- 1Department of Molecular Systems Biology, Helmholtz-Centre for Environmental Research—UFZ GmbH, Leipzig, Germany
- 2Institute for Bioanalysis, Coburg University of Applied Sciences and Arts, Coburg, Germany
- 3Bayreuth Center of Ecology and Environmental Research (BayCEER), University of Bayreuth, Bayreuth, Germany
- 4Forest Entomology, Swiss Federal Research Institute WSL, Birmensdorf, Switzerland
- 5Department of Environmental Systems Science, Institute of Terrestrial Ecosystems, ETH Zürich, Zurich, Switzerland
- 6UNESCO Biosphere Reserve Thuringian Forest, Schmiedefeld am Rennsteig, Germany
- 7Department of Bio- and Environmental Sciences, International Institute Zittau, Technical University of Dresden, Zittau, Germany
- 8Department of Ecology and Ecosystem Management, Technical University of Munich, Freising, Germany
Deadwood provides an important carbon source in forests and wooded ecosystems and, accordingly, forest management strategies discuss the enrichment of deadwood amount and diversity by different tree species. To investigate the decomposition processes of enriched deadwood, we simultaneously placed 3,669 size-standardized and gamma sterilized wood specimens of 13 tree species (Populus tremula, Tilia cordata, Prunus avium, Betula pendula, Carpinus betulus, Fraxinus excelsior, Quercus robur, Fagus sylvatica, Acer platanoides, Larix decidua, Pinus sylvestris, Picea abies, and Pseudotsuga menziesii) at a total of 300 forest and grassland plots in three regions in Germany covering large gradients of management intensity and environmental conditions. After 1 year, mass loss was calculated and its relationship with wood traits and environmental conditions was assessed to determine the most important factors. Mass loss was overall higher in forest compared to grassland habitats, with wood traits as the most important driver, followed by region and environmental factors related to microclimate. However, management intensity was less relevant to explain the mass loss in both habitats. Our results suggest that decomposition of enriched deadwood, even after removal of endophytes, is influenced by the same drivers (positively by moisture and abundance of macronutrients, negatively by lignin and phenol concentration) as naturally occurring wood. Furthermore, due to the immense and standardized experimental setting, our study contributes to a better understanding of the important drivers of mass loss in different tree species and thus provides the basis for predictions of the carbon cycle in a changing world.
Introduction
Temperate and boreal forests store approximately 500 megatons of carbon in above-ground biomass (Myneni et al., 2001). After tree death, much of the carbon remains as deadwood, which is slowly decomposed (Franklin et al., 1987). Decomposition processes of deadwood convert carbon and micronutrients (including nitrogen) that are mainly transferred into the atmosphere in the form of carbon dioxide (CO2) (Kipping et al., 2022), are incorporated into biomass (Harmon et al., 1986) or enter the soil as organic material through leaching (Russell et al., 2015). European forest and grassland environments are governed by anthropogenic management strategies that strongly influence the amounts and quality of coarse woody debris (Josefsson et al., 2010; Ruokolainen et al., 2018). Recent studies recommended strategies of active deadwood enrichment with higher importance on deadwood quality and diversity (Baber et al., 2016; Vogel et al., 2020). Deadwood is an important habitat for saproxylic species and changes the functional composition of these communities (Seibold et al., 2015). Therefore deadwood enrichment promotes the maintenance of forest biodiversity (Doerfler et al., 2018) and studies have already shown positive effects on biodiversity for microorganisms and beetles (Baber et al., 2016; Vogel et al., 2020).
The main important drivers of natural deadwood decomposition were ranked on a global scale, and tree species had the strongest impact, followed by exogenous factors such as climate and location-specific parameters, deadwood size and the openness of canopies (Harmon et al., 2020). Tree species as the main driver of decomposition process was described frequently (Cornwell et al., 2009; Weedon et al., 2009; Freschet et al., 2012; Pietsch et al., 2014; Hu et al., 2018; Parisi et al., 2018) and are based on respective wood traits, which differ in their chemical composition and morphology. Wood traits are more important at the initial deadwood decay while their influence fades along successional decay (Oberle et al., 2020). Wood traits such as nitrogen, sulfur, and magnesium concentration promote deadwood decomposition process, while higher concentrations of extractives, lignin and phenol have an inhibitory effect (Kahl et al., 2017).
Exogenous factors such as edaphic conditions, macro- and microclimate have been shown to shape the occurrence of wood dwelling organisms and deadwood decaying microbial communities, which in turn affect the rate of deadwood decay (Chambers et al., 2000; Zanne et al., 2015). Empirical studies have shown that local environmental conditions, particularly temperature and moisture, exert a strong positive effect on the deadwood decomposition rate (Moore et al., 1999; Kueppers and Harte, 2005). The strong positive correlation between temperature and wood decay is directly linked to microbial community compositions (Bradford et al., 2014), which indirectly alters decomposition processes (Harmon et al., 2020). Moreover, increasing decomposition rates were observed with increasing moisture, as long as the wood moisture content was between 20 and 80% (vol./vol.) (Cornelissen et al., 2012). The effects of temperature and moisture were controversial in different forest ecosystems, indicating a need for further research. Temperature showed the strongest effect on decay rates and tends to be more important than tree species and decomposer diversity in subtropical forests (Pietsch et al., 2019). In turn, moisture had a greater effect in mediterranean forests (Criquet et al., 2002).
Furthermore, habitat structure may affect deadwood decomposition, which is caused directly or indirectly by habitat-related environmental factors. For example, deadwood exposed in forest stands of the same tree species decomposes faster than in forest stands of other tree species, as postulated by the home-field advantage hypothesis (Ayres et al., 2009). In addition, forests with increasing canopy openness showed higher decomposition rates (Janisch et al., 2005; Shorohova and Kapitsa, 2014; Harmon et al., 2020). Open canopies and open habitats such as grasslands lead to increased photodegradation by solar radiation (George et al., 2005) and faster alterations of local weather situations. Few studies examined deadwood decomposition rates across a gradient from mature forest to open land (Dossa et al., 2020), where mass loss and termite infestation of deadwood increased in open tropical montane compared to closed tropical forest sites. In contrast, wood decomposition across a gradient at a forest to shrubland ecotone in temperate regions showed increased microbial community activities near forests, which were decreasing with increasing distance (Smith and Peay, 2021). These correlative approaches provide indications for potential mechanisms, but conclusions about causal relationships are limited. Standardized, systematic field experiments on wood decomposition of multiple tree species under different environmental conditions based on a highly replicated design would allow for a better mechanistic understanding of wood decay but were lacking so far.
Most of Europe is dominated by deciduous broadleaved forests or mixed coniferous and broadleaved forests, but also grassland is partly used as pasturelands and agricultural sites, and open woodland makes up a substantial proportion of the cultural landscape (Bohn et al., 2000). German forests are dominated by the tree species Picea, Pinus, Fagus, and Quercus (BMEL, 2021), with most of these tree species and additionally Acer, Tilia, or Ulmus occur in small proportions in grasslands (Vera et al., 2006; Centeri et al., 2016). Majority of grasslands are the consequence of deforestation (Feurdean et al., 2018), and are partly used as woodland pastures or orchard meadows where trees were left or planted for shading and fruit food source for humans and animals (Centeri et al., 2016). As trees occur in semi-natural grassland, wood decomposition is a relevant process in these habitats which greatly differs from forests in terms of environmental conditions and thus is of great importance to understand the main drivers of carbon cycling.
In this study, we aim to investigate the degradation processes of enriched deadwood from 13 different tree species. In addition, we expect to gain a deeper mechanistic understanding of natural deadwood decomposition concerning wood traits and environmental conditions by using a highly replicated and well-standardized experiment. This is conducted as part of the Biodiversity Exploratories with 300 standardized field plots in three different regions of Germany (Fischer et al., 2010). Each region contains field plots in grassland and forest habitats for which highly resolved data on anthropogenic management intensity and environmental data are available. This enabled us to analyse plot-associated factors on early stage deadwood decomposition processes between both habitat types (grassland and forest habitat) with the same macroclimate and edaphic conditions and under defined gradients of management intensity. Standardized deadwood specimens of 13 tree species were exposed (Lasota et al., 2019; Noll et al., 2019), which minimize the complexity of deadwood and enable a more reliable analysis (Lasota et al., 2019) of factors that govern deadwood mass loss. Moreover, deadwood specimens were sterilized (hereinafter referred to as enriched wood) to eliminate wood-inhabiting endophytes, as these have been found to contribute to the home-field advantage hypothesis in the early deadwood decay phase (Carroll, 1988; Song et al., 2017). Thus, the deadwood specimens of the 13 tree species could only be colonized by the local plot-specific decomposer community. Therefore, we were interested in whether the current biodiversity of each of the 300 plots is equally capable of decomposing the deadwood and what plot-associated factors may explain any deviations. We hypothesize that enriched deadwood undergoes the same decomposing processes as naturally occurring deadwood and, consequently, wood traits are the most important driver of deadwood decay in both habitat types, followed by environmental factors. However, we expect mass loss to be higher in forest habitats compared to grassland habitats, as habitat conditions like temperature, moisture, soil conditions and the presence and abundance of saprotrophic organisms are more favorable in forest habitats.
Materials and methods
Field plots
The study was conducted within the Biodiversity Exploratories, a large-scale and long-term project to investigate the relationship between biodiversity and ecosystem processes in response to land-use intensity. In 2006–2009, three study regions were established in different German protected areas (Figure 1B). For these three regions, a detailed inventory of the forest and grassland habitats was carried out, as well as the forest management type and main tree species or the land use intensity was determined by interviewing farmers and landowners [for additional details, see Fischer et al. (2010)]. To study deadwood decomposition, this work used a total of 300 study plots (100 plots per region, including 50 plots in forest of 100 × 100 m each and 50 plots in grassland of 50 × 50 m each) which provided additional information on biodiversity and were subject to regular environmental monitoring (Figures 1A, C). The size differences between grassland and forest plots are based on the selection criteria of consistency and constancy within a plot.
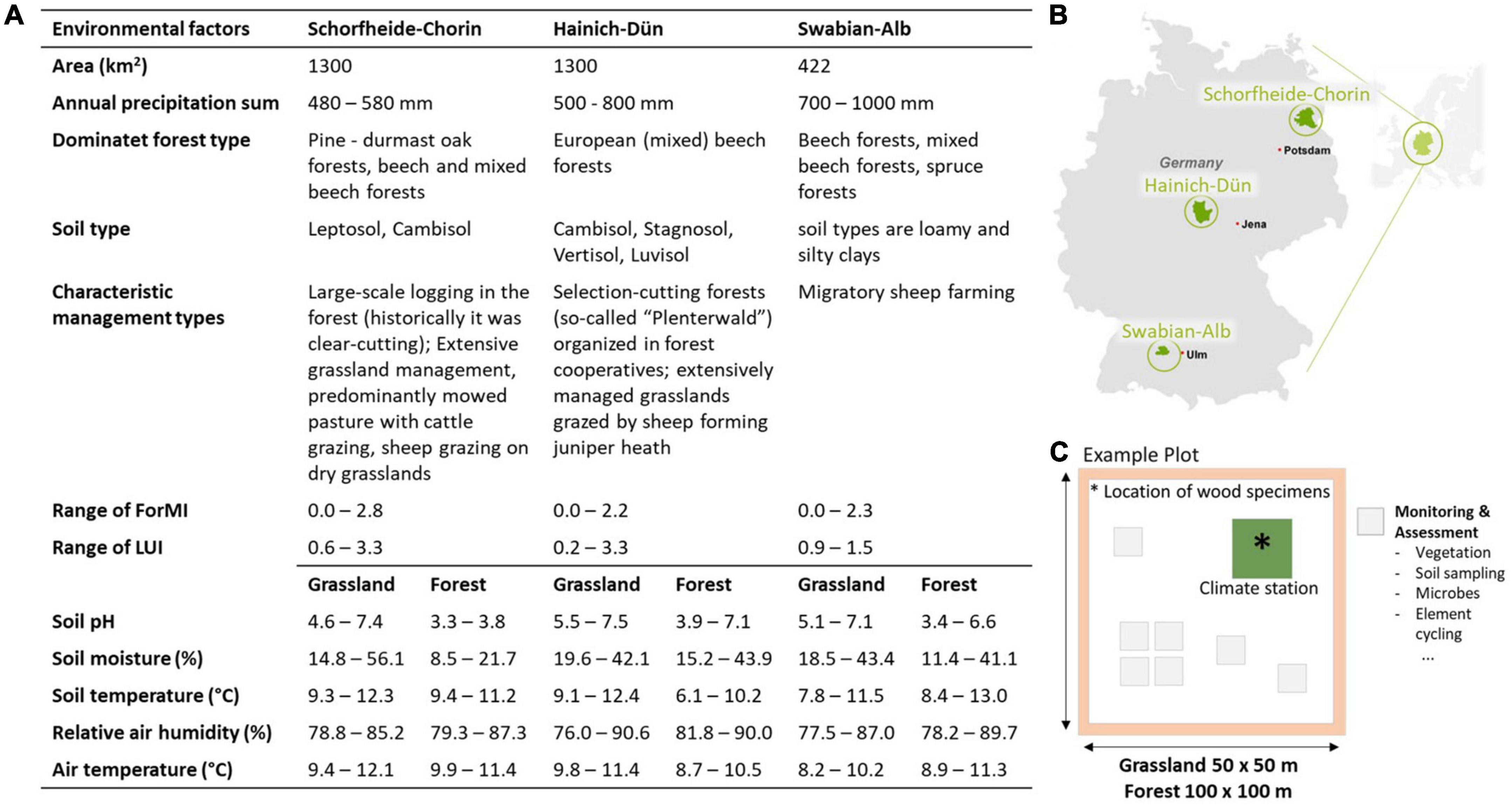
Figure 1. Range of environmental factors (A) and region (B) of the Exploratories Schorfheide-Chorin, Hainich-Dün, and Swabian-Alb in Germany. Each region consists of 50 forest and 50 grassland plots, which are structured in a similar pattern, each with a climate station where all wood specimens were placed (see Supplementary Figure 1) and additional stations for monitoring and assessment (C). LUI, land-use intensity, ForMI: forest management intensity. Details for the indices can be found in Table 1 and Supplementary Tables 2, 3 and the definition of indices by Blüthgen et al. (2012), Kahl and Bauhus (2014).
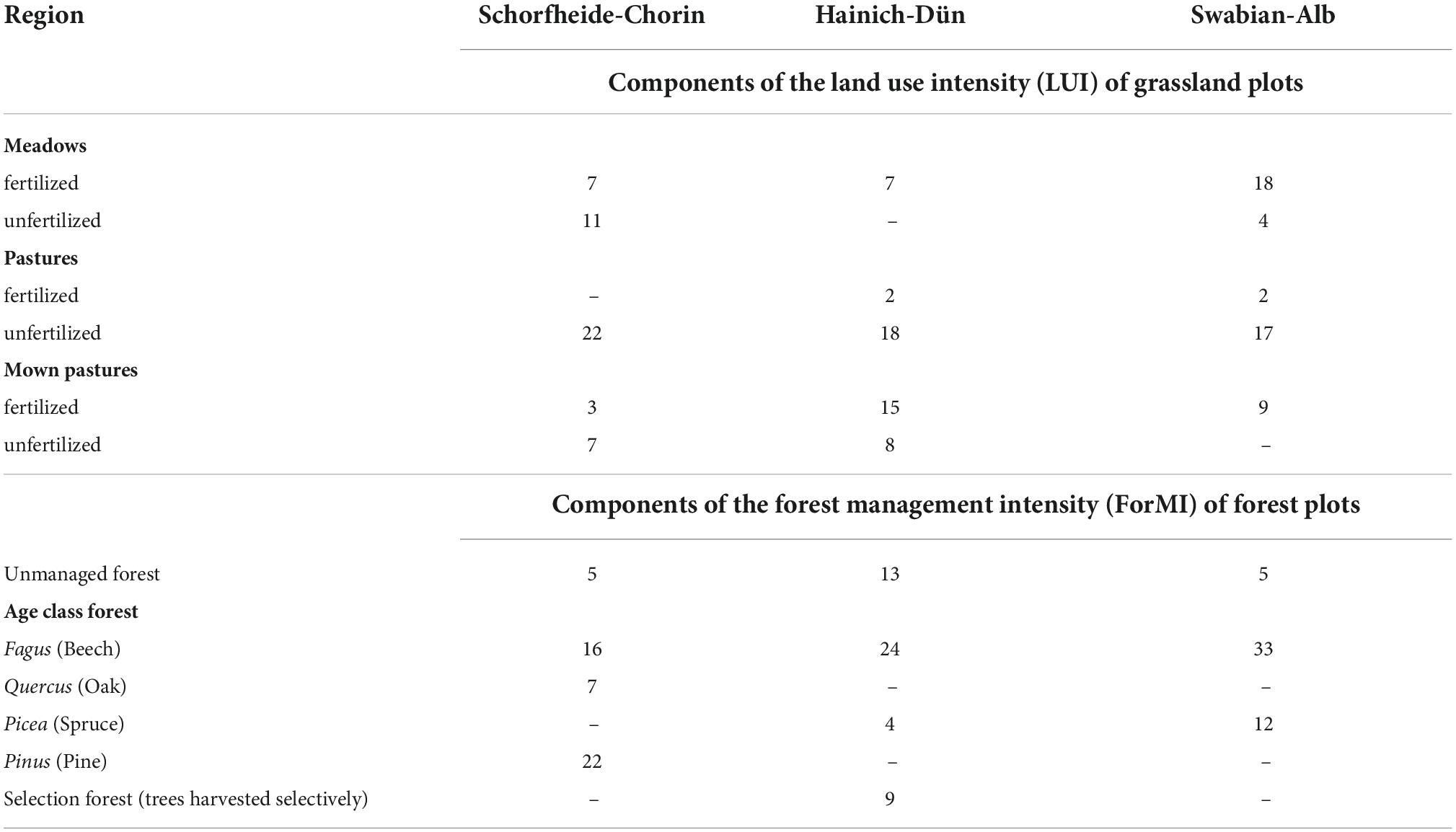
Table 1. Number of individual plots with contrasting land-use and management types for grassland and forest habitats in three regions.
The Exploratory UNESCO Biosphere Reserve Schorfheide-Chorin (52°47′25′′–53° 13′26′′N/13°23′27′′–14°08′53′′E, 3–140 m a.s.l.) is characterized by extensive hilly young moraine landscape with numerous lakes, small bodies of water and bogs, as well as dry sandy knoll with subcontinental dry grassland. Forest range from pine-durmast-oak forest, to mixed beech forest and beech forest. The Exploratory National Park Hainich and surrounding Hainich-Dün area (50°56′14′′–51°22′43′′N/10°10′24′′–10°46′45′′E, 285–550 m a.s.l.) are one of the largest continuous deciduous forest areas that are dominated by European beech forests. Forest areas are surrounded by small settlements, arable land, meadows and pastures and limestone grassland. The Exploratory UNESCO Biosphere Reserve Swabian-Alb (48°20′28′′–48°32′02′′N/9°10′49′′–09°35′54′′E, 460–860 m a.s.l.) is located on sub-montane and montane plateaus. This region is characterized by small-scale mosaic of forests with in-between meadows, sheep pastures and semi-arid grasslands. Forest types range from natural old beech forests, mixed beech-spruce forests and beech or spruce monocultures.
Sample collection
In total, 3,900 deadwood specimens [50 (longitudinal) × 25 × 15 mm] from 13 different tree species were prepared from the outer part of the corresponding tree trunk without bark. To eliminate potential endophytes, all specimens were sterilized in airtight packages by gamma radiation of 70 kGy (Gammaservice, Radeberg, Germany) as outlined earlier (Muszynski et al., 2021). Thereafter each deadwood specimen was precisely weighed under kiln-dried conditions (48 h at 103°C) to record its initial mass (see mass loss). In April 2017, wood specimens (13 tree species × 50 grassland plots and 50 forest plots × 3 regions) were placed above ground so that the transverse surface was exposed to both soil and air. For safety and recovery, the samples were covered with a net (mesh size of 0.5 cm, please see Supplementary Figure 1) and exposed for approximately 1 year in the region Schorfheide-Chorin (397 days), Hainich-Dün (460 days), and Swabian-Alb (414 days). However, 3,669 deadwood specimens were recovered after exposure from a total of 300 specimens per tree species (Populus tremula (n = 280), Tilia cordata (n = 286), Prunus avium (n = 285), Betula pendula (n = 285), Carpinus betulus (n = 293), Fraxinus excelsior (n = 285), Quercus robur (n = 280), Fagus sylvatica (n = 279), Acer platanoides (n = 286), Larix decidua (n = 276), Pinus sylvestris (n = 277), Picea abies (n = 270), and Pseudotsuga menziesii (n = 287) (for details see Supplementary Tables 2, 3).
Mass loss
After exposure, each deadwood specimen was collected from each plot and cleaned of adhering soil and/or fungal mycelium with a brush. Each wood specimen was then separately packed, transported to the laboratory, dried for over 48 h in a climatic chamber at 103 ± 2°C, and then placed in a desiccator (Lasota et al., 2019). Thereafter, the weight of wood specimen was measured, and drying was repeated until a weight constancy was revealed. The percentage mass loss was determined by dividing the dry weight after exposure from the initial dry weight from every single wood specimen.
Environmental factors
Since the beginning of the Biodiversity Exploratories, environmental factors have been recorded on each plot in all three regions (Figure 1). Each plot contains a 3 × 3 m weather documentation system (climate station) in the plot center, where all weather data were collected and also our deadwood specimens were placed (see Figure 1C). These weather data included relative air humidity (%, vol/vol) at 2 m above ground, soil moisture (%, wt/wt) at 10 cm below surface, air temperature (°C) at 10 cm above surface, soil temperature (°C) at 10 cm below surface and soil pH. The average value of each environmental factor per plot was calculated for our exposure year and used for further statistical analyses (see Figure 1 for macroclimate and Supplementary Data Sheet 2 for microclimate).
Management intensities
The forests of the Biodiversity Exploratories include three main management groups: (1) unmanaged, mature forests, which are not used and mostly protected for at least 60 years, (2) uneven-aged selection cutting forest where trees are harvested selectively, and (3) age-class forests, where harvest occur at 80 years intervals for coniferous forests and 100–120 years intervals for beech forests (Table 1). For anthropogenic management intensity calculation, the forest plots were characterized by the combined forest management index ForMI (Kahl and Bauhus, 2014), and its components: proportion of harvested wood volume (Iharv), proportion of tree species that are not part of the natural community (Inonat), and proportion of deadwood including saw cuts (Idwcut). Each component was ranked in a range between 0 (no sign of management) and 1 (intensive management) and then summarized to the ForMI.
Grasslands are divided into three main categories of land use: (1) meadows, (2) pastures and (3) mown pastures, and were characterized by the combined land-use intensity index LUI (Blüthgen et al., 2012). The LUI summarizes the standardized intensity of the components of total grazing, total mowing, and total fertilization.
Tree species-specific wood traits
As part of the Biodiversity Exploratories, Kahl et al. (2017) analysed the wood properties of the 13 tree species used in this study (Kahl et al., 2017). To test the potential effect of wood traits in detail, concentrations of carbon, nitrogen, phosphorous, sulfur, potassium, calcium, magnesium, manganese, organic extractives, phenol, and lignin were taken from Kahl et al. (2017), which calculated the average value per tree species (Supplementary Table 1). In addition, initial wood density was analysed within this study (see above section “Sample collection”) by measuring the weight of each deadwood specimen before and after exposure at kiln-dried conditions. Our wood density findings were in line with previous findings (Fengel and Wegener, 2011).
Statistical analyses
The statistical analyses and data visualization were performed using R v4.0.2 (R Core Team, 2016). In the first step (Model 1), we tested for the total effect of tree species, habitat type (forest and grassland), region (Schorfheide-Chorin, Swabian-Alb, and Hainich-Dün), and anthropogenic management intensities (LUI and ForMI) on mass loss. Therefore, we conducted linear mixed effects models using the lmer function in lmerTest package (Kuznetsova et al., 2017). For the first model, mass loss was used as response factors. Tree species, habitat type, region and management intensity and their interaction were used as fixed factors and PlotID as random factor. Anthropogenic management intensity values in forest and grassland habitats were normalized within each habitat type to achieve comparable scales. Continuous factors were standardized (scaled to zero mean and unit variance) using the decostand function in the vegan package (Oksanen et al., 2017). The first model results were presented as type III ANOVA (analysis of variance) table with p-values for F-tests after single term deletions (drop1 function) and by using Satterthwaite’s method for denominator degrees of freedom. Based on these model results, the adjusted mean effect size and corresponding 95% confidence intervals, were additionally generated with emmeans function in the package emmeans (Lenth et al., 2018) and illustrated with the field observed data using the ggplot2 package (Wickham et al., 2016). Associated significances were also performed using the emmeans function and denominator degrees of freedom are determined based on Satterthwaite’s method.
To examine in detail the influencing drivers underlying the overall effects, the second step (Model 2) assessed the importance of wood traits, environmental factors, region, and management intensity for mass loss to subsequently determine their estimated effect size. Accordingly to the strong influence of habitat types, all of the following analyses of the second model were conducted separately for forest and grassland habitats. To avoid multicollinearity, we first evaluated the Spearman correlations among all fixed-effect factors and excluded one of each factor pair with a correlation >0.7 (Tabchnick and Fidell, 1996). As potassium and sulfur, calcium and phosphorus, and carbon and phosphorus concentration were highly correlated (0.91, 0.73, and −0.78, respectively) only sulfur, calcium and carbon concentrations were kept for subsequent analyses. Thereafter, we used all selected variables and conducted separate models based on the combined management intensities ForMI/LUI and its components. These separate models resulted in the following variables used in the global model: ForMI (Iharv, Inonat, Idwcut)/LUI (grazing, mowing, fertilization), main tree species, wood density, carbon, nitrogen, sulfur, calcium, magnesium and lignin concentration, soil pH, relative air humidity, soil moisture, air temperature, and soil temperature. In a second step, we applied the multimodel inference approach as proposed by Grueber et al. (2011) based on a global model (lmer) including all selected and standardized (see above) fixed-effect factors with mass loss as a dependent factor and PlotID and TreeSpecies as random effects. We conducted automated model selection with subsets of the global model by the dredge function based on AICc (small-sample-size corrected version of Akaike information criterion) in the R package MuMIn (Barton, 2020). We subsequently selected the best models based on ΔAIC < 2 (substantial support) and ΔAIC < 4 and ΔAIC < 7 (some support) as suggested by Burnham et al. (2011) using the get.models function. The relative importance of each independent factor was assessed by calculating the cumulative Akaike weights of models containing a particular predictor. In the last step, we used all factors with an importance value >0.75 in a final model with a model structure like the global model to calculate their estimated effect size. The model estimates of the important fixed effects were plotted using the package sjPlot (Lüdecke, 2020). Additionally, to determine the predictive capacity of all models, the R-Squared was calculated by using r2_nakagawa function (Lüdecke et al., 2020).
Results
Decay rate of 13 different tree species differed in grassland and forest plots (model 1)
Mass loss differed significantly among tree species (Figure 2). Region, tree species identity and habitat type, and the interactions with tree species were the important drivers of mass loss (Table 2). The effect of tree species identity on mass loss strongly depended on region, habitat type, and anthropogenic management intensity (Table 2), indicating that the effect of tree species was affected by these environmental factors to varying degrees. Moreover, anthropogenic management intensity alone did not show a significant effect on mass loss (Table 2), nor the interaction of habitat type and management intensity (Table 2). In summary, the first model after single-term deletion had a prediction capacity of 65%, including the interactions (Supplementary Table 5).
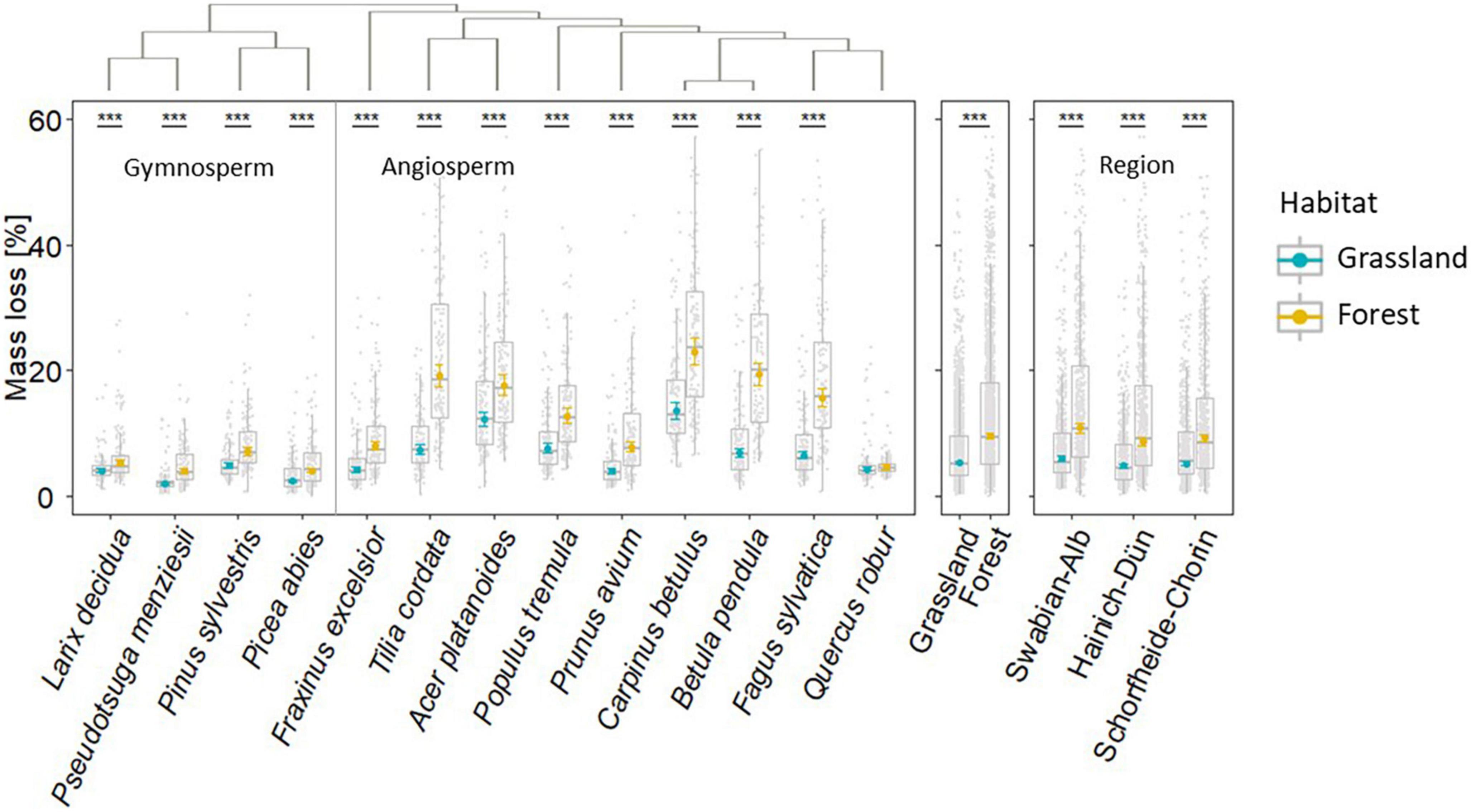
Figure 2. Mass loss of deadwood of 13 temperate tree species exposed in 150 grassland and 150 forest habitats (n = 3,669). Gray dots indicates measured field observation data and yellow/blue symbols the model-adjusted mean and 95% confidence intervals of each mean. Plot details were summarized in materials and methods section. Significant differences in mass loss within, between grassland and forest habitats and regions based on linear mixed effect model using Satterthwaite’s method (***p ≤ 0.001). Tree species-specific phylogenetic information (above mass loss) was taken from Kahl et al. (2017). For detailed significance results see Table 2 and Supplementary Table 4.
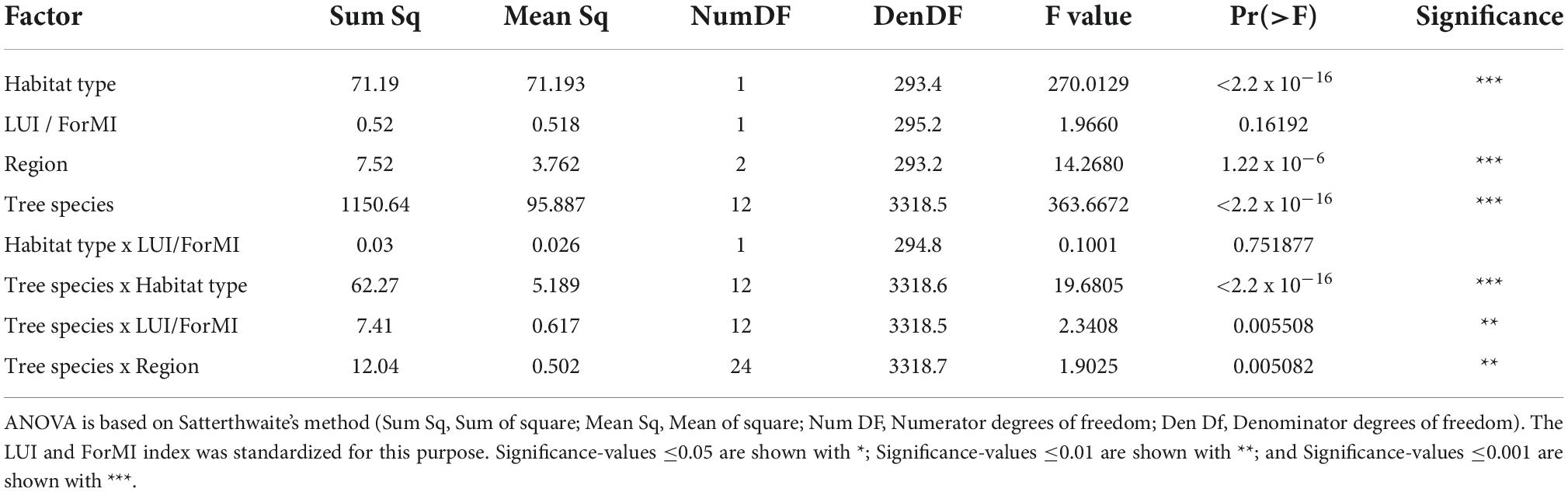
Table 2. Results of linear mixed effect model for regions (Schorfheide-Chorin, Swabian-Alb, and Hainich-Dün), habitat types (grassland and forest), management intensity (LUI, land use intensity index or ForMI, forest management intensity) and their interactions on the mass loss of deadwood from 13 temperate tree species (Model 1; n = 3669).
Mass loss of all tree species was significantly higher in forest than in grassland habitats (Figure 2). The four gymnosperms L. decidua, P. sylvestris, P. abies, and P. menziesii had a much lower mass loss in grassland as well as in forest habitats than the majority of angiosperm trees (Figure 2 and Supplementary Table 4). In addition, Q. robur and P. menziesii had the lowest mass loss in forest and grassland habitats, respectively. In contrast, A. platanoides and C. betulus had the highest mass loss in grassland habitats, while C. betulus, A. platanoides and B. pendula had the highest mass loss in forest habitats.
Degree of mass loss in each habitat type is linked to region, plot specific and tree species-specific traits (model 2)
After reducing the variables following a model average procedure separately for each habitat, we investigated important factors of regions, tree species-specific traits as well as plot-associated environmental factors (weather and edaphic conditions) as the main drivers of mass loss in both habitat types (Figures 3, 4). Based on ΔAIC < 2 region, relative air humidity, soil pH, wood density and concentration of lignin, carbon, calcium, sulfur and phenol showed high importance in forests, whereas region, soil moisture, and concentration of lignin, carbon, calcium and sulfur were most important in grassland habitats (Figure 3 and Supplementary Figures 2–5). Based on the second model including these factors, mass loss was positively affected by the wood traits such as carbon, sulfur and calcium concentrations in a similar pattern for both habitat types (Figure 4 and Table 3). Moreover, high phenol concentrations and wood density were positively associated with mass loss, but negatively with soil pH in forest habitats (Figure 4 and Table 3). In addition, mass loss was positively associated with soil moisture in grasslands and relative air humidity in forest habitats. In both habitat types, mass loss was negatively affected by lignin concentration, and was lower in the regions Hainich-Dün and Schorfheide-Chorin compared to Swabian-Alb. Mass loss in Hainich-Dün was higher than in Schorfheide-Chorin in grasslands, but lower in forests (Figures 2, 4 and Table 3). The final model had a predictive capacity of 62 and 61% for grassland and forest habitats, respectively (Supplementary Table 5).
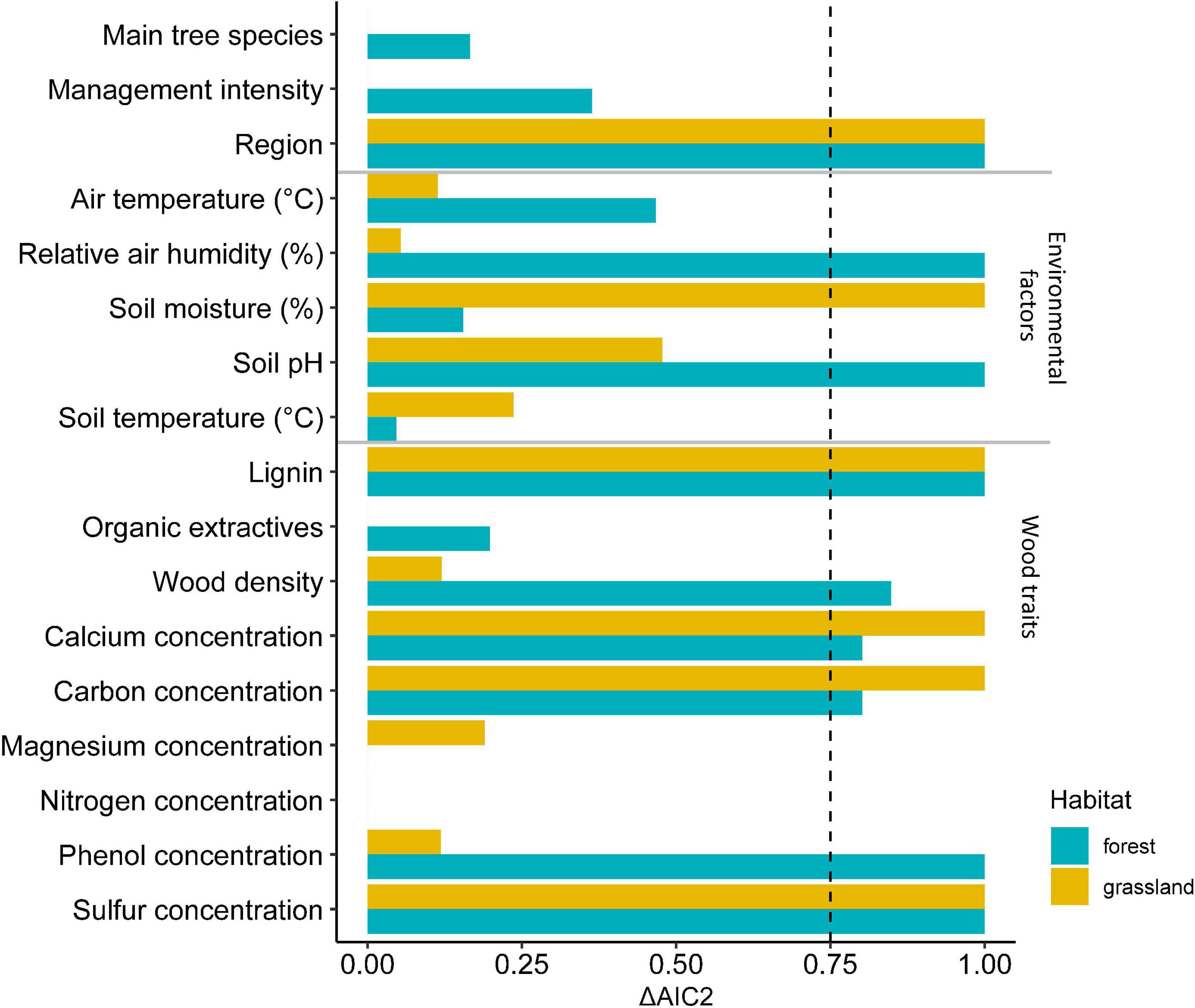
Figure 3. Importance factors (assessed by the cumulative Akaike information criterion (AIC) weights of models containing a particular predictor) of fixed variables in explaining decay rates of the 13 different tree species based on model averaging with ΔAIC < 2. Values are shown separately for grassland and forest habitat and used with an importance value > 0.75 in a final model (Figure 4). For details see Supplementary Figures 2–5 in the Supplementary material.
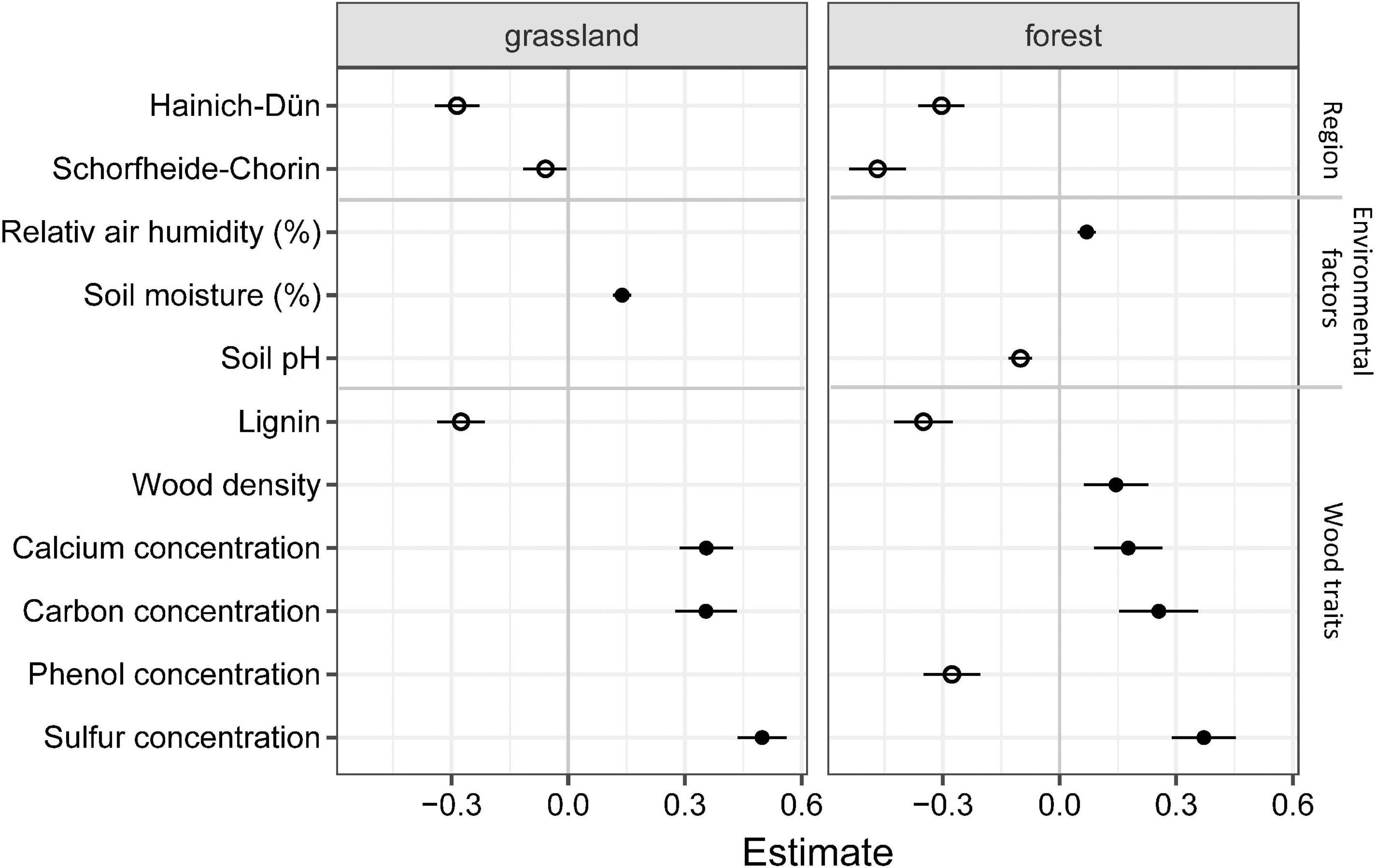
Figure 4. Model estimates of the final linear mixed effect model (Model 2) to elucidate drivers of mass loss in forest and grassland habitats. Model-averaging procedure based on Akaike information criterion (AIC) and calculation for selection of importance factors (value > 0.75; details see Supplementary Figures 2–5). Effect mean size and confidence intervals are indicated for the parameters included in the model set (all models included). Detailed results of the final model are shown in Table 3.
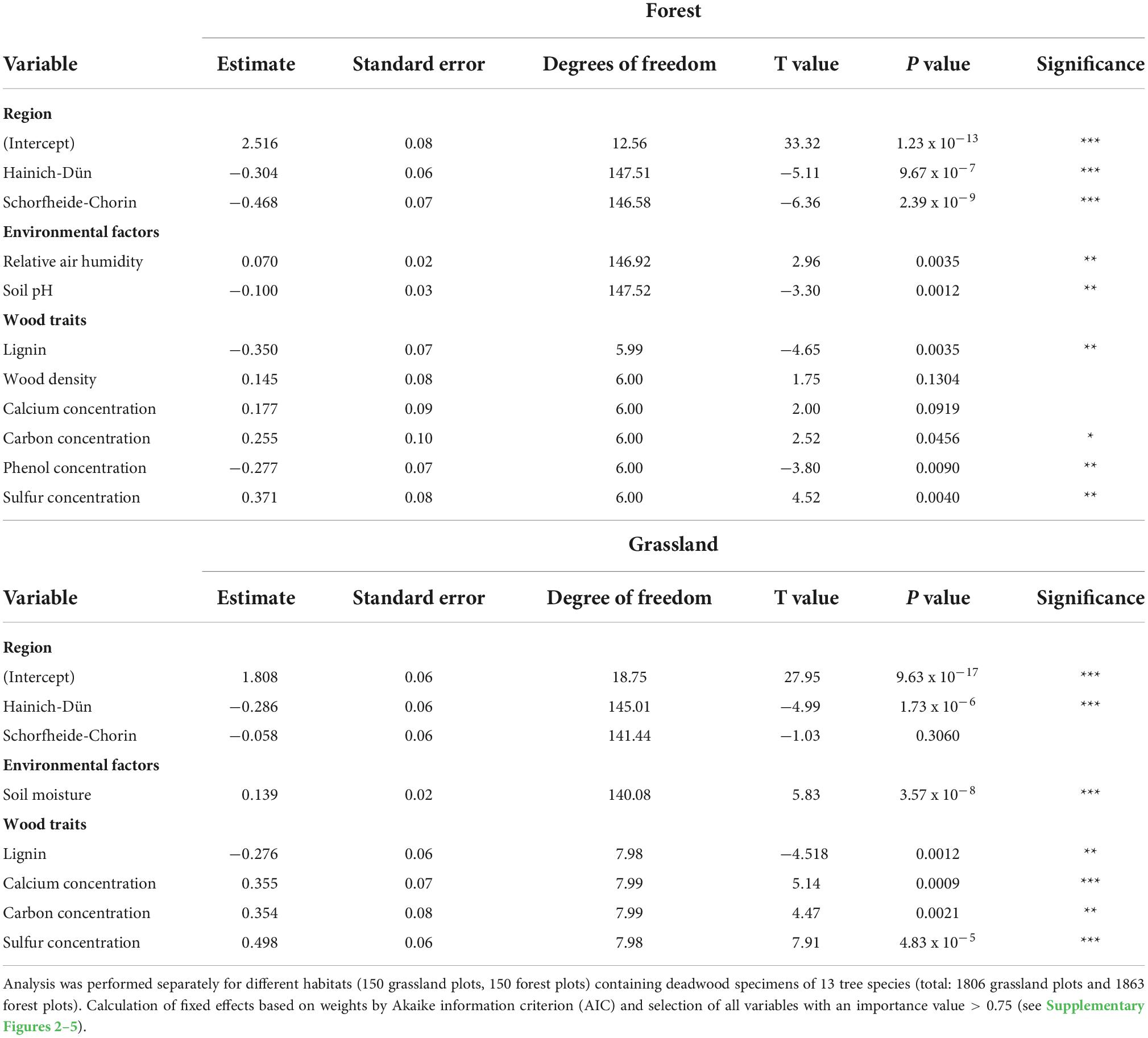
Table 3. Results of final models for importance variables of tree species traits, environmental factors and land-use intensity on mass loss (Model 2).
Discussion
This study serves to investigate decomposition of enriched deadwood and validate reported drivers of mass loss by using a highly replicated experimental setting. The experiment was carried out in all three regions during the same time frame, so that seasonal cycle was similar for all samples. The results revealed, that the rate of enriched deadwood decay is most strongly affected by tree species due to different respective wood traits, regardless of habitat type, i.e., forest and grassland and region. In addition, mass loss is shaped by environmental factors differing between habitat type and region. In contrast, management intensity was less important for mass loss (Table 2). These findings indicate similar decomposing processes for enriched and naturally occurring deadwood and support previous observational studies conducted in forest habitats. Furthermore, it shows their generalizability of processes across habitats and regions and thus contributes substantially to a better mechanistic understanding of the drivers of deadwood decomposition.
Globally, microbes are the key deadwood decay agents (Lee et al., 2020) and they are already partly present as endophytes in many tree species (Magaudda et al., 2001; Song et al., 2017; Purahong et al., 2019). Gamma sterilization was applied to all standardized deadwood specimens, which is a very effective treatment to inactivate all endophytes and other wood inhabiting organisms (Sharman and Smith, 1970; Freitag and Morrel, 1998). Therefore, the decomposing substrates were only exposed to saprotrophic communities without any prior presence of endophytes. Cline and colleagues showed that the effect of endophytes was more pronounced in the early stage of deadwood decay than in the late stage (Cline et al., 2018), indicating the priority effect of pivotal importance in the early stage of deadwood decay. Since our study addresses the early stages of decay, gamma radiation ensured a valuable method to establish equal initial conditions.
Early stage deadwood degradation was more efficient in forest than in grassland habitats (Figure 2 and Table 2), indicating that the functional guilds for deadwood decay were also present in grassland, but more abundant or with increased activity in forest habitats as described earlier in the same environments (Kaiser et al., 2016). According to the home-field advantage hypothesis tree species-specific decomposer communities were more frequent in forests with the same tree species identity than in forests of other tree species identities (Purahong et al., 2019), indicating that the presence and abundances also in forest habitats are unequally distributed. Moreover, a recent study revealed that these 13 tree species were mainly resource-specific colonized by a defined set of fungi and prokaryotes as revealed by bipartite interaction networks in forest habitats (Moll et al., 2021). The presence of specialized deadwood decaying fungi is of higher importance for deadwood decay than respective accompanying prokaryotic community compositions (Moll et al., 2021). Therefore, the colonization patterns of deadwood decomposing microorganisms are of pivotal importance for mass loss. While the effect of the deadwood decaying fungal communities during the conversion from forest habitats to grassland habitats by diebacks (Fukasawa et al., 2019) or after wildfires (Molinas-González et al., 2017) has been studied, knowledge on deadwood decay in grassland, which is less connected to the forest source of wood decomposing organisms, is so far scarce. Recently a study on the same Swabian-Alb plots showed that fungi were superior in the colonization of mineral grassland plots. These fungi were more efficient to incorporate dead root-derived carbon into their biomass compared to bacterial community members (Kandeler et al., 2019). Therefore, a broad diversity of fungal decomposing community members is also present in these grassland habitats but less productive in the deadwood decomposition. However, to assess these decomposing microorganisms exact relevance and productivity in grassland, further studies for phylogenetic identity and productivity are required.
The variance in deadwood mass loss within tree species across plots was much higher in the forest habitats than in the grassland habitats (Figure 2). As the experimental setting was similar for all sterilized deadwood specimens, this can be mainly attributed to plot-specific colonization patterns of deadwood decomposing microorganisms and their biotic interactions (Figure 4). Interestingly the variability in mass loss of gymnosperms was lower compared to angiosperms in both habitat types (except for Q. robur) (Figure 2), which is in line with the annual decay rate of similar wood logs exposed at the same plots (Kahl et al., 2017).
Besides, mass loss at the early decay stage showed region-based differences. The mean mass loss and estimated effect size were higher in both forest and grassland habitats of Swabian-Alb than those of Schorfheide-Chorin or Hainich-Dün, but was more similar to each other in grassland habitats (Figures 2, 4). Swabian-Alb had the highest precipitation rates, followed by Hainich-Dün and Schorfheide-Chorin leading to higher soil moisture and air humidity. As the region Schorfheide-Chorin is placed on sandy soils with moraines, the water holding capacity is lower compared to Hainich-Dün or Swabian-Alb. Soil moisture in grassland and air humidity in forest habitats were positive drivers of mass loss (Figure 4), which is in line with previous studies as increased moisture content had a linear or almost linear relationship to mass loss of deadwood (Kubartová et al., 2015; Zelinka et al., 2020). Moreover, increasing soil pH was a negative driver for mass loss in forest habitats, which was attributed in another study for leaching from phenolic compounds of silver fir and beech deadwood (Stutz et al., 2017).
Surprisingly, anthropogenic management intensity showed no effect in the early decay stages, neither overall nor separately for forest or grassland habitats (Figure 4 and Table 2). Because fresh wood is usually colonized by a large set of organisms and biodiversity is directly affected by management intensity (Bässler and Müller, 2010), we expected a biodiversity-based effect of management intensity. Moreover, the decomposition rate is strongly affected by microbial activity in the early stage of deadwood decay, which in turn depends on environmental factors (Kueppers and Harte, 2005). Therefore, even if the biodiversity was not changed in each plot an activity-based effect of management intensity was expected subordinate effect. It is possible, that these effects would be minimized by prior sterilization of the deadwood samples.
As tree species was an important driver of mass loss (Table 2), the majority of tree species-specific traits were also significantly correlated to mass loss (Figure 4 and Table 3), which was shown previously (Kahl et al., 2017). Lignin concentration was negatively correlated to mass loss in both habitat types (Figure 4), supporting early research on deadwood decomposition (Meentemeyer, 1978). Lignin is a recalcitrant carbon compound and requires specific enzymatic cleavage for its degradation, which is mainly produced by members of the division Basidiomycota (Janusz et al., 2017). Also, the quality of lignin addressed as phenol concentration was negatively correlated in forest habitats (Figure 4), which is in line with previous findings (Talbot et al., 2012; Kahl et al., 2017). In turn, carbon concentration was positively correlated to mass loss in both habitats (Figure 4). As deadwood decomposing communities gain their energy by carbon metabolism, a positive correlation to carbon concentration is evident. In the early stages of decomposition, readily degradable and accessible carbon compounds are primarily degraded, promoting a positive correlation with total carbon concentration. Lignin, on the other hand, is degraded less rapidly and conversion rates are known to be low, explaining the opposite effects of lignin content on mass loss. In addition, other macronutrients such as sulfur and calcium also had a positive effect on mass loss. Sulfur and compounds like cysteine, sulfonates and sulfate are known to increase fungal mycelium growth and proliferation, which could support a higher decomposition rate (Schmalenberger et al., 2011; Kahl et al., 2017), but further information is required to fully understand the underlying processes. An increased calcium concentration contribute to enzyme activities and osmoregulation in microorganisms, indicating that these concentrations are rate-limiting for deadwood decay. As deadwood is composed of a large set of abiotic tree species-specific traits, single parameters can hardly be disentangled from each other. Additionally, mass loss in forest habitats correlated positively with wood density (Figure 4), as already found previously (Kahl et al., 2017; Hu et al., 2018). Such relationship between mass loss and high wood density changes during decomposition stages (Oberle et al., 2020). Since the higher mass loss in forest habitats is associated with advancing decomposition, the positive relationship with wood density could already be observed here, while nothing was yet visible in grassland. In general, mass loss was lower in grassland than in forest habitats, but was influenced by similar wood traits as well as environmental factors.
Conclusion
The paradigm that tree species-specific wood traits are of pivotal importance for decay and hence mass loss (Lee et al., 2020; Oberle et al., 2020) is supported and extended by our study to more tree species and more habitats, i.e., forest and grassland plots. Therefore, the generalized findings from forest habitats can also be used to predict mass loss in grassland habitats, which was to the best of our knowledge, not addressed in such a highly replicated study so far.
In addition, a detailed analysis of decomposition drivers revealed the same direction of influence factors for enriched deadwood compared to naturally occurring deadwood (Kahl et al., 2017). Environmental factors such as temperature and moisture and wood traits such as macronutrients were positively related to accelerated deadwood decay, while lignin and phenol were negatively related. Future research should clarify which microbial communities are able to colonize enriched deadwood to link phylogenetic identity to ecosystem service productivity, which will help predict future management strategies in a world with biodiversity loss and climate change.
Data availability statement
The original contributions presented in this study are included in the article/Supplementary material, further inquiries can be directed to the corresponding author.
Author contributions
MN, NJ, HK, and TK conceived the ideas and designed methodology. FM, NJ, SM, and MN collected the data. LK, FM, and MG analysed the data. MN, LK, and MG led wrote the manuscript. WW contributed by additional funding and proofreading of the manuscript. All authors contributed critically to the drafts and gave final approval for publication.
Funding
This work was funded by the DFG Priority Program 1374 “Infrastructure-Biodiversity-Exploratories” (grant numbers: NO 834/5-4 and JE 724/7-4).
Acknowledgments
We thank the managers of the three Exploratories, Kirsten Reichel-Jung, Katrin Lorenzen, Miriam Teuscher, and all former managers for their work in maintaining the plot and project infrastructure; Christiane Fischer and Jule Mangels for giving support through the central office, Michael Owonibi for managing the central data base, and Markus Fischer, Eduard Linsenmair, Dominik Hessenmöller, Daniel Prati, Ingo Schöning, François Buscot, Ernst-Detlef Schulze, and the late Elisabeth Kalko for their role in setting up the Biodiversity Exploratories project. We thank the administration of the Hainich national park, the UNESCO Biosphere Reserve Swabian-Alb and the UNESCO Biosphere Reserve Schorfheide-Chorin as well as all landowners for the excellent collaboration. Field work permits were issued by the responsible state environmental offices of Baden-Württemberg, Thüringen, and Brandenburg. We thank Björn Hoppe and Claus Bässler for fruitful discussions. For support by field work, sample preparation, and analysis we thank Rahel Bauer, Sophia Bender, Anna Danzberger, Jessy-Lee Fournes, Celina Gern, Carolin Gold, Viktoria Göbel, Christof Hartung, Christian Heby, Nicholas Holzscheck, Maik Klaedtke, Ines Kleemeier, Viviane Kraus, Michael Langer, Gina-Marie Meyer, Sarah Neuroth, Debora Obergfäll, Gülsan Önder, Nicola Pfeuffer, Maike Reimann, Roxana Röthing, Christoph Rudisch, Isabel Sandner, Henry Scheibel, Felix Schneider, Johanna Stenglein, Tessa Wolter, and Fabienne Zapf.
Conflict of interest
The authors declare that the research was conducted in the absence of any commercial or financial relationships that could be construed as a potential conflict of interest.
Publisher’s note
All claims expressed in this article are solely those of the authors and do not necessarily represent those of their affiliated organizations, or those of the publisher, the editors and the reviewers. Any product that may be evaluated in this article, or claim that may be made by its manufacturer, is not guaranteed or endorsed by the publisher.
Supplementary material
The Supplementary Material for this article can be found online at: https://www.frontiersin.org/articles/10.3389/ffgc.2022.1020737/full#supplementary-material
References
Ayres, E., Steltzer, H., Simmons, B. L., Simpson, R. T., Steinweg, J. M., Wallenstein, M. D., et al. (2009). Home-field advantage accelerates leaf litter decomposition in forests. Soil Biol. Biochem. 41, 606–610. doi: 10.1016/j.soilbio.2008.12.022
Baber, K., Otto, P., Kahl, T., Gossner, M. M., Wirth, C., et al. (2016). Disentangling the effects of forest-stand type and dead-wood origin of the early successional stage on the diversity of wood-inhabiting fungi. For. Ecol. Manag. 377, 161–169. doi: 10.1016/j.foreco.2016.07.011
Bässler, C., and Müller, J. (2010). Importance of natural disturbance for recovery of the rare polypore Antrodiella citrinella Niemela & Ryvarden. Fungal biol. 114, 129–133. doi: 10.1016/j.funbio.2009.11.001
Blüthgen, N., Dormann, C. F., Prati, D., Klaus, V. H., Kleinebecker, T., Hölzel, N., et al. (2012). A quantitative index of land-use intensity in grasslands: Integrating mowing, grazing and fertilization. Basic Appl. Ecol. 13, 207–220. doi: 10.1016/j.baae.2012.04.001
BMEL (2021). The forests in Germany. Selected results of the third national inventory. Available online at: https://www.bmel.de/SharedDocs/Downloads/EN/Publications/german-forests.pdf?__blob=publicationFile&v=7 (accessed October 30, 2022).
Bohn, U., Neuhäusl, R., Gollub, G., Hettwer, C., Neuhäusloá, Z., Raus, T., et al. (eds) (2000). Karte der natürlichen vegetation Europas/map of the natural vegetation of Europe. Maßstab/scale 1: 2 500 000. Münster: Landwirtschaftsverlag.
Bradford, M. A., Warren, I. I. R. J., Baldrian, P., Crowther, T. W., Maynard, D. S., Oldfield, E. E., et al. (2014). Climate fails to predict wood decomposition at regional scales. Nat. Clim. Change 4, 625–630. doi: 10.1038/nclimate2251
Burnham, K. P., Anderson, D. R., and Huyvaert, K. P. (2011). AIC model selection and multimodel inference in behavioral ecology: Some background, observations, and comparisons. Behav. Ecol. Sociobiol. 65, 23–35. doi: 10.1007/s00265-010-1029-6
Carroll, G. (1988). Fungal endophytes in stems and leaves: From latent pathogen to mutualistic symbiont. Ecology 69, 2–9. doi: 10.2307/1943154
Centeri, C., Renes, H., Roth, M., Kruse, A., Eiter, S., Kapfer, J., et al. (2016). Wooded grasslands as part of the European agricultural heritage. Biocult. Divers. Eur. 5, 75–103. doi: 10.1007/978-3-319-26315-1_4
Chambers, J. Q., Higuchi, N., Schimel, J. P., Ferreira, L. V., and Melack, J. M. (2000). Decomposition and carbon cycling of dead trees in tropical forests of the central Amazon. Oecologia 122, 380–388. doi: 10.1007/s004420050044
Cline, L. C., Schilling, J. S., Menke, J., Groenhof, E., Kennedy, P. G., and Gallery, R. (2018). Ecological and functional effects of fungal endophytes on wood decomposition. Funct. Ecol. 32, 181–191. doi: 10.1111/1365-2435.12949
Cornelissen, J. H., Sass-Klaassen, U., Poortler, L., Geffen, K. V., Logtestijn, R. S. P. V., Hal, J. V, et al. (2012). Controls on coarse wood decay in temperate tree species: Birth of the LOGLIFE experiment. AMBIO 41, 231–245. doi: 10.1007/s13280-012-0304-3
Cornwell, W. K., Cornelissen, J. H. C., Allison, S. D., Bauhus, J., Eggleton, P., Preston, C. M., et al. (2009). Plant traits and wood fates across the globe: Rotted, burned, or consumed? Glob. Change Biol. 15, 2431–2449. doi: 10.1111/j.1365-2486.2009.01916.x
Criquet, S., Tagger, S., Vogt, G., and Le Petit, J. (2002). Endoglucanase and β-glycosidase activities in an evergreen oak litter: Annual variation and regulating factors. Soil Biol. Biochem. 34, 1111–1120. doi: 10.1016/S0038-0717(02)00045-7
Doerfler, I., Gossner, M. M., Müller, J., Seibold, S., and Weisser, W. W. (2018). Deadwood enrichment combining integrative and segregative conservation elements enhances biodiversity of multiple taxa in managed forests. Biol. Conserv. 228, 70–78. doi: 10.1016/j.biocon.2018.10.013
Dossa, G. G., Paudel, E., Schaefer, D., Zhang, J.-L., Cao, K.-F., Xu, J.-C., et al. (2020). Quantifying the factors affecting wood decomposition across a tropical forest disturbance gradient. For. Ecol. Manag. 468:118166. doi: 10.1016/j.foreco.2020.118166
Fengel, D., and Wegener, G. (2011). Wood: Chemistry, ultrastructure, reactions. Berlin: Walter de Gruyter.
Feurdean, A., Ruprecht, E., Molnár, Z., Hutchinson, S. M., and Hickler, T. (2018). Biodiversity-rich European grasslands: Ancient, forgotten ecosystems. Biol. Conserv. 228, 224–232. doi: 10.1016/j.biocon.2018.09.022
Fischer, M., Bossdorf, O., Gockel, S., Hänsel, F., Hemp, A., Hessenmöller, D., et al. (2010). Implementing large-scale and long-term functional biodiversity research: The biodiversity exploratories. Basic Appl. Ecol. 11, 473–485. doi: 10.1016/j.baae.2010.07.009
Franklin, J. F., Shugart, H. H., and Harmon, M. E. (1987). Tree death as an ecological process. BioScience 37, 550–556. doi: 10.2307/1310665
Freitag, C. M., and Morrel, J. J. (1998). Use of gamma radiation to eliminate fungi from wood. For. Prod. J. 48, 76–78.
Freschet, G. T., Weedon, J. T., Aerts, R., van Hal, J. R., and Cornelissen, J. H. C. (2012). Interspecific differences in wood decay rates: Insights from a new short-term method to study long-term wood decomposition. J. Ecol. 100, 161–170. doi: 10.1111/j.1365-2745.2011.01896.x
Fukasawa, Y., Ando, Y., Oishi, Y., Matsukura, K., Okano, K., Song, Z., et al. (2019). Effects of forest dieback on wood decay, saproxylic communities, and spruce seedling regeneration on coarse woody debris. Fungal Ecol. 41, 198–208. doi: 10.1016/j.funeco.2019.05.004
George, B., Suttie, E., Merlin, A., and Deglise, X. (2005). Photodegradation and photostabilisation of wood – the state of the art. Polym. Degrad. Stab. 88, 268–274. doi: 10.1016/j.polymdegradstab.2004.10.018
Grueber, C. E., Nakagawa, S., Laws, R. J., and Jamieson, I. G. (2011). Multimodel inference in ecology and evolution: Challenges and solutions. J. Evol. Biol. 24, 699–711. doi: 10.1111/j.1420-9101.2010.02210.x
Harmon, M. E., Fasth, B. G., Yatskov, M., Kastendick, D., Rock, J., and Woodall, C. W. (2020). Release of coarse woody detritus-related carbon: A synthesis across forest biomes. Carbon Balance Manag. 15, 1–21. doi: 10.1186/s13021-019-0136-6
Harmon, M. E., Franklin, J. F., Swanson, F. J., Sollins, P., Gregory, S. V., Lattin, J. D., et al. (1986). Ecology of coarse woody debris in temperate ecosystems. Adv. Ecol. Res. 15, 133–302. doi: 10.1016/S0065-2504(08)60121-X
Hu, Z., Michaletz, S. T., Johnson, D. J., McDowell, N. G., Huang, Z., Zhou, X., et al. (2018). Traits drive global wood decomposition rates more than climate. Glob. Chang. Biol. 24, 5259–5269. doi: 10.1111/gcb.14357
Janisch, J. E., Harmon, M. E., Chen, H., Fasth, B., and Sexton, J. (2005). Decomposition of coarse woody debris originating by clearcutting of an old-growth conifer forest. Écoscience 12, 151–160. doi: 10.2980/i1195-6860-12-2-151.1
Janusz, G., Pawlik, A., Sulej, J., Swiderska-Burek, U., Jarosz-Wilkolazka, A., and Paszczynski, A. (2017). Lignin degradation: Microorganisms, enzymes involved, genomes analysis and evolution. FEMS Microbiol. Rev. 41, 941–962. doi: 10.1093/femsre/fux049
Josefsson, S., Leonardsson, K., Gunnarsson, J. S., and Wiberg, K. (2010). Bioturbation-driven release of buried PCBs and PBDEs from different depths in contaminated sediments. Environ. Sci. Technol. 44, 7456–7464. doi: 10.1021/es100615g
Kahl, T., and Bauhus, J. (2014). An index of forest management intensity based on assessment of harvested tree volume, tree species composition and dead wood origin. Nat. Conserv. 7, 15–27. doi: 10.3897/natureconservation.7.7281
Kahl, T., Arnstadt, T., Baber, K., Bässler, C., Bauhus, J., Borken, W., et al. (2017). Wood decay rates of 13 temperate tree species in relation to wood properties, enzyme activities and organismic diversities. For. Ecol. Manag. 391, 86–95. doi: 10.1016/j.foreco.2017.02.012
Kaiser, K., Wemheuer, B., Korolkow, V., Wemheuer, F., Nacke, H., Schöning, I., et al. (2016). Driving forces of soil bacterial community structure, diversity, and function in temperate grasslands and forests. Sci. Rep. 6:33696. doi: 10.1038/srep33696
Kandeler, E., Gebala, A., Boeddinghaus, R. S., Müller, K., Rennert, T., Soares, M., et al. (2019). The mineralosphere–succession and physiology of bacteria and fungi colonising pristine minerals in grassland soils under different land-use intensities. Soil Biol. Biochem. 136:107534. doi: 10.1016/j.soilbio.2019.107534
Kipping, L., Gossner, M. M., Koschorreck, M., Muszynski, S., Maurer, F., Weisser, W. W., et al. (2022). Emission of CO2 and CH4 from 13 deadwood tree species is linked to tree species identity and management intensity in forest and grassland habitats.. Glob. Biogeochem. Cycles 36:e2021GB007143. doi: 10.1029/2021GB007143
Kubartová, A., Ottosson, E., and Stenlid, J. (2015). Linking fungal communities to wood density loss after 12 years of log decay. FEMS Microbiol. Ecol. 91:fiv032. doi: 10.1093/femsec/fiv032
Kueppers, L. M., and Harte, J. (2005). Subalpine forest carbon cycling: Short- and long-term influence of climate and species. Ecol. Appl. 15, 1984–1999. doi: 10.1890/04-1769
Kuznetsova, A., Brockhoff, P. B., and Christensen, R. H. B. (2017). lmerTest package: Tests in linear mixed effects models. J. Stat. Softw. 82, 1–26. doi: 10.18637/jss.v082.i13
Lasota, S., Stephan, I., Horn, M. A., Otto, W., and Noll, M. (2019). Copper in wood preservatives delayed wood decomposition and shifted soil fungal but not bacterial community composition. Appl. Environ. Microbiol. 85, 1–13. doi: 10.1128/AEM.02391-18
Lee, M. R., Oberle, B., Olivas, W., Young, D. F., and Zanne, A. E. (2020). Wood construction more strongly shapes deadwood microbial communities than spatial location over 5 years of decay. Environ. Microbiol. 22, 4702–4717. doi: 10.1111/1462-2920.15212
Lenth, R., Singmann, H., Love, J., Buerkner, P., and Herve, M. (2018). Emmeans: Estimated marginal means, aka least-squares means. R package Version, 1.1, 3.
Lüdecke, D. (2020). sjPlot: Data visualization for statistics in social science. R Package Version, 2.1.
Lüdecke, D., Makowski, D., Waggoner, P., and Patil, I. (2020). Package ‘performance’: R package 0.7.1.
Magaudda, G., Adamo, M., and Rocchetti, F. (2001). Damage caused by destructive insects to cellulose previously subjected to gamma-ray irradiation and artificial ageing. Restaurator 22:41. doi: 10.1515/REST.2001.242
Meentemeyer, V. (1978). Macroclimate and lignin control of litter decomposition rates. Ecology 59, 465–472. doi: 10.2307/1936576
Molinas-González, C., Castro, J., and Leverkus, A. (2017). Deadwood decay in a burnt mediterranean pine reforestation. Forests 8:158. doi: 10.3390/f8050158
Moll, J., Heintz-Buschart, A., Bässler, C., Hofrichter, M., Kellner, H., Buscot, F., et al. (2021). Amplicon sequencing-based bipartite network analysis confirms a high degree of specialization and modularity for fungi and prokaryotes in deadwood. mSphere 6, e856–e820. doi: 10.1128/mSphere.00856-20
Moore, T. R., Trofymow, J. A., Taylor, B., Prescott, C., Camiré, C., Duschene, L., et al. (1999). Litter decomposition rates in Canadian forests. Glob. Change Biol. 5, 75–82. doi: 10.1046/j.1365-2486.1998.00224.x
Muszynski, S., Maurer, F., Henjes, S., Horn, M. A., and Noll, M. (2021). Fungal and bacterial diversity patterns of two diversity levels retrieved from a late decaying Fagus sylvatica under two temperature regimes. Front. Microbiol. 11:151. doi: 10.3389/fmicb.2020.548793
Myneni, R. B., Dong, J., Tucker, C. J., Kaufmann, R. K., Kauppi, P. E., Liski, J., et al. (2001). A large carbon sink in the woody biomass of Northern forests. Proc. Natl. Acad. Sci. U.S.A. 98, 14784–14789. doi: 10.1073/pnas.261555198
Noll, M., Buettner, C., and Lasota, S. (2019). Copper containing wood preservatives shifted bacterial and fungal community compositions in pine sapwood in two field sites. Int. Biodeterior. Biodegradation. 142, 26–35. doi: 10.1016/j.ibiod.2019.04.007
Oberle, B., Lee, M. R., Myers, J. A., Osazuwa-Peters, O. L., Spasojevic, M. J., Walton, M. L., et al. (2020). Accurate forest projections require long-term wood decay experiments because plant trait effects change through time. Glob. Change Biol. 26, 864–875. doi: 10.1111/gcb.14873
Oksanen, J., Blanchet, F. G., Friendly, M., Kindt, R., Legendre, P., McGlinn, D., et al. (2017). Vegan: Community ecology package. R Package Version 2.5-3.
Parisi, F., Pioli, S., Lombardi, F., Fravolini, G., Marchetti, M., and Tognetti, R. (2018). Linking deadwood traits with saproxylic invertebrates and fungi in European forests–a review. iForest 11, 423–436. doi: 10.3832/ifor2670-011
Pietsch, K. A., Eichenberg, D., Nadrowski, K., Bauhus, J., Buscot, F., Purahong, W., et al. (2019). Wood decomposition is more strongly controlled by temperature than by tree species and decomposer diversity in highly species rich subtropical forests. Oikos 128, 701–715. doi: 10.1111/oik.04879
Pietsch, K. A., Ogle, K., Cornelissen, J. H. C., Cornwell, W. K., Bönisch, G., Craine, J. M., et al. (2014). Global relationship of wood and leaf litter decomposability: The role of functional traits within and across plant organs. Glob. Ecol. Biogeogr. 23, 1046–1057. doi: 10.1111/geb.12172
Purahong, W., Kahl, T., Krüger, D., Buscot, F., and Hoppe, B. (2019). Home-field advantage in wood decomposition is mainly mediated by fungal community shifts at “home” versus “away”. Microb. Ecol. 78, 725–736. doi: 10.1007/s00248-019-01334-6
Ruokolainen, A., Shorohova, E., Penttilä, R., Kotkova, V., and Kushnevskaya, H. (2018). A continuum of dead wood with various habitat elements maintains the diversity of wood-inhabiting fungi in an old-growth boreal forest. Eur. J. For. Res. 137, 707–718. doi: 10.1007/s10342-018-1135-y
Russell, M. B., Fraver, S., Aakala, T., Gove, J. H., Woodall, C. W., D’Amato, A. W., et al. (2015). Quantifying carbon stores and decomposition in dead wood: A review. For. Ecol. Manag. 350, 107–128. doi: 10.1016/j.foreco.2015.04.033
Schmalenberger, A., Pritzkow, W., Ojeda, J. J., and Noll, M. (2011). Characterization of main sulfur source of wood-degrading basidiomycetes by S K-edge X-ray absorption near edge spectroscopy (XANES). Int. Biodeterior. Biodegradation 65, 1215–1223. doi: 10.1016/j.ibiod.2011.08.013
Seibold, S., Bässler, C., Brandl, R., Gossner, M. M., Thorn, S., Ulyshen, M. D., et al. (2015). Experimental studies of dead-wood biodiversity–A review identifying global gaps in knowledge. Biol. Conserv. 191, 139–149. doi: 10.1016/j.biocon.2015.06.006
Sharman, C. V., and Smith, R. S. (1970). Gamma radiation sterilization of ponderosa pine and birch sapwood. Wood Fiber Sci. 1970, 134–143.
Shorohova, E., and Kapitsa, E. (2014). Influence of the substrate and ecosystem attributes on the decomposition rates of coarse woody debris in European boreal forests. For. Ecol. Manag. 315, 173–184. doi: 10.1016/j.foreco.2013.12.025
Smith, G. R., and Peay, K. G. (2021). Multiple distinct, scale-dependent links between fungi and decomposition. Ecol. Lett. 24, 1352–1362. doi: 10.1111/ele.13749
Song, Z., Kennedy, P. G., Liew, F. J., Schilling, J. S., and Bennett, A. (2017). Fungal endophytes as priority colonizers initiating wood decomposition. Funct. Ecol. 31, 407–418. doi: 10.1111/1365-2435.12735
Stutz, K. P., Dann, D., Wambsganss, J., Scherer-Lorenzen, M., and Lang, F. (2017). Phenolic matter from deadwood can impact forest soil properties. Geoderma 288, 204–212. doi: 10.1016/j.geoderma.2016.11.014
Tabchnick, B. G., and Fidell, L. S. (1996). Using multivariate statistics. New York, NY: Harper Collins.
Talbot, J. M., Yelle, D. J., Nowick, J., and Treseder, K. K. (2012). Litter decay rates are determined by lignin chemistry. Biogeochemistry 108, 279–295. doi: 10.1007/s10533-011-9599-6
R Core Team (2016). R: A language and environment for statistical computing (Computer software manual). Vienna: R Foundation for Statistical Computing.
Vera, F. W. M., Bakker, E. S., and Olff, H. (2006). Large herbivores: Missing partners of western European light-demanding tree and shrub species? Conserv. Biol. 11, 203–231. doi: 10.1017/CBO9780511617461.009
Vogel, S., Gossner, M. M., Mergner, U., Müller, J., and Thorn, S. (2020). Optimizing enrichment of deadwood for biodiversity by varying sun exposure and tree species: An experimental approach. J. Appl. Ecol. 57, 2075–2085. doi: 10.1111/1365-2664.13648
Weedon, J. T., Cornwell, W. K., Cornelissen, J. H. C., Zanne, A. E., Wirth, C., and Coomes, D. A. (2009). Global meta-analysis of wood decomposition rates: A role for trait variation among tree species? Ecol. Lett. 12, 45–56. doi: 10.1111/j.1461-0248.2008.01259.x
Wickham, H., Chang, W., and Wickham, M. H. (2016). Ggplot2: Create elegant data visualisations using the grammar of graphics. R package Version, 2.1
Zanne, A. E., Oberle, B., Dunham, K. M., Milo, A. M., Walton, M. L., and Young, D. F. (2015). A deteriorating state of affairs: How endogenous and exogenous factors determine plant decay rates. J. Ecol. 103, 1421–1431. doi: 10.1111/1365-2745.12474
Keywords: deadwood decomposition, mass loss, forest and grassland habitats, management intensities, region, wood traits, model averaging approach
Citation: Kipping L, Maurer F, Gossner MM, Muszynski S, Kahl T, Kellner H, Weiser WW, Jehmlich N and Noll M (2022) Drivers of deadwood decay of 13 temperate tree species are similar between forest and grassland habitats. Front. For. Glob. Change 5:1020737. doi: 10.3389/ffgc.2022.1020737
Received: 16 August 2022; Accepted: 17 November 2022;
Published: 07 December 2022.
Edited by:
Zuoqiang Yuan, Northernwest Polytechnical University, ChinaReviewed by:
Ivika Ostonen, University of Tartu, EstoniaIsabella De Meo, Council for Agricultural and Economics Research (CREA), Italy
Soledad Méndez, CONICET Instituto de Investigaciones Fisiológicas y Ecologicas Vinculadas a la Agricultura (IFEVA), Argentina
Copyright © 2022 Kipping, Maurer, Gossner, Muszynski, Kahl, Kellner, Weiser, Jehmlich and Noll. This is an open-access article distributed under the terms of the Creative Commons Attribution License (CC BY). The use, distribution or reproduction in other forums is permitted, provided the original author(s) and the copyright owner(s) are credited and that the original publication in this journal is cited, in accordance with accepted academic practice. No use, distribution or reproduction is permitted which does not comply with these terms.
*Correspondence: Matthias Noll, bWF0dGhpYXMubm9sbEBocy1jb2J1cmcuZGU=