- 1Department of Sustainable Resources Management, SUNY College of Environmental Science and Forestry, Syracuse, NY, United States
- 2Department of Environmental Biology, SUNY College of Environmental Science and Forestry, Syracuse, NY, United States
- 3College of Forest Resources and Environmental Science, Michigan Technological University, Houghton, MI, United States
Trait-based analyses provide powerful tools for developing a generalizable, physiologically grounded understanding of how forest communities are responding to ongoing environmental changes. Key challenges lie in (1) selecting traits that best characterize the ecological performance of species in the community and (2) determining the degree and importance of intraspecific variability in those traits. Recent studies suggest that globally evident trait correlations (trait dimensions), such as the leaf economic spectrum, may be weak or absent at local scales. Moreover, trait-based analyses that utilize a mean value to represent a species may be misleading. Mean trait values are particularly problematic if species trait value rankings change along environmental gradients, resulting in species trait crossover. To assess how plant traits (1) covary at local spatial scales, (2) vary across the dominant environmental gradients, and (3) can be partitioned within and across taxa, we collected data on 9 traits for 13 tree species spanning the montane temperate—boreal forest ecotones of New York and northern New England. The primary dimension of the trait ordination was the leaf economic spectrum, with trait variability among species largely driven by differences between deciduous angiosperms and evergreen gymnosperms. A second dimension was related to variability in nitrogen to phosphorous levels and stem specific density. Levels of intraspecific trait variability differed considerably among traits, and was related to variation in light, climate, and tree developmental stage. However, trait rankings across species were generally conserved across these gradients and there was little evidence of species crossover. The persistence of the leaf economics spectrum in both temperate and high-elevation conifer forests suggests that ecological strategies of tree species are associated with trade-offs between resource acquisition and tolerance, and may be quantified with relatively few traits. Furthermore, the assumption that species may be represented with a single trait value may be warranted for some trait-based analyses provided traits were measured under similar light levels and climate conditions.
Introduction
One of the major challenges facing ecologists today is how to accurately model forest community dynamics, which respond to and affect ongoing, intensifying changes in the global climate system (Parmesan and Yohe, 2003; Chen et al., 2011; Gottfried et al., 2012). Trait-based ecological methods have the potential to link plant functional traits with generalizable metrics of community structure and ecosystem services (McGill et al., 2006; Suding et al., 2008; Shipley et al., 2016). A small number of plant traits have been identified as consistently correlated with ecological variation in plant physiology and performance; these traits also allow for efficient characterization of community function (Reich et al., 1997; Reich, 2014; Díaz et al., 2016). Whether and how interspecific trait covariation manifests itself locally is context-dependent and still not fully understood for many plant communities (Funk and Cornwell, 2013). Moreover, a current challenge in functional ecology is accounting for variation within species, which can reflect local adaptation and/or plastic responses to changing conditions that dictate plant performance and ecosystem function (Nicotra et al., 2010; Valladares et al., 2014). Thus, identifying locally relevant trait correlations that lead to trait dimensions and quantifying the importance and drivers of intraspecific vs. interspecific trait variability locally are two key steps to developing trait-based models of plant community response to change.
Global trait dimensions generally characterize differences in plant diversity based on different sets of correlated traits. For example, the leaf economics spectrum (LES) characterizes differences in leaves (specific leaf area, leaf lifespan, leaf nitrogen/phosphorous concentration, photosynthetic capacity, and dark respiration rate) along a trait continuum reflects a fundamental tradeoff between resource acquisition (i.e., fast growth) and conservation (i.e., slow growth) (Wright et al., 2004). A similar trait dimension for wood traits (e.g., wood density, etc.) has been suggested (i.e., the wood economic spectrum), which also balances growth against conservative attributes such as stress resistance and mechanical strength (Chave et al., 2009). Other established trait dimensions include plant size (Díaz et al., 2016) and plant branching architecture (i.e., Corner’s Rules), which weights wood growth against photosynthetic area (White, 1983; Westoby et al., 2002; Messier et al., 2017a). While empirical support for such trait dimensions is strong at the global scale, recent studies have suggested trait covariation seen globally may weaken at smaller spatiotemporal scales (Wright and Sutton-Grier, 2012; Messier et al., 2017a; Derroire et al., 2018). For example, Messier et al. (2017b) concluded that the strength of trait relationships underlying the leaf economics spectrum weaken in temperate deciduous forests due do the importance of variation in the local environment. Other studies found fine-scale covariation among plant functional traits (leaf nitrogen concentration, photosynthetic rate, and water-use efficiency) along distinct local environmental gradients (Álvarez-Yépiz et al., 2017). Thus, to properly utilize trait dimensions in future studies, their existence and strength at local scales must be investigated in greater detail.
Most trait-based models of ecological communities have also used mean trait values for each species, under the assumption that interspecific trait variation outweighs intraspecific trait variation (ITV, McGill et al., 2006; Shipley et al., 2016). However, the importance of ITV has been increasingly documented (Luo et al., 2016; Roos et al., 2019), and the degree of ITV varies considerably between functional traits (Jung et al., 2014; Burton et al., 2017). ITV may arise from a plant’s plasticity to its environment over its lifetime, or through local adaptation through successive generations, although the specific responses of plant functional traits will vary based on the relative impact of acclimation vs. adaptation (Siefert et al., 2015). For example, traits for which developmental stage is a major source of ITV may vary dramatically over the individual’s lifespan, responding in part to changing environmental variables such as light, temperature, precipitation, and wind (Lusk, 2004; Körner, 2007; Fajardo and Piper, 2011; Spasojevic et al., 2014). If different species have contrasting responses to environmental gradients or life stages, this could lead to species crossover (Figure 1); i.e., shifts in trait value rankings between species along an environmental gradient. Species crossover in turn could affect the utility of generalizing plant trait responses, and subsequently community assembly in distinct or changing environments. Despite the fact that such trait shifts may have consequences for community composition, functional diversity and ecosystem processes (Violle et al., 2012), ITV is rarely considered and often explicity removed from studies (Pérez-Harguindeguy et al., 2013).
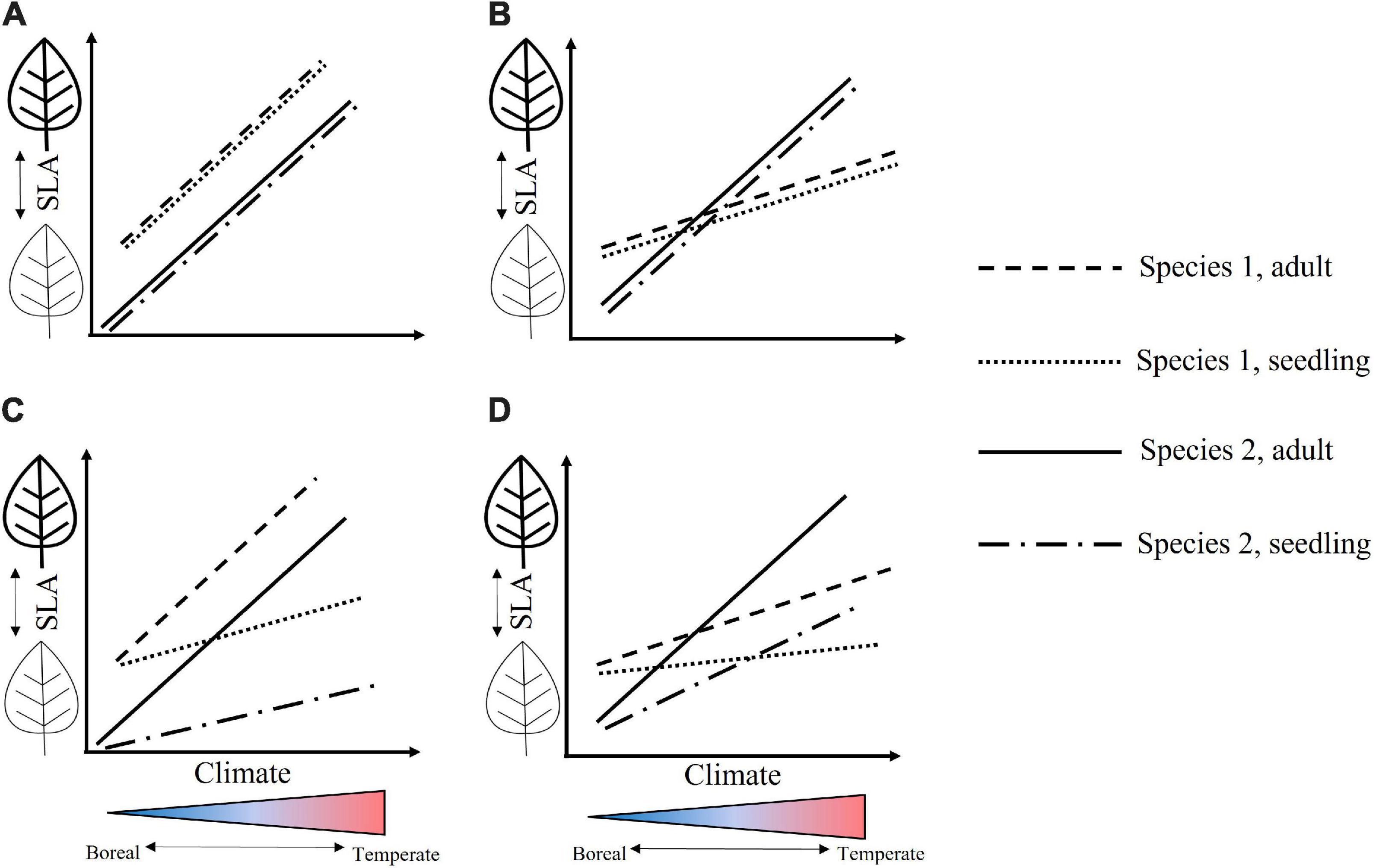
Figure 1. Hypothetical responses of specific leaf area (SLA) across an environmental gradient. In communities where species traits respond equivalently to a climate variable across species and their developmental stages, (A) mean trait values (shown as full or dashed lines) will parallel one another across the environmental gradient. (B) Species with differing responses to a climate variable (equally across life stages) may show shifts in trait rankings along an environmental gradient, or over time in a changing environment, resulting in species crossover. (C) Species whose responses are based on developmental stage may show contrasting patterns of species crossover. (D) Species may show more complex trait rank shifts if developmental stages have differing responses to a climate variable.
Major sources of ITV include environmental gradients such as temperature and light (Lusk, 2004; Albert et al., 2010; Jung et al., 2014; Burton et al., 2017), however, its impact across moderate environmental gradients has been shown to be insufficient to cause species crossover, suggesting that using a single mean value for a specific trait may be valid. Specific leaf area (SLA) and stem specific density (SSD) have previously shown lower ITV across environmental gradients of temperature and light, while leaf nitrogen and phosphorous content (LNC, LPC) have been shown to be more variable across environmental gradients (Siefert et al., 2015; Luo et al., 2016; Burton et al., 2017; Roos et al., 2019; Umaña and Swenson, 2019; Dong et al., 2020). Globally, the influence of light on leaf trait plasticity has also been understudied (Keenan and Niinemets, 2016), and within species trait-trait relationships may run counter to the LES in response to light (e.g., lower SLA with increasing light, Burton et al., 2017). For example, Anderegg et al. (2018) concluded that LES leaf trait covariation is scale dependent and supported by weak physiological tradeoffs that may be overwhelmed by intraspecific trait plasticity. Understanding how ITV of important functional traits is organized by major sources of trait variation across environmental gradients is thus vital for understanding its relevance in structuring current and future plant communities.
In this study, we measured the degree of trait covariation and the importance of intraspecific trait variation in overstory and understory communities across environmental gradients (in climate and canopy openness) spanning five mountains and four states in the northeast United States. We examined nine functional traits associated with leaf, stem, and structural trait dimensions (Table 1; Weiher et al., 1999; Wright et al., 2004; Pérez-Harguindeguy et al., 2013) and how they respond to environmental variability across a topo-climatic gradient to address the following questions: (1) Which trait dimensions are present at local scales in montane forests? (2) How is trait variation partitioned within and among tree taxa? (3) How do traits vary across large environmental gradients of temperature and canopy openness vs. growth stage (overstory trees compared to seedlings and saplings)? We then tested the following hypotheses:
H1: Three recognized trait dimensions (i.e., LES, wood economic spectrum, and architectural restraints) will be weakly represented and driven by differences between temperate and montane boreal communities.
H2: Trait variance partitioned to a taxon is negatively proportional to its scale (i.e., finer taxonomic classifications are responsible for less variation), and with the exception of leaf chemical traits, relatively little variance would be proportioned as ITV.
H3: Environmental variables will impact trait values uniformly by species, resulting in distinct shift in trait values over the measured gradients but no species crossover (i.e., changes in trait rankings across the environmental gradient).
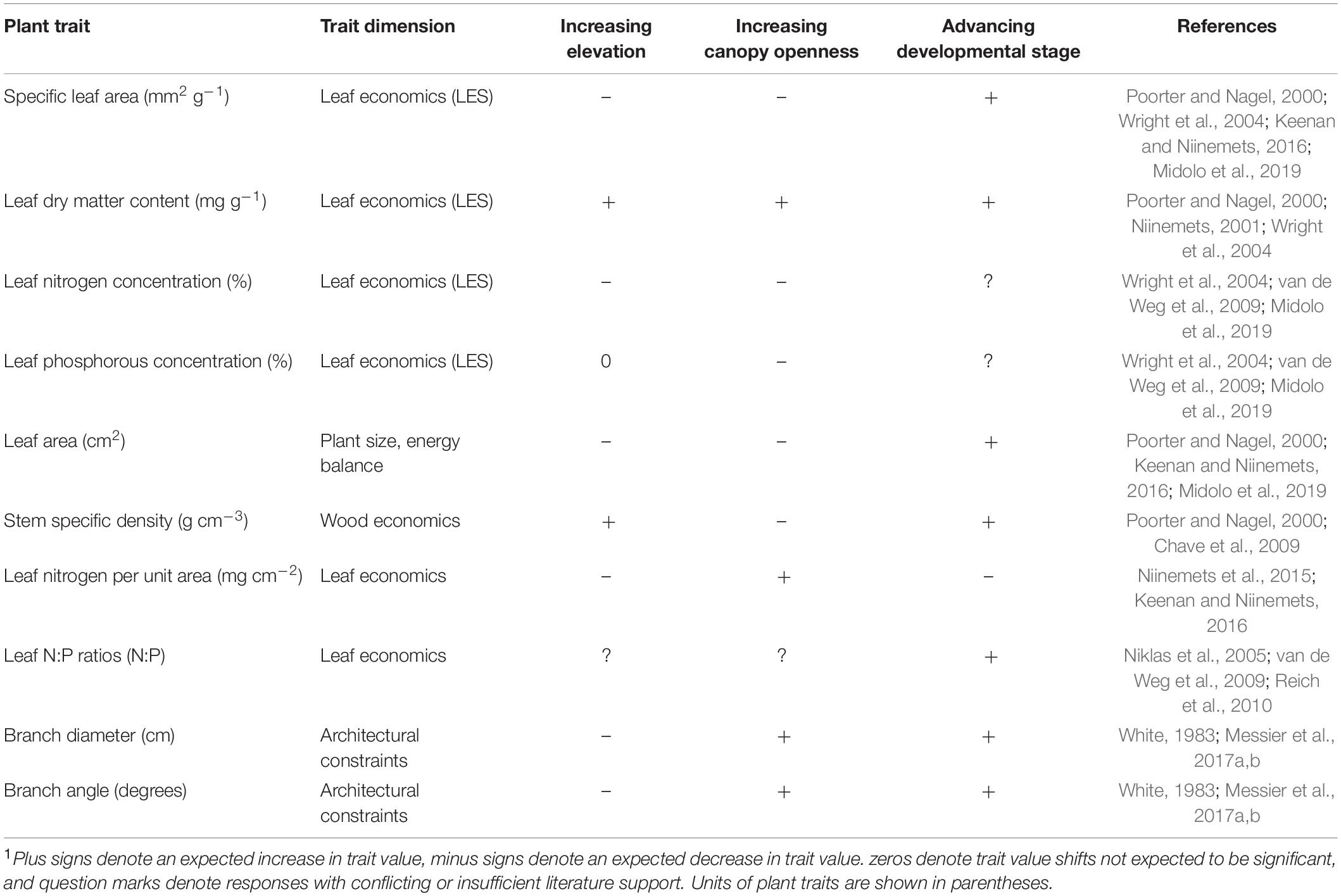
Table 1. Hypothesized trait value responses of specific plant traits to gradients in elevation, canopy openness, and developmental stage1.
Materials and Methods
Study Areas
This study was conducted within and between transects located along elevational gradients on each of five mountains (Mount Bigelow, Mount Whiteface, Mount Madison, Mount Abraham, and Killington Peak) located in four northeastern United States states (Maine, New York, New Hampshire, and Vermont), at elevations ranging from 400 to 1,150 m above sea level (masl). Northern hardwood forests generally occur at lower elevations and primarily consist of shade tolerant species like sugar maple (Acer saccharum), American beech (Fagus grandifolia), and eastern hemlock (Tsuga canadensis), with occasional inclusions of red maple (Acer rubrum) and striped maple (Acer pensylvanicum). These temperate forests transition to Appalachian spruce-fir forests dominated by shade tolerant boreal species such as red spruce (Picea rubens), balsam fir (Abies balsamea). Species found in association with northern hardwood and high elevation conifer forest communities include shade intolerant species such as paper birch (Betula papyrifera), mountain paper birch (Betula cordifolia), American mountain-ash (Sorbus americana), and quaking aspen (Populus tremuloides) and mid-tolerants such as yellow birch (Betula alleghaniensis) and black cherry (Prunus serotina). The elevation of the ecotone depends on latitude, and it decreases on average 100 masl for every 1° increase in latitude (Cogbill and White, 1991). Here, the elevation of the ecotone ranged between 690 to 910 masl (Wason and Dovciak, 2017), depending on the mountain sampled, with an average ecotone elevation around 785 masl. Average annual temperatures across all sites ranged from 2 to 5.8°C (based on 1961–1990 climate normals, ClimateNA), however, the region has experienced an average climate warming of 0.25°C per decade since 1970, with climate projections predicting sustained warming (Hayhoe et al., 2007; Huntington et al., 2009). Soils generally consisted of spodosols of pH 5–6 at lower elevations, which became more acidic and shallower with increasing elevation; soil organic matter also increased with increasing elevation (Siccama, 1974).
Study Design and Field Sampling
To determine patterns of trait variability, we collected leaf and stem samples of each species present within previously established transects (Wason and Dovciak, 2017), and in forest gaps adjacent to these transects (Beeles et al., 2021), at three distinct stages of plant development (i.e., seedlings, saplings, and mature trees). Transects were previously established running along a contour line of constant elevation; six transects ranging in elevation from 500 to 1,000 masl were located on each mountain (or 600–1,000 masl on Killington Peak and Mount Abraham, where suitable stands were not present at 500 masl). All transects at Mount Bigelow, Mount Abraham, and Killington Peak were sampled, however, the 1,000 masl site on Mount Whiteface, and the 700 masl site on Mount Madison were not sampled due to logistical constraints that limited site access. In total, 26 transects were fully sampled. The species sampled were determined before entering the field; for each transect, we selected the species with the highest relative dominance (measured as relative basal area) within a transect, then selected species with successively less relative basal area within a transect, until 80% of the cumulative relative species dominance for the transect was met (Pakeman and Quested, 2007). Relative species dominances within each transect were previously collected in subplots for mature trees using the point centered quarter method, as described in Wason and Dovciak (2017) (Supplementary Figure 1). This sampling design is premised on the mass ratio hypothesis, which predicts that a species contribution to community function is proportional to its relative dominance (Grime, 1998); sampled species number thus varied between transects (2–7 species) based on their species composition. Mature trees within a transect were sampled by selecting accessible trees > 10 cm in DBH, as determined by a visual inspection of trees present. Additionally, species were opportunistically sampled between transects if they represented an individual at the climatic limit of that species’ range on a mountain to maximize the sampled climate range. A full list of species, along with the elevation and climatic range they were sampled within, is included in Supplementary Table 1.
We collected samples of tree seedlings and saplings from each species within the established transects and associated gap plots. Seedling and sapling size cutoffs were based on established diameter at breast height (DBH) measurements used for previous vegetation surveys (Cottam and Curtis, 1956; Holway et al., 1969); seedlings were classified as < 2.5 cm DBH, saplings were classified as between 2–10 cm DBH. Species relative dominances for saplings were determined previously (Beeles et al., 2021); we thus used the same 80% cumulative relative species dominance method as described previously for mature trees to determine which saplings to measure. Seedling dominances within each transect were previously measured as frequency; we sampled up to the five most frequent species per transect, which in all cases exceeded 80% of the cumulative relative frequency for each transect. We sampled tree seedlings and saplings within the closest associated forest gaps located adjacent to each transect. Canopy gaps were previously identified using satellite imagery. Climate normals (1981–2010) for each GPS location associated with sampled individuals were generated using annual temperature estimates for each sample point were generated using ClimateNA (Wang et al., 2016). In total, one sample, consisting of a branch with multiple leaves or several hundred needles, was taken per target species, canopy condition, and life stage for each transect. Seedling and saplings that represented the climatic limit of that species’ range on a mountain were also sampled, as previously described for mature trees.
Field Measurements
Fully expanded leaves still connected to their twig (to minimize water loss) were collected from understory trees using garden shears, while canopy leaves were collected using the shotgun method. Most samples collected were of healthy leaves, however, leaves were occasionally sampled that exhibited evidence of insect damage or disease; in these cases, the healthiest leaves from the collected sample were selected for traits analysis. Samples were wrapped in a moist paper towel, put into a plastic bag, and stored in a cooler with ice. Branch angle was measured in the field on attached foliage using a protractor on the first branch that was leafless at its base but bore secondary branches that had leaves. Branch diameter was recorded as an average of two perpendicular measurements to the nearest 0.1 mm, taken directly below where branch angle was measured, using calipers in the field. Plant height was measured as distance from the ground to the highest live tissue using a meter stick for seedlings and saplings, and a range finder for mature trees. GPS points were taken within 15 m of each sampled plant, and forest canopy density was quantified using a densiometer. Densiometer readings were taken approximately 1.4 m off the ground and above each plant, unless several suitable plants were located within 2 m of one another, when readings were taken at an average distance between sampled plants. No densiometer readings were taken for canopy trees. Densiometer measurements were generalized by separating samples into quintiles before statistical analyses to include canopy trees within the dataset, which were assumed to be in the highest canopy openness quintile (80–100%). More information on leaf and stem sampling procedures can be found within established protocols (Pérez-Harguindeguy et al., 2013).
Lab Traits Measurement
All traits were measured using protocols described previously (Pérez-Harguindeguy et al., 2013). Fresh leaves were weighed, dried at 60°C for a minimum of 72 h, and reweighed. Leaf images was taken using a flatbed scanner set at 300 DPI and were measured for leaf area using ImageJ (Schindelin et al., 2012). A total of four leaves per plant was used as the unit of replication for all leaf traits, except for conifer species and broadleaved samples with excessively small leaves, where a mass-based cutoff was used (2 g fresh-weight) to ensure adequate sample for chemical analyses. Stem samples were collected either by clipping an approximate 10 cm sample of branch tissue using garden shears for stem specific density, or by using an increment borer for trunk sapwood density. For stem specific density samples, surface detritus (i.e., lichens, loose bark, cobwebs, etc.) was removed, and stem volume was measured using the water-displacement method. For trunk sapwood density samples, tree cores had their sapwood measured for volume using the water-displacement method. Branch and stem samples were weighed, dried at 70°C for a minimum of 72 h, and reweighed to calculate stem specific density. Leaves were dried and ground with a ball grinder, and sent to Brookside Laboratories (New Bremen, OH, United States) for tissue chemistry analysis (specifically nitrogen and phosphorous) using the dry-ashing method. While leaf nitrogen per unit mass (LNC) is most commonly used metric for examining leaf nitrogen, leaf nitrogen per unit area (Narea) is known to demonstrate high plasticity with respect to light levels; thus, both LNC and Narea were thus examined in all analyses due to the wide variety of light conditions within the study (Ellsworth and Reich, 1993; Niinemets et al., 2015; Keenan and Niinemets, 2016).
Climate Data
All climate metrics were obtained from PRISM climate data covering the period 1961–1990 that were down-scaled using the spatial interpolation software Climate NA, based on an tree samples’ geographic coordinates and elevation as recorded by handheld GPS (Wang et al., 2016; PRISM Climate Group, Oregon State U, 2020). Potential evapotranspiration (PET) was selected as the relevant climate metric from the available climate variables because it incorporates temperature, humidity, and solar radiation; PET was estimated using Hargreaves reference evaporation metric (Hargreaves and Allen, 2003).
Statistical Analyses
Non-metric Multidimensional Scaling
We used non-metric multidimensional scaling (NMS) to characterize inter- and intraspecific relationships among traits. NMS is a highly flexible non-parametric ordination method preferred for studies exploring patterns within multiple responses that do not meet parametric assumptions. All multivariate analyses were completed using software PC-ORD, version 7, using the workflow described by Peck (McCune and Grace, 2002 p.; Peck, 2016). The main and second matrices were first relativized by maximum to scale our responses prior to using the autopilot function to determine the appropriate dimensionality for the analysis. The autopilot was set to medium and was run four times to assure consistent results. Comparisons between the scree plots of these separate runs suggested that a two-dimensional NMS was appropriate. We then reran the NMS procedure four times at two dimensions and compared ordinations and randomization tests; any runs with excessive stress or large p-values were rejected and rerun. Stress vs. iteration numbers were also examined between runs to ensure the number had stabilized. Once all NMS analyses showed consistent results, the run with the lowest stress was selected for interpretation. The resulting ordination was plotted using PC-ORD software, with the functional traits overlaid using the biplot option. The NMS itself was run on a subset of the collected data (408 samples) excluding branch architecture traits and any samples missing data for the included functional traits or explanatory variables. The data subset excluded architectural traits as the sampling of these traits was restricted to seedlings and saplings.
Variance Partitioning Analysis
We used linear mixed effects models to determine the taxonomic levels responsible for functional trait variation and importance of intraspecific variation, using the R package “lmerTest” (Kuznetsova et al., 2017). Models were set with a fixed intercept and nested random effects for division (angiosperm vs. gymnosperm), order, family, genus, and species, following established procedures (Burton et al., 2017; Messier et al., 2017a). Residual variation from these models was interpreted as intraspecific variation. Variance components were summed, and each taxonomic level value was divided by the total to yield a percent variance explained. Variance partitioning analyses were performed in R Studio version 4.0.3 (R Core Team, 2020).
Guided Stepwise Linear Mixed Effects Model Selection
We used a model comparison and selection process (Burnham and Anderson, 2003) to assess the influence of light, climate conditions, and plant development on trait variation within species. We used a stepwise process to compare a limited number of plausible linear mixed effects models that added terms in order of their empirical support in previous studies (Burton et al., 2014; Pettit et al., 2019). We compared model AICc values, a bias corrected version of Akaike’s information criterion (AIC) for small samples to determine best-fit models, using the R package “AICcmodelavg” (Mazerolle, 2020). Models that did not differ substantially (Δ AICc < 2), the simpler of the two models was selected. If adding any additional fixed effects did not improve the model, the model from the previous step in the selection process was used to compare subsequent models. We additionally estimated and compared the variance explained by included fixed effects (marginal; R2m) and the full model (conditional; R2c) to determine the importance of the included fixed effects in explaining trait variation (Nakagawa and Schielzeth, 2013). We also calculated semi-partial R2’s (R2β), which measure the relative contributions of fixed effects relative to other variables within a linear mixed effects model (Edwards et al., 2008).
To account for the study’s sampling design, models included nested random effects for sample location (e.g., elevation contour within mountain of origin) when the trait data supported nested random effects. If, at the beginning of the model selection process, a model’s random effects structure was too complex for the trait data to support, we only included mountain as a random effect for all successive models. The random effect’s structure was thus held constant when comparing between the null model and alternatives of a single plant trait. Because of occasional inconsistencies in trait sampling, some samples lacked information for certain traits or environmental data. Trait models were thus selected using samples with complete trait and environment data, causing the sample size for each species to differ between traits. A summary of the sample number by species and trait for each linear mixed effects model can be found in Supplementary Table 2. All stepwise linear mixed models were created and analyzed in R Studio version 4.0.3 (R Core Team, 2020).
Step 1: Species—First, we compared a null, intercept only model to a model using species as a main effect. Because the study was designed to assess species trait variation, controlling for species was the first and most important step in the model selection process. The best model from this step was carried forward to step two.
Step 2: Canopy openness—Next, we assessed alternative models that examined different hypotheses involving the importance of fine scale effects in light availability. We examined the effect of light availability by adding a fixed effect representing quintiles of canopy openness to the model and examined the two-way interaction of canopy openness and species. Additionally, we tested for the possibility of species cross-over by comparing models with and without interaction terms between species and the variable of interest. The best model (Δ AICc < 2, if any) from step two was carried forward to step three.
Step 3: Climate—The effect of climate was examined using an annual average of PET. As before, we assessed two-way interactions between climate and other fixed effects if they were included as main effects within the best-fit model from the previous step. To test the hypothesis that trait relationships to climate depend on light availability, we additionally examined a model with two-way interactions between climate and canopy openness. Any model including a climate and light interaction assumed all species respond similarly to interactions between light and climate to a model with a three-way interaction (i.e., species × climate × light) that allows individualistic responses among species.
Step 4: Development—Lastly, we compared alternative models that added effects for plant development to the best-fit model. The effect of plant development was assessed using a categorical variable for life-stage based on the study’s sampling design (i.e., seedling, sapling, mature trees). We also compared potential two-way interactions with life stage and any other included fixed effects. We examined all two-way interactions between fixed effects because they appeared to represent ecologically valid hypotheses.
Best-fit models containing interaction terms provide potential evidence for species crossover. To further examine such models containing significant species interactions, we ran a series of post hoc pairwise comparisons of the estimated marginal means (i.e., predicted model averages within a model’s reference grid, where all combinations of factor levels within a model are calculated), using the R package, “emmeans” (Lenth et al., 2021). Results from the pairwise comparisons of these estimated marginal means were then adjusted using the Benjamini–Hochberg false discovery rate adjustment for multiple comparisons to minimize the possibility of type 1 error (Benjamini and Hochberg, 1995). This multiple correction type was an appropriate correction method because of the high number of comparisons performed that, given the size of the dataset, would force alternative methods to be overly conservative and inflate type 2 error. All post hoc analyses were performed in R studio 4.0.3 (R Core Team, 2020).
Results
Trait Dimensions
The ordination of functional traits showed a clear delineation between gymnosperm samples with negative PC scores, and angiosperm samples with neutral and positive PC scores, as well as a delineation as between temperate and high-elevation conifer forest communities (Figure 2A). The overlay of traits in the ordination confirms the first axis was associated with the LES; deciduous angiosperms with short leaf lifespans had acquisitive traits including higher SLA, LNC, while evergreen conifers with long leaf lifespans had conservative traits with opposite trends. This axis explains most of the variation within the ordination (r2 = 0.79, i.e., 79% of explained variance). The second axis explained a small but significant component of variation within the ordination (r2 = 0.13) and was primarily associated with SSD and N:P. This second axis was related to environmental variation and differences in traits between life stages (Figures 2B,C, respectively). There was considerable overlap between species within phylogenetic divisions (i.e., angiosperms and gymnosperms); however, there was noticeable, systematic variation in samples due to canopy openness and life stage. Species exhibiting similar life strategies also appeared to cluster together; for example, shade-intolerant angiosperms such as S. americana were more related in multivariate trait space with each other than to shade-tolerant species such as A. saccharum and F. grandifolia. Three traits, LDMC, LPC, and Narea, were influenced by both axes of the ordination.
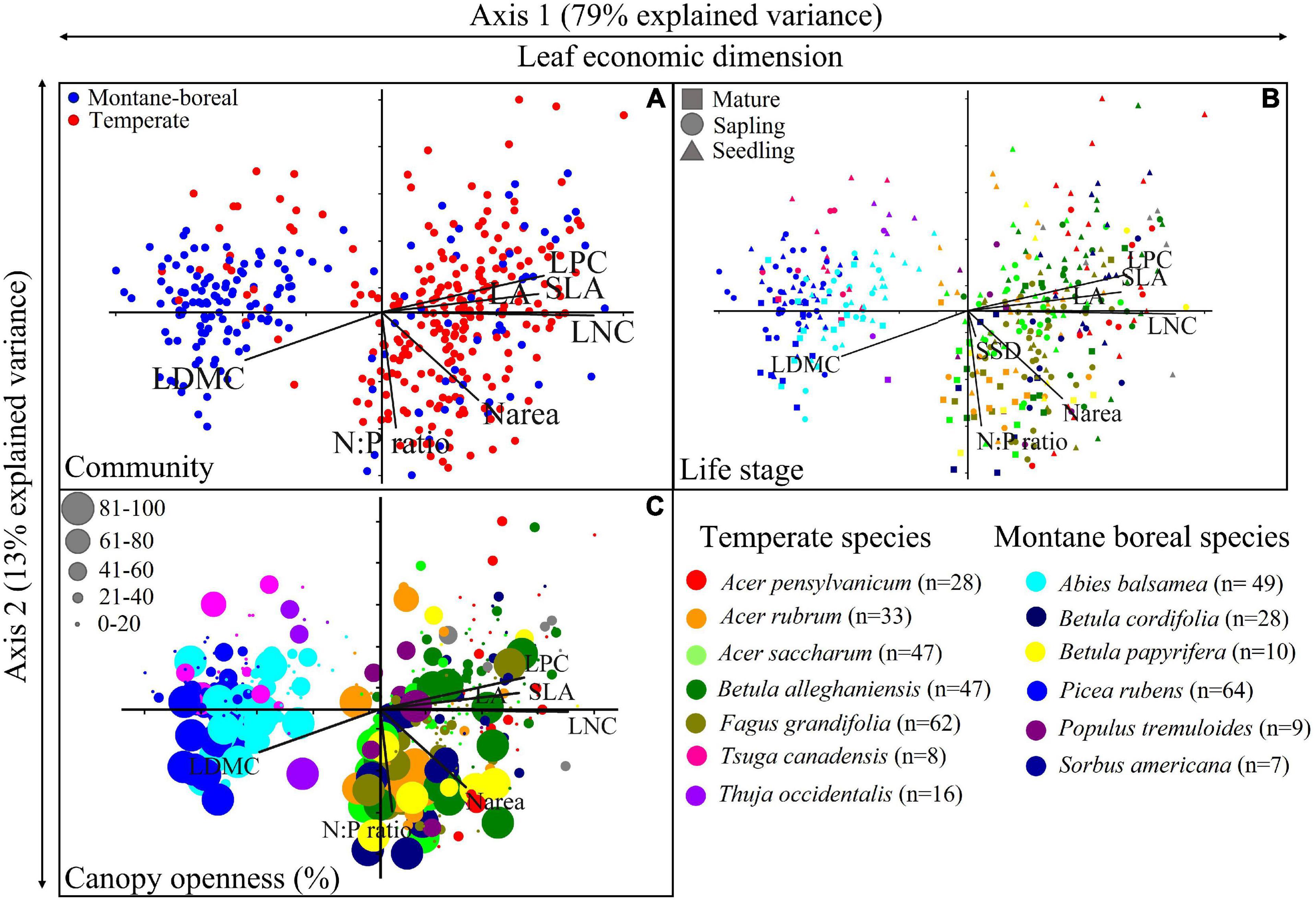
Figure 2. Ordination of functional traits in relation to different taxonomic and structural groupings. (A) Ordination of individual trees by community type with functional traits overlaid. (B) Canopy openness for individual trees is highlighted using circle size, with species identified by color. (C) Life stage of individual trees is highlighted using circle size, with species identified by color. Sample size within the ordination is shown in the legend next the scientific name of the species. Trait acronyms; Specific leaf area (SLA), leaf area (LA), leaf dry matter content (LDMC), leaf nitrogen concentration (LNC), leaf phosphorous concentration (LPC), leaf nitrogen per unit area (Narea), stem specific density (SSD), leaf nitrogen to phosphorus ratio (N:P).
Influence of Intraspecific Trait Variation Between Traits
The relative importance of ITV ranged from 8% for leaf area to 68% for branch angle, with a majority of trait variation concentrated at the division level between angiosperms and gymnosperms (Figure 3). Analysis of trait variation across taxa showed that with the exception of branching angle, interspecific trait variation exceeded ITV. Most traits had less than 50% of their variance explained by ITV; however, influence of ITV was not consistent among traits. Traits related to LES typically were less variable within species ITV, while traits related to architectural constraints and stem economic spectrum were more variable within species. Some traits showed no variation explained by certain taxonomic levels due to the low species diversity of the studied transitional temperate-boreal ecotone forests, where an individual species may also be the only representative of an entire family.
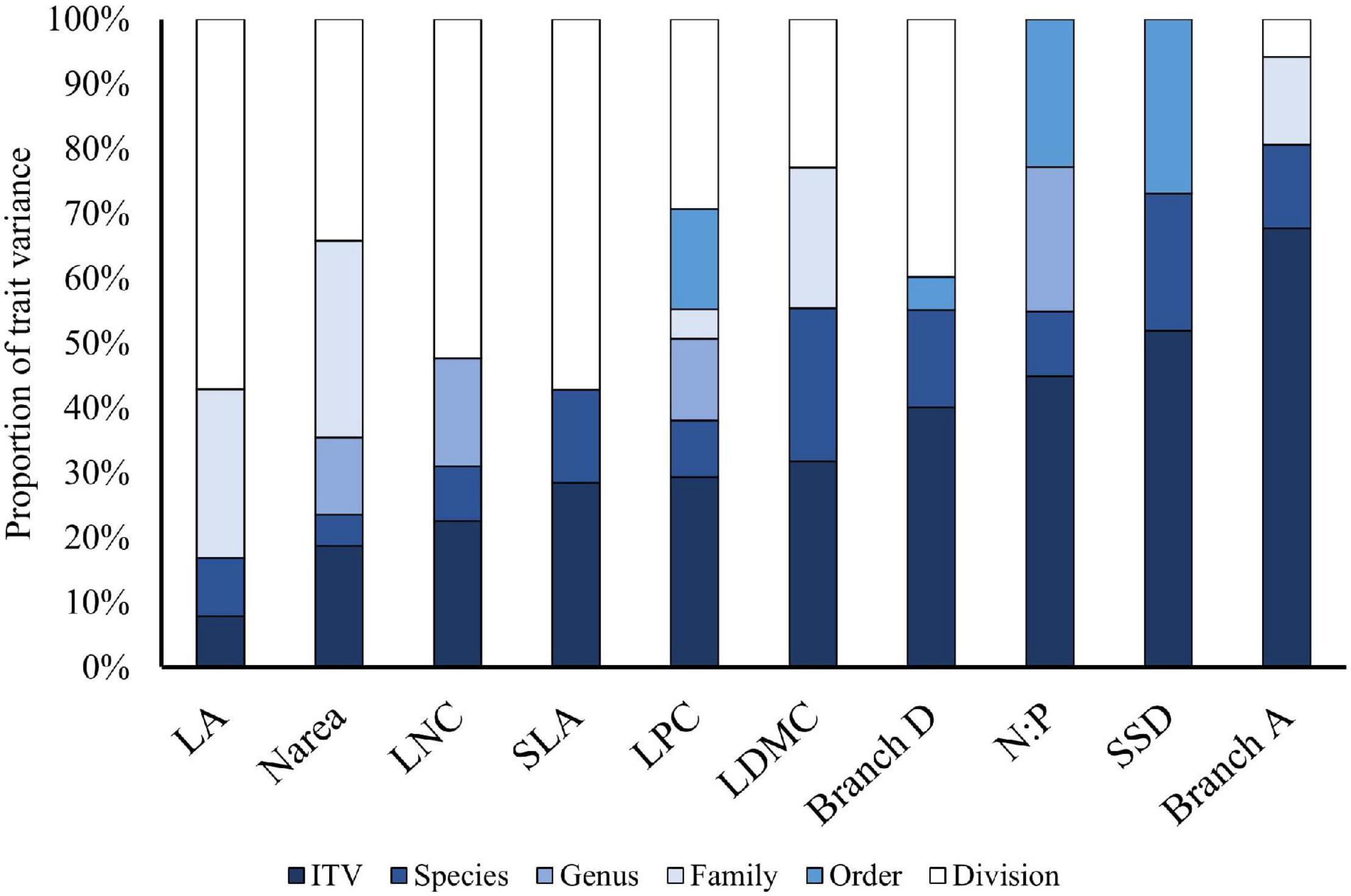
Figure 3. Variance decomposition of measured leaf and stem economic traits across different taxonomic levels from communities spanning the temperate-boreal ecotone of the northeast United States.
Trait-Environment Relationships
Leaf economics: Variation in traits associated with leaf economics were primarily driven by differences between species based on semi-partial coefficients (R2β, ranged from 0.096 to 0.981), however, all leaf traits were also related to other variables (Figure 4 and Table 2). The selected models for SLA, LNC, Narea and LPC indicated that variance was also related to canopy openness (R2β = 0.132, 0.083, 0.063, and 0.188, respectively), with LNC additionally being influenced by climate (R2β = 0.121), and SLA and Narea additionally being influenced by life stage (R2β = 0.112 and 0.091, respectively). SLA, LNC, and LPC decreased with increasing canopy openness, while Narea increased with increasing canopy openness; the trends in SLA and Narea with increasing light are noteworthy because within species trends become more acquisitive with increasing light, running counter to the global LES. The selected models for LDMC and N:P were weakly related to light (R2β = 0.055 and R2β = 0.012, respectively), life-stage (R2β = 0.008 and 0.002, respectively), and interactions between species and life-stage (R2β = 0.107 and 0.099, respectively). Model estimates for both LDMC and N:P increased with increasing canopy openness. Subsequent ANOVA’s of LDMC and N:P ratio best-fit models showed evidence for significant interactions of species with life-stage for N:P, and LDMC (p < 0.05), suggesting the possibility of species crossover. To examine the potential for species crossover further, we ran a post hoc analysis of the estimated marginal means for the interactions within these models, using a false discovery rate adjustment for multiple comparisons. There was no evidence for consistent species trait rank shifts for N:P; for LDMC, several species whose trait values were equivalent as seedlings diverged in mature trees (Table 3). However, while there may be evidence for separate slopes models for LDMC and N:P, no changes in trait values with life stage resulted in species crossover.
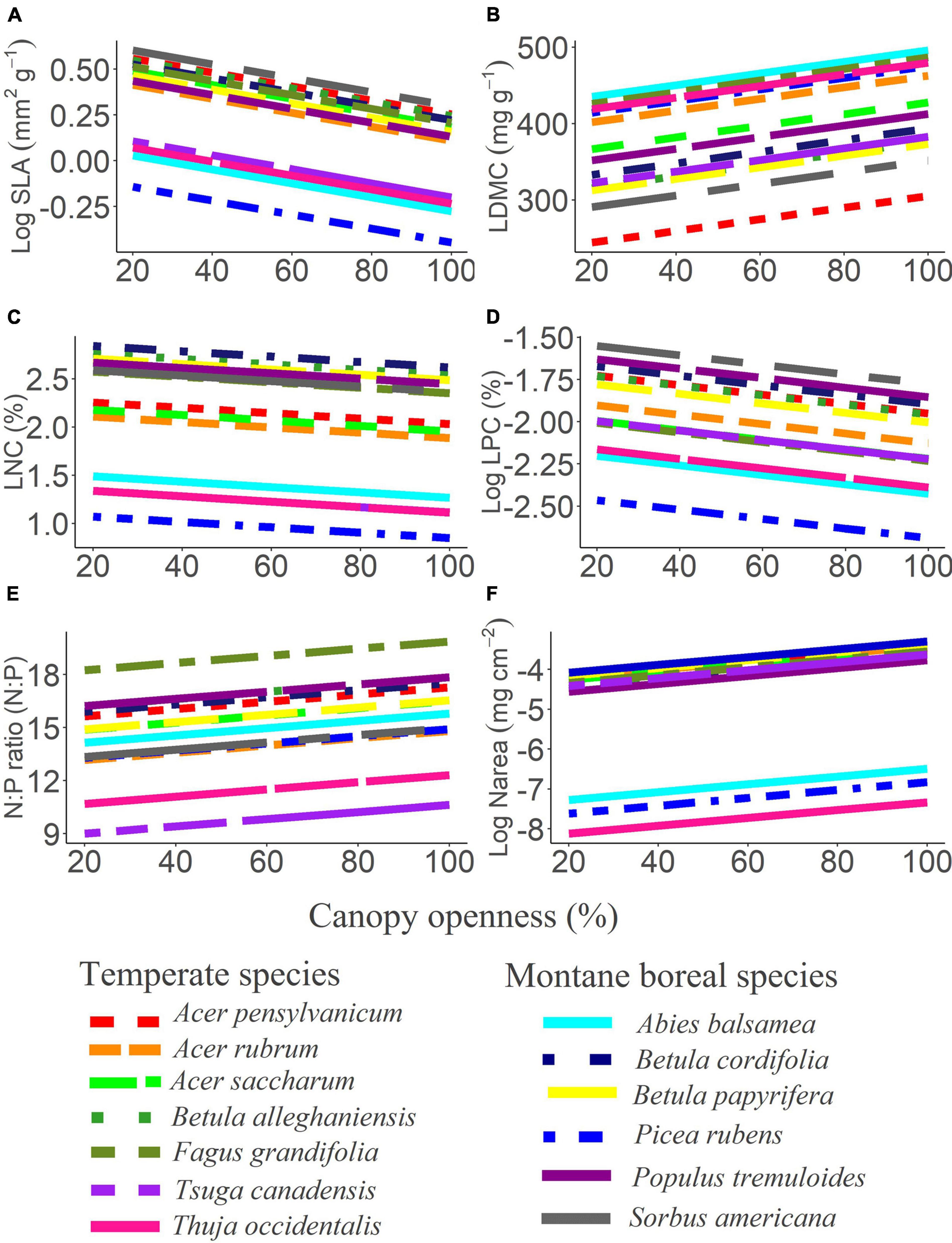
Figure 4. Estimated relationships of measured functional traits to gradients of light. Lines show model estimates for each species from the best-fit model for (A) specific leaf area (SLA), (B) leaf dry matter content (LDMC), (C) leaf nitrogen concentration (LNC), (D) leaf phosphorous concentration (LPC), (E) nitrogen to phosphorous ratios (N:P ratio), and (F) leaf nitrogen per unit area (Narea).
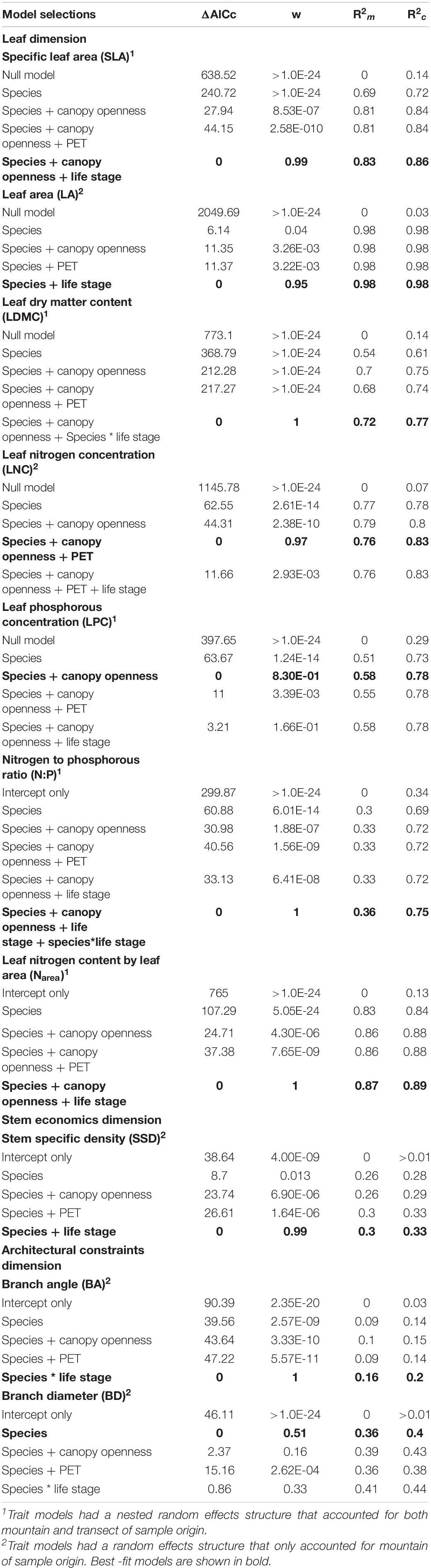
Table 2. Comparison of delta AICc (the difference between AICc values of an alternate model against the best-fit model for a given trait), Akaike weights (w), and marginal R2 (R2m) and conditional R2 (R2c) of best-fit trait models from each model selection step, where models with an ΔAICc of 0 are the best-fit model for a given trait.
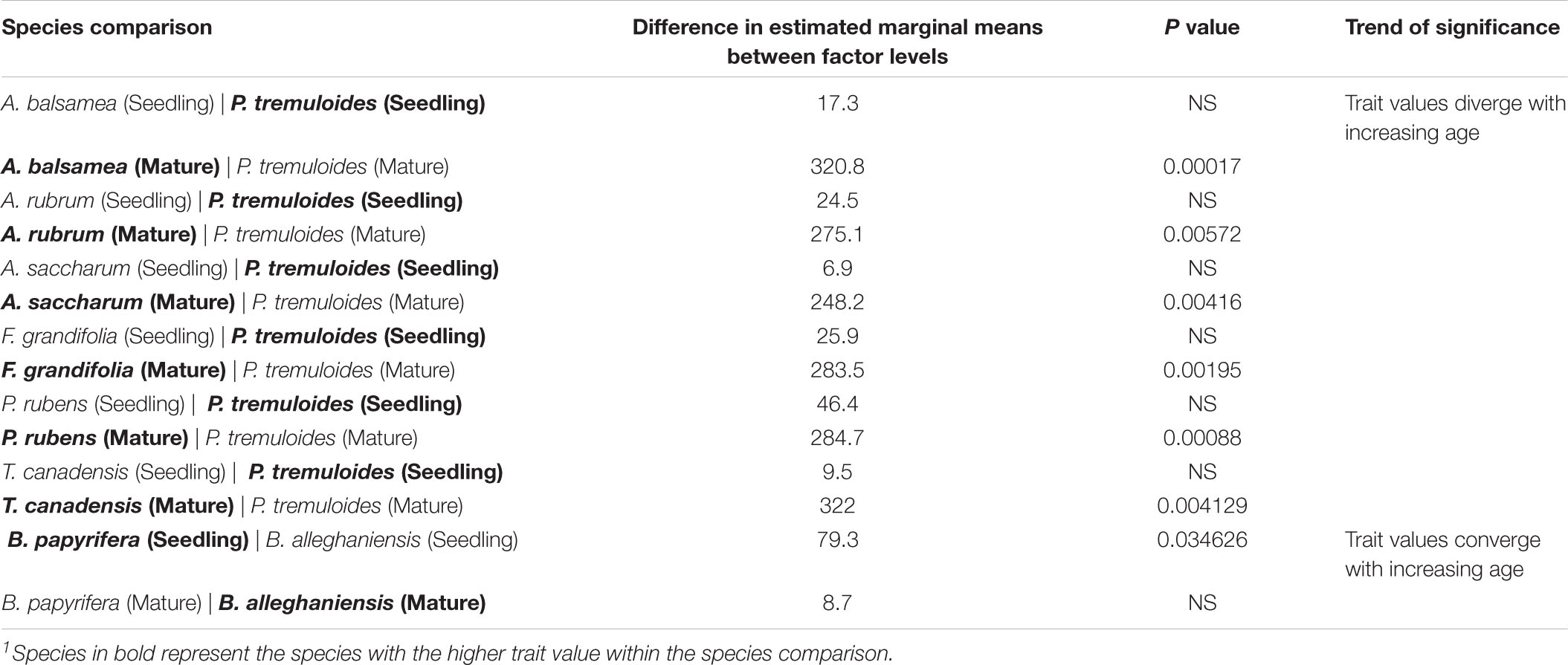
Table 3. Subset of post hoc pairwise comparisons of the LDMC estimated marginal means across life stage for the selected best-fit model, where trait values significantly diverge with age1.
Stem economics: Variation in traits associated with stem economics (i.e., SSD) was influenced by species (R2β = 0.268) and weakly influenced by life-stage (R2β = 0.063).
Architectural constraints: Variation in traits associated with architectural constraints were also primarily driven by differences between species (R2β ranged from 0.096 to 0.27), with branch diameter exclusively being driven by differences between species; however, branch angle was influenced by life-stage (R2β = 0.005), and additionally by an interaction between species and life-stage (R2β = 0.018).
Variation Explained by Fixed Effects
Best-fit models had fixed effects that explained between 14 and 98% of total variance (Table 2). Marginal R2 values across all models were generally large, suggesting that only a small proportion of variation was due to the random effects. Only the LPC and N:P ratio models were improved by more than 20% by including random effects. Adding species as a fixed effect resulted in the largest improvement for all models. Except for the species and light interaction in the LDMC model, all subsequent model terms increased the variance explained by less than 10%. Light was the next most significant fixed effect in most models, explaining an additional 1–7% more variance when included. Climate and life-stage were the least important variables included in the model, contributing relatively equal amounts of explanatory power in models. Significant species–variable interactions indicative of species crossover were present in the best-fit models of three traits (LDMC, branch angle, and N:P ratio); however, only the interaction between species and light in the LDMC best-fit model substantially increased the variance explained by the model.
Discussion
Our results showed that despite intraspecific variation in LES traits, tree species spanning the montane temperate-boreal ecotone in the northeastern United States were primarily distinguished along the leaf economics spectrum (LES). Within species variation in LES traits along gradients of light ran counter to the global LES (i.e., increases in resources resulted in shifts toward the conservative rather than acquisitive end of the LES). A second dimension reflected inter and intraspecific variation in leaf N:P and stem SSD. Levels of intraspecific trait variability (ITV) were generally low and related to climate and light, and differed among seedlings, saplings and overstory trees. Traits related to leaf chemistry, stem economics and branching architecture had higher levels of ITV, which may warrant consideration in future studies. Overall, there was very little evidence of trait crossover, suggesting species maintain competitive hierarchies across Leaf N:P and SSD gradients in climate conditions and resources assessed here.
The second axis in the ordination showed a dimension orthogonal to the LES, which is not related to the division between angiosperms and gymnosperms. This axis is primarily structured by variation in leaf N:P ratios and SSD; high levels of intraspecific variation in these traits may thus be influencing this axis. First, N:P is also influenced strongly with nutrient availability (Güsewell, 2004; Reich and Oleksyn, 2004), which is known to vary across the northeastern United States from a combination of parent material weathering and N deposition (Richardson, 2004; Crowley et al., 2012). Second, SSD increases as trees transition from seedling to sapling and overstory trees. Recent global studies of community level trait covariation have found that at the community level, SSD and N:P are more strongly influenced by environmental variables than other commonly studied plant traits, with SSD increasing with PET and N:P increasing with growing-season temperature (Bruelheide et al., 2018).
Our variance partitioning analysis showed that most leaf traits generally had low levels of ITV, indicating that differences in trait values were primarily driven by taxonomic differences and not responses to environmental conditions. These trends generally correlate with previous research (Burton et al., 2017; Anderegg et al., 2018). The majority of interspecific trait variation was explained at the division level; however, there were considerable differences among traits. Stem and architecture traits had greater levels of intraspecific trait variation. Architectural traits are known to be highly plastic in response to resource availability; for example, branching angle increases with light (White, 1983; Ackerly and Donoghue, 1998; Messier et al., 2017a). However, we were unable to assess branching architecture traits from mature trees because of the logistical constraints involving sampling the forest canopy. Because these traits showed some of the highest levels of ITV of the traits measured, and that plant architecture traits have been previously shown to indicate tradeoffs in plant life strategy (Messier et al., 2017a), more research is required to determine how these traits vary over the plant’s lifespan.
In contrast with expectations based on the global LES which contrasts acquisitive species with high SLA, LNC from conservative species with high LDMC, SLA and LNC decreased while LDMC increased with light. These trends have been noted previously (Burton et al., 2017), and may result from physiological tradeoffs that lead to context dependent relationships between traits and resource utilization (Anderegg et al., 2018). Increased SLA in shade may result in more efficient light harvesting per unit biomass (Lusk et al., 2008; Burton et al., 2017) while increases in Narea and LDMC in conjunction with lower SLA in canopy openings reflect increases in photosynthetic capacity (and resource acquisition). As expected, trait models were most improved by accounting for taxonomy; however, variation within species was also related to light for many traits assessed here. Our study thus reiterates the importance of accounting for light in trait studies at local spatial scales within forest ecosystems.
Within species, leaf nitrogen content (LNC) decreased with potential evapotranspiration (PET) and associated increases in mean annual temperature (and decreases in elevation). This trend may reflect plant’s ability to increase photosynthetic capacity at higher elevations whenever growing seasons are short (Körner, 2007; Reich and Oleksyn, 2004). A legacy of nitrogen deposition at high elevation in the northeast may also lead to increases in LNC (Aber et al., 2003). ITV due to climate was also a component in many best-fit trait models, despite the climate gradient of our study only spanning a portion of the species’ total realized niches. Future trait-based studies in similar communities should thus take ITV into account when examining patterns in LNC, particularly where the climate gradient is comparable or exceeds that found in our study.
Despite the LDMC, BA, and N:P best-fit models containing interactions terms indicative of species crossover, we did not find much evidence that such trait rank shifts were statistically significant. Observed trait rank shifts were restricted to instances where species trait values diverged in older developmental stages. The most consistent trend was for quaking aspen (Populus tremuloides) where LDMC decreased with increasing older stages relative to other species. Lower LDMC is generally associated with reduced leaf lifespan and greater potential growth rates (Ryser, 1996; Wilson et al., 1999) and may thus indicate a shift in plant strategy toward resource acquisition as the individual ages. Interpretation of P. tremuloides functional traits may be confounded by the species’ propensity for polyploidy, which can modify an individual tree’s structure and physiology based on ploidy level (Greer et al., 2018). Because the shifts in LDMC were weak and primarily restricted to a single species, our results confirm that LDMC in the northeast may be modeled with a single mean trait value over similar spatial scales.
Conclusion
We show here that trees species in northeastern montane forests are aligned with the leaf economics spectrum (LES) despite intraspecific variation. That is, variation in light and canopy openness resulted in within-species variation in LES traits that runs counter to expectations based on tradeoffs underlying the LES. Despite this intraspecific variation, trait correlations underlying the LES explained the majority of variation at the multivariate level. Interestingly, the LES is not only present at moderate spatial scales that span the TBE, but consistent within biomes (i.e., temperate and montane boreal forests). Therefore, the LES trait dimension may indeed be applicable to local-scale studies of community assembly in temperate and high elevation boreal forests. We did not find conclusive evidence for the presence of other global trait dimensions locally (White, 1983; Westoby et al., 2002; Chave et al., 2009; Díaz et al., 2016; Messier et al., 2017a). However, other traits not assessed here could explain additional dimensions of functional differentiation such as root traits (Kramer-Walter et al., 2016; Ma et al., 2018), and plant size (e.g., plant height, seed size, Díaz et al., 2016). The coordination of LES traits suggests that measuring a small number of associated traits may sufficiently represent plant performance at local scales. The relative importance of ITV was not consistent between traits, suggesting it should be evaluated on a trait-by-trait basis. High levels of ITV for N:P and SSD may explain the second axis of our ordination; within species variability in N:P and SSD may thus be more important for explaining distributions along elevation gradients than other commonly measured traits. Lastly, we found limited evidence for species crossover, supporting the findings of previous studies (Burton et al., 2017). Consequently, single trait values may be used in future trait-based studies within a similar range of light and climate conditions, with the exception of trait variation in life stage for LDMC and N:P, where trait values should take into account a individuals life stage. Accounting for ITV related to life stage and environmental variation may be particularly important, even in the absence of cross-over, for modeling studies conducted at smaller spatial scales, such as physiological and gap-based models of tree growth and forest dynamics.
Data Availability Statement
The raw data supporting the conclusions of this article will be made available by the authors, without undue reservation.
Author Contributions
JB, MH, and MD contributed to the conception and design of the study. MH and JZ collected the field data. MH performed the statistical analyses and wrote the drafts of the manuscript. All authors contributed to manuscript revision, read and approved the submitted version.
Funding
Funding for this research was made possible through USDA National Institute of Food and Agriculture McIntire-Stennis Program NYZ1165802 and a research grant from the United States National Science Foundation NSF-BCS-GSS-1759724.
Conflict of Interest
The authors declare that the research was conducted in the absence of any commercial or financial relationships that could be construed as a potential conflict of interest.
Publisher’s Note
All claims expressed in this article are solely those of the authors and do not necessarily represent those of their affiliated organizations, or those of the publisher, the editors and the reviewers. Any product that may be evaluated in this article, or claim that may be made by its manufacturer, is not guaranteed or endorsed by the publisher.
Acknowledgments
We would like to thank the New York Department of Environmental Conservation, the Green Mountain National Forest and the White Mountain National Forest units of the United States Forest Service, and the Maine Bureau of Parks and Lands for their assistance in securing research permits. We would also like to thank Jordon Tourville and Jay Wason for their constructive comments regarding field sampling protocols.
Supplementary Material
The Supplementary Material for this article can be found online at: https://www.frontiersin.org/articles/10.3389/ffgc.2021.754063/full#supplementary-material
References
Aber, J. D., Goodale, C. L., Ollinger, S. V., Smith, M. L., Magill, A. H., Martin, M. E., et al. (2003). Is nitrogen deposition altering the nitrogen status of northeastern forests? BioScience 53:375.
Ackerly, D. D., and Donoghue, M. J. (1998). Leaf size, sapling allometry, and corner’s rules: phylogeny and correlated evolution in maples (Acer). Am. Nat. 152, 767–791. doi: 10.1086/286208
Ackerly, D., Knight, C., Weiss, S., Barton, K., and Starmer, K. (2002). Leaf size, specific leaf area and microhabitat distribution of chaparral woody plants: contrasting patterns in species level and community level analyses. Oecologia 130, 449–457. doi: 10.1007/s004420100805
Albert, C. H., Thuiller, W., Yoccoz, N. G., Soudant, A., Boucher, F., Saccone, P., et al. (2010). Intraspecific functional variability: extent, structure and sources of variation. J. Ecol. 98, 604–613. doi: 10.1016/s0065-2881(02)43006-4
Álvarez-Yépiz, J., Búrquez, A., Martínez-Yrízar, A., Teece, M., Yépez, E. A., and Dovciak, M. (2017). Resource partitioning by evergreen and deciduous species in a tropical dry forest. Oecologia 183, 607–618. doi: 10.1007/s00442-016-3790-3
Anderegg, L. D. L., Berner, L. T., Badgley, G., Sethi, M. L., Law, B. E., and HilleRisLambers, J. (2018). Within-species patterns challenge our understanding of the leaf economics spectrum. Ecol. Lett 21, 734–744. doi: 10.1111/ele.12945
Beeles, K., Tourville, J., and Dovciak, M. (2021). Characterizing canopy openness across large forested landscapes using spherical densiometer and smartphone hemispherical photography. J. For. doi: 10.1093/jofore/fvab046
Benjamini, Y., and Hochberg, Y. (1995). Controlling the false discovery rate: a practical and powerful approach to multiple testing. J. R. Stat. Soc. Ser. B 57, 289–300.
Bruelheide, H., Dengler, J., Purschke, O., Lenoir, J., Jiménez-Alfaro, B., Hennekens, S. M., et al. (2018). Global trait–environment relationships of plant communities. Nat. Ecol. Evol. 2, 1906–1917. doi: 10.1038/s41559-018-0699-8
Burnham, K. P., and Anderson, D. R. (2003). Model Selection and Multimodel Inference: A Practical Information-Theoretic Approach. Berlin: Springer Science & Business Media.
Burton, J. I., Ganio, L. M., and Puettmann, K. J. (2014). Multi-scale spatial controls of understory vegetation in Douglas-fir–western hemlock forests of western Oregon, USA. Ecosphere 5:art151.
Burton, J. I., Perakis, S. S., Brooks, J. R., and Puettmann, K. J. (2020). Trait integration and functional differentiation among co-existing plant species. Am. J. Bot. 107, 628–638. doi: 10.1002/ajb2.1451
Burton, J. I., Perakis, S. S., McKenzie, S. C., Lawrence, C. E., and Puettmann, K. J. (2017). Intraspecific variability and reaction norms of forest understorey plant species traits (M Tjoelker, Ed). Funct. Ecol. 31, 1881–1893.
Chave, J., Coomes, D., Jansen, S., Lewis, S. L., Swenson, N. G., and Zanne, A. E. (2009). Towards a worldwide wood economics spectrum. Ecol. Lett. 12, 351–366. doi: 10.1111/j.1461-0248.2009.01285.x
Chen, I.-C., Hill, J. K., Ohlemuller, R., Roy, D. B., and Thomas, C. D. (2011). Rapid range shifts of species associated with high levels of climate warming. Science 333, 1024–1026. doi: 10.1126/science.1206432
Cogbill, C. V., and White, P. S. (1991). The latitude-elevation relationship for spruce-fir forest and treeline along the Appalachian Mountain chain. Vegetatio 94, 153–175.
Cottam, G., and Curtis, J. T. (1956). The use of distance measures in phytosociological sampling. Ecology 37, 451–460. doi: 10.1371/journal.pone.0199980
Crowley, K. F., McNeil, B. E., Lovett, G. M., Canham, C. D., Driscoll, C. T., Rustad, L. E., et al. (2012). Do Nutrient limitation patterns shift from nitrogen toward phosphorus with increasing nitrogen deposition across the Northeastern United States? Ecosystems 15, 940–957.
Derroire, G., Powers, J. S., Hulshof, C. M., Varela, L. E. C., and Healey, J. R. (2018). Contrasting patterns of leaf trait variation among and within species during tropical dry forest succession in Costa Rica. Sci. Rep. 8:285. doi: 10.1038/s41598-017-18525-1
Díaz, S., Kattge, J., Cornelissen, J. H. C., Wright, I. J., Lavorel, S., Dray, S., et al. (2016). The global spectrum of plant form and function. Nature 529, 167–171.
Dong, N., Prentice, I. C., Wright, I. J., Evans, B. J., Togashi, H. F., Caddy-Retalic, S., et al. (2020). Components of leaf-trait variation along environmental gradients. New Phytol. 228, 82–94. doi: 10.1111/nph.16558
Edwards, L. J., Muller, K. E., Wolfinger, R. D., Qaqish, B. F., and Schabenberger, O. (2008). An R2 statistic for fixed effects in the linear mixed model. Stat. Med. 27, 6137–6157. doi: 10.1002/sim.3429
Ellsworth, D. S., and Reich, P. B. (1993). Canopy structure and vertical patterns of photosynthesis and related leaf traits in a deciduous forest. Oecologia 96, 169–178. doi: 10.1007/BF00317729
Fajardo, A., and Piper, F. I. (2011). Intraspecific trait variation and covariation in a widespread tree species (Nothofagus pumilio) in southern Chile. New Phytol. 189, 259–271. doi: 10.1111/j.1469-8137.2010.03468.x
Funk, J. L., and Cornwell, W. K. (2013). Leaf traits within communities: context may affect the mapping of traits to function. Ecology 94, 1893–1897. doi: 10.1890/12-1602.1
Gottfried, M., Pauli, H., Futschik, A., Akhalkatsi, M., Barančok, P., Alonso, J. L. B., et al. (2012). Continent-wide response of mountain vegetation to climate change. Nat. Clim. Change 2, 111–115.
Greer, B. T., Still, C., Cullinan, G. L., Brooks, J. R., and Meinzer, F. C. (2018). Polyploidy influences plant–environment interactions in quaking aspen (Populus tremuloides Michx.). Tree Physiol. 38, 630–640. doi: 10.1093/treephys/tpx120
Grime, J. P. (1998). Benefits of plant diversity to ecosystems: immediate, filter and founder effects. J. Ecol. 86, 902–910.
Güsewell, S. (2004). N: P ratios in terrestrial plants: variation and functional significance. New Phytol. 164, 243–266. doi: 10.1111/j.1469-8137.2004.01192.x
Hargreaves, G. H., and Allen, R. G. (2003). History and evaluation of hargreaves evapotranspiration equation. J. Irrig. Drain. Eng. 129, 53–63.
Hayhoe, K., Wake, C. P., Huntington, T. G., Luo, L., Schwartz, M. D., Sheffield, J., et al. (2007). Past and future changes in climate and hydrological indicators in the US Northeast. Clim. Dyn. 28, 381–407.
Holway, J., Scott, J., and Nicholson, S. (1969). Vegetation of the Whiteface Mountain Region of the Adirondacks. Report 92. Albany, NY: Atmospheric Sciences Research Center, 1–49.
Huntington, T. G., Richardson, A. D., McGuire, K. J., and Hayhoe, K. (2009). Climate and hydrological changes in the northeastern United States: recent trends and implications for forested and aquatic ecosystems. Can. J. For. Res. 39, 199–212.
Jung, V., Albert, C. H., Violle, C., Kunstler, G., Loucougaray, G., and Spiegelberger, T. (2014). Intraspecific trait variability mediates the response of subalpine grassland communities to extreme drought events. J. Ecol. 102, 45–53.
Keenan, T. F., and Niinemets, Ü (2016). Global leaf trait estimates biased due to plasticity in the shade. Nat. Plants 3:16201. doi: 10.1038/nplants.2016.201
Körner, C. (2007). The use of ‘altitude’ in ecological research. Trends Ecol. Evol. 22, 569–574. doi: 10.1016/j.tree.2007.09.006
Kramer-Walter, K. R., Bellingham, P. J., Millar, T. R., Smissen, R. D., Richardson, S. J., and Laughlin, D. C. (2016). Root traits are multidimensional: specific root length is independent from root tissue density and the plant economic spectrum. J. Ecol. 104, 1299–1310.
Kuznetsova, A., Brockhoff, P. B., and Christensen, R. H. B. (2017). lmerTest package: tests in linear mixed effects models. J. Stat. Softw. 82, 1–26.
Lenth, R., Singmann, H., Love, J., Buerkner, P., and Herve, M. (2021). emmeans: Estimated Marginal Means, aka Least-Squares Means Version 1.7.1-1.
Luo, Y.-H., Liu, J., Tan, S.-L., Cadotte, M. W., Wang, Y. H., Xu, K., et al. (2016). Trait-based community assembly along an elevational gradient in subalpine forests: quantifying the roles of environmental factors in inter- and intraspecific variability. PLoS One 11:e0155749. doi: 10.1371/journal.pone.0155749
Lusk, C. H. (2004). Leaf area and growth of juvenile temperate evergreens in low light: species of contrasting shade tolerance change rank during ontogeny. Funct. Ecol. 18, 820–828.
Lusk, C. H., Reich, P. B., Montgomery, R. A., Ackerly, D. D., and Cavender-Bares, J. (2008). Why are evergreen leaves so contrary about shade? Trends Ecol. Evol. 23, 299–303. doi: 10.1016/j.tree.2008.02.006
Ma, Z., Guo, D., Xu, X., Lu, M., Bardgett, R. D., Eissenstat, D. M., et al. (2018). Evolutionary history resolves global organization of root functional traits. Nature 555, 94–97.
Mazerolle, M. J. (2020). AICcmodavg: Model Selection and Multimodel Inference Based on (Q)AIC(c) Version 2.3-1.
McCune, B., and Grace, J. B. (2002). Analysis of Ecological Communities. Gleneden Beach, OR: MjM Software Design.
McGill, B., Enquist, B., Weiher, E., and Westoby, M. (2006). Rebuilding community ecology from functional traits. Trends Ecol. Evol. 21, 178–185.
Messier, J., Lechowicz, M. J., McGill, B. J., Violle, C., and Enquist, B. J. (2017a). Interspecific integration of trait dimensions at local scales: the plant phenotype as an integrated network (H Cornelissen, Ed). J. Ecol. 105, 1775–1790.
Messier, J., McGill, B. J., Enquist, B. J., and Lechowicz, M. J. (2017b). Trait variation and integration across scales: is the leaf economic spectrum present at local scales? Ecography 40, 685–697. doi: 10.1002/eap.2064
Midolo, G., Frenne, P. D., Hölzel, N., and Wellstein, C. (2019). Global patterns of intraspecific leaf trait responses to elevation. Glob. Change Biol. 25, 2485–2498. doi: 10.1111/gcb.14646
Nakagawa, S., and Schielzeth, H. (2013). A general and simple method for obtaining R2 from generalized linear mixed-effects models. Methods Ecol. Evol. 4, 133–142. doi: 10.1093/sysbio/syy060
Nicotra, A. B., Atkin, O. K., Bonser, S. P., Davidson, A. M., Finnegan, E. J., Mathesius, U., et al. (2010). Plant phenotypic plasticity in a changing climate. Trends Plant Sci. 15, 684–692.
Niinemets, Ü (2001). Global-scale climatic controls of leaf dry mass per area, density, and thickness in trees and shrubs. Ecology 82, 453–469.
Niinemets, Ü, Keenan, T. F., and Hallik, L. (2015). A worldwide analysis of within-canopy variations in leaf structural, chemical and physiological traits across plant functional types. New Phytol. 205, 973–993. doi: 10.1111/nph.13096
Niklas, K. J., Owens, T., Reich, P. B., and Cobb, E. D. (2005). Nitrogen/phosphorus leaf stoichiometry and the scaling of plant growth. Ecol. Lett. 8, 636–642.
Pakeman, R. J., and Quested, H. M. (2007). Sampling plant functional traits: what proportion of the species need to be measured? Appl. Veg. Sci. 10, 91–96. doi: 10.3389/fpls.2017.00843
Parmesan, C., and Yohe, G. (2003). A globally coherent fingerprint of climate change impacts across natural systems. Nature 421, 37–42. doi: 10.1038/nature01286
Peck, J. (2016). Peck: Multivariate Analysis for Ecologists: Step-By-Step. Glendale Beach, OR: MjM Software Design.
Pérez-Harguindeguy, N., Díaz, S., Garnier, E., Poorter, H., Jaureguiberry, P., Bret-Harte, M. S., et al. (2013). New handbook for standardised measurement of plant functional traits worldwide. Austral. J. Bot. 61, 167–234.
Pettit, J. M., Burton, J. I., DeRose, R. J., Long, J. N., and Voelker, S. L. (2019). Epidemic spruce beetle outbreak changes drivers of Engelmann spruce regeneration. Ecosphere 10:e02912.
Poorter, H., and Nagel, O. (2000). The role of biomass allocation in the growth response of plants to different levels of light, CO2, nutrients and water: a quantitative review. Funct. Plant Biol. 27:595.
PRISM Climate Group, Oregon State U. (2020). Available online at: https://prism.oregonstate.edu/normals/ (Accessed December 5, 2020).
R Core Team (2020). R: A Language and Environment for Statistical Computing. R Foundation for Statistical Computing. Vienna.
Reich, P. B. (2014). The world-wide ‘fast–slow’ plant economics spectrum: a traits manifesto. J. Ecol. 102, 275–301.
Reich, P. B., and Oleksyn, J. (2004). Global patterns of plant leaf N and P in relation to temperature and latitude. Proc. Natl. Acad. Sci. U.S.A. 101, 11001–11006. doi: 10.1073/pnas.0403588101
Reich, P. B., Oleksyn, J., and Wright, I. J. (2010). Evidence of a general 2/3-power law of scaling leaf nitrogen to phosphorus among major plant groups and biomes. Proc. R. Soc. B. 277, 877–883.
Reich, P. B., Walters, M. B., and Ellsworth, D. S. (1997). From tropics to tundra: global convergence in plant functioning. Proc. Natl. Acad. Sci. U.S.A. 94, 13730–13734. doi: 10.1073/pnas.94.25.13730
Reich, P. B., Walters, M. B., Tjoelker, M. G., Vanderklein, D., and Buschena, C. (1998). Photosynthesis and respiration rates depend on leaf and root morphology and nitrogen concentration in nine boreal tree species differing in relative growth rate. Funct. Ecol. 12, 395–405.
Richardson, A. D. (2004). Foliar chemistry of balsam fir and red spruce in relation to elevation and the canopy light gradient in the mountains of the northeastern United States. Plant Soil 260, 291–299.
Roos, R. E., van Zuijlen, K., Birkemoe, T., Klanderud, K., Lang, S. I., Bokhorst, S., et al. (2019). Contrasting drivers of community-level trait variation for vascular plants, lichens and bryophytes across an elevational gradient. Funct. Ecol. 33, 2430–2446.
Ryser, P. (1996). The importance of tissue density for growth and life span of leaves and roots: a comparison of five ecologically contrasting grasses. Funct. Ecol. 10, 717–723.
Schindelin, J., Arganda-Carreras, I., Frise, E., Kaynig, V., Longair, M., Pietzsch, T., et al. (2012). Fiji: an open-source platform for biological-image analysis. Nat. Methods 9, 676–682. doi: 10.1038/nmeth.2019
Shipley, B., De Bello, F., Cornelissen, J. H. C., Laliberté, E., Laughlin, D. C., and Reich, P. B. (2016). Reinforcing loose foundation stones in trait-based plant ecology. Oecologia 180, 923–931. doi: 10.1007/s00442-016-3549-x
Siccama, T. G. (1974). Vegetation, soil, and climate on the green mountains of vermont. Ecol. Monogr. 44, 325–349.
Siefert, A., Violle, C., Chalmandrier, L., Albert, C. H., Taudiere, A., Fajardo, A., et al. (2015). A global meta-analysis of the relative extent of intraspecific trait variation in plant communities (J Chase, Ed). Ecol. Lett. 18, 1406–1419. doi: 10.1111/ele.12508
Spasojevic, M. J., Yablon, E. A., Oberle, B., and Myers, J. A. (2014). Ontogenetic trait variation influences tree community assembly across environmental gradients. Ecosphere 5:art129.
Suding, K. N., Lavorel, S., Chapin, F. S., Cornelissen, J. H., Díaz, S., Garnier, E., et al. (2008). Scaling environmental change through the community-level: a trait-based response-and-effect framework for plants. Glob. Change Biol. 14, 1125–1140.
Umaña, M. N., and Swenson, N. G. (2019). Intraspecific variation in traits and tree growth along an elevational gradient in a subtropical forest. Oecologia 191, 153–164. doi: 10.1007/s00442-019-04453-6
Valladares, F., Matesanz, S., Guilhaumon, F., Araújo, M. B., Balaguer, L., Benito-Garzón, M., et al. (2014). The effects of phenotypic plasticity and local adaptation on forecasts of species range shifts under climate change. Ecol. Lett. 17, 1351–1364. doi: 10.1111/ele.12348
van de Weg, M. J., Meir, P., Grace, J., and Atkin, O. K. (2009). Altitudinal variation in leaf mass per unit area, leaf tissue density and foliar nitrogen and phosphorus content along an Amazon-Andes gradient in Peru. Plant Ecol. Divers. 2, 243–254.
Violle, C., Enquist, B. J., McGill, B. J., Jiang, L. I. N., Albert, C. H., Hulshof, C., et al. (2012). The return of the variance: intraspecific variability in community ecology. Trends Ecol. Evol. 27, 244–252. doi: 10.1016/j.tree.2011.11.014
Wang, T., Hamann, A., Spittlehouse, D., and Carroll, C. (2016). Locally downscaled and spatially customizable climate data for historical and future periods for North America. PLoS One 11:e0156720. doi: 10.1371/journal.pone.0156720
Wason, J. W., and Dovciak, M. (2017). Tree demography suggests multiple directions and drivers for species range shifts in mountains of Northeastern United States. Glob. Change Biol. 23, 3335–3347. doi: 10.1111/gcb.13584
Weiher, E., van der Werf, A., Thompson, K., Roderick, M., Garnier, E., and Eriksson, O. (1999). Challenging theophrastus: a common core list of plant traits for functional ecology. J. Veg. Sci. 10, 609–620.
Weng, E., Farrior, C. E., Dybzinski, R., and Pacala, S. W. (2017). Predicting vegetation type through physiological and environmental interactions with leaf traits: evergreen and deciduous forests in an earth system modeling framework. Glob. Change Biol. 23, 2482–2498. doi: 10.1111/gcb.13542
Westoby, M., Falster, D. S., Moles, A. T., Vesk, P. A., and Wright, I. J. (2002). Plant ecological strategies: some leading dimensions of variation between species. Annu. Rev. Ecol. Syst. 33, 125–159.
White, P. S. (1983). Corner’s rules in eastern deciduous trees: allometry and its implications for the adaptive architecture of trees. Bull. Torrey Bot. Club 110, 203–212.
Wilson, P. J., Thompson, K., and Hodgson, J. G. (1999). Specific leaf area and leaf dry matter content as alternative predictors of plant strategies. New Phytol. 143, 155–162.
Wright, I. J., Dong, N., Maire, V., Prentice, I. C., Westoby, M., Díaz, S., et al. (2017). Global climatic drivers of leaf size. Science 357, 917–921. doi: 10.1126/science.aal4760
Wright, I. J., Reich, P. B., Westoby, M., Ackerly, D. D., Baruch, Z., Bongers, F., et al. (2004). The worldwide leaf economics spectrum. Nature 428, 821–827.
Keywords: climate change, environmental gradients, leaf economic spectrum, functional traits, trait dimensions, intraspecific trait variability (ITV)
Citation: Hecking MJ, Zukswert JM, Drake JE, Dovciak M and Burton JI (2022) Montane Temperate-Boreal Forests Retain the Leaf Economic Spectrum Despite Intraspecific Variability. Front. For. Glob. Change 4:754063. doi: 10.3389/ffgc.2021.754063
Received: 05 August 2021; Accepted: 16 December 2021;
Published: 14 January 2022.
Edited by:
Veronica De Micco, University of Naples Federico II, ItalyReviewed by:
Lara Reale, University of Perugia, ItalyHans Beeckman, Royal Museum for Central Africa, Belgium
Copyright © 2022 Hecking, Zukswert, Drake, Dovciak and Burton. This is an open-access article distributed under the terms of the Creative Commons Attribution License (CC BY). The use, distribution or reproduction in other forums is permitted, provided the original author(s) and the copyright owner(s) are credited and that the original publication in this journal is cited, in accordance with accepted academic practice. No use, distribution or reproduction is permitted which does not comply with these terms.
*Correspondence: Matthew J. Hecking, bWgyNDM2QGNvcm5lbGwuZWR1