- 1Durrell Institute of Conservation and Ecology (DICE), School of Anthropology and Conservation, University of Kent, Canterbury, United Kingdom
- 2Institute for Tropical Biology and Conservation, Universiti Malaysia Sabah, Kota Kinabalu, Malaysia
The expansion of oil palm agriculture has contributed to biodiversity loss in Southeast Asia and elsewhere in the tropics. Riparian reserves (areas of native forest along waterways) have the potential to maintain forest biodiversity and associated ecological processes within these agricultural landscapes. Using acoustic sampling, we investigated the value of riparian reserves for insectivorous bats in oil palm plantations in Sabah, Malaysian Borneo. We compared general bat activity, foraging activity, and species occupancy between riparian areas in forest and riparian reserves in oil palm plantations. Overall bat activity varied little between riparian reserves in oil palm and riparian forest. Rather, activity was greatest in areas with a high forest canopy, irrespective of how much forest was available within or outside the riparian reserve. Bat foraging activity, as well as the occupancy of two species, was greatest in the forest sites, and while bats were detected in the oil palm riparian reserves, both foraging and occupancy were more associated with topographic ruggedness than forest amount or height. Our results indicate that habitat structure within riparian reserves may be more important than reserve size for supporting insectivorous bat diversity within oil palm landscapes. These findings provide important insights into the extent of the ecological benefits provided by conservation set-asides in forest-agricultural landscapes in the tropics.
Introduction
The intensification and expansion of agriculture are major causes of tropical biodiversity declines (Phalan et al., 2013). Since the tropics support large numbers of species and some of the world's last pristine habitats, the ongoing biodiversity crisis occurs disproportionately in these regions (Sodhi et al., 2013; Rosa et al., 2016). Here industrial commodity crops, including but not limited to oil palm (Elaeis guineensis), are becoming widespread. In the last decade palm oil production has more than doubled and demand is likely to rise, due to both its status as the world's primary and cheapest vegetable oil and its use as a biofuel feedstock (Meijaard et al., 2018). Southeast Asia dominates as the epicenter of oil palm production, with Indonesia and Malaysia being the leading producers (Meijaard et al., 2018). Seventeen percent of Malaysian plantations are thought to have needed forest clearing (Pirker et al., 2016). With oil palm plantations being a poor biodiversity substitute for native forest habitat (Fitzherbert et al., 2008), this raises concerns about the environmental sustainability of the crop, and the associated negative impacts agricultural expansion is having on tropical biodiversity.
One way to mitigate biodiversity losses in oil palm is to maximize natural habitat within estates by retaining habitat remnants as conservation set-asides (Meijaard et al., 2018). Protected forest patches are promoted within the Roundtable on Sustainable Palm Oil certification standard (RSPO, 2018), and while these improve prospects for terrestrial mammals, patches are rarely large enough to sustain viable populations individually (Deere et al., 2020). Therefore, improving habitat area and connectivity between these patches is paramount, and will be particularly important for species with limited dispersal capabilities that are unable to traverse large expanses of open, agricultural land (Carroll et al., 2004).
Protecting riparian reserves—areas of native vegetation alongside rivers—is one potential way to help join up forest patches in tropical agriculture, but to date has been poorly studied in the context of oil palm (Luke et al., 2019). Riparian reserves (also known as buffers), are typically protected to maintain hydrology (Tabacchi et al., 2000) and nutrient regulation (Naiman et al., 2010), which can lead to potential co-benefits for biodiversity (Mitchell et al., 2018). Many tropical countries therefore protect riparian areas in some form, but there is a weak evidence-base supporting the size, or width, of the protection zone (Luke et al., 2019). Further evidence is considered a high priority within the oil palm research community (Padfield et al., 2019).
To date, bats have been poorly studied in the context of oil palm in part because they are difficult to capture and study in open habitats. However, bats make ideal indicator taxa since they are globally distributed, taxonomically stable, and can be monitored regularly to determine population trends if acoustic data are used (Jones et al., 2009). In addition, as primary invertebrate predators, bats are potentially important indicators of invertebrate abundance and their responses to environmental change. Bats comprise around a third of Southeast Asia's mammal diversity (Kingston, 2010), and the conservation status of 17% of these species is currently unknown (IUCN, 2019). The main threats to Asia's bats mirror those listed for other taxa; namely, deforestation, habitat fragmentation, and disturbance, and 22% are assessed as threatened (IUCN, 2019). If these threats continue, Southeast Asia is projected to lose 40% of its bat species by the end of the century (Lane et al., 2006).
In paleotropical forests high bat diversity is associated with structurally complex, highly heterogeneous and lesser disturbed forest habitats (Struebig et al., 2013). Diversity and population viability are also greatest in larger forest patches (Struebig et al., 2008, 2011). However, habitat disturbance and fragmentation affect bat species in different ways, with those in the families Hipposideridae and Rhinolophidae, and vespertilionid subfamilies Kerivoulinae and Murininae, being most affected (Struebig et al., 2008). The high sensitivity of these taxa is thought to be due to adaptations in wing morphology and echolocation call design that restrict their movements and foraging capabilities in more open habitats (Kingston et al., 2003b). Nevertheless, recapture distances and radiotracking studies confirm that some hipposiderid and rhinolophid species can commute several kilometers in a single night (Struebig et al., 2008), demonstrating that there is also substantial variation in species responses within these groups. In contrast, recapture, and radiotracking studies of Kerivoula species rarely exceed 1 km, and declines in abundance tends to be associated with the loss of tree cavities in disturbed forests since many of these species use tree features for roosting (Struebig et al., 2013). Other sub-components of insectivorous bat assemblages, such as those that forage in forest edges or open spaces outside or above the forest, may be able to adapt to disturbed habitats, and are frequently detected in farmland (Kingston et al., 2003a).
The importance of riparian reserves for bats has not been explicitly studied in the Southeast Asian tropics. However, in other parts of the world bats are known to benefit from protected waterways in agricultural areas. In the neotropics, for example, bats use riparian reserves as stepping stones between larger patches of vegetation (de Peña-Cuéllar et al., 2015), and in Britain reserves are known to be used for foraging due to the large number of insects supported by rivers (Vaughan et al., 1996). In Mexico, riparian reserves were found to host a higher abundance and diversity of bats compared to other landscape features (Galindo-González and Sosa, 2003). Additionally, a study in Swaziland found that as well as high abundance and diversity there was also higher activity and species richness in riparian reserves compared to the surrounding savannah landscape (Monadjem and Reside, 2008).
Here we investigate the use of riparian areas by palaeotropical bats in order to determine the optimum habitat features to support their conservation in Southeast Asian agricultural landscapes. We base our study in a region with widespread oil palm agriculture (Sabah, Malaysian Borneo), and use acoustic survey techniques as an alternative to traditional capture methods, which are known to poorly sample many insectivorous species, particularly in more open habitats. Specifically, we investigate how (i) total bat activity, and (ii) foraging activity varies between the riparian areas in forest and riparian reserves in oil palm, for all species and between call-types. We then identify the structural characteristics important for (iii) predicting bat activity, and (iv) species-specific occurrence for a subset of taxa.
Materials and Methods
Study Area
Research was conducted in and around the Stability of Altered Forest Ecosystems Project1 within the Kalabakan Forest Reserve of Sabah, Borneo. The SAFE Project is a landscape experiment tracking changes to biodiversity and ecosystem functioning as logged mixed-dipterocarp forest is converted to plantation. Forest across the landscape has been logged multiple times since the 1970s, and during the fieldwork the remaining core areas of forest were being salvage-logged in preparation for additional plantation.
We sampled 15 riparian areas, comprising eight forested rivers in the SAFE project area, and seven riparian reserves in the surrounding oil palm estates, which were planted between 2000 and 2015 (Figure 1, Table S1). Forest sites were protected from further logging, but many remained highly disturbed from previous logging events. In Malaysia, riparian protection policies vary by state, with the prescribed reserve width in farmland depending on the size of the river and landscape context. In Sabah, companies are required to protect between 5 and 100 m, with many reserves in oil palm being at least 20 m wide from each riverbank (Luke et al., 2019). Therefore, the width of the riparian reserves in the oil palm varied substantially between and also within rivers, ranging from 0 m (i.e., completely devoid of forest vegetation) in river ROP2, to >200 m in parts of R14 and R16 - considering both riverbanks. All rivers were sampled at least twice (Table 1).
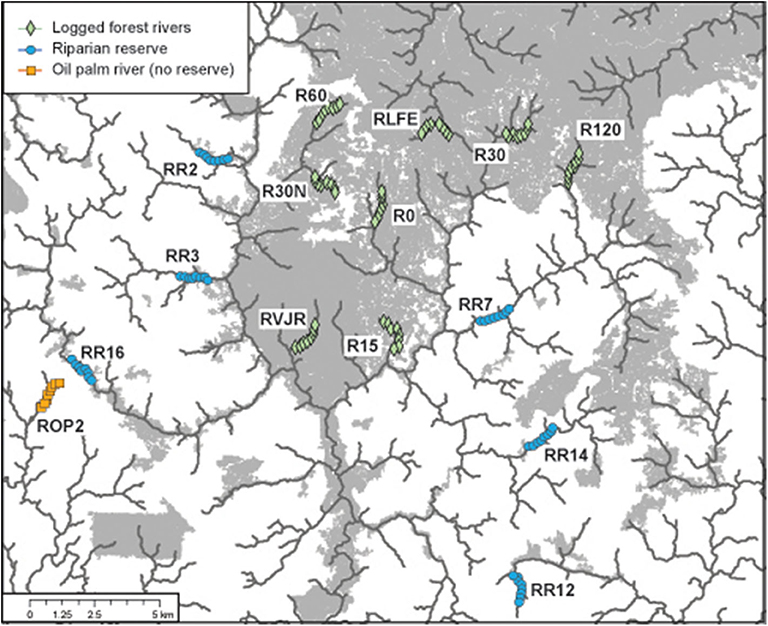
Figure 1. Location of the 15 rivers sampled for bats via acoustic monitoring in the Stability of Altered Forest Ecosystems (SAFE) landscape of Sabah, Malaysian Borneo. Each site comprised eight recording points, which were sampled multiple times. Forested area in gray (derived from Hansen et al., 2013 data for 2014); oil palm estates in white. Lines denote the river courses.
Acoustic Sampling
We implemented acoustic surveys along each river and the associated riparian forest/reserve using a walking-point-transect design. At each site we recorded bats at eight sampling points positioned ~200 m apart along a 2 km stretch of river. Surveys were undertaken in the early evening during peak bat activity, commencing just before sunset (18:01–18:23, according to standardized times in Kota Kinabalu2). As heavy rainfall has implications for bat activity as well as the viability of recording equipment, we restricted surveys to rain-free nights—the number of nights abandoned due to rain was more than double that of the sample size achieved. At each point, we recorded bat activity for 10 min (80 min of recording per night), using a full-spectrum EM3+ ultrasonic recorder (Wildlife Acoustics Inc.). The detector was set to record intermittently, with sounds >18 db and >18 kHz triggering a recording. The sampling rate was set at 384 kHz, thus allowing frequencies up to 192 kHz to be recorded. The maximum duration of recordings was set at 10 s. We considered one trigger to be a bat pass of the microphone and used this as an index of bat activity. Triggers and associated data were stored on SDHC memory cards as raw WAV files.
The EM3+ microphone is omnidirectional, with optimal recordings ±30° vertically and 60° horizontally to the unit. As with all acoustic devices, the detection zone of the microphone varied for different species, depending on sound attenuation and vegetation clutter around the river. For this reason, we broke up the 10 min of recording into three components at each point: 4 min in the center of the river, and 3 on each riverbank ~5 m from the river edge. This minimized bias to species utilizing merely the river, whilst also maximizing detection of those bats in vegetation nearby. All rivers were sampled on at least two occasions, visiting sites sequentially from one end of the river to the other. Five rivers had four visits, five had three visits and five had two visits, with surveys spanning across 2014 and 2015. All visits used the same sampling points, and all recordings were processed following the same method. When logistically possible we sampled the rivers starting at the opposite end of the transect on the second sample in order to control for differing levels of bat activity over the period of the evening. For example, we expected higher bat activity earlier in the evening, around and just after dusk, with declining activity as the evening progressed.
Processing Acoustic Data
Data from each night were processed using the SonoBat SM2 Batch Attributer (Wildlife Acoustics PLC.), and then scrubbed using the SonoBat Batch Scrubber V5.2 to remove noise files. The remaining 16,530 files were processed using SonoBat 3.2p Batch Processor, and each sound file manually inspected, then accepted or rejected as a genuine bat trigger. An independent trigger file was accepted as a genuine bat call if there was a minimum of three pulses. To quantify bat activity at each river we used the number of genuine bat microphone triggers, noting that this could represent a single bat responsible for multiple triggers. During processing, we identified feeding buzzes through visual interpretation of the call and from playback.
We used SonoBat call analysis software to automatically extract the following call parameters: pulse duration, and highest frequency and lowest frequency of the dominant harmonic. All calls were manually inspected alongside the call parameters to classify them into four call-types: constant frequency (CF; e.g., Rhinolophidae and Hipposideridae), frequency modulating quasi-constant frequency (FMqCF; e.g., Molossidae and several Vespertilioninae genre such as the bamboo bats, Tylonycteris), broad-band frequency modulating (FMb; e.g., Kerivoulinae and Murininae), and multi-harmonic frequency modulating (MHFM; e.g., Megaderma spasma and Nycteris tragata). Species utilizing CF, FMb, or MHFM calls are known to forage in cluttered narrow-spaces; CF species use flutter detection to find prey, whereas species using FMb and MHFM are active and passive gleaners, respectively (Denzinger and Schnitzler, 2013; Table S2). These three call types are therefore characteristic of species widely regarded as forest interior insectivores. On the other hand, species using FMqCF calls are characterized by aerial hawking to capture prey and are more commonly associated with forest edge and open spaces (Denzinger and Schnitzler, 2013).
It was not possible to identify most calls to the species-level due to insufficient local knowledge of the echolocation calls for many species. However, horseshoe and leaf-nosed bats (Rhinolophidae and Hipposideridae respectively, and all CF species) could be confidently identified using the species-specific peak parameters of their constant frequency tone (Table 2). These call parameters were taken from a library of recordings from bats recorded in the landscape or elsewhere in northern Borneo. Analysis was therefore restricted to the level of call-type for most bats, with the addition of species-based analysis for CF bats.
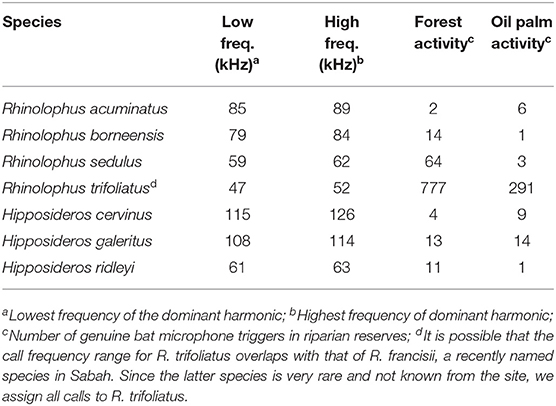
Table 2. Call parameters of constant frequency (CF) bats identified in this study along with their activity in the riparian reserves.
Environmental Characteristics of Riparian Sites
Potential covariates were extracted at each point on every river, providing eight data points per river—i.e., 128 over the landscape. Riparian reserve width, applicable to only sites in the oil palm, was estimated at each point from LiDAR imagery at 5 m resolution (described in Swinfield et al., 2020). The width of the river channel, measured in the field using a laser rangefinder (Leica Rangemaster CRF 1000), was subtracted from this estimate to determine the actual land surface within the reserve. The covariate used, RiparianWidth, is the mean width on a single side of the river, since this is typically referenced in environmental policy documents.
Previous research in the project area highlighted forest structural and landscape characteristics as important predictors of bat community composition (Struebig et al., 2013), and so two additional covariates were also extracted from each sample point. Average topographic ruggedness (Topography) was measured within 50 m of each point using Shuttle Radar Topography Mission data, according to Wilson et al. (2007). Mean canopy height (CanopyHeight), also sourced from the LiDAR data, was used as a proxy for forest quality. Taller forests in the landscape tend to have more trees, higher biomass, and greater vertical and horizontal complexity, all measures of forest structure and quality that are highly correlated (Deere et al., in review). Both variables were extracted to points at a spatial scale of 50 m as this matched those used for a study on birds at the site (Mitchell et al., 2018) as we assumed bats and birds would be using the landscape in similar ways.
Statistical Analysis
For each point, we summed total activity or foraging activity for all call data combined, as well as grouping by call-type. Due to the unequal sample effort between rivers (Table 1) and high numbers of zeros in the dataset (which could not be remedied via zero-inflation models), this was calculated as a mean value across visits for each sampling point, e.g., sum of total bat passes recorded at the point per night divided by the number of nights recorded. We then applied generalized linear mixed effect models (GLMMs) with poisson error distributions to explore the potential determinants of bat activity across sites. In addition, for a subset of CF bats that could be identified to species level, we applied hierarchical Bayesian multi-species occupancy modeling to determine whether there were patterns in species occurrence between rivers. Occupancy modeling was better suited to species-level analyses given the propensity for zero counts.
Activity
The GLMMs were first applied to determine whether bat activity differed between forest and oil palm sites, and whether this was associated with the covariates. A second analysis was then undertaken using the subset of the data from oil palm riparian reserves to determine whether the width of the reserve influenced bat activity relative to the other covariates. These analyses were conducted for all bat activity, CF calls, and FMqCF calls—thus six GLMMs in total. We did not work with data for the other call types due to insufficient numbers of bat passes.
In order to avoid collinearity in the GLMMs, only non-correlated variables were included (rs <0.5). The final fixed covariates were HabitatType (“forest” vs. “oil palm,” categorical with two levels), TimeafterSunset (continuous), Topography (continuous), CanopyHeight (continuous) and RiparianWidth (continuous). To incorporate the dependency among observations of the same river, we used River-ID as a random intercept. HabitatType was used only in the first GLMM to assess covariates across sites collectively [Equation (1:3)]. As RiparianWidth is only applicable to oil palm reserves, we only included this covariate in the models focusing on those reserves [Equation (4:7)] (Table S3).
The package “lme4” (Bates et al., 2015) in the software R (R Core Team, 2014) was used to fit the models in Equation (1:7) (Table S3) using a Nelder-Mead optimizer from the package “optimx” (Nash, 2014). We checked dispersion using the package “blmeco” (Korner-Nievergelt et al., 2015; > 1.4; Table S4; overdispersion <0.75). We centered and standardized covariates prior to analysis. We then undertook likelihood ratio tests to determine which covariates from the top five models were statistically important (Table S5). Considering the results of the likelihood ratio tests, the best fit model was chosen for each call group to analyse the relationship between activity including all covariates identified as significant (Tables S3, S5). We then used the package “multcomp” (Hothorn et al., 2008) to perform a multiple comparison test on these final models.
Data were too zero-inflated to explore foraging activity further with GLMMs. Therefore, we used the non-parametric Mann-Whitney U test to compare foraging activity between the forest and oil palm, as the data were not normally distributed (riparian forests: W= 0.843, p < 0.001). Foraging activity was examined as a proportion of total activity at any given point.
Occupancy
We used hierarchical Bayesian multi-species occupancy models adapted from Deere et al. (2017) to investigate species-specific occurrences in relation to environmental conditions, while accounting for imperfect detection (MacKenzie et al., 2002). This was only possible for seven species that could be identified from the acoustic data. Our framework linked occurrence and detection models for individual species via a hierarchical component that modeled regression coefficients as realizations from a common community-level distribution using hyper-parameters, which assume species respond to environmental conditions in a similar way. Since some species (e.g., Rhinolophus trifoliatus) were much more abundant than rare or elusive species (e.g., Hipposideros ridleyi), their occupancy estimates can be used to improve the estimation precision of the latter where detection records are limited (Pacifici et al., 2014).
We modeled the occurrence of species i at site j using the binary variable, zi, j, where 1 indicates species presence and 0 denotes species absence. The occurrence state was specified as the outcome of a Bernoulli process, zi, j~ Bern(ψi, j), where ψi,j represents the latent occupancy state. The true occurrence state is imperfectly observed so we incorporated a second Bernoulli process, xi, j, k~ Bern(zi, j), where xi, j, k represents the observed detection histories, and pi, j, k is the detection probability of species i, at site j for temporal replicate k. Within this framework, detection probability is conditional on species presence (zi, j= 1), therefore it is not possible to estimate detection probability at a site where a species is absent (zi,j = 0). Our approach assumes abundance of a species does not affect the probability of detection (Royle and Dorazio, 2008). To conduct the analysis, we specified models of the form:
Occupancy and detection probabilities were modeled with intercepts on the logit scale, specific for each species. Five models were used to compare the fit of singular habitat characteristics in explaining species occurrence [Models 2, 3 & 4], against cumulative habitat characteristics [Models 1 & 5]. Covariate selection was partially informed by the results of the GLMM (Table S4). Covariates were centered and standardized prior to analysis to place them on a comparable scale and improve model convergence.
Models were specified using uninformative priors for intercept, variance, and slope parameters. We specified River as a random spatial effect for each species specifically. For each model we ran three parallel MCMC chains for 100,000 iterations each, discarding the first half during the burn-in process and thinning posterior samples by 50. The Gelman-Ruben statistic was assessed to ensure convergence (<1.1 for all parameters; Gelman and Hill, 2007). Widely applicable AIC was subsequently calculated for each model, following Gelman et al. (2013), and used for model selection [Model 5] (<2 ΔAIC; Table S6). This model was then used to describe occupancy and detectability.
Results
Over 49 survey nights, we recorded 24,228 sound files, which were manually identified as 5,696 genuine bat triggers. Bats with frequency modulating quasi-constant frequency calls (FMqCF) were the most active amongst the community, constituting 70% of total activity recorded. Seven CF taxa were identified and constituted 23.7% of all calls (Table 1). The remaining call-types (FMb and MHFM) comprised 6.3% of activity, and so are not explored further due to insufficient data.
Activity
Total Activity and Foraging Activity Between Habitat Types
There was no overall difference in bat activity between forest and oil palm rivers for all calls combined, and also CF species and FMqCF species analyzed separately (Figures 2A–C). Similarly, there was no overall difference in the proportion of foraging activity for activity overall (W = 442, p = 0.964) or for FMqCF species (W = 465.5, p = 0.763; Figures 2D–F). However, the foraging activity of CF species (expressed as a proportion of total activity at a given point) was significantly greater alongside the forest rivers than the rivers in the oil palm (W = 345, p= 0.017).
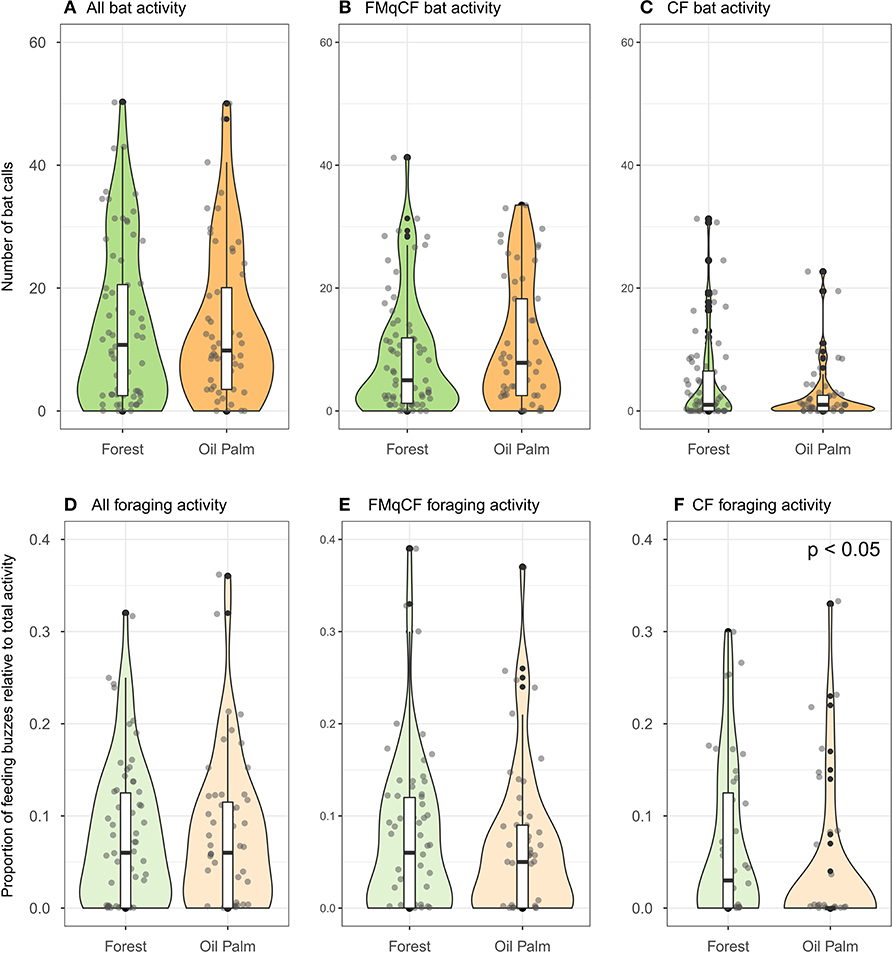
Figure 2. Violin plots and boxplots demonstrating variation in bat activity and foraging activity between rivers in contiguous forest vs. those in oil palm for all species recorded in the study. Points represent bat activity per sampling point (Forest = 72 points across 9 sites; Oil palm = 56 points across 7 sites), and are jittered to improve presentation of the data distribution. (A–C) Represent bat total activity; (D–F) represent foraging activity.
Total Activity in Relation to Habitat Covariates
Considering all study sites and total activity assessed together, the GLMMs revealed bats to be more active at sites with a higher forest canopy (Figure 3; Table S7), a pattern that was also evident for activity of FMqCF-calling bats, but not for CF-calling bats when assessed separately (Table S5). Detection was negatively affected by time-of-recording, with greater activity detected closer to sunset for CF bats, FMqCF bats, and all call groups combined. Habitat type (i.e., forest vs. oil palm) was not identified as an important factor driving activity for any call group.
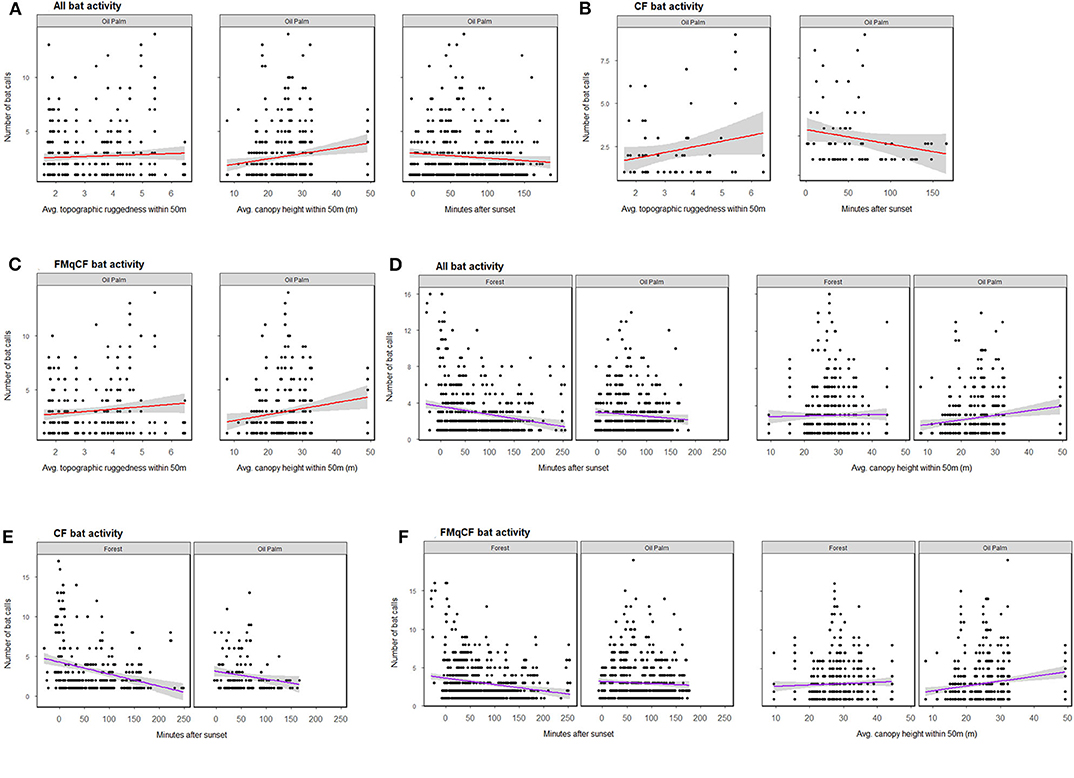
Figure 3. The relationship between bat activity (per sample point) and significant habitat covariates as determined by GLMM analysis for each call group per habitat type. (A–C) Show relationships from GLMMs assessing oil palm exclusively; (D–F) show relationships within both forest and oil palm. Shading represents 95% confidence interval.
When the GLMMs were repeated for the oil palm subset of the data to explore the role of riparian reserve width, bats were again shown to be more active in oil palm reserves with a higher forest canopy, but also those with greater topographic ruggedness. Different habitat covariates were identified to be important for different call groups in oil palm reserves (Figure 3; Table S7). However, activity appeared independent of the width of oil palm reserves for all call groups assessed (Table S5).
Site Occupancy
Occupancy modeling revealed a significant response to the covariates for five of the seven taxa that could be identified to species level (Figure 4). Two species, Hipposideros cervinus and H. galeritus, had a greater occupancy probability at sites that were more rugged topographically. The detectability of H. cervinus, as well as three Rhinolophus species, also declined as time progressed after sunset. The other species exhibited no clear occupancy pattern across the wider landscape or in relation to the covariates investigated.
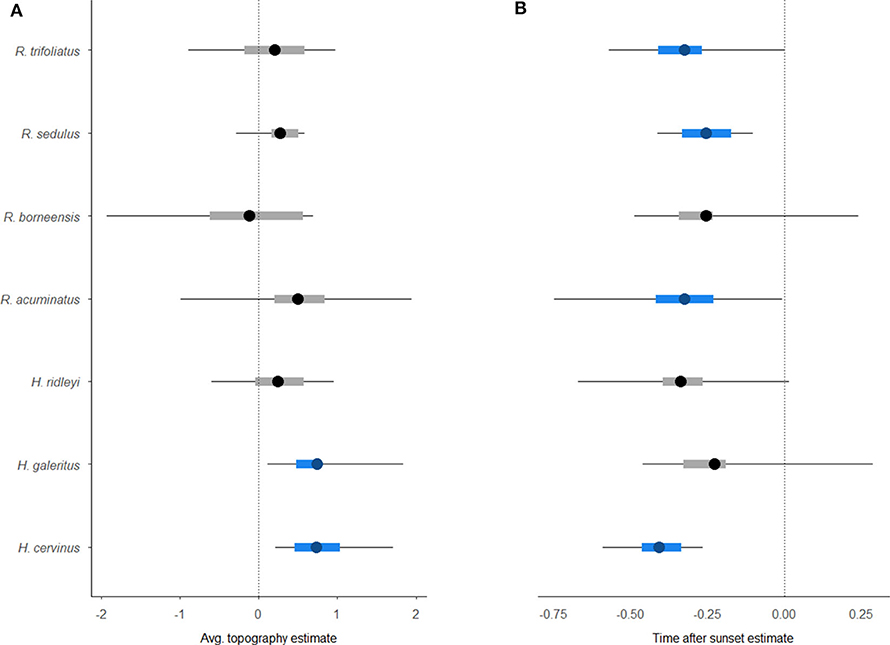
Figure 4. Caterpillar plots of the output from the hierarchical Bayesian multi-species occupancy model [4] (including 95% Bayesian credible interval). Graphs show species-specific baseline occupancy estimates for environmental covariates (A) Topography, and (B) detection covariate TimeafterSunset. Significant associations are highlighted in blue.
Discussion
We demonstrate that riparian reserves in oil palm plantations support comparable levels of insectivorous bat activity to riparian areas in neighboring continuous forest. However, our results demonstrate variation in the importance of specific habitat covariates for different groups of bats, as defined by their echolocation call type. The timing of the survey was an important predictor in all of our analyses. In addition, bat activity was driven by canopy height (i.e., a measure of forest quality), and appeared especially important for bats using FMqCF calls. Topography also had an important influence on activity of bats using CF calls in the oil palm riparian reserves. We also note lower foraging activity recorded in oil palm compared to forested riparian sites for CF species. Overall, our analyses reveal that topography and forest quality may be more important than the width of the riparian reserve in driving bat activity alongside rivers in oil palm reserves. These findings support a previous study of captured bats that demonstrated the role of canopy height in structuring the bat community of disturbed tropical forest (Struebig et al., 2013).
Bat Activity in Riparian Reserves
Bats characterized by FMqCF calls are suited to forest edges and more open habitats, and were the most active group across all study sites, indicating that these bats are quite tolerant to extensive habitat disturbance. Indeed, Struebig et al. (2013) studied changes in bat assemblages across a forest disturbance gradient and found that 85% of edge/open species were captured in repeatedly logged forest. Elsewhere in the tropics, Meyer et al. (2004) also found that FMqCF bats are more abundant/active in open, partially cluttered habitat, such as that typical of heavily logged forest.
We also found that CF bats—those using flutter detection and often perceived to be specialists of cluttered forest environments—were also at high densities in riparian reserves in oil palm compared to the rivers in contiguous forest sites. It is possible that CF activity in riparian reserves is inflated as bats use these landscape features to commute to other sites while avoiding the agricultural land. Differences in foraging activity between the habitat types support this assertion, but we require further recordings in adjacent oil palm estates to be more certain. Forests that are repeatedly logged also tend to comprise fewer and smaller trees with scarcer roosting opportunities in tree cavities (Struebig et al., 2013); making it more likely that tree-roosting CF bats (e.g., R. sedulus, H. ridleyi; Table S2) are ‘passing through' rather than resident in riparian reserves. In theory, the low wing loading and aspect ratios typical of CF bats makes flying long distances energetically expensive (Kingston et al., 2003b), although some cave-roosting CF taxa (e.g., H.cervinus) have narrower wings and have been recaptured in forest fragments 10–12 km from continuous forest sites (Struebig et al., 2008). Therefore, landscape connectivity may also have a role to play in the long-term effectiveness of riparian reserves for these species.
Foraging Activity in Riparian Reserves
There was high variability in foraging activity across riparian reserves as well as between forest and oil palm (Figure 2; Table 1). Oil palm plantations typically have negative consequences on invertebrates (Turner and Foster, 2009), including the reduction in moth diversity (VunKhen, 2006), Diptera and Hemiptera (Edwards et al., 2014), which are known to be prey for insectivorous bats in Sabah (Hemprich-Bennett et al., 2020). Previous studies in this landscape indicate that riparian reserve assemblages of some invertebrate groups, namely ants, and dung beetles, are somewhat similar to those in nearby forest provided riparian reserves are sufficiently wide (Luke et al., 2019). It is therefore feasible that the prey-base in riparian reserves may be sufficient to support some bat species at least, particularly those using FMqCF calls.
Importance of Habitat Structure
Changes to different structural components of riparian reserve habitats have differing implications for bat species. The long constant-frequency CF component of Rhinolophid and Hipposiderid bats generates a continuous input of environmental information (Russo et al., 2018). Consequently, these taxa are able to navigate more cluttered environments of lower canopy forests (Monadjem and Reside, 2008), and therefore may be less affected by canopy height than bats using other call types. On the other hand, FMqCF bats are restricted to less cluttered environments; predominantly foraging along the edge, in gaps, or above forest (Altringham, 1996; Table S2). Monadjem and Reside (2008) found the inverse relationship between canopy height and FMqCF bats in Acacia-dominated savanna in Swaziland, where higher activity was recorded in riparian reserves with lower canopies. This may be due to differences in structural complexity between savannah and rainforest. However, Monadjem & Reside also proposed that, unlike birds, bats discriminate between microhabitats rather than larger-scale habitat features. Topographic complexity and canopy height are known to predict the variation in local microclimate within the SAFE project (Jucker et al., 2018), e.g., taller canopies reduce diurnal variation in microclimatic. This could benefit aerial invertebrates for which abundance positively correlates with canopy height in repeatedly logged forest in Malaysia (Akutsu et al., 2007). Species, such as the Lesser bamboo bat (Tylonycteris pachypus), which utilize quasi-constant frequency (FMqCF) calls may adjust the relative dominance of each component on environmental demand, e.g., reduce the FM component and increase the CF component in open spaces (Kalko and Schnitzler, 1993; Altringham, 1996). This adaptability allows bats that employ this echolocation technique to exploit multiple habitat types, thereby reducing their susceptibility to disturbance. As bat activity is correlated with prey availability (Kusch et al., 2004; Müller et al., 2012), higher prey abundance in higher canopy forest may be indirectly driving the increase in FMqCF bat activity observed within our study.
Areas with higher topographic ruggedness are more difficult to access for logging. As such, it is likely that rugged areas provide an indirect benefit to wildlife by providing a refuge for species that require mature forest, rather than directly affecting their survival. Although riparian reserve width did not directly affect bat activity or species occurrence at river sites, forest extent can be important for maintaining a taller forest canopy. Edge effects can lead to increased mortality of mature trees, which in turn leads to a decrease in canopy height (Laurance et al., 2018). Therefore, riparian reserves should still be as wide as possible to reduce the long-term risk from edge-effects. The minimum 40 m width threshold on each riverbank recommended for birds may be appropriate in this regard, because reserves of this size also represent greater tree biomass and higher forest canopies (Mitchell et al., 2018). This seems all the more important when considering that bat activity was highest in the most topographically-rugged oil palm reserve sites, which were typically rocky and steep terrain prone to tree falls and landslides.
Detection
Both the GLMM and occupancy analysis highlighted the importance of considering the influence of time in acoustic survey designs. The emergence time and hours of peak activity vary between bat species and different foraging guilds (Jones and Rydell, 1994). Our study confirms sampling effort may not be comparable if surveys are not controlled for temporal variation. Different call-types are also not sampled equally using acoustic monitoring due to the differences in intensity of their echolocation calls (Hayes, 2000). For example, species utilizing FMqCF calls, which are high intensity, were recorded abundantly, but other call-types may not have been detected as easily. Forest-dwelling species recorded by capture surveys in the forest, such as Megaderma spasma and Nycteris tragata have low intensity (MHFM) calls, whilst Vespertilionidae (Kerivoula and Myotis spp.) have low to intermediate intensity calls (FMb) (Waters and Jones, 1995). More cluttered environments also reduce the detection distance for echolocation calls (Russo et al., 2018). Therefore, both their call-type and their preferred habitat are likely to contribute to these species being under-represented in our sampling. While this somewhat contravenes a key assumption of the occupancy models applied here (i.e., that detection is not influenced by abundance), we note that the conclusions of these models supported those of the GLMMs, and so we consider the outcome robust. Live trapping could be used to complement acoustic sampling to better represent these species, although we maintain that this remains logistically prohibitive in many tropical landscapes, particularly in and around rivers.
Conclusions
There is growing recognition that riparian reserves have a positive role to play in biodiversity conservation within tropical agricultural landscapes, but the evidence base remains weak (Luke et al., 2019). Our study confirms that these reserves in oil palm support comparable levels of bat activity to otherwise forested river sites. As palm oil production continues to rise, it is important to use evidence-based decision-making to inform more sustainable practices. With this in mind, our study highlights the importance of considering simple measures of habitat structure in the design of riparian reserves since taller vegetation supported higher levels of activity. If reflective of other taxa, this indicates that the designation and protection of riparian reserves should continue to be promoted on biodiversity grounds, with the onus on taller, more structurally complex forest, being retained alongside rivers within plantations.
Data Availability Statement
Publicly available datasets were analyzed in this study. This data can be found here: https://zenodo.org/record/3901505.
Ethics Statement
The animal study was reviewed and approved by School of Anthropology and Conservation Research Ethics Committee, University of Kent.
Author Contributions
MS and HB conceived and designed the study. KM, SM, and MS conducted the fieldwork. KM and SB processed the bat calls. NY and DS performed the statistical analysis. NY, KM, and MS wrote the manuscript. All authors contributed to manuscript revision, read, and approved the submitted version.
Funding
This study was funded by the UK Natural Environment Research Council (NERC) via the Human Modified Tropical Forests Programme (NE/K016407/1; http://lombok.hmtf.info). SM was supported by a Ph.D. scholarship jointly funded by University of Kent and NERC. NY was supported by NERC through the EnvEast DTP scholarship (grant number NE/L002582/1).
Conflict of Interest
The authors declare that the research was conducted in the absence of any commercial or financial relationships that could be construed as a potential conflict of interest.
Acknowledgments
We thank the Sabah Biodiversity Council, Sabah Forest Department, Yayasan Sabah, and Benta Wawasan Sdn Bhd. for research permissions and site access and the many research assistants at the SAFE project site that helped with the bat surveys. We also thank Nick Deere for statistical advice regarding the occupancy analysis and the Coomes Lab at University of Cambridge for access to LiDAR information.
Supplementary Material
The Supplementary Material for this article can be found online at: https://www.frontiersin.org/articles/10.3389/ffgc.2020.00073/full#supplementary-material
Footnotes
References
Akutsu, K., Khen, C. V., and Toda, M. J. (2007). Assessment of higher insect taxa as bioindicators for different logging-disturbance regimes in lowland tropical rain forest in Sabah, Malaysia. Ecol. Res. 22, 542–550. doi: 10.1007/s11284-006-0052-6
Bates, D., Mächler, M., Bolker, B., and Walker, S. (2015). Fitting linear mixed-effects models using lme4. J. Stat. Soft. 67, 1–48. doi: 10.18637/jss.v067.i01
Carroll, C., Noss, R. F., Paquet, P. C., and Schumaker, N. H. (2004). Extinction debt of protected areas in developing landscapes. Conserv. Biol. 18, 1110–1120. doi: 10.1111/j.1523-1739.2004.00083.x
de Peña-Cuéllar, E., Benítez-Malvido, J., Avila-Cabadilla, L. D., Martínez-Ramos, M., Estrada, A., et al. (2015). Structure and diversity of phyllostomid bat assemblages on riparian corridors in a human-dominated tropical landscape. Ecol. Evol. 5, 903–913. doi: 10.1002/ece3.1375
Deere, N. J., Guillera-Arroita, G., Baking, E. L., Bernard, H., Pfeifer, M., Reynolds, G., et al. (2017). High carbon stock forests provide co-benefits for tropical biodiversity. J. Appl. Ecol. 55, 997–1008. doi: 10.1111/1365-2664.13023
Deere, N. J., Guillera-Arroita, G., Platts, P. J., Mitchell, S. L., Baking, E. L., Bernard, H., et al. (2020). Implications of zero-deforestation commitments: forest quality and hunting pressure limit mammal persistence in fragmented tropical landscapes. Conserv. Lett. doi: 10.1111/conl.12701. [Epub ahead of print].
Denzinger, A., and Schnitzler, H. (2013). Bat guilds, a concept to classify the highly diverse foraging and echolocation behaviors of microchiropteran bats. Front. Physiol. 4:164. doi: 10.3389/fphys.2013.00164
Edwards, D. P., Magrach, A., Woodcock, P., Ji, Y., Lim, N. T.-, Edwards, F. A., et al. (2014). Selective-logging and oil palm: multitaxon impacts, biodiversity indicators, and trade-offs for conservation planning. Ecol. Appl. 24, 2029–2049. doi: 10.1890/14-0010.1
Fitzherbert, E. B., Struebig, M. J., Morel, A., Danielsen, F., Brühl, C. A., Donald, P. F., et al. (2008). How will oil palm expansion affect biodiversity? Trends Ecol. Evol. 23, 538–545. doi: 10.1016/j.tree.2008.06.012
Galindo-González, J., and Sosa, V. J. (2003). Frugivorous bats in isolated trees and riparian vegetation associated with human-made pastures in a fragmented tropical landscape. Southwest Nat. 48, 579–90. doi: 10.1894/0038-4909(2003)048<0579:FBIITA>2.0.CO;2
Gelman, A., and Hill, J. (2007). Data Analysis using Regression and Multilevel/Hierarchical Models. Cambridge University Press. doi: 10.1017/CBO9780511790942
Gelman, A., Hwang, J., and Vehtari, A. (2013). Understanding predictive information criteria for Bayesian models. arXiv [statME]. doi: 10.1007/s11222-013-9416-2
Hansen, M. C., Potapov, P. V., Moore, R., Hancher, M., Turubanova, S. A., Tyukavina, A., et al. (2013). High-resolution global maps of 21st-century forest cover change. Science 15, 850–853. doi: 10.1126/science.1244693
Hayes, J. P. (2000). Assumptions and practical considerations in the design and interpretation of echolocation-monitoring studies. Acta Chiropt 2, 225–236.
Hemprich-Bennett, D. R., Kemp, V. A., Blackman, D., Struebig, M. J., Lewis, O. T., Rossiter, S. J., et al. (2020). Altered structure and stability of bat-prey interaction networks in logged tropical forests revealed by metabarcoding. bioRxiv 2020:000331 doi: 10.1101/2020.03.20.000331
Hothorn, T., Bretz, F., and Westfall, P. (2008). Simultaneous inference in general parametric models. Biom. J. 50, 346–363. doi: 10.1002/bimj.200810425
Jones, G., Jacobs, D. S., Kunz, T. H., Willig, M. R., and Racey, P. A. (2009). Carpe noctem: the importance of bats as bioindicators. Endanger Species Res. 8, 93–115. doi: 10.3354/esr00182
Jones, G., and Rydell, J. (1994). Foraging strategy and predation risk as factors influencing emergence time in echolocating bats. Philos. Trans. R. Soc. Lond. B Biol. Sci. 346, 445–455. doi: 10.1098/rstb.1994.0161
Jucker, T., Hardwick, S. R., Both, S., Elias, D. M. O., Ewers, R. M., Milodowski, D. T., et al. (2018). Canopy structure and topography jointly constrain the microclimate of human-modified tropical landscapes. Glob. Chang. Biol. 24, 5243–5258. doi: 10.1111/gcb.14415
Kalko, E. K. V., and Schnitzler, H.-U. (1993). Plasticity in echolocation signals of European pipistrelle bats in search flight: implications for habitat use and prey detection. Behav. Ecol. Sociobiol. 33, 415–428. doi: 10.1007/BF00170257
Kingston, T. (2010). Research priorities for bat conservation in Southeast Asia: a consensus approach. Biodivers. Conserv. 19, 471–484. doi: 10.1007/s10531-008-9458-5
Kingston, T., Francis, C. M., Akbar, Z., and Kunz, T. H. (2003a). Species richness in an insectivorous bat assemblage from Malaysia. J. Trop Ecol. 19, 67–79. doi: 10.1017/S0266467403003080
Kingston, T., Jones, G., Akbar, Z., and Kunz, T. H. (2003b). Alternation of Echolocation Calls in 5 Species of Aerial-Feeding Insectivorous Bats from Malaysia. J. Mammal. 84, 205–15. doi: 10.1644/1545-1542(2003)084<0205:AOECIS>2.0.CO;2
Korner-Nievergelt, F., Roth, T., von Felten, S., Guélat, J, Almasi, B., and Korner-Nievergelt, P. (2015). Bayesian Data Analysis in Ecology using Linear Models with R, BUGS and Stan. Academic Press.
Kusch, J., Weber, C., Idelberger, S., and Koob, T. (2004). Foraging habitat preferences of bats in relation to food supply and spatial vegetation structures in a western European low mountain range forest. Folia Zool. 53, 113–128.
Lane, D. J. W., Kingston, T., and Lee, B. P. Y.-H. (2006). Dramatic decline in bat species richness in Singapore, with implications for Southeast Asia. Biol. Conserv. 131, 584–593. doi: 10.1016/j.biocon.2006.03.005
Laurance, W. F., Camargo, J. L. C., Fearnside, P. M., Lovejoy, T. E., Williamson, G. B., Mesquita, R. C. G., et al. (2018). An Amazonian rainforest and its fragments as a laboratory of global change: Amazonian fragments and global change. Biol. Rev. 93, 223–247. doi: 10.1111/brv.12343
Luke, S. H., Slade, E. M., Gray, C. L., Annammala, K. V., Drewer, J., Williamson, J., et al. (2019). Riparian buffers in tropical agriculture: scientific support, effectiveness and directions for policy (T Siqueira, ed). J. Appl. Ecol. 56, 85–92. doi: 10.1111/1365-2664.13280
MacKenzie, D. I., Nichols, J. D., Lachman, G. B., Droege, S., Royle, J. A., et al. (2002). Estimating site occupancy rates when detection probabilities are less than one. Ecology 83, 2248–2255. doi: 10.1890/0012-9658(2002)083[2248:ESORWD]2.0.CO;2
Meijaard, E., Garcia-Ulloa, J., Sheil, D., Wich, S. A., Carlson, K. M., Juffe-Bignoli, D., et al. (2018). Oil palm and biodiversity: a situation analysis by the IUCN Oil Palm Task Force. Gland: International Union for Conservation of Nature and Natural Resources (IUCN). doi: 10.2305/IUCN.CH.2018.11.en
Meyer, C. F. J., Schwarz, C. J., and Fahr, J. (2004). Activity patterns and habitat preferences of insectivorous bats in a West African forest–savanna mosaic. J. Trop. Ecol. 20, 397–407. doi: 10.1017/S0266467404001373
Mitchell, S. L., Edwards, D. P., Bernard, H., Coomes, D., Jucker, T., Davies, Z. G., et al. (2018). Riparian reserves help protect forest bird communities in oil palm dominated landscapes. J. Appl. Ecol. 55, 2744–2755. doi: 10.1111/1365-2664.13233
Monadjem, A., and Reside, A. (2008). The influence of riparian vegetation on the distribution and abundance of bats in an African savanna. Acta Chiropterol. 10, 339–348. doi: 10.3161/150811008X414917
Müller, J., Mehr, M., Bässler, C., Fenton, M. B., Hothorn, T., Pretzsch, H., et al. (2012). Aggregative response in bats: prey abundance versus habitat. Oecologia 169, 673–684. doi: 10.1007/s00442-011-2247-y
Naiman, R. J., Decamps, H., and McClain, M. E. (2010). Riparia: Ecology, Conservation, and Management of Streamside Communities. London: Elsevier.
Nash, J. C. (2014). On best practice optimization methods in R. J. Stat. Soft. 60, 1–14. Available online at: http://www.jstatsoft.org/v60/i02/
Pacifici, K., Zipkin, E. F., Collazo, J. A., Irizarry, J. I., Dewan, A., et al. (2014). Guidelines for a priori grouping of species in hierarchical community models. Ecol. Evol. 4, 877–888. doi: 10.1002/ece3.976
Padfield, R., Hansen, S., Davies, Z. G., Ehrensperger, A., Slade, E. M., Evers, S., et al. (2019). Co-producing a research agenda for sustainable palm oil. Front. Forests Glob. Change. doi: 10.3389/ffgc.2019.00013
Phalan, B., Bertzky, M., Butchart, S. H. M., Donald, P. F., Scharlemann, J. P. W., Stattersfield, A. J., et al. (2013). Crop expansion and conservation priorities in tropical countries. PLoS ONE 8:e51759. doi: 10.1371/journal.pone.0051759
Pirker, J., Mosnier, A., Kraxner, F., Havlík, P., and Obersteiner, M. (2016). What are the limits to oil palm expansion? Glob. Environ. Change 40, 73–81. doi: 10.1016/j.gloenvcha.2016.06.007
R Core Team (2014). R: A Language and Environment for Statistical Computing. Vienna: R Foundation for Statistical Computing. Available online at: http://www.R-project.org/
Rosa, I. M. D., Smith, M. J., Wearn, O. R., Purves, D., and Ewers, R. M. (2016). The environmental legacy of modern tropical deforestation. Curr. Biol. 26, 2161–2166. doi: 10.1016/j.cub.2016.06.013
Royle, J. A., and Dorazio, R. M. (2008). Hierarchical Modeling and Inference in Ecology. New York, NY: Academic Press.
RSPO (2018). RSPO Principles and Criteria for the Production of Sustainable Palm Oil 2018. Kuala Lumpur: RSPO.
Russo, D., Ancillotto, L., and Jones, G. (2018). Bats are still not birds in the digital era: echolocation call variation and why it matters for bat species identification. Can. J. Zool. 96, 63–78. doi: 10.1139/cjz-2017-0089
Sodhi, N. S., Brook, B. W., and Bradshaw, J. (2013). Tropical Conservation Biology. Oxford: John Wiley and Sons.
Struebig, M. J., Kingston, T., Petit, E. J., Le Comber, S. C., Zubaid, A., Mohd-Adnan, A., et al. (2011). Parallel declines in species and genetic diversity in tropical forest fragments. Ecol. Lett. 14, 582–590. doi: 10.1111/j.1461-0248.2011.01623.x
Struebig, M. J., Kingston, T., Zubaid, A., Mohd-Adnan, A., and Rossiter, S. J. (2008). Conservation value of forest fragments to palaeotropical bats. Biol. Conserv. 141, 2112–2126. doi: 10.1016/j.biocon.2008.06.009
Struebig, M. J., Turner, A., Giles, E., Lasmana, F., Tollington, S., Bernard, H., et al. (2013). Quantifying the biodiversity value of repeatedly logged rainforests. Adv. Ecol. Res. 48, 183–224. doi: 10.1016/B978-0-12-417199-2.00003-3
Swinfield, T., Both, S., Riutta, T., Bongalov, B., Elias, B., Majalap-Lee, N., et al. (2020). Imaging spectroscopy reveals the effects of topography and logging on the leaf chemistry of tropical forest canopy trees. Glob. Change Biol. 26, 989–1002. doi: 10.1111/gcb.14903
Tabacchi, E., Hélène, L. L. G., Planty-Tabacchi, A., Muller, A.-M., Decamps, E., et al. (2000). Impacts of riparian vegetation on hydrological processes. Hydrol. Process 14, 2959–2976. doi: 10.1002/1099-1085(200011/12)14:16/17<2959::AID-HYP129>3.0.CO;2-B
Turner, E. C., and Foster, W. A. (2009). The impact of forest conversion to oil palm on arthropod abundance and biomass in Sabah, Malaysia. J. Trop. Ecol. 25, 23–30. doi: 10.1017/S0266467408005658
Vaughan, N., Jones, G., and Harris, S. (1996). Effects of sewage effluent on the activity of bats (Chiroptera: Vespertilionidae) foraging along rivers. Biol. Conserv. 78, 337–343. doi: 10.1016/S0006-3207(96)00009-2
VunKhen, C. (2006). Impacts of forest conversion on biodiversity as indicated by moths. Malay Nat. J. 57, 383–418.
Waters, D. A., and Jones, G. (1995). Echolocation call structure and intensity in five species of insectivorous bats. J. Exp. Biol. 198, 475–489.
Keywords: chiroptera, Southeast Asia, palm oil, riparian buffer, acoustic monitoring, habitat fragmentation, land-use change, biodiversity
Citation: Mullin KE, Yoh N, Mitchell SL, Basrur S, Seaman DJI, Bernard H and Struebig MJ (2020) Riparian Reserves Promote Insectivorous Bat Activity in Oil Palm Dominated Landscapes. Front. For. Glob. Change 3:73. doi: 10.3389/ffgc.2020.00073
Received: 31 December 2019; Accepted: 20 May 2020;
Published: 02 July 2020.
Edited by:
Janice Ser Huay Lee, Nanyang Technological University, SingaporeReviewed by:
Susan M. Tsang, American Museum of Natural History, United StatesSarah Anne-Leigh Scriven, University of York, United Kingdom
Susannah Fleiss, University of York, United Kingdom, in collaboration with reviewer SA-LS
Copyright © 2020 Mullin, Yoh, Mitchell, Basrur, Seaman, Bernard and Struebig. This is an open-access article distributed under the terms of the Creative Commons Attribution License (CC BY). The use, distribution or reproduction in other forums is permitted, provided the original author(s) and the copyright owner(s) are credited and that the original publication in this journal is cited, in accordance with accepted academic practice. No use, distribution or reproduction is permitted which does not comply with these terms.
*Correspondence: Matthew J. Struebig, bS5qLnN0cnVlYmlnQGtlbnQuYWMudWs=
†These authors have contributed equally to this work