- 1Department of Soil, Water, and Environmental Science, University of Arizona, Tucson, AZ, United States
- 2School of Forest Resources and Conservation, University of Florida, Gainesville, FL, United States
- 3Department of Ecology and Evolutionary Biology, University of Colorado, Boulder, CO, United States
- 4Cooperative Institute for Research in Environmental Sciences, University of Colorado, Boulder, CO, United States
- 5Department of Biosystems Engineering, University of Arizona, Tucson, AZ, United States
As the world's population grows, global food production will need to increase. While food production efficiency has increased in recent decades through pathogen control, climate change poses new challenges in crop protection against pathogens. Understanding the natural geographical distribution and dispersal likelihood of fungal plant pathogens is essential for forecasting disease plant spread. Here we used cultivation-independent techniques to identify fungal plant pathogens in 1,289 near-surface dust samples collected across the United States. We found that overall fungal pathogen community composition is more related to environmental conditions (in particular soil pH, precipitation and frost) than to agricultural hosts and practices. We also delimited five susceptibility geographical areas in the United States where different sets of pathogens tend to occur.
Introduction
As our global population continues to grow, the demand for food will also increase with the concomitant needs to optimize crop yield and minimize crop loss to plant diseases (Gilland, 2002; Parry et al., 2004; Savary et al., 2019). It is estimated that yield losses to plant diseases represent ~12% of total crop production worldwide (Reeleder, 2003). Developed areas that practice intensive agriculture based on irrigation, fertilization and monoculture invest increasing amounts of economic resources into crop protection. For example, in the United States alone, where pesticides are applied at a cost of $11.2 billion per year, crop losses to plant disease equal $9.1 billion a year (Agrios, 2005). The prevalence and severity of outbreaks caused by virulent fungal plant pathogens have increased during the past two decades and are recognized as emergent threats to food security worldwide (Fisher et al., 2012; Santini et al., 2013). Additionally, modern agricultural expansion based on a limited genetic diversity of crops increases the risks of global disease spread (Brown and Hovmøller, 2002). Thus, it is of utmost importance to understand the geographical distribution of fungal plant pathogens.
The geographical distributions of fungal plant pathogens are impacted by crop management, climatic, biotic and other environmental factors, as well as their host distribution and susceptibility levels (Borer et al., 2016). For example, local plant community structure and diversity may support different levels of plant pathogen diversity by favoring specialist or generalist pathogens, or decreasing the density of susceptible hosts (Bever et al., 2015). Overall, individual fungal species show strong spatial patterns reflecting an array of environmental adaptations and dispersal limitations (Reich et al., 1997; Peay et al., 2012; Barberán et al., 2015). Fungal spores are disseminated in dust by air currents from meters to hundreds of kilometers depending on air turbulence, velocity, UV radiation, and desiccation tolerance until they are deposited or washed out by rain (Aylor, 2003). While fungal dispersion over short distances is common (Peay et al., 2010), fungal spores can be transported thousands of kilometers by humans, plants, and animals (via seeds and feathers), or by high wind events, such as hurricanes (Golan and Pringle, 2017). There are numerous historical examples of long-distance spread of plant pathogenic fungi (Aylor, 1986). For example, Cryphonectria parasitica, the causative agent of chestnut blight, was accidentally introduced from Japan to North America in the early twentieth century, and by the 1920s had devastated chestnut trees across the eastern United States (Milgroom and Lipari, 1995). Although fungal spores are generally short-lived and susceptible to UV radiation and desiccation (Aylor, 2003), there are some reported cases of long-distance dispersal via “wind highways” (Smith et al., 2013). For example, the pathogen causing coffee leaf rust (Hemileia vastatrix) was probably wind-dispersed from Africa to Brazil in the 1970s (Bowden et al., 1971). Another example is the dispersal via African dust plumes of the causal agent of the aspergillosis (Aspergillus sydowii) in Caribbean coral reefs (Shinn et al., 2000). The ability to track the long-distance dispersal and continental-scale geographical distribution of fungal pathogens is essential for forecasting disease plant spread and for establishing effective quarantine measures (Brown and Hovmøller, 2002; Schmale and Ross, 2015). However, because the geographic range of fungal pathogens is typically documented as a function of disease incidence, there is little information on the natural distribution and likelihood of dispersal across spatial scales for most plant pathogens. Such information would ultimately assist farmers and practitioners with decisions about fungicide use, sanitation, and quarantine.
Using nearly 1,300 near-surface dust samples collected across the United States, we explored the geographical distribution of putative fungal plant pathogens detected by cultivation-independent methods and analyzed using a combination of machine learning tools. Our goals were to understand: (1) the impact of environmental and agricultural factors on the composition of fungal plant pathogen communities, and (2) the potential of delimiting geographical regions based on the presence or absence of fungal plant pathogens in dust. This information can be used to inform regional to continental-scale epidemiology and to predict the future effects of climate change on potential plant pathogen distributions.
Materials and Methods
Dust Samples, Molecular Analyses, and Sequence Processing
The data used in this study were collected through a citizen science project called Wild Life of Our Homes (yourwildlife.org) from March 2012 to May 2013. Volunteers across the United States sampled the upper door trim of the outside surface of an exterior home door using sterile swabs. Door trims served as a passive collector of near-surface dust since outside door frames are rarely cleaned. 1,289 dust samples were PCR amplified targeting the first nuclear ribosomal internal transcribed spacer region (ITS1) using the ITS1-F (GTGCCAGCMGCCGCGGTAA) and ITS2 (GCTGCGTTCTTCATCGATGC) fungal-specific primer pair (Schoch et al., 2012). Amplicons were sequenced on an Illumina MiSeq platform. Sequences were clustered at 97% similarity using UPARSE (Edgar, 2013) to generate a community table of phylotypes by sample. Taxonomic classification was determined using the Ribosomal Database Project (RDP) naïve Bayesian classifier (Wang et al., 2007) against the UNITE database (Abarenkov et al., 2010). We used FUNGuild to identify putative fungal plant pathogens (Nguyen et al., 2016) and confirmed the taxonomic assignment using BLAST against the NCBI nucleotide database (Altschul et al., 1997). Although we used state-of-the-art methods for sequence processing, taxonomic, and functional assignment, we acknowledge the limitations of ITS amplicon short reads for classifying fungi (Nilsson et al., 2019). For example, not all members of a given species are necessarily pathogenic as closely related taxa can have both pathogenic and non-pathogenic lifestyles (Rodriguez et al., 2009). Thus, we refer to these taxa as “putative fungal plant pathogens” as we recognize that identifying pathogens based on ITS gene sequence similarity alone is not sufficient to definitely determine whether a given fungus is, indeed, a fungal plant pathogen. Full details describing the molecular methods and sequence processing can be found in Barberán et al. (2015). The data used for this study are associated with NCBI BioProject PRJNA280363 and are publicly accessible at http://dx.doi.org/10.6084/m9.figshare.1270900.
Sample Characterization
Latitude and longitude coordinates were generated from home addresses. These coordinates were then used to acquire georeferenced variables for each household (mean annual temperature, mean annual precipitation, days of frost per year, elevation, soil pH, dust production, plant diversity, net primary productivity, and human population) from the British Atmospheric Data Center (http://badc.nerc.ac.uk), the NASA Earth Observations (http://neo.sci.gsfc.nasa.gov), the NASA Socioeconomic Data and Applications Center (http://sedac.ciesin.columbia.edu) and the World Soil Information (http://www.isric.org) as described in Barberán et al. (2015). We used the United States Department of Agriculture (USDA) statistics service (www.nass.usda.gov) to obtain county level information on crop production (apples, barley, berries, bulbs, cherries, citrus, corn, cotton, cucumbers, floriculture, grapes, beans, hay, legumes, lettuce, melons, oats, olives, peanuts, peaches, pears, peppers, potatoes, pumpkins, rice, rye, sorghum, soybean, sugar beets, tobacco, tomatoes, tree nuts, vegetables, and wheat) as well as economic- and production-related variables (total animal stock, chemicals used, fertilizers used, machinery used, tractors used, total crop economic yield, grain storage capacity). These estimates are developed from data collected through sample surveys conducted each year and the census of agriculture conducted every 5 years.
Data Analysis
We used variation partitioning (Peres-Neto et al., 2006) to understand the overall association between fungal plant pathogen community composition (using the Bray-Curtis distance metric) and explanatory variables (environmental, agricultural or the shared effect of both).
After gaining a better understanding of overall fungal plant pathogen community patterns, we investigated the explanatory power of individual variables using an extension of random forest analysis called gradient forest analysis (Ellis et al., 2012). Random forest is an ensemble of regression trees that randomly selects a subset of the data and partitions this subset based on the most strongly associated predictor. At each node, a random subset of the total number of predictors is considered for partitioning. At the end, the final tree prediction is given by the average value of the data within each branch of the tree (Breiman, 2001). Gradient forest analysis is an extension of random forest for multiple response variables (in our study, abundance of fungal plant pathogens). A function is built for each response variable, in this case each species of plant pathogen, and then an aggregate function is created for all species correcting for biases with correlated explanatory variables (i.e., conditional importance) (Strobl et al., 2008). This analysis provides information on the overall importance of each predictor across all the response variables and compositional turnover functions for each predictor.
Next, we investigated how the presence or absence of fungal plant pathogens in dust could be used to delimit distinct geographical areas across the United States. We applied Latent Dirichlet Allocation (LDA) to the putative fungal plant pathogen presence/absence data (Valle et al., 2018). LDA is a probabilistic model that reduces complex assemblages into sets of distinct component partitions based on groups of species that tend to co-occur. In the LDA model, each dust sample has an associated vector of probabilities which describes the membership to each partition. Similarly, each partition is characterized by a vector of probabilities which describes the association of each species with that particular partition. When fitting LDA, we set the maximum number of partitions to 20 and identified five main partitions after eliminating partitions that were very uncommon. This analysis allows us to demarcate susceptibility areas where different sets of fungal plant pathogens tend to occur.
All statistical analyses were carried out in the R environment (www.r-project.org/) using the vegan (https://cran.r-project.org/web/packages/vegan), gradientForest (http://gradientforest.r-forge.r-project.org/), and Rlda (https://cran.r-project.org/web/packages/Rlda/) packages. Interactive maps depicting the spatial distribution of the most abundant putative fungal plant pathogens in dust were generated using Tableau (www.tableau.com) and can be publicly accessed at http://dx.doi.org/10.6084/m9.figshare.1270900.
Results
From more than 50,000 total fungal phylotypes (1,400 fungal phylotypes per sample on average), we identified a total of 386 different species of putative fungal plant pathogens across all 1,289 dust samples. The average number of different putative fungal plant pathogens (i.e., richness) per outdoor dust sample was 45, and the average relative abundance of total fungal pathogens per sample was 21.5% (Figures 1A,B, respectively). The most abundant species of fungal plant pathogens in dust were identified as Cladosporium sphaerospermum, Alternaria alternata, Aureobasidium pullulans, and Epicoccum nigrum (Figure 1C).
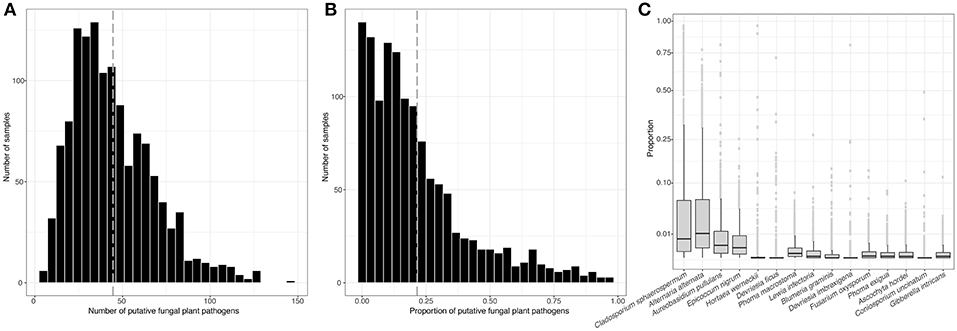
Figure 1. Number of different putative fungal plant pathogens (richness) in individual dust samples (mean = 45) (A). Abundance of putative fungal plant pathogens in dust samples (mean = 0.215) (B). Means represented as vertical lines for (A,B). Relative abundance of the most abundant species of fungal plant pathogens in dust samples (C). Note that the y-axis in (C) is square root scale.
Explaining Geographical Fungal Plant Pathogen Patterns in Dust
Variation partitioning provided the overall explanatory power between the three broad categories of environmental (mainly climate and soil), agricultural (mainly potential hosts and practices), and the shared effect of environmental and agricultural explanatory variables. Environmental variables alone explained 12% of the variation in community composition of putative fungal plant pathogens in dust, followed by the shared explanatory power of environmental and agricultural variables (7%), and finally agricultural variables alone (3%) (Figure 2A).
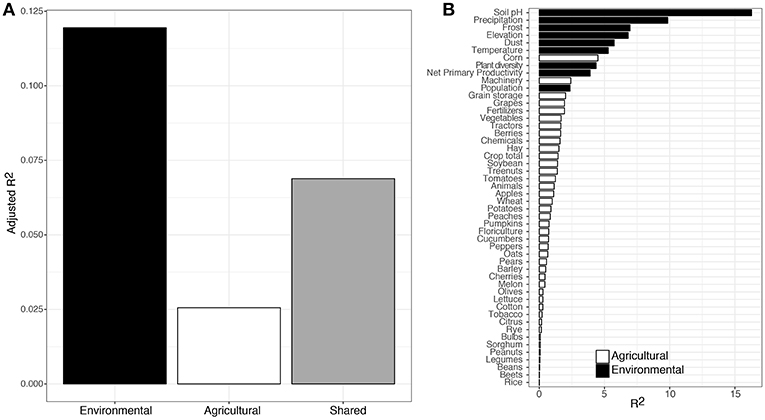
Figure 2. Variance partitioning results showing the explanatory power of environmental (black) and agricultural (white) variables in relation to fungal plant pathogen community composition in dust samples (A). Gradient forest analysis results showing the overall importance of individual variables on fungal plant pathogen community composition (B).
We explored the specific explanatory power for each variable using gradient forest analysis. Overall, environmental variables accounted for more explanatory power than agricultural variables (Figure 2B). The most important environmental variables were soil pH, mean annual precipitation, and number of days of frost per year (Supplementary Figure 1). Although less important than these environmental variables, the most important agricultural variables were corn production, machinery used, and volume of grain storage (Figure 2B).
Delimiting Susceptibility Areas Based on the Presence of Fungal Plant Pathogens in Dust
After Latent Dirichlet Allocation (LDA) analysis, five geographical partitions were identified based on the presence or absence of putative fungal plant pathogens in outdoor dust across the USA (Figure 3 and see http://dx.doi.org/10.6084/m9.figshare.1270900 for individual distribution maps of the most abundant pathogens). The Eastern partition was characterized by the presence of Alternaria alternata, Cladosporium sphaerospermum, Mycosphaerella punctiformis (=Ramularia endophylla), Aureobasidium pullulans and Monographella cucumerina (=Plectosphaerella cucumerina), among others. The Northern partition area was dominated by Geastrumia polystigmatis, Teratosphaeria capensis (=Neodevriesia capensis), Coniothyrium sidae, Harknessia capensis, and Microdiplodia miyakei. The species that best described the Western geographical area were Coniothyrium multiporum (=Neoconiothyrium multiporum), Collybia cirrhata (=Collybia cookei), Taphrina wiesneri, Valsa malicola, and Phoma exigua (=Boeremia exigua). The Northwestern Coastal area was characterized by Mycosphaerella ulmi, Laurobasidium hachijoense, Valsa ceratosperma (=Cytospora ceratosperma), Cylindrocladiella hahajimaensis, and Mycosphaerella latebrosa. The Southern central partition was defined by the presence of Taphrina dearnessii, Chalara holubovae, Setosphaeria turcica (=Exserohilum turcicum), Mycosphaerella populicola, and Athelia bombacina.
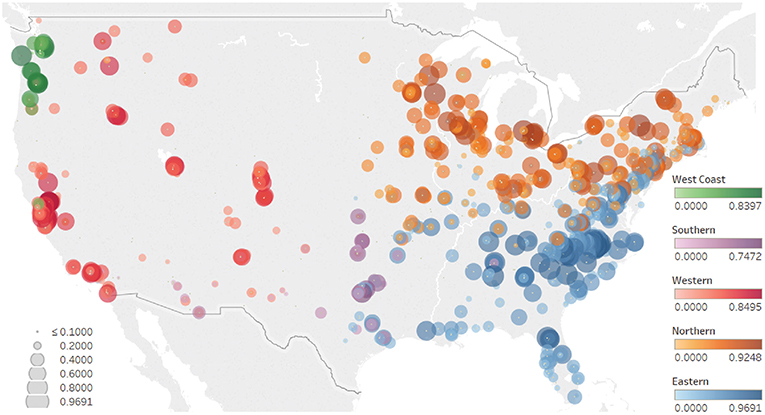
Figure 3. Map showing the results of the Latent Dirichlet Allocation (LDA) model. Size represents the probability of each dust sample being associated with a particular partition. Color represents the geographical partition: Northern (orange), Eastern (blue), Southern (purple), Western (red), and West Coast (green).
Discussion
By analyzing the fungal DNA recovered from dust samples, we were able to document the large-scale geographical patterns of fungal plant pathogens. On average, 21.5% of the total fungi community in dust was taxonomically assigned to a fungal plant pathogen. This is in agreement with a previous study that found that fungal plant pathogens account for 5–27% of the total culturable population in African dust (Kellogg et al., 2004). The most abundant and widespread fungal plant pathogens in settled dust across the US were ubiquitous, opportunistic, airborne, and stress-adaptable species such as Cladosporium sphaerospermum, Alternaria alternate, and Aureobasidium pullulans (Gonzalez-Martin et al., 2014). However, we acknowledge that passively collected dust alone might not recover the complete diversity of fungal plant pathogen inocula as different sampler types differ in their collection potential (Chen et al., 2018). Cladosporium sphaerospermum is a cosmopolitan saprotroph and secondary colonizer of dead or dying plant tissue that frequently dwells in nutrient poor environments like dust (Zalar et al., 2007). This airborne fungus produces melanin that serves as a protective mechanism against UV radiation (Ng et al., 2012), and thus, might enable its long-distance dispersal and survival in dust. Alternaria alternata is an opportunistic pathogen on numerous plant hosts that causes leaf spots, rots, and blights (Akimitsu et al., 2003). Both Alternaria and Cladosporium spores can induce allergies, the incidence and prevalence of which is on the rise (Rook, 2013). Aureobasidium pullulans is a black, ubiquitous, and extremely stress-tolerant fungus found in different environments such as the surface of plant leaves (i.e., phyllosphere), polluted waters, hypersaline habitats, and indoor dust (Gostinčar, 2011). These specific examples show the pervasiveness of fungal plant pathogens in dust, and are in concordance with previous reports showing that plant pathogenic fungi in dust samples are frequently dominated by the ubiquitous genera Aspergillus, Cladosporium, Alternaria, and Penicillium (Gonzalez-Martin et al., 2014).
Although there exists a long history of research on atmospheric fungal transport in dust (Wolf, 1943; Gorbushina et al., 2007), changes in intense agricultural practices, climate and land-use change, and global atmospheric systems have recently affected the amount of dust present in the atmosphere and transportation patterns (Gonzalez-Martin et al., 2014). Thus, understanding large-scale distributional patterns of fungal pathogens in dust is important not just for potential aid in mitigating crop infection and yield loss, but also for predicting disease in natural plant communities. Soilborne and dust-dispersed pathogens play a critical role in shaping plant community diversity and composition because they are able to mediate plant species' coexistence through trade-offs between competitive ability and pathogen defense (Mordecai, 2011; Bever et al., 2015). Here we showed that overall fungal pathogen community composition in dust is more strongly linked to environmental conditions (in particular soil pH, precipitation, and frost) than to potential agricultural hosts and agricultural practices. This means that to understand environmental transmission of pathogens in dust, we should focus on the ecological processes not only within the plant but also outside the plant (Shaw and Osborne, 2011). This is corroborated by population dynamics models that stress the importance of the pathogen's growth in the non-host environment on disease amplification (Thrall et al., 1997). Besides these environmental effects, mathematical models show how the mismatch between the dispersal of both plants and pathogens might be modulating the strength of plant-soil feedbacks (Revilla et al., 2013). These ideas and conceptual framework call for a holistic understanding of the natural history of fungal plant pathogen communities in order to better prevent agricultural losses due to pathogens.
To date most plant disease models have focused on specific populations rather than plant pathogen community-level data (Schmale and Ross, 2015; Borer et al., 2016). Usually, these predictive models map positive occurrences of disease and then relate this information to measurements of environmental factors and host occurrence (Aylor, 2003). For example, the detailed process-based quantitative potato late blight pathogen (Phytophthora infestans) disease forecast model estimates severity as a function of weather (Hijmans et al., 2000). Another example is the aerial transport model of soybean rust (Phakopsora pachyrhizi) from South America to the United States (Isard et al., 2005). Our results do not discount the importance of host occurrence and agricultural practices in predicting specific disease outbreaks or susceptibility, particularly for crops under traditional farming practices, like monoculture and/or high plant density of the same physiological and phenological features, which allow for rapid pathogen coevolution (Savary et al., 2019). As predicted by some of the analytical models, these practices may lead to larger outbreaks, faster crop resistance, and yield instability (Shipton, 1977). While crop diversity and pathogen adaptation play important roles in yield amounts, both production methods and environmental variability certainly contribute to annual variations in crop losses (Scholthof, 2007). Baseline information on the community-level distribution of pathogens in dust might be able to forecast how changes in climate and agricultural practices might result in future outbreaks.
By linking the geographical distribution of multiple plant pathogens across the United States, we were able to delimit five different areas of susceptibility influenced by different sets of fungal pathogens. For example, although Epicoccum nigrum has a global spread, its geographical distribution in dust was closely linked to the Northern partition (Figures 4A,B). This saprophytic fungus is commonly found in dust and grows in cereals and corn (see Figure 4C for the distribution of corn production across the US). Stemphylium solani the causal agent for gray leaf spot in tomatoes has been reported all over the world (Cedeño and Carrero, 1997). However, our results based on molecular signatures in dust suggest that its area of influence in the US is mainly restricted to California. A similar Northwest Coast and California delimited distribution was observed in the widespread saprophytic species Cladosporium tenuissimum (maps can be publicly accessed at http://dx.doi.org/10.6084/m9.figshare.1270900), although this fungus has been isolated specially in tropical areas (Batta, 2004). However, we acknowledge that this study represents a simple snapshot of the fungal plant pathogen inocula in settled dust and these patterns might not reflect seasonal dynamics (Chen et al., 2018). We found some other cases of pathogen geographical distributions completely mismatched that of their potential hosts. For example, Ascochyta hordei map aligns closely to the Southern partition (Figures 4D,E), however its main crop host, barley (Sprague and Johnson, 1950), is primarily in northern border states (Figure 4F). Similarly, other studies have found that potato blight (Phytophthora infestans) occurs wherever potato is grown, but is problematic in a substantially smaller geographic area (Hijmans et al., 2000). This illustrates how some fungi have the potential to reside on a wide range of non-crop plant species as pathogens or saprotrophs (Rai and Agarkar, 2016), and how the natural distribution of fungal plant pathogens is not solely dependent on environment or crops, but there is a combination of factors delimiting their biogeography and potential dispersal.
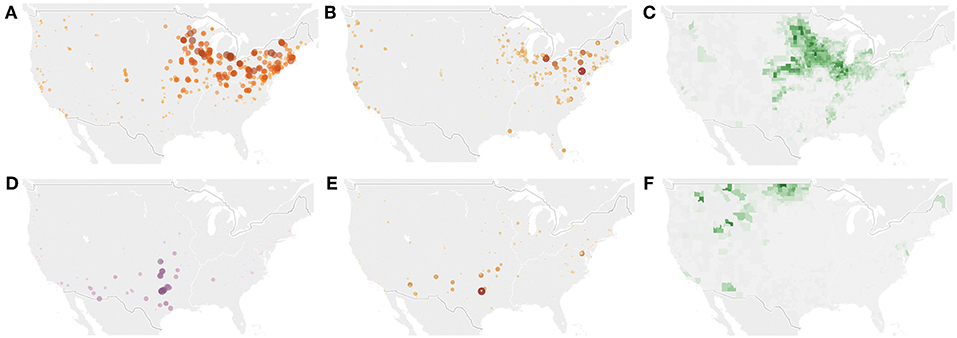
Figure 4. Map of the probability to be associated with the Northern partition (A). Relative abundance of Epicoccum nigrum in dust samples (B). County-level corn production data from the United States Department of Agriculture (USDA) (C). Map of the probability to be associated with the Southern partition (D). Relative abundance of Ascochyta hordei in dust samples (E). County-level barley production data from the United States Department of Agriculture (USDA) (F).
Data Availability
The datasets generated for this study can be found in the figshare (https://figshare.com/articles/1000homes/1270900).
Author Contributions
KD, DV, and JU'R performed data analysis, and wrote the manuscript. NF wrote the manuscript. AB conceived the study, performed data analysis, and wrote the manuscript.
Conflict of Interest Statement
The authors declare that the research was conducted in the absence of any commercial or financial relationships that could be construed as a potential conflict of interest.
Acknowledgments
We thank the volunteers who participated in the Wild Life of Our Homes study for collecting home dust samples. We thank Steph Dietzel for her assistance with Tableau.
Supplementary Material
The Supplementary Material for this article can be found online at: https://www.frontiersin.org/articles/10.3389/fevo.2019.00304/full#supplementary-material
References
Abarenkov, K., Henrik Nilsson, R., Larsson, K.-H., Alexander, I. J., Eberhardt, U., Erland, S., et al. (2010). The UNITE database for molecular identification of fungi - recent updates and future perspectives. N. Phytol. 186, 281–285. doi: 10.1111/j.1469-8137.2009.03160.x
Akimitsu, K., Peever, T. L., and Timmer, L. W. (2003). Molecular, ecological and evolutionary approaches to understanding Alternaria diseases of citrus. Mol. Plant Pathol. 4, 435–446. doi: 10.1046/j.1364-3703.2003.00189.x
Altschul, S. F., Madden, T. L., Schäffer, A. A., Zhang, J., Zhang, Z., Miller, W., et al. (1997). Gapped BLAST and PSI-BLAST: a new generation of protein database search programs. Nucleic Acids Res. 25, 3389–402. doi: 10.1093/nar/25.17.3389
Aylor, D. E. (1986). A framework for examining inter-regional aerial transport of fungal spores. Agric. For. Meteorol. 38, 263–288. doi: 10.1016/0168-1923(86)90017-1
Aylor, D. E. (2003). Spread of plant disease on a continental scale: role of aerial dispersal of pathogens. Ecology 84, 1989–1997. doi: 10.1890/01-0619
Barberán, A., Ladau, J., Leff, J. W., Pollard, K. S., Menninger, H. L., Dunn, R. R., et al. (2015). Continental-scale distributions of dust-associated bacteria and fungi. Proc. Natl. Acad. Sci. U.S.A. 112, 5756–5761. doi: 10.1073/pnas.1420815112
Batta, Y. A. (2004). Cladosporium tenuissimum Cooke (Deuteromycotina: Hyphomycetes) as a causal organism of new disease on cucumber fruits. Eur. J. Plant Pathol. 110, 1003–1009. doi: 10.1007/s10658-004-1604-7
Bever, J. D., Mangan, S. A., and Alexander, H. M. (2015). Maintenance of plant species diversity by pathogens. Annu. Rev. Ecol. Evol. Syst. 46, 305–325. doi: 10.1146/annurev-ecolsys-112414-054306
Borer, E. T., Laine, A.-L., and Seabloom, E. W. (2016). A multiscale approach to plant disease using the metacommunity concept. Annu. Rev. Phytopathol. 54, 397–418. doi: 10.1146/annurev-phyto-080615-095959
Bowden, J., Gregory, P. H., and Johnson, C. G. (1971). Possible wind transport of coffee leaf rust across the Atlantic Ocean. Nature 229, 500–501. doi: 10.1038/229500b0
Brown, J. K. M., and Hovmøller, M. S. (2002). Aerial dispersal of pathogens on the global and continental scales and its impact on plant disease. Science. 297, 537–541. doi: 10.1126/science.1072678
Cedeño, L., and Carrero, C. (1997). First report of tomato gray leaf spot caused by Stemphylium solani in the Andes region of Venezuela. Plant Dis. 81, 1332–1332. doi: 10.1094/PDIS.1997.81.11.1332B
Chen, W., Hambleton, S., Seifert, K. A., Carisse, O., Diarra, M. S., Peters, R. D., et al. (2018). Assessing performance of spore samplers in monitoring aeromycobiota and fungal plant pathogen diversity in Canada. Appl. Environ. Microbiol. 84, e02601–e02617. doi: 10.1128/AEM.02601-17
Edgar, R. C. (2013). UPARSE: highly accurate OTU sequences from microbial amplicon reads. Nat. Methods 10, 996–998. doi: 10.1038/nmeth.2604
Ellis, N., Smith, S. J., and Pitcher, C. R. (2012). Gradient forests: calculating importance gradients on physical predictors. Ecology 93, 156–168. doi: 10.1890/11-0252.1
Fisher, M. C., Henk, D. A., Briggs, C. J., Brownstein, J. S., Madoff, L. C., McCraw, S. L., et al. (2012). Emerging fungal threats to animal, plant and ecosystem health. Nature 484, 186–194. doi: 10.1038/nature10947
Gilland, B. (2002). World population and food supply: can food production keep pace with population growth in the next half-century? Food Policy 27, 47–63. doi: 10.1016/S0306-9192(02)00002-7
Golan, J. J., and Pringle, A. (2017). Long-distance dispersal of fungi. Microbiol. Spectr. 5, 309–333. doi: 10.1128/microbiolspec.FUNK-0047-2016
Gonzalez-Martin, C., Teigell-Perez, N., Valladares, B., and Griffin, D. W. (2014). The global dispersion of pathogenic microorganisms by dust storms and its relevance to agriculture. Adv Agronomy 127, 1–41. doi: 10.1016/B978-0-12-800131-8.00001-7
Gorbushina, A. A., Kort, R., Schulte, A., Lazarus, D., Schnetger, B., Brumsack, H.-J., et al. (2007). Life in Darwin's dust: intercontinental transport and survival of microbes in the nineteenth century. Environ. Microbiol. 9, 2911–2922. doi: 10.1111/j.1462-2920.2007.01461.x
Gostinčar, C. (2011). Evolution of fungal pathogens in domestic environments? Fungal Biol. 115, 1008–1018. doi: 10.1016/j.funbio.2011.03.004
Hijmans, R. J., Forbes, G. A., and Walker, T. S. (2000). Estimating the global severity of potato late blight with GIS-linked disease forecast models. Plant Pathol. 49, 697–705. doi: 10.1046/j.1365-3059.2000.00511.x
Isard, S. A., Gage, S. H., Comtois, P., and Russo, J. M. (2005). Principles of the atmospheric pathway for invasive species applied to soybean rust. Bioscience 55, 851–861. doi: 10.1641/0006-3568(2005)055[0851:POTAPF]2.0.CO;2
Kellogg, C. A., Griffin, D. W., Garrison, V. H., Peak, K. K., Royall, N., Smith, R. R., et al. (2004). Characterization of aerosolized bacteria and fungi from desert dust events in Mali, West Africa. Aerobiologia 20, 99–110. doi: 10.1023/B:AERO.0000032947.88335.bb
Milgroom, M. G., and Lipari, S. E. (1995). Spatial analysis of nuclear and mitochondrial RFLP genotypes in populations of the chestnut blight fungus, Cryphonectria parasitica. Mol. Ecol. 4, 633–642. doi: 10.1111/j.1365-294X.1995.tb00264.x
Mordecai, E. A. (2011). Pathogen impacts on plant communities: unifying theory, concepts, and empirical work. Ecol. Monogr. 81, 429–441. doi: 10.1890/10-2241.1
Ng, K. P., Yew, S. M., Chan, C. L., Soo-Hoo, T. S., Na, S. L., Hassan, H., et al. (2012). Sequencing of Cladosporium sphaerospermum, a Dematiaceous fungus isolated from blood culture. Eukaryot. Cell 11, 705–706. doi: 10.1128/EC.00081-12
Nguyen, N. H., Song, Z., Bates, S. T., Branco, S., Tedersoo, L., Menke, J., et al. (2016). FUNGuild: an open annotation tool for parsing fungal community datasets by ecological guild. Fungal Ecol. 20, 241–248. doi: 10.1016/j.funeco.2015.06.006
Nilsson, R. H., Anslan, S., Bahram, M., Wurzbacher, C., Baldrian, P., and Tedersoo, L. (2019). Mycobiome diversity: high-throughput sequencing and identification of fungi. Nat. Rev. Microbiol. 17, 95–109. doi: 10.1038/s41579-018-0116-y
Parry, M., Rosenzweig, C., Iglesias, A., Livermore, M., and Fischer, G. (2004). Effects of climate change on global food production under SRES emissions and socio-economic scenarios. Glob. Env. Chang. 14, 53–67. doi: 10.1016/j.gloenvcha.2003.10.008
Peay, K. G., Garbelotto, M., and Bruns, T. D. (2010). Evidence of dispersal limitation in soil microorganisms: isolation reduces species richness on mycorrhizal tree islands. Ecology 91, 3631–3640. doi: 10.1890/09-2237.1
Peay, K. G., Schubert, M. G., Nguyen, N. H., and Bruns, T. D. (2012). Measuring ectomycorrhizal fungal dispersal: macroecological patterns driven by microscopic propagules. Mol. Ecol. 21, 4122–4136. doi: 10.1111/j.1365-294X.2012.05666.x
Peres-Neto, P. R., Legendre, P., Dray, S., and Borcard, D. (2006). Variation partitioning of species data matrices: estimation of fractions. Ecology 87, 2614–2625. doi: 10.1890/0012-9658(2006)87[2614:VPOSDM]2.0.CO;2
Rai, M., and Agarkar, G. (2016). Plant–fungal interactions: what triggers the fungi to switch among lifestyles? Crit. Rev. Microbiol. 42, 428–438. doi: 10.3109/1040841X.2014.958052
Reeleder, R. D. (2003). Fungal plant pathogens and soil biodiversity. Can. J. Soil Sci. 83, 331–336. doi: 10.4141/S01-068
Reich, P. B., Walters, M. B., Ellsworth, D. S., Smith, D. P., Branco, S., Glassman, S. I., et al. (1997). From tropics to tundra: global convergence in plant functioning. Proc. Natl. Acad. Sci. U.S.A. 94, 13730–13734. doi: 10.1073/pnas.94.25.13730
Revilla, T. A., Veen, G. F., Eppinga, M. B., and Weissing, F. J. (2013). Plant–soil feedbacks and the coexistence of competing plants. Theor. Ecol. 6, 99–113. doi: 10.1007/s12080-012-0163-3
Rodriguez, R. J., White, J. F. Jr, Arnold, A. E., and Redman, R. S. (2009). Fungal endophytes: diversity and functional roles. New Phytol. 182, 314–330. doi: 10.1111/j.1469-8137.2009.02773.x
Rook, G. A. (2013). Regulation of the immune system by biodiversity from the natural environment: an ecosystem service essential to health. Proc. Natl. Acad. Sci. U.S.A. 110, 18360–18367. doi: 10.1073/pnas.1313731110
Santini, A., Ghelardini, L., De Pace, C., Desprez-Loustau, M. L., Capretti, P., Chandelier, A., et al. (2013). Biogeographical patterns and determinants of invasion by forest pathogens in Europe. N. Phytol. 197, 238–250. doi: 10.1111/j.1469-8137.2012.04364.x
Savary, S., Willocquet, L., Pethybridge, S. J., Esker, P., McRoberts, N., and Nelson, A. (2019). The global burden of pathogens and pests on major food crops. Nat. Ecol. Evol. 3, 430–439. doi: 10.1038/s41559-018-0793-y
Schmale, D. G. III., and Ross, S. D. (2015). Highways in the sky: scales of atmospheric transport of plant pathogens. Annu. Rev. Phytopathol. 53, 591–611. doi: 10.1146/annurev-phyto-080614-115942
Schoch, C. L., Seifert, K. A., Huhndorf, S., Robert, V., Spouge, J. L., André Levesque, C., et al. (2012). Nuclear ribosomal internal transcribed spacer (ITS) region as a universal DNA barcode marker for Fungi. Proc. Natl. Acad. Sci. U.S.A. 109, 6241–6246. doi: 10.1073/pnas.1117018109
Scholthof, K.-B. G. (2007). The disease triangle: pathogens, the environment and society. Nat. Rev. Microbiol. 5, 152–156. doi: 10.1038/nrmicro1596
Shaw, M. W., and Osborne, T. M. (2011). Geographic distribution of plant pathogens in response to climate change. Plant Pathol. 60, 31–43. doi: 10.1111/j.1365-3059.2010.02407.x
Shinn, E. A., Smith, G. W., Prospero, J. M., Betzer, P., Hayes, M. L., Garrison, V., et al. (2000). African dust and the demise of Caribbean coral reefs. Geophys. Res. Lett. 27, 3029–3032. doi: 10.1029/2000GL011599
Shipton, P. J. (1977). Monoculture and soilborne plant pathogens. Annu. Rev. Phytopathol. 15, 387–407. doi: 10.1146/annurev.py.15.090177.002131
Smith, D. J., Timonen, H. J., Jaffe, D. A., Griffin, D. W., Birmele, M. N., Perry, K. D., et al. (2013). Intercontinental dispersal of bacteria and archaea by transpacific winds. Appl. Environ. Microbiol. 79, 1134–1139. doi: 10.1128/AEM.03029-12
Sprague, R., and Johnson, A. G. (1950). Ascochyta leaf spots of cereals and grasses in the United States. Mycologia 42, 523–553. doi: 10.1080/00275514.1950.12017858
Strobl, C., Boulesteix, A., Kneib, T., Augustin, T., and Zeileis, A. (2008). Conditional variable importance for random forests. BMC Bioinformatics. 9:307. doi: 10.1186/1471-2105-9-307
Thrall, P. H., Bever, J. D., Mihail, J. D., and Alexander, H. M. (1997). The population dynamics of annual plants and soil-borne fungal pathogens. J. Ecol. 85, 313–328. doi: 10.2307/2960504
Valle, D., Albuquerque, P., Zhao, Q., Barberán, A., and Fletcher, R. J. (2018). Extending the latent dirichlet allocation model to presence/absence data: a case study on North American breeding birds and biogeographical shifts expected from climate change. Glob. Chang. Biol. 24, 5560–5572. doi: 10.1111/gcb.14412
Wang, Q., Garrity, G. M., Tiedje, J. M., and Cole, J. R. (2007). Naive Bayesian classifier for rapid assignment of rRNA sequences into the new bacterial taxonomy. Appl. Environ. Microbiol. 73, 5261–5267. doi: 10.1128/AEM.00062-07
Wolf, F. T. (1943). The microbiology of the upper air. Bull. Torrey Bot. Club 70, 1–14. doi: 10.2307/2481676
Zalar, P., de Hoog, G. S., Schroers, H.-J., Crous, P. W., Groenewald, J. Z., and Gunde-Cimerman, N. (2007). Phylogeny and ecology of the ubiquitous saprobe Cladosporium sphaerospermum, with descriptions of seven new species from hypersaline environments. Stud. Mycol. 58, 157–183. doi: 10.3114/sim.2007.58.06
Keywords: fungal plant pathogen, dust, dispersal, biogeography, machine learning, crop, agriculture
Citation: Dietzel K, Valle D, Fierer N, U'Ren JM and Barberán A (2019) Geographical Distribution of Fungal Plant Pathogens in Dust Across the United States. Front. Ecol. Evol. 7:304. doi: 10.3389/fevo.2019.00304
Received: 16 May 2019; Accepted: 30 July 2019;
Published: 09 August 2019.
Edited by:
Luciano Bosso, University of Naples Federico II, ItalyReviewed by:
Wen Chen, Agriculture and Agri-Food Canada (AAFC), CanadaCristina Gonzalez-Martin, Universidad de La Laguna, Spain
Copyright © 2019 Dietzel, Valle, Fierer, U'Ren and Barberán. This is an open-access article distributed under the terms of the Creative Commons Attribution License (CC BY). The use, distribution or reproduction in other forums is permitted, provided the original author(s) and the copyright owner(s) are credited and that the original publication in this journal is cited, in accordance with accepted academic practice. No use, distribution or reproduction is permitted which does not comply with these terms.
*Correspondence: Albert Barberán, YmFyYmVyYW5AZW1haWwuYXJpem9uYS5lZHU=