- 1Department of Ecology and Evolutionary Biology, University of Arizona, Tucson, AZ, United States
- 2Department of Biology, University of Miami, Miami, FL, United States
- 3Department of Biogeochemical Processes, Max Planck Institute for Biogeochemistry, Jena, Germany
- 4Embrapa Amazônia Oriental, Santarém, Brazil
Both plant physiology and atmospheric chemistry are substantially altered by the emission of volatile isoprenoids (VI), such as isoprene and monoterpenes, from plant leaves. Yet, since gaining scientific attention in the 1950’s, empirical research on leaf VI has been largely confined to laboratory experiments and atmospheric observations. Here, we introduce a new field instrument designed to bridge the scales from leaf to atmosphere, by enabling precision VI detection in real time from plants in their natural ecological setting. With a field campaign in the Brazilian Amazon, we reveal an unexpected distribution of leaf emission capacities (EC) across the vertical axis of the forest canopy, with EC peaking in the mid-canopy instead of the sun-exposed canopy surface, and moderately high emissions occurring in understory specialist species. Compared to the simple interpretation that VI protect leaves from heat stress at the hot canopy surface, our results encourage a more nuanced view of the adaptive role of VI in plants. We infer that forest emissions to the atmosphere depend on the dynamic microenvironments imposed by canopy structure, and not simply on canopy surface conditions. We provide a new emissions inventory from 52 tropical tree species, revealing moderate consistency in EC within taxonomic groups. We highlight priorities in leaf volatiles research that require field-portable detection systems. Our self-contained, portable instrument provides real-time detection and live measurement feedback with precision and detection limits better than 0.5 nmolVI m–2leaf s–1. We call the instrument ‘PORCO’ based on the gas detection method: photoionization of organic compounds. We provide a thorough validation of PORCO and demonstrate its capacity to detect ecologically driven variation in leaf emission rates and thus accelerate a nascent field of science: the ecology and ecophysiology of plant volatiles.
Introduction
Forest-atmosphere interactions are shaped not only by the exchange of carbon, water, and energy, but also by the biological production of organic trace gasses (Laothawornkitkul et al., 2009; Unger, 2014; Rieksta et al., 2020; Tani and Mochizuki, 2021). For example, volatile isoprenoids (VI)—including 5C isoprene and a diversity of 10C monoterpenes—protect plants from diverse abiotic and biotic stresses (Fineschi et al., 2013), and contribute to the formation of atmospheric aerosols (Heald et al., 2008). These ‘secondary organic aerosols’ formed through atmospheric chemistry with organic gasses—most conspicuous in the blue haze that forms over maritime forests in hot conditions—affect the radiative forcing of the climate (Carslaw et al., 2013) and are the source of up to half of all cloud condensation nuclei (Carslaw et al., 2010).
Understanding which plant species emit VI and how they are distributed across landscapes is critical to determining regional and global emissions (Klinger et al., 1998; Harley et al., 2004; Rinnan et al., 2020; Seco et al., 2020; Purser et al., 2021). The most abundant organic gas emitted from the biosphere, isoprene, is produced by only approximately one-fifth of terrestrial plant species (Fini et al., 2017), including one-third or more of tropical trees (Taylor et al., 2018). Monoterpene emissions are less abundant but more reactive than isoprene (Heald et al., 2008), and their distribution among species—conspicuous from conifers (pine scent) but cryptic from broadleaf trees—is poorly known (Guenther, 2013; Feng et al., 2019; Jardine et al., 2020a). Models of VI emissions rely on inventories of species measurements from the field to estimate regional fractions of emitting vegetation (Guenther, 2013). This presents a challenge for modeling areas that are under-sampled due to remoteness or high species diversity.
Tropical forests are estimated to emit more VI to the atmosphere, globally, than temperate and boreal forests combined (Guenther, 2013; Hantson et al., 2017; Yáñez-serrano et al., 2020). Variation in species compositions among sites produces substantial variation in emitter fractions, which has been linked to variation in measured forest emissions (Klinger et al., 1998; Harley et al., 2004). Given that only one percent of the roughly fifty-thousand species of trees in tropical forests (Slik et al., 2015) have been sampled (Taylor et al., 2018), accurately representing emitter fractions seems a daunting task. The task may be simplified by seeking ecological mechanisms that distribute VI emitting trees across tropical landscapes. For example, a recent study estimated that the proportion of isoprene emitting trees in tropical forests increases twofold from cool, dry sites to warm, wet sites, representing a mechanism for variation in forest emission capacity (Taylor et al., 2018). This pattern may arise due to better photosynthetic performance at high temperatures among isoprene emitting species compared to non-emitting species (Taylor et al., 2019), consistent with the long-held hypothesis that isoprene enhances plant thermal tolerance (Singsaas et al., 1997; Sharkey and Yeh, 2001; Behnke et al., 2007; Sharkey and Monson, 2017). While a promising start, the ecological analyses by Taylor et al. (2018, 2019) relied on published species emissions inventories, most of which were carried out without the aim of ecological hypothesis testing. To both narrow uncertainty in estimates of forest emitter fractions (Taylor et al., 2018), and understand how VI may shape future diversity and function in tropical forests, many more species measurements are needed in contexts that inform the ecological and evolutionary structure of emissions (Monson et al., 2013; Sharkey and Monson, 2014).
Despite intensive scientific interest beginning in the 1950’s (Rasmussen and Went, 1964; Sanadze, 2004), research on VI emissions has remained largely confined to laboratory and greenhouse experiments on plants (Sharkey and Monson, 2017), and atmospheric observations and modeling (Sharkey and Monson, 2014). The main impediment to the incorporation of VI into ecological research has arguably been a lack of instrumentation optimized for emission measurements in field settings (Tholl et al., 2006). There are currently two general approaches to field sampling of leaf VI emissions. The first is an ‘offline’ approach, in which gas samples are collected in the field, and later analyzed in the lab. Typically, leaves are placed in flow-through chambers such as commercial photosynthesis cuvettes or less quantitative enclosures, and gas samples are collected from the cuvette exhaust in bags or adsorption cartridges for later analysis by mass spectrometry (Tholl et al., 2006; Niinemets et al., 2010a; Alves et al., 2014; Jardine et al., 2020b; Rieksta et al., 2020). The advantages of this method include control of the leaf environment using a sophisticated leaf cuvette, precision quantification of emissions, and the ability to distinguish VI species. The disadvantages include limited sample sizes due to the high cost of adsorption cartridges, the requirement for offsite analysis, a lack of live measurement feedback, and the potential chemical degradation of stored samples during transport. The second common method employs portable, ‘online’ detection instruments such as handheld photoionization detectors (PIDs), which quantify organic gas concentrations in real time, but lack the ability to distinguish gas species. A portable online detector provides significant advantages in remote areas, affording unlimited sample sizes and live measurement feedback (Klinger et al., 1998, 2002; Harley et al., 2004). However, low detection precision necessitates high sample concentrations, achieved by high-volume cuvettes that lack environmental control, often enclosing whole branches. Results are typically treated as qualitative, distinguishing only strong emitters from non-emitters.
Here, we present a prototype instrument for precision online detection of light-dependent (photosynthetically driven) leaf VI emissions in the field, with a lower detection limit (LDL) equal to 0.4 nmolVI m–2leaf s–1. We field-validate the instrument with a measurement campaign in the Brazilian Amazon, from which we produce an emissions inventory of 52 tropical tree species, and estimate the vertical distribution of emission capacities within the forest canopy. We call the instrument “PORCO,” after the detection method—photoionization of organic compounds. Custom, light-controlled leaf cuvettes are combined with optimized photoionization detection for high measurement precision and repeatability. Our measurements represent the dominant VI—predominantly isoprene, and smaller amounts of monoterpenes—as these are typically the only ionizable, light-dependent compounds emitted by tree leaves at rates that exceed our detection limit. The components and methods are adaptable to a range of real-time gas-sampling objectives where required mixing-ratio detection limits are around 5 ppb or greater (e.g., growth-chamber air, headspace analysis), and to systems requiring microenvironmental control (e.g., moss growth chambers). The adaptations unique to PORCO enable greater sample sizes, lower LDLs, real-time data, and better distinction between leaves and species for more nuanced ecological hypothesis testing than was previously possible in a field portable system.
Materials and Equipment
Overview of the ‘PORCO’ System
PORCO measures emissions from intact leaves attached to branches (Figure 1) in a manner similar to field measurements for leaf photosynthesis (Hunt, 2003). Leaves are enclosed in custom acrylic cuvettes with custom light-emitting-diode (LED) panels providing photosynthetically active radiation (PAR: 90% red, 660 nm; 10% blue, 460 nm) to the leaf to drive photosynthesis and organic gas emissions. Air is purified and pumped to the cuvette inlet at a controlled rate. Cuvette air is subsampled from an outlet while excess flow is exhausted at a loose seal around the leaf petiole. Sample air is drawn through tubing embedded in ice to remove water vapor, and into a commercial photoionization detector (PID). The PID quantifies hydrocarbon gas concentrations, logs its readings at 1 Hz, and displays live numerical data as it is logged. Emission rates from the leaf are calculated as non-steady-state fluxes, with a LDL of approximately 0.4 nmol m–2 s–1. The entire system is battery powered and mounted on an external-frame backpack for field portability. For a list of major system components and sources, see Supplementary Table 1.
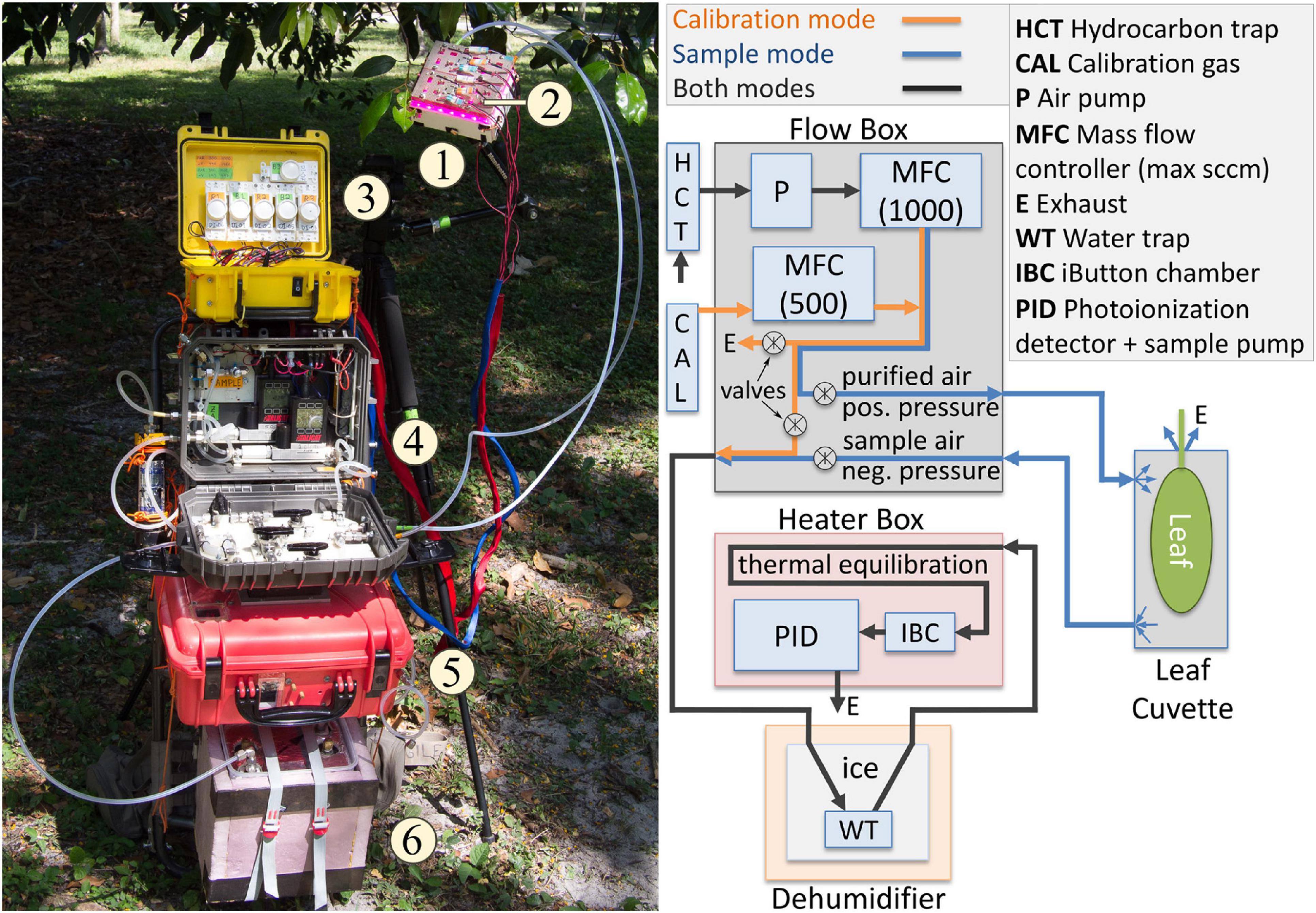
Figure 1. Overview of PORCO components and flow paths. (1) Several acrylic leaf cuvettes accommodate different leaf shapes and sizes. (2) Light emitting diode (LED) panels produce photosynthetically active light. (3) Photon flux density at the leaf is regulated by electrical current control to the light panels. (4) A Flow Box regulates air flow rates and pathways for calibration and sampling via a pump, mass-flow controllers, valve manifold, hydrocarbon trap, and calibration gas. The hydrocarbon trap purifies ambient air for use in calibration and flow-through sampling. (5) Enclosed in a temperature-controlled case, a photoionization detector (PID) with an internal air pump draws sample air from the cuvette. (6) Humidity of calibration air or sample air returning from the leaf cuvette is kept constant by conditioning through chilled tubing in ice and water. The heater, Flow Box, and light control system are each powered by independent lithium ion batteries. The PID contains its own lithium ion battery that powers its internal pump, computer, and sensor components. The PID displays live measurement data, viewable through the enclosure window, in the form of an uncalibrated numerical reading that varies at 1 Hz as it is recorded to the datalog. Data post-processing is performed by custom software in R.
Methods
Detecting Leaf Volatiles by Photoionization
PORCO employs a commercial, handheld PID with sensitivity to hydrocarbon gasses in parts-per-billion (ppb), the ppbRAE-3000 (Honeywell International Inc., Charlotte, NC, United States). The PID method employs an ultraviolet (UV) lamp to ionize hydrocarbons in the sample gas stream (Rae Systems Inc, 2013a). The separated electrons and positive ions migrate to electrodes in the sensor housing, inducing an electrical current. Gas concentrations are linearly proportional to the induced current.
Photoionization detectors have previously been used for qualitative measurements of leaf hydrocarbon emissions in remote field settings, where portability and online detection are significant advantages (Klinger et al., 1998, 2002; Harley et al., 2004). The use of PIDs for quantitative emission measurements has been impeded by several factors. Until recently, portable commercial PIDs were limited to parts-per-million (ppm) sensitivity (Rae Systems Inc, 2013a). Measurements suffer from high signal variability (measurement error), often referred to as ‘PID drift,’ even when measuring a constant calibration gas (Rae Systems Inc, 2013b). Environmentally controlled cuvettes in photosynthesis machines insufficiently amplify emission signals for detection by PIDs, especially if sources of measurement error are not controlled. The following sections describe the adaptations unique to PORCO that overcome previous limitations to PID use, enabling quantitative leaf emission measurements with high precision and low detection limits in real-time under field conditions.
Isolation of Isoprene and Monoterpenes in the PID Signal
The PID is a generalist sensor with variable responsivity to a diversity of ionizable compounds, and which reports a single value representing the integrated measurement (i.e. the sensor is ‘non-distinguishing’). While plant leaves can emit a high diversity of compounds in a light-dependent manner, only isoprene and monoterpenes are commonly emitted at rates detectable by the PID in PORCO. Under standard leaf measurement conditions (1000 μmol m–2 s–1 PAR, 30°C) isoprene emission rates range from 1 to >100 nmol m–2 s–1, while a smaller fraction of plant species emit light-dependent monoterpenes at rates that rarely exceed 1 nmol m–2 s–1 but can occasionally reach rates similar to isoprene (Kesselmeier and Staudt, 1999; Klinger et al., 2002; Jardine et al., 2020a). The remainder of the most common volatile organic compounds that can be emitted by mature, photosynthetically active plant leaves in significant quantities are sesquiterpenes, acetaldehyde, formaldehyde, methanol, acetone, acetic acid, formic acid, and ethylene (Kesselmeier and Staudt, 1999; Laothawornkitkul et al., 2009). Emission rates of each of these compounds are typically <0.1–1 nmol m–2 s–1, except for methanol, which can reach several nmol m–2 s–1 (Kesselmeier and Staudt, 1999; Kesselmeier, 2001; Jardine et al., 2011; Bracho-Nunez et al., 2013; Kim et al., 2020a). Sesquiterpenes are highly reactive and would be unlikely to survive the tubing path length to the PID sensor (Niinemets et al., 2011). The PID sensor employed in PORCO (with a 10.6 eV UV lamp) is unresponsive to methanol, and at least ten times less responsive to the remaining compounds relative to isoprene, except acetone to which the PID is twice as responsive as isoprene (Rae Systems Inc, 2013c). The most commonly identified monoterpene in light-dependent emissions is alpha-pinene (Guenther, 2013), to which the PID is twice as responsive as isoprene (Rae Systems Inc, 2013c). Leaf emission rates detected by the PID in excess of 0.5–1 nmol m–2 s–1 are therefore expected to nearly exclusively comprise isoprene and small amounts of monoterpenes. Accordingly, PORCO measurements are scaled relative to the PID responsivity to isoprene and reported as isoprene equivalents unless stated otherwise.
PID Sensitivities, Control, and Calibration
Photoionization detector accuracy is affected by measurement error arising from internal and environmental causes (Rae Systems Inc, 2013b). Measurement error manifests via variation in the ‘baseline’ signal (calibration intercept, or signal from purified ‘zero’ air) and sensor ‘responsivity’ (calibration slope, or baseline-subtracted response to ionizable gas). Both the baseline signal and responsivity vary in response to temperature, humidity, and time (intrinsic drift). In PORCO, PID signal stability and measurement accuracy are enhanced by custom calibration techniques, temperature and humidity control, and data post-processing.
Because measurement error can be positive or negative, monitoring and correcting it requires a continuous non-zero data stream, ideally a raw voltage signal from the instrument. However, commercial PIDs will only log internally processed data based on onboard calibrations. The solution employed in PORCO is to conduct what we term a ‘PID initialization’ that results in its generation of ‘pseudo-raw data.’ Pseudo-raw data is the standard PID datalog in putative units of ppb, but adjusted to be a better representation of the raw electrical signal. This returns control of data interpretation to the user, so that corrections for environmental sensitivities and drift can be applied in data post-processing.
The PID initialization to generate pseudo-raw data is conducted by performing an onboard ‘zero’ calibration after intentionally reducing the PID’s baseline signal. This can be achieved by one or a combination of the following: apply a zero-air airstream with high humidity; reduce the temperature of the instrument; or install a UV bulb with a lower strength (bulbs of any given model vary in output, and trials will quickly demonstrate which of two bulbs is stronger). All three methods reduce sensor voltage and thereby the signal that the onboard computer interprets as ‘zero.’ Upon return to the configuration used during sampling, purified air will read far above zero, thus enabling the perception of any decrease in baseline signal. Similarly, an arbitrary ‘span’ (non-zero concentration) calibration can be employed to reduce the minimum signal interval from 1 ppb to less than 1 ppb. For example, conducting a span calibration set to 1000 ppb while providing 500 ppb of calibration gas will induce the instrument to return signal at 0.5 ppb intervals (essentially increasing the sensor ‘gain’). At some point, however, signal noise at the sensor exceeds the apparent resolution of the signal. Note that while the initialization procedure uses the onboard calibration features of the PID, this process is only used to induce the PID to display and log arbitrarily scaled pseudo-raw data, as a solution to the deficiencies of the instrument’s logging method described above and demonstrated in the subsequent analyses in this section. After this point, no further use of the PID onboard calibration system is required. From here onward in the text, the term ‘calibration’ refers to the standard procedure in which gas dilutions are applied, and the sensor baseline and responsivity coefficients are determined from the logged data in post-processing, and applied to sample data (see section “Field Data Processing”).
To demonstrate the consequences of not controlling for environmental sensitivities and drift, we performed calibration experiments to quantify the effects of humidity, temperature, and time on the PID baseline signal (calibration intercept) and responsivity (calibration slope) (Figures 2, 3). In the first experiment, the PID was stabilized at a given temperature in a thermally controlled enclosure, and exposed to hydrocarbon-purified air (‘zero-air’) with a series of distinct relative humidity values. Dilutions of isobutylene calibration gas in zero-air were applied, and the process was repeated at a higher temperature (Figures 2A,B,D). In the second experiment, the PID was stabilized at three different temperatures for a full day each, at constant relative humidity, and isobutylene dilutions were applied every few hours (Figures 2C,E,F).
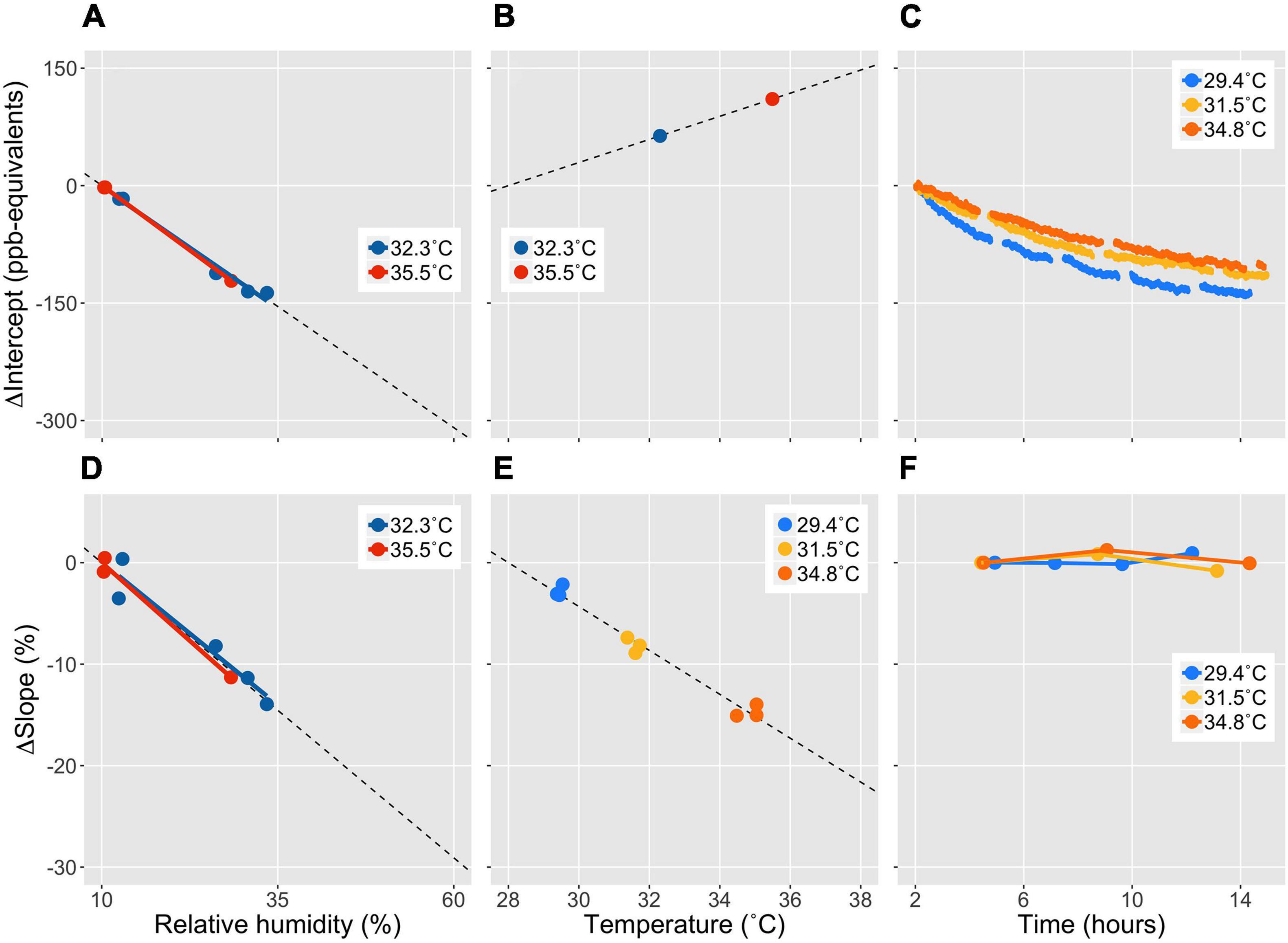
Figure 2. Sensitivity of PID calibration intercept (baseline signal) and slope (responsivity to isobutylene gas) to relative humidity, temperature, and time. To isolate each effect, the results in each panel are expressed as deltas relative to a common reference point. To compare the effect sizes of the different mechanisms of measurement error, the data are plotted across a typical range of diurnal variation of relative humidity (RH, 50%) and air temperature (T, 10°C) experienced during outdoor sampling. The negative sensitivity of the calibration intercept to RH (A) is about twice as strong as its positive sensitivity to T (B) and negative sensitivity to operation time (C) over the representative diurnal ranges. The negative sensitivity of the calibration slope to RH (D) is about 1.5 times the negative sensitivity to T (E) over the specified ranges, while no temporal drift was detected (F). We found no interactive effects between T and RH (A,D). To remove the effect of variable RH during T-sensitivity experiments, the calibration coefficients from (B,E) were adjusted to represent a common RH based on (A,D). Intercepts (A,B) were corrected for temporal drift (C), while the slopes in (E) are derived from three full-day experiments at different temperatures (F).
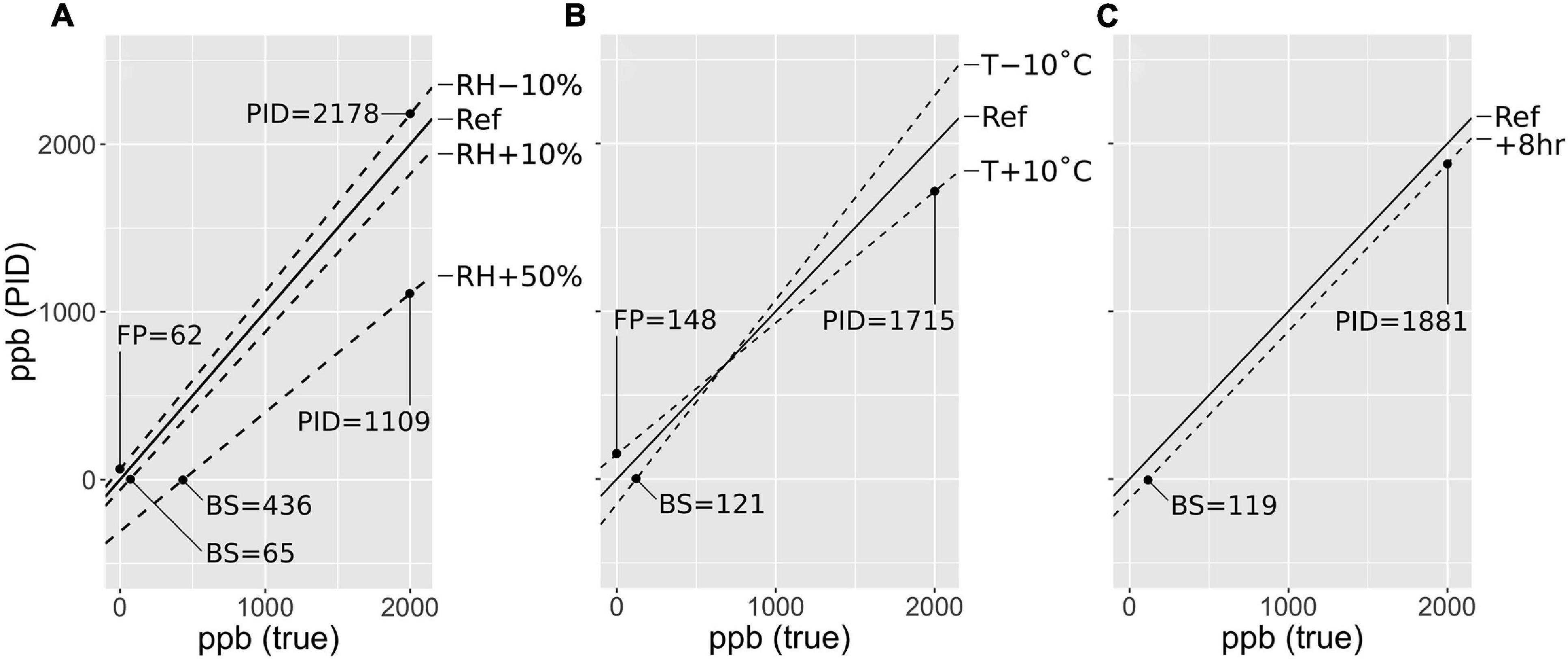
Figure 3. A rough guide for assessing the importance of controlling for PID measurement error (commonly called ‘PID drift’) for a given application and environment. This figure shows estimated outcomes of PID measurements of a gas ranging from 0 to 2000 ppb using the standard onboard calibration method, and measuring under varying sample relative humidity (RH, A), instrument temperature (T, B), and time (C). Conditions are specified at the right of each panel, with ‘Ref’ representing conditions during PID calibration. Sensitivities are derived from Figure 2. PID measurement error can manifest as ‘blind spots’ (BS) where the PID reads zero for non-zero gas concentrations, false positives (FP) where the PID reads non-zero for zero gas concentrations, and inaccuracies in non-zero gas readings (sensor responsivity). RH will be the most important concern for most users, though direct sun exposure can cause large changes in T. Controlling or correcting for PID measurement error will be most important for applications requiring lower detection limits, precision, and accuracy better than approximately 500–1000 ppb. This should be used only as a rough guide, as PID sensitivities likely differ between sensors.
Photoionization detector baseline signal was negatively sensitive to relative humidity of the measured air stream (Figure 2A), positively sensitive to enclosure temperature (Figure 2B), and negatively sensitive to instrument run time (Figure 2C). PID responsivity to calibration gas was negatively sensitive to relative humidity (Figure 2D), negatively sensitive to temperature (Figure 2E), and not sensitive to instrument run time (Figure 2F). Extrapolating the modeled sensitivities (linear regressions, dashed lines in Figure 2) across a 50% relative humidity range, and a 10°C temperature range, the relative importance of each factor can be assessed in terms of typical ambient environmental variation encountered during a day of outdoor field sampling. Relative humidity emerges as the most important driver of PID measurement error, causing a reduction in baseline signal equivalent to 300 ppb (Figure 2A), and a 30% reduction in sensor responsivity (Figure 2D) across a 50% range of relative humidity. This source of measurement error is especially relevant to sampling gasses from leaf enclosures, during which transpiration can rapidly increase sample humidity.
The importance of PID measurement error for a given sampling objective depends on the measurement conditions and the LDLs and precision required. To facilitate such an evaluation, Figure 3 shows modeled responses of a PID with a traditional on-board zero and span calibration to gas concentrations between 0 and 2000 ppb. Deviations of PID readings from true concentrations are modeled based on the responses of both baseline signal and responsivity to humidity, temperature, and time shown in Figure 2. For example, if sample humidity is 50% greater than calibration humidity, a false zero will be observed up to true concentrations of 436 ppb (the ‘blind spot’), and 2000 ppb will be interpreted as 1109 ppb (Figure 3A). An instrument temperature 10°C higher than during calibration will impose a false positive of 148 ppb in purified air, but will underestimate 2000 as 1715 ppb (Figure 3B). Note especially that the standard calibration technique necessarily imposes a mix of environmental conditions: purified ambient air (at ambient T and RH) is used for the zero, while the span is provided by direct application of calibration gas from the bottle. The calibration gas is both dry (near 0% RH) and cold due to decompression—the cooling of the sensor causes the typical downward drift in signal observed after completing the span and before disconnecting the PID from the gas bottle. Note that Figure 3 should be treated only as a rough guide, as sensitivities may vary considerably between sensors and calibration configurations.
While these analyses demonstrate the potential to measure the sources of PID measurement error and apply corrections in data post-processing, the best results are achieved by first maximizing environmental control. For example, temperature measurement of the sample air cannot be used to correct for thermal sensitivity unless sample and instrument are in thermal equilibrium. In PORCO, PID temperature sensitivity is mitigated by controlling instrument and sample-gas temperatures. The PID is enclosed in an insulated case, in which the air is heated to an above-ambient constant temperature (± 0.5°C) with a thermostat-controlled Peltier device (Figure 4). Sample (and zero or calibration) gas is equilibrated to the enclosure temperature by routing through coils of stainless steel tubing upstream of the PID (Figures 1, 4). Relative humidity is held low and constant (approximately 10% with precision ± 0.5% at the PID inlet) by routing sample air through tubing embedded in ice and water in an insulated enclosure (Figure 1 and Supplementary Figure 1), which maintains a vapor-saturated air space at constant temperature. Relative humidity drops when the air is subsequently heated prior to entering the PID, where humidity is logged with an iButton (Figure 1). This method produces constant humidity over 16 h of continuous run time in hot (30–40°C air) conditions. A small chamber at the bottom of a V-shaped tubing path in the dehumidifier catches condensed water and allows sample gas to pass above any liquid instead of traveling through it (Supplementary Figure 1). Isoprene and other common isoprenoids emitted by plants such as alpha-pinene (a monoterpene) have minimal water-solubility (Martins et al., 2017; Kim et al., 2020b), so interference by liquid water is negligible. While some PIDs offer an optional on-board correction for humidity and temperature (toggle in the software; Rae Systems Inc, 2013a), this should be disabled as the correction is small relative to the magnitude of sensitivities, and introduces unaccountable noise into the pseudo-raw data. We find that humidity removal by condensation is the most effective strategy, as our trials with desiccants such as Drierite (W. A. Hammond Drierite Co. Ltd.) showed significant interference with sample gasses.
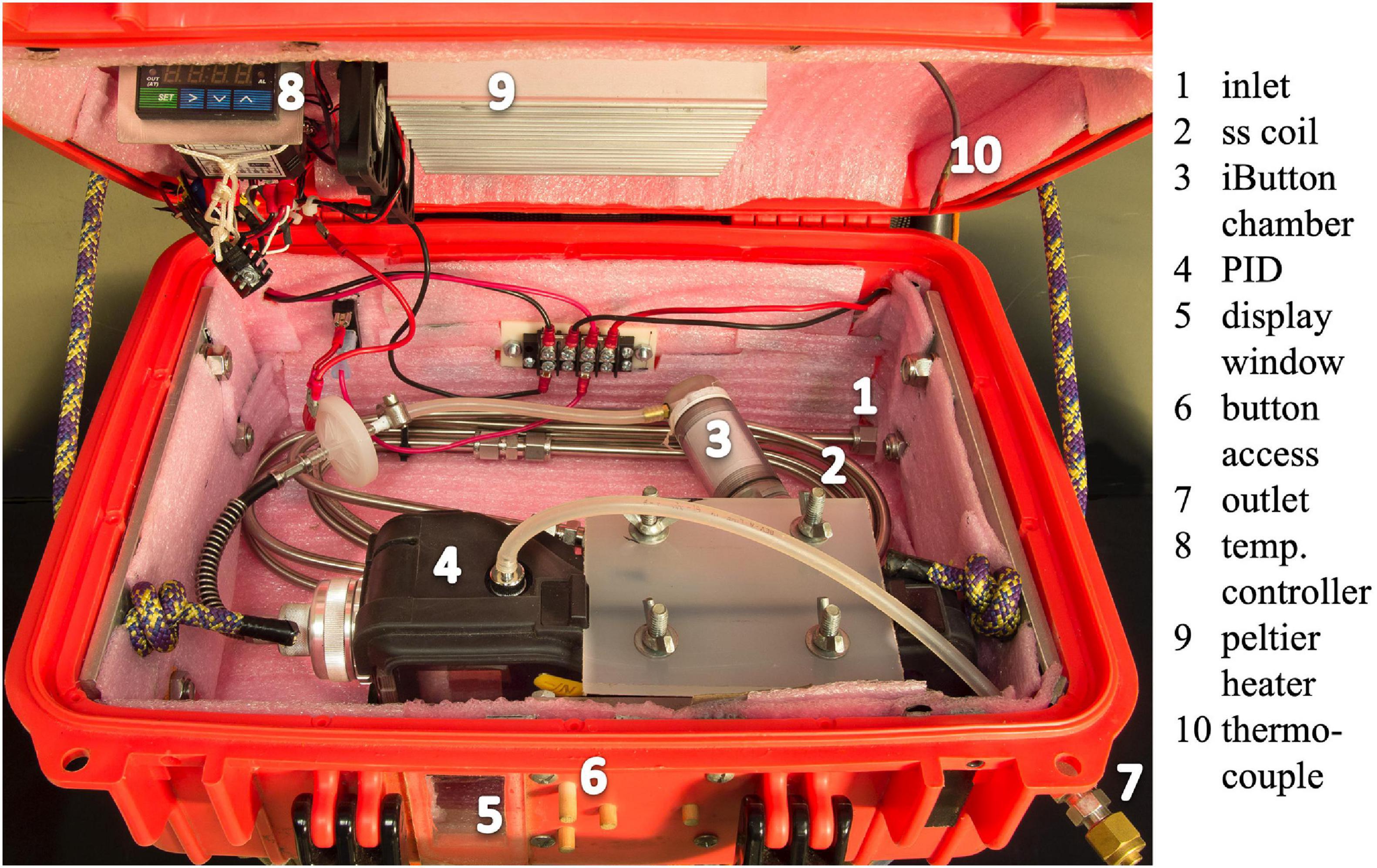
Figure 4. The temperature-control box provides thermal regulation of the PID and sample gas. The PID is fixed inside an insulated enclosure with a battery-powered Peltier heater. A thermocouple-equipped thermostat regulates the heater to maintain a fixed above-ambient temperature inside the enclosure. Sample gas enters the enclosure by tubing connection to a bulkhead union. Sample gas is pre-heated to match the enclosure temperature via coils of stainless steel (ss) tubing. Gas temperature and humidity are measured with an iButton in a PTFE-insulated chamber upstream of the PID. An acrylic window enables viewing of the PID display. Wooden pins at the box interface provide access to the buttons on the PID without opening the enclosure. PID sample exhaust is piped to an outlet port, which can be connected to alternative sampling media (e.g., bags, adsorption cartridges).
Photoionization detectors have different responsivities to different gasses due to variation in ionization energy among compounds. Measurements of different compounds can be scaled by the relative responsivity of the PID to isobutylene calibration gas using published correction factors (CF) specific to different UV lamp voltages (Rae Systems Inc, 2013c). However, empirical CF may differ between sensors or sample configurations. We find that the PID (using a 10.6 eV UV lamp) is 2.25 times more responsive to isoprene than isobutylene (CF = 0.44, Supplementary Figure 2), compared to 1.59 times (CF = 0.63) reported by Rae Systems (Rae Systems Inc, 2013c). Rarely, some compounds can interfere with PID responsivity (Rae Systems Inc, 2013a). Volatiles from silicone lubricants in valves, for example, can cause chronic signal reduction (‘sensor poisoning’) requiring cleaning of the sensor and sample path to recover responsivity (data not shown). These considerations are particularly important when sample gas contains variable mixtures of compounds, and when choosing materials that contact the sample gas (Niinemets et al., 2011). PORCO employs stainless steel and PTFE or equivalent tubing. Other materials are minimized and tested to ensure non-interference.
PORCO-PID Validation Against Fast Isoprene Sensor
We compared the performance of the PORCO-PID to the Fast Isoprene Sensor (FIS, Hills Scientific, Boulder, CO, United States) (Guenther and Hills, 1998) in a series of tandem calibrations and leaf measurements made in the lab. The FIS uses chemiluminescence in an ozone chamber to isolate the signal of isoprene from other volatiles, and is commonly used for leaf-cuvette based experiments in the lab (Monson et al., 2016; Lantz et al., 2019a). The instruments were installed in series such that the FIS sampled from exhaust air at the PID outlet. The PID was integrated in the standard PORCO configuration (Figure 1). Humidity in the sample airstream of both instruments was stabilized via the PORCO dehumidifier upstream of the PID (Figure 1 and Supplementary Figure 1). Isoprene standard dilutions were mixed upstream to generate stepped calibration curves (Figure 5). Isoprene emission was sampled from four plant leaves, using a LI-6400 leaf cuvette (LI-COR, Inc., Lincoln, NE, United States) for environmental control. The PID sampled from a tee in the cuvette return-air path. Direct calibrations to the FIS showed the inline PID had no effect on FIS calibration linearity or signal-to-noise ratio, but the PID did reduce isoprene concentrations at the FIS by 8.2% (Supplementary Figure 3). By calibrating and measuring leaves under the same instrument configuration, signal degradation by the PID was held constant and thereby nullified.
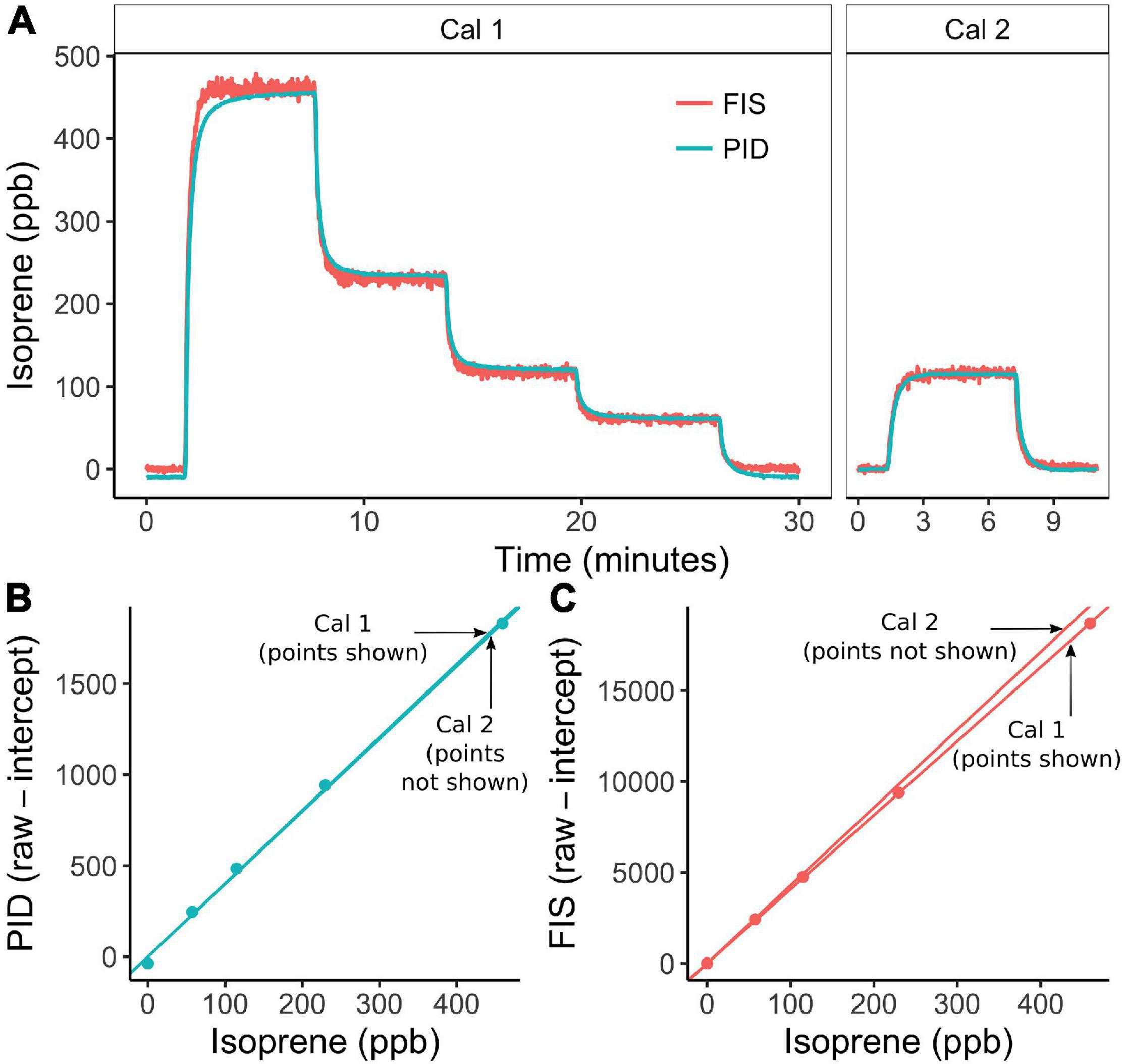
Figure 5. Inter-comparison between the photoionization detector (PID) employed in PORCO and the Fast Isoprene Sensor (FIS) shows comparable precision and signal stability in response to isoprene calibration gas. The PID sampled from an upstream gas mixture, while the FIS sampled from the PID exhaust. (A) Response of each instrument to dilutions of isoprene standard used to produce the calibration curves in (B,C). The instrument responses in (A) are visualized in calibrated units of ppb so that instrument signals are aligned and their agreement and stability can be compared. Cal 1 was performed prior to a series of leaf samples, and Cal 2 afterward, with an elapsed time between calibrations of approximately 4 h. Points in (B,C) are 15 and 30 s averages of PID and FIS raw signals respectively, from the end of each dilution response plateau. Noise at 1 s resolution is higher in the FIS (A), but the linearity of the FIS response (C) is slightly better than that of the PID (b). PID responsivity (slope) drifted less (B) than FIS responsivity (C), but the FIS baseline (intercept) was more stable over time (not shown, see discussion of temporal PID drift in Main Text).
The PID and FIS showed strong agreement in the time-series of isoprene standard (Figure 5) and leaf emission measurements (Figure 6 and Supplementary Figure 4). Compared to the FIS, the PID showed less signal noise at 1 Hz, slightly poorer linearity of response to isoprene standard dilutions, and less drift in responsivity (calibration slope) over time (Figure 5). The PID showed more drift in baseline signal (not shown, but see Figure 2C). Both instruments captured the subtle signal of a post-illumination isoprene emission burst (Li and Sharkey, 2013) from an oak leaf (Figure 6A). Averaged emission rates estimated by the two instruments from the final two minutes of each leaf measurement strongly agreed (linear regression, r2 = 0.999, Figure 7). The PID underestimated emission rates relative to the FIS by 12% (slope [PID∼FIS] = 0.88). The reduction in PID responsivity in sample vs. calibration mode is attributable to a pressure difference between calibration and sample configurations, with a higher flow rate and more resistant excess-flow path producing positive pressure at the PID during calibration (see diagram in Figure 1 showing positive and negative-pressure flow paths). This discrepancy can be quantified and corrected for any given sample configuration (see section “Cuvette Validation”). Best field practices include calibrating and sampling at similar sample-air pressures.
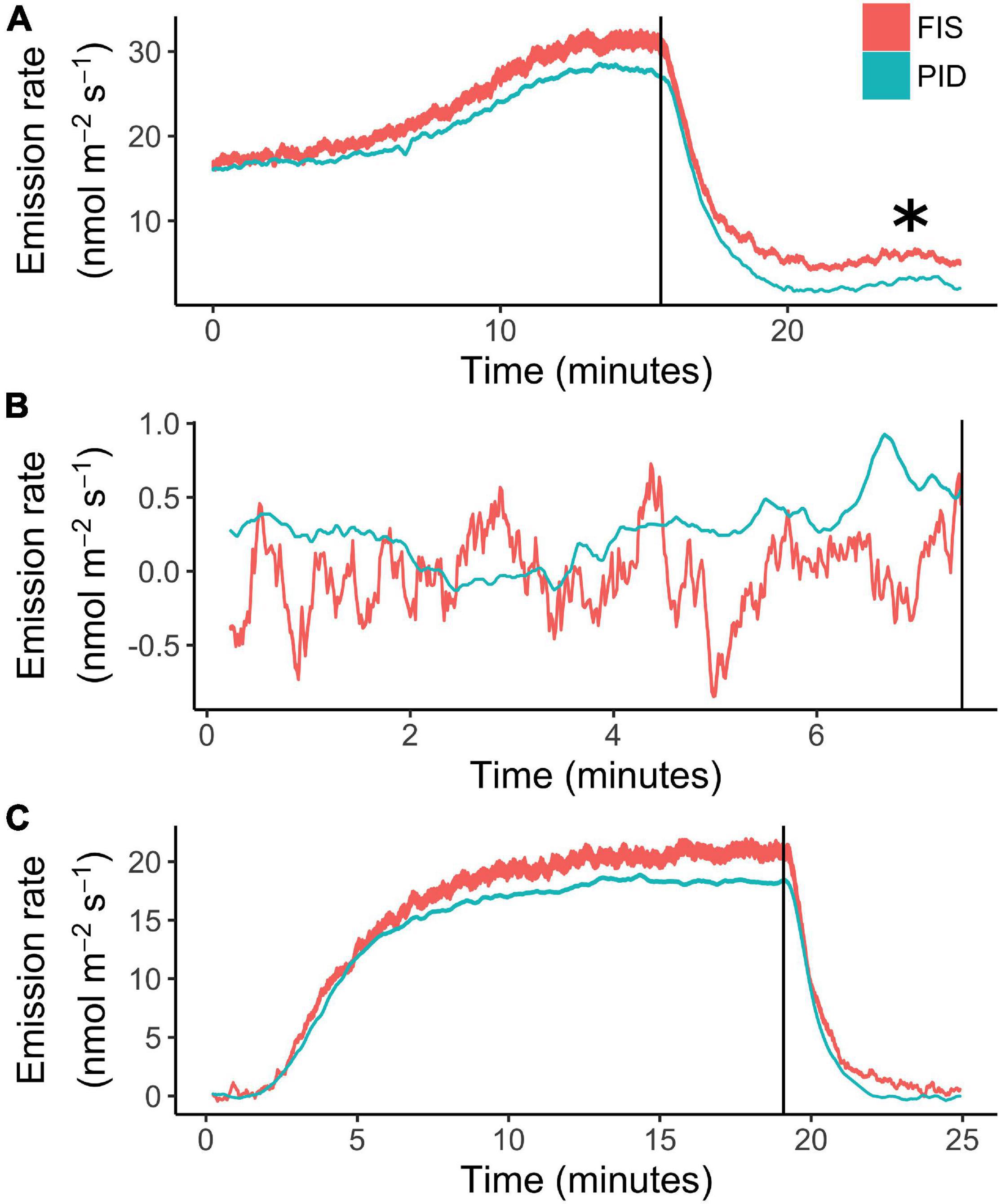
Figure 6. Tandem real-time measurements of isoprene emission from tree leaves by photoionization detector (PID) employed in PORCO, and a Fast Isoprene Sensor (FIS). The instruments were set up in series, whereby the leaf environment was controlled by a Licor-6400 leaf cuvette, the cuvette return-air was subsampled by the PID, and the FIS subsampled air exhausted from the PID outlet. PID and FIS calibrations were performed simultaneously under the same series configuration. Time series data are ribbons, bounded by the emission rates estimated from pre- and post-measurement calibrations, demonstrating confidence relative to sensor responsivity drift. The asterisk in (A) indicates a post-illumination isoprene emission burst captured by both instruments. Species are (A) a temperate oak, Quercus sp., (B) an ornamental shrub, Hibiscus rosa-sinensis, and (C) a tropical tree, Malpighia glabra. Leaf (A) was acclimated to light before emission sampling. For leaves (B,C), cuvette light was turned on at time 0. Vertical lines mark the time at lights-off [cuvette was opened at lights-off in (B)].
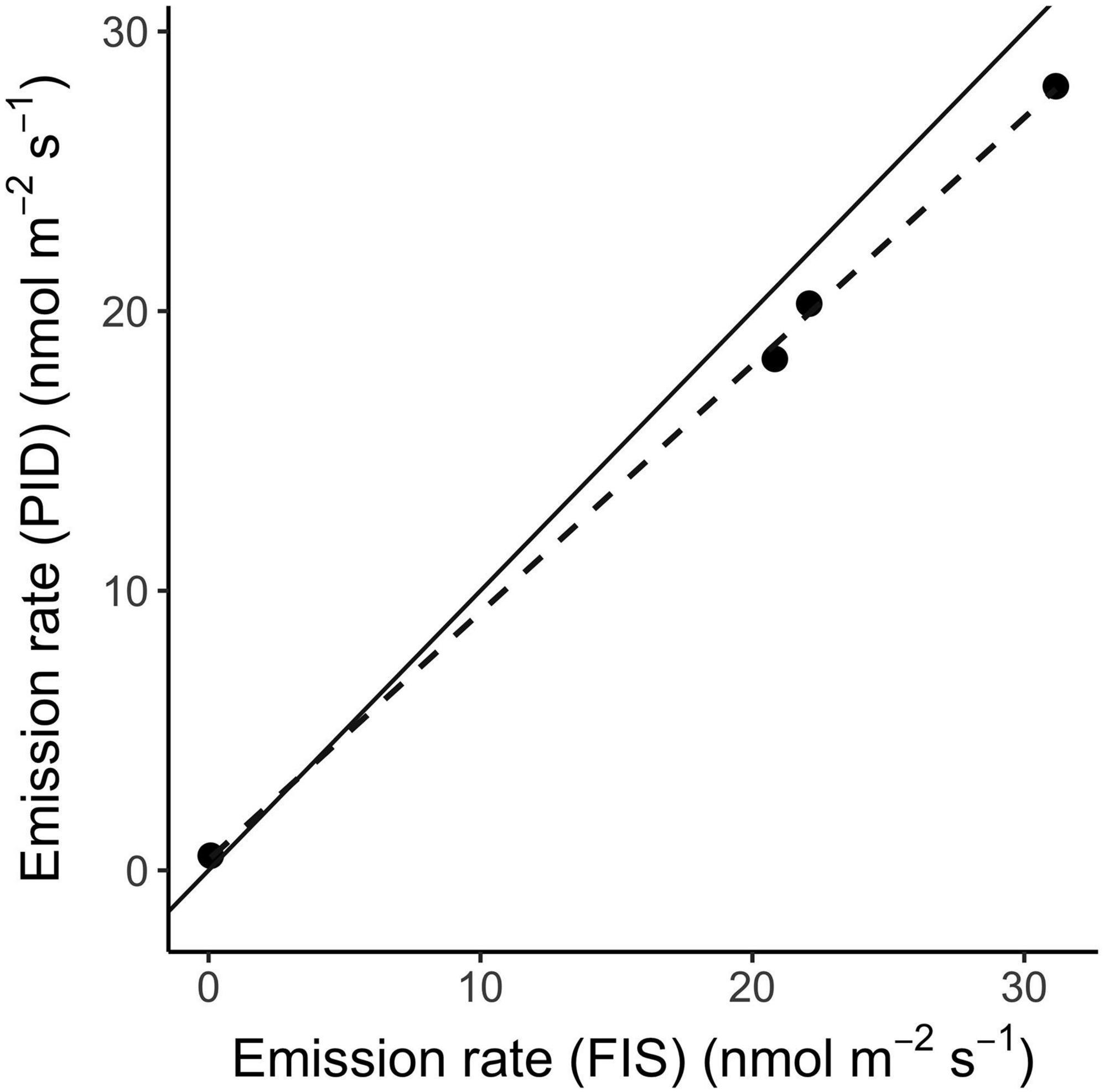
Figure 7. Isoprene emission rates calculated from photoionization detector (PID) and Fast Isoprene Sensor (FIS) readings, averaged over the final two minutes of leaf illumination from four leaf samples (including those depicted in Figure 6 and an additional sample from Malpighia glabra, Supplementary Figure 4). The solid line is 1:1. The dashed line shows a linear regression through the data points (r2 = 0.999, slope = 0.88).
The correlation between PID- and FIS-derived isoprene concentrations using all data points from 1 s resolution leaf measurements was similar to that shown in Figure 7 (Supplementary Figure 5, linear regression: r2 = 0.971, slope [PID∼FIS] = 0.88). The regression residuals indicating sensor consistency over short timescales had a relatively large range of 9.7 ppb (97.5−2.5 percentiles), attributable primarily to signal noise from both instruments. We estimated signal noise by fitting a smoothing function to the PID and FIS data from all leaf measurements (loess fit with span = 0.1; Supplementary Figure 5B). The range of signal noise (loess residuals; 97.5−2.5 percentiles) was significantly higher for the FIS (8.3 ppb) compared to the PID (1.7 ppb) (ANOVA on bootstrap estimates of variance of the residuals: p << 0.001; see Supplementary Figure 5).
Leaf Cuvettes
Cuvettes used for capturing volatile emissions from plant leaves vary from translucent bags enclosing whole branches (Padhy and Varshney, 2005; Geron et al., 2016; Purser et al., 2020) to highly environmentally controlled leaf enclosures such as those employed in commercial photosynthesis instruments (Tholl et al., 2006; Alves et al., 2014; Jardine et al., 2015). While PORCO-PID adaptations can improve real-time field sampling precision from a diversity of cuvettes, PORCO employs custom leaf cuvettes with design features that optimize precision and detection limits relative to the sensitivities of the PID. The cuvettes also provide a low-cost solution for quantitative leaf emission sampling using other gas-detection devices. Leaf illumination can be provided from ambient light, or using the custom modular LED panels described in section “Leaf Light Environment.”
Cuvette Design
To quantify emission rates, sampled trace gas concentrations must exceed the LDL of the detector. Emitted gasses accumulate inside a cuvette at a rate dependent on the leaf size, emission rate, cuvette volume, and the replacement rate of cuvette air. The PORCO cuvettes were designed to achieve a LDL equal to a leaf area-based emission rate (ER) of 1 nmol m–2 s–1 or better within 60 s of measurement from a majority of leaf types found among tropical rainforest trees. A 60 s LDL of ER = 1 is a conservative goal that allows for confident distinction of weak emissions from non-emission over slightly longer (minutes) measurement times under non-ideal conditions such as sub-optimally sized leaves, reduced physiological activity of cut branches, or environmental instability causing PID measurement error. We evaluated cuvette designs in terms of the ratio of ER to the concentration of the emitted gas accumulated in the cuvette after 60 s, which we term the ‘signal amplification factor’ (SAF). Given typical signal noise of up to ± 2 ppb of isoprene, a confident LDL for the PORCO-PID is approximately 5 ppb. Therefore, a minimum SAF = 5 is optimal for detection of ER = 1 (i.e., 5 ppb is accumulated in 60 s). For comparison, for the LI-6400 leaf cuvette, SAF = 1.5 due to the small cuvette volume relative to a standard flow rate (550 sccm), resulting in LDL = 3.3 nmol m–2 s–1. Moreover, at a given emission rate, gas concentrations in the LI-6400 cuvette reach steady state in less than 1 min, while concentrations continue to increase in larger cuvettes beyond 1 min, giving a greater potential to exceed LDLs.
Because the flow rate through the cuvette must exceed the sampling rate of the PID (300 or 500 mLpm), increasing SAF requires increasing the amount of leaf area enclosed, while minimizing the increase in cuvette volume. We designed three cuvettes that provide SAF > 5 by fully enclosing entire leaves or leaflets (of compound leaves), with distinct shapes that accommodate leaf types of a large majority of tropical tree species.
Cuvette Construction
Custom PORCO leaf cuvettes (Figure 8) are rigid acrylic boxes that enclose entire leaves. The petiole or twig traverses a port by which leaves maintain connection to their branch. Operation is a flow-through system where pure air is pumped through an inlet port, and sample air is drawn by the pump in the PID via an outlet port (diagram in Figure 1). Excess flow is exhausted through a partial-seal around the leaf petiole (‘petiole gasket,’ Figure 8). An internal fan ensures even air mixing, necessary for non-steady-state flux calculations. All internal parts are acrylic, stainless steel, or PTFE to minimize adsorption and release of trace gasses, except for the small plastic mixing fan (with a brushless motor, which minimizes ozone production), which does not produce significant interference, as demonstrated by stable background signals during both dark and illuminated cuvette blank measurements. The acrylic initially produced significant background concentrations of ionizable gasses, but this was successfully eliminated by baking cuvettes in a drying oven at 60°C for 5 days. A roof mounting system accommodates modular LED panels and a light diffusor. Reflective siding ensures even light distribution and minimal interference from ambient light.
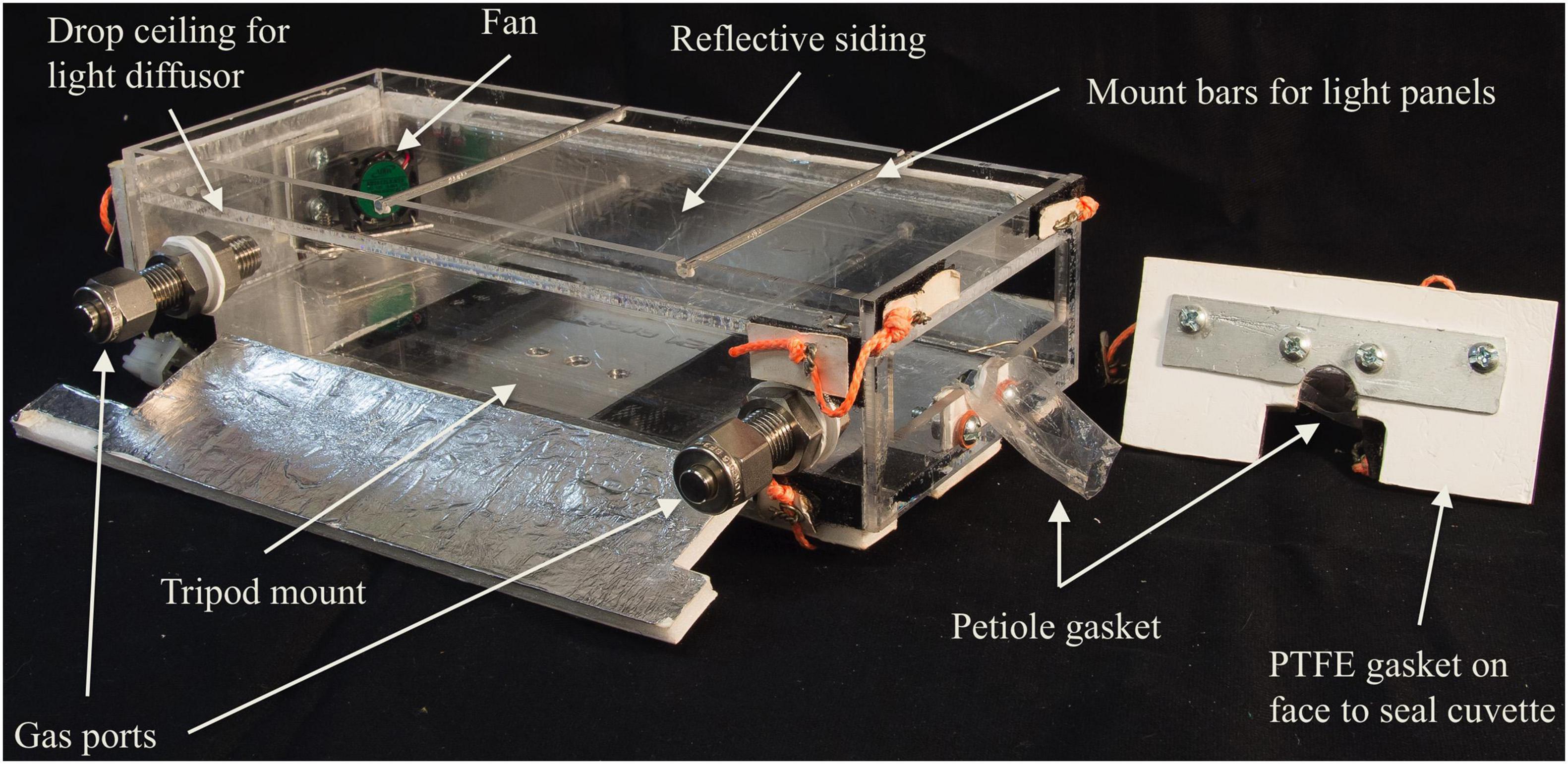
Figure 8. One of three uniquely shaped leaf cuvettes, designed to accommodate a range of leaf shapes and sizes. The leaf is inserted into the opening at right, and the petiole settled into the U-shaped slot. Tensioned cords seal the faceplate with the PTFE gasket to the front of the box, with its inverted U-shaped slot and petiole gasket opposing those on the cuvette face. The petiole gasket is loosely sealed around the petiole with cord such that excess flow (150 mLpm or greater) can escape, but diffusive gas exchange with outside air is not permitted. Light panels mount to the top of the cuvette to drive photosynthesis. Reflective siding ensures even light distribution and minimal interference from ambient light.
Cuvette Validation
Constant leaf emission inside a cuvette should produce an asymptotic increase in gas concentration. The shape of the concentration curve depends on the emission rate, cuvette volume, and flow rate. With a known flow rate and cuvette volume, the emission rate can be calculated from any portion of the curve. Assumptions about the shape of the curve can be validated by simulating leaf emissions by conveying calibration gas to the cuvette inlet (Figure 9). Insufficient air mixing would reduce the effective mixing volume, producing an initial increase in concentration that is faster than expected. Our simulated emissions show an initial increase that is slightly slower than expected. Insufficient excess flow out of the petiole gasket may produce a diluted curve if the seal is poor, due to mixing with outside ambient air. However, tightening the petiole gasket seal or increasing the flow rate to the cuvette does not alter the results (data not shown), suggesting that excess flow through the loosely sealed petiole gasket under normal sampling conditions is sufficient to mitigate dilution.
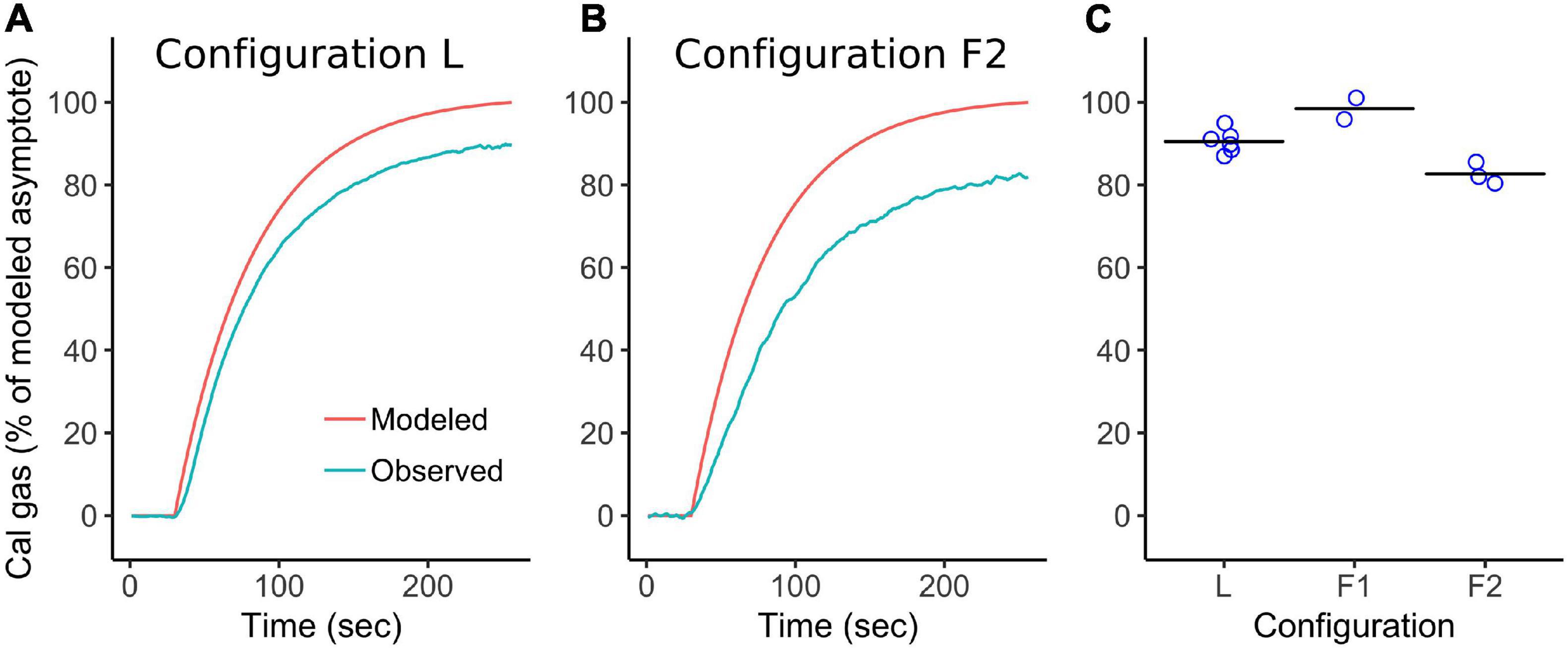
Figure 9. Calibration gas is piped to leaf cuvettes to simulate leaf emissions in order to validate emission calculations from curves of concentration changes. Calibration correction factors are derived from emission simulations to correct for the effect of pressure differential between calibration and sample modes on PID sensor readings. (A) Example of a simulated emission showing expected (modeled) and observed cuvette concentrations measured by PID under a laboratory configuration. (B) Example of a simulated emission under the F2 field configuration. (C) Observed concentrations at 210 sec as a percentage of expected concentrations during simulated emissions under three different plumbing configurations, one lab, two field. Mean values [horizontal lines in (C)] are used to correct sample readings for the effect of the pressure drop for a given sampling configuration.
Simulated emissions show that the largest measurement bias arises from pressure differences caused by different plumbing configurations. The balance of pressure from the zero-air pump (positive) and PID pump (negative) differ between calibration and sample modes (Figure 1), and between different sample-plumbing configurations. During calibration, positive pressure is determined by the total flow rate and the resistance of the excess-flow exhaust path (coiled 1/8” tubing). During sampling, the PID pump pulls air from the cuvette through a relatively long pathway via the dehumidifier and thermal equilibration tubing (Figure 1), causing a pressure drop. We have not attempted to measure pressure in order to quantify this effect. Pressure effects are particularly evident when changing flow modes by turning valves, which inevitably causes a transient spike in PID readings (Supplementary Figure 6). If valves are turned too quickly, or the flow path is momentarily blocked, this reading spike can be extremely large and persist in the form of an asymptotic recovery toward the previous baseline signal over a half hour or more. Strong negative pressure could also exacerbate the effect of small leaks in sample path connections, though we note that sample dilution caused by leaks is inconsistent with the observation of reduced signal at the PID (after switching from calibration to sample mode) relative to an independent instrument sampling directly from the PID exhaust (i.e. the independent sensor should have seen the same reduction in signal, see section “PORCO-PID validation against Fast Isoprene Sensor”). Pressure effects are plausibly related to the fact that the PID pump, ionization bulb, and sensor are all powered by the same battery, and therefore a change in pump effort caused by a change in flow pressure causes a change in sensor voltage. Different calibration and sample configurations therefore produce a corresponding offset of observation from expectation during simulated emissions. The offset is consistent within configurations (Figure 9C), allowing calculation of a configuration-specific correction factor for leaf emission estimates. Calibrating exclusively via simulated emissions would obviate the need for correction factors, but this requires much more calibration gas, which is in limited supply in the field due to the logistical challenge of transporting compressed gas cannisters.
Leaf Light Environment
PORCO is designed to measure emissions of light-dependent hydrocarbons, i.e., those responsive to light via the dependency of their production on photosynthetic metabolism (Laothawornkitkul et al., 2009). For comparability of measurements, leaf illumination by photosynthetically active radiation (PAR) is typically standardized to 1000 μmol photons m–2 leaf s–1 (Monson et al., 1995; Niinemets et al., 2010b). In PORCO, PAR is provided by custom panels of light emitting diodes (LEDs) mounted atop the leaf cuvettes (see section “Standardized Leaf Measurement with PORCO”). The light panels were designed to mimic the peak light wavelengths and ratios employed in the LI-6400 leaf chamber: 90% red light with peak output at 670 nm, and 10% blue light at 465 nm. The PORCO light panels use Cree Xlamp “Photo Red” (peak output at 650–670 nm) and “Royal Blue” (450–465 nm) LEDs (Cree Inc., Durham, NC, United States). Light ratios and total quantity are regulated based on a calibrated relationship between measured PAR output and electrical current to the bulbs (Supplementary Figure 7). During measurements, electrical current to each bulb color on each panel is adjusted to target values via dimmer dials, based on voltage read across precision inline resistors (yellow box in Figure 1). Electrical current-controllers maintain a steady photon flux density at the leaf plane.
The modular LED panels can be adapted to a diversity of cuvettes. In PORCO, up to three panels are used at a time depending on cuvette size. The LED panels could be easily applied to custom cuvettes adapted for other purposes, such as moss or algal growth chambers.
Field Data Processing
The continuous pseudo-raw data logged at 1 Hz from the PID is processed with custom code in R (Wickham, 2016; R Core Team, 2020). Figure 10 illustrates the processing flow from a day of field measurements. During measurements, the user records start and end times for each type of sample, such as zero-air, calibration air, cuvette blanks, and leaf samples. Data processing is applied to specific time brackets in the time-series output based on the type of measurement recorded. A signal lag of 21 s from the cuvette to the PID is accounted for. Emission rate estimates are standardized to 30°C.
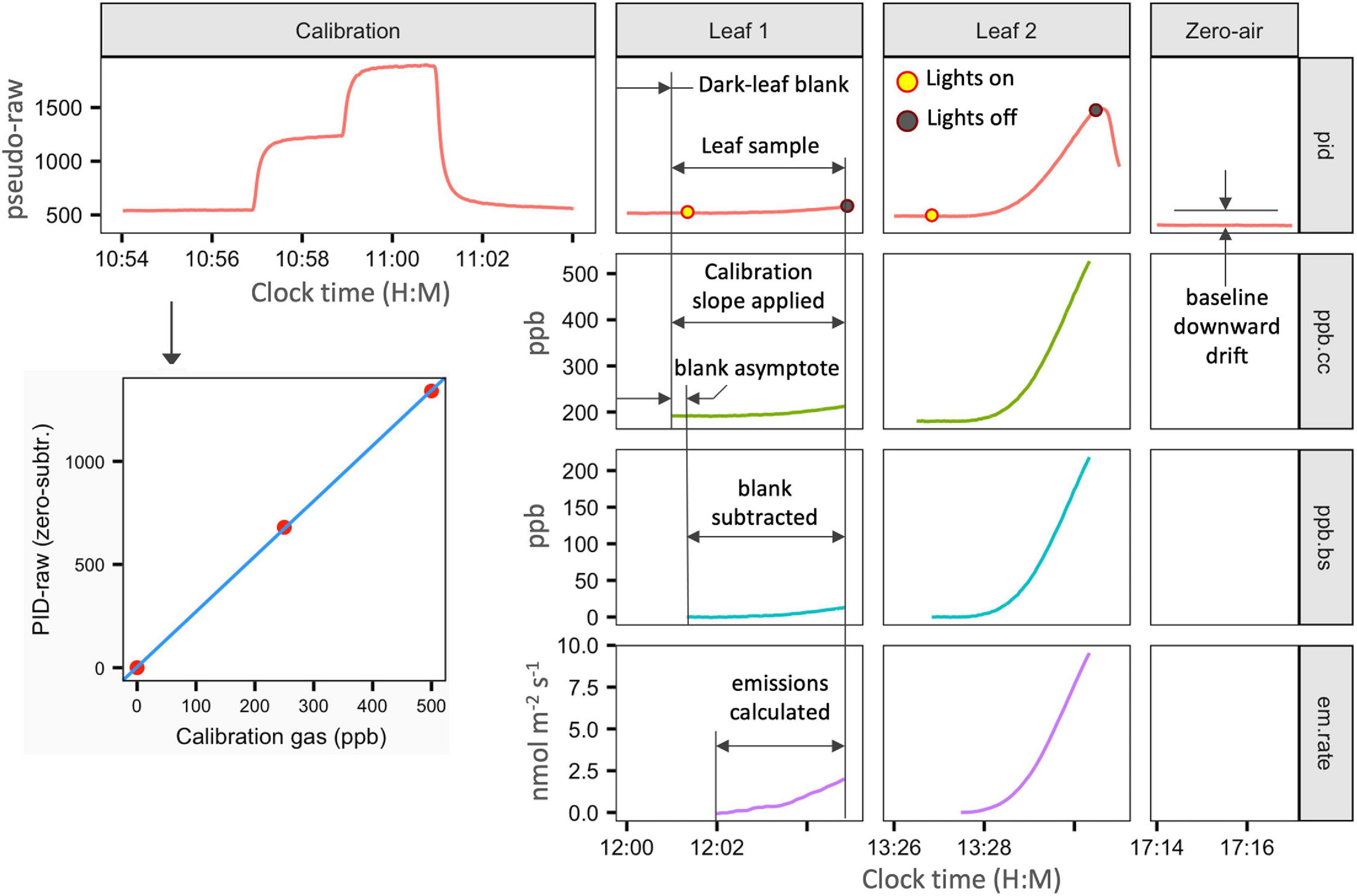
Figure 10. Demonstration of the data processing flow from selected time segments during a day of measurements. Data shown is actual field data from a tropical rainforest. The timing of all calibration and sampling activities are manually noted with 1 s resolution and entered into standardized tables for data processing. Custom processing code in R applies calculations and data transformations according to the activity specified for each time bracket. A calibration determines the responsivity coefficients for conversion of PID pseudo-raw data to gas concentrations. With a leaf in the cuvette, the background is allowed to stabilize for several minutes. Leaf measurement data is converted to calibration-corrected ppb (ppb.cc) by the calibration slope only. The asymptotic tail of the cuvette blank (stabilization period with the leaf in darkness) is then subtracted from the subsequent leaf-sample period (ppb.bs). The relative responsivity to isoprene (0.44 compared to isobutylene calibration gas) is also applied. Emission rate (em.rate) is then calculated as a non-steady-state flux from the time-series of ppb.bs data.
Field Calibration
Linear calibration curves are made by measuring isobutylene standard diluted in purified (‘zero’) air. The air streams are regulated at desired flow rates by mass-based flow controllers (Alicat Scientific, Tucson, AZ, United States) and joined before exhausting through coiled eighth-inch tubing to minimize ambient interference, while the PID samples the mixture from a tee junction. Calibrations are performed at the beginning of each day of measurements, and frequently at the end of each day to ensure responsivity stability. Periodic zero-air readings can be used to interpolate and subtract baseline drift (Figure 2C).
Leaf Emission Rate Calculation
For standard leaf measurements with PORCO cuvettes, the stabilized signal from the cuvette with the leaf installed and lights off (‘cuvette blank’) is used as the baseline for emission calculations. Leaf emissions are calculated as a non-steady state flux determined from linear regressions of gas concentration changes over time along a 40 s sliding window of data following the moment the cuvette lights are turned on (Figure 10). Specifically, fluxes are calculated based on the following equation:
Where C is the concentration of VI in ppb (ppbVI = nmolVI molair–1), V is the volume of the cuvette in molair, and Fin and Fout are the flows of VI (nmol s–1) into (from leaf emission) and out of (via the purified air stream) the cuvette. Fout at any moment is Fair C, the product of the flow rate of purified air (mol s–1) and C. Rearranging the above equation gives leaf VI emission rate Fin (nmol s–1) as:
We estimate the average leaf emission over a 40 s time window using (estimated via a linear regression of C against time) and , where the overbars indicate an average over the time window. The first VI emission rate (nmol s–1) is calculated at t = 40 s, and the process is iterated, advancing the window by one second until the end of the measurement.
Temperature Standardization of Leaf Emission Rates
Emission rates of light-dependent VI vary with temperature as a function of enzyme reaction kinetics (Guenther et al., 1993). For comparability, standardized emission measurements are typically performed at 30°C. Due to the difficulty of obtaining tight temperature control in larger cuvettes, a common approach is to record the measurement temperature and scale emission rates to a 30°C equivalent based on an empirical temperature response curve of emissions (Niinemets et al., 2011; Purser et al., 2021). In PORCO, cuvette temperature is measured by an iButton shaded with a PTFE roof. Cuvette temperature varies with ambient temperature and is elevated by the LED lights, resulting in average measurement temperatures of 3.7°C above shaded ambient temperatures (Supplementary Figure 8). Cuvette temperature at the end of the measurement is used to standardize emission rates to a 30°C equivalent based on a temperature sensitivity coefficient (CT) representing the temperature dependence of isoprene described by Guenther et al. (1993):
where CT is the coefficient for a given temperature, T is measurement temperature (Kelvin) represented by cuvette temperature, TS is a normalizing temperature set to 301 K, R is a gas constant (8.314 J K–1 mol–1), and T1 (95,000 J mol–1), T2 (230,000 J mol–1), and T3 (314 K) are empirical coefficients. The coefficients at cuvette temperature (CTCUV) and 30°C (C30) are used to scale the emission rate at cuvette temperature (ETCUV) to 30°C (E30) by E30 = ETCUV(C30/CTCUV).
Cuvette temperatures from tropical field measurements (see section “Field Measurements From 52 Tropical Tree Species”) ranged from 31.7° to 37.0°C (5 and 95% quantiles, respectively), and the associated scaling factors (C30/CTCUV) ranged from 0.54 to 0.83 (5 and 95% quantiles, respectively) (Supplementary Figure 8). While temperature standardization helps to compare emission rates between studies (e.g., all of our measurements at higher temperatures are scaled downward), the small range of variation in scaling factors implies that the effect of temperature variability on emission rates during tropical field measurements with PORCO is small compared to the variation in emission rate capacities spanning three orders of magnitude among tree leaves (Supplementary Figure 9).
Integrated System
Integrating all of these components into a single, portable system allows us to bring the laboratory to the leaf. For the first time, a system that can be carried and operated by a single person under field conditions can provide precise, real-time information about plant volatile emissions, allowing unlimited sampling. In contrast to ‘offline’ sampling methods requiring subsequent laboratory analysis, PORCO provides live measurement feedback and the ability to view processed results after each measurement day, allowing the user to make informed adjustments to their sampling efforts while in the field. PORCO has the potential to accelerate a nascent field of science: the ecology and ecophysiology of plant volatiles.
Standardized Leaf Measurement With PORCO
PORCO measures emissions from leaves that are attached to branches and under conditions suitable for photosynthesis (Figure 11). Measurement preparation begins with choosing a cuvette for the leaf that maximizes the ratio of leaf area to horizontal cuvette area without bending the leaf edges. Purified ambient air is conveyed to the cuvette inlet port via a mass-flow controller, while the PID draws air from the outlet port. The LED panels are attached to the cuvette, and electrical current is adjusted to produce the desired light output (1000 PAR for standardized measurements). The lights are then switched off.
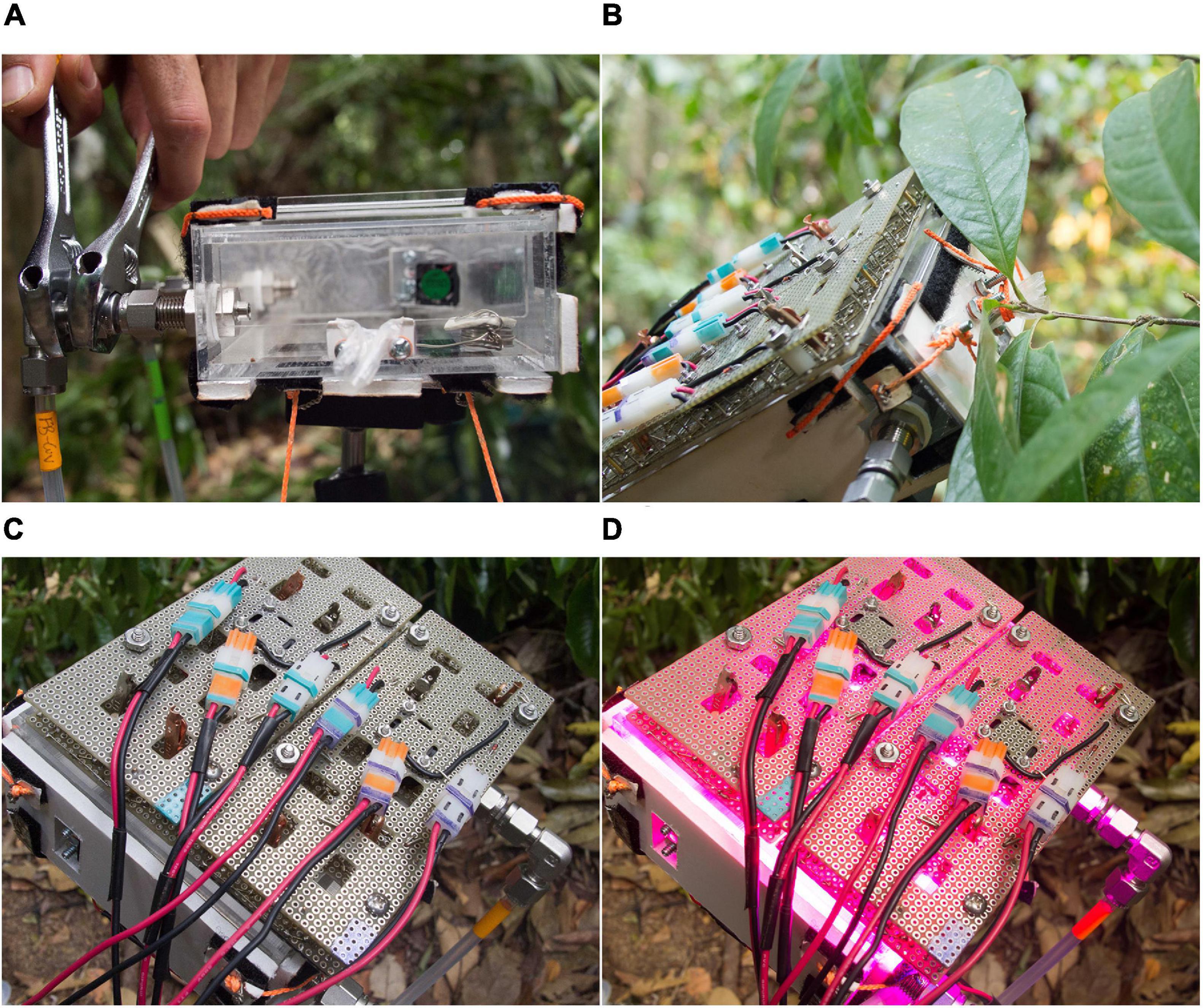
Figure 11. To measure emissions from a leaf, the appropriate cuvette is chosen (A), maximizing the amount of leaf area relative to cuvette volume. The leaf is installed while still attached to its branch, and the cuvette faceplate fixed with the petiole gasket loosely sealed around the leaf petiole (B). The leaf sits in darkness until ambient air is cleared (C, the ‘dark-leaf blank’ period in Figure 10). Then the lights are switched on, set to deliver 1000 μmol m–2 s–1 PAR (D).
The leaf is installed by carefully positioning the cuvette to enclose the leaf, using an articulating and locking arm mount to closely match the cuvette orientation to leaf orientation, minimizing leaf disturbance. The cuvette faceplate is installed and the petiole loosely sealed by the petiole gasket. Purified air is conveyed to the cuvette at a rate ≥ 150 sccm above the PID sampling rate (either approximately 500 or 300 mLpm depending on PID pump-speed setting). Excess flow is exhausted from a loose seal around the leaf petiole to resist diffusion of ambient air into the cuvette. The leaf is left in darkness inside the closed cuvette for sufficient time to clear ambient air and achieve a stable background signal (6–9 min depending on cuvette volume). The LED lights are then switched on, driving photosynthesis and VI emissions if the leaf is an emitter.
For standardized measurements at 1000 PAR, leaves are illuminated for 3.5 min. Longer illumination periods at high light levels are avoided due to the potential to overheat the cuvette. Continued monitoring following lights-off allows confirmation of weak emission peaks, and analysis of species variation in post-illumination emission dynamics (e.g., Figure 6A). Live emission information can be qualitatively determined during a measurement by monitoring the numerical output on the PID display (a single number varying at 1 Hz, equivalent to the datalog) through the enclosure window (Figure 4). Careful observation allows the distinction of even weak emitters from non-emitters in real time, as well as the detection of anomalous signal drift that would contribute to a bad measurement. When measurements are completed, leaves are scanned to determine their area, then dried and weighed so that emission rates can be scaled by either leaf area or mass.
Field Measurements From 52 Tropical Tree Species
The primary components of PORCO, excluding leaf cuvettes, are mounted on an external-frame backpack for portability. The system can be carried by one person through a forest, up a canopy tower, or hauled by ropes into trees or canopy walkways (Figure 12). All of the plastic cases can be closed during transport with all air-flow components still running to maintain PID stability. The backpack system weighs 23.5 kg. For tall trees, branches can be cut, carefully lowered, re-cut under water to maintain the transpiration stream, and then measured on the ground. Where accessible, the portability of PORCO allows in situ measurements of tree leaves, which eliminates the potential for undesired physiological responses to branch cutting.
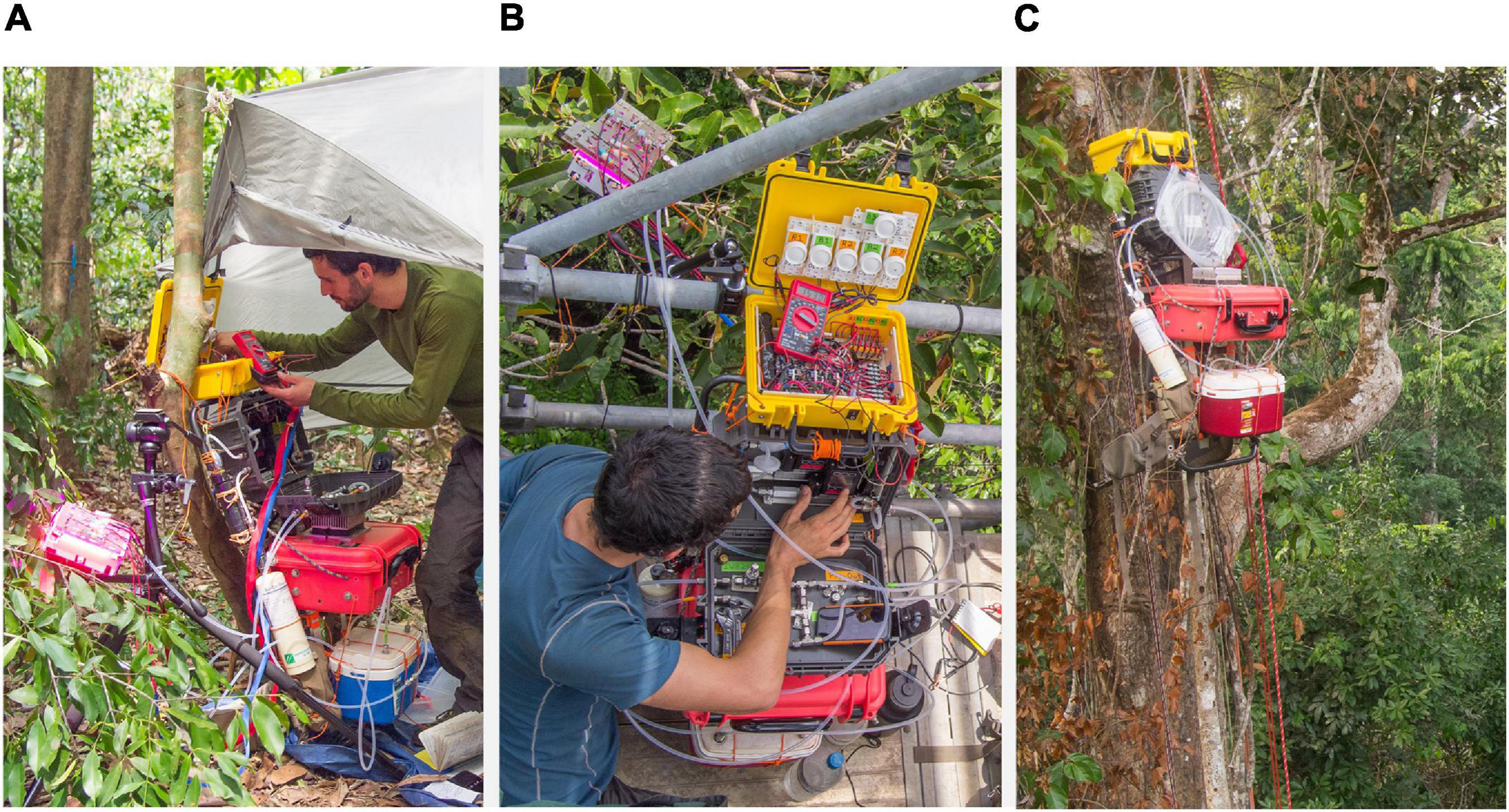
Figure 12. Field measurements with PORCO in a tropical forest: (A) on the ground with cut branches; (B) on a canopy walk-up tower for in situ measurements on intact branches; (C) hauled into the canopy for in situ measurements from a suspended walkway. Subject in images is the lead author of the present study.
We used our uniquely portable detection system to quantify leaf VI emissions from tropical trees at a field site in the eastern Amazon, the “k67” eddy flux tower site in the Tapajos National Forest near Santarém, PA, Brazil (Rice et al., 2004), during the dry season (August to December) of 2015. In 27 days of field sampling, we obtained high quality emission data from 138 leaves from 67 trees and 52 species (Figure 13). All species were professionally identified from pressed specimens (Herbario IAN of Embrapa in Belém, PA, Brazil), and taxonomic names were standardized by the Taxonomic Name Resolution Service (Boyle et al., 2013) (accessed August 22, 2020, Supplementary Table 2).
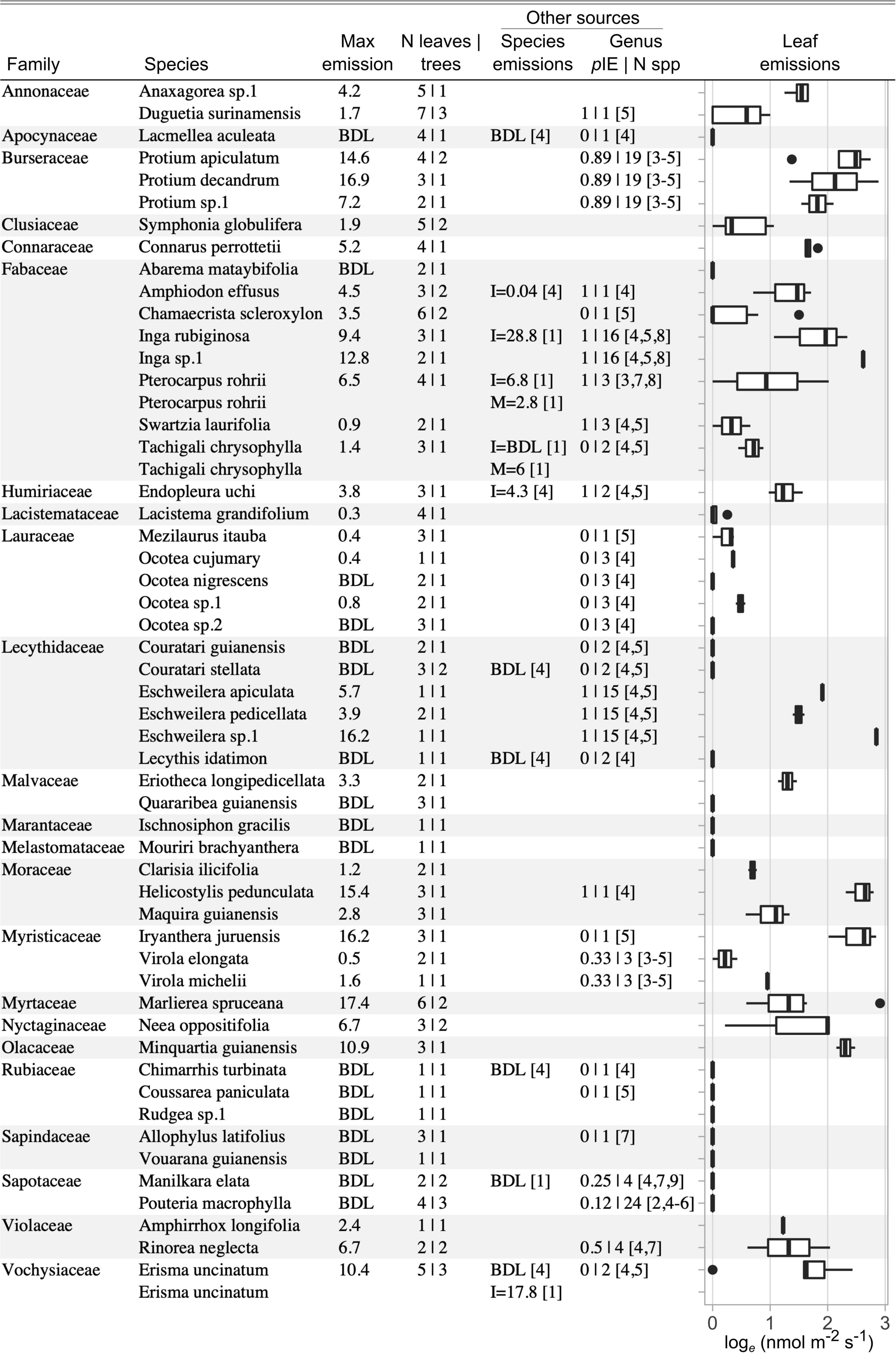
Figure 13. Volatile isoprenoid emission rates from 52 tree species at an eastern Amazon site, the Tapajós National Forest, PA, Brazil. Emission rates are calculated from the end of a 210 s period of leaf illumination at 1000 PAR. Detectable emission rates were subsequently standardized to 30°C based on a standard temperature response curve. Max emission per species is provided (tabular, in nmolVI m–2leaf s–1), as well as the distributions of emissions from all leaf measurements (graphical, loge transformed). Validation measurements by GC-MS from this study and published estimates for isoprene (I) and monoterpenes (M) from a previous study at the same site are provided for shared species. Genus level data from literature is provided in units of proportion of measured species that emit isoprene (pIE) along with the number of species measured (N spp.). Numbered references: [1] Present study GC-MS validation measurements; [2] Bracho-Nunez et al. (2013); [3] Geron et al. (2002); [4] Harley et al. (2004); [5] Jardine et al. (2020a); [6] Klinger et al. (2002); [7] Klinger et al. (1998); [8] Taylor et al. (2018); [9] Varshney and Singh (2003).
This emissions inventory increases tropical species coverage by approximately 10% relative to the literature compilation by Taylor et al. (2018). Such inventory datasets contribute to research on the phylogenetic distribution of VI emissions (Harley et al., 1999; Monson et al., 2013), and can be used to refine the ‘emitter fractions’ (proportion of emitting leaf area in a forest) used in emissions and atmospheric chemistry models (Geron et al., 1994; Guenther, 1997). The ability to monitor sensor drift and leaf emission dynamics in real time, and to immediately process emission data without waiting for lab results, allowed the informed adjustment of sampling efforts in the field to ensure adequate coverage of sampling targets and the resolution of any questionable results. We observed detectable (>0.4 nmol m–2 s–1), light-dependent emissions from 67% of sampled species. It is likely that emission rates lower than ca. 1 nmol m–2 s–1 comprise a diversity of compounds, some of which may be passively released by rising temperature when the leaf is illuminated (Guenther et al., 1995; Laothawornkitkul et al., 2009). However, measurements subsequent to this campaign have shown that careful measurement of highly aromatic leaves that lack the capacity for light-dependent VI emissions (e.g., Retrophyllum, Myrcia, and Zanthoxylum spp.) do not produce detectable volatiles during a standard PORCO measurement (data not shown). Emission rates > 1 nmol m–2 s–1 are very likely to be predominantly composed of isoprene, and less commonly, smaller amounts of light-dependent monoterpenes (Keller and Lerdau, 1999; Kuhn et al., 2002; Harrison et al., 2013; Jardine et al., 2020a). We observed strong light-dependent emissions (>1 nmol m–2 s–1) from 56% of sampled species. Consistent with the findings of Taylor et al. (2018), VI emissions show some taxonomic consistency in Figure 13. Emission rates ranged from consistently less than 1 nmol m–2 s–1 in the Lauraceae family, to frequently exceeding 10 nmol m–2 s–1 in the genus Protium. The highest observed emission rate was from Marlierea spruceana, 17.4 nmol m–2 s–1.
Validation of Field Measurements
Visual analysis of processed leaf-emissions data demonstrate background signal noise to rarely exceed ± 0.2 nmol m–2 s–1. All emission curves exceeding 0.4 nmol m–2 s–1 were distinctly different from background fluctuations by 210 s of illumination (Figure 14). We therefore assign a conservative uniform detection limit of 0.4 nmol m–2 s–1. Emission estimates may subsequently be scaled below 0.4 when standardized to 30°C equivalent rates.
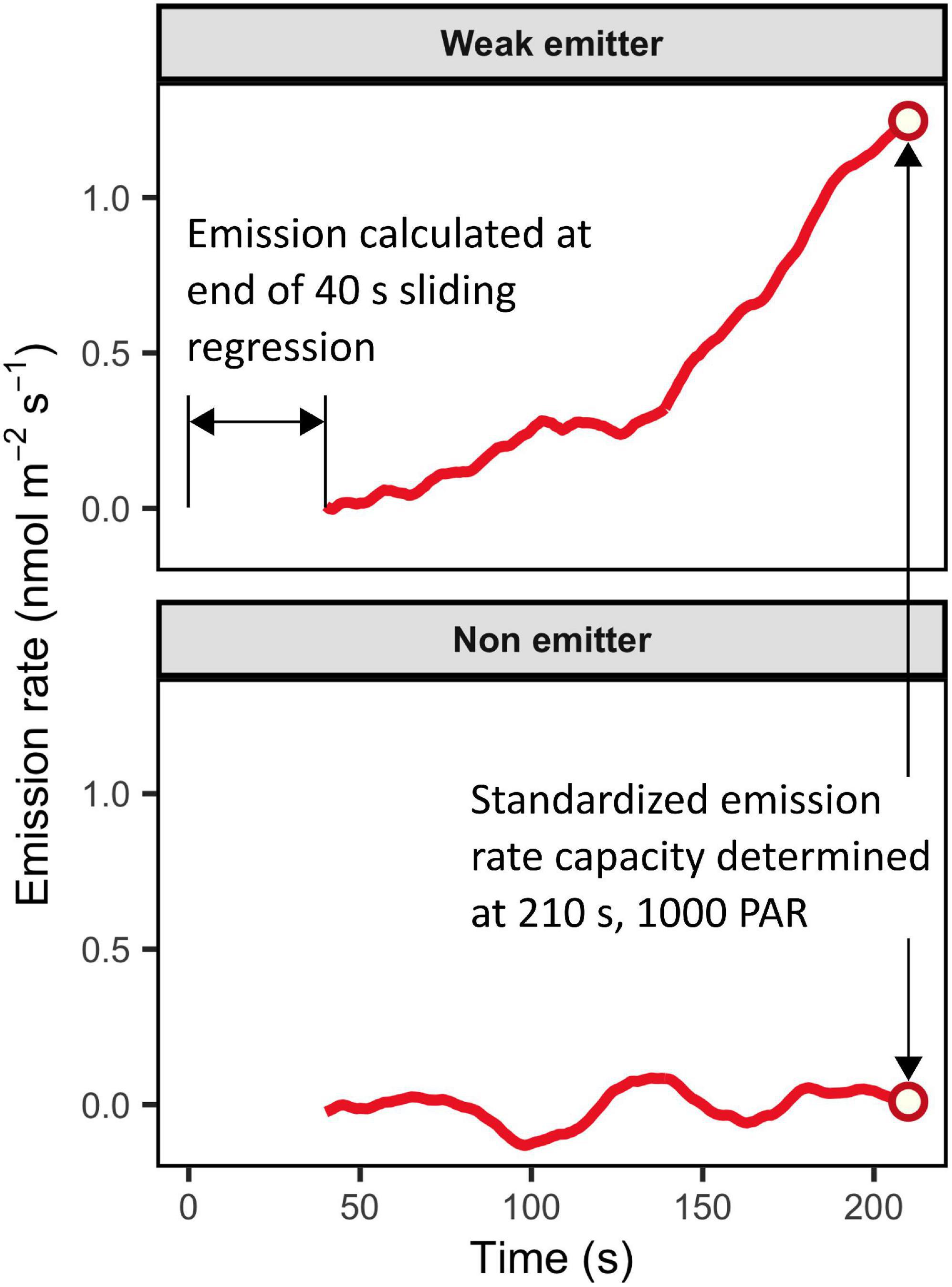
Figure 14. Leaf emission rates are calculated as non-steady-state fluxes based on concentration changes determined by a 40 s sliding-window regression. Background signal noise produces noise in emission estimates around ± 0.2 nmol m–2 s–1. Emission rates of 0.4 nmol m–2 s–1 by 210 s of measurement time are clearly differentiable from zero.
Much of the variation in emission rates can be expected to occur at the scale of individual branches (Monson and Fall, 1989; Harley et al., 1996; Funk et al., 2006), which largely determine the light and thermal microenvironment to which leaves are acclimated (Chazdon and Fetcher, 1984; Niinemets et al., 2010b). Accordingly, we observed that where within-species variation was highest (Figure 13), species data was amalgamated from measurements of multiple distinct trees or branches from different light environments. We tested whether PORCO measurements are sufficiently consistent to detect ecologically driven variation at the branch scale, as distinguished from leaf level variation and detection precision. We found that between-branch variation (standard deviation) in mean emission rates exceeded within-branch (between-leaf) variation by a factor of 2.8 (t-test, p << 0.001, Supplementary Figure 10), demonstrating that PORCO is able to detect ecologically driven variation in leaf emission rates.
Emission measurements using PORCO show good agreement with other data sources for the same species and genera (Figure 13). Independent data was available at the species-level for 11 of our measured species. These included our own validation measurements from several of the same individual trees (ref. [1] in Figure 13), in which leaves were acclimated to 1000 PAR and 30°C until photosynthetic stability was achieved (approximately 2 min) in a Walz photosynthesis chamber head (Heinz Walz GmbH, Effeltrich, Germany), followed by 10 min of subsampling cuvette gas onto adsorbent cartridges for subsequent analysis by GC-MS, similar to the methods of Jardine et al. (2015). Seven of our measured species were previously measured at the same site by Harley et al. (2004), using a combination of controlled and uncontrolled cuvettes for sampling onto adsorbent cartridges and analysis by gas chromatography. PORCO measurements agreed with independent data sources for all 11 shared species in terms of detectable emissions (six species) versus emissions below detection limit (BDL, five species), except E. uncinatum, reported as BDL by Harley et al. (2004), but confirmed as an emitter by independent measurements on multiple individuals during this campaign.
Quantitative emission rates derived from PORCO were correlated with our GC-MS validation measurements (linear regression on maximum emission rates from five species as reported in Figure 13: p = 0.05, r2 = 0.68, Supplementary Figure 11), though PORCO estimates were lower. Due to the short duration of measurements beginning with dark-acclimated leaves, emissions during PORCO measurements do not reach physiological steady state (see emission curves in Figures 10, 14), and will tend to be systematically lower than those derived from longer measurements that approach steady state. PORCO measurements also agreed well with published data at the genus-level (Figure 13), obtained from a literature compilation of tropical isoprene emission measurements (Taylor et al., 2018, 2019; Supplementary Table 2). For species with weak or undetected emissions by PORCO, a low proportion of species from the corresponding genus (“congeners”) were reported as emitters in the literature. Conversely, stronger emissions corresponded to a higher proportion of congeners reported as emitters in the literature (Figure 13). Likely due to the low detection limit achieved with PORCO, we report a higher proportion of species with light-dependent VI emissions (67% above detection limit; 56% > 1 nmol m–2 s–1) compared to the inventory at the same site by Harley et al. (2004) (46% of 26 species, after correcting their reporting of E. uncinatum from non-emitter to emitter). Our proportion of detectable emissions closely matches a recent inventory of Amazonian trees based entirely on GC-MS measurements, reporting light-dependent VI emissions from 67% of species (Jardine et al., 2020a).
Of the four emitting species independently quantified by GC-MS and PORCO, two emitted only isoprene, one a mixture of isoprene and a smaller quantity of alpha-pinene (a monoterpene), and one entirely alpha-pinene (Figure 13). Because PIDs have different sensitivities to different compounds (see section “PID Sensitivities, Control, and Calibration”), we report PORCO measurements calibrated as isoprene-equivalents. The relative responsivity of the ppbRAE 3000 PID to alpha-pinene is reported to be twice that of isoprene (Rae Systems Inc, 2013c). PORCO is therefore expected to overestimate total VI emissions in proportion to the ratio of monoterpenes to isoprene in the sample. Although we detected monoterpenes from two out of five species measured by GC-MS, light-dependent monoterpene emissions are uncommon from tropical trees relative to isoprene, and are most commonly emitted at rates one-to-two orders of magnitude lower than isoprene (Supplementary Figure 12) (Kesselmeier and Staudt, 1999; Jardine et al., 2020a). Moreover, light-dependent monoterpene emissions are evidenced to play a similar role to isoprene in the leaf, providing an advantage to detecting both compound classes in ecological studies (Copolovici et al., 2005; Vickers et al., 2009a). See the “Discussion” section for an expansion on the expected biases and advantages resulting from combined detection of isoprene and monoterpenes.
Results
Unexpected Vertical Profile of Emission Capacities in a Tropical Forest
If VI emissions are linked to adaptations for leaf thermal tolerance (Sharkey et al., 2008; Vickers et al., 2009a; Taylor et al., 2019), then species with high emission capacities can be expected to congregate in the upper canopy where light availability and solar heating of leaves is greatest (Niinemets et al., 2010a). Results from our field inventory of tropical trees reveal an unexpected vertical distribution, with the highest emission capacities (Figure 15A) and the greatest proportion of emitting species (Figure 15B) found in the mid-canopy region, and surprisingly high emissions found among the smallest trees. We cannot attribute this trend to an over-sampling of canopy species at sub-canopy positions, because we sampled most trees at sizes within the maximum size class for their species. For example, the highest emission rates were observed among mid-canopy specialist groups Protium, Myristicaceae, and Myrtaceae, while low to moderate emissions were observed from the canopy dominants Erisma and Tachigali (Figure 13). Two species in the Violaceae family showed consistently moderate emissions, and they are exclusively understory specialists, never exceeding 10 cm diameter and often found in fully shaded environments. Our interpretation of the vertical profile of emission capacities (Figure 15A) requires that the typical preponderance of isoprene over monoterpenes does not systematically vary across the profile, affecting the scaling of emissions detected by PID. While we detected monoterpenes in upper-canopy trees and may therefore slightly overestimate emissions there, we know of no systematic studies across canopy depths, nor theoretical support for a shift in emission compositions across microenvironments. Such variation in emission compositions would have little affect on our estimates of the proportion of species that emit significant VI across the profile (Figure 15B).
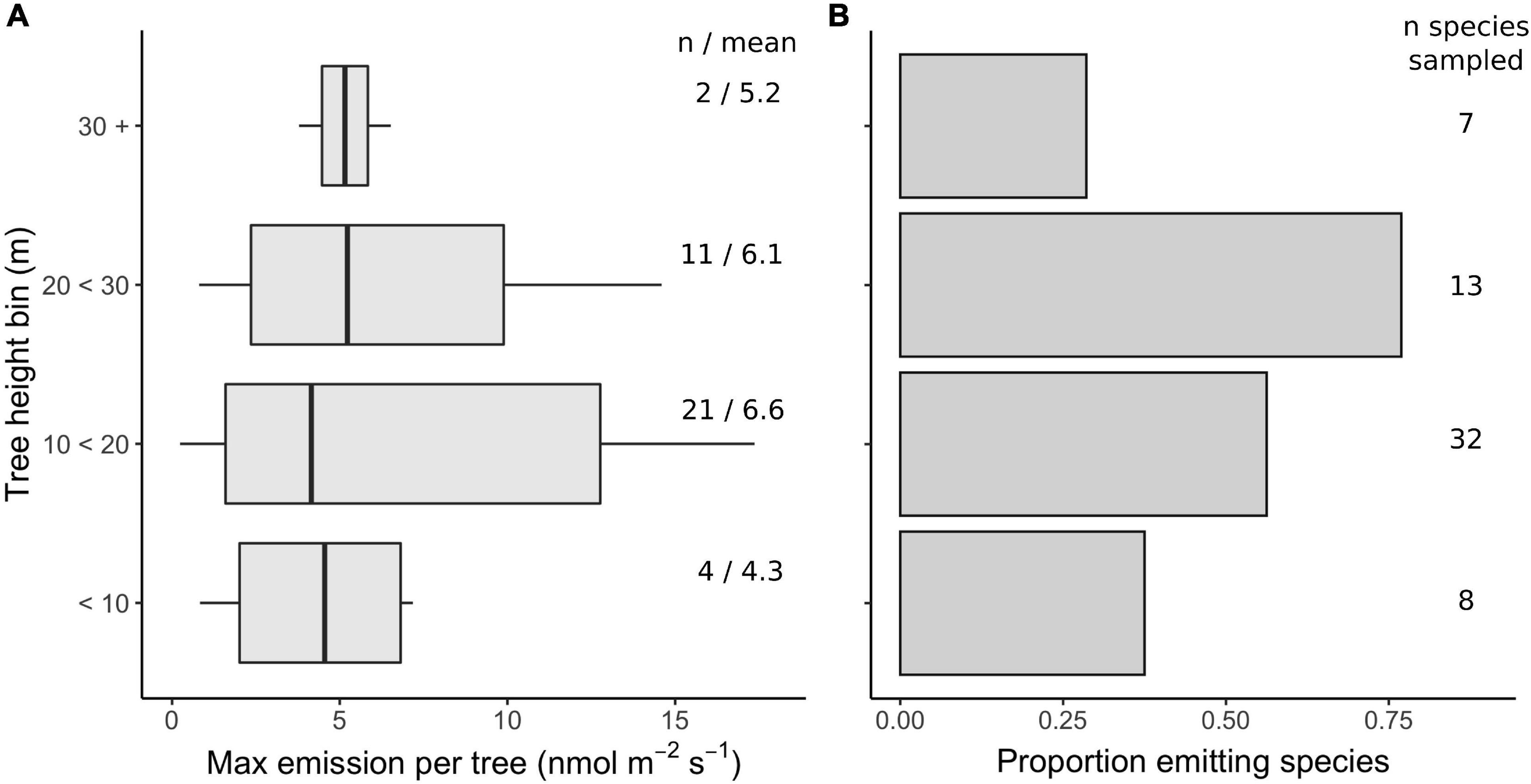
Figure 15. Isoprenoid emission capacities peaked in the mid-canopy among strongly emitting species (those with maximum emission rates > 1 nmol m–2 s–1). Boxplots in (A) show the highest detectable leaf-level emission rate measured per tree among trees binned by height (typically 1–3 leaves measured per tree, standardized at 3.5 min, 1000 PAR, and 30°C). Strong emissions are even observed in the understory. Bars in (B) show the proportion of species with emission rates > 1 nmol m–2 s–1, binned by the maximum tree height among measured individuals of each species.
This vertical distribution of emission capacities suggests a more nuanced view of the adaptive significance of VI emissions for tropical trees. As an on-demand trait, VI emission may be adaptive for temporally dynamic thermal environments (Sharkey et al., 2008), rather than chronically hot ones. In the mid-canopy, leaves experience alternating shade and direct sun exposure as the sun traverses overhead canopy material and gaps. This view presents a challenge for modeling emissions from tropical forests. Forest emissions will depend on the influence of forest structure on dynamic sub-canopy light environments (Niinemets et al., 2010a; Way and Pearcy, 2012; Smith et al., 2019), and cannot be represented as simple steady-state responses to canopy surface conditions. It is worth further exploring how such subtleties in the ecological context of emissions relate to other emerging, nuanced views of the role of VI in dynamic regulation of gene expression (Lantz et al., 2019b; Zuo et al., 2019) and growth-defense tradeoffs in species’ ecological strategies (Monson et al., 2021).
Discussion
Contrasting PORCO to Commercial Instruments: Advantages and Limitations
PORCO adaptations enable unprecedented in situ detection of leaf volatile emissions with the precision and detection limits required to resolve physiological and ecological drivers of VI emissions from terrestrial vegetation. Compared to other in situ sampling methods, PORCO fills a unique niche with its particular balance of detection capabilities. The ideal detection system would feature: portability; online (real-time) data detection and display; precision detection with low detection limits; leaf environmental control; and the capability to distinguish between gas species. The sections above demonstrate PORCO’s portability and quantify the precision of its online detection capabilities. Its partial leaf environmental control and non-distinguishing sensor represent tradeoffs relative to other methods.
Both precision online detection and compound differentiation can be achieved with a proton transfer reaction mass spectrometer (PTR-MS), but this system is very difficult to mobilize to the field, requiring an improvised laboratory setting (e.g., Alves et al., 2016; Sarkar et al., 2020). The Fast Isoprene Sensor (Guenther and Hills, 1998; Hanson and Sharkey, 2001) is more robust and portable than a PTR-MS, and provides online detection of isoprene specifically, but requires AC power and large oxygen tanks to generate ozone for the sensor reaction chamber. PIDs used ‘out of the box’ (not adapted) provide portable online detection, but have poor detection limits due to low signal:noise ratios. Such use requires large, environmentally uncontrollable cuvettes that can enclose many leaves in order to obtain high gas concentrations and overcome sensor noise. The best alternative method currently is to use small adsorbent cartridges to sample gas from the exhaust of commercial leaf cuvettes of photosynthesis instruments that have excellent control of leaf environmental conditions, including light, temperature, CO2, and humidity (e.g., Jardine et al., 2020a, b). Drawbacks include offline detection (samples are sent to a mass spectrometry lab for analysis), risk of signal degradation during sample transport, expense of cartridges (several hundred USD each), and the limitation of sample sizes to available cartridges and analysis turnaround times.
PORCO leaf cuvettes optimize detection precision and lower-limits, while providing precise light control. However, tight temperature control in larger cuvettes is a challenge. The LED panels warm the air by about 4°C above ambient by 3.5 min. PORCO can alternatively sample from commercial leaf cuvettes, combining precise emission detection with better leaf environmental control (see section “PORCO-PID validation against Fast Isoprene Sensor”). Drawbacks include an increase in LDL (see section “Cuvette design”), and the need to obtain, carry, and maintain a second instrument system in the field. The advantages of PORCO can be combined with an ability to distinguish compounds by simultaneously sampling onto adsorbent cartridges from the partially ionized PID exhaust (see Supplementary Figure 3), or separately from the leaf cuvettes.
Combined Detection of Isoprene and Monoterpenes
Detection of the dominant light-dependent VI—isoprene and monoterpenes—in a combined signal represents a small source of ambiguity in measurements while at the same time offering certain advantages. Consider first that the uncertainty in the VI emission potential of an unmeasured tree spans three-to-four orders of magnitude (from tenths to tens or >100 nmol m–2 s–1). The PORCO PID is twice as responsive to the most commonly detected monoterpene species (alpha-pinene) compared to isoprene (see section “Isolation of Isoprene and Monoterpenes in the PID Signal”), such that a VI emission rate estimated by PORCO could be overestimated by a maximum of a factor of two in the extreme case that the gas exclusively contains monoterpenes. However, evidence and known mechanisms support the expectation that monoterpenes are rare in light-dependent emissions. A survey of 113 Amazonian tree species found that 62% emitted isoprene, 15% emitted monoterpenes, and only 5% were exclusive monoterpene emitters (Jardine et al., 2020a). Where present, monoterpene emission rates from tropical trees tend to be one-to-two orders of magnitude lower than isoprene emission rates (see Supplementary Figure 12), most often <1 nmol m–2 s–1 (Kesselmeier and Staudt, 1999; Klinger et al., 2002; Kuhn et al., 2002; Jardine et al., 2020a). Atmospheric observations support the dominance of isoprene emissions from tropical forests (Yanez-Serrano et al., 2015; Alves et al., 2016; Sarkar et al., 2020) and worldwide (Guenther, 2013). The relative rarity of light-dependent monoterpenes can be attributed in part to their metabolic cost—while isoprene (5C) is a simple transformation of its five-carbon precursor, each monoterpene molecule (10C) is composed of more complex derivations of two of the same precursor, costing twice the carbon and more than twice the reducing power of isoprene (Gershenzon, 1994). VI emission estimates from PORCO are therefore most commonly a precise representation of isoprene emission rates, and where gas mixtures are present, represent an overestimate of total VI by a factor typically much less than two. This is an acceptable range of confidence given the uncertainty range of three-to-four orders of magnitude for an unmeasured tree, the paucity of field measurements from remote forests, and the limitations to field deployment of currently available gas-distinguishing methods. While we focus here on tropical forests, the application of PORCO to other systems requires confirmation of the composition and consistency of emitted gasses. The interpretation of PORCO measurements can be further refined by future studies that seek to determine the taxonomic groups and environments within which light dependent monoterpene emissions reach rates comparable to isoprene.
Detecting total light-dependent VI offers some advantages over isoprene-specific methods. Light-dependent monoterpene emissions are derived from the same metabolic pathway as isoprene and are likely to play a similar physiological role in the leaf. Alpha-pinene has been shown to enhance photosynthetic thermal tolerance in a manner similar to isoprene (Copolovici et al., 2005). Similar to recent conclusions about isoprene (Zuo et al., 2019; Monson et al., 2021), emerging evidence suggests that monoterpenes act as signaling mechanisms to coordinate cellular responses to abiotic and biotic stresses (Riedlmeier et al., 2017; Frank et al., 2021). Due to their similar metabolic dependencies, forest emissions of light-dependent VI can be modeled with a single function based on photosynthetic electron transport rates, while applying a simple fractionation to apportion into the prominent gas species (Hantson et al., 2017). The fact that PORCO does not fail to detect monoterpene emissions when they occur can be an advantage for informing VI-based ecophysiological adaptations and emissions modeling.
Accessing New Frontiers in the Ecology and Ecophysiology of Plant Volatiles
Despite the well demonstrated critical roles of volatile isoprenoid emissions in plant physiology and atmospheric chemistry, the field research required to bridge the scales from leaf to atmosphere has been limited by a lack of adequate field instrumentation. The adaptations presented in PORCO resolve key limitations, allowing an increased rate of species sampling and the in situ measurement precision required to address important but previously inaccessible questions in ecology, evolution, and atmospheric science. For example, modeling forest emissions requires that we understand the landscape distributions of emitting species (Guenther, 1997; Sharkey and Monson, 2014). The present and future distributions of heat-tolerant emitters may also be a key determinant of the sensitivity of forest carbon uptake to climate warming (Feeley et al., 2020; Smith et al., 2020). Limited data suggests that the enhanced thermal tolerance of isoprene emitting species shapes their distributions across tropical landscapes and through time (Taylor et al., 2019), but the quantified uncertainty in emitter distributions is high due to limited species sampling (Taylor et al., 2018). A conspicuous loss of emitters toward dry climates (Klinger et al., 1998; Taylor et al., 2018) remains enigmatic, and may be linked to unexplored coordination between emissions and leaf carbon-economic and hydraulic strategies (Taylor et al., 2018).
Field campaigns will enable targeted hypothesis testing, and species inventories such as reported here (Figure 13) increase the potential to correlate emissions with other published datasets describing species thermal tolerance strategies and other ecophysiological traits (Wright et al., 2004, 2010; Perez et al., 2018; Perez and Feeley, 2020) or demographic responses to climate (Enquist and Enquist, 2011; Duque et al., 2015; Fadrique et al., 2018). While the diversity of tropical forests is overwhelming (Slik et al., 2015), targeted sampling can resolve the phylogenetic (evolutionary) distribution of emissions among species (Harley et al., 1999; Monson et al., 2013), which will allow for more informed inference of unmeasured species (Taylor et al., 2018). The vertical profile of emission capacities presented here suggests that canopy structure may mediate forest emissions by determining spatiotemporal variation in light environments of the middle and low canopy (e.g., Smith et al., 2019). The generality of this vertical distribution and the mechanisms driving it should be explored further. PORCO has the portability and speed of online detection required to observe how emissions respond to temporally dynamic sun exposure beneath the canopy surface. The ease of deployment of PORCO can enable repeat measurements to disentangle the effects of leaf age and environment (Niinemets et al., 2010b; Alves et al., 2014) that contribute to a ‘cryptic phenology’ of emissions from evergreen forests (Alves et al., 2016, 2018; Albert et al., 2019). Understanding emission responses to leaf age, macro and microenvironmental variation, and the mechanisms underpinning species distributions will aid in scaling efforts based on physiological emissions models (Unger et al., 2013; Morfopoulos et al., 2014) and remote sensing (Zheng et al., 2015, 2017) in order to better resolve the role of emissions in biosphere-anthroposphere-climate interactions (Unger et al., 2017).
PORCO adaptations could also benefit applied research. VI-emitting crops may be more resistant to drought and ozone, but at a cost to growth rate (Vickers et al., 2009b; Ryan et al., 2014). With the efficient field sampling enabled by PORCO, crop strains could be monitored for selection of optimum emission rates. The emission rates of urban trees can be monitored to understand their impacts on air quality for human health (Wang et al., 2013; Montoya et al., 2020; Purser et al., 2020; Zheng et al., 2020). Our new system brings the laboratory to the leaf, opening a new frontier in research toward a mechanistic understanding of volatile isoprenoid emissions from plants.
Data Availability Statement
The original contributions presented in the study are included in the article/Supplementary Materials, further inquiries can be directed to the corresponding author/s.
Ethics Statement
Written informed consent was obtained from the individual(s) for the publication of any potentially identifiable images or data included in this article.
Author Contributions
TT designed and built the instrument, carried out the fieldwork, performed the data analyses, and wrote the manuscript. WW advised on electrical components and engineering techniques during instrument development. EA assisted in fieldwork and provided the field validation measurements via cartridge sampling and GC-MS analysis. RO facilitated field access, field data access, and plant specimen identification and storage. SS was the first-author’s primary advisor during instrument development and fieldwork and advised in all aspects of the project. All the authors contributed to manuscript revision, read, and approved the submitted version.
Funding
Financial support for this study was provided to TT, WW, and SS by grant NSF-PIRE #OISE-0730305 and the DOE GoAmazon project award #DE-SC0008383 and TT by NSF grant #NSF-PRFB-1711997, and #1754163.
Conflict of Interest
The authors declare that the research was conducted in the absence of any commercial or financial relationships that could be construed as a potential conflict of interest.
Acknowledgments
We thank Kolby Jardine for advising in the early development stages; Steve Bissel for engineering support; Russel K. Monson for advising and access to validation instrumentation; the botanical personnel at Herbario-IAN, Embrapa, in Belém, PA, Brazil, for identifying our plant specimens free of charge to support TT’s dissertation research; Tiago Martins for his dedicated, reliable help in the field; Mick Eltringham and Neill Prohaska for canopy access assistance; Tucson Store Fixtures for careful construction of the leaf cuvettes according to our designs; the University of Arizona’s Biosphere 2 facility; Professor Julio Tota at the Universidade Federal do Oeste do Pará, Brazil for laboratory space during instrument development. Parts of this article content were published online previously in the Ph.D. dissertation of TT (Taylor, 2017). The submitted form of this article was posted as a pre-print on bioRxiv.
Supplementary Material
The Supplementary Material for this article can be found online at: https://www.frontiersin.org/articles/10.3389/ffgc.2021.668228/full#supplementary-material
References
Albert, L. P., Restrepo-Coupe, N., Smith, M. N., Wu, J., Bryant, C. C., Prohaska, N., et al. (2019). Cryptic phenology in plants: case studies, implications, and recommendations. Global Change Biol. 25, 3591–3608.
Alves, E. G., Harley, P., Gonçalves, J. F. D. C., Eduardo, C., and Jardine, K. (2014). Effects of light and temperature on isoprene emission at different leaf developmental stages of Eschweilera coriacea in central Amazon. Acta Amazonica 44, 9–18.
Alves, E. G., Jardine, K., Tota, J., Jardine, A., Maria Yãnez-Serrano, A., Karl, T., et al. (2016). Seasonality of isoprenoid emissions from a primary rainforest in central Amazonia. Atmospheric Chem. Phys. 16, 3903–3925.
Alves, E. G., Tóta, J., Turnipseed, A., Guenther, A. B., Bustillos, J. O. W. V., Santana, R. A., et al. (2018). Leaf phenology as one important driver of seasonal changes in isoprene emission in central Amazonia. Biogeosciences 15, 4–5.
Behnke, K., Ehlting, B., Teuber, M., Bauerfeind, M., Louis, S., Hänsch, R., et al. (2007). Transgenic, non-isoprene emitting poplars don’t like it hot. Plant J. 51, 485–499. doi: 10.1111/j.1365-313X.2007.03157.x
Boyle, B., Hopkins, N., Lu, Z., Raygoza Garay, J. A., Mozzherin, D., Rees, T., et al. (2013). The taxonomic name resolution service: an online tool for automated standardization of plant names. BMC Bioinformatics 14:16. doi: 10.1186/1471-2105-14-16
Bracho-Nunez, A., Knothe, N. M., Welter, S., Staudt, M., Costa, W. R., Liberato, M. A. R., et al. (2013). Leaf level emissions of volatile organic compounds (VOC) from some Amazonian and Mediterranean plants. Biogeosciences 10, 5855–5873. doi: 10.5194/bg-10-5855-2013
Carslaw, K. S., Boucher, O., Spracklen, D. V., Mann, G. W., Rae, J. G. L., Woodward, S., et al. (2010). A review of natural aerosol interactions and feedbacks within the Earth system. Atmospheric Chem. Phys. 10, 1701–1737. doi: 10.5194/acp-10-1701-2010
Carslaw, K. S., Lee, L. A., Reddington, C. L., Pringle, K. J., Rap, A., Forster, P. M., et al. (2013). Large contribution of natural aerosols to uncertainty in indirect forcing. Nature 503, 67–71. doi: 10.1038/nature12674
Chazdon, R. L., and Fetcher, N. (1984). Photosynthetic light environments in a lowland tropical rain forest in costa rica. J. Ecol. 72, 553–564. doi: 10.2307/2260066
Copolovici, L. O., Filella, I., Llusià, J., and Niinemets, Ü, and Peñuelas, J. (2005). The capacity for thermal protection of photosynthetic electron transport varies for different monoterpenes in Quercus ilex. Plant Physiol. 139, 485–496. doi: 10.1104/pp.105.065995
Duque, A., Stevenson, P. R., and Feeley, K. J. (2015). Thermophilization of adult and juvenile tree communities in the northern tropical Andes. Proc. Natl. Acad. Sci. U S A. 112, 10744–10749. doi: 10.1073/pnas.1506570112
Enquist, B. J., and Enquist, C. A. F. (2011). Long-term change within a Neotropical forest: assessing differential functional and floristic responses to disturbance and drought. Global Change Biol. 17, 1408–1424. doi: 10.1111/j.1365-2486.2010.02326.x
Fadrique, B., Báez, S., Duque, Á, Malizia, A., Blundo, C., Carilla, J., et al. (2018). Widespread but heterogeneous responses of Andean forests to climate change. Nature 564, 207–212. doi: 10.1038/s41586-018-0715-9
Feeley, K. J., Bravo-Avila, C., Fadrique, B., Perez, T. M., and Zuleta, D. (2020). Climate-driven changes in the composition of new world plant communities. Nature Climate Change 10, 965–970. doi: 10.1038/s41558-020-0873-2
Feng, Z., Yuan, X., Fares, S., Loreto, F., Li, P., Hoshika, Y., et al. (2019). Isoprene is more affected by climate drivers than monoterpenes: a meta-analytic review on plant isoprenoid emissions. Plant Cell Environ. 42, 1939–1949. doi: 10.1111/pce.13535
Fineschi, S., Loreto, F., Staudt, M., and Peñuelas, J. (2013). “Diversification of volatile isoprenoid emissions from trees: evolutionary and ecological perspectives,” in Biology, Controls and Models of Tree Volatile Organic Compound Emissions, eds Ü Niinemets and R. K. Monson (Berlin: Springer), doi: 10.1007/978-94-007-6606-8
Fini, A., Brunetti, C., Loreto, F., Centritto, M., Ferrini, F., and Tattini, M. (2017). Isoprene responses and functions in plants challenged by environmental pressures associated to climate change. Front. Plant Sci. 8:1281. doi: 10.3389/FPLS.2017.01281
Frank, L., Wenig, M., Ghirardo, A., Krol, A., Vlot, A. C., Schnitzler, J., et al. (2021). Isoprene and β-caryophyllene confer plant resistance via different plant internal signaling pathways. Plant Cell Environ. 44, 1151–1164. doi: 10.1111/pce.14010
Funk, J. L., Giardina, C. P., Knohl, A., and Lerdau, M. T. (2006). Influence of nutrient availability, stand age, and canopy structure on isoprene flux in a Eucalyptus saligna experimental forest. J. Geophys. Res. Biogeosci. 111, 1–10. doi: 10.1029/2005JG000085
Geron, C., Daly, R., Harley, P., Rasmussen, R., Seco, R., Guenther, A., et al. (2016). Large drought-induced variations in oak leaf volatile organic compound emissions during PINOT NOIR 2012. Chemosphere 146, 8–21. doi: 10.1016/j.chemosphere.2015.11.086
Geron, C., Guenther, A., Greenberg, J., Loescher, H. W., Clark, D., and Baker, B. (2002). Biogenic volatile organic compound emissions from a lowland tropical wet forest in Costa Rica. Atmospheric Environ. 36, 3793–3802. doi: 10.1016/S1352-2310(02)00301-1
Geron, C. D., Guenther, A. B., and Pierce, T. E. (1994). An improved model for estimating emissions of volatile organic compounds from forests in the eastern United States. J. Geophys. Res. 99, 12773–12791. doi: 10.1029/94JD00246
Gershenzon, J. (1994). Metabolic costs of terpenoid accumulation in higher plants. J. Chem. Ecol. 20, 1281–1328. doi: 10.1007/BF02059810
Guenther, A. (1997). Seasonal and spatial variations in natural volatile organic compound emissions. Ecol. Appl. 7, 34–45.
Guenther, A. (2013). Biological and chemical diversity of biogenic volatile organic emissions into the atmosphere. ISRN Atmospheric Sci. 2013, 1–27. doi: 10.1155/2013/786290
Guenther, A., Hewitt, C. N., Erickson, D., Fall, R., Geron, C., Graedel, T., et al. (1995). A global model of natural volatile organic compound emissions. J. Geophys. Res. 100:8873. doi: 10.1029/94JD02950
Guenther, A. B., and Hills, A. J. (1998). Eddy covariance measurement of isoprene fluxes. J. Geophys. Res. Atmospheres 103, 13145–13152. doi: 10.1029/97JD03283
Guenther, A. B., Zimmerman, P. R., Harley, P. C., Monson, R. K., and Fall, R. (1993). Isoprene and monoterpene emission rate variability: model evaluations and sensitivity analyses. J. Geophys. Res. 98, 12609–12617.
Hanson, D. T., and Sharkey, T. D. (2001). Effect of growth conditions on isoprene emission and other thermotolerance-enhancing compounds. Plant Cell Environ. 24, 929–936. doi: 10.1046/j.1365-3040.2001.00744.x
Hantson, S., Knorr, W., Schurgers, G., Pugh, T. A. M., and Arneth, A. (2017). Global isoprene and monoterpene emissions under changing climate, vegetation, CO2 and land use. Atmospheric Environ. 155, 35–45. doi: 10.1016/j.atmosenv.2017.02.010
Harley, P., Guenther, A., and Zimmerman, P. (1996). Effects of light, temperature and canopy position on net photosynthesis and isoprene emission from sweetgum (Liquidambar styraciflua) leaves. Tree Physiol. 16, 25–32. doi: 10.1093/treephys/16.1-2.25
Harley, P., Vasconcellos, P., Vierling, L., Pinheiro, C. C. D. S., Greenberg, J., Guenther, A., et al. (2004). Variation in potential for isoprene emissions among neotropical forest sites. Global Change Biol. 10, 630–650. doi: 10.1111/j.1529-8817.2003.00760.x
Harley, P. C., Monson, R. K., and Lerdau, M. T. (1999). Ecological and evolutionary aspects of isoprene emission from plants. Oecologia 118, 109–123. doi: 10.1007/s004420050709
Harrison, S. P., Morfopoulos, C., Dani, K. G. S., Prentice, I. C., Arneth, A., Atwell, B. J., et al. (2013). Volatile isoprenoid emissions from plastid to planet. New Phytol. 197, 49–57. doi: 10.1111/nph.12021
Heald, C. L., Henze, D. K., Horowitz, L. W., Feddema, J., Lamarque, J.-F., Guenther, A., et al. (2008). Predicted change in global secondary organic aerosol concentrations in response to future climate, emissions, and land use change. J. Geophys. Res. 113:D05211. doi: 10.1029/2007JD009092
Hunt, S. (2003). Measurements of photosynthesis and respiration in plants. Physiol. Plant 117, 314–325. doi: 10.1034/j.1399-3054.2003.00055.x
Jardine, A. B., Jardine, K. J., Fuentes, J. D., Martin, S. T., Martins, G., Durgante, F., et al. (2015). Highly reactive light-dependent monoterpenes in the Amazon. Geophys. Res. Lett. 42, 1576–1583. doi: 10.1002/2014GL062573
Jardine, K., Yañez Serrano, A., Arneth, A., Abrell, L., Jardine, A., Artaxo, P., et al. (2011). Ecosystem-scale compensation points of formic and acetic acid in the central Amazon. Biogeosciences 8, 3709–3720. doi: 10.5194/bg-8-3709-2011
Jardine, K. J., Zorzanelli, R. F., Gimenez, B. O., Oliveira Piva, L. R., de, Teixeira, A., et al. (2020a). Leaf isoprene and monoterpene emission distribution across hyperdominant tree genera in the Amazon basin. Phytochemistry 175:112366. doi: 10.1016/j.phytochem.2020.112366
Jardine, K. J., Zorzanelli, R. F., Gimenez, B. O., Robles, E., and de Oliveira Piva, L. R. (2020b). Development of a portable leaf photosynthesis and volatile organic compounds emission system. MethodsX 7:100880. doi: 10.1016/j.mex.2020.100880
Keller, M., and Lerdau, M. (1999). Isoprene emission from tropical forest canopy leaves. Global Biogeochem. Cycles 13, 19–29. doi: 10.1029/1998GB900007
Kesselmeier, J. (2001). Exchange of short-chain oxygenated volatile organic compounds (VOCs) between plants and the atmosphere: a compilation of field and laboratory studies. J. Atmospheric Chem. 39, 219–233. doi: 10.1023/A:10632302076
Kesselmeier, J., and Staudt, M. (1999). Biogenic Volatile Organic Compunds (VOC): an overview on emission, physiology and ecology. J. Atmospheric Chem. 33, 23–88. doi: 10.1023/A:1006127516791
Kim, S., Chen, J., Cheng, T., Gindulyte, A., He, J., He, S., et al. (2020a). PubChem in 2021: new data content and improved web interfaces. Nucleic Acids Res. 49, 1388–1395. doi: 10.1093/nar/gkaa971
Kim, S.-Y., Kim, J.-C., Park, C.-R., and Son, Y.-S. (2020b). Emission characteristics of biogenic volatile organic compounds from representative plant species of the Korean peninsula – focused on aldehydes. Atmospheric Res. 236:104840. doi: 10.1016/j.atmosres.2020.104840
Klinger, L. F., Greenberg, J., Guenther, A., Zimmerman, P., M’Bangui, M., and Kenfack, D. (1998). Patterns in volatile organic compound emissions along a savanna-rainforest gradient in central Africa. J. Geophys. Res. 103, 1443–1454. doi: 10.1029/97JD02928
Klinger, L. F., Li, Q. J., Guenther, A. B., Greenberg, J. P., Baker, B., and Bai, J. H. (2002). Assessment of volatile organic compound emissions from ecosystems of China. J. Geophys. Res. Atmospheres 107, 4603–4624. doi: 10.1029/2001JD001076
Kuhn, U., Rottenberger, S., Biesenthal, T., Wolf, A., Schebeske, G., Ciccioli, P., et al. (2002). Isoprene and monoterpene emissions of Amazônian tree species during the wet season: direct and indirect investigations on controlling environmental functions. J. Geophys. Res. 107, 1–13. doi: 10.1029/2001JD000978
Lantz, A. T., Allman, J., Weraduwage, S. M., and Sharkey, T. D. (2019a). Isoprene: new insights into the control of emission and mediation of stress tolerance by gene expression. Plant Cell Environ. 42, 2808–2826. doi: 10.1111/pce.13629
Lantz, A. T., Solomon, C., Gog, L., McClain, A. M., Weraduwage, S. M., Cruz, J. A., et al. (2019b). Isoprene suppression by CO2 is not due to triose phosphate utilization (TPU) limitation. Front. Forests Global Change 2:8. doi: 10.3389/ffgc.2019.00008
Laothawornkitkul, J., Taylor, J. E., Paul, N. D., and Hewitt, C. N. (2009). Biogenic volatile organic compounds in the Earth system: tansley review. New Phytol. 183, 27–51. doi: 10.1111/j.1469-8137.2009.02859.x
Li, Z., and Sharkey, T. D. (2013). Metabolic profiling of the methylerythritol phosphate pathway reveals the source of post-illumination isoprene burst from leaves. Plant, Cell Environ. 36, 429–437. doi: 10.1111/j.1365-3040.2012.02584.x
Martins, M. A. R., Silva, L. P., Ferreira, O., Schröder, B., Coutinho, J. A. P., and Pinho, S. P. (2017). Terpenes solubility in water and their environmental distribution. J. Mol. Liquids 241, 996–1002. doi: 10.1016/j.molliq.2017.06.099
Monson, R. K., and Fall, R. (1989). Isoprene emission from aspen leaves: influence of environment and relation to photosynthesis and photorespiration. Plant Physiol. 90, 267–274. doi: 10.1104/pp.90.1.267
Monson, R. K., Jones, R. T., Rosenstiel, T. N., and Schnitzler, J. P. (2013). Why only some plants emit isoprene. Plant Cell Environ. 36, 503–516. doi: 10.1111/pce.12015
Monson, R. K., Lerdau, M. T., Sharkey, T. D., Schimel, D. S., and Fall, R. (1995). Biological aspects of constructing volatile organic-compound emission inventories. Atmospheric Environ. 29, 2989–3002. doi: 10.1016/1352-2310(94)00360-W
Monson, R. K., Neice, A. A., Trahan, N. A., Shiach, I., McCorkel, J. T., and Moore, D. J. P. (2016). Interactions between temperature and intercellular CO2 concentration in controlling leaf isoprene emission rates. Plant Cell Environ. 39, 2404–2413. doi: 10.1111/pce.12787
Monson, R. K., Weraduwage, S. M., Rosenkranz, M., Schnitzler, J. P., and Sharkey, T. D. (2021). Leaf isoprene emission as a trait that mediates the growth-defense tradeoff in the face of climate stress. Oecologia doi: 10.1007/s00442-020-04813-7
Montoya, O. L. Q., Niño-Ruiz, E. D., and Pinel, N. (2020). On the mathematical modelling and data assimilation for air pollution assessment in the Tropical Andes. Environ. Sci. Poll. Res. 27, 35993–36012. doi: 10.1007/s11356-020-08268-4
Morfopoulos, C., Sperlich, D., Peñuelas, J., Filella, I., Llusià, J., Medlyn, B. E., et al. (2014). A model of plant isoprene emission based on available reducing power captures responses to atmospheric CO2. New Phytol. 203, 125–139. doi: 10.1111/nph.12770
Niinemets, Ü, Arneth, A., Kuhn, U., Monson, R. K., Peñuelas, J., and Staudt, M. (2010a). The emission factor of volatile isoprenoids: stress, acclimation, and developmental responses. Biogeosciences 7, 2203–2223. doi: 10.5194/bg-7-2203-2010
Niinemets, Ü, Copolovici, L., and Hüve, K. (2010b). High within-canopy variation in isoprene emission potentials in temperate trees: implications for predicting canopy-scale isoprene fluxes. J. Geophys. Res. Biogeosci. 115, 1–19. doi: 10.1029/2010JG001436
Niinemets, Ü, Kuhn, U., Harley, P. C., Staudt, M., Arneth, A., Cescatti, A., et al. (2011). Estimations of isoprenoid emission capacity from enclosure studies: measurements, data processing, quality and standardized measurement protocols. Biogeosciences 8, 2209–2246. doi: 10.5194/bg-8-2209-2011
Padhy, P. K., and Varshney, C. K. (2005). Isoprene emission from tropical tree species. Environ. Pollut. 135, 101–109. doi: 10.1016/j.envpol.2004.10.003
Perez, T. M., and Feeley, K. J. (2020). Photosynthetic heat tolerances and extreme leaf temperatures. Funct. Ecol. 34, 2236–2245. doi: 10.1111/1365-2435.13658
Perez, T. M., Valverde-barrantes, O., Bravo, C., Hogan, J. A., Pardo, C. J., Taylor, T. C., et al. (2018). Botanic gardens are an untapped resource for studying the functional ecology of tropical plants. Philos. Trans. R. Soc. Lond. B Biol. Sci. 374:20170390. doi: 10.1098/rstb.2017.0390
Purser, G., Drewer, J., Heal, M. R., Sircus, R. A. S., Dunn, L. K., and Morison, J. I. L. (2021). Isoprene and monoterpene emissions from alder, aspen and spruce short-rotation forest plantations in the United Kingdom. Biogeosciences 18, 2487–2510.
Purser, G., Heal, M. R., White, S., Morison, J. I. L., and Drewer, J. (2020). Differences in isoprene and monoterpene emissions from cold-tolerant eucalypt species grown in the UK. Atmospheric Pollut. Res. 11, 2011–2021. doi: 10.1016/j.apr.2020.07.022
R Core Team (2020). R: A Language and Environment for Statistical Computing. Vienna: R Foundation for Statistical Computing.
Rae Systems Inc. (2013a). Technical Note TN-106 Correction Factors, Ionization Energies, and Calibration Characteristics. San Jose, CA: RAE Systems Inc.
Rae Systems Inc. (2013b). Technical Note TN-165: Combating Drift in Portable and Fixed PIDs. San Jose, CA: RAE Systems Inc.
Rasmussen, R., and Went, F. W. (1964). Volatile organic matter of plant origin in the atmosphere. Science 144:566. doi: 10.1126/science.144.3618.566-a
Rice, A. H., Pyle, E. H., Saleska, S. R., Hutyra, L., Keller, M., Camargo, P. B., et al. (2004). Carbon balance and vegetation dynamics in an old-growth amazonian forest. Ecol. Appl. 14, S55–S71. doi: 10.1890/02-6006
Riedlmeier, M., Ghirardo, A., Wenig, M., Knappe, C., Koch, K., Georgii, E., et al. (2017). Monoterpenes support systemic acquired resistance within and between plants. Plant Cell 29, 1440–1459. doi: 10.1105/tpc.16.00898
Rieksta, J., Li, T., Junker, R. R., Jepsen, J. U., Ryde, I., and Rinnan, R. (2020). Insect herbivory strongly modifies mountain birch volatile emissions. Front. Plant Sci. 11:558979. doi: 10.3389/fpls.2020.558979
Rinnan, R., Iversen, L. L., Tang, J., Vedel-Petersen, I., Schollert, M., and Schurgers, G. (2020). Separating direct and indirect effects of rising temperatures on biogenic volatile emissions in the Arctic. Proc. Natl. Acad. Sci. U S A. 117, 32476–32483. doi: 10.1073/pnas.2008901117
Ryan, A. C., Hewitt, C. N., Possell, M., Vickers, C. E., Purnell, A., Mullineaux, P. M., et al. (2014). Isoprene emission protects photosynthesis but reduces plant productivity during drought in transgenic tobacco (Nicotiana tabacum) plants. New Phytol. 201, 205–216. doi: 10.1111/nph.12477
Sanadze, G. A. (2004). Biogenic isoprene (a review). Russian J. Plant Physiol. 51, 729–741. doi: 10.1023/B:RUPP.0000047821.63354.a4
Sarkar, C., Guenther, A. B., Park, J., Seco, R., Alves, E., Batalha, S., et al. (2020). PTR-TOF-MS eddy covariance measurements of isoprene and monoterpene fluxes from an eastern Amazonian rainforest. Atmospheric Chem. Phys. 20, 7179–7191. doi: 10.5194/acp-20-7179-2020
Seco, R., Holst, T., Sillesen Matzen, M., Westergaard-Nielsen, A., Li, T., Simin, T., et al. (2020). Volatile organic compound fluxes in a subarctic peatland and lake. Atmospheric Chem. Phys. 20, 13399–13416. doi: 10.5194/acp-20-13399-2020
Sharkey, T. D., and Monson, R. K. (2014). The future of isoprene emission from leaves, canopies and landscapes. Plant Cell Environ. 37, 1727–1740. doi: 10.1111/pce.12289
Sharkey, T. D., and Monson, R. K. (2017). Isoprene research - 60 years later, the biology is still enigmatic. Plant Cell Environ. 40, 1671–1678. doi: 10.1111/pce.12930
Sharkey, T. D., Wiberley, A. E., and Donohue, A. R. (2008). Isoprene emission from plants: why and how. Ann. Bot. 101, 5–18. doi: 10.1093/aob/mcm240
Sharkey, T. D., and Yeh, S. (2001). Isoprene emission from plants. Ann. Rev. Plant Physiol. Plant Mol. Biol. 52, 407–436.
Singsaas, E. L., Lerdau, M., Winter, K., and Sharkey, T. D. (1997). Isoprene increases thermotolerance of isoprene-emitting species. Plant Physiol. 115, 1413–1420. doi: 10.1104/pp.115.4.1413
Slik, J. W. F., Arroyo-Rodriguez, V., Shin-Ichiro, A., Alvarez-loayza, P., Alves, L. F., Ashton, P., et al. (2015). An estimate of the number of tropical tree species. Proc. Natl. Acad. Sci. U S A. 112, 7472–7477. doi: 10.1073/pnas.1512611112
Smith, M. N., Stark, S. C., Taylor, T. C., Ferreira, M. L., de Oliveira, E., Restrepo-Coupe, N., et al. (2019). Seasonal and drought related changes in leaf area profiles depend on height and light environment in an Amazon forest. New Phytol. 222, 1284–1297. doi: 10.1111/nph.15726
Smith, M. N., Taylor, T. C., van Haren, J., Rosolem, R., Restrepo-Coupe, N., Adams, J., et al. (2020). Empirical evidence for resilience of tropical forest photosynthesis in a warmer world. Nat. Plants 6, 1225–1230. doi: 10.1038/s41477-020-00780-2
Tani, A., and Mochizuki, T. (2021). Review: exchanges of volatile organic compounds between terrestrial ecosystems and the atmosphere. J. Agricul. Meteorol. 77, 66–80. doi: 10.2480/agrmet.D-20-00025
Taylor, T. C. (2017). Thesis: Leaf Volatile Emissions Structure Tree Community Assembly and Mediate Climate Feedbacks in Tropical Forests. thesis, Ph.D Tucson, AZ: University of Arizona.
Taylor, T. C., Mcmahon, S. M., Smith, M. N., Boyle, B., Violle, C., Haren, J., et al. (2018). Isoprene emission structures tropical tree biogeography and community assembly responses to climate. New Phytol. 220, 435–446. doi: 10.1111/nph.15304
Taylor, T. C., Smith, M. N., Slot, M., and Feeley, K. J. (2019). The capacity to emit isoprene differentiates the photosynthetic temperature responses of tropical plant species. Plant Cell Environ. 42, 2448–2457. doi: 10.1111/pce.13564
Tholl, D., Boland, W., Hansel, A., Loreto, F., Röse, U. S. R., and Schnitzler, J. P. (2006). Practical approaches to plant volatile analysis. Plant J. 45, 540–560. doi: 10.1111/j.1365-313X.2005.02612.x
Unger, N. (2014). On the role of plant volatiles in anthropogenic global climate change. Geophys. Res. Lett. 41, 8563–8569. doi: 10.1002/2014GL061616
Unger, N., Harper, K., Zheng, Y., Kiang, N. Y., Aleinov, I., Arneth, A., et al. (2013). Photosynthesis-dependent isoprene emission from leaf to planet in a global carbon-chemistry-climate model. Atmospheric Chem. Phys. 13, 10243–10269. doi: 10.5194/acp-13-10243-2013
Unger, N., Yue, X., and Harper, K. L. (2017). Aerosol climate change effects on land ecosystem services. Faraday Discussions 200, 121–142. doi: 10.1039/c7fd00033b
Varshney, C. K., and Singh, A. P. (2003). Isoprene emission from Indian trees. J. Geophys. Res. 108:4803. doi: 10.1029/2003JD003866
Vickers, C. E., Gershenzon, J., Lerdau, M. T., and Loreto, F. (2009a). A unified mechanism of action for volatile isoprenoids in plant abiotic stress. Nat. Chem. Biol. 5, 283–291. doi: 10.1038/nchembio.158
Vickers, C. E., Possell, M., Cojocariu, C. I., Velikova, V. B., Laothawornkitkul, J., Ryan, A., et al. (2009b). Isoprene synthesis protects transgenic tobacco plants from oxidative stress. Plant Cell Environ. 32, 520–531. doi: 10.1111/j.1365-3040.2009.01946.x
Wang, J. L., Chew, C., Chang, C. Y., Liao, W. C., Lung, S. C. C., Chen, W. N., et al. (2013). Biogenic isoprene in subtropical urban settings and implications for air quality. Atmospheric Environ. 79, 369–379. doi: 10.1016/j.atmosenv.2013.06.055
Way, D. A., and Pearcy, R. W. (2012). Sunflecks in trees and forests: from photosynthetic physiology to global change biology. Tree Physiol. 32, 1066–1081. doi: 10.1093/treephys/tps064
Wright, I. J., Reich, P. B., Westoby, M., Ackerly, D. D., Baruch, Z., Bongers, F., et al. (2004). The worldwide leaf economics spectrum. Nature 428, 821–827. doi: 10.1038/nature02403
Wright, S. J., Kitajima, K., Kraft, N. J. B., Reich, P. B., Wright, I. J., Bunker, D. E., et al. (2010). Functional traits and the growth-mortality trade-off in tropical trees. Ecology 91, 3664–3674. doi: 10.1890/09-2335.1
Yáñez-serrano, A. M., Bourtsoukidis, E., Alves, E. G., Bauwens, M., Stavrakou, T., Llusià, J., et al. (2020). Amazonian biogenic volatile organic compounds under global change. Global Change Biol. 26, 4722–4751. doi: 10.1111/gcb.15185
Yanez-Serrano, A. M., Nölscher, A. C., Williams, J., Wolff, S., Alves, E., Martins, G. A., et al. (2015). Diel and seasonal changes of biogenic volatile organic compounds within and above an Amazonian rainforest. Atmos. Chem. Phys. 15, 3359–3378. doi: 10.5194/acp-15-3359-2015
Zheng, Y., Thornton, J. A., Lee, Ng, N., Cao, H., Henze, D. K., et al. (2020). Long-term observational constraints of organic aerosol dependence on inorganic species in the southeast US. Atmospheric Chem. Phys. 20, 13091–13107. doi: 10.5194/acp-20-13091-2020
Zheng, Y., Unger, N., Barkley, M. P., and Yue, X. (2015). Relationships between photosynthesis and formaldehyde as a probe of isoprene emission. Atmospheric Chem. Phys. 15, 8559–8576. doi: 10.5194/acp-15-8559-2015
Zheng, Y., Unger, N., Tadić, J. M., Seco, R., Guenther, A. B., Barkley, M. P., et al. (2017). Drought impacts on photosynthesis, isoprene emission and atmospheric formaldehyde in a mid-latitude forest. Atmospheric Environ. 167, 190–201. doi: 10.1016/j.atmosenv.2017.08.017
Keywords: isoprene, volatile organic compounds, detection, cuvette, microenvironment, ecophysiology, atmospheric chemistry, forest structure
Citation: Taylor TC, Wisniewski WT, Alves EG, Oliveira Junior RC and Saleska SR (2021) A New Field Instrument for Leaf Volatiles Reveals an Unexpected Vertical Profile of Isoprenoid Emission Capacities in a Tropical Forest. Front. For. Glob. Change 4:668228. doi: 10.3389/ffgc.2021.668228
Received: 15 February 2021; Accepted: 31 May 2021;
Published: 09 July 2021.
Edited by:
Mark Potosnak, DePaul University, United StatesReviewed by:
Roger Seco, University of Copenhagen, DenmarkMalcolm Possell, The University of Sydney, Australia
Copyright © 2021 Taylor, Wisniewski, Alves, Oliveira Junior and Saleska. This is an open-access article distributed under the terms of the Creative Commons Attribution License (CC BY). The use, distribution or reproduction in other forums is permitted, provided the original author(s) and the copyright owner(s) are credited and that the original publication in this journal is cited, in accordance with accepted academic practice. No use, distribution or reproduction is permitted which does not comply with these terms.
*Correspondence: Tyeen C. Taylor, dHllZW4udGF5bG9yQGdtYWlsLmNvbQ==