Assessing Pathways of Climate Change Effects in SpaDES: An Application to Boreal Landbirds of Northwest Territories Canada
- 1Faculty of Forestry, The University of British Columbia, Vancouver, BC, Canada
- 2Pacific Forestry Centre, Canadian Forest Service, Natural Resources Canada, Victoria, BC, Canada
- 3School of Environmental Studies, University of Victoria, Victoria, BC, Canada
- 4Department of Wood and Forest Sciences, Laval University, Quebec City, QC, Canada
- 5Canadian Wildlife Service, Environment and Climate Change Canada, Yellowknife, NT, Canada
- 6Department of Renewable Resources, University of Alberta, Edmonton, AB, Canada
- 7Northern Forestry Centre, Canadian Forest Service, Natural Resources Canada, Edmonton, AB, Canada
- 8Wildlife Research Division, Environment and Climate Change Canada, Quebec City, QC, Canada
- 9FOR-CAST Research & Analytics, Calgary, AB, Canada
- 10Landscape Science and Technology Division, Environment and Climate Change Canada, Ottawa, ON, Canada
- 11Canadian Wildlife Service, Environment and Climate Change Canada, Whitehorse, YT, Canada
- 12Department of Biological Sciences, University of Alberta, Edmonton, AB, Canada
- 13Canadian Wildlife Service, Environment and Climate Change Canada, Saskatoon, SK, Canada
- 14Yukon Research Centre, Yukon University, Whitehorse, YT, Canada
Distributions of landbirds in Canadian northern forests are expected to be affected by climate change, but it remains unclear which pathways are responsible for projected climate effects. Determining whether climate change acts indirectly through changing fire regimes and/or vegetation dynamics, or directly through changes in climatic suitability may allow land managers to address negative trajectories via forest management. We used SpaDES, a novel toolkit built in R that facilitates the implementation of simulation models from different areas of knowledge to develop a simulation experiment for a study area comprising 50 million ha in the Northwest Territories, Canada. Our factorial experiment was designed to contrast climate effects pathways on 64 landbird species using climate-sensitive and non-climate sensitive models for tree growth and mortality, wildfire, and landbirds. Climate-change effects were predicted to increase suitable habitat for 73% of species, resulting in average net gain of 7.49 million ha across species. We observed higher species turnover in the northeastern, south-central (species loss), and western regions (species gain). Importantly, we found that most of the predicted differences in net area of occupancy across models were attributed to direct climate effects rather than simulated vegetation change, despite a similar relative importance of vegetation and climate variables in landbird models. Even with close to a doubling of annual area burned by 2100, and a 600 kg/ha increase in aboveground tree biomass predicted in this region, differences in landbird net occupancy across models attributed to climate-driven forest growth were very small, likely resulting from differences in the pace of vegetation and climate changes, or vegetation lags. The effect of vegetation lags (i.e., differences from climatic equilibrium) varied across species, resulting in a wide range of changes in landbird distribution, and consequently predicted occupancy, due to climate effects. These findings suggest that hybrid approaches using statistical models and landscape simulation tools could improve wildlife forecasts when future uncoupling of vegetation and climate is anticipated. This study lays some of the methodological groundwork for ecological adaptive management using the new platform SpaDES, which allows for iterative forecasting, mixing of modeling paradigms, and tightening connections between data, parameterization, and simulation.
Introduction
The North American boreal forest represents approximately 48% of the continent’s forests (Smith et al., 2018), and it provides breeding habitat for half of North American bird species (Wells and Blancher, 2011). In recent decades, the southern boreal forest has seen rapid economic development from forestry, agriculture, oil, bitumen and gas, with attendant consequences for the region’s biodiversity (Hebblewhite, 2017; Mahon et al., 2019; Stewart et al., 2020). However, in the northern parts of the boreal forest, climate change is perhaps the greatest threat to biodiversity (Price et al., 2013). The region is warming at twice the global average rate (Masson-Delmotte et al., 2019), which is expected to increase the frequency, extent, and severity of droughts and wildfires (Boulanger et al., 2014; Gauthier et al., 2015; Masson-Delmotte et al., 2019). An overall decrease in tree productivity and total biomass may be expected in these northern regions (Boulanger et al., 2017), with projected shifts from mid- and late-successional conifers (Picea spp.) to fire-adapted pioneer species of genus Populus and Pinus. Major vegetation transitions of this nature have been documented (Wang et al., 2020), with a combination of frequent fire and drought (Whitman et al., 2019) as well as permafrost thaw (Helbig et al., 2016) potentially playing major roles. As a result, climate change may alter the diversity and abundance of many terrestrial taxa (Dawson et al., 2011), including landbirds (Stralberg et al., 2015a; Cadieux et al., 2020).
More than half of boreal landbird species have shown declines in abundance over the last five decades (Rosenberg et al., 2019) and climate change may exacerbate these trends. According to Stralberg et al. (2015a), projected changes in end-of-century breeding habitat for boreal landbirds range from an average 40% change based on bioclimatic models alone (with 45% of species decreasing), to a projected 20–60% contraction when considering vegetation lags due to climate change (70–100% of species decreasing). Similarly, Bateman et al. (2020a) estimated that 98% of 48 modeled boreal landbird species were at moderate to high risk of range size contraction due to climate change, based on bioclimatic niche models. Climate change may cause range and/or population changes through several mechanisms, with different implications for management. Climate change might act indirectly, through climate-induced changes to vegetation or wildfire and resulting change to habitat, or directly, through non-vegetation mediated climatic effects. The relative importance of these pathways has important implications for management. For example, if climate-caused declines are indirect, land managers can use tree-planting, vegetation restoration, or fuel limitation as on the-ground adaptation strategies to reduce the system’s vulnerability to the effects of climate change (Bauduin et al., 2020), and consequently improve conservation. If climate-caused declines are direct, more drastic adaptation actions such as species translocation, as well as mitigation strategies that can change climate trajectories in the short-term may be needed to meet conservation objectives. Quantifying the relative importance of these alternate pathways is a matter of urgency given current and projected declines in boreal landbirds (Tremblay et al., 2018; Stralberg et al., 2019; Cadieux et al., 2020).
Separating direct from indirect pathways of climate effects poses a significant challenge for standard statistical modeling approaches to both predicting and forecasting wildlife distribution. Interactions and feedback loops (or simply “feedbacks”) among different drivers of change are not yet fully understood, but have the potential to greatly influence outcomes (Bush and Lemmen, 2019; Wilcox et al., 2019; Turetsky et al., 2020). It has been shown, for example, that the forecasted direction of species’ movements under climate change in some cases suggests northern retractions rather than the expected expansions (e.g., Clason et al., 2020). Our current ability to forecast how the various pathways of climate may affect landbird occupancy in boreal forests is limited. Landbird projections to date have been mostly based on statistical correlations between landbird abundance and climate and vegetation covariates, without including feedbacks between climate, habitat, and disturbance regimes (but see Tremblay et al., 2018; Cadieux et al., 2019, 2020). More realistic near-term forecasts can come from dynamic simulations where ecological elements can interact during the forecast period. Interactions and feedbacks could allow, for example, annual vegetation changes to affect annual wildfire forecasts, which in turn affect subsequent vegetation (Marchal et al., 2017a,b, 2019). Absent this, forecasts may diverge too greatly from the upcoming future, potentially resulting in ineffective management actions and misapplication of scarce conservation resources. To address such challenges in the field of predictive ecology (Peters, 1982; Clark, 2001; Dietze et al., 2018; Yates et al., 2018; Dietze and Lynch, 2019; White et al., 2019) and conservation biology (Travers et al., 2019), and improve both uncertainty assessment and conservation resource allocation, the ability to integrate dynamic simulation models such as of forest growth and mortality, wildfire, and wildlife distribution is essential. Spatial Discrete Event Simulation (SpaDES) has been developed with such purposes in mind (Chubaty and McIntire, 2021; McIntire et al., 2021).
Spatial Discrete Event Simulation is a platform that facilitates the use of the PERFICT approach, which stands for Predictive Ecology that is built on the concepts of Reusability, Free availability, and Interoperability of models, which are built around a Continuous workflow, and Tested automatically (McIntire et al., 2021). In a nutshell, the SpaDES platform allows wrapping models (typically implemented in R scripts) into modules and schedules the execution of these modules, allowing for different models (e.g., from siloed areas of knowledge) to interact through their shared inputs and outputs. For example, both forest age and species composition influence the estimation of wildfire parameters (i.e., ignition, escape, and spread probabilities), which in turn, are used to simulate landscape burning modifying both the forest age and its composition (i.e., via serotiny). Continuing the simulation, the new forest composition and age modify the following year’s fire parameter probabilities. Species distribution models are then influenced by the forest composition and potentially other parameters for each simulation year, and can eventually also play a role in modifying both forest growth and wildfire regimes if biological processes such as seed dispersal are modeled. Because SpaDES is implemented in R, it allows tight coupling of data import, processing, modeling, and analyses with simulation components.
Focusing on the Taiga Plains ecozone within the Northwest Territories, Canada, we used this novel and dynamic simulation platform to (i) begin teasing apart the individual effects of direct and indirect climate-change pathways, and (ii) forecast the combined direct and indirect effects of climate pathways, including their interactions and feedbacks, on landbirds. To answer such complex ecological forecasting questions, we developed a simulation experiment of climate sensitive/non-climate sensitive models for (i) forest growth, (ii) wildfire, and (iii) landbird densities. We used existing modules for forest growth and wildfire and wrapped the landbirds’ statistical models into a module, as described below. Considering the large simulated effects of climate on both vegetation (Boulanger et al., 2017) and wildfire regime in several boreal regions (Boulanger et al., 2014; Gauthier et al., 2015; Masson-Delmotte et al., 2019; Cadieux et al., 2020), we anticipated that all pathways would contribute substantially to the effect of climate on landbird species forecasted occupancy. Using SpaDES allowed us to integrate statistical and geospatial simulations to generate tightly coupled data-simulation forecasts covering large spatial and temporal extents (McIntire et al., 2021) to begin investigating the pathways through which climate change will affect boreal landbird species.
Materials and Methods
Study Area and Species
The Taiga Plains ecozone within the Northwest Territories is located in northwestern Canada, and encompasses approximately 50 million ha (Supplementary Appendix I Figure A1). This vast area is primarily composed of upland (50%) and lowland (15%) boreal forests, intermixed with many lakes and small waterbodies (15%), with the remaining 20% land cover composed mostly of low vegetation (i.e., shrubs, herbs, barren ground), ice, and human development (Ecosystem Classification Group, 2007). The predominant tree species are black spruce (Picea mariana), white spruce (Picea glauca), jack pine (Pinus banksiana), paper birch (Betula papyrifera), trembling aspen (Populus tremuloides), and tamarack (Larix laricina) (Ecosystem Classification Group, 2007).
The Northwest Territories hosts nearly 300 breeding landbird species (Lepage, 2019), with twenty of these considered Species at Risk (SAR) at the federal and/or territorial government levels (GNWT, 2019). Our present analysis focused on 64 of these landbird species (Table 1), including two SAR. The species selected for modeling were chosen primarily based on the availability of survey data within the Northwest Territories and the availability of density offsets (Sólymos et al., 2013) to standardize point-count survey estimates (see section “Landbird Models”).
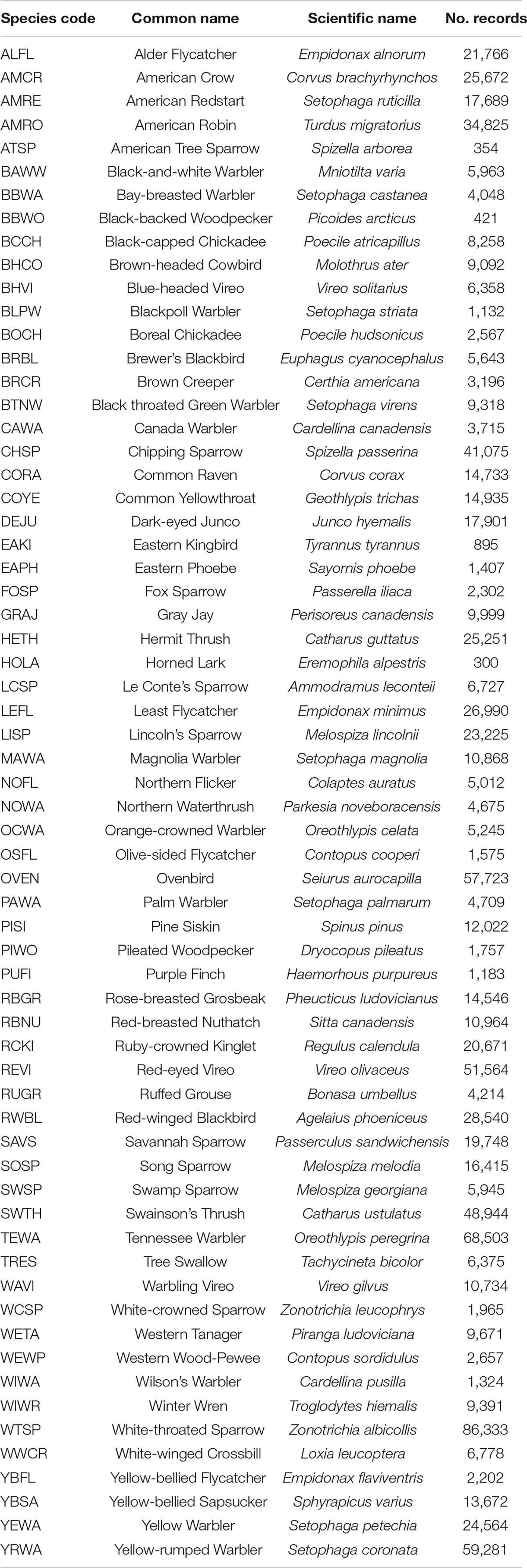
Table 1. Landbird species code, common and scientific names, and the number of records (number of individuals detected in point count surveys) per species in the dataset used to build the landbird statistical models.
Simulation Experiment
We developed statistical and simulation models for boreal landbirds, wildfire, and forest growth using the SpaDES modeling framework (Chubaty and McIntire, 2021; Figure 1), implemented as a suite of packages in R (R Core Team, 2019). Even though many of the drivers used in the geospatial simulation models (specifically LandR-Biomass and FireSense; see below) have been estimated using statistical approaches, we distinguish “simulation models” as iterative, dynamic models whose subsequent iteration depends on the previous state. This describes the vegetation and fire models, but not the “statistical” landbird models, for which predictions are derived solely from the covariates, which may or be dynamic or static, at the time of prediction (e.g., as “simulated” by the fire and vegetation models).
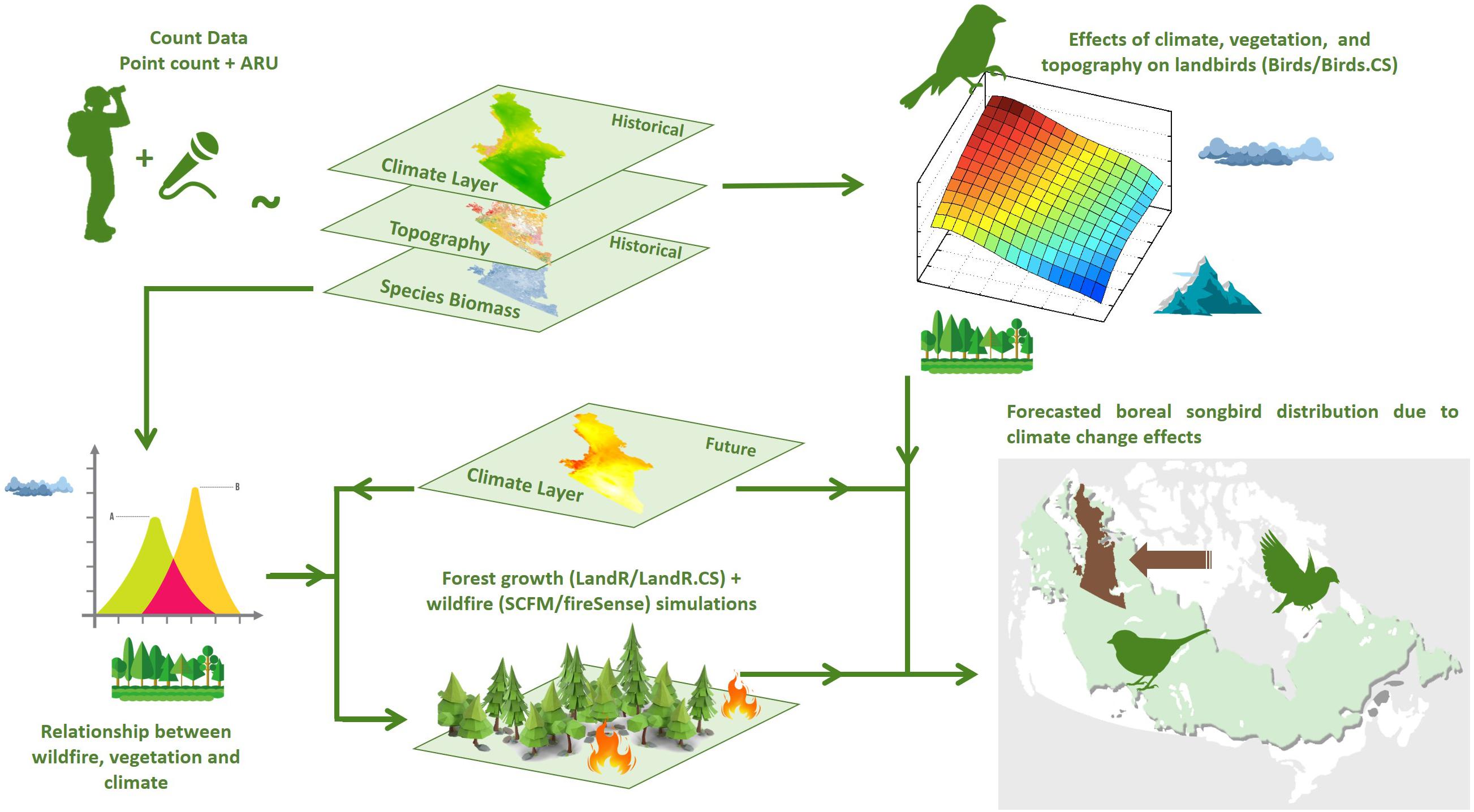
Figure 1. Study framework connecting boreal landbird model (Birds/Birds.CS) fitting, forest growth (LandR/LandR.CS), and wildfire (SCFM/FireSense) simulation and landbird occupancy forecasting. Point-count data to generate landbird models was compiled from the database of the Boreal Avian Modelling Project (Barker et al., 2015), supplemented with data from Automated Recording Units (ARUs; WildTrax, 2019).
We developed or adapted three types of ecological models: (i) forest growth and succession (simulation model); (ii) wildfire (simulation model); and (iii) landbird species densities (statistical models). We developed pairs of each of these three model types: “climate-sensitive” (CS) variants in which climate covariates are explicit, and “non-climate-sensitive” (non-CS) variants where climate covariates are excluded (although climate may act implicitly, e.g., via vegetation covariates), for a total of six models: two forest growth succession models (CS and non-CS), two wildfire models (CS and non-CS), and two landbird density (CS and non-CS). To disentangle climate pathways, we developed a fully factorial simulation experiment involving the three model types with two levels each (CS and non-CS), resulting in 2 × 2 × 2 = 8 treatment combinations (labeled I to VIII; Table 2 and Figure 2). By developing six unique models, we are implementing a “best-in-type” approach to forecasting. Such a novel approach involves building two distinct models (CS and non-CS) for each model type (tree growth, wildfire, and landbird) rather than one “full” model of each type where the non-CS forecast is simply the full model with fixed climate covariates at their mean. The best-in-type approach proposed here partitions more of the variance to non-climate covariates in the non-CS model than it does in its climate-sensitive counterpart, since there is likely some degree of collinearity between these covariates. While some of the extra partitioned variance will be noise, some of it will be a signal incorrectly attributed to the climate covariates in the CS models. This results in a more informative non-CS model than a single “full” model type. A caveat to this approach is that the “control” model (in the present work, the non-CS) is not merely a simplified version of the “experimental” model (in the present work, the CS model with no climate covariates): they are alternative fits to data with different assumptions. As discussed below, we focused on assessing the pathways of climate change effects on landbirds (i.e., differences between models) rather than exploring forecasted climate-sensitive landbird occupancy (i.e., forecasts of one model). In this case, using a “full control model” that would only include factors that are not changing over time (i.e., our non-CS model I with included static climate variables) would result in mostly unchanging predictions, which are not relevant for the purpose of this study. Therefore, while our control model could include climate variables, it would not present different outcomes from the one used. Our used approach has the advantage of allowing researchers to reuse models from any arbitrary source, regardless of the area of research and method used, e.g., computer simulation or machine learning, in this case.
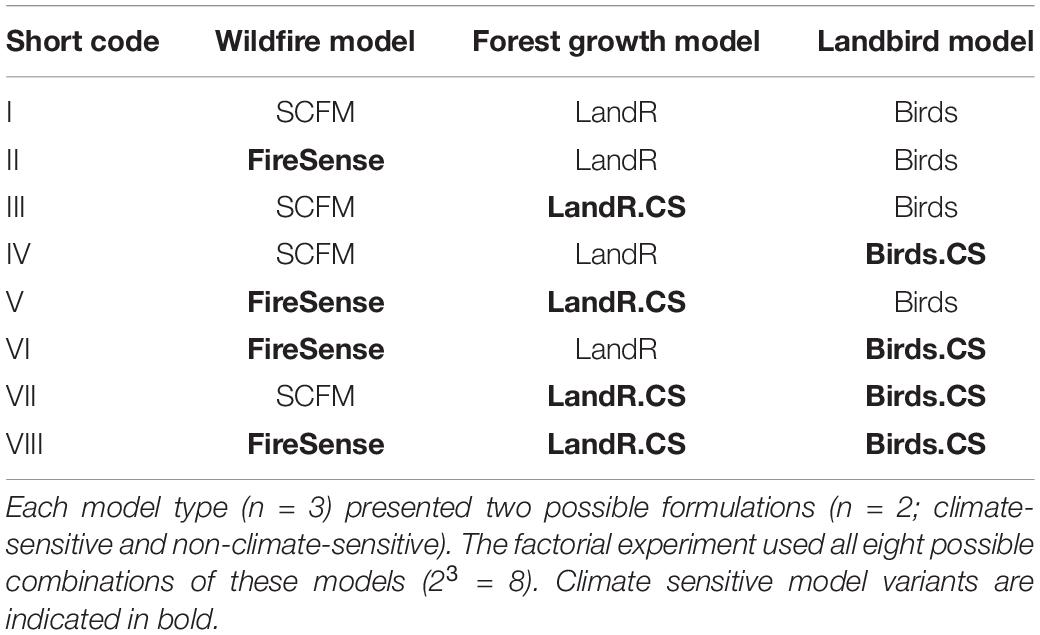
Table 2. Wildfire, forest growth and landbird models used in a factorial simulation experiment to explore the pathways through which climate change might drive responses and forecast the net effect of climate change on different boreal landbird species.
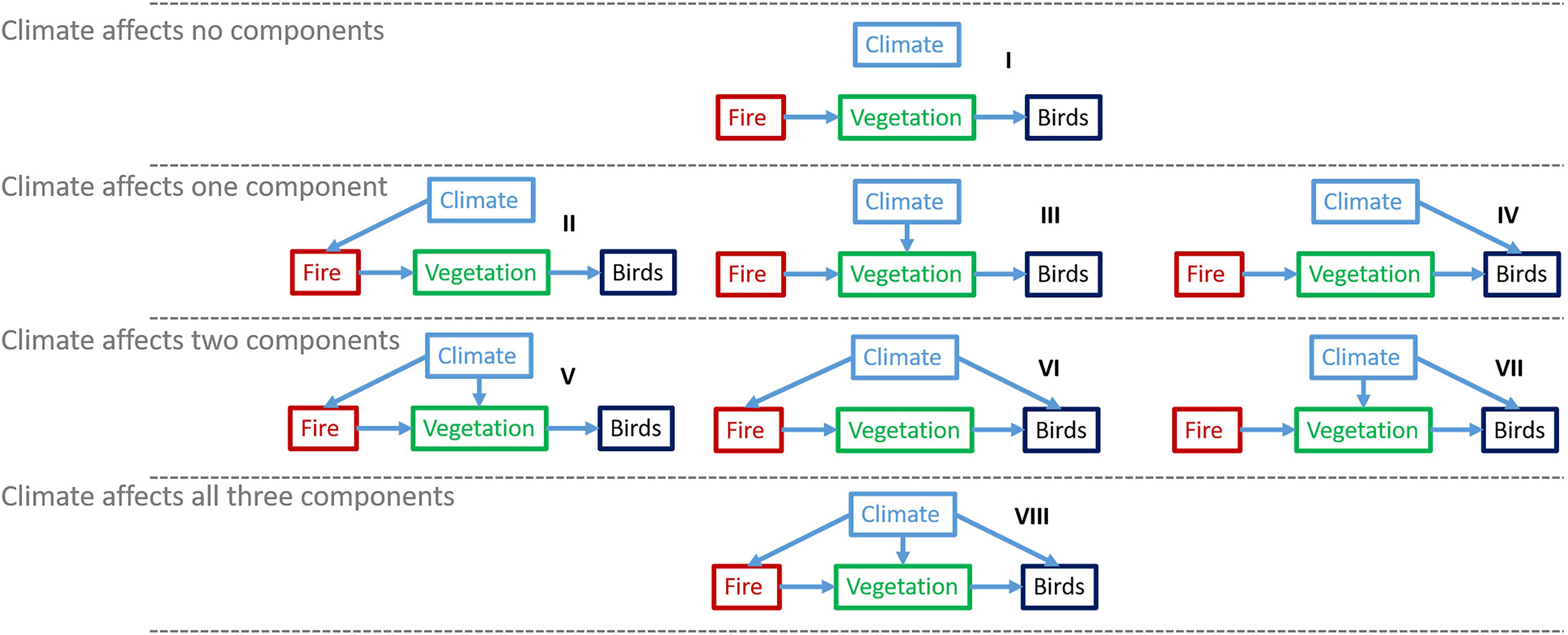
Figure 2. Framework of the factorial experiment of vegetation (green), wildfire (red), and landbird (black) models. This factorial experiment covers the eight possible combinations of the two forms of the three models. The arrows represent the pathways via which climate might affect landbirds (“Birds”). Each graphical representation of the model combinations used can be matched with the model numbers (roman numbers) in Table 2.
We ran 90-year simulations at an annual time step from 2011 through 2100. We used a common spatial resolution of 250 m across all models to align with the resolution of the input stand biomass layers. For each of the eight treatment combinations, we ran 10 replicate simulations to incorporate the inherent stochasticity of the wildfire and vegetation dynamics models. Finally, for each component of this study we have selected, to our knowledge, the best available models given the available data and process models, and our objectives.
Climate Data
We obtained historical climate normals (1981–2010) from ClimateNA (Wang et al., 2016) and https://adaptwest.databasin.org/ to match as closely as possible the years of data in each of the vegetation, fire and bird databases and used these for parameterizing each of the climate-sensitive components of the respective models. We obtained annual (2011–2100) climate projection layers, downscaled to a 1-km grid cell resolution using the ClimateNA software, from forecasts of the Community Climate System Model version 4 (CCSM4) general circulation model from the U.S. National Center for Climate Research. CCSM4 is a coupled climate model that simulates earth’s climate system. It was chosen due to its high spatial resolution (0.9424° latitude, 1.2500° longitude) and because it has shown good overall performance in other regions when compared to other climate models with available annual forecasts (Ahmed et al., 2019) and its averaged precipitation and temperature changes are is the closest to the ensemble model for our study region (Fajardo et al., 2020). We used climate forecasts under Representative Concentration Pathway (RCP) 8.5, which may be considered as a worst-case scenario of climate change for 2100 based on a continuation of current policies and economic incentives (Hausfather and Peters, 2020). We chose RCP 8.5 as evidence suggests that the average increase in temperature in the present century will likely exceed RCP 4.5 (Sherwood et al., 2020), supporting instead the use of RCP 8.5 for forecasts (Schwalm et al., 2020). We focus our current work on assessing uncertainty due to climate effect pathways, rather than other sources of uncertainty, such as choice of climate model or emissions scenario. The climate data was post-processed to match the resolution of the vegetation layers by applying a bilinear interpolation using the function postProcess from the reproducible R package (McIntire and Chubaty, 2021).
Models
Forest Growth and Succession Models
For non-CS and CS forest growth and succession models we used the LandR Biomass and LandR.CS model suites, respectively. The LandR Biomass (“LandR” hereafter) model (Supplementary Appendix II), is an implementation of the LANDIS-II biomass succession model (v3.2.1; Scheller and Mladenoff, 2004) in R. LandR has some minor model algorithm differences compared to LANDIS-II, notably simultaneous within-year growth across cohorts, rather than sequential. As with LANDIS-II, there are many tree species-level traits to parameterize. We parameterized tree species’ growth curve and mortality shape based on permanent sample plot data (Supplementary Appendix II and Acknowledgments Section). For other species-level traits (longevity, sexual maturity, ability to resprout), we used the best available estimates from the literature and trait values used in LANDIS-II applications (Supplementary Appendix II). Briefly, during simulations using LandR, one or more cohorts (defined as the biomass of a given tree species with a given age in a pixel) is created and maintained as a non-linear function of growth and mortality equations (i.e., growth curves and within-pixel competition), dispersal dynamics, and responses to disturbances, such as wildfire (i.e., mortality, serotiny, and resprouting; more details in Supplementary Appendix II). The main output from LandR consists of annual tables and maps of the biomass and age of every tree species cohort in every pixel with at least one cohort.
To create the CS version of LandR, we modified cohort growth and mortality equations following the statistical approach developed in Luo et al. (2019). Rather than using their simultaneous multivariate approach, from which we could not correctly predict without developing our own custom statistical routines, we fit two separate univariate models for plot-level biomass growth increment (t/year) and biomass mortality (t/year) as functions of spatially explicit climate variables. Specifically, we first calculated the annual growth and mortality from permanent sample plots (Supplementary Appendix II) located in the Boreal and Taiga Plains ecozone. Even though there are permanent sample plots in the Northwest Territories, the (i) minimum three repeated measurements to fit a statistical model for growth and mortality are still lacking, (ii) there are not enough sampling plots to be modeled without supplementation from other portions of the ecoregion, and most importantly, (iii) there are concerns regarding the time disparity between repeated measures for these permanent sample plots and the reference years from other permanent sample plots. Therefore, we used data from across the southern part of the ecoregion in Alberta and Saskatchewan to be able to model the climate effects on growth and mortality. This also allowed us to encompass some of the warmer future climates that might characterize our study area in the future. We then related plot-level biomass growth increment and mortality to annual temperature anomalies (ATA) and an annual Climate Moisture Index (CMI; Hogg, 1994), accounting for cohort age (sensu Chen et al., 2016). We used a Generalized Linear Mixed Model (GLMM) and a Generalized Additive Model specifying the Location, Scale, and Shape of the data distribution (GAMLSS; gamlss R package; Rigby and Stasinopoulos, 2005), for growth increment and mortality, respectively. We then calculated CMI and ATA using historical monthly temperature and precipitation for summer months (Supplementary Appendix I Figure A2). For each driver (tree growth or tree mortality), we calculated the annual “climate effect ratio” (CER), which is the ratio of the growth (or mortality) under each annual future climate and the growth (or mortality) under the averaged historic climate, for each pixel. We finally multiplied the LandR-derived growth and mortality by this CER. This gives, for example, no change in growth (or mortality) when a future year has the same climate as the historical average, because the ratio is 1. As future climate changes, this ratio can go above or below 1, resulting in deviations from the non-CS stand-level growth (or mortality). We did not apply the CER to cohorts under 20 years of age because they were not represented in the permanent sample plots, lacking information on their climate sensitivity. We, therefore, determined their growth and mortality using the default LANDIS-II equations. We also placed upper and lower limitations on this ratio (1.66 and 1/1.66 = 0.6, respectively, which allows for up to a 66% increase or decrease in growth and mortality) in an attempt to balance two opposing sources of forecasting error: minimizing cases where we deemed that the extrapolation would be too great and not allowing for future climate to have unrealistically severe effects on growth and mortality. In other words, we used a percentile to estimate the effect of climate on growth/mortality for climate sensitive forest growth models, where we divided the current climate effects by reference climate effects. We used these upper and lower limits to circumvent problems caused by very small denominators as well as constraining predictions outside the data range used to generate the model. We selected these thresholds to be slightly outside the range of the most extreme predictions made within the first few decades of projected climate values. We attempted to extract tree species-specific CER, but there were insufficient sample sizes for most species. As a result, we used plot-level growth increment and mortality.
We ran the above dynamic forest growth models for all “treed” pixels, including treed wetlands. Land cover classes modeled were the ones classified as “treed” by Latifovic and Pouliot (2005), i.e., 1–15, 20, 32, 34, 35, and 39. Even though 34 and 35 are classified as burned, we converted those to the nearest-neighbor’s forested class to allow for forest regeneration. We used Beaudoin et al. (2014) dataset as our starting layers for biomass (for details, please refer to Supplementary Appendix II). Data were not supplemented with the Northwest Territory’s forest inventory data due to the small spatial coverage of this dataset in comparison to the size of our study area. We did not simulate dynamic vegetation changes for non-treed locations: non-vegetated, non-treed wetlands, shrublands, herb dominated, grasslands, croplands, and lichen dominated land cover classes, which summed to 35% of the total landscape cover. These were held static during simulations as we did not have access to a sufficiently fine-grained dynamic forest growth model within the study area.
Wildfire Models
We used two landscape fire models, SCFM and FireSense to represent non-CS and CS fire models, respectively. SCFM is a three-stage landscape fire model (Cumming et al., 1998; Armstrong and Cumming, 2003). It simulates wildfire as a process of ignition (fires that ignited at a given time period), escape from ignition pixels to neighboring cells (the binary probability of escaping or extinguishing), and spread from escaped pixels across a rasterized landscape (if a fire escapes, what is the probability it will burn the neighboring cells). This approach is a variant of percolation models (Hargrove et al., 2000). Fires are extinguished when no further spreading occurs. Each of the three stages is probabilistic, and the probabilities were estimated from historical fire data between 1965 and 2016 (Canadian Forest Service, 2019). We considered lightning-caused fires only, as human-caused fires have been negligible within our study area (Canadian Forest Service, 2019). We estimated ignition rate (fires per 100 km2 yr–1 – translated to pixel-level probability of ignition) from all known fires of all sizes. We estimated the pixel-level escape probability as the ratio of fires >1 pixel to the number of fires. We calculated the mean size of escaped (i.e., realized) fires (>1 pixel area burned) from historic fire data obtained from the Canadian National Fire Database (Canadian Forest Service, 2019). We estimated the spread probability using a calibration approach whereby we simulated approximately 100,000 fires with different landscape-constant spread probabilities. We fitted a shape-constrained Generalized Additive Model (Pya and Wood, 2015) to the scatterplot of spread probabilities simulated fire sizes. From this curve, we determined the landscape-specific, pixel-level spread probability that reproduces the historical mean fire size for the landscape. All pixels were either flammable with their given ignition, escape, and spread probabilities (not only treed land cover classes, but also lower vegetation ones) or non-flammable (water bodies, ice and snow, rocks and non-vegetated areas such as human development). The model simulates stand-replacing fires only, and the only influence of climate and vegetation on SCFM is indirect through their influence on the historical fire regime.
FireSense also simulates fires through percolation as described for SCFM, but with ignition, escape, and spread probabilities that vary spatially and temporally as a function of annual fire weather and vegetation. We developed statistical models to predict the simulation parameters from these covariates, using methods adapted from Marchal et al. (2017a,b, 2019). We used Monthly Drought Codes from annual Global Climate Model projections (sensu Bergeron et al., 2010) as annual fire weather covariates. For ignition and escape, we classified the historical and projected landscapes based on land cover classes (Latifovic and Pouliot, 2005), while for spread, the most complex model of the three, we classified the historical and projected landscapes into one of the four land cover classes, following this order: (i) young (<15 years since disturbance), (ii) deciduous leading, (iii) conifer leading, and (iv) other; the pixel “leading” tree species or land cover class is that accounting the largest proportion of total species biomass.
Landbird Models
We used landbird density models fit by the Boreal Avian Modelling (BAM) project (Cumming et al., 2010; Barker et al., 2015) with avian point count data from the Boreal and Taiga Plains ecozone [as represented by Bird Conservation Region 6 (Bird Studies Canada and NABCI, 2014; Supplementary Appendix I Figure A1)]. Data were compiled as part of the BAM project and include data from both publicly available and independent projects within the boreal and hemi-boreal regions of North America. These human-based avian point-count surveys were conducted between 1993 and 2018. The BAM database was supplemented with point-count data from autonomous recording units (ARU) deployed in the Northwest Territories and Alberta between 2012 and 2018 by the Canadian Wildlife Service and the University of Alberta Bioacoustic Unit (WildTrax, 2019). The final database used to fit the bird models comprised 126,621 point-counts (5.5% of which were from ARUs), from a total of 42,612 sampling stations. This resulted in 975,527 individual bird records (Table 1) for the species modeled in this study. Of these point-counts, 63% took place in Alberta, 9% in British Columbia, 11% in Manitoba, 6% in Saskatchewan, and 11% in the Northwest Territories. Even though there is unbalanced spatial coverage due to the challenging nature of data collection in remote Northern forests, the data coming exclusively from the Northwest Territories still comprised almost 4,700 unique sampling stations. To minimize the influence of this spatial data imbalance on the landbird models, we weighted individual point-count observations (counts) according to the inverse of the number of surveys conducted within a 5 km × 5 km window around the survey station (including repeat visits to the same station), thereby down-weighting the influence of individual surveys in heavily sampled areas.
For each landbird species (n = 64; Table 1), we developed two species density models: non-CS (vegetation and terrain covariates only) and CS (vegetation, terrain, and climate covariates). Even though we recognize that the non-CS model is not necessarily insensitive to climate, its effect would be mostly indirect, and we chose to use a consistent naming convention (CS vs. non-CS) for our factorial experiment. These count models were fit as boosted regression trees (BRT) with a Poisson distribution using the gbm.step function in the dismo package (Hijmans et al., 2011), following methods outlined in Stralberg et al. (2015b). We used ten-fold cross-validation to assess model robustness to sampling bias. The covariates (Table 3) used for the bird model fitting were either: (i) assumed static over the simulated period and not allowed to change, e.g., water bodies, wetland, urban/agriculture, water proportion, human development proportion (Latifovic and Pouliot, 2005); (ii) static, i.e., topographic ruggedness (Sappington et al., 2007); or (iii) dynamic and allowed to change, e.g., tree species, biomass, age, and climate covariates. Tree species biomass covariates for fitting the bird models were derived from predicted biomass layers for 2001 or 2011 (Beaudoin et al., 2014, 2017). Pre-2006 sampling events were associated with the 2001 biomass data, whereas events from 2006 and up were associated with the 2011 biomass data. Tree species used for biomass covariates included the main tree species present in the study area: paper birch, tamarack, white spruce, black spruce, jack pine, and trembling aspen.
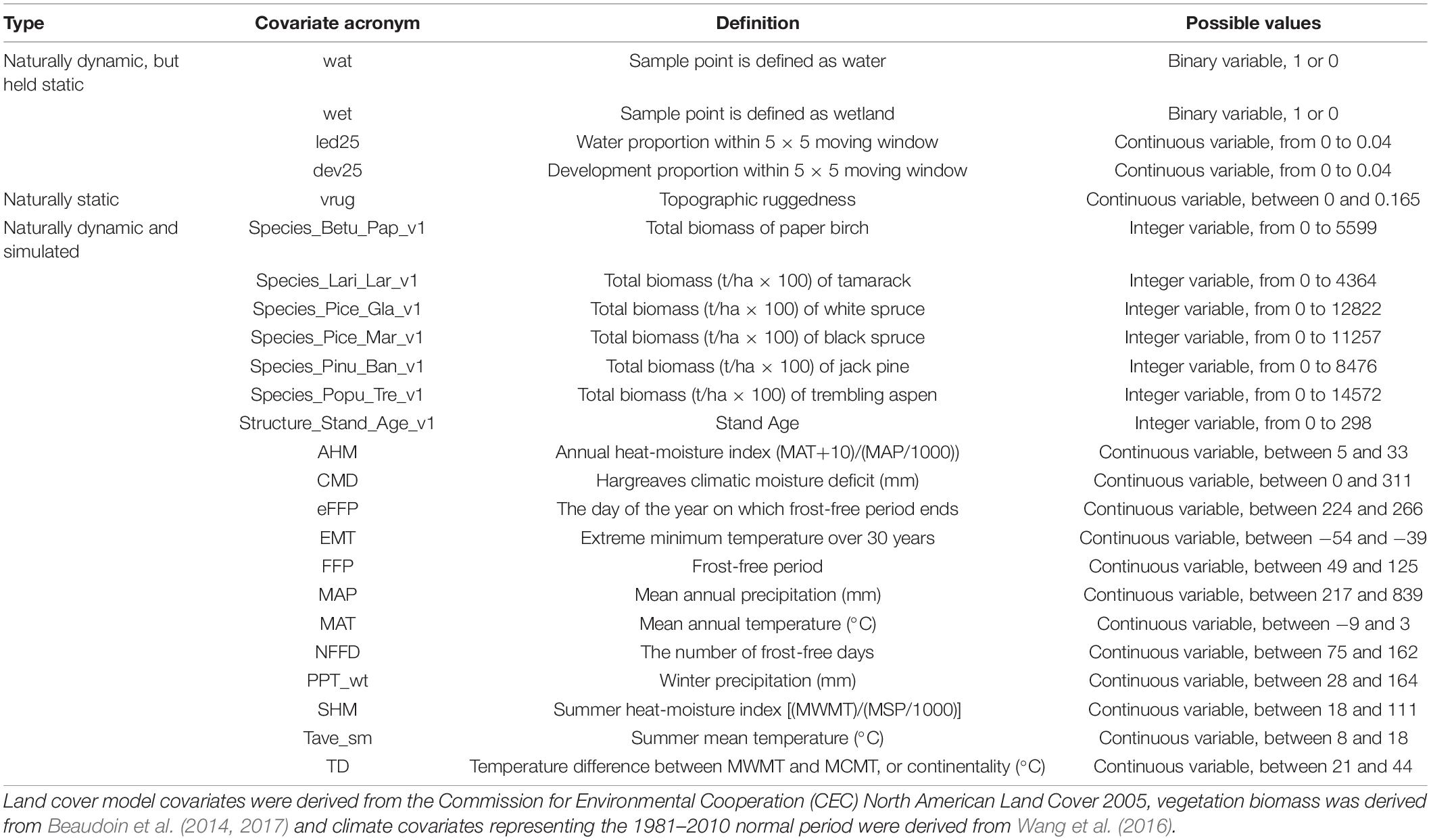
Table 3. Type of covariates, covariate acronyms, definitions, and range of possible values for the covariates used to build the landbird models.
To standardize the landbird density models, we accounted for differences in sampling protocol and covariate effects on landbird species detectability using statistical offsets. This included the effects of time of day and day of year on the probability of availability given presence, and the effects of tree cover and land-cover type on the probability of detection given availability (Sólymos et al., 2013). Offsets were calculated based on removal and distance-sampling models (Sólymos et al., 2018). The adjustments appeared as offsets in the BRTs so that expected values represented species density. We assumed that ARU detectability rates were similar to those of human observers (after Van Wilgenburg et al., 2017; Yip et al., 2017). We assessed potential correlation between predictors using Pearson correlation coefficients between climate, topographic, and vegetation covariates. We did not include covariates that presented stronger correlation than 0.9 with other covariates (sensu Stralberg et al., 2015b).
We also summed relative importance of vegetation and climate variables (i.e., predictors) to assess the combined relative importance of climate vs. vegetation (and assumed-static covariates) in each species’ model. The summed relative importance for climate predictors was compared with the net effect on occupancy (see below) using linear regression.
Quantifying Direct and Indirect Climate Effects on Landbird Occupancy
Our analyses were based on changes in mapped predicted densities within the study region, over the course of a simulation. Predicted densities in 2011 included large areas of very low (effectively zero) densities, outside of species’ known distributions (BirdLife International, 2021), as well as very small areas with very high densities (up to three orders of magnitude higher densities than average). This increased the complexity of the estimation of density changes between scenarios. To address this, we converted species’ density maps into occupancy maps by applying species-specific density thresholds defined by historically identified high density areas, i.e., areas where predicted densities exceeded the mean predicted density within the model-building area (sensu Stralberg et al., 2015a). Although the thresholds used to define occupancy may affect pixel-level changes, the relative magnitudes of direct, indirect, and net climatic effects are likely insensitive to the choice of threshold. Changes in thresholds used to define occupancy would likely modify the magnitude of change in occupancy, but not the qualitative differences in change observed among the three pathways. We generated these occupancy maps for 2011 and 2100, for each species, treatment, and replicate resulting in 160 maps per species (2 years × 8 treatments × 10 replicates).
For each landbird species, treatment and replicate, we subtracted the 2011 occupancy map from the 2100 occupancy map. The resulting 80 maps representing the changes in occupancy for each of the 64 species had pixels coded 1, −1, or 0, depending whether a pixel changed from 0 to 1 (gain of the given species in that pixel), changed from 1 to 0 (loss of the given species in that pixel), or did not change, respectively. For each pathway effect (direct, indirect via vegetation, indirect via fire), we stacked the 80 maps into two groups (CS and non-CS), resulting in 40 maps per pathway effect of the CS model versions, and 40 per pathway effect of the non-CS versions (i.e., vegetation pathway – CS group = III, V, VII, VIII and non-CS group = I, II, IV, VI; fire pathway – CS group = II, V, VI, VIII and non-CS group = I, III, IV, VII; direct pathway – CS group = IV, VI, VII, VIII and non-CS group = I, II, III, V; see Table 2 and Figure 2). For estimating the net effect of climate, i.e., cumulative and additive effects of climate change pathways, we only used the full CS (Scenario VIII) and the full non-CS (Scenario I) models, which resulted in two groups of 10 maps per species. We then estimated the per-pixel probabilities of gain and loss for each of these groups (two additional maps), separately:
Pgain = number of replicates with gain/total number of replicates (i.e., either 40 if individual pathway effects, or 10 for the net effects)
Ploss = number of replicates with loss/total number of replicates
This resulted in 16 maps per species: one gain and one loss of occupied area maps for each of the two climate grouping, CS and non-CS, for each of the four pathway and net effects [(i) direct, (ii) indirect via vegetation, (iii) indirect via fire, and (iv) net effect, i.e., 2 × 2 × 4]. For each species, we multiplied probabilities by pixel area to estimate the mean areas (in ha) of species gain and loss per pathway. Then, for each species we subtracted areas of species loss from areas of species gain, to estimate the total climate effect on occupancy for each pathway. A schematic representation of this process is found in Figure 3. Information regarding code and data to reproduce the analysis can be found in a GitHub repository1. Finally, we mapped the change in the number of species (i.e., species turnover) between 2100 and 2011 due to net climate effects by calculating the difference in occupancy between the full CS (VIII) and full non-CS (I) models across species (Figure 2).
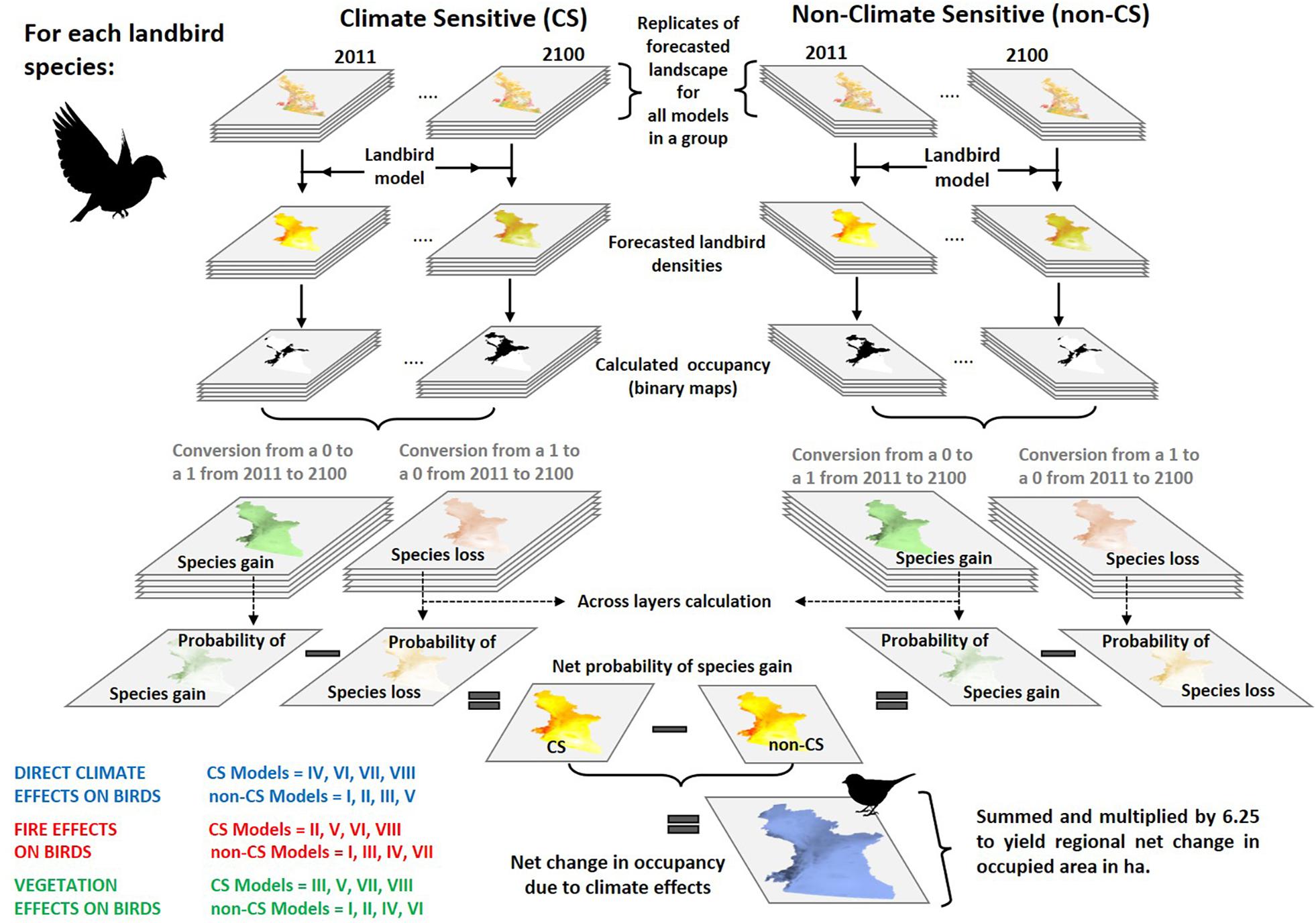
Figure 3. Scheme describing the steps taken to calculate change in occupancy of landbird species by direct and indirect climate effects (summarized in Figure 6) from the forecasted landscape.
Results
Landbird Model Performance and Variable Importance
Model performance varied widely across landbird species and models. Pseudo-R2 values ranged from 0.02 (WIWA) to 0.459 (SAVS) for non-climate sensitive models, and from 0.079 (NOFL) to 0.705 (ATSP) for climate-sensitive models (Supplementary Appendix III). The total relative importance of vegetation variables averaged 59% in non-climate-sensitive models (with a mean pseudo-R2 of 0.21) and 24% in climate-sensitive models (with a mean pseudo-R2 of 0.25; Supplementary Appendix III). Climate-sensitive landbird models showed widely varying relative importance of climatic variables, ranging from 19% (OVEN) to 91% (WCSP) across species (Supplementary Appendix I Figure A3 and Supplementary Appendix III).
Climate Effects on Forest Growth and Mortality and Wildfire
The CS forest growth simulation model showed a net increase in tree biomass of 400 kg/ha (15.4%; Figure 4) compared to results from the non-CS simulation model. CS fire simulations alone resulted in a net increase in tree biomass of 100 kg/ha, representing a 13% increase across the whole study area compared to results from the non-CS fire simulations (Figure 4). Another 100 kg/ha increase can be accounted for by interactions between fire and vegetation in the combined CS models. Thus, the net climate effect on tree biomass was an increase of 600 kg/ha in 2100 (Figure 4). Net climate effects on biomass changes can be spatially seen in Supplementary Appendix I Figure A4. The distribution of pixel-level leading tree species showed fairly modest changes, with higher deciduous conversion in the southeastern portion and more conversion to conifer in the central and eastern portions (Supplementary Appendix I Figure A5), with small increases in black-spruce dominated mixed stands, and small decreases in mixed stands dominated by white spruce and trembling aspen (see Supplementary Appendix I for detailed results).
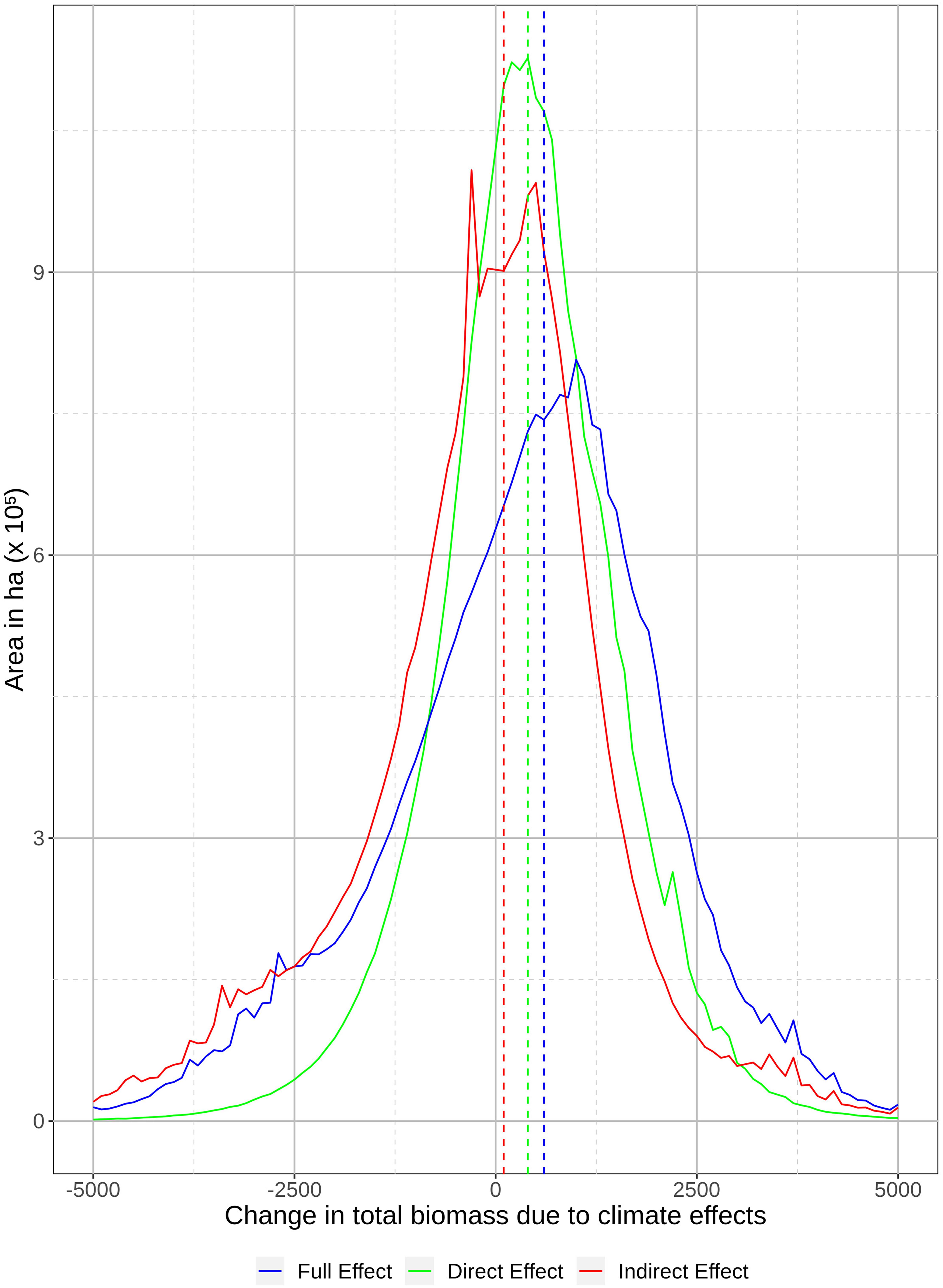
Figure 4. Distribution of area (number of pixels multiplied by resolution) difference of simulated tree species biomass (kg/ha) across models and replicates within the study area in 2100. Dashed lines represent median of biomass differences due to direct climate effects on vegetation (+400 kg/ha; green), indirect effects via fire (+100 kg/ha; red), and the total net effects (+600 kg/ha; blue).
Comparing CS to non-CS fire simulations, mean annual area burned increased by 280 thousand ha in 2100 (Figure 5). This represents a climate-driven increase of almost 90% relative to historical conditions (1965–2016). Given the modest changes in fuel type abundances reported above, this increase is attributable to the direct effects of climate warming on fire activity.
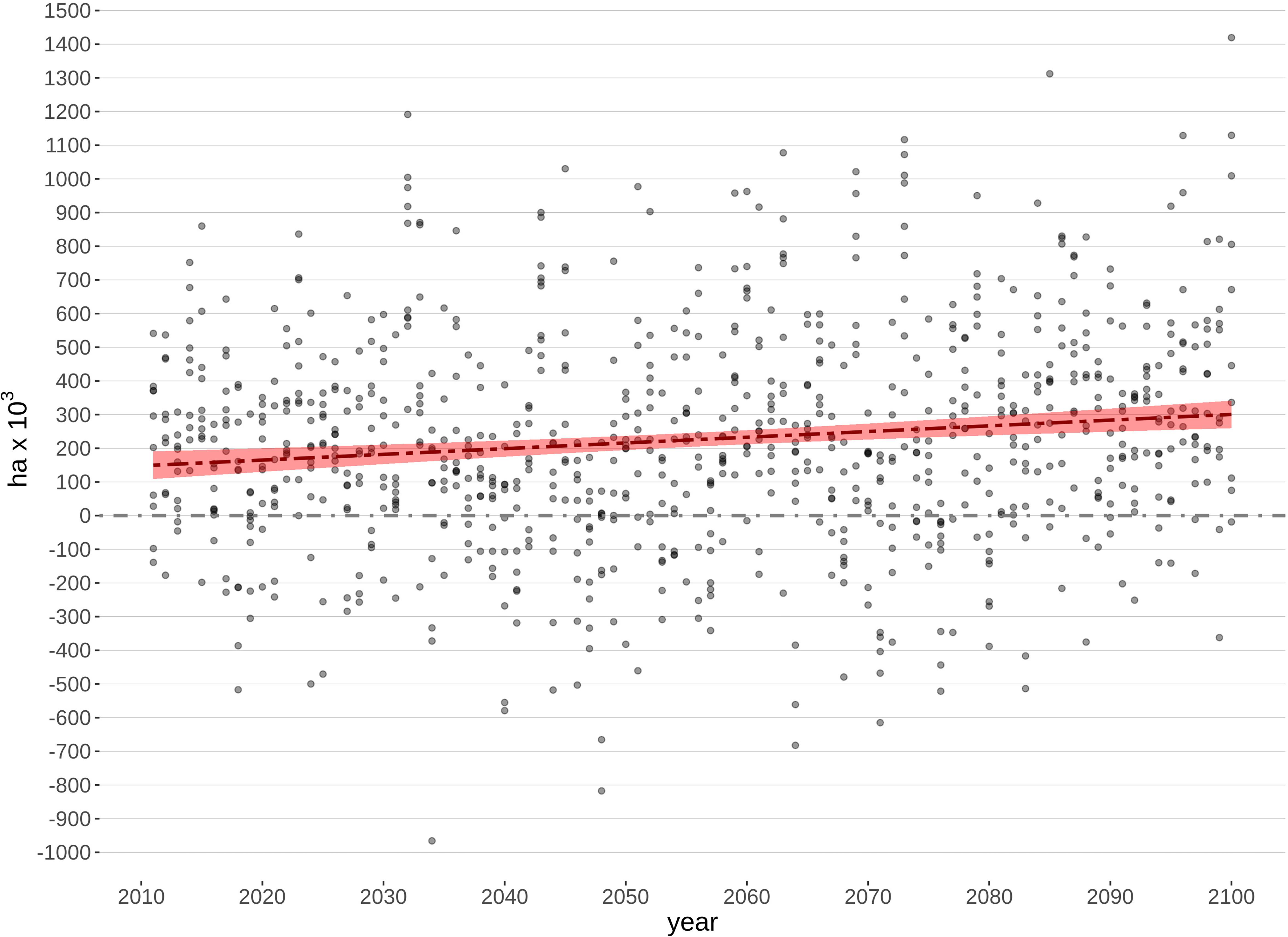
Figure 5. Difference in mean area burned from 2011 to 2100 when simulating landscape change and wildfire between with climate-sensitive and non-climate-sensitive models [Scenarios (II, V, VI, VIII) – (I,III,IV,VII)] for the Taiga Plains Ecozone within the Northwest Territories (dots). The red line represents the fitted linear regression of values through time (y = 1.7x – 3258.2; p = 0.00003).
Climate Effects on Landbird Distributions
Climate change was projected to positively affect average landbird occupancy area in the Northwest Territories by about 7.39 million ha. This area represents approximately 15% of the Taiga Plains ecozone within the Northwest Territories (Figure 6A and Supplementary Appendix IV). Over all species, we forecasted a positive mean difference of 7.49 million ha exclusively due to the direct effects of climate change (dashed blue vertical line on Figure 6B), which were partially offset by a negative mean difference of 97 thousand ha due to indirect effects of climate via fire (dashed red vertical line on Figure 6B). Effects of climate via changes in forest growth and mortality were negligible (a positive mean difference of 4,250 ha; dashed green vertical line on Figure 6B). Overall, the direct effect of climate on landbirds was almost two orders of magnitude greater than the indirect effects, based on simulated changes in landbird distributions (Figure 6). We reiterate that results presented here are not simulated forecasts of future occupancy but instead represent the difference between forecasted occupancy with CS and non-CS models. Even though in some cases the present results might match forecasted occupancy (for example, if the forecasts of non-CS models are unchanging through time) the present results (Figure 6) should not be interpreted as future distribution shifts or range expansions and contractions without careful consideration of the control model (model I).
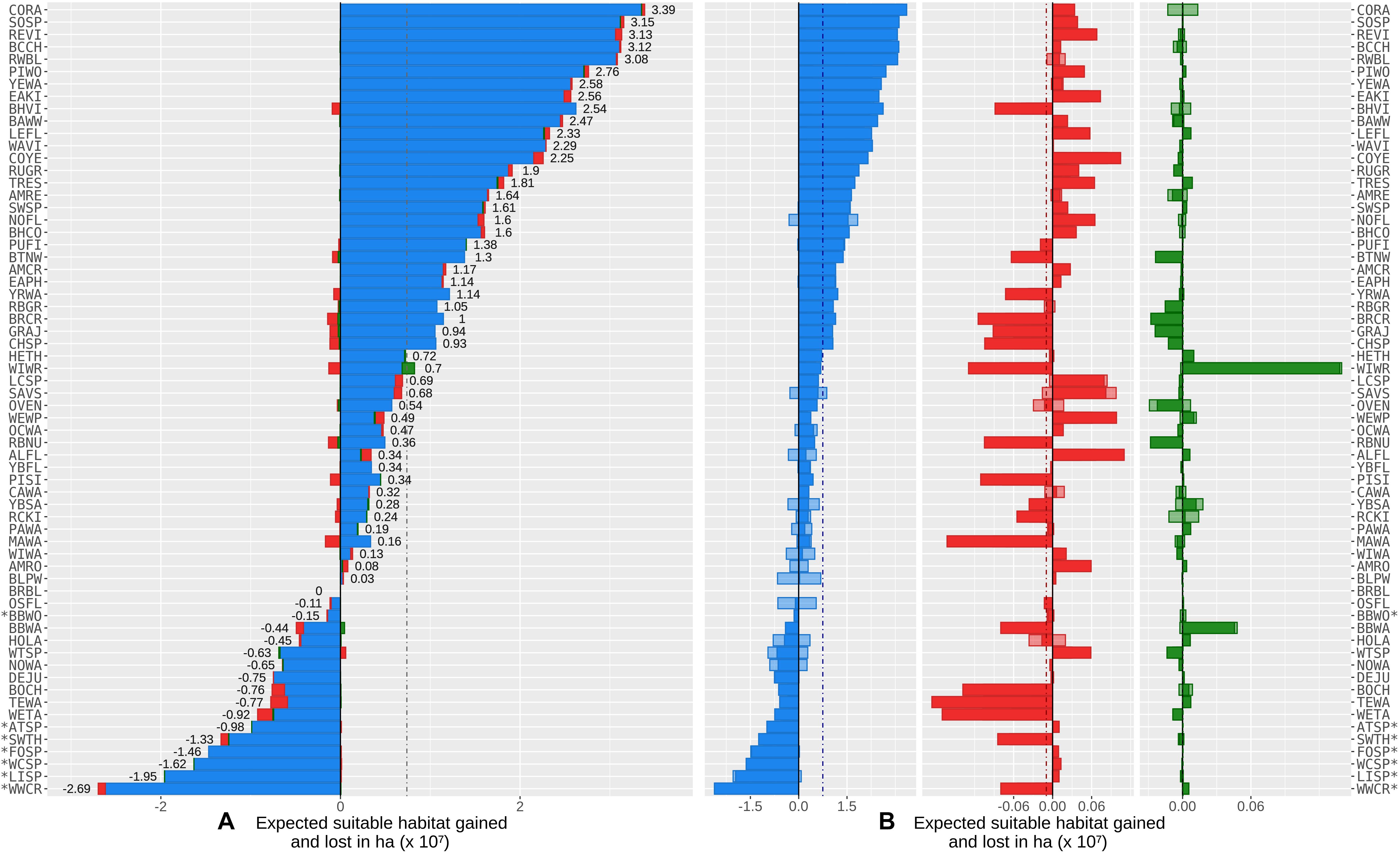
Figure 6. Direct and indirect effects of climate change on the distributions of 64 landbird species within the study area (Supplementary Appendix I Figure A1). Effects are measured as the absolute or relative differences between paired climate-sensitive and non-climate sensitive models (Table 1) in the changes in pixel occupancy between 2011 and 2100, in terms of probabilities calculated over replicates. Left to right, (A) the net direct effects (Scenarios [IV, VI, VII, VIII] – [I, II, III, V], Table 2); blue), and indirect effects via fire (Scenarios [II, V, VI, VIII] – [I, III, IV, VII], Table 2; red) and via forest change (Scenarios [III, V, VII, VIII] – [I, II, IV, VI], Table 2; green). Net effects are the difference between the climate sensitive model and non-climate sensitive model predictions, after calculating the total net area (gained minus lost); (B) light colored bars represent mean expected areas gained (positive) and lost (negative) for each one of the bird species due to direct (blue) and indirect effects of climate (red via fire, green via forest change). Full color bars depict the net effect (gained minus lost) of each of these pathways. The dotted lines represent the average across species. Species for which the net differences in abundance between paired climate-sensitive and non-climate sensitive models (i.e., losses higher than gains) were higher than 90% are marked with an “*”.
Approximately 19% of the landbird species showed both positive and negative direct effects of climate as measured by the gained and lost areas (blue bars on Figures 6A,B). Most species (75%) showed a net area gain to direct effect of climate when comparing variants CS vs. non-CS (Figure 6A), while for 25% there was a net area loss due to direct effects of climate, when making the same comparison. The indirect effect of climate acting through fire or vegetation on landbird distribution varied considerably among species, generating important spatial variation in local gain and loss of occupied area (Figures 6, 7). The net climate effects across species were not correlated with the relative importance of climate variables in the CS models (Figure 8).
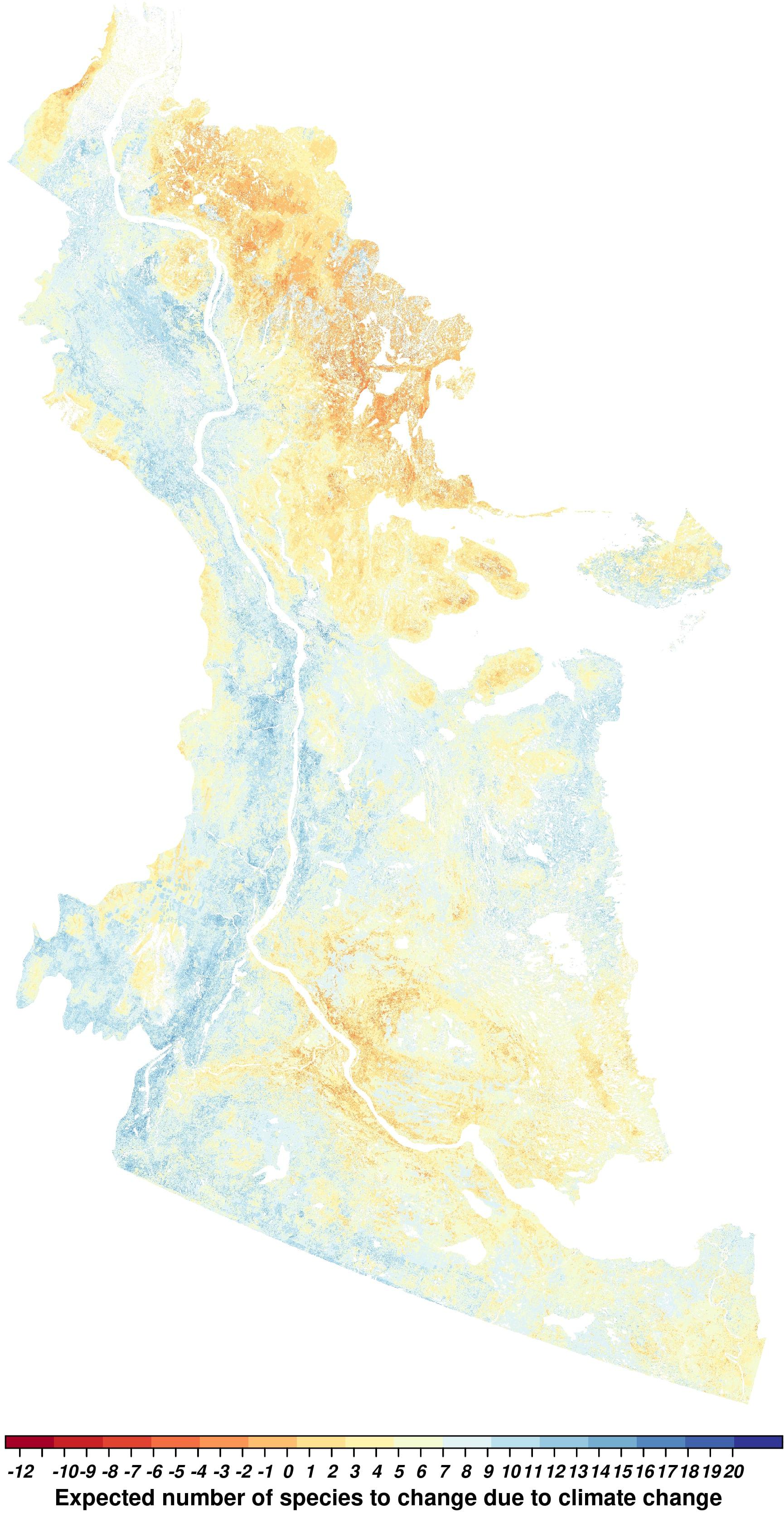
Figure 7. Expected change in the number of species due to net effects of climate change (difference of the change from 2011 to 2100 between full climate-sensitive models and full non-climate-sensitive models) in the Taiga Plains ecozone within the Northwest Territories, Canada. Yellow to red values represent species losses, and yellow to blue values represent species gains.
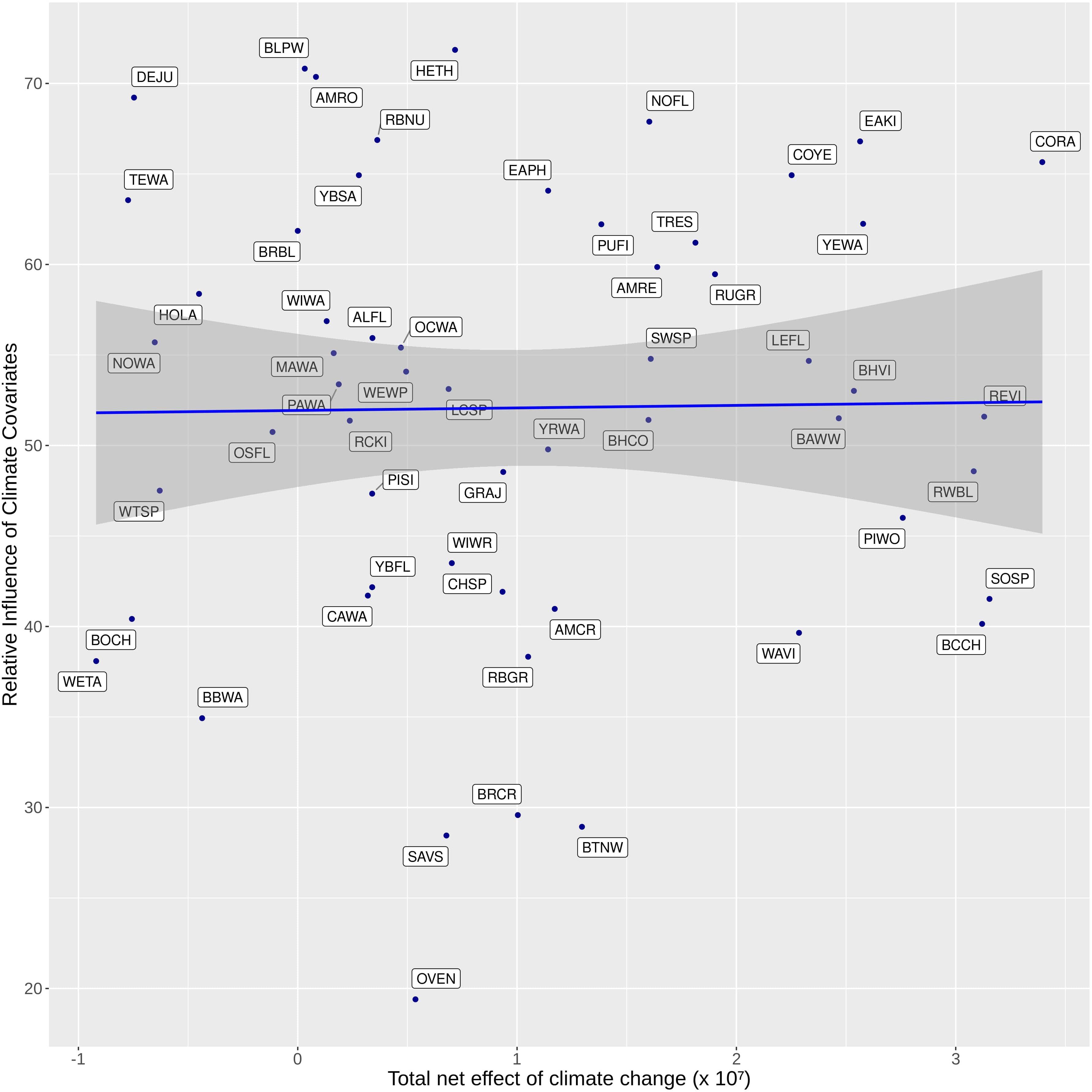
Figure 8. Non-significant correlation (blue line) between relative importance of climate covariates in the climate-sensitive avian species distribution models and total net effect of climate change in terms of area – calculated as the difference of the area affected by climate change calculated between climate-sensitive and non-climate-sensitive predictions in 2100 – for 64 boreal landbird species within the Bird Conservation Region 6 within the Northwest Territories.
The forecasted effect of climate (CS vs. non-CS variants) suggested a net reduction in richness of up to 12 and seven species in the south-central and northeastern regions, respectively (Figure 7). In contrast, the western regions of the study area showed an increase of up to 20 species when comparing the CS vs. non-CS variants. Species turnover was highest in the northeastern, south-central (higher species loss) and western regions (higher species gain) of the study area (Figure 7).
Discussion
Understanding the relative importance of key pathways through which climate change affects ecosystems is important for informing potential adaptation and mitigation measures. The abundance and distribution of landbirds – and wildlife in general – are affected by climate change through the effects of climate on their habitat, more specifically on vegetation, i.e., indirect climate effects (Wisz et al., 2013), but also directly through, e.g., physiological responses to temperature and precipitation (Riddell et al., 2021). Understanding the relative influence of direct and indirect effects enables wildlife managers to identify opportunities to address negative effects of changing climate on valued ecological indicators (Bauduin et al., 2020). We present a novel evaluation of the relative influences of three potential climate-change pathways (vegetation, wildfire, and climate) on future landbird distributions in Northwest Territories, Canada. Direct climate effects were approximately two orders of magnitude more important in explaining predicted changes in landbird occupancy than the indirect pathways. Despite a 90% simulated climate-driven increase in annual area burned by 2100, this did not translate into major vegetation changes across our large study area. Models simulated an average increase of 600 kg/ha in tree biomass, but with only modest changes in leading tree species due to climate change. These results suggest that actions directed at indirect pathways such as wildfire suppression or forest management may not be enough to effectively mitigate landbird species distributional changes under climate change.
In our models, climate drives both forest growth and mortality (i.e., via climate-affected modifiers to these parameters), as well as the probabilities of wildfire ignition, escape and spread. Yet, the indirect effects of climate change on species distribution were still marginal compared to direct effects. This might be explained by vegetation lags. Rapid climate change, accompanied by a relatively slow vegetation change, could result in a state of disequilibrium between climate and vegetation (Wu et al., 2015). Stralberg et al. (2015a) drew attention to the importance of considering lags in vegetation responses to changes in climate when evaluating the effects of climate change on the distributions of boreal landbirds. In theory, the more important the vegetation lag, the more landbird species are likely to experience important range contraction, due to reductions in suitable habitat (Stralberg et al., 2015a). Still, based on the simulated changes in forest growth, mortality, and wildfire regime, our results suggest that vegetation lags may be longer than the simulation time span used in this study, and that direct and/or indirect effects of climate will not necessarily impose important range contractions to most of the 64 species we studied, as previously proposed. Farther south, in Alberta, Cadieux et al.’s (2019) projections suggest larger changes in species composition, reflecting shorter lags in vegetation response to climate change.
Projected shifts in climate and habitat resulted in species distribution patterns with 47 of 64 (73%) of species projected to show an increase in the area they occupy and 16 of 64 (25%) of the species projected to show a decrease in the area occupied within the study area by 2100 when comparing CS vs. non-CS variants. Of the 47 species which net climate effects would increase the occupied area, 72% (34 species) currently have their range centroid south of our study area, supporting previous results projecting northward range shifts of migratory birds (Hitch and Leberg, 2007; Langham et al., 2015; Bateman et al., 2020b; McCaslin and Heath, 2020). Interestingly, these results might point to slower rates of change in mountain ranges, as well as the ability for species to move up slopes to suitable habitats. Species whose models project increases in its habitat extent generally have diverse habitat associations; however, species with the largest net gains (“winners”) are primarily associated with deciduous forests, e.g., Black-capped Chickadee (Poecile atricapillus), Yellow Warbler (Setophaga petechia), Black-and-white Warbler (Mniotilta varia), Least Flycatcher (Empidonax minimus), Warbling Vireo (Vireo gilvus), and Ruffed Grouse (Bonasa umbellus). Species whose models project the largest net losses (“losers”) are species associated with: (1) conifer forest, such as White-winged Crossbill (Loxia leucoptera), Boreal Chickadee (Poecile hudsonicus); (2) non-forested habitats, such as Lincoln’s Sparrow (Melospiza lincolnii), Fox Sparrow (Passerella iliaca); or (3) treeline-tundra habitats such as American Tree Sparrow (Spizelloides arborea), Horned Lark (Eremophila alpestris). These outcomes align with other simulation studies in the western boreal forests (Mahon et al., 2016; Cadieux et al., 2020), and highlight the importance of simulating landscape change with climate sensitive components when forecasting potential future species distributions. Still, two important caveats should be considered when interpreting these results. First, only forested habitats were simulated by the forest growth and mortality model which might influence expected changes in non-forested habitat, and consequently, on landbird species associated with these environments. Second, the extent of current range of landbird species could also influence expected gains and losses for species with narrow ranges within the data set, for example White-crowned Sparrow (Zonotrichia leucophrys). Although this species occurs within the Northwest Territories, it is uncommon across all of the northern region of Alberta, from which much of the data to fit the landbird statistical models was collected.
In light of the highly variable magnitudes of potential vegetation change in the North (e.g., Rehfeldt et al., 2012), further studies should aim to better understand temporal response of both forested and non-forested habitats, and wildfire regime to climate change, including lag responses of vegetation to climate change, and further assess the implications of climate and landscape change on landbird communities.
The lack of a relationship between importance of climate variables in the species distribution models and the net climate change effects in simulation (Figure 8) implies that our forecasted changes in landbird occupancy are not simply a consequence of the strength of climate variables in the CS models. It may be explained by the fact that the predicted densities from a species distribution model are the product of the magnitude of the covariate effect (i.e., the importance of climate variables combined with the explanatory power of the model and the underlying simulated landscape covariates). This result (Figure 8) emphasizes the importance of actually performing forecasts (i.e., simulating landscape changes and forecasting species concurrent changes in occupancy) to understand plausible future outcomes (Dietze et al., 2018); it is not sufficient to infer possible futures directly from coefficients of species distribution models.
We recognize that there may be mismatches between the specific vegetation changes simulated and those captured by the landbird models, and that our results are a direct outcome of the particular forest growth and mortality, and wildfire models used. Our models also do not account for important ecological processes such as permafrost thaw or changes in surface hydrology (Helbig et al., 2016), nor do they capture or simulate extreme weather events (Tanner et al., 2017), that are likely to change with climate. We also could improve our models and obtain more refined landbird forecasts if including other explicit biological processes such as landbirds’ dispersal capacity and potential changes in species interactions due to future climate change (i.e., mismatches in ecological networks). These processes could all alter avian habitat quantity and quality through direct and indirect mechanisms. Our choice of occupancy thresholds for landbirds may also have resulted in over- or under-estimation of species gains, losses, and turnover. However, this should not have influenced the overall patterns observed or our main finding, i.e., relatively small effects of indirect vs. direct climate pathways on landbird occupancy across our study area.
To our knowledge, this study represents the first example of a fully reproducible and reusable modeling workflow, covering every step from acquisition and assimilation of raw data, through the parameter estimation of all statistical models, the specification of simulation experiments, and their execution to generate the samples of forecasts. This study highlights the platform’s ability to actually perform (i) yearly simulation of ecological processes (i.e., forest growth and wildfire) with (ii) existing feedbacks when forecasting species (i.e., landbird) distribution, in contrast to using a scenario based approach for forecasting, which neglects the effects ecological feedbacks among ecological processes, and (iii) on the same platform, as opposed to using one platform for the landscape simulation and another for wildlife modeling (sensu Regos et al., 2018), which improves both model reusability and nimbleness. This study also (iv) allowed us to account for direct effects of climate on both wildfire regimes and forest growth, and (v) represents both the vegetation component with a high level of details (i.e., several species cohorts per pixel), both of which have been previously identified as a limitation of other platforms and models (De Cáceres et al., 2013). Our workflow, using the SpaDES platform, allows for frequent updates and revisions, even to input datasets far upstream from the simulations. Further, at every step, we were able to include expertise of multiple subject experts to update and correct components of different models. We mixed numerous statistical modeling tools (e.g., BRTs, GAMLSS, GLM, GAMS, non-linear mixed effects models) and simulation paradigms (e.g., percolation processes, continuous tree propagule dispersal, forest stand growth modeling), using the best science currently available from each discipline. The SpaDES platform allowed us to integrate these diverse tools in a single data-driven paradigm with reusable modular components (Chubaty and McIntire, 2021; McIntire et al., 2021). This platform is especially well suited to address the new generation of the ecological forecasting paradigm (Dietze et al., 2018; Stall et al., 2019; Bodner et al., 2020) and the implementation of the PERFICT approach (McIntire et al., 2021). This approach can, for example, help reduce model overfitting by enabling faster and iterative re-evaluation and updating of models and model fit. This can also be done, not only by the original model creators but also other researchers, when new data becomes available, speeding up scientific advances. This nimble approach can improve science-based decision making processes, where increasingly complex ecological processes and management objectives can be addressed (McIntire et al., 2021).
This study, and the use of the SpaDES framework, sets the stage for continued testing and development of models and hypotheses to inform land management. The two avian model variants used for this experiment, although not necessarily state-of-the-art models, were appropriate for forecasting because they only required external climate inputs, and tree species composition and age. This made these models particularly well suited for integration with ecological process forecasting, as they did not include covariates for which forecasted data is not available. As with most forecasting studies, there were a large number of ecological processes that were held static or not included in our simulations (e.g., wetland dynamics, permafrost change, extreme events, and anthropogenic disturbances), each of which may have impacts on our estimates of direct versus indirect climate effects on landbirds. A crucial element of our simulation system was the close connection between data and models, and between statistical data analysis and the parameterization of the ecological process models used in the simulation. SpaDES is the only platform, to our knowledge, that could handle our entire workflow. This study – and the models used and developed here – will become part of an iterative, continuously improving forecasting process (sensu Dietze et al., 2018; White et al., 2019). Future development will weave in forecast validation and integrate ecological processes (mentioned above), improving the capacity of these and other models to forecast likely impacts of climatic change and other processes of management concern.
Data Availability Statement
The original contributions presented in the study are included in the article/Supplementary Material, further inquiries can be directed to the corresponding author.
Author Contributions
TM worked on the integration of models and development of the analysis. EM worked on the development of the analysis. DS, RP, SV, ML, CM, EB, SH, JT, and FSc worked on the development and assessment of the landbird-associated components. EM, SC, and IE worked on the development and assessment of wildfire-associated components. EM, CB, IE, and AC worked on the development and assessment of vegetation-associated components. All authors worked on the design, concept, and writing of the manuscript.
Funding
This research was funded by a Discovery Grant of the Natural Sciences and Engineering Research Council of Canada (NSERC) and the Canadian Forest Service ADM Innovation Fund (Natural Resources Canada) to EM, by Strategic Partnership, Discovery and Engage of the Natural Sciences and Engineering Research Council of Canada (NSERC) grants held by SC, and by research contracts awarded to SC and EM by Environment and Climate Change Canada. We acknowledge funding from a Mitacs Accelerate Award No. IT10289 and Mitacs Elevate Fellowship No. IT12391, in partnership with fRI Research, both awarded to CB.
Conflict of Interest
The authors declare that the research was conducted in the absence of any commercial or financial relationships that could be construed as a potential conflict of interest.
Publisher’s Note
All claims expressed in this article are solely those of the authors and do not necessarily represent those of their affiliated organizations, or those of the publisher, the editors and the reviewers. Any product that may be evaluated in this article, or claim that may be made by its manufacturer, is not guaranteed or endorsed by the publisher.
Acknowledgments
This manuscript was enhanced through discussions with the Predictive Ecology research group at the Canadian Forest Service. We gratefully thank J. Marchal for his input and contribution to this integrated workflow. We thank the Alberta Ministry of Agriculture and Forestry and the Saskatchewan Ministry of Environment for collecting and archiving the permanent sample plot datasets. This publication is a contribution of the Boreal Avian Modelling (BAM) Project, an international research collaboration targeting the ecology, management, and conservation of boreal birds. We acknowledge BAM’s members, avian and biophysical Data Partners, and funding agencies (including Environment and Climate Change Canada), listed in full at: www.borealbirds.ualberta.ca/about-us/partners-sponsors. The Canadian Wildlife Service is particularly grateful to many indigenous partners that have granted the permission and helped with landbird surveys conducted on their traditional territories across the Northwest Territories. We also thank the two reviewers for all suggested improvements to this study.
Supplementary Material
The Supplementary Material for this article can be found online at: https://www.frontiersin.org/articles/10.3389/fevo.2021.679673/full#supplementary-material
Footnotes
References
Ahmed, K., Sachindra, D. A., Shahid, S., Demirel, M. C., and Chung, E.-S. (2019). Selection of multi-model ensemble of general circulation models for the simulation of precipitation and maximum and minimum temperature based on spatial assessment metrics. Hydrol. Earth Syst. Sci. 23, 4803–4824. doi: 10.5194/hess-23-4803-2019
Armstrong, G. W., and Cumming, S. G. (2003). Estimating the cost of land base changes due to wildfire using shadow prices. For. Sci. 49, 719–730. doi: 10.1093/forestscience/49.5.719
Barker, N. K. S., Fontaine, P. C., Cumming, S. G., Stralberg, D., Westwood, A., Bayne, E. M., et al. (2015). Ecological monitoring through harmonizing existing data: lessons from the boreal avian modelling project. Wildlife Soc. Bull. 39, 480–487. doi: 10.1002/wsb.567
Bateman, B. L., Taylor, L., Wilsey, C., Wu, J., LeBaron, G. S., and Langham, G. (2020a). Risk to North American birds from climate change-related threats. Conserv. Sci. Pract 2:e243. doi: 10.1111/csp2.243
Bateman, B. L., Wilsey, C., Taylor, L., Wu, J., LeBaron, G. S., and Langham, G. (2020b). North American birds require mitigation and adaptation to reduce vulnerability to climate change. Conserv. Sci. Pract. 2:e242. doi: 10.1111/csp2.242
Bauduin, S., Cumming, S. G., St-Laurent, M.-H., and McIntire, E. J. B. (2020). Integrating functional connectivity in designing networks of protected areas under climate change: a caribou case-study. PLoS One 15:e0238821. doi: 10.1371/journal.pone.0238821
Beaudoin, A., Bernier, P. Y., Guindon, L., Villemaire, P., Guo, X. J., Stinson, G., et al. (2014). Mapping attributes of Canada’s forests at moderate resolution through kNN and MODIS imagery. Can. J. For. Res. 44, 521–532. doi: 10.1139/cjfr-2013-0401
Beaudoin, A., Bernier, P. Y., Villemaire, P., Guindon, L., and Guo, X. (2017). Tracking forest attributes across Canada between 2001 and 2011 using a k nearest neighbors mapping approach applied to MODIS imagery. Can. J. For. Res. 48, 85–93. doi: 10.1139/cjfr-2017-0184
Bergeron, Y., Cyr, D., Girardin, M. P., and Carcaillet, C. (2010). Will climate change drive 21st century burn rates in Canadian boreal forest outside of its natural variability: collating global climate model experiments with sedimentary charcoal data. Int. J. Wildland Fire 19:1127. doi: 10.1071/WF09092
Bird Studies Canada, and NABCI (2014). Bird Conservation Regions. Bird Studies Canada on behalf of the North American Bird Conservation Initiative. Available online at: https://www.birdscanada.org/bird-science/nabci-bird-conservation-regions (Accessed February 10, 2021)
BirdLife International (2021). IUCN Red List for Birds. BirdLife International, Available online at: http://www.birdlife.org (Accessed December 15, 2020)
Bodner, K., Fortin, M., and Molnár, P. K. (2020). Making predictive modelling ART: accurate, reliable, and transparent. Ecosphere 11:e03160. doi: 10.1002/ecs2.3160
Boulanger, Y., Gauthier, S., and Burton, P. J. (2014). A refinement of models projecting future Canadian fire regimes using homogeneous fire regime zones. Can. J. For. Res. 44, 365–376. doi: 10.1139/cjfr-2013-0372
Boulanger, Y., Taylor, A. R., Price, D. T., Cyr, D., McGarrigle, E., Rammer, W., et al. (2017). Climate change impacts on forest landscapes along the Canadian southern boreal forest transition zone. Landsc. Ecol. 32, 1415–1431. doi: 10.1007/s10980-016-0421-7
Bush, E., and Lemmen, D. S. (2019). Canada’s Changing Climate Report. Ottawa, ONT: Government of Canada, Available online at: https://changingclimate.ca/CCCR2019/ (Accessed April 13, 2021).
Cadieux, P., Boulanger, Y., Cyr, D., Taylor, A. R., Price, D. T., Sólymos, P., et al. (2020). Projected effects of climate change on boreal bird community accentuated by anthropogenic disturbances in western boreal forest, Canada. Divers. Distrib. 26, 668–682. doi: 10.1111/ddi.13057
Cadieux, P., Boulanger, Y., Cyr, D., Taylor, A. R., Price, D. T., and Tremblay, J. A. (2019). Spatially explicit climate change projections for the recovery planning of threatened species: the Bicknell’s Thrush (Catharus Bicknelli) as a case study. Glob. Ecol. Conserv. 17:e00530. doi: 10.1016/j.gecco.2019.e00530
Canadian Forest Service (2019). Canadian National Fire Database – Agency Fire Data. Edmonton: Canadian Forest Service,
Chen, H. Y. H., Luo, Y., Reich, P. B., Searle, E. B., and Biswas, S. R. (2016). Climate change-associated trends in net biomass change are age dependent in western boreal forests of Canada. Ecol. Lett. 19, 1150–1158. doi: 10.1111/ele.12653
Chubaty, A. M., and McIntire, E. J. B. (2021). SpaDES: Develop and Run Spatially Explicit Discrete Event Simulation Models. R Package Version 2.0.4. Available online at: http://cran.r-project.org/package=SpaDES (accessed January 26, 2021).
Clark, J. S. (2001). Ecological forecasts: an emerging imperative. Science 293, 657–660. doi: 10.1126/science.293.5530.657
Clason, A. J., McIntire, E. J. B., and Burton, P. J. (2020). Latitudinal limit not a cold limit: cold temperatures do not constrain an endangered tree species at its northern edge. J. Biogeogr 47, 1398–1412. doi: 10.1111/jbi.13822
Cumming, S. G., Demarchi, D., and Walters, C. (1998). A grid-based spatial model of forest dynamics applied to the boreal mixedwood region. Sustain. For. Manage. Netw. Available online at: https://doi.org/10.7939/R35N33 (Accessed April 13, 2021).
Cumming, S. G., Lefevre, K. L., Bayne, E., Fontaine, T., Schmiegelow, F. K. A., and Song, S. J. (2010). Toward conservation of Canada’s Boreal Forest Avifauna: design and application of ecological models at continental extents. Avian Conserv. Ecol. 5:art8. doi: 10.5751/ACE-00406-050208
Dawson, T. P., Jackson, S. T., House, J. I., Prentice, I. C., and Mace, G. M. (2011). Beyond predictions: biodiversity conservation in a changing climate. Science 332, 53–58. doi: 10.1126/science.1200303
De Cáceres, M., Brotons, L., Aquilué, N., and Fortin, M.-J. (2013). The combined effects of land-use legacies and novel fire regimes on bird distributions in the Mediterranean. J. Biogeogr. 40, 1535–1547. doi: 10.1111/jbi.12111
Dietze, M., and Lynch, H. (2019). Forecasting a bright future for ecology. Front. Ecol. Environ. 17:3. doi: 10.1002/fee.1994
Dietze, M. C., Fox, A., Beck-Johnson, L. M., Betancourt, J. L., Hooten, M. B., Jarnevich, C. S., et al. (2018). Iterative near-term ecological forecasting: needs, opportunities, and challenges. Proc. Natl. Acad. Sci. U.S.A. 115, 1424–1432. doi: 10.1073/pnas.1710231115
Ecosystem Classification Group (2007). Ecological Regions of the Northwest Territories: Taiga Plains. Yellowknife, NT: Government of Northwest Territories.
Fajardo, J., Corcoran, D., Roehrdanz, P. R., Hannah, L., and Marquet, P. A. (2020). GCM compareR: a web application to assess differences and assist in the selection of general circulation models for climate change research. Methods Ecol. Evol. 11, 656–663. doi: 10.1111/2041-210X.13360
Gauthier, S., Bernier, P., Kuuluvainen, T., Shvidenko, A. Z., and Schepaschenko, D. G. (2015). Boreal forest health and global change. Science 349, 819–822. doi: 10.1126/science.aaa9092
Hargrove, W. W., Gardner, R. H., Turner, M. G., Romme, W. H., and Despain, D. G. (2000). Simulating fire patterns in heterogeneous landscapes. Ecol. Modell. 135, 243–263. doi: 10.1016/S0304-3800(00)00368-9
Hausfather, Z., and Peters, G. P. (2020). Emissions – the ‘business as usual’ story is misleading. Nature 577, 618–620. doi: 10.1038/d41586-020-00177-3
Hebblewhite, M. (2017). Billion dollar boreal woodland caribou and the biodiversity impacts of the global oil and gas industry. Biol. Conserv. 206, 102–111. doi: 10.1016/j.biocon.2016.12.014
Helbig, M., Pappas, C., and Sonnentag, O. (2016). Permafrost thaw and wildfire: equally important drivers of boreal tree cover changes in the Taiga Plains, Canada. Geophys. Res. Lett. 43, 1598–1606. doi: 10.1002/2015GL067193
Hijmans, R., Philips, S., Leathwich, J., and Elith, J. (2020). dismo: Species Distribution Modeling. R Package Version 1.4-4. Available online at: https://CRAN.R-project.org/package=dismo (accessed January 26, 2021).
Hitch, A. T., and Leberg, P. L. (2007). Breeding distributions of North American bird species moving north as a result of climate change. Conserv. Biol. 21, 534–539. doi: 10.1111/j.1523-1739.2006.00609.x
Hogg, E. H. (Ted) (1994). Climate and the southern limit of the western Canadian boreal forest. Can. J. For. Res. 24, 1835–1845. doi: 10.1139/x94-237
Langham, G. M., Schuetz, J. G., Distler, T., Soykan, C. U., and Wilsey, C. (2015). Conservation status of North American birds in the face of future climate change. PLoS One 10:e0135350. doi: 10.1371/journal.pone.0135350
Latifovic, R., and Pouliot, D. (2005). Multitemporal land cover mapping for Canada: methodology and products. Can. J. Rem. Sens. 31, 347–363. doi: 10.5589/m05-019
Lepage, D. (2019). Checklist of Birds of Northwest Territories. Available online at: https://avibase.bsc-eoc.org/checklist.jsp?lang=EN&p2=1&list=aou&synlang=®ion=CAnt&version=text&lifelist=&highlight=0 (Accessed August 2, 2019).
Luo, Y., McIntire, E. J. B., Boisvenue, C., Nikiema, P., and Chen, H. Y. H. (2019). Climatic change only stimulated growth for trees under weak competition in central boreal forests. J. Ecol. 108, 36–46. doi: 10.1111/1365-2745.13228
Mahon, C. L., Holloway, G., Sólymos, P., Cumming, S. G., Bayne, E. M., Schmiegelow, F. K. A., et al. (2016). Community structure and niche characteristics of upland and lowland western boreal birds at multiple spatial scales. For. Ecol. Manage. 361, 99–116. doi: 10.1016/j.foreco.2015.11.007
Mahon, C. L., Holloway, G. L., Bayne, E. M., and Toms, J. D. (2019). Additive and interactive cumulative effects on boreal landbirds: winners and losers in a multi-stressor landscape. Ecol. Appl. 29:e01895. doi: 10.1002/eap.1895
Marchal, J., Cumming, S. G., and McIntire, E. J. B. (2017a). Exploiting Poisson additivity to predict fire frequency from maps of fire weather and land cover in boreal forests of Québec, Canada. Ecography 40, 200–209. doi: 10.1111/ecog.01849
Marchal, J., Cumming, S. G., and McIntire, E. J. B. (2017b). Land cover, more than monthly fire weather, drives fire-size distribution in Southern Québec forests: implications for fire risk management. PLoS One 12:e0179294. doi: 10.1371/journal.pone.0179294
Marchal, J., Cumming, S. G., and McIntire, E. J. B. (2019). Turning down the heat: vegetation feedbacks limit fire regime responses to global warming. Ecosystems 23, 204–216. doi: 10.1007/s10021-019-00398-2
Masson-Delmotte, V., Zhai, P., Pörtner, H.-O., Roberts, D., Skea, J., Shukla, P. R., et al. (2019). Global Warming of 1.5°C. An IPCC Special Report on the Impacts of Global Warming of 1.5°C Above Pre-Industrial Levels and Related Global Greenhouse Gas Emission Pathways, in the Context of Strengthening the Global Response to the Threat of Climate Change, Sustainable Development, and Efforts to Eradicate Poverty. Geneva: IPCC.
McCaslin, H. M., and Heath, J. A. (2020). Patterns and mechanisms of heterogeneous breeding distribution shifts of North American migratory birds. J. Avian Biol. 51:jav.02237. doi: 10.1111/jav.02237
McIntire, E. J. B., and Chubaty, A. M. (2021). Reproducible: A Set of Tools that Enhance Reproducibility Beyond Package Management. R Package Version 1.2.1.9000. Available online at: https://CRAN.R-project.org/package=reproducible (accessed January 26, 2021).
McIntire, E. J. B., Chubaty, A. M., Cumming, S. G., Barros, C., Micheletti, T., Eddy, I., et al. (2021). Predictive ecology: a re-imagined foundation and the tools for ecological models. Authorea [Preprint]. doi: 10.22541/au.161709444.40008325/v1
Peters, R. H. (1982). “Useful concepts for predictive ecology,” in Conceptual Issues in Ecology, ed. E. Saarinen (Dordrecht: Springer Netherlands), 215–227. doi: 10.1007/978-94-009-7796-9_8
Price, D. T., Alfaro, R. I., Brown, K. J., Flannigan, M. D., Fleming, R. A., Hogg, E. H., et al. (2013). Anticipating the consequences of climate change for Canada’s boreal forest ecosystems. Environ. Rev. 21, 322–365. doi: 10.1139/er-2013-0042
Pya, N., and Wood, S. N. (2015). Shape constrained additive models. Stat. Comput. 25, 543–559. doi: 10.1007/s11222-013-9448-7
R Core Team (2019). R: a Language and Environment for Statistical Computing. Vienna: R Foundation for Statistical Computing. Available online at: https://www.R-project.org/ (accessed September 16, 2021)
Regos, A., Clavero, M., D’Amen, M., Guisan, A., and Brotons, L. (2018). Wildfire–vegetation dynamics affect predictions of climate change impact on bird communities. Ecography 41, 982–995. doi: 10.1111/ecog.02990
Rehfeldt, G. E., Crookston, N. L., Sáenz-Romero, C., and Campbell, E. M. (2012). North American vegetation model for land-use planning in a changing climate: a solution to large classification problems. Ecol. Appl. 22, 119–141. doi: 10.1890/11-0495.1
Riddell, E. A., Iknayan, K. J., Hargrove, L., Tremor, S., Patton, J. L., Ramirez, R., et al. (2021). Exposure to climate change drives stability or collapse of desert mammal and bird communities. Science 371, 633–636. doi: 10.1126/science.abd4605
Rigby, R. A., and Stasinopoulos, D. M. (2005). Generalized additive models for location, scale and shape (with discussion). J. R. Stat. Soc. C 54, 507–554. doi: 10.1111/j.1467-9876.2005.00510.x
Rosenberg, K. V., Dokter, A. M., Blancher, P. J., Sauer, J. R., Smith, A. C., Smith, P. A., et al. (2019). Decline of the North American avifauna. Science 366, 120–124. doi: 10.1126/science.aaw1313
Sappington, J. M., Longshore, K. M., and Thompson, D. B. (2007). Quantifying landscape ruggedness for animal habitat analysis: a case study using bighorn sheep in the Mojave desert. J. Wildlife Manage. 71, 1419–1426. doi: 10.2193/2005-723
Scheller, R. M., and Mladenoff, D. J. (2004). A forest growth and biomass module for a landscape simulation model, LANDIS: design, validation, and application. Ecol. Modell. 180, 211–229. doi: 10.1016/j.ecolmodel.2004.01.022
Schwalm, C. R., Glendon, S., and Duffy, P. B. (2020). Reply to Hausfather and Peters: RCP8.5 is neither problematic nor misleading. Proc. Natl. Acad. Sci. U.S.A. 117, 27793–27794. doi: 10.1073/pnas.2018008117
Sherwood, S. C., Webb, M. J., Annan, J. D., Armour, K. C., Forster, P. M., Hargreaves, J. C., et al. (2020). An assessment of Earth’s climate sensitivity using multiple lines of evidence. Rev. Geophys. 58, 73–77. doi: 10.1029/2019RG000678
Smith, W. B., Cuenca Lara, R. A., Delgado Caballero, C. E., Godínez Valdivia, C. I., Kapron, J. S., Leyva Reyes, J. C., et al. (2018). The North American forest database: going beyond national-level forest resource assessment statistics. Environ. Monit. Assess. 190:350. doi: 10.1007/s10661-018-6649-8
Sólymos, P., Matsuoka, S. M., Bayne, E. M., Lele, S. R., Fontaine, P., Cumming, S. G., et al. (2013). Calibrating indices of avian density from non-standardized survey data: making the most of a messy situation. Methods Ecol. Evol. 4, 1047–1058. doi: 10.1111/2041-210X.12106
Sólymos, P., Matsuoka, S. M., Cumming, S. G., Stralberg, D., Fontaine, P., Schmiegelow, F. K. A., et al. (2018). Evaluating time-removal models for estimating availability of boreal birds during point count surveys: sample size requirements and model complexity. Condor 120, 765–786. doi: 10.1650/CONDOR-18-32.1
Stall, S., Yarmey, L., Cutcher-Gershenfeld, J., Hanson, B., Lehnert, K., Nosek, B., et al. (2019). Make scientific data FAIR. Nature 570, 27–29. doi: 10.1038/d41586-019-01720-7
Stewart, F. E. C., Nowak, J. J., Micheletti, T., McIntire, E. J. B., Schmiegelow, F. K. A., and Cumming, S. G. (2020). Boreal Caribou can coexist with natural but not industrial disturbances. J. Wild. Management 84, 1435–1444. doi: 10.1002/jwmg.21937
Stralberg, D., Bayne, E. M., Cumming, S. G., Sólymos, P., Song, S. J., and Schmiegelow, F. K. A. (2015a). Conservation of future boreal forest bird communities considering lags in vegetation response to climate change: a modified Refugia approach. Divers. Distrib. 21, 1112–1128. doi: 10.1111/ddi.12356
Stralberg, D., Matsuoka, S. M., Hamann, A., Bayne, E. M., Sólymos, P., Schmiegelow, F. K. A., et al. (2015b). Projecting boreal bird responses to climate change: the signal exceeds the noise. Ecol. Appl. 25, 52–69. doi: 10.1890/13-2289.1
Stralberg, D., Berteaux, D., Drever, C. R., Drever, M., Naujokaitis-Lewis, I., Schmiegelow, F. K. A., et al. (2019). Conservation planning for boreal birds in a changing climate: a framework for action. Avian Conserv. Ecol. 14:13. doi: 10.5751/ACE-01363-140113
Tanner, E. P., Elmore, R. D., Fuhlendorf, S. D., Davis, C. A., Dahlgren, D. K., and Orange, J. P. (2017). Extreme climatic events constrain space use and survival of a ground-nesting bird. Glob. Change Biol. 23, 1832–1846. doi: 10.1111/gcb.13505
Travers, H., Selinske, M., Nuno, A., Serban, A., Mancini, F., Barychka, T., et al. (2019). A manifesto for predictive conservation. Biol. Conserv. 237, 12–18. doi: 10.1016/j.biocon.2019.05.059
Tremblay, J. A., Boulanger, Y., Cyr, D., Taylor, A. R., Price, D. T., and St-Laurent, M.-H. (2018). Harvesting interacts with climate change to affect future habitat quality of a focal species in eastern Canada’s boreal forest. PLoS One 13:e0191645. doi: 10.1371/journal.pone.0191645
Turetsky, M. R., Abbott, B. W., Jones, M. C., Anthony, K. W., Olefeldt, D., Schuur, E. A. G., et al. (2020). Carbon release through abrupt permafrost thaw. Nat. Geosci. 13, 138–143. doi: 10.1038/s41561-019-0526-0
Van Wilgenburg, S. L., Sólymos, P., Kardynal, K. J., and Frey, M. D. (2017). Paired sampling standardizes point count data from humans and acoustic recorders. ACE 12:art13. doi: 10.5751/ACE-00975-120113
Wang, J. A., Sulla-Menashe, D., Woodcock, C. E., Sonnentag, O., Keeling, R. F., and Friedl, M. A. (2020). Extensive land cover change across Arctic–Boreal Northwestern North America from disturbance and climate forcing. Glob. Change Biol. 26, 807–822. doi: 10.1111/gcb.14804
Wang, T., Hamann, A., Spittlehouse, D., and Carroll, C. (2016). Locally downscaled and spatially customizable climate data for historical and future periods for North America. PLoS One 11:e0156720. doi: 10.1371/journal.pone.0156720
Wells, J. V., and Blancher, P. J. (2011). “Global role for sustaining bird populations,” in Boreal birds of North America: a Hemispheric View of Their Conservation Links and Significance. Studies in Avian Biology, ed. J. V. Wells (Berkeley, CA: University of California Press), 7–22.
White, E. P., Yenni, G. M., Taylor, S. D., Christensen, E. M., Bledsoe, E. K., Simonis, J. L., et al. (2019). Developing an automated iterative near-term forecasting system for an ecological study. Methods Ecol. Evol. 10, 332–344. doi: 10.1111/2041-210X.13104
Whitman, E., Parisien, M.-A., Thompson, D. K., and Flannigan, M. D. (2019). Short-interval wildfire and drought overwhelm boreal forest resilience. Sci. Rep. 9:18796. doi: 10.1038/s41598-019-55036-7
Wilcox, E. J., Keim, D., de Jong, T., Walker, B., Sonnentag, O., Sniderhan, A. E., et al. (2019). Tundra shrub expansion may amplify permafrost thaw by advancing snowmelt timing. Arctic Sci. 5, 202–217. doi: 10.1139/as-2018-0028
WildTrax (2019). User Guide: Autonomous Recording Units. Version 2019-10-4. Alberta: Alberta Biodiversity Monitoring Institute.
Wisz, M. S., Pottier, J., Kissling, W. D., Pellissier, L., Lenoir, J., Damgaard, C. F., et al. (2013). The role of biotic interactions in shaping distributions and realised assemblages of species: implications for species distribution modelling. Biol. Rev. 88, 15–30. doi: 10.1111/j.1469-185X.2012.00235.x
Wu, D., Zhao, X., Liang, S., Zhou, T., Huang, K., Tang, B., et al. (2015). Time-lag effects of global vegetation responses to climate change. Glob. Change Biol. 21, 3520–3531. doi: 10.1111/gcb.12945
Yates, K. L., Bouchet, P. J., Caley, M. J., Mengersen, K., Randin, C. F., Parnell, S., et al. (2018). Outstanding challenges in the transferability of ecological models. Trends Ecol. Evol. 33, 790–802. doi: 10.1016/j.tree.2018.08.001
Keywords: landscape simulation, ecological forecasting, vegetation, fire, decision support, forest management, reproducibility, reusability
Citation: Micheletti T, Stewart FEC, Cumming SG, Haché S, Stralberg D, Tremblay JA, Barros C, Eddy IMS, Chubaty AM, Leblond M, Pankratz RF, Mahon CL, Van Wilgenburg SL, Bayne EM, Schmiegelow F and McIntire EJB (2021) Assessing Pathways of Climate Change Effects in SpaDES: An Application to Boreal Landbirds of Northwest Territories Canada. Front. Ecol. Evol. 9:679673. doi: 10.3389/fevo.2021.679673
Received: 12 March 2021; Accepted: 08 September 2021;
Published: 04 October 2021.
Edited by:
Daniel de Paiva Silva, Goiano Federal Institute (IFGOIANO), BrazilReviewed by:
Miguel Ângelo Marini, University of Brasilia, BrazilAlejandra Morán-Ordóñez, Ecological and Forestry Applications Research Center (CREAF), Spain
Copyright © 2021 Micheletti, Stewart, Cumming, Haché, Stralberg, Tremblay, Barros, Eddy, Chubaty, Leblond, Pankratz, Mahon, Van Wilgenburg, Bayne, Schmiegelow and McIntire. This is an open-access article distributed under the terms of the Creative Commons Attribution License (CC BY). The use, distribution or reproduction in other forums is permitted, provided the original author(s) and the copyright owner(s) are credited and that the original publication in this journal is cited, in accordance with accepted academic practice. No use, distribution or reproduction is permitted which does not comply with these terms.
*Correspondence: Tatiane Micheletti, tati.micheletti@gmail.com
†ORCID: Tatiane Micheletti, orcid.org/0000-0003-4838-8342; Frances E. C. Stewart, orcid.org/0000-0001-9344-8346; Steven G. Cumming, orcid.org/0000-0002-7862-2913; Samuel Haché, orcid.org/0000-0003-3952-009X; Diana Stralberg, orcid.org/0000-0003-4900-024X; Junior A. Tremblay, orcid.org/0000-0003-4930-0939; Ceres Barros, orcid.org/0000-0003-4036-977X; Ian M. S. Eddy, orcid.org/0000-0001-7397-2116; Alex M. Chubaty, orcid.org/0000-0001-7146-8135; Mathieu Leblond, orcid.org/0000-0002-2833-6265; Rhiannon F. Pankratz, orcid.org/0000-0003-1345-6624; C. L. Mahon, orcid.org/0000-0003-0283-057; Steven L. Van Wilgenburg, orcid.org/0000-0002-6742-4567; Erin M. Bayne, orcid.org/0000-0002-0679-4521; Fiona Schmiegelow, orcid.org/0000-0002-8219-8684; Eliot J. B. McIntire, orcid.org/0000-0002-6914-8316