- 1Department of Biological Sciences, Dartmouth College, Hanover, NH, United States
- 2Department of Natural Resources and the Environment, University of New Hampshire, Durham, NH, United States
- 3Department of Biology, Young Harris College, Young Harris, GA, United States
Mycorrhizal fungi can affect soil organic matter cycling through several mechanisms including priming, nutrient competition, and direct enzyme production. Differences in nutrient foraging strategies between ectomycorrhizal (EcM) and arbuscular mycorrhizal (AM) fungi produce divergent belowground dynamics: where EcM can take up organic nitrogen and directly break down soil organic matter (SOM) by producing enzymes, AM fungi are limited to scavenging mineral N. EcM-associated tree species also have leaf litter with relatively higher ratios of carbon to nitrogen (C:N), and belowground saprotrophic communities more dominated by fungi. Consequently, free-living microbes in EcM-dominated soils should experience nitrogen limitation, with subsequent increases in enzyme production and decreased carbon use efficiency (CUE). However, the relative importance of the effects of substrate quality and fungal community composition on enzyme production and CUE are unclear. To assess this distinction, we sampled the organic horizon and 10 cm of the mineral horizon in northern temperate forest soils along a gradient of EcM dominance. We characterized fungal community composition by measuring EcM relative abundances from extracted fungal DNA and the fungal to bacterial (F:B) ratios from phospholipid fatty acid (PLFA) analysis. We assessed soil substrate quality as the soil C:N ratio. Soil microbial functions were measured as potential activities of five hydrolytic and two oxidative enzymes, and microbial CUE. We found that the fungal community, represented by either the F:B ratio, EcM relative abundance, or both, affected CUE and six measured enzyme activities, while the C:N ratio affected only oxidative and chitin-targeting extracellular enzyme activities. Our results highlight the use of EcM relative dominance as a predictor of soil microbial community composition and function independent of substrate quality.
Introduction
Global patterns show significant correlation between the mycorrhizal associations of canopy trees and soil carbon (C) and nitrogen (N) storage (Averill et al., 2014; Zhu et al., 2018; Lu and Hedin, 2019). Trees generally form symbioses with either arbuscular mycorrhizal (AM) or ectomycorrhizal (EcM) fungi where in return for increased soil nutrient uptake via mycorrhizal hyphae, plants exude a significant portion of their photo-assimilated carbon belowground to their mycorrhizal symbionts (Simard et al., 2003; Smith and Read, 2009; Ouimette et al., 2019). AM and EcM fungi have different nutrient acquisition strategies: while EcM fungi can more actively pursue soil organic N and compete with the surrounding soil microbial community, AM fungi are restricted to passively obtaining inorganic N after it has been liberated by other soil decomposers (Phillips et al., 2013). These strategies likely have divergent effects on the free-living bacterial and fungal saprotrophs thought to be primarily responsible for organic matter decomposition (Cheeke et al., 2017; Verbruggen et al., 2017; Lang et al., 2019). In order to understand the mycorrhizal controls on soil organic matter cycling observed at the global scale, we need to investigate how dominant mycorrhizal type (EcM versus AM) affects the function of soil microbial communities.
Mycorrhizal fungi can indirectly, and sometimes directly, affect soil extracellular enzyme activity (EEA), an important step in soil organic matter (SOM) decay. Many species of EcM fungi are able to produce enzymes that degrade plant cell walls, aiding in their ability to more directly access and compete for nutrients contained within organic matter (Cullings and Courty, 2009; Kohler et al., 2015). Given that mycorrhizal hyphal biomass can represent a third or more of the total microbial biomass in forest soils (Högberg and Högberg, 2002), the overall effect of EcM enzyme production should have a significant effect on organic matter decay (sensu Zak et al., 2019) and nutrient liberation even if their enzyme production rate is less than that of saprotrophic fungi (Talbot et al., 2008; Lindahl and Tunlid, 2015; Martin et al., 2016). Both EcM and AM fungi affect the surrounding soil microbial community by priming free-living saprotrophs with labile exudates to induce extracellular enzyme production and subsequent nutrient release (Hodge et al., 2001; Talbot et al., 2008; Kaiser et al., 2015; Gorka et al., 2019). This mycorrhizal-mediated priming mechanism can drastically increase or decrease depending on available soil nutrients, leading to shifts in the extracellular enzyme activity (EEA) of free-living microbes. Excess N, for example, has been shown to significantly decrease mycorrhizal-mediated exudation and EEA likely because plants decrease mycorrhizal access to photosynthate (Sinsabaugh et al., 2005; Högberg et al., 2010; Gorka et al., 2019).
This interaction between mycorrhizal priming, competition for nutrients, and differences in enzyme production is likely to affect microbial carbon use efficiency (CUE), the proportion of processed C that is incorporated into microbial biomass versus respired as CO2. Specifically, microbes with access to an adequate supply of nutrients and easily assimilable substrates are expected to allocate more acquired C to biomass and less to CO2 during respiration (Cotrufo et al., 2013; Sinsabaugh et al., 2013; Geyer et al., 2016). These circumstances promoting elevated community CUE are hypothesized to be greater where mycorrhizal-mediated exudation rates are also higher. AM fungi are likely to have higher exudation rates relative to EcM fungi as they must induce free-living saprotrophic microbes to produce exoenzymes and liberate nutrients (Hodge et al., 2001; Kaiser et al., 2015). In EcM-dominated forests, competition for N between saprotrophic and mycorrhizal fungi has been associated with lower soil respiration and more C in the organic horizon, likely due to a suppression of saprotrophic microbial growth and biomass (Verbruggen et al., 2017; Zhu et al., 2018), potentially reducing CUE (Anthony et al., 2020). Further, increasing fungal:bacterial ratios in soils, which can be seen with increasing EcM dominance, have also been associated with less efficient community decomposition as saprotrophic fungi are less efficient than bacteria on average (Cheeke et al., 2017; Silva-Sánchez et al., 2019; Soares and Rousk, 2019). While C exudation by mycorrhizal fungi could promote a high microbial CUE in their saprotrophic neighbors, the activity of EcM fungi specifically may instead offset this positive effect by enhancing competition for N or by restricting exudation when N availability is high (Gorka et al., 2019; Hestrin et al., 2019).
Disentangling the effects of mycorrhizal fungi on the free-living soil microbial community is further complicated by differences in the quality of AM and EcM tree leaf litter, an important C and nutrient source for saprotrophic microbes. AM-associated trees usually have high quality, lower carbon:nitrogen (C:N) leaf litter and EcM-associated trees have lower quality, higher C:N leaf litter (Cornelissen et al., 2001; Sun et al., 2018). The quality of organic substrates may affect CUE and enzyme production since low-quality substrates contain fewer nutrients and are relatively slow to break down (Saiya-Corka et al., 2002; Cotrufo et al., 2013). For example, litter with a low C:N degrades quickly and has been found to promote more efficient microbial growth or faster litter decomposition, but without a consistent increase in enzyme production (Sinsabaugh et al., 2002; Frey et al., 2013; Lashermes et al., 2016). These findings suggest that substrate quality, CUE, and enzyme production are likely linked to interactions between mycorrhizal fungi and their free-living soil microbial community (Allison, 2012b). Yet, the degree to which substrate quality and dominant mycorrhizal type influence soil microbial function remains poorly described.
In order to understand how mycorrhizal associations affect soil microbial function, we assessed two hypothesized drivers of mycorrhizal control, fungal community composition and substrate quality, and related them to soil microbial functions—community CUE and potential extracellular enzyme activities. We measured these potential drivers and functions along a gradient of EcM-associated tree basal area (subsequently referred to as EcM basal area) in northern temperate forests. We characterized (i) changes in fungal community composition (assessed here as EcM relative abundance, AM biomass, and fungal:bacterial ratios) and (ii) substrate quality (assessed as soil C:N) with soil horizon (organic and top 10 cm of mineral soil). We tested three hypotheses: (i) With increasing EcM basal area, soil C:N, EcM relative abundance, and fungal to bacterial (F:B) would all increase, while AM biomass would decrease; (ii) the relative importance of substrate quality on extracellular enzyme activities would decrease from the organic to the mineral soil, because the organic horizon contains more complex organic substrates requiring enzymatic degradation; and (iii) the fungal community composition would be a more important driver of CUE than substrate quality. Specifically, we predicted that the CUE of the free-living microbial community would decrease as EcM became more dominant due to EcM-driven competition for N and subsequent saprotrophic growth limitation. We further predicted a decline in free-living community CUE where EcM dominate, because we expected a concomitant increase in overall fungal dominance (i.e., increasing F:B), and saprotrophic fungi are less efficient per unit substrate than bacteria.
Materials and Methods
Study Sites
We collected soils across gradients of EcM basal area at two sites, one in Norwich, Vermont (43.72°N, 72.35°W) and one in Hanover, New Hampshire (43.72°N, 72.16°W) on 22 May 2019, during the early growing season. These sites are classified as mixed hardwood forest on inceptisols. We collected soils from 10, 10 m radius plots across a gradient of EcM basal area (Supplementary Table S1). Within each plot, we measured the diameter at breast height (1.4 m) of all trees greater than 10 cm in diameter and identified each tree to species. We assigned all trees as EcM or AM based on their family (Supplementary Table S1). The basal area of Salicaceae was assumed to be 50% EcM, and only one species from our plots, Populus tremuloides, was in this family. EcM species in the lowest EcM basal area plot were represented mostly by Betula paperifera, while mid-range EcM basal area plots included an increasing presence of Fagus grandifolia, P. tremuloides, and Quercus Rubra. The highest EcM basal area plots were dominated by Tsuga canadensis.
Soil Collection and Processing
At each site, we collected soil cores of the organic horizon, which ranged in thickness from 4 to 12 cm, and the corresponding top 10 cm of mineral soil. We collected soils cores from two spots at the center of our circular plots and combined those cores for analysis. To collect the cores, we first cut out and set aside the O horizon within the footprint of a 5 cm diameter stainless steel corer, which we then used to collect the mineral soil. Samples were transported back to the lab on ice and stored at 4°C before further processing. Organic horizon samples were root picked and thoroughly mixed while mineral samples were sieved through a 2 mm mesh. Subsamples were dried overnight at 100°C, ground, and later analyzed for percent soil C and N using an ECS 4010 CHNSO analyzer (Costech Analytical Technologies, Inc., Valencia, CA, United States). Soil pH was measured with a 1:1 ratio of soil to deionized water from the thawed organic horizon and mineral soils using a benchtop pH meter (Mettler Toledo, Columbus, OH, United States).
Extracellular Enzyme Assays
We performed fluorometric and colorimetric assays of EEA using methods modified from German et al. (2011) and Allison (2012a). We measured EEA for hydrolases: β-glucosidase (BG; glucose from cellulose), cellobiohydrolase (CB; disaccahrides from cellulose), N-acetyl-glucosaminidase (NAG; chitin), Leucine-aminopeptidase (LAP; amino acids from proteins), and acid phosphatase (AP; phosphate ions from organic compounds); and two oxidases: phenol oxidase (PO) and peroxidase (PER). In brief, soil subsamples (1 g) from thawed soils were homogenized using an immersion blender with 125 ml of deionized water, to ensure capturing in situ soil pH. 200 μl of sample homogenate was pipetted into 96-well plates with 50 μl of each 4-Methylumbelliferone labeled-fluorogenic substrate in replicates of four and incubated in the dark at 12°C (Koch et al., 2007). Fluorescence was measured on a microplate spectrophotometer (365 nm excitation, 450 nm emission) for hydrolases after addition of 15 μl of 1 M NaOH. For PO and PER, the samples were mixed with 50 μl L-3,4-dihydroxyphenylalanine (L-DOPA) or 50 μl L-DOPA and 20 μl hydrogen peroxide, respectively, incubated in the dark at 12°C for 18 h, and then their absorbance (460 nm) was measured on the microplate reader. We used a principle component analysis (PCA) to group enzymes for subsequent statistical analyses based on relatedness across samples (Supplementary Figure S1). BG and CB were summed to create a hydrolytic activity metric as these enzymes target plant derived, carbon-based molecules (Emilson et al., 2016), and LAP and AP were grouped as nutrient-targeting enzymes given their similar PCA loadings (Supplementary Figure S1 and Supplementary Table S2).
Microbial Carbon Use Efficiency
We measured CUE of the organic and mineral soil microbial communities following an 18O tracing method, where CUE is estimated based on 18O-water incorporation into microbial DNA (Spohn et al., 2016; Geyer et al., 2019). Microbial communities were first acclimated by pre-incubating samples for 5 days at 12°C. We added a dilution of labeled 18O-water and 16O-water to 0.3 g of soil from the same sample in separate 2 ml microcentrifuge tubes. Dilutions of 18O-water were determined by optimizing for a 20 atom% label in each sample without exceeding the average water holding capacity of the mineral and organic soils (see Data Availability Statement section for link to data repository which contains spreadsheet “18O_CUEcalcs.SCxTS.2019.xls,” tab name “prep calcs,” for full dilution calculations). In short, we aimed for soils to be moist, but not saturated to avoid anaerobic conditions. We then inserted the microcentrifuge tubes into 50 ml glass incubation bottles, which were sealed and flushed with CO2-free air prior to a 48 h incubation at 12°C. Following incubation, we measured 10 ml of headspace CO2 using an EGM-5 CO2 gas analyzer (PP-Systems, Amesbury, MA, United States). We removed microcentrifuge tubes from bottles and extracted microbial DNA following modified methods, where the entire supernatant was transferred in order to extract total DNA as opposed to a subsample, and using materials from the Qiagen DNeasy Powersoil extraction protocol and kit. An important modification step is to use all the supernatant in every step (instead of a subset), as the final calculations are based on the amount of 18O incorporated in the DNA. We added 70 μl of the final extractions to silver capsules (5 × 3.5 mm) along with 100 μl of 10 ng ml–1 salmon sperm to raise the oxygen to detection level. We then dried samples at 60°C overnight and sent the capsules in a 96-well plate to the UC Davis Stable Isotope facility for 18O analysis on an elementar PyroCube (Elementar Analysensysteme GmbH, Hanau, Germany) and interfaced to an Isoprime VisION (Isoprime Ltd., Stockport, United Kingdom, a unit of Elementar Analysensysteme GmbH, Hanau, Germany).
In parallel, we analyzed total organic carbon (TOC) in microbial cells by performing a chloroform fumigation and subsequent TOC extraction on duplicate 15 mg thawed soil samples (Vance et al., 1987). One set of samples was fumigated with chloroform overnight to lyze microbial cells. TOC was subsequently extracted from both fumigated and duplicate non-fumigated soil using 0.5 M KCL. The extracts were diluted to a ratio of 1:4 for TOC analysis on a Sievers M5310 C Laboratory TOC analyzer (SUEZ, Paramous, NJ, United States).
DNA Extraction and Bioinformatics
Fungal DNA was extracted from organic horizon and mineral soils using the Quiagen PowerSoil Kit (Qiagen Science Inc., Germantown, MD, United States). DNA extracts were sent to the University of Wisconsin-Madison Biotechnology Center where they were prepared similarly to the process detailed in Illumina’s 16s Metagenomic Sequencing Library Preparation Protocol, Part # 15044223 Rev. B (Illumina Inc., San Diego, CA, United States). The ITS region was amplified using the forward primers ITS (CTTGGTCATTTAGAGGAAGTAA) and reverse primer ITS (TCCTCCGCTTATTGATATGC) (Gardes and Bruns, 1993). Duplicate PCR reactions were conducted under the conditions outlined in Caporaso et al. (2011). PCR products were cleaned using an AxyPrep Mag PCR clean-up kit (Axygen Biosciences, Union City, CA, United States). Quality and quantity of the cleaned products were assessed using an Agilent DNA 1000 kit (Agilent Technologies, Santa Cara, CA, United States) and Qubit dsDNA HS Assay Kit (Thermofisher Scientific), respectively. Libraries were pooled in an equimolar fashion prior to sequencing, and paired-end, 300 bp sequencing was performed using Illumina MiSeq (v3). Sequences were processed using R software (R Core Team, 2019, version 3.6.1), with the Dada2 package and following the Dada2 ITS-specific pipeline (Callahan et al., 2016). The ITS1 region was extracted using itsxpress for downstream community analysis (Rivers et al., 2018). Unique amplicon sequence variants (ASVs) were identified to the lowest confident taxonomic level using a Naïve Bayesian Classifer from the UNITE ITS database (Wang et al., 2007). Singletons and non-fungal sequences were removed and samples were rarefied to a sequencing depth of 16,803 using the “rrarefy” function in R (“vegan” package; Oksanen et al., 2018). Thereafter, ASV relative abundance was calculated for each sample. EcM ASVs were identified using the fungal functional group assignment software, FUNGuild (Nguyen et al., 2016). The relative abundance of EcM fungi within the sequenced fungal community (referred to as EcM relative abundance hereafter) was calculated as the sum of the relative abundance of EcM ASVs divided by the sum of all relative abundance ASVs. EcM richness was calculated as the number of EcM ASVs per sample. The corresponding ASV dataset (filename “SCxTS_ASV_taxonomy.csv”) prior to rarefication can be accessed at the location specified in “Data Access.”
Phospholipid Fatty Acid Analysis
We estimated the biomass of AM fungi and the ratio of bacterial relative abundance to fungal relative abundance (F:B) from soil samples using phospholipid fatty acid (PLFA) analysis by gas chromatography mass spectroscopy (Microbial ID, Inc., Newark, DE, United States). Thawed subsamples were processed using standard methods (Buyer and Sasser, 2012). Peaks for AM fungi (16:1ω5) and saprophytic fungi (18:2ω6) were identified using the Sherlock System (MIDI, Inc., Newark, DE, United States). Though no method of analyzing fungal biomass and dominance in soil is without limitations (Strickland and Rousk, 2010), we used these metrics to reflect the relative composition of the microbial community along our mycorrhizal gradient.
Statistical Analysis
All statistical analyses were performed using R software (R Core Team, 2019, version 3.6.1). We used linear mixed modeling in lme4 (Bates et al., 2015) to predict C:N and fungal community metric responses to EcM basal area, as well as microbial functional responses (potential enzyme activities and CUE) to C:N and fungal community metrics. We used a PCA to calculate and visualize the importance of community metrics: EcM relative abundance explained the most variation along axis 1, while the F:B ratio explained the most variation along axis 2 (Supplementary Figure S1 and Supplementary Table S4). Thus, we included EcM relative abundance and F:B as predictors in the linear mixed models for microbial functions. Specifically, linear mixed model predictors included the main effects of C:N ratio, EcM relative abundance, and the F:B ratio, and their interactions with soil horizon. We checked predictors for autocorrelation by calculating their correlation coefficients: all coefficients were less than 0.54. Predictor variables were scaled in order to compare effect sizes among estimates. Given that pH was highly correlated with several of our focal predictors in the EcM basal area models, pH was omitted from microbial function models (Supplementary Table S3). While we recognize that pH is an important control on microbial community composition, it was not part of our hypotheses; our intention was to investigate how community composition affects microbial function, which is downstream of the pH effect. We included sample core, because the mineral and organic horizon samples come from the same core at each plot, as a random effect in each model. We performed model selection by sequentially removing interactions and then predictors with the least significant p-value and verifying that the AIC value for the newest model did not increase relative to the previous model (Bolker et al., 2009). We verified model variance homogeneity by plotting model residuals by each of the model predictors at each step of the model selection process, and model normality by plotting model residuals in a histogram. We also analyzed model fit by calculating the marginal and conditional coefficient of variation using the function R. squarredGLMM (Supplementary Table S5; Barton, 2009). We visualized significant responses by plotting model partial residuals for the response variable by the raw predictor values. Plots of the raw response variables by the predictors are available in Supplementary Figures S3–S6.
Results
Substrate Quality and Microbial Community Composition Along the EcM Basal Area Gradient
With increasing EcM basal area, which ranged from 0 to 79.4%, we found that the soil C:N ratio increased in both the organic, ranging from 12.9 to 32.4, and mineral horizon, ranging from 13.9 to 29.4 (p = 0.001; Figure 1A and Supplementary Table S3). However, patterns in fungal community composition were less clear. The F:B ratio increased with EcM basal area, but this pattern was stronger in the organic, ranging from 0.041 to 0.123, relative to mineral horizon, ranging from 0.036 to 0.077 (p < 0.001, p = 0.026; Figure 1B and Supplementary Table S3). We verified that F:B was not driven by changes in just fungal or bacterial relative abundance, and was positively correlated with the relative fungal abundance and negatively correlated with relative bacterial abundance (r = 0.89, p < 0.001; r = −0.83, p < 0.001). We also found that F:B and EcM relative abundance were not significantly correlated (r = 0.28, p = 0.231). EcM relative abundance, which ranged from 5.20 to 85.94 in the organic and from 2.96 to 75.38 in the mineral horizon, increased with EcM basal area in the mineral, but not organic horizon (p = 0.019; Figure 1C and Supplementary Table S3). AM biomass (nmol g–1), which ranged from 24.31 to 40.53 in the organic and from 4.12 to 12.57 in the mineral, decreased in the mineral but not in the organic horizon (p = 0.051; Figure 1D and Supplementary Table S3). AM biomass was included as an estimate of AM abundance because the ITS sequencing approach we employed is biased against AM fungi. Fungal richness was not related to EcM basal area (Supplementary Table S3).
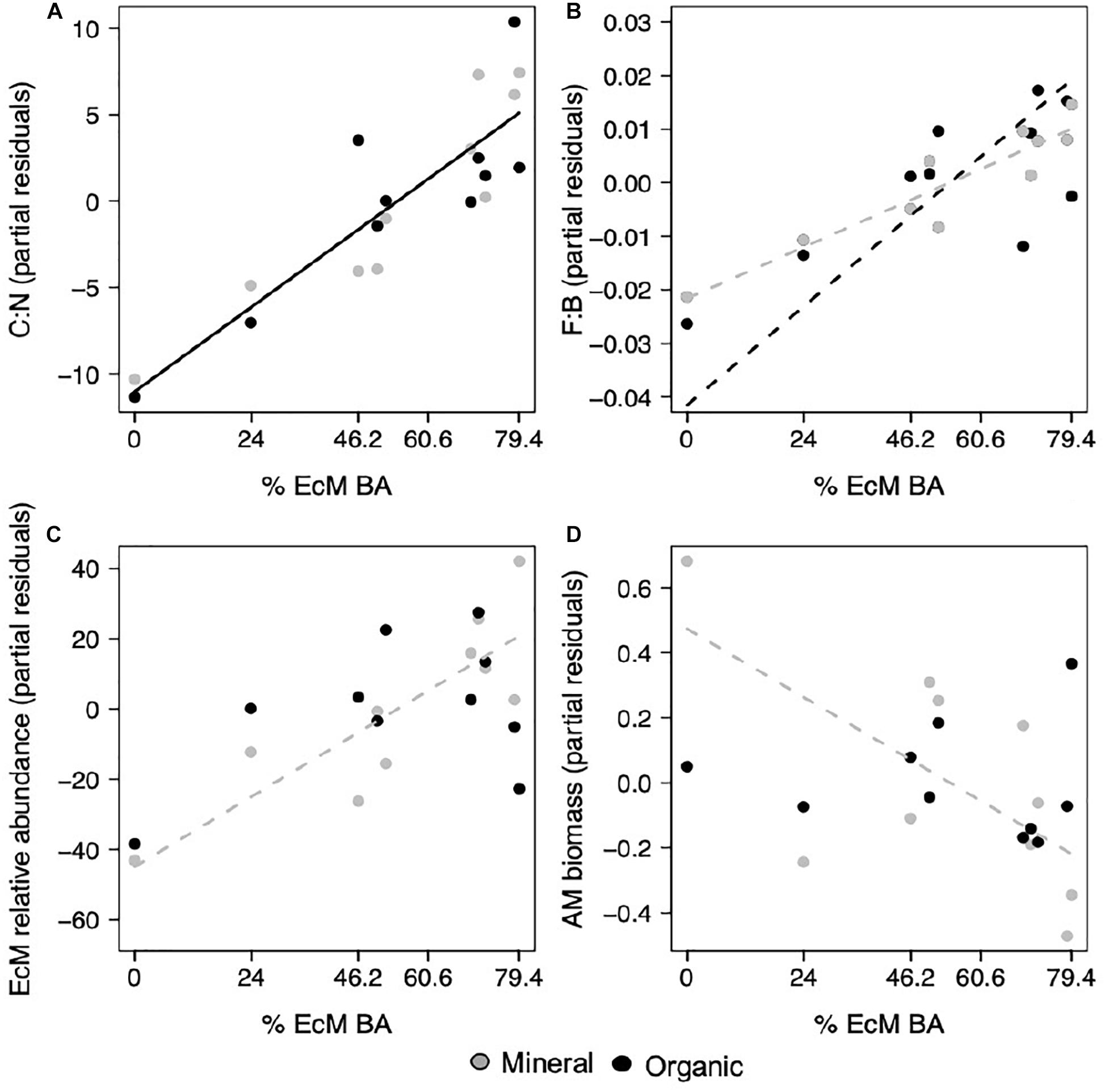
Figure 1. With increasing percent EcM basal area in sample plots, (A) the carbon to nitrogen ratio increased in both horizons, (B) the fungal to bacterial ratio increased with a greater response in the organic relative to mineral horizon, (C) EcM relative abundance increased in the mineral horizon, and (D) AM biomass nmol g–1 decreased in the mineral horizon. Points represent regression partial residuals. Black solid lines represent linear relationships with no horizon interaction, while dashed lines represent individual horizon responses where the mineral horizon is represented by gray and the organic horizon by black lines (p < 0.05).
The Effect of Fungal Community on Microbial Function
Most extracellular enzyme activities were related to either F:B or EcM relative abundance, but not both. Specifically, several enzymes had opposing relationships with F:B. PER, an enzyme class that depolymerizes lignin polymers, decreased significantly with increasing F:B in both horizons (p = 0.007; Figure 2B and Table 1). In contrast, AP + LAP, enzymes that target phosphorus-containing organic compounds and peptide chains, respectively, as well as PO, which oxidizes lignin monomers, increased significantly with increasing F:B in the mineral horizon (p = 0.007, p = 0.019, respectively; Figures 2C,D) and marginally in the organic horizon (p = 0.093, p = 0.092, respectively). Enzymes targeting simple C compounds (BG + CB) and chitin-bound N (NAG) were not affected by the F:B ratio. In contrast EcM relative abundance affected only simple C-degrading enzyme (BG + CB) activities (p = 0.024, respectively; Figure 3B).
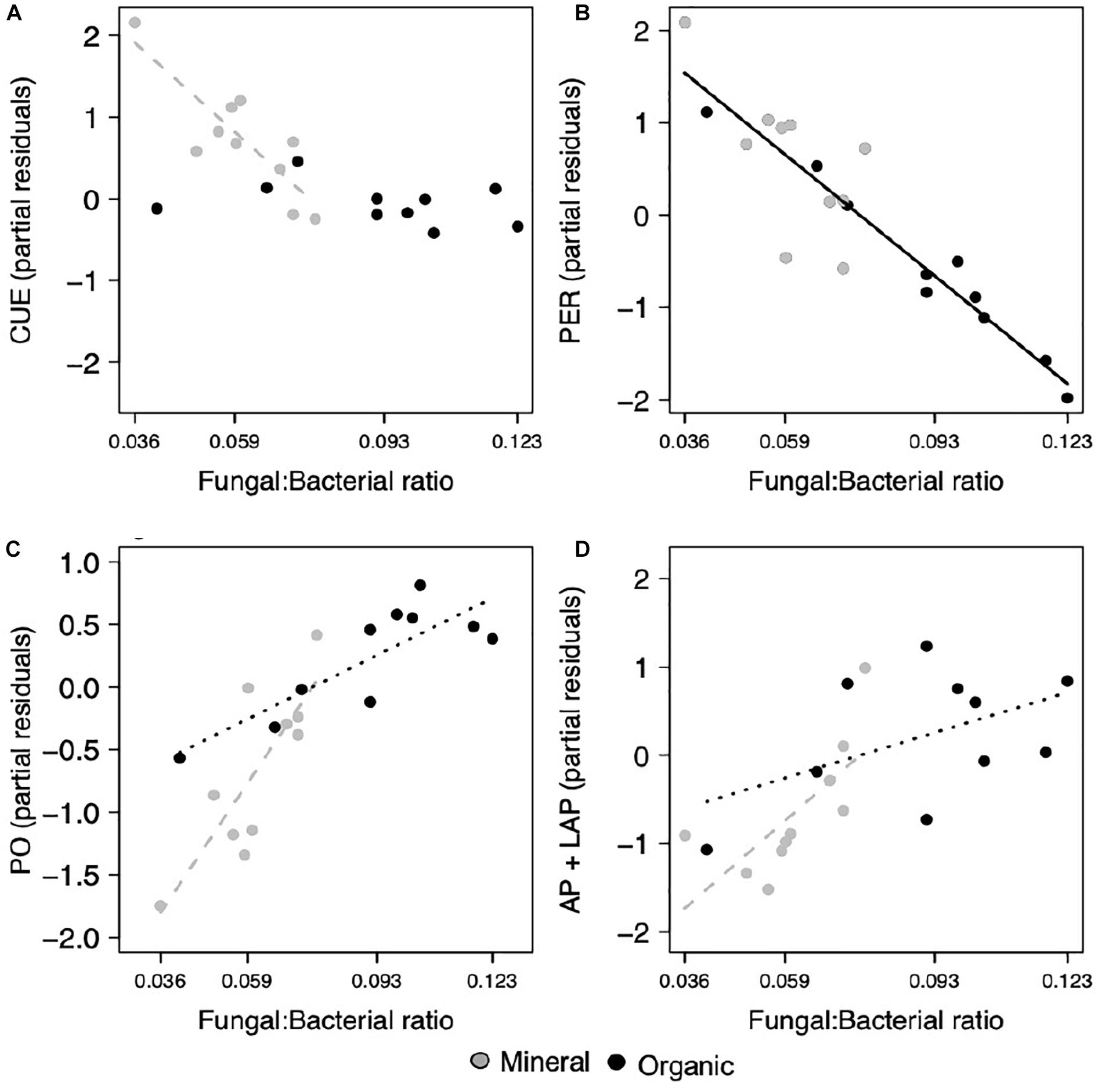
Figure 2. Microbial functions responded to the fungal to bacterial ratio (F:B), where (A) CUE decreased in the mineral horizon, (B) PER activity decreased across both horizons, (C) PO activity and the combined activities of (D) AP and LAP increased in the mineral horizon and with marginal significance, increased in the organic horizon. Points represent the partial residuals of each response variable plotted against F:B ratio. Black solid lines represent linear relationships with no horizon interaction, while dashed lines represent individual horizon responses where the mineral horizon is represented by gray and the organic horizon by black (p < 0.05). Dotted lines represent marginally significant relationships within an individual horizon (p < 0.1).
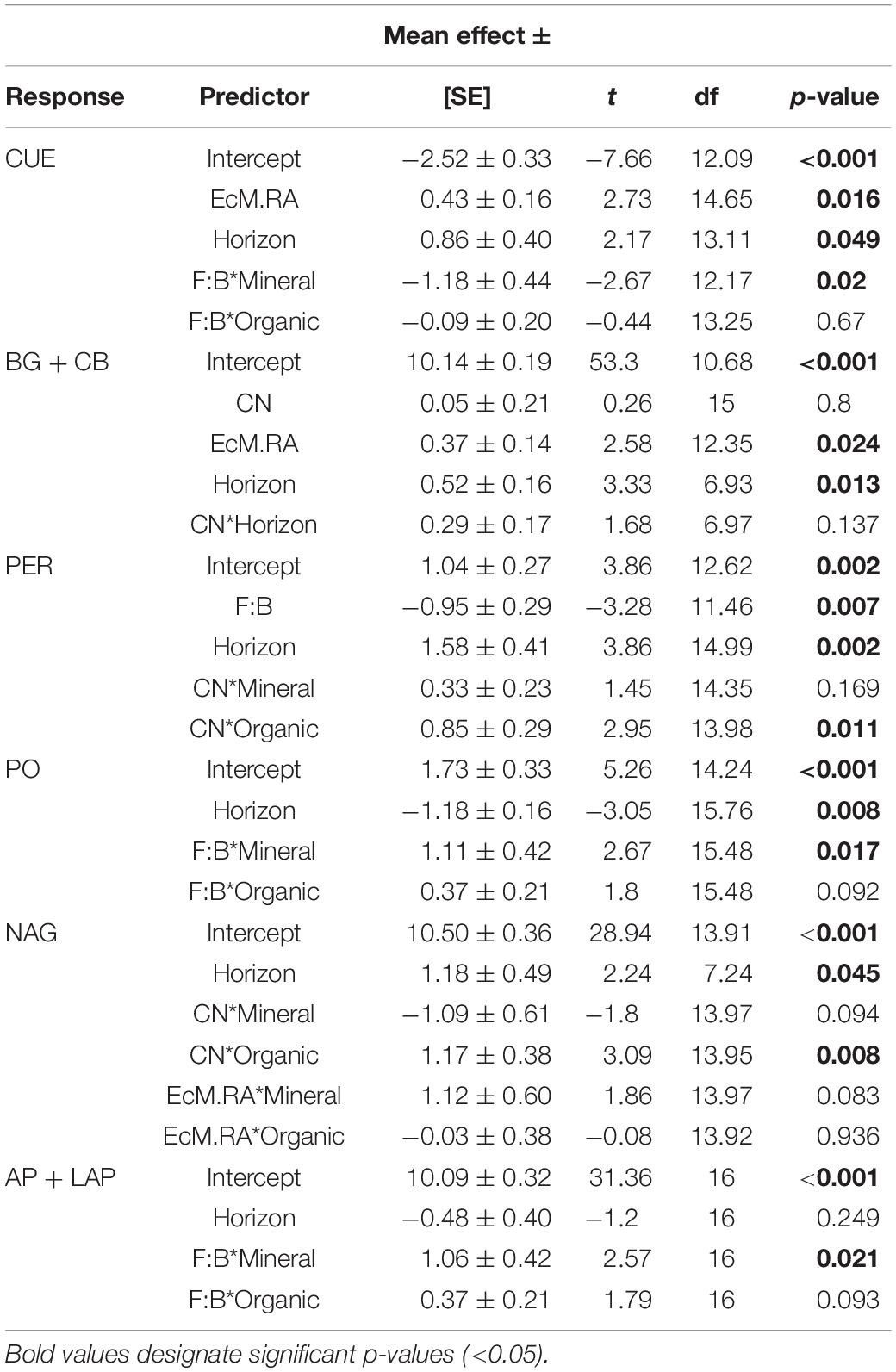
Table 1. Mean effect sizes ± standard error (SE), t-values, degrees of freedom (df), and p-values for microbial function linear mixed effects model outputs.
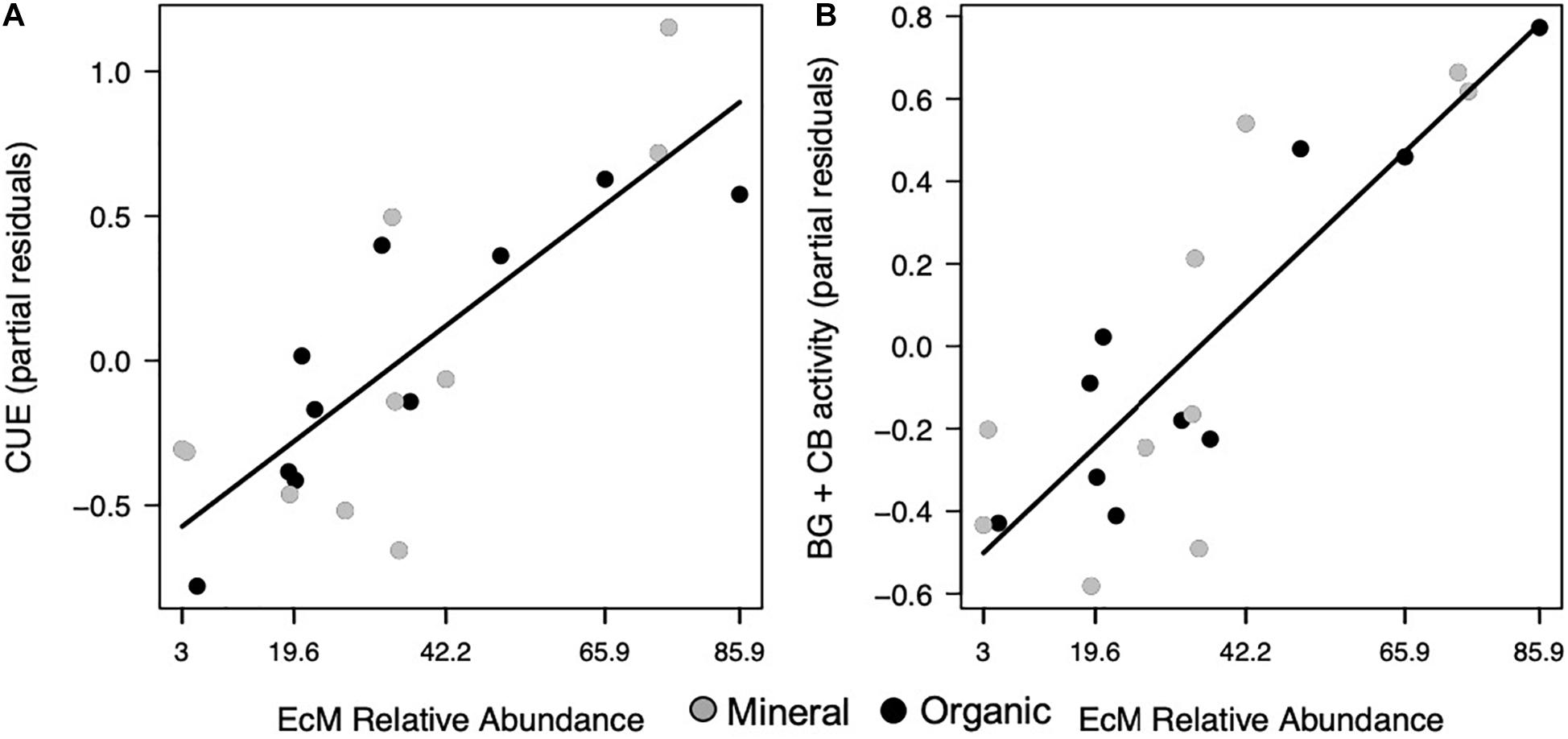
Figure 3. Microbial functions responded to EcM relative abundance, where (A) CUE and (B) combined activities of BG and CB increased in both horizons. Points represent the partial residuals of each response variable plotted against EcM relative abundance. Black solid lines represent linear relationships with no horizon interaction (p < 0.05).
Increases in F:B were associated with lower microbial CUE in the mineral horizon (p = 0.012; Table 1), but did not affect CUE in the organic horizon (Figure 2A). In comparison, CUE increased with EcM relative abundance across both horizons (p = 0.016; Figure 3A and Table 1). The effect size for F:B was 2.8 times the effect size for EcM relative abundance.
The Effect of Substrate Quality on Microbial Function
Microbial functions were less affected by the soil C:N ratio, with significant effects only observed in the organic horizon. Only two enzymes, PER (p = 0.021; Figure 4A) and NAG (p = 0.010; Figure 4B), increased in activity as soil C:N increased. Microbial CUE was not affected by the soil C:N ratio (Table 1).
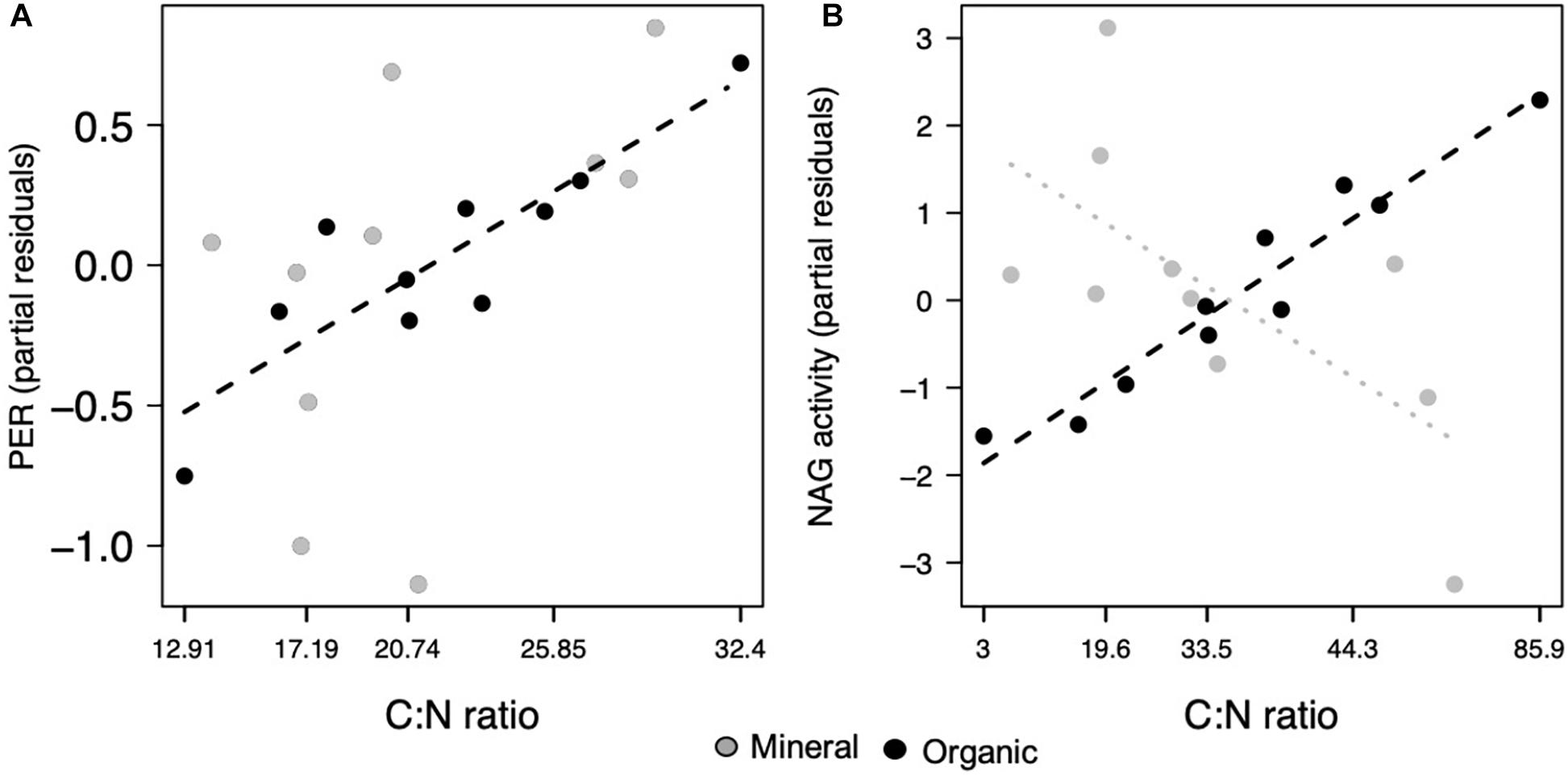
Figure 4. Microbial functions responded to the ratio of carbon to nitrogen (C:N), where peroxidase increased in both horizons and (A) NAG decreased in the mineral and (B) increased in the organic horizon. Points represent the partial residuals of response variables plotted against C:N. Dashed lines represent individual horizon responses where the mineral horizon is represented by gray and the organic horizon, black lines (p < 0.05). Dotted lines represent marginally significant relationships (p < 0.1).
Discussion
We compared the effects of fungal community composition and substrate quality on soil microbial function across a gradient of EcM tree basal area. We demonstrated distinct microbial functional signatures of soils dominated by EcM versus AM trees, and we identified fungal community structure, rather than substrate quality, as the major driver of divergent microbial functions.
Substrate Quality and Microbial Community Composition Change With EcM Basal Area
Our first hypothesis, as to how substrate quality and fungal community composition would change along a gradient of EcM basal tree area was supported in the mineral horizon for AM biomass and EcM relative abundance, and across both horizons for C:N and the F:B ratio. The increase in soil C:N was likely due in part to the relatively lower quality (i.e., higher C:N) leaf litter of the dominant EcM-associated tree species (Phillips et al., 2013; Sun et al., 2018). Concurrently, EcM fungi can actively increase soil C:N ratios by their ability to mine and take up organic N (Averill et al., 2014; Sterkenburg et al., 2018; Zhu et al., 2018).
The increase in EcM relative abundance and corresponding decrease in AM biomass in the mineral horizon confirm that the basal area of trees reflects the abundance of their associated mycorrhizal symbionts. However, this relationship may have been weaker in the organic horizon due to potential vertical stratification of mycorrhizal-associated root tips and foraging extramatrical mycelium (Genney et al., 2006). For instance, studies from Boreal systems have shown vertical separation of EcM and saprotrophic fungi, indicating the importance analyzing both mineral and organic soil horizons in order to understand EcM fungal effects (Rosling et al., 2003; Lindahl et al., 2007).
The F:B ratio increased with EcM basal area across both horizons, suggesting that soil below EcM-associated trees harbors a more fungal-dominated community than soil below AM-associated trees (Cheeke et al., 2017), as the F:B ratio was strongly and positively correlated with fungal relative abundance. Increasing saprotrophic fungal dominance could have been affected by the concurrent decreasing litter quality along the EcM gradient. Fungi have a lower N requirement than bacteria and can use the relatively low-N substrates available in EcM-dominated plots, giving them a competitive advantage despite having lower growth efficiency than bacteria (Fierer et al., 2009; Güsewell and Gessner, 2009; Silva-Sánchez et al., 2019). It is also possible that this increasing F:B signature was affected by elevated production of EcM mycelium and biomass, as the PLFA analysis does not distinguish between EcM and saprotrophic fungi. However, the non-significant correlation between F:B and EcM relative abundance supports our interpretation of the effects of F:B as being driven primarily by saprotrophic fungi.
Effect of Substrate Quality Versus Fungal Community Composition on Microbial Function
Our analysis showed that fungal community composition is a more important driver of microbial function along a gradient of EcM basal area than substrate quality. Although changes in the C:N ratio were associated with increases in two enzymes (NAG and PER), the majority of soil microbial functions exhibited stronger relationships with fungal community metrics. The positive response of NAG to increasing C:N in the organic horizon may reflect the need to liberate N from organic sources, such as chitin in fungal necromass (Gorka et al., 2019; Schnecker et al., 2019), while PER activity likely increased with C:N as the microbial community degraded more complex substrates such as lignin (Rinkes et al., 2013; Lin et al., 2017), which is generally enriched in EcM tree litter relative to that of AM trees (Sun et al., 2018). However, PER activity also responded negatively to an increasing F:B ratio across both soil horizons, with a greater effect size and significance relative to the effect of C:N. Therefore, although PER production is sensitive to local substrate quality, microbial community structure is of greater importance.
Fungal Community Components Differentially Affect EEA
Most extracellular enzyme activities responded to either the F:B ratio or the relative abundance of EcM fungi, but not both, suggesting EcM fungi and saprotrophic fungi control specific groups of enzymes. Specifically, the combined activities of BG + CB increased with EcM relative abundance, but not the F:B ratio, suggesting that this particular suite of enzymes, which degrade plant starch and cellulose, is regulated directly by EcM fungi in these soils rather than both EcM and saprotrophic fungi. This group of enzymes is likely directly produced by EcM fungi, induced by EcM priming, or a combination of both mechanisms (Kotroczó et al., 2014; Kohler et al., 2015). The lack of relationship between EcM relative abundance and the oxidative enzymes PER and PO is not surprising; during evolution from saprotrophic ancestors, most EcM taxa lost the ability to produce oxidative enzymes responsible for lignin degradation (Martin et al., 2016).
Differences in microbial community composition could explain the increase in PO activity and decrease in PER activity with higher F:B. Fungal taxa that are proficient in producing POs (e.g., laccase), but lack the ability to produce PERs were likely present in these soils (Sinsabaugh, 2010; Martin et al., 2016). Moreover, declining PER activity with F:B suggests that PER production at our site may be dominated by bacteria. Recent research has found that bacteria produce a family of lignin-degrading PERs that are structurally unrelated to fungal PERs (de Gonzalo et al., 2016). Thus, as F:B increased, relatively fewer bacteria may have resulted in lower PER activity.
The combined activities of AP + LAP, which target phosphorus-containing organic compounds and peptide chains, respectively, also increased with the F:B ratio, and did not respond to substrate quality. These results indicate that nutrient-targeting enzyme production is not necessarily induced by the nitrogen availability in soil (Waring, 2013). Instead, saprotrophic fungi appear to be important for degrading N and P containing organic compounds (Talbot et al., 2015). Further, EcM-dominated ecosystems are hypothesized to have developed more fungal-dominated free living communities because their relatively greater enzymatic capacities are needed to break down the lower quality leaf litter (Cheeke et al., 2017). Given that both F:B and C:N increased along the EcM basal area gradient, it is possible that these two seemingly different mechanisms actually overlap as fungi are found in, and contribute to, high soil C:N through a relatively higher average biomass C:N ratio than bacteria (Lauber et al., 2008; Zhang and Elser, 2017).
Carbon Use Efficiency
The lack of microbial CUE response to soil C:N is surprising given the theoretical framework and evidence that predicts microbial CUE to decrease with increasing substrate C:N (Manzoni et al., 2012; Cotrufo et al., 2013). Instead, we observed an increase in microbial CUE with EcM relative abundance, indicating that the free-living microbial community grows more efficiently where EcM are more dominant. The free-living microbes in EcM-dominated soils likely rely more on photosynthate C through mycorrhiza-mediated priming rather than the surrounding SOM (Schimel, 2003; Fransson et al., 2016; Frey, 2019). We therefore suggest that the effects of EcM on the free-living community override the importance of soil N availability for microbial CUE in this system.
Given substantial evidence showing lower soil N content and N mineralization rates in EcM-dominated soils (Phillips et al., 2013; Lin et al., 2017; Cotrufo et al., 2019), we expected to find a negative association between EcM relative abundance and free-living microbial CUE, as microbes are predicted to grow less efficiently with increasing ratios of C to nutrient availability (Manzoni et al., 2012). Moreover, EcM have been shown to compete with free-living saprotrophs for N (i.e., the “Gadgil effect”; Averill et al., 2014), which has been associated with declines in free-living community CUE (Anthony et al., 2020). Instead, in this study, we observed a strong positive correlation between EcM relative abundance and free-living microbial CUE. This positive response could have been driven by considerable EcM exudate secretion to the surrounding microbial community (Phillips and Fahey, 2006; Frey, 2019), outweighing the potential negative effects of nutrient competition between the free-living community and EcM (Phillips and Fahey, 2005; Fransson et al., 2016; Soong et al., 2020). This mechanism is further supported by a study which found that EcM exudate production decreased under elevated soil N conditions, followed by a decrease in microbial growth and biomass (Gorka et al., 2019). These results suggest that soils with greater soil N concentrations, as in our AM-dominated plots, may have less root exudation, which would suppress the biomass production of free-living microbes (Soares and Rousk, 2019). Moreover, it is increasingly recognized that the Gadgil effect is only observed under specific environmental conditions (Fernandez and Kennedy, 2016), such as in systems with highly recalcitrant litter types (e.g., pine litter with high lignin:N and high C:N; Smith and Wan, 2019) and where certain EcM taxa characterized by competitive traits are present (Fernandez et al., 2020). Instead, our results suggest that in the temperate forest soils we studied, EcM may predominantly be involved in the facilitation of free-living microbial growth and efficiency.
While microbial CUE responded positively to EcM relative abundance across horizons, it also decreased with the F:B ratio in the mineral horizon, suggesting divergent effects of different members of the fungal community. This lower CUE in soil with a higher F:B ratio supports other findings that as free-living communities shift to higher F:B ratios, the community becomes less efficient (Silva-Sánchez et al., 2019; Soares and Rousk, 2019). Some studies have found the opposite effect, where CUE increased with increasing fungal dominance or depended on substrate availability; however, these laboratory-based studies added individual simple substrates and did not capture how the soil microbial community interacts with complex native substrates (Lipson et al., 2009; Keiblinger et al., 2010). Importantly, the negative effect of F:B ratio on free-living microbial CUE in the mineral horizon was three times stronger than the positive effect of EcM relative abundance. Consequently, though the effect of EcM fungi on the free-living community CUE may be positive via priming, the higher F:B in soil beneath EcM-associated trees may result in lower overall microbial CUE in mineral soils, as was found in a recent global analysis (Anthony et al., 2020).
A relative increase in soil microbial CUE has been hypothesized as a driver of long-term soil C storage and an important piece of information to include in models projecting future C sequestration potential (Kallenbach et al., 2016). The distribution of AM and EcM trees is projected to change across the continental United States in response to a number of factors including climate change and nitrogen deposition, with an increase in AM dominance in the New England area expected (Jo et al., 2019). Our results show that microbial CUE is strongly related to mycorrhizal association, both positively via EcM priming and negatively due to increasing fungal dominance, with a net negative effect on CUE in mineral soil. Therefore, a potential shift toward AM-dominated forests that would reduce soil EcM relative abundance as well as F:B, could cause conceivable net increases in mineral soil CUE. Increased CUE likely leads to a larger proportion of soil C becoming stored in organo-mineral associations (Cotrufo et al., 2013)—an effect that should be strongest in mineral soil (Craig et al., 2018). Therefore, we support continued efforts to use mycorrhizal associations as an effective and relatively simple proxy for predicting soil carbon dynamics at broad scales (Sulman et al., 2019).
The generalizability of these results needs to be tested at other sites and other times of the year. Our EcM basal area gradient was dominated by Pinaceae in the higher EcM-dominant plots. Consequently, the relationships described here may differ from those in forests dominated by other EcM families such as Fagaceae. Because the microbial functional data were analyzed on soils from only one time point, in the early growing season, it is possible that results could differ at other times of year, such as leaf-fall or the spring thaw. The phenology of mycorrhizal fungi function is very much an open question. We are confident, however, in our fungal community analysis. We also extracted DNA and analyzed ITS data from soils at the same plots in 2018, and we found a very similar EcM relative abundance relationship along the EcM basal area gradient.
Conclusion
The trends we observed along our gradient of EcM basal area support the conclusion that fungal community composition is a stronger driver of soil microbial functions than organic matter quality. First, we found that EcM and the saprotrophic free-living community had divergent effects on soil enzyme activities with BG + CB being driven by EcM fungi and AP + LAP and PO being driven by saprotrophic fungi. Second, we found that the decreases in CUE with increasing fungal dominance along the EcM basal area gradient likely outweighed the positive effects of priming by EcM fungi in mineral soil. This latter finding requires further research that disentangles the effects of EcM relative abundance alone versus fungal dominance associated with EcM-associated trees on microbial CUE. Overall, our results indicate that including mycorrhizal plant functional types in models of the soil carbon cycle is warranted and future modeling efforts should focus on integrating the fungal community.
Data Availability Statement
The datasets generated for this study are be found in the ESS_Drive repository: https://data.ess-dive.lbl.gov/view/doi:10.15485/1659374.
Author Contributions
AF led field sample collection and processing, and data analysis and writing. AL and EW contributed to interpreting results and writing. EW also led bioinformatics for the ITS data. KG contributed to CUE methods optimization, and CUE data production and analysis. CH conceived of the experimental design and contributed to data analysis and writing. All authors contributed to the article and approved the submitted version.
Funding
This work was supported as part of the Terrestrial Ecosystem Science Program by the Office of Science, Office of Biological and Environmental Research, of the U.S. Department of Energy under contract DE-SC0020228. In addition, KG and EW were supported by grants to S. D. Frey from the U.S. Department of Energy Genomic Science Program (DE-S0016590) and the U.S. Department of Agriculture National Institute of Food and Agriculture through the New Hampshire Agricultural Experiment Station (Hatch 1003421).
Conflict of Interest
The authors declare that the research was conducted in the absence of any commercial or financial relationships that could be construed as a potential conflict of interest.
Acknowledgments
We thank Owen Krol for help in the field and work on CUE optimization, Paul Zietz for running carbon and nitrogen analyses, Microbial ID for producing PLFA and NLFA data, the UC Davis Stable Isotope Facility for 18O data production, and the University of Wisconsin Biotechnology Center for DNA sequencing.
Supplementary Material
The Supplementary Material for this article can be found online at: https://www.frontiersin.org/articles/10.3389/ffgc.2020.569945/full#supplementary-material
References
Allison, S. (2012a). Fluorimetric and Oxidative Enzyme Assay Protocol. Allison Lab. Available online at: https://allison.bio.uci.edu/protocols/fluorimetricenzymeprotocol.pdf (accessed August 1, 2019).
Allison, S. D. (2012b). A trait-based approach for modelling microbial litter decomposition. Ecol. Lett. 15, 1058–1070. doi: 10.1111/j.1461-0248.2012.01807.x
Anthony, M. A., Crowther, T. W., Maynard, D. S., van den Hoogen, J., and Averill, C. (2020). Distinct assembly processes and microbial communities constrain soil organic carbon formation. One Earth 2, 349–360. doi: 10.1016/j.oneear.2020.03.006
Averill, C., Turner, B. L., and Finzi, A. C. (2014). Mycorrhiza-mediated competition between plants and decomposers drives soil carbon storage. Nature 505, 543–545. doi: 10.1038/nature12901
Barton, K. (2009). Mu-MIn: Multi-model Inference. R Package Version 14317. Available online at: http://R-Forge.R-project.org/projects/mumin/ (accessed August 24, 2020).
Bates, D., Mächler, M., Bolker, B., and Walker, S. (2015). Fitting linear mixed-effects models using lme4. J. Stat. Softw. 67:1406. doi: 10.18637/jss.v067.i01
Bolker, B. M., Brooks, M. E., Clark, C. J., Geange, S. W., Poulsen, J. R., Stevens, M. H. H., et al. (2009). Generalized linear mixed models: a practical guide for ecology and evolution. Trends Ecol. Evol. 24, 127–135. doi: 10.1016/j.tree.2008.10.008
Buyer, J. S., and Sasser, M. (2012). High throughput phospholipid fatty acid analysis of soils. Appl. Soil Ecol. 61, 127–130. doi: 10.1016/j.apsoil.2012.06.005
Callahan, B. J., McMurdie, P. J., Rosen, M. J., Han, A. W., Johnson, A. J. A., and Holmes, S. P. (2016). DADA2: high resolution sample inference from Illumina amplicon data. Nat. Methods 13, 581–583. doi: 10.1038/nmeth.3869
Caporaso, J. G., Lauber, C. L., Walters, W. A., Berg-Lyons, D., Lozupone, C. A., Turnbaugh, P. J., et al. (2011). Global patterns of 16S rRNA diversity at a depth of millions of sequences per sample. Proc. Natl. Acad. Sci. U.S.A. 108, 4516–4522. doi: 10.1073/pnas.1000080107
Cheeke, T. E., Phillips, R. P., Brzostek, E. R., Rosling, A., Bever, J. D., and Fransson, P. (2017). Dominant mycorrhizal association of trees alters carbon and nutrient cycling by selecting for microbial groups with distinct enzyme function. New Phytol. 214, 432–442. doi: 10.1111/nph.14343
Cornelissen, J., Aerts, R., Cerabolini, B., Werger, M., and van der Heijden, M. (2001). Carbon cycling traits of plant species are linked with mycorrhizal strategy. Oecologia 129, 611–619. doi: 10.1007/s004420100752
Cotrufo, M. F., Ranalli, M. G., Haddix, M. L., Six, J., and Lugato, E. (2019). Soil carbon storage informed by particulate and mineral-associated organic matter. Nat. Geosci. 12, 989–994. doi: 10.1038/s41561-019-0484-6
Cotrufo, M. F., Wallenstein, M. D., Boot, C. M., Denef, K., and Paul, E. (2013). The microbial efficiency-matrix stabilization (MEMS) framework integrates plant litter decomposition with soil organic matter stabilization: do labile plant inputs form stable soil organic matter? Glob. Change Biol. 19, 988–995. doi: 10.1111/gcb.12113
Craig, M. E., Turner, B. L., Liang, C., Clay, K., Johnson, D. J., and Phillips, R. P. (2018). Tree mycorrhizal type predicts within-site variability in the storage and distribution of soil organic matter. Glob. Change Biol. 24, 3317–3330. doi: 10.1111/gcb.14132
Cullings, K., and Courty, P.-E. (2009). Saprotrophic capabilities as functional traits to study functional diversity and resilience of ectomycorrhizal community. Oecologia 161, 661–664. doi: 10.1007/s00442-009-1434-6
de Gonzalo, G., Colpa, D. I., Habib, M. H. M., and Fraaije, M. W. (2016). Bacterial enzymes involved in lignin degradation. J. Biotechnol. 236, 110–119. doi: 10.1016/j.jbiotec.2016.08.011
Fernandez, C. W., and Kennedy, P. G. (2016). Revisiting the ‘Gadgil effect’: do interguild fungal interactions control carbon cycling in forest soils? New Phytol. 209, 1382–1394. doi: 10.1111/nph.13648
Fernandez, C. W., See, C. R., and Kennedy, P. G. (2020). Decelerated carbon cycling by ectomycorrhizal fungi is controlled by substrate quality and community composition. New Phytol. 226, 569–582. doi: 10.1111/nph.16269
Emilson, C. E., Kreutzweiser, D. P., Gunn, J. M., and Mykytczuk, N. C. S. (2016). Effects of land use on the structure and function of leaf-litter microbial communities in boreal streams. Freshw. Biol. 61, 1049–1061. doi: 10.1111/fwb.12765
Fierer, N., Strickland, M. S., Liptzin, D., Bradford, M. A., and Cleveland, C. C. (2009). Global patterns in belowground communities. Ecol. Lett. 12, 1238–1249. doi: 10.1111/j.1461-0248.2009.01360.x
Fransson, P., Andersson, A., Norström, S., Bylund, D., and Bent, E. (2016). Ectomycorrhizal exudates and pre-exposure to elevated CO2 affects soil bacterial growth and community structure. Fungal Ecol. 20, 211–224. doi: 10.1016/j.funeco.2016.01.003
Frey, S. D. (2019). Mycorrhizal Fungi as Mediators of Soil Organic Matter Dynamics. Annu. Rev. Ecol. Evol. Syst. 50, 237–259. doi: 10.1146/annurev-ecolsys-110617-062331
Frey, S. D., Lee, J., Melillo, J. M., and Six, J. (2013). The temperature response of soil microbial efficiency and its feedback to climate. Nat. Clim. Change 3, 395–398. doi: 10.1038/nclimate1796
Gardes, M., and Bruns, T. D. (1993). ITS primers with enhanced specificity for basidiomycetes–application to the identification of mycorrhizae and rusts. Mol. Ecol. 2, 113–118. doi: 10.1111/j.1365-294x.1993.tb00005.x
Genney, D. R., Anderson, I. C., and Alexander, I. J. (2006). Fine-scale distribution of pine ectomycorrhizas and their extramatrical mycelium. New Phytol. 170, 381–390. doi: 10.1111/j.1469-8137.2006.01669.x
German, D. P., Weintraub, M. N., Grandy, A. S., Lauber, C. L., Rinkes, Z. L., and Allison, S. D. (2011). Optimization of hydrolytic and oxidative enzyme methods for ecosystem studies. Soil Biol. Biochem. 43, 1387–1397. doi: 10.1016/j.soilbio.2011.03.017
Geyer, K. M., Dijkstra, P., Sinsabaugh, R., and Frey, S. D. (2019). Clarifying the interpretation of carbon use efficiency in soil through methods comparison. Soil Biol. Biochem. 128, 79–88. doi: 10.1016/j.soilbio.2018.09.036
Geyer, K. M., Kyker-Snowman, E., Grandy, A. S., and Frey, S. D. (2016). Microbial carbon use efficiency: accounting for population, community, and ecosystem-scale controls over the fate of metabolized organic matter. Biogeochemistry 127, 173–188. doi: 10.1007/s10533-016-0191-y
Gorka, S., Dietrich, M., Mayerhofer, W., Gabriel, R., Wiesenbauer, J., Martin, V., et al. (2019). Rapid transfer of plant photosynthates to soil bacteria via ectomycorrhizal hyphae and its interaction with nitrogen availability. Front. Microbiol. 10:168. doi: 10.3389/fmicb.2019.00168
Güsewell, S., and Gessner, M. O. (2009). N?: P ratios influence litter decomposition and colonization by fungi and bacteria in microcosms. Funct. Ecol. 23, 211–219. doi: 10.1111/j.1365-2435.2008.01478.x
Hestrin, R., Hammer, E. C., Mueller, C. W., and Lehmann, J. (2019). Synergies between mycorrhizal fungi and soil microbial communities increase plant nitrogen acquisition. Commun. Biol. 2:233. doi: 10.1038/s42003-019-0481-8
Hodge, A., Campbell, C. D., and Fitter, A. H. (2001). An arbuscular mycorrhizal fungus accelerates decomposition and acquires nitrogen directly from organic material. Nature 413, 297–299. doi: 10.1038/35095041
Högberg, M. N., Briones, M. J. I., Keel, S. G., Metcalfe, D. B., Campbell, C., Midwood, A. J., et al. (2010). Quantification of effects of season and nitrogen supply on tree below-ground carbon transfer to ectomycorrhizal fungi and other soil organisms in a boreal pine forest. New Phytol. 187, 485–493. doi: 10.1111/j.1469-8137.2010.03274.x
Högberg, M. N., and Högberg, P. (2002). Extramatrical ectomycorrhizal mycelium contributes one-third of microbial biomass and produces, together with associated roots, half the dissolved organic carbon in a forest soil. New Phytol. 154, 791–795. doi: 10.1046/j.1469-8137.2002.00417.x
Jo, I., Fei, S., Oswalt, C. M., Domke, G. M., and Phillips, R. P. (2019). Shifts in dominant tree mycorrhizal associations in response to anthropogenic impacts. Sci. Adv. 5:eaav6358. doi: 10.1126/sciadv.aav6358
Kaiser, C., Kilburn, M. R., Clode, P. L., Fuchslueger, L., Koranda, M., Cliff, J. B., et al. (2015). Exploring the transfer of recent plant photosynthates to soil microbes: mycorrhizal pathway vs direct root exudation. New Phytol. 205, 1537–1551. doi: 10.1111/nph.13138
Kallenbach, C. M., Frey, S. D., and Grandy, A. S. (2016). Direct evidence for microbial-derived soil organic matter formation and its ecophysiological controls. Nat. Commun. 7, 1–10. doi: 10.1038/ncomms13630
Keiblinger, K. M., Hall, E. K., Wanek, W., Szukics, U., Hämmerle, I., Ellersdorfer, G., et al. (2010). The effect of resource quantity and resource stoichiometry on microbial carbon-use-efficiency. FEMS Microbiol. Ecol. 73, 430–440. doi: 10.1111/j.1574-6941.2010.00912.x
Koch, O., Tscherko, D., and Kandeler, E. (2007). Temperature sensitivity of microbial respiration, nitrogen mineralization, and potential soil enzyme activities in organic alpine soils. Glob. Biogeochem. Cycles 21:GB4017. doi: 10.1029/2007GB002983
Kohler, A., Kuo, A., Nagy, L. G., Morin, E., Barry, K. W., Buscot, F., et al. (2015). Convergent losses of decay mechanisms and rapid turnover of symbiosis genes in mycorrhizal mutualists. Nat. Genet. 47, 410–415. doi: 10.1038/ng.3223
Kotroczó, Z., Veres, Z., Fekete, I., Krakomperger, Z., Tóth, J. A., Lajtha, K., et al. (2014). Soil enzyme activity in response to long-term organic matter manipulation. Soil Biol. Biochem. 70, 237–243. doi: 10.1016/j.soilbio.2013.12.028
Lang, A. K., Jevon, F. V., Ayres, M. P., and Hatala Matthes, J. (2019). Higher soil respiration rate beneath arbuscular mycorrhizal trees in a northern hardwood forest is driven by associated soil properties. Ecosystems 23, 1243–1253. doi: 10.1007/s10021-019-00466-7
Lashermes, G., Gainvors-Claisse, A., Recous, S., and Bertrand, I. (2016). Enzymatic strategies and carbon use efficiency of a litter-decomposing fungus grown on maize leaves, stems, and roots. Front. Microbiol. 7:1315. doi: 10.3389/fmicb.2016.01315
Lauber, C. L., Strickland, M. S., Bradford, M. A., and Fierer, N. (2008). The influence of soil properties on the structure of bacterial and fungal communities across land-use types. Soil Biol. Biochem. 40, 2407–2415. doi: 10.1016/j.soilbio.2008.05.021
Lin, G., McCormack, M. L., Ma, C., and Guo, D. (2017). Similar below-ground carbon cycling dynamics but contrasting modes of nitrogen cycling between arbuscular mycorrhizal and ectomycorrhizal forests. New Phytol. 213, 1440–1451. doi: 10.1111/nph.14206
Lindahl, B. D., Ihrmark, K., Boberg, J., Trumbore, S. E., Högberg, P., Stenlid, J., et al. (2007). Spatial separation of litter decomposition and mycorrhizal nitrogen uptake in a boreal forest. New Phytol. 173, 611–620. doi: 10.1111/j.1469-8137.2006.01936.x
Lindahl, B. D., and Tunlid, A. (2015). Ectomycorrhizal fungi – potential organic matter decomposers, yet not saprotrophs. New Phytol. 205, 1443–1447. doi: 10.1111/nph.13201
Lipson, D. A., Monson, R. K., Schmidt, S. K., and Weintraub, M. N. (2009). The trade-off between growth rate and yield in microbial communities and the consequences for under-snow soil respiration in a high elevation coniferous forest. Biogeochemistry 95, 23–35. doi: 10.1007/s10533-008-9252-1
Lu, M., and Hedin, L. O. (2019). Global plant–symbiont organization and emergence of biogeochemical cycles resolved by evolution-based trait modelling. Nat. Ecol. Evol. 3, 239–250. doi: 10.1038/s41559-018-0759-0
Manzoni, S., Taylor, P., Richter, A., Porporato, A., and Ågren, G. I. (2012). Environmental and stoichiometric controls on microbial carbon-use efficiency in soils: research review. New Phytol. 196, 79–91. doi: 10.1111/j.1469-8137.2012.04225.x
Martin, F., Kohler, A., Murat, C., Veneault-Fourrey, C., and Hibbett, D. S. (2016). Unearthing the roots of ectomycorrhizal symbioses. Nat. Rev. Microbiol. 14, 760–773. doi: 10.1038/nrmicro.2016.149
Nguyen, N. H., Song, Z., Bates, S. T., Branco, S., Tedersoo, L., Menke, J., et al. (2016). FUNGuild: an open annotation tool for parsing fungal community datasets by ecological guild. Fungal Ecol. 20, 241–248. doi: 10.1016/j.funeco.2015.06.006
Ouimette, A. P., Ollinger, S. V., Lepine, L. C., Stephens, R. B., Rowe, R. J., Vadeboncoeur, M. A., et al. (2019). Accounting for carbon flux to mycorrhizal fungi may resolve discrepancies in forest carbon budgets. Ecosystems 23, 715–729. doi: 10.1007/s10021-019-00440-3
Oksanen, J., Guillaume Blanchet, F., Kindt, R., Legendre, P., Minchin, P., O’hara, R., et al. (2018). Vegan: Community Ecology Package. Httpscranr-Proj.
Phillips, R. P., Brzostek, E., and Midgley, M. G. (2013). The mycorrhizal-associated nutrient economy: a new framework for predicting carbon-nutrient couplings in temperate forests. New Phytol. 199, 41–51. doi: 10.1111/nph.12221
Phillips, R. P., and Fahey, T. J. (2005). Patterns of rhizosphere carbon flux in sugar maple (Acer saccharum) and yellow birch (Betula allegheniensis) saplings. Glob. Change Biol. 11, 983–995. doi: 10.1111/j.1365-2486.2005.00959.x
Phillips, R. P., and Fahey, T. J. (2006). Tree species and mycorrhizal associations influence the magnitude of rhizosphere effects. Ecology 87, 1302–1313. doi: 10.1890/0012-9658(2006)87[1302:tsamai]2.0.co;2
R Core Team (2019). R: A Language and Environment for Statistical Computing. Vienna: R Foundation for Statistical Computing. Available online at: https://www.R-project.org/
Rinkes, Z. L., Sinsabaugh, R. L., Moorhead, D. L., Grandy, A. S., and Weintraub, M. N. (2013). Field and lab conditions alter microbial enzyme and biomass dynamics driving decomposition of the same leaf litter. Front. Microbiol. 4:260. doi: 10.3389/fmicb.2013.00260
Rivers, A. R., Weber, K. C., Gardner, T. G., Liu, S., and Armstrong, S. D. (2018). ITSxpress: software to rapidly trim internally transcribed spacer sequences with quality scores for marker gene analysis. F1000Research 7:1418. doi: 10.12688/f1000research.15704.1
Rosling, A., Landeweert, R., Lindahl, B. D., Larsson, K.-H., Kuyper, T. W., Taylor, A. F. S., et al. (2003). Vertical distribution of ectomycorrhizal fungal taxa in a podzol soil profile. New Phytol. 159, 775–783. doi: 10.1046/j.1469-8137.2003.00829.x
Saiya-Corka, K. R., Sinsabaugha, R. L., and Zakb, D. R. (2002). The effects of long term nitrogen deposition on extracellular enzyme activity in an Acer saccharum forest soil. Soil Biol. Biochemistry 34, 1309–1315.
Schimel, J. (2003). The implications of exoenzyme activity on microbial carbon and nitrogen limitation in soil: a theoretical model. Soil Biol. Biochem. 35, 549–563. doi: 10.1016/S0038-0717(03)00015-4
Schnecker, J., Bowles, T., Hobbie, E. A., Smith, R. G., and Grandy, A. S. (2019). Substrate quality and concentration control decomposition and microbial strategies in a model soil system. Biogeochemistry 144, 47–59. doi: 10.1007/s10533-019-00571-8
Silva-Sánchez, A., Soares, M., and Rousk, J. (2019). Testing the dependence of microbial growth and carbon use efficiency on nitrogen availability, pH, and organic matter quality. Soil Biol. Biochem. 134, 25–35. doi: 10.1016/j.soilbio.2019.03.008
Simard, S. W., Jones, M. D., and Durall, D. M. (2003). “Carbon and Nutrient Fluxes Within and Between Mycorrhizal Plants,” in Mycorrhizal Ecology, eds M. G. A. van der Heijden and I. R. Sanders (Berlin: Springer), 33–74. doi: 10.1007/978-3-540-38364-2_2
Sinsabaugh, R. L. (2010). Phenol oxidase, peroxidase and organic matter dynamics of soil. Soil Biol. Biochem. 42, 391–404. doi: 10.1016/j.soilbio.2009.10.014
Sinsabaugh, R. L., Carreiro, M. M., and Repert, D. A. (2002). Allocation of extracellular enzymatic activity in relation to litter composition, N deposition, and mass loss. Biogeochemistry 60, 1–24. doi: 10.1023/A:1016541114786
Sinsabaugh, R. L., Gallo, M. E., Lauber, C., Waldrop, M. P., and Zak, D. R. (2005). Extracellular enzyme activities and soil organic matter dynamics for northern hardwood forests receiving simulated nitrogen deposition. Biogeochemistry 75, 201–215. doi: 10.1007/s10533-004-7112-1
Sinsabaugh, R. L., Manzoni, S., Moorhead, D. L., and Richter, A. (2013). Carbon use efficiency of microbial communities: stoichiometry, methodology and modelling. Ecol. Lett. 16, 930–939. doi: 10.1111/ele.12113
Smith, G. R., and Wan, J. (2019). Resource-ratio theory predicts mycorrhizal control of litter decomposition. New Phytol. 223, 1595–1606. doi: 10.1111/nph.15884
Soares, M., and Rousk, J. (2019). Microbial growth and carbon use efficiency in soil: links to fungal-bacterial dominance, SOC-quality and stoichiometry. Soil Biol. Biochem. 131, 195–205. doi: 10.1016/j.soilbio.2019.01.010
Soong, J. L., Fuchslueger, L., Marañon-Jimenez, S., Torn, M. S., Janssens, I. A., Penuelas, J., et al. (2020). Microbial carbon limitation: the need for integrating microorganisms into our understanding of ecosystem carbon cycling. Glob. Change Biol. 26, 1953–1961. doi: 10.1111/gcb.14962
Spohn, M., Klaus, K., Wanek, W., and Richter, A. (2016). Microbial carbon use efficiency and biomass turnover times depending on soil depth – Implications for carbon cycling. Soil Biol. Biochem. 96, 74–81. doi: 10.1016/j.soilbio.2016.01.016
Sterkenburg, E., Clemmensen, K. E., Ekblad, A., Finlay, R. D., and Lindahl, B. D. (2018). Contrasting effects of ectomycorrhizal fungi on early and late stage decomposition in a boreal forest. ISME J. 12, 2187–2197. doi: 10.1038/s41396-018-0181-2
Strickland, M. S., and Rousk, J. (2010). Considering fungal:bacterial dominance in soils – Methods, controls, and ecosystem implications. Soil Biol. Biochem. 42, 1385–1395. doi: 10.1016/j.soilbio.2010.05.007
Sulman, B. N., Shevliakova, E., Brzostek, E. R., Kivlin, S. N., Malyshev, S., Menge, D. N. L., et al. (2019). Diverse mycorrhizal associations enhance terrestrial C storage in a global model. Glob. Biogeochem. Cycles 33, 501–523. doi: 10.1029/2018GB005973
Sun, T., Hobbie, S. E., Berg, B., Zhang, H., Wang, Q., Wang, Z., et al. (2018). Contrasting dynamics and trait controls in first-order root compared with leaf litter decomposition. Proc. Natl. Acad. Sci. U.S.A. 115, 10392–10397. doi: 10.1073/pnas.1716595115
Talbot, J. M., Allison, S. D., and Treseder, K. K. (2008). Decomposers in disguise: mycorrhizal fungi as regulators of soil C dynamics in ecosystems under global change. Funct. Ecol. 22, 955–963. doi: 10.1111/j.1365-2435.2008.01402.x
Talbot, J. M., Martin, F., Kohler, A., Henrissat, B., and Peay, K. G. (2015). Functional guild classification predicts the enzymatic role of fungi in litter and soil biogeochemistry. Soil Biol. Biochem. 88, 441–456. doi: 10.1016/j.soilbio.2015.05.006
Vance, E. D., Brookes, P. C., and Jenkinson, D. S. (1987). An extraction method for measuring soil microbial biomass C. Soil Biol. Biochem. 19, 703–707. doi: 10.1016/0038-0717(87)90052-6
Verbruggen, E., Pena, R., Fernandez, C. W., and Soong, J. L. (2017). Mycorrhizal Interactions With Saprotrophs and Impact on Soil Carbon Storage in Mycorrhizal Mediation of Soil. Amsterdam: Elsevier, 441–460. doi: 10.1016/B978-0-12-804312-7.00024-3
Wang, Q., Garrity, G. M., Tiedje, J. M., and Cole, J. R. (2007). Naive Bayesian classifier for rapid assignment of rRNA sequences into the new bacterial taxonomy. Appl. Environ. Microbiol. 73, 5261–5267. doi: 10.1128/AEM.00062-07
Waring, B. G. (2013). Exploring relationships between enzyme activities and leaf litter decomposition in a wet tropical forest. Soil Biol. Biochem. 64, 89–95. doi: 10.1016/j.soilbio.2013.04.010
Zak, D. R., Pellitier, P. T., Argiroff, W., Castillo, B., James, T. Y., Nave, L. E., et al. (2019). Exploring the role of ectomycorrhizal fungi in soil carbon dynamics. New Phytol. 223, 33–39. doi: 10.1111/nph.15679
Zhang, J., and Elser, J. J. (2017). Carbon:nitrogen:phosphorus stoichiometry in fungi: a Meta-analysis. Front. Microbiol. 8:1281. doi: 10.3389/fmicb.2017.01281
Keywords: mycorrhizal fungi, forest soil, carbon use efficiency, enzymes, fungal:bacterial, carbon:nitrogen
Citation: Fitch AA, Lang AK, Whalen ED, Geyer K and Hicks Pries C (2020) Fungal Community, Not Substrate Quality, Drives Soil Microbial Function in Northeastern U.S. Temperate Forests. Front. For. Glob. Change 3:569945. doi: 10.3389/ffgc.2020.569945
Received: 05 June 2020; Accepted: 22 September 2020;
Published: 22 October 2020.
Edited by:
Stephanie N. Kivlin, The University of Tennessee, Knoxville, United StatesReviewed by:
Edward Robert Brzostek, West Virginia University, United StatesAntra Boca, Latvia University of Agriculture, Latvia
Copyright © 2020 Fitch, Lang, Whalen, Geyer and Hicks Pries. This is an open-access article distributed under the terms of the Creative Commons Attribution License (CC BY). The use, distribution or reproduction in other forums is permitted, provided the original author(s) and the copyright owner(s) are credited and that the original publication in this journal is cited, in accordance with accepted academic practice. No use, distribution or reproduction is permitted which does not comply with these terms.
*Correspondence: Amelia A. Fitch, YW1lbGlhLmEuZml0Y2guZ3JAZGFydG1vdXRoLmVkdQ==