spatial distribution and Supplementary Materials S1, S2). A further 183 roadkill reports from citizens were located on the N3 surveyed by the road patrol.
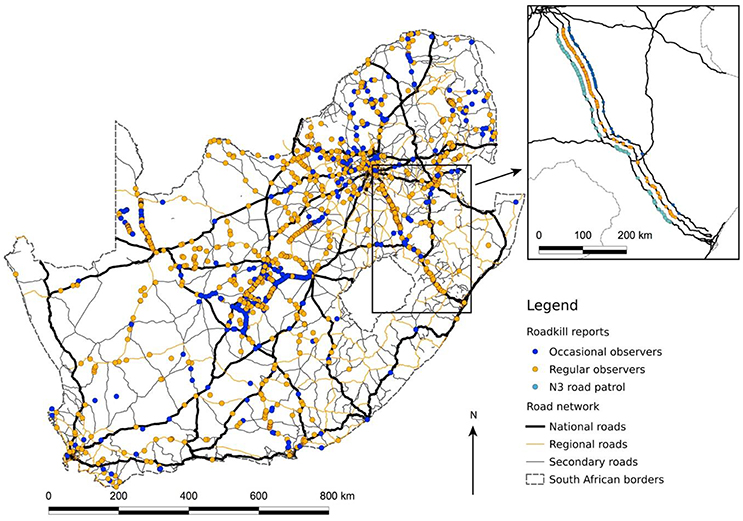
Figure 1. Spatial distribution of roadkill reports collected from occasional (dark blue dots) and regular (yellow dots) reporters in South Africa and along the N3 (insert) where roadkill reports were also provided by trained personnel conducting regular road patrols (light blue dots).
Data Categorization
Each species in the dataset was categorized according to taxonomic order. Domestic species (cat Felis catus, dog Canis lupus familiaris and livestock) were pooled into a group labeled “domestic,” and unknown/ unidentifiable mammal species were grouped into “Mammalia”. Three body mass classes were defined to account for carcass detection probability, using the average adult female body mass for African mammals (Skinner and Chimimba, 2005): very small (<2 kg), small (2–10 kg) and medium to large (>10 kg). Unknown species were assigned to a size class labeled “unknown.” Based on the National Red List of mammals in South Africa (Child et al., 2016) each species in the dataset was assigned to a Red List threat category. Domestic species and generic Mammalia were assigned a Not Determined (ND) Red List status.
Data Analysis
Citizen Science vs. Road Patrol Data
To assess the potential of the citizen scientist's contribution to roadkill surveys, we first compared the data provided by citizen scientists (both regular and occasional observers) to that compiled by systematic road patrols. For this analysis, we considered only the citizen science data collected on the road section where road transects were conducted by the road patrols (i.e., the N3 highway). The dataset was thus composed of 183 reports from citizen scientists compared to 209 reports provided by the N3 road patrol (Figure 1). We compared species size, taxonomic category and Red List category between these two datasets using Chi-square tests with false-discovery-rate correction for multiple comparisons. Analyses were performed in R software version 3.2.2 (R Core Team, 2016) using the function “chisqPostHoc” from the “NCStats” package (Ogle, 2015).
Regular vs. Occasional Observers' Data
To further assess the value of random roadkill reports, we compared data submitted by regular and occasional observers across South Africa. We used Chi-square tests with false-discovery-rate correction for multiple comparisons to test for difference in terms of size, taxonomic category and Red List category.
Cluster Analysis
To compare the spatial patterns between the three different types of observer (occasional, regular and road patrol), we conducted a cluster analysis using the KDE+ method defined in Bíl et al. (2015). For this analysis, datasets included only roadkill reports that provided a GPS location (N = 1,836, 137 for N3 road patrol, 505 for occasional and 1194 for regular reporters). Of these, only a portion could be clearly associated to a specific road from the Open Street Map data (http://download.geofabrik.de/africa/south-africa.html). Our dataset for comparison between trained and untrained observers reporting roadkill on the N3 highway consisted of 137 reports submitted by road patrols and 1874 from citizen scientists respectively. The remaining reports from our dataset provided a comparison of 872 (regular reporters) and 345 (occasional reporters) reports representing the South African road network (total length ~170,000 km, accounting for ~25% of the South African road network; Karani, 2008).
Results
From 2011 to 2014, a total of 2,642 mammalian roadkill incidents were reported on roads (totaling ~170,000 km) in South Africa, comprising 102 mammalian species from 14 orders (Supplementary Material S1). Of the 287 species on the National Red List, 24.7% (n = 71) were reported killed. Of these, 78.9% were of Least Concern (LC, n = 56), 11.3% were Near Threatened (NT, n = 8), 5.6% were Vulnerable (VU, n = 4), and 4.2% were Endangered (EN, n = 3). A total of 196 observers contributed toward the survey and we identified nine regular observers who provided 67.6% (n = 1,647) of the total citizen scientist dataset (n = 2,433). The majority of citizen reports were submitted via email (77.2%, n = 1,879) and on the smartphone-application (19.5%, n = 45). Social media (1.4%, n = 33) and direct reporting through SMS or phone call (1.5%, n = 37) provided only a small fraction of the data.
Roadkill Patterns Identified from the Citizen Scientist (Occasional and Regular) vs. Road Patrol Data
Along the same section of the N3 highway, trained road patrols and citizen scientists (from both occasional and regular reports totaling 183 reports) reported a total of 31 and 35 mammalian species, respectively, across eight and nine taxonomic groups (Supplementary Material S2). The vast majority of the road patrol reports were of small and medium sized species (Figure 2A), in NT and ND Red List categories (Figure 2B). The distribution of Red List category (χ2 = 21.7, df = 2, p < 0.001) from road patrol reports was significantly different from that reported by citizen scientists. Citizen scientists reported more roadkill of medium-size and species falling in the Red List category EN (Figures 2A,B). Additionally, they reported less very-small and NT species (Figure 2B). The frequencies of taxonomic groups reported differed significantly between road patrols and citizen scientists (χ2 = 26.9, df = 8, p < 0.001), the latter reporting more Carnivora and domestic mammals and fewer Rodentia and unknown mammals than road patrols (Figure 2C).
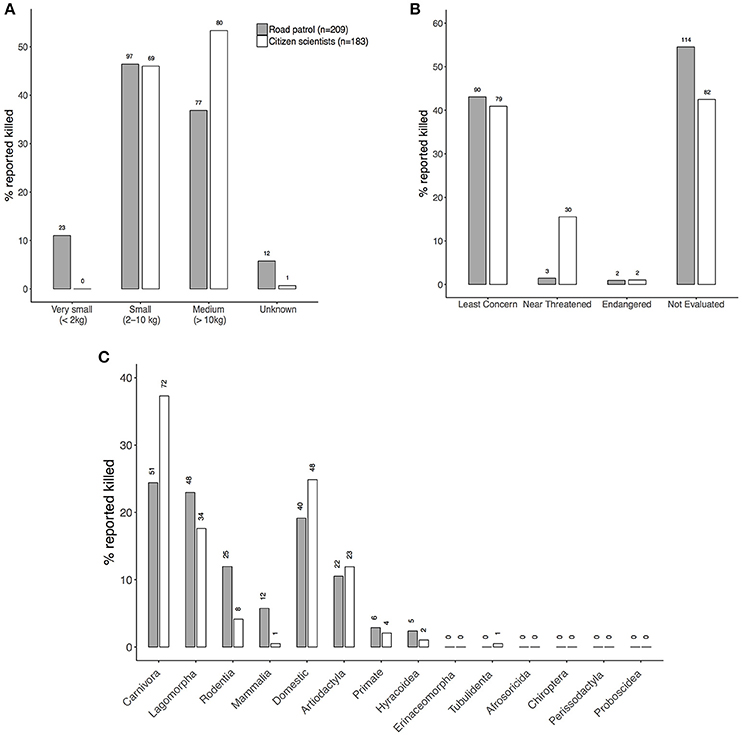
Figure 2. Comparison of the distributions of (A) sizes, (B) Red List category of species and (C) taxonomic groups reported killed along a 431 km section of the N3 road by road patrols (white) and citizen scientists (gray). Figures above bars represent the number reported in each category.
The top three roadkill species reported by road patrols (unknown rabbit sp Leporidae sp., 22.1%; domestic dog Canis lupus familiaris, 11.5%; and black-backed jackal Canis mesomelas 10.5%; Supplementary Material S2) were among the top four species reported by citizen scientists, followed by serval (Leptailurus serval, 14.8%).
Using the KDE+ method, we identified eight and 11 significant clusters of roadkill from the road patrol and citizen scientist reports respectively. Using a threshold strength of 0.4 to define biologically relevant clusters, seven road patrol clusters were relevant as were eight citizen scientist clusters. These clusters represent 10.9% (n = 15) and 12.1% (n = 21) of the roadkill reported by road patrol and citizens respectively. Most of these clusters (four from road patrols and seven from citizens) were located within a section of ~41 km along the northern part of the surveyed road (Figure 3), representing ~10% of the total distance surveyed (431 km).
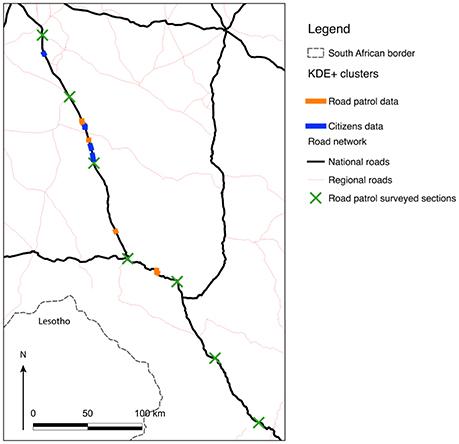
Figure 3. Clusters of roadkill reported on the N3 identified from the road patrol (orange) and citizens (blue) reports using KDE+ method. Only clusters with a strength superior to 0.4 are shown.
Comparison of Regular and Occasional Observers' Data
Taxonomic and Trait Patterns
The body size of the majority of the species reported killed by both regular and occasional observers was small (Figure 4A) and of LC Red List category (Figure 4B). Carnivora was the order most often reported for both citizen scientist observer types, with Lagomorpha and Rodentia forming the remaining bulk (Supplementary Material S1, Figure 4C). There was no significant difference in the frequency distribution of size (χ2 = 0.9, df = 3, p > 0.05), Red List categories (χ2 = 2.7, df = 3, p > 0.05) or taxonomic groups (χ2 = 18.7, df = 13, p > 0.05) reported between occasional and regular observers.
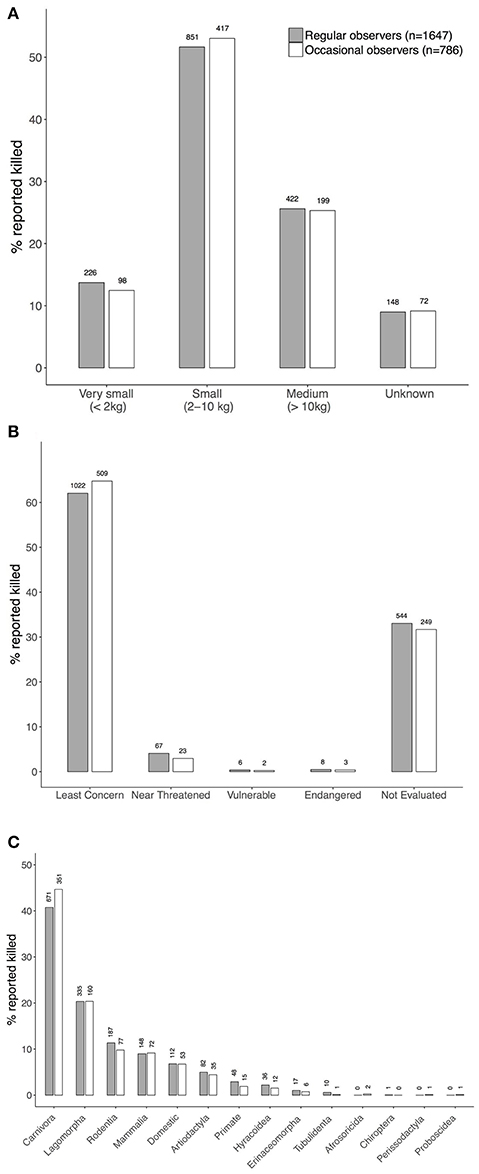
Figure 4. Comparison of the distributions of (A) sizes, (B) Red List category of species and (C) taxonomic groups reported killed across South Africa by regular (gray) and occasional (white) observers. Figures above bars represent the number reported in each category.
A total of 70 species were reported by occasional observers compared to a total of 88 for regular reporters. In both datasets, scrub hare (Lepus saxatilis; regular n = 169 and occasional n = 85) was the most prevalent species, followed by unknown species (regular n = 148 and occasional n = 72). Bat-eared fox (Otocyon megalotis) ranked fourth (n = 123) and third (n = 66) respectively for regular and occasional observers, followed by black-backed jackal, which ranked fifth for both regular (n = 107) and occasional (n = 48) observers. Aardwolf (Proteles cristata) ranked sixth (n = 82) and fourth (n = 52) respectively for regular and occasional observers, whilst unknown rabbit species (Leporidae) ranked third (n = 127) in the regular observers' dataset but was sixth (n = 42) in the occasional observers' dataset (Supplementary Material S1).
Spatial Patterns
From the regular and occasional reports, the KDE+ method allowed for the detection of 45 and 14 roadkill clusters respectively. Of these, 30 clusters for regular reporters and 9 clusters for occasional reporters had a strength superior to 0.4 (Figure 5). These clusters represent roadkill reports from 43.1% (n = 73) of regular and 3.6% (n = 18) of occasional citizen scientist participants. Nearly half of the clusters from both regular (n = 14) and occasional (n = 2) observers were located in the center of the country (Bloemfontein-Kimberley region; Figure 5) along sections totaling ~550 km of both national and regional roads. Four regular observers' clusters were also located on the N3 (Figure 5).
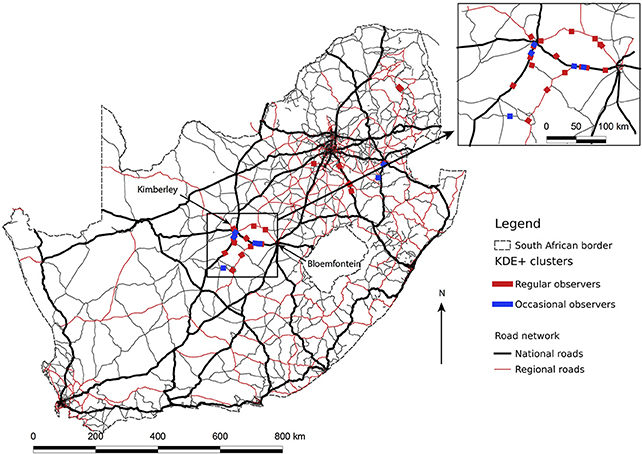
Figure 5. Clusters of roadkill reported across South Africa from regular (red) and occasional (blue) reports using KDE+ method. The insert shows the Bloemfontein-Kimberley region where most of the clusters are concentrated. Only clusters with a strength superior to 0.4 are shown.
Discussion
Summary of Key Findings
Data quality gathered by citizen scientists can be compromised (Floridi, 2012) due to inaccuracies in reporting and lack of scientific understanding (Batini and Scannapieca, 2006) and thus should be interpreted with caution. Our results suggest that data collected by ad hoc citizen scientists (i.e., occasional reporters) can be as accurate as those obtained by trained or informed reporters in terms of broad spatial patterns and species identification. Citizen scientists (i.e., occasional and regular reporters) who gathered roadkill data in South Africa between 2011 and 2014, largely agreed with the data from that of trained road patrols. Furthermore, the regular, informed reporters conveyed similar roadkill patterns as the occasional reporters.
Caro et al. (2000) noted during roadkill counts in California that small mammals (<10 kg) are more difficult to see when driving at normal speed (~100 km/h−1). Furthermore, these smaller species are often quickly removed from roads by scavengers or become problematic to identify by observers as they become “flattened” by vehicles using the roads (Hels and Buchwald, 2001), thus making the recording of roadkill less accurate. In the case of our study, South African citizen scientists were less likely to report smaller species than the road patrols along the N3 highway. This is in line with our assumption that citizen scientist data would be biased toward larger species (>10 kg). In addition, citizen scientists might also lose motivation or put less effort in to reporting unidentified or “low profile” species, assuming that the information is not scientifically valuable (Lukyanenko et al., 2016). Our data show that road patrols frequently report a high number of very-small and unidentified species. This is likely due to them driving at slower speeds (<60 km/h−1) as well as the training they receive from the EWT in species identification and collecting roadkill data (Collinson, 2016, pers. obs.).
A third of the roadkill clusters identified by occasional reporters were found in locations that agreed with those of regular reporters. The same was true when comparing data from road patrols to both citizen scientist reports (occasional and regular) as well as those of regular and occasional reports. Clusters of roadkill (i.e., roadkill hotspots) were concentrated on the same road sections in South Africa which represents 0.3% of the road network analyzed (~170,000 km). By comparison, 70% of clusters were not close to the clusters identified using data from the other reporting group (road patrol vs. citizens or regular vs. occasional reporters). These most likely resulted from the difference in sampling efforts by both types of citizen reporters. In our opinion, the spatial extent of the area surveyed by the 187 occasional observers (~765,000 km of the entire road network in South Africa; Karani, 2008) is expected to be larger than the one sampled by the nine regular observers (~170,000 km of road); smaller and less utilized roads are likely to have received a different sampling effort from both user groups. Our sample size from the occasional reporters (n = 505) was insufficient in terms of monitoring the country's road network, and consequently, a larger sample size is required to enable cluster analysis of the occasional data. We propose that the clusters identified as “roadkill hotspots” could be the focus of further and more detailed study that concentrate on the fine-scale patterns of roadkill and factors potentially responsible for the high intensity of collisions. Thus, while citizen scientists may not identify all the clusters noted by trained observers, these data can provide an early warning system for potential roadkill hotspots and data management (Shilling et al., 2015).
The role of citizen science in research and monitoring is increasing globally as a data collection tool (Lukyanenko et al., 2016) despite skepticism of the data produced by non-experts (Swanson et al., 2016). Our analysis demonstrates that the assumption that we target only “educated citizens” for the collection of roadkill data (Batini and Scannapieca, 2006) appears to be unnecessary. In this large-scale study of citizen science data collection patterns in South Africa, we conclude that the biases that may be present in our data (Floridi, 2012) are not significant enough to ignore the immense value of citizen science projects. Our study demonstrates that ad hoc citizen science has the potential to map roadkill occurrence and identify hotspots in a reliable and robust manner compared to that of trained road patrols and informed reporters. However, we propose that ad hoc citizen science data should only be used to identify general patterns or trends. As Lukyanenko et al. (2016) states, “Citizen science is about writing a story where citizens contribute to the plot. Experienced researchers should then assume the role of directing the actors and writing the dialogue.”
Recommendations
We outline below recommendations to improve the accuracy, sampling effort and the motivation of the citizen scientist.
(1) Data accuracy: Our dataset did not allow for species identification verification, since not all data were submitted with a photograph. We therefore propose that all similar projects encourage the submission of a photograph that can be verified by an expert, or cross-referenced by other citizen scientists (Swanson et al., 2015, 2016).
(2) Sampling effort: The sampling effort of data collected by both the citizen scientists and the road patrols was not recorded and we were therefore unable to correct for sampling effort bias; this leads to potential bias in both spatial distribution and species reporting. We therefore propose that (where possible) sample effort is encouraged with data submitted on not only where roadkill occurs but also roadkill absence (Shilling et al., 2015). For example, a smartphone application can either automatically record distances driven, or prompt users to report these data.
(3) Training and feedback: Citizen scientists contribute data because they want to make a difference and learn something new (Bonney et al., 2009; Silvertown et al., 2013; van der Wal et al., 2016). Thus, training opportunities (either online or in person), and feedback (not simply in scientific journals) can be invaluable ways of retaining and attracting citizen scientists across the globe. Face-to-face training will also provide the opportunity to explain the value in submitting all data, including the reporting of small and/or unidentifiable carcasses.
(4) Vary the tools: Not all potential citizen scientists are likely to prefer the same method of collecting data. Thus, some individuals will be engaged by an interactive website (Swanson et al., 2015, 2016), while others prefer a smartphone app, and another group may prefer email communication (as was the case in this study).
Despite the limitations associated with reporting efforts, the EWT's citizen science project has established the first national database for animal road mortalities. This will guide future management decisions on mitigating the negative impacts of roads and provide a platform from which future studies can be designed.
Author Contributions
We acknowledge the following for their contributions toward this manuscript: Data centralization and cleaning: WC and LR; Data analysis: SP; Manuscript writing: SP, LR, WC, and AIR.
Funding
We thank the Directorate Research Development at the University of the Free State for financial support to SP. We also thank Bridgestone SA, N3 Toll Concession (N3TC) and Bakwena Platinum Corridor Concession for their financial support of the EWT's project.
Conflict of Interest Statement
The authors declare that the research was conducted in the absence of any commercial or financial relationships that could be construed as a potential conflict of interest.
Acknowledgments
This research was initiated by the Endangered Wildlife Trust, with funding from Bridgestone SA, N3 Toll Concession (N3TC) and Bakwena Platinum Corridor Concession. We are grateful to Carsten Abrolat for the design of the Road Watch smartphone application and to our regular and occasional data reporters for their invaluable contributions. Our deepest thanks to Jirí Sedoník for his help in running the KDE+ cluster analysis.
Supplementary Material
The Supplementary Material for this article can be found online at: https://www.frontiersin.org/articles/10.3389/fevo.2018.00015/full#supplementary-material
References
Andrews, K. M., and Gibbons, J. W. (2005). How do highways influence snake movement? Behavioral responses to roads and vehicles. Copeia 2005, 772–782. doi: 10.1643/0045-8511(2005)005[0772:HDHISM]2.0.CO;2
Baker, P. J., Harris, S., Robertson, C. P. J., Saunders, G., and White, P. C. L. (2004). Is it possible to monitor mammal population changes from counts of road traffic casualties? An analysis using Bristol's red foxes vulpes vulpes as an example. Mammal Rev. 34, 115–130. doi: 10.1046/j.0305-1838.2003.00024.x
Barthelmess, E. L., and Brooks, M. S. (2010). The influence of body-size and diet on road-kill trends in mammals. Biodivers. Conserv. 19, 1611–1629. doi: 10.1007/s10531-010-9791-3
Batini, C., and Scannapieca, M. (2006). Data quality; Concepts, Methodoclogies and Techniques. New York, NY: Springer.
Bíl, M., Andrášik, R., Svoboda, T., and Sedoník, J. (2015). The KDE+ software: a tool for effective identification and ranking of animal-vehicle collision hotspots along networks. Landsc. Ecol. 31, 231–237. doi: 10.1007/s10980-015-0265-6
Bird, T. J., Bates, A. E., Lefcheck, J. S., Hill, N. A., Thomson, R. J., Edgar, G. J., et al. (2014). Statistical solutions for error and bias in global citizen science datasets. Biol. Conserv. 173, 144–154. doi: 10.1016/j.biocon.2013.07.037
Bonney, R., Cooper, C. B., Dickinson, J., Kelling, S., Phillips, T., Rosenberg, K. V., et al. (2009). Citizen science: a developing tool for expanding science knowledge and scientific literacy. Bioscience 59, 977–984. doi: 10.1525/bio.2009.59.11.9
Caro, T. M., Shargel, J. A., and Stoner, C. J. (2000). Frequency of medium-sized mammal road kills in an agricultural landscape in California. Am. Midland Nat. 144, 362–369. doi: 10.1674/0003-0031
Child, M. F., Roxburgh, L., Do Linh San, E., Raimondo, D., Davies-Mostert, H. T., and Raimondo, D. (eds.) (2016). The Red List of Mammals of South Africa, Lesotho and Swaziland. Johannesburg: South African National Biodiversity Institute and Endangered Wildlife Trust, South Africa.
Coffin, A. W. (2007). From roadkill to road ecology: a review of the ecological effects of roads. J. Trans. Geogr. 15, 396–406. doi: 10.1016/j.jtrangeo.2006.11.006
Collinson, W. J., Parker, D. M., Bernard, R. T., Reilly, B. K., and Davies-Mostert, H. T. (2014). Wildlife road traffic accidents: a standardized protocol for counting flattened fauna. Ecol. Evol. 4, 3060–3071. doi: 10.1002/ece3.1097
D'Amico, M., Périquet, S., Roman, J., and Revilla, E. (2015a). Road avoidance responses determine the impact of heterogeneous road networks at a regional scale. J. Appl. Ecol. 53, 181–190. doi: 10.1111/1365-2664.12572
D'Amico, M., Roman, J., de los Reyes, L., and Revilla, E. (2015b). Vertebrate road-kill patterns in Mediterranean habitats: who, when and where. Biol. Conserv. 191, 234–242. doi: 10.1016/j.biocon.2015.06.010
Forman, R. T., Sperling, D., Bissonette, J. A., Clevenger, A. P., Cutshall, C. D., Dale, V. H., et al. (2003). Road Ecology: Science and Solutions. Washington, DC: Island Press.
Guinard, E., Prodon, R., and Barbraud, C. (2015). “Case study: a robust method to obtain defendable data on wildlife mortality,” in Handbook of Road Ecology, eds R. van der Ree, D. J. Smith, and C. Grilo (Oxford, UK: John Wiley & Sons), 96–100.
Harris, L. D., and Scheck, J. (1991). From implications to applications: the dispersal corridor principle applied to the conservation of biological diversity. Nat. Conserv. 2, 189–220.
Hels, T., and Buchwald, E. (2001). The effect of road kills on amphibian populations. Biol. Conserv. 99, 331–340. doi: 10.1016/S0006-3207(00)00215-9
Hobday, A. J., and Minstrell, M. L. (2008). Distribution and abundance of roadkill on Tasmanian highways: human management options. Wildlife Res. 35, 712–715. doi: 10.1071/WR08067
Huijser, M. P., and Bergers, P. (2000). The effect of roads and traffic on hedgehog (Erinaceus europaeus) populations. Biol. Conserv. 95, 111–116. doi: 10.1016/S0006-3207(00)00006-9
Jaarsma, C. F., van Langevelde, F., and Botma, H. (2006). Flattened fauna and mitigation: traffic victims related to road, traffic, vehicle, and species characteristics. Transport. Res. 11, 264–276. doi: 10.1016/j.trd.2006.05.001
Karani, P. (2008). Impacts of Roads on the Environment in South Africa. DBSA—Development Bank of Southern Africa, Midrand, South Africa [Online]. Available online at: https://www.dbsa.org/EN/About-Us/Publications/Documents/Environmental%20implications%20of%20the%20road%20network%20in%20South%20Africa.pdf [Accessed Dec 2016].
Lukyanenko, R., Parsons, J., and Wiersma, Y. F. (2016). Emerging problems of data quality in citizen science. Conserv. Biol. 30, 447–449. doi: 10.1111/cobi.12706
Ogle, D. H. (2015). NCStats: Helper Functions for Statistics at Northland College. R package version 0.4.4.
Ramp, D., Caldwell, J., Edwards, K. A., Warton, D., and Croft, D. B. (2005). Modelling of wildlife fatality hotspots along the Snowy Mountain Highway in New South Wales, Australia. Biol. Conserv. 126, 474–490. doi: 10.1016/j.biocon.2005.07.001
R Core Team (2016). R: A Language and Environment for Statistical Computing. Vienna: R Foundation for Statistical Computing. Version 3.3.2.
Santos, S. M., Carvalho, F., and Mira, A. (2011). How long do the dead survive on the road? Carcass persistence probability and implications for road-kill monitoring surveys. PLoS ONE 6:e25383. doi: 10.1371/journal.pone.0025383
Shilling, F., Prekins, S. E., and Collinson, W. J. (2015). “Wildlife/roadkill observation and reporting systems,” in Handbook of Road Ecology, 1st Edn., eds R. van der Ree, D. J. Smith and C. Grilo (West Sussex, UK: John Wiley & Sons, Ltd.), 492–501.
Silvertown, J., Buesching, C. D., Jacobson, S., and Rebelo, T. (2013). “Citizen science and nature conservation,” in Key Topics in Conservation Biology 2, 1st Edn., eds D. W. Macdonald and K. J. Willis (West Sussex, UK, John Wiley & Sons, Ltd.), 127–142.
Skinner, J. D., and Chimimba, C. T. (2005). The Mammals of the Southern African Subregion. Cambridge, UK; Cambridge University Press.
Slater, F. M. (2002). An assessment of wildlife road casualties–the potential discrepancy between numbers counted and numbers killed. Web Ecol. 3, 33–42. doi: 10.5194/we-3-33-2002
Swanson, A., Kosmala, M., Lintott, C., and Packer, C. (2016). A generalized approach for producing, quantifying, and validating citizen science data from wildlife images. Conserv. Biol. 30, 520–531. doi: 10.1111/cobi.12695
Swanson, A., Kosmala, M., Lintott, C., Simpson, R., Smith, A., and Packer, C. (2015). Snapshot Serengeti, high-frequency annotated camera trap images of 40 mammalian species in an African savanna. Sci. Data 2, 150026–150014. doi: 10.1038/sdata.2015.26
Taylor, B. D., and Goldingay, R. L. (2004). Wildlife road-kills on three major roads in north-eastern New South Wales. Wildlife Res. 31, 83–89. doi: 10.1071/WR01110
Trombulak, S., and Frissell, C. (2000). Review of ecological effects of roads on terrestrial and aquatic communities. Conserv. Biol. 14, 18–30. doi: 10.1046/j.1523-1739.2000.99084.x
van der Wal, R., Sharma, N., Mellish, C., Robinson, A., and Siddharthan, A. (2016). The role of automated feedback in training and retaining biological recorders for citizen science. Conserv. Biol. 30, 550–561. doi: 10.1111/cobi.12705
Keywords: spatial clusters, mammals, mortality, road ecology, wildlife-vehicle collisions
Citation: Périquet S, Roxburgh L, le Roux A and Collinson WJ (2018) Testing the Value of Citizen Science for Roadkill Studies: A Case Study from South Africa. Front. Ecol. Evol. 6:15. doi: 10.3389/fevo.2018.00015
Received: 15 March 2017; Accepted: 02 February 2018;
Published: 19 February 2018.
Edited by:
Edgar Van Der Grift, Wageningen Environmental Research, NetherlandsReviewed by:
Susan C. Cook-Patton, Nature Conservancy, United StatesMelissa Renee McHale, Colorado State University, United States
Copyright © 2018 Périquet, Roxburgh, le Roux and Collinson. This is an open-access article distributed under the terms of the Creative Commons Attribution License (CC BY). The use, distribution or reproduction in other forums is permitted, provided the original author(s) and the copyright owner are credited and that the original publication in this journal is cited, in accordance with accepted academic practice. No use, distribution or reproduction is permitted which does not comply with these terms.
*Correspondence: Wendy J. Collinson, d2VuZHljQGV3dC5vcmcuemE=