- G.B. Pant National Institute of Himalayan Environment (NIHE), Almora, Uttarakhand, India
Analyzing plant species richness across a broad geographic gradient is critical for understanding the patterns and processes of biodiversity. In view of this, a species richness map was developed by stacking the ranges of 51 tree species along an elevational gradient in the Western Himalaya using stacked species distribution models (SSDMs). Among modeling algorithms available in SSDMs, random forest and artificial neural networks exhibited the best performance (r = 0.81, p < 0.001). The predicted tree species richness distribution pattern revealed a mid-elevation peak at around 2,000 m asl, which is in concordance with the observed richness pattern (R2 = 0.94, p < 0.001). Additionally, structural equation models (SEMs) were used to confirm the key factors that influence tree richness. The results based on SEMs confirm that the elevational pattern of predicted tree species richness is explained by mutual effects of water–energy availability, climate, and habitat heterogeneity. This study also validates that the impact of moisture on tree species richness coincides geographically with climate factors. The results have revealed that water–energy-related variables are likely to impact the species richness directly at higher elevations, whereas the effect is more likely to be tied to moisture at lower elevations. SSDMs provide a good tool to predict a species richness pattern and could help in the conservation and management of high biodiverse areas at different spatial scales. However, more investigation is needed to validate the SSDMs in other parts of the Himalayan region to provide a comprehensive synoptic perspective of Himalayan biodiversity at a larger scale.
Introduction
Species distribution modeling (SDM) is a tool that offers robust estimates of biodiversity mapping using field-based observations of species localities. In recent years, SDM has become a useful approach for predicting species distribution ranges, based on the relationships between species records and environmental variables (Pineda and Lobo, 2009). Mapping of species richness using SDM has increased significantly and gaining the attention of policy planners in forest management and conservation (Fitzpatrick et al., 2008; Colombo and Joly, 2010; Schmidt-Lebuhn et al., 2012; Benito et al., 2013; Brown et al., 2015; D’Amen et al., 2015; Pouteau et al., 2018). It is well known that the assessment of plant species richness in any ecosystem is critical for long-term management and conservation initiatives (Gutiérrez and Huth, 2012; Rawal et al., 2018; Negi et al., 2019). A hump-shaped pattern or a higher number of species at mid-elevation has been reported as a prominent pattern across the mountain regions (Stevens, 1992; Rahbek, 1995; Grytnes and McCain, 2013; Guo et al., 2013; Negi et al., 2021). Several hypotheses, e.g., energy and water availability, energy–water balance, seasonality, and habitat heterogeneity, have been proposed to explain the elevational pattern of species richness. The habitat heterogeneity hypothesis states that an increase in heterogeneity leads to an increase in species richness (MacArthur and MacArthur, 1961). Similarly, the energy hypothesis states that greater availability of energy is an optimum condition for enhancing species richness and climate stability (Turner et al., 1987; Hawkins et al., 2003; Panda et al., 2017).
The Himalaya, a global biodiversity hotspot (Myers, 1988) and a climatically sensitive region, supports more than 10,500 species of flowering plants, which are distributed across the subtropical foothills to high alpine climatic zones. These regions are important to study species richness patterns across a wider geographical coverage (Rana and Rawat, 2017). Studies based on field observations and overlapping elevational ranges along an elevational gradient in the Himalaya show a common pattern of a mid-elevation peak in the species richness (Vetaas and Grytnes, 2002; Bhattarai and Vetaas, 2003, 2006; Oommen and Shanker, 2005; Acharya et al., 2011; Kluge et al., 2017; Manish et al., 2017; Thakur et al., 2021). Field observation-based studies along the elevational gradients in the Himalaya report the highest number of species at the mid-elevations (Kharkwal et al., 2005; Sharma et al., 2018; Rana et al., 2019a; Bhat et al., 2020). The causes of such variations include a primary role of water–energy dynamics (Acharya et al., 2011), evolutionary history (Rana et al., 2019b), and disturbances (Negi et al., 2018a,b; Thakur et al., 2021; Rana et al., 2022). However, spatial and climatic patterns are postulated to explain these patterns of variation in species richness over elevational gradients (Connell and Orias, 1964). Panda et al. (2017) suggested that energy variables (i.e., potential evapotranspiration and temperature seasonality) provide a better explanation than water variables (i.e., aridity index and precipitation of the driest quarter) in explaining plant species richness in the Western Himalaya. Similarly, a combination of ambient energy (i.e., air temperature, solar radiation, and potential evapotranspiration) and water availability (i.e., soil water content and precipitation) was found to be the main drivers of the elevational pattern of species richness in the Eastern Himalaya (Manish et al., 2017). Recent studies from the Himalayan region indicate that the freezing line and disturbance also play important roles in driving the species turnover along the elevational gradient (Rana et al., 2022). However, the specific causes for the hump structure of association between plant species richness and elevation are difficult to decipher due to the intricate linkages among these processes (Acharya et al., 2011). Thus, currently, many hypotheses still remain to be tested for habitat heterogeneity and climate stability, which can provide important insights into explaining species richness patterns (Pan et al., 2016).
Many assessments of biodiversity patterns along elevational gradients rely on field data gathered in transects or plots. Species richness maps based on field observations have been proven to be the best alternative for identifying biodiversity-rich areas with complete geographical coverage (Murray-Smith et al., 2009; Oliveira et al., 2019; Testolin et al., 2021). Identification of degraded regions, sensitive and species-specific habitats (Negi et al., 2018a), and hotspots of invasive species (Gallardo-Cruz et al., 2009; Bellard et al., 2013; Pouteau et al., 2018) through spatially explicit methods is useful for climate change adaptation, restoration of degraded lands, and conservation of specific habitat or region (Bellard et al., 2013).
Modeling species richness using SDMs is frequently used to anticipate how potential plant species richness in mountains may alter due to climate change. Although only a few research studies focus on the use of SDMs to predict richness patterns along elevational gradients (Syfert et al., 2018), no attempts have been made to predict a spatial pattern of species richness using SDM approach in the Indian Himalayan region (IHR). The true ranges of most plant species are unknown due to a limited number of their recorded locations. This has prompted for using a valid species distribution model for mapping, using field-based data on species locale. In such cases, the use of SDM without knowing the true species ranges of plant species can add ambiguity in predictions for research and policy issues, such as conservation priority. Thus, this study is an attempt to prove that SDM algorithms are a useful approach for predicting true species richness patterns at a spatial scale. This was followed by testing the ecological hypotheses related to water, energy, climate, and habitat heterogeneity to identify determinants of tree species richness in the Western Himalaya.
Materials and methods
Study area and species data
The study area located in Pithoragarh district (Uttarakhand) in the Western Himalaya (Figure 1) has a unique importance in terms of diverse and unique flora and fauna (Oli and Zomer, 2011). It is situated at the confluence of the Western, Central, and Trans-Himalayan bioregions, forming an intersection of flora, fauna, and human cultures. The study area has a wide elevational gradient ranging from 412 to 7,132 m asl constituting the vegetation types covering subtropical to alpine regions. It is also an important ecological barrier depending on varying topographies from the outer Siwalik to the inner high Himalaya and consists of a variety of plant diversity covering subtropical to alpine vegetation (Saxena et al., 1985). The region is attitudinally divisible into subtropical (300 to 1,500 m), temperate (1,500 to 3,500 m), and alpine (>3,500 m) zones (Saxena et al., 1985). The mean annual temperature of the area ranges from 30 to 12°C lower to higher altitudinal ranges (Thakur et al., 2020). Vegetation sampling was carried out during 2016–2019 along an elevational gradient ranging from 800 to 4,000 m asl at 33 sites of an approximate interval of 100 m in the Pithoragarh district. We have used the phytosociological method (Misra, 1968, Muller Dombois and Ellenberg, 1974; Negi et al., 2018a; Rawal et al., 2018) to sample trees species. The sample plots were randomly laid at the interval of 100 m elevation along the altitude. Within 100 m elevation, we have randomly placed three plots of 2500 m2 each, and within one random plot (2,500 m2), five quadrats of 10 × 10 m (100 m2 size each) were placed for tree sampling. Thus, a total of 99 plots (2,500 m2 size) were laid along 33 elevations or sites (800–4,000 m asl) along the elevational gradient in the studied area. Trees with a circumference at breast height (cbh) > 30 cm were considered for sampling. A total of 2,193 geocoordinates for 51 tree species were recorded using a global positioning system (Supplementary Table 1).
Environmental data
A comprehensive set of environmental variables that were considered to be the main factors influencing the distribution of plant species (Dirnböck et al., 2003; Körner, 2012) were used in this study. We have used 23 environmental variables to understand the relative importance of climate, water, energy, and habitat heterogeneity for explaining spatial variation in tree species richness at the regional scale (Supplementary Table 2). We extracted 19 bioclimatic variables from Worldclim21 at the resolution ∼1 km2 (Fick and Hijmans, 2017) and elevation from USGS GTOPO-302 with a resolution of 30 arc seconds. The slope and aspect were extracted from an elevation layer using the “slope” and “aspect” tools in the spatial analyst tools of ArcGIS 10.1. In addition, the normalized difference vegetation index (NDVI), which has been suggested to be useful in predicting plant species distribution and richness (Williams et al., 2009; Cramer and Verboom, 2017), was also derived from the public domain Sentinel-2B of 10-m-resolution images for pre-monsoon and post-monsoon seasons. The data were extracted on March 10, 2019, and October 19, 2019, from the open-source Earth Resources Observation and Science (EROS) Data Center of the US Geological Survey (USGS) archive.3 All the environmental layers were resampled to 1 km2 using bilinear transformation to maintain the uniformity. To deal with collinearity, we have performed the multicollinearity test among the predictor variables using the “usdm” package in R (Naimi, 2015) and retained only variables with a variance inflation factor (VIF) less than 8 or correlation coefficient r ≥ 0.8 (Supplementary Figure 1). Based on the multicollinearity test, we used a non-collinear set of predictor variables, i.e., precipitation seasonality (PS), mean diurnal range (MDR), mean temperature of driest quarter (MTDQ), precipitation of warmest quarter (PWQ), slope, aspect, and normalized difference vegetation index (NDVI) in the final ensemble model (Supplementary Figure 2). These variables were categorized into water, energy, climate stability, and habitat heterogeneity based on the potential importance of factors to plant species richness. The MTDQ surrogates as energy availability (Panda et al., 2017; Gao and Liu, 2018), PWQ as water availability (Panda et al., 2017; Gao and Liu, 2018), MDR and PS as climate stability (Gao and Liu, 2018), and NDVI, slope, and aspect as habitat heterogeneity (Mouchet et al., 2015; Nieto et al., 2015).
Stack species distribution modeling
As uncertainty in distribution predictions can skew policymaking and planning, fitting a number of alternative statistical approaches finds an agreement among SSDM projections (Marmion et al., 2009; Gritti et al., 2013). The SSDM is ideal for extrapolation of predicted species richness, even with a small number of presence locations with a narrow environmental range (Cayuela et al., 2009; Williams et al., 2009; Mateo et al., 2010). We used the SSDM approach and implemented the R package “SSDM” which integrates multiple individual SDMs to produce a community-level model (Ferrier and Guisan, 2006). The SSDM package provides eight different methods, namely, the generalized linear model (GLM), generalized additive model (GAM), multivariate adaptive regression splines (MARS), generalized boosted regression model (GBM), classification tree analysis (CTA), random forest (RF), support vector machines (SVM), and artificial neural networks (ANN), to generate a distribution model for a given species. To get the binary map, we used the sensitivity–specificity equality (SES) to compute the binary map threshold (Schmitt et al., 2017). We first produced individual binary maps for each species using these modeling algorithms. We have then checked values of area under the curve (AUC) and kappa metrics that evaluate the accuracy of the individual model for each species. The AUC metric is a widely used statistic for assessing the discriminatory capacity of species distribution models (Barbet-Massin et al., 2012; Leroy et al., 2018). This metric assesses the balance between a true-positive rate (sensitivity) and a false-positive rate (100 – specificity), which is a threshold-independent metric that varies from 0 to 1, where values near or equal to 1 represent that the models reached excellent discriminatory power and values around 0.5 represent species predictions no better than a random distribution. The model needs the presence and absence data of the occurrence data. Many studies proved that the presence–absence of biotic data in species distribution models tended to perform better than the presence-only models (Barbet-Massin et al., 2012; Schmitt et al., 2017; Leroy et al., 2018). It is believed that presence–absence models will yield more reliable results if the presence data that are available seem insufficient or uncertain. To reduce over-prediction and extrapolation into unknown areas can be minimized by absence and/or pseudo-absence points (Barbet-Massin et al., 2012). We randomly created pseudo-absence data of each species with an equal number of its presence data randomly within the study. We summed individual binary maps generated using the SSDM approach for all species, and the final model was selected for AUC > 0.90 to produce an ensemble species richness map. The accuracy (disagreements between the prediction and reality) of the species richness maps across the sets of spatial grains was assessed using the observed occurrence data of the species. We analyzed the proportion of accurate richness predictions and true negatives, true positives, and similarities in species richness using the species richness error, prediction success, and Cohen’s kappa coefficient (Schmitt et al., 2017). The work flow of modeling approach is shown in Figure 2. Subsequently, we developed the partial dependency plots to better explain the relationship between tree species richness and predictor variables. The partial dependency plots represent the influence of predictor variable on target variable (richness in our case) while controlling the average effects of other predictor variables (Mouchet et al., 2015). For interpretation, we have smoothed the response variables with a spline fit (Mouchet et al., 2015).
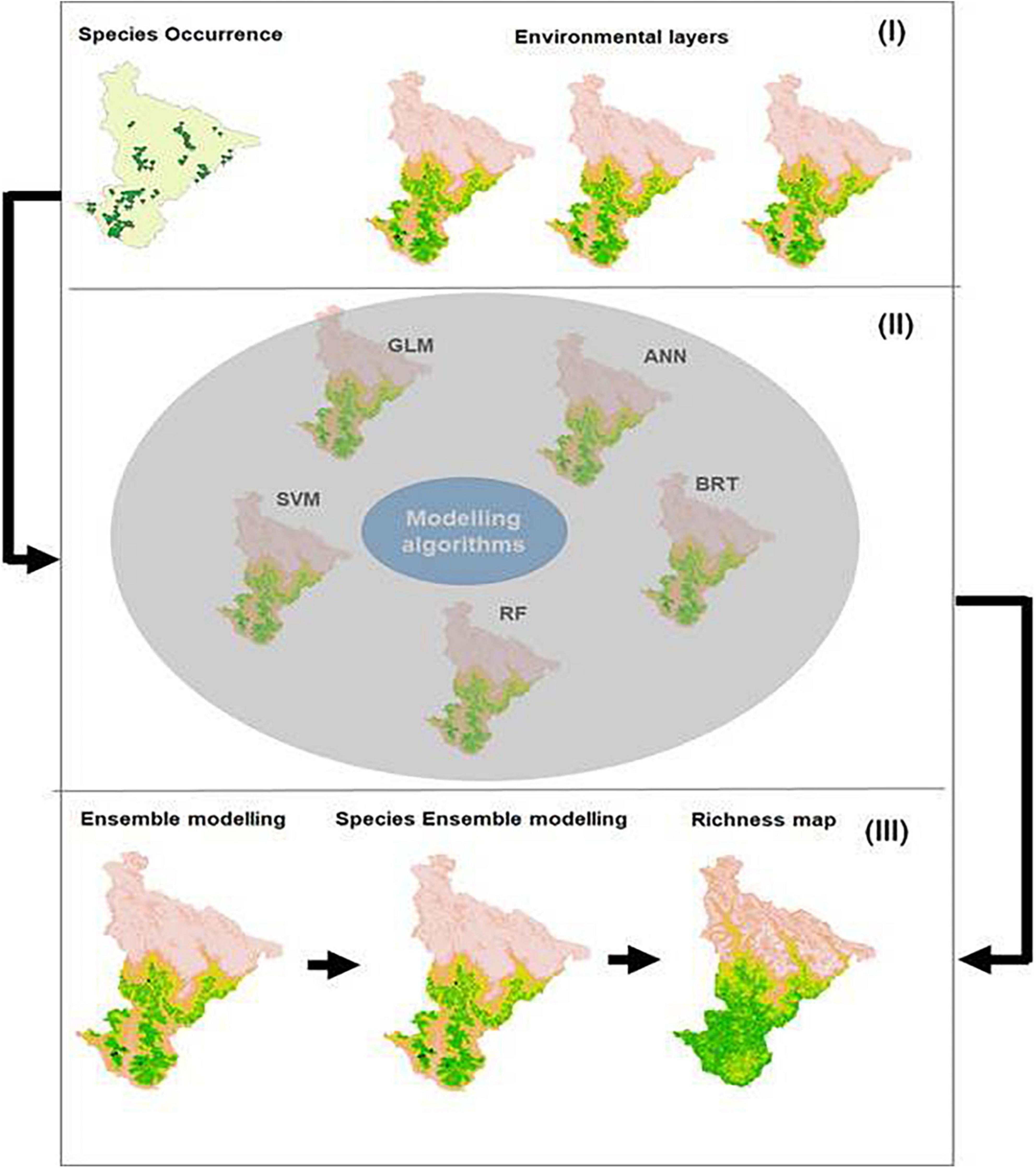
Figure 2. Methodological flow diagram of SSDM approach. (I) Input species occurrences and environmental layers; (II) modeling algorithms for deriving binary maps; and (III) ensembling binary maps to produce community-level richness.
Species richness–environment relationship
To validate the relative importance of environmental factors for determining tree species richness generated from SSDM, we have used the structural equation model (SEM) approach. SEM is a sophisticated and reliable regression model commonly employed in ecological research (Panda et al., 2017; Rana et al., 2019b). SEM general methodology entails the creation of a multivariate dependence model that can be statistically validated using field data. This multivariate technique not only aids in a model selection among alternatives, but also presents a quick and efficient solution to a succession of overlapping regression associations. The analysis was carried out in R using the packages “lavaan,” “semPlot,” and “lavaan” from the CRAN repository (Rosseel, 2012). We compared tree species richness to each group of ecological determinants. Then, for each ecological driver, we investigated the combination of environmental variables with tree species richness. The beta coefficient, R2 value, and root mean square error were used to assess the model’s performance.
Results
Pattern of species richness
The SSDM approach successfully predicted a tree species richness map with a spatial resolution of 1 km2 under the present climatic environments (Figure 3). We extracted pixel values of predicted richness with an elevation to derive the elevational pattern of tree species. The observed pattern shows higher synchronicity (R2 = 0.94, p < 0.001) with an expected richness pattern (Supplementary Figure 3). The elevational pattern of predicted species richness showed a positively skewed hump-shaped pattern with a richness of 20–27 species peaked at approximately 2000 m asl. The predicted richness was about 10–15 species between 2,000 and 3,000 m asl elevation. However, above 3,000 m asl, the tree richness recorded was far less, ranging from 2 to 6 species (Figure 4). Consequently, the predicted richness shows a sharp decline in the occurrence of species above 2,000 m asl.
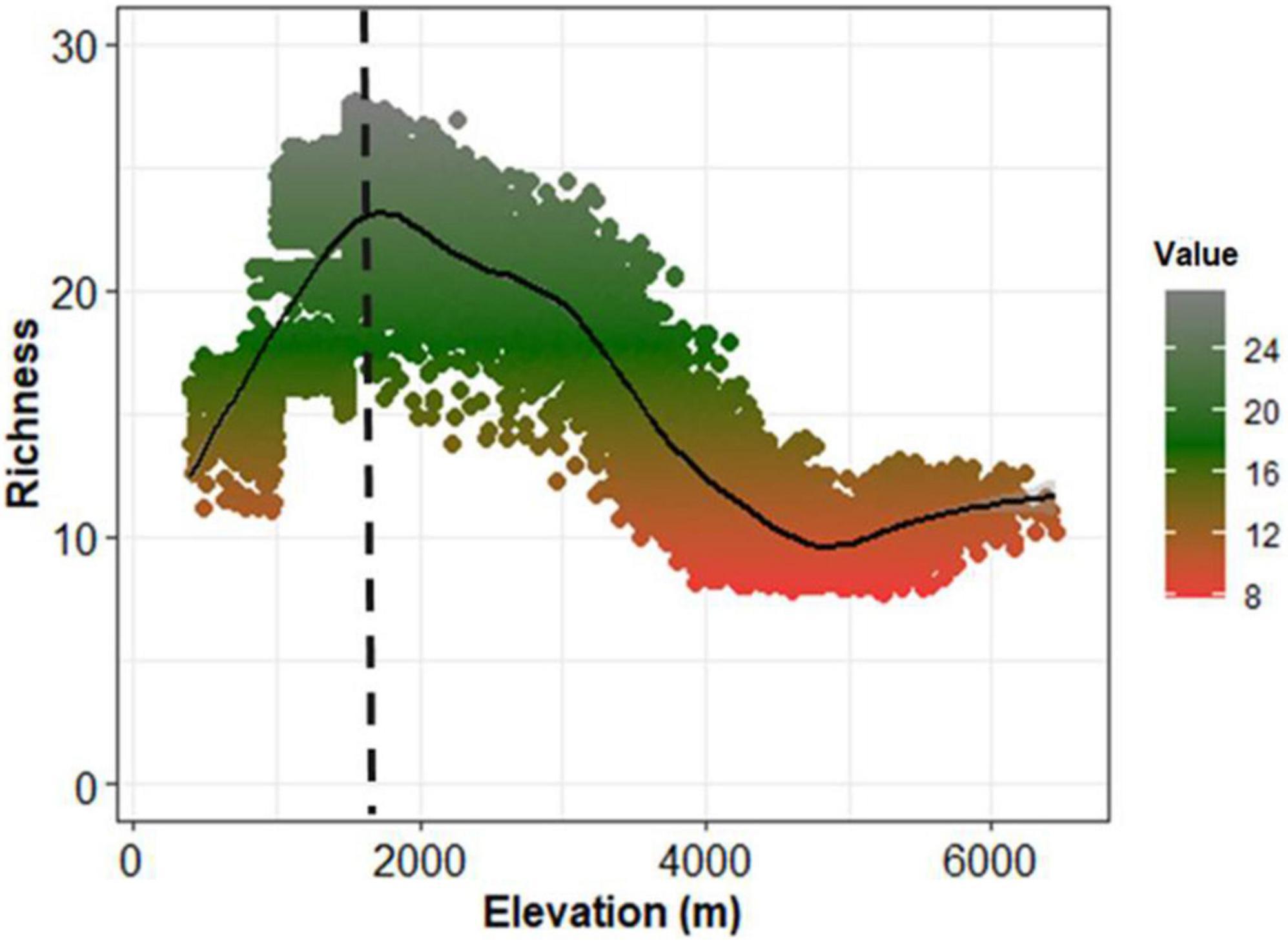
Figure 4. Elevational pattern of predicted tree species richness showing positively skewed humped. Dotted black line indicates the richness peak.
Variables determining tree species richness
Interestingly, all variables explained marginal variance (about 11–16%) to explain tree species richness in the region (Supplementary Figure 4). Habitat heterogeneity variables, i.e., NDVI, aspect, and slope, explained 16.46, 15.18, and 11.90% of the variance, respectively, in explaining tree species richness. Water (PWQ) and energy (MTDQ) variables contributed 14.28 and 12.96% of the variance, respectively, to explain tree species richness. Climate stability variables, i.e., MDR and PS, have explained 15.90 and 13.32% of the variance, respectively, to explain tree species richness. These results indicate that the predicated richness patterns are explained differently by various factors, but their relative importance varies. The SEMs adequately fitted the output of key predictor variables for the water–energy, habitat heterogeneity, and climate stability models. The SEM of habitat heterogeneity, water–energy, and climate stability explained 36, 23, and 32% of the variance, respectively, in explaining tree species richness. The habitat heterogeneity model shows a strong significant positive correlation (r = 0.76, p < 0.001) between NDVI and tree species richness and aspect (r = –0.23, p < 0.05) and a weak negative correlation with slope (r = –0.05, p > 0.05) (Figure 5A). Similarly, a water–energy model based on MTDQ shows a positive relationship (r = 0.52, p < 0.05) between tree species richness (Figure 5B). In a climate stability model, a strong positive relationship (r = 0.80, p < 0.001) between PS and tree species richness was found (Figure 5C). The partial dependency plots show a sharp increase in richness values with an increase in NDVI values above 0.25, indicating higher values of NDVI sustain higher tree species (Figure 6A). These plots also reflect a relationship between MDR and predicted tree species richness that generates a hump-shaped curve with the maximum predicted species richness at an approximate increase in MDR between 10 and 12°C (Figure 6B). The richness varies between 10 and 12 species with an increase in MDR from 8 to 13°C (Figure 6B). For MTDQ, a tree species richness pattern increased with an increase in MTDQ (Figure 6C). The relationship between tree species richness and PS shows a parabolic response and increased with an increase in PS ranging from 80 to 120 mm (Figure 6D); it reflected an inverse response for species richness values up to 15 species (Figure 6D). The predicted richness and PWQ relationship has revealed an accelerating unimodal pattern peaked at a PWQ value of 750 mm (Figure 6E). Overall, this research contribution reveales a combined effect of water, energy, and habitat heterogeneity and climate stability, which have a greater role in explaining tree species richness in the mountain region.
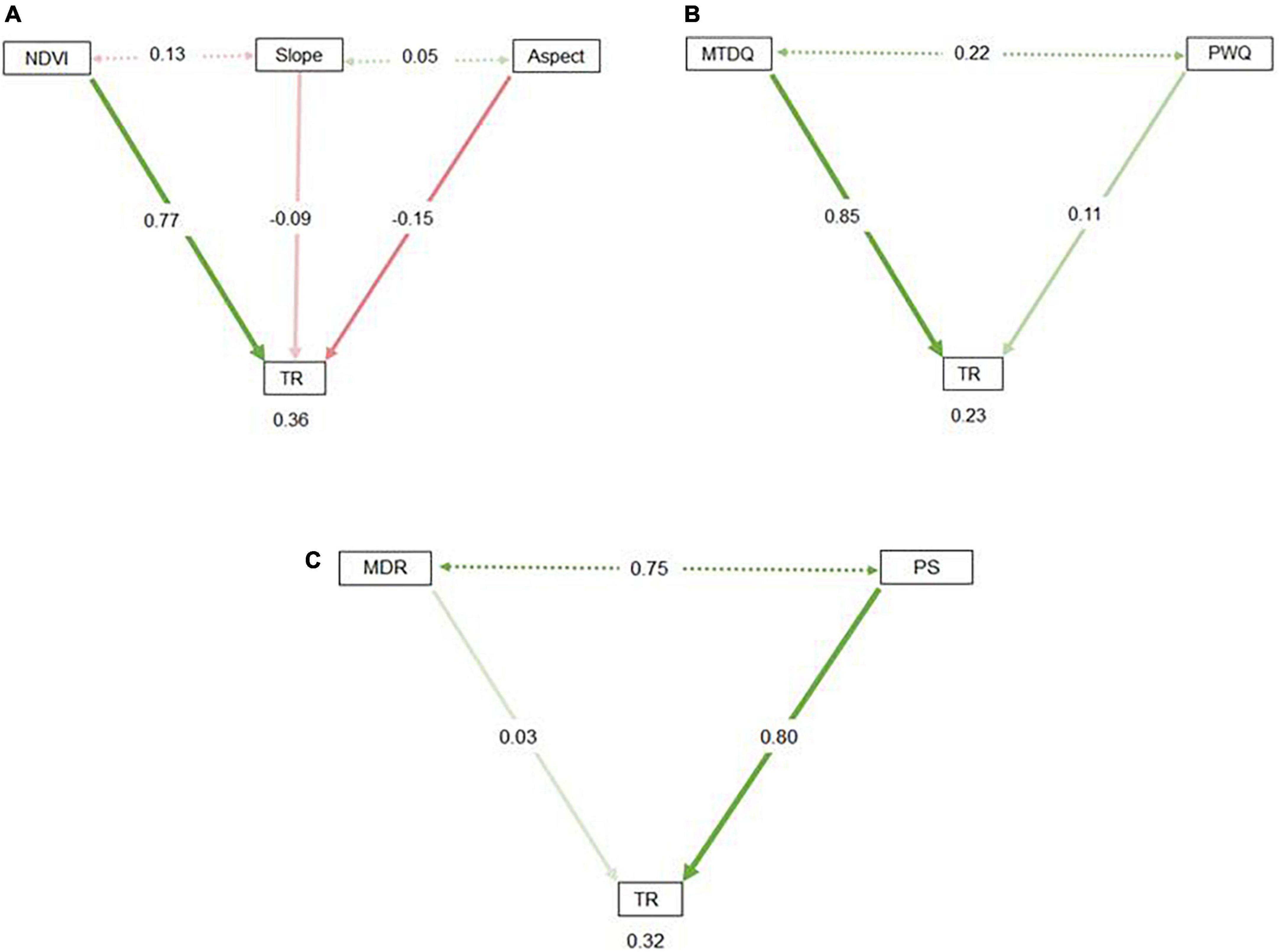
Figure 5. SEM output to evaluate the significance of environmental determinants: (A) Habitat heterogeneity, (B) water–energy, and (C) climate stability on tree richness (TR).
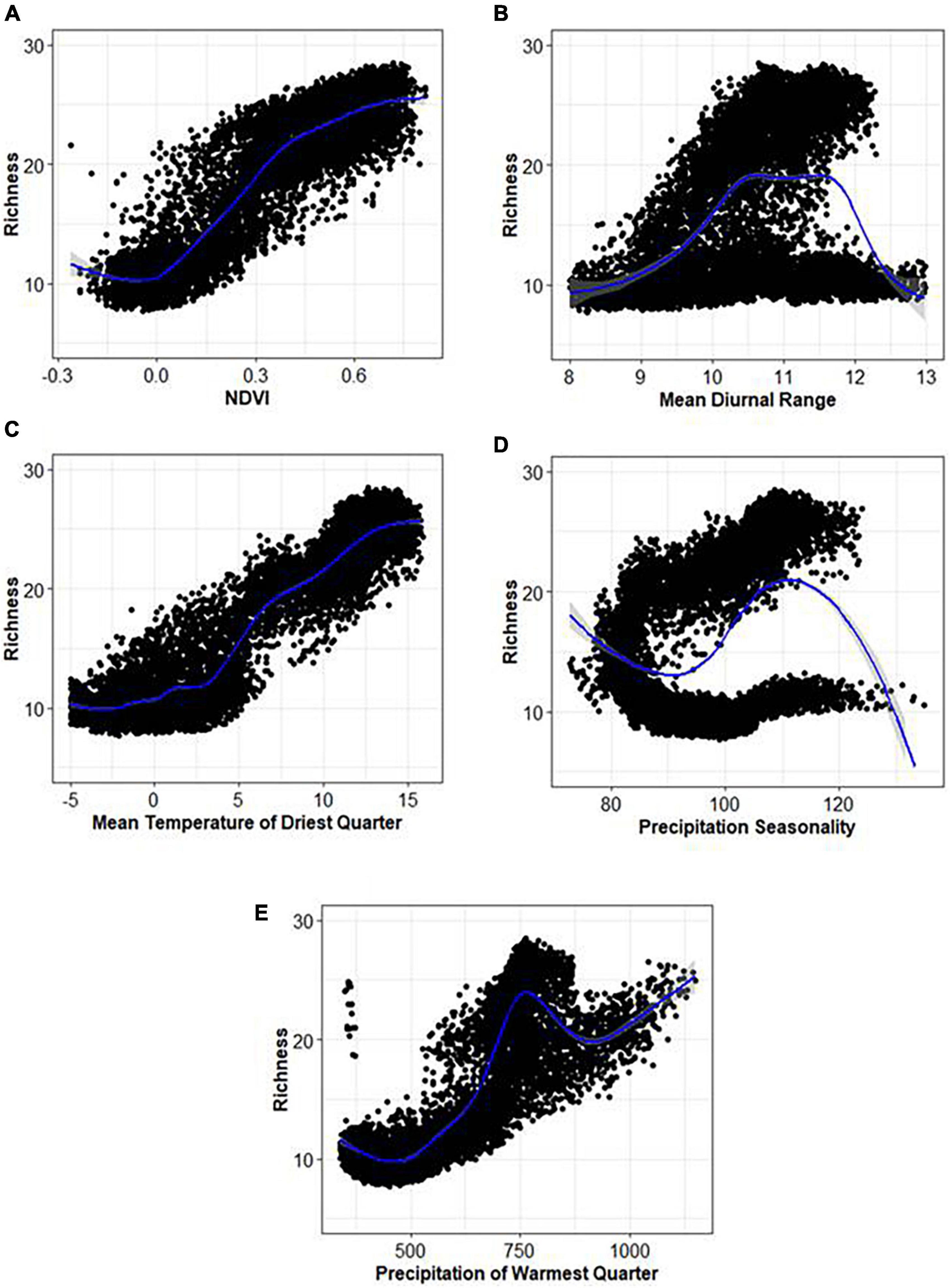
Figure 6. (A–E) Examples of partial dependency plot showing the relationships between the predicted tree species richness with important predictor variables.
Model evaluation and validation
The relative success of all the models was evaluated in predicting tree species richness by comparing mean AUC values and kappa statistics. Among all the models, RF and ANN generated results with the maximum mean AUC and kappa values >0.90. The cross-model correlations showed a high correlation between RF and ANN models (r = 0.81; Supplementary Table 3); thus, we used an ensemble model of these two variables to obtain a reliable predicted richness map. It was also reflected in the investigation of maps and correlation statistics that RF and ANN generated similar results, in terms of both AUC scores and the physical locations where species occurrences were projected. The final model exhibiting a high kappa value (0.99) signifies that the model performance was excellent (Supplementary Table 4). The mean richness error, i.e., the difference between actual and predicted richness, is quite low (4.95), revealing a superior utility of the map (Supplementary Table 4).
Discussion
Pattern of species richness
Mapping spatial pattern of species richness using ground observations is important for biodiversity monitoring, and conservation, as well as developing management and adaptation strategies (Rodríguez et al., 2007). The predicted pattern of tree species richness using SSDM showed a positive skewed hump-shaped pattern. The hump-shaped association predicted by SSDM between species richness and elevation is consistent with various earlier investigations (Krömer et al., 2013; Syfert et al., 2018). The results of this study reveal that tree species richness peaks at around 2,000 m containing 20–27 taxa; however, most other Himalayan studies show that a richness peaks at much lower elevations: (i) 1,000 m (Bhattarai and Vetaas, 2006), (ii) 600–1,000 m (Behera and Kushwaha, 2006), (iii) 1,500 m (Acharya et al., 2011), and (iv) 500–1,000 m (Rana et al., 2019a). In the study region, a mid-elevation zone is dominated by various tree genera such as Quercus, Rhododendron, Pinus, and Alnus with a sharp decline in species richness toward higher elevations. The uppermost limits in our sampling at around 4,000 m in the region were dominated by Abies pindrow, Betula utilis, and Pinus wallichiana. This indicates that subalpine trees are primarily sink populations in the region and are unable to cope with the harsh and stressful open subalpine environment. Findings from other parts of the Himalayan region have revealed that the elevation ranging between 1,500 and 2,000 m is an essential transition zone between subtropical and temperate flora (Rana et al., 2019a). If we examine the species–area relationships of the region, the lower half of the region is anticipated to have more species due to the larger area available for species (Supplementary Figure 5). The present results have reflected that the richness was high at 2,000 m and in consonance with the previous field-based studies from the Himalaya (Vetaas and Grytnes, 2002; Bhattarai et al., 2004). The higher peak of tree species richness in our study can thus be attributed to the habitat specificity of tree species and a low level of anthropogenic pressure. Generally, mountain regions with a wider elevational gradient exhibited a unimodal pattern of diversity, and this kind of pattern is globally well-recognized for the respective transects (Rahbek, 1995; Gaston, 2000; Arya et al., 2017; Rana et al., 2019a). Previous studies have suggested that elevational patterns of plant richness may be influenced by biotic processes, environmental heterogeneity, climate, and evolutionary history (McCain and Grytnes, 2010; Rana et al., 2019a).
In a study from Nepal, Bhattarai and Vetaas (2006) observed a decreasing trend of trees above 1500 m asl with narrow elevational ranges at the transition zone of the gradient with a wider elevational range in the middle. This is due to boundary effects, i.e., a change in environmental or climatic conditions at lower and higher elevational zones (Feng et al., 2016). In addition, optimum water (precipitation seasonality in our study) and temperature (mean diurnal range in our study) create more favorable conditions, which results in more plant species at mid-elevation (McCain, 2007). Even at high elevations, the decline in species richness may be due to the decreasing temperature, precipitation, and humidity (Sinha et al., 2018). Furthermore, we observed that a declining pattern of predicted tree species richness after 2000 m is also supported by observed tree species richness over the Himalayan region (Stevens, 1992; Kharkwal et al., 2005; Rodríguez et al., 2007; Malik, 2014; Malik and Nautiyal, 2016; Sharma et al., 2018; Sinha et al., 2018; Rana et al., 2019a; Bhat et al., 2020). This suggests that the SSDM has the potential utility to predict the observed richness pattern using field-based plant species data.
Role of habitat heterogeneity
Habitat heterogeneity is widely recognized as a key driver of high species richness in mountain environments, as it promotes diversification, reduces extinction, and provides niche space for species cohabitation (Stein et al., 2014). Environmental variables related to habitat heterogeneity (slope, aspect, and especially NDVI) are critical in explaining the overall tree species richness patterns. In a study, Pouteau et al. (2018) reported that the inclusion of remotely sensed derived NDVI provides a better prediction of plant species richness because of its multispectral nature. Interestingly, high NDVI values were observed around 2,000 m asl elevation in our study region strongly explaining a mid-elevation peak in tree species richness (Supplementary Figure 6A). The wider elevational gradient in our study site shows variations in NDVI values and climatic conditions which depicted a different zonation of vegetation types. Feilhauer et al. (2012) have also emphasized that a wider elevational gradient and a clear floristic and climatic gradient have a clear association between NDVI and plant species richness. Forests may have a diverse range of vegetation types with significant internal heterogeneity, which is likely to support a greater number of ecological groupings, such as species associated with clear-cuts, broadleaf or coniferous, forest floors (Ellenberg and Leuschner, 2010). We found evidence for habitat heterogeneity, implying that a greater variety of vegetation types should support a greater number of species. Slope and elevation have an important role in the species composition of the region due to a change in the incoming solar radiation (Gallardo-Cruz et al., 2009; Scherrer and Körner, 2011), which changes over short horizontal distances. The small change in the distribution of solar radiation and water moisture with a change in the slope may contribute to a difference in the micro-climate of the area. This will directly or indirectly change the plant composition and species richness (Vetaas, 2000; Gallardo-Cruz et al., 2009; Moeslund et al., 2013). In those places where rainfall does not exceed 400 mm per year, species richness is comparatively high on damp north-facing slopes compared with sun-exposed south-facing slopes (Vetaas, 1992; Sternberg and Shoshany, 2001). However, at higher elevations, energy inputs may be an important limiting factor. Our findings are consistent with these facts that changes in land-use patterns between contrasting slopes may reflect varied vegetation structures and plant diversity (Burnett et al., 1998). The land-use patterns, microclimatic conditions, and their interactions potentially regulate vegetation structure and abiotic factors (Shrestha and Vetaas, 2009). Our findings, as well as prior research on other plant groups (Shrestha and Vetaas, 2009; Shrestha et al., 2018), found support for the role of habitat heterogeneity in maintaining high richness in the mountain region.
Role of water and energy
The energy (MTDQ) and water variables (PWQ) have explained much variance to define the distribution of tree species richness in this study. The MTDQ and PWQ explained 14.28 and 12.96% of the variance, respectively, in tree species richness. Both MTDQ and PWQ values show a declining trend with a more pronounced decline after 2,000 m asl along the elevational gradient in the region (Supplementary Figures 6B,C). These findings suggest that richness patterns around 2,000 m asl are the best-suited environments for the growth of tree species, and they are confined under exceptionally severe conditions (such as extremely cold temperatures) at high elevations and warmer climates at low elevations (i.e., subtropical climate). Despite the availability of sufficient energy, water stress has a negative impact on species richness, and with optimal water availability, plants would use the maximal photon flux required for their physiological processes (Panda et al., 2017). This can be explained by the area’s cold and dry climates, which may have resulted in a high biotic reliance on energy (Panda et al., 2017). Interaction between water and energy, either directly or indirectly (via plant productivity), provides a strong explanation for globally extensive plant diversity along the gradients (Hawkins et al., 2003). Thus, energy and water can better explain the hump-shaped pattern of tree species richness as reflected in Figures 6C,E. Sufficient energy and moisture stimulate photosynthesis in plants, which increases plant species richness through physiological processes (Bhattarai and Vetaas, 2003; Hawkins et al., 2003; Currie et al., 2004). Similar observations have been reported from other mountain regions of the world (O’Brien, 1998; Hawkins et al., 2003; Marini et al., 2008; Gao and Liu, 2018; Lu et al., 2018; Pandey et al., 2020a,b) and from the Himalaya (Panda et al., 2017; Vetaas et al., 2019; Pandey et al., 2020b). However, if we had also evaluated the lowest portion of the gradient, our general positive association between species richness and temperature along the elevational gradient might have assumed a different shape (Bhattarai et al., 2004). Despite the fact that the energy (MTDQ) and water variables (PWQ) may underlie such cumulative species–energy connections, knowledge of their relative contributions is limited so far, and further investigation is necessary to gain insight into these mechanisms. Thus, integrating water–energy variables is critical for future biodiversity research.
Role of climate stability
Climatic stability is an important driving force in shaping the geographical distribution of plant species in mountain regions. Our results have shown that MDR and PS (representing climate stability) influenced the tree species richness pattern in the Himalayan region. In the study region, we observed that areas with a moderate mean MDR are more favorable for the survival of tree species around 2,000 m asl (Supplementary Figure 6D). The high and low values of MDR are an indication of high and low moisture, respectively. Vetaas (1993) has suggested that ideal moisture conditions at mid-elevation may be associated with the existence of the cloud zone, where a significant amount of water is deposited directly onto plants from clouds and light mist consequently decreases sunlight and evapotranspiration, resulting in a higher atmospheric humidity that may promote high species richness. Contrary to this, high moisture at higher elevations and substantial diurnal temperature during growing seasons (as depicted in Figure 6B) could trigger physical harm to the plants and their reproductive success. This suggests that stable climates enhance species richness as has previously been reported in other mountain regions (Gao and Liu, 2018; Zhao et al., 2018).
Conclusion, limitations, and implications of the study
The current SSDM analysis demonstrates the relevance of water, energy, climate, and habitat heterogeneity in determining the mid-elevation peak in species richness, paving the way for the next step of extending the model inference regarding diversity patterns to a larger range of the Himalaya. The water–energy, climate stability, and habitat heterogeneity variables had significant effects in explaining plant species richness. This is implying that climate and land cover should be incorporated as explanatory factors in models of species richness along elevational gradients. The mapped richness is baseline data for the region and can be updated with more robust field observations. Investigation of the relationship between elevation and plant distribution ranges by putting hypotheses related to habitat heterogeneity and climate stability will improve the understanding of ecological drivers of plants in the Himalayan region. Furthermore, the SSDM approach has shown the potential utility to predict the observed richness pattern which would be useful for biodiversity management and conservation planning in the region. Due to the lack of accurate absence information in the data used for SSDMs, it is expected that some plant absence records that appear to be accurately predicted are actually incorrect, thereby increase the predictive success of performance metrics. In addition, it is obvious that additional surveys would improve the dataset.
The mapped species richness can also be used to identify biodiversity-rich areas for conservation and decision-making. Further, the areas with low species richness can be identified for restoration and management planning. The plantation of habitat-specific tree species in degraded areas would be useful for the mitigation of climate change. These maps can be utilized as a potential input for assessing the vulnerability of forests under current and contrasting future climate change scenarios. Therefore, mapping areas of biological importance along with their respective degrees of knowledge is the first step toward effective conservation planning.
Data availability statement
The original contributions presented in this study are included in the article/Supplementary material, further inquiries can be directed to the corresponding author.
Author contributions
ST: conceptualization, field study, data curation, validation, analysis, and writing – original draft. RD: data analysis, software, and writing and editing in first draft. VSN: writing – review and editing. IDB: writing – review and editing, and supervising.
Acknowledgments
We thank Sunil Nautiyal, Director, G.B. Pant National Institute of Himalayan Environment (NIHE), Kosi-Katarmal, Almora, for encouragement and facilities. We also thank late R. S. Rawal for the conceptualization of the study and guidance. Partial funding from the Department of Science and Technology (DST), Government of India, under NMSHE—Task Force 3 “Forest Resources and Plant Biodiversity” Phase II, and ICIMOD under KSLCDI-II is gratefully acknowledged.
Conflict of interest
The authors declare that the research was conducted in the absence of any commercial or financial relationships that could be construed as a potential conflict of interest.
Publisher’s note
All claims expressed in this article are solely those of the authors and do not necessarily represent those of their affiliated organizations, or those of the publisher, the editors and the reviewers. Any product that may be evaluated in this article, or claim that may be made by its manufacturer, is not guaranteed or endorsed by the publisher.
Supplementary material
The Supplementary Material for this article can be found online at: https://www.frontiersin.org/articles/10.3389/ffgc.2022.1022082/full#supplementary-material
Footnotes
References
Acharya, B. K., Chettri, B., and Vijayan, L. (2011). Distribution pattern of trees along an elevation gradient of Eastern Himalaya, India. Acta Oecol. 37, 329–336. doi: 10.1016/j.actao.2011.03.005
Arya, V., Kumar, B., and Rawat, J. S. (2017). Tree species diversity, community composition and distribution across six forest stands of Uttarakhand, Central Himalaya, India. Ind. J. Ecol. 44, 722–728.
Barbet-Massin, M., Jiguet, F., Albert, C. H., and Thuiller, W. (2012). Selecting pseudo-absences for species distribution models: how, where and how many? Methods Ecol. Evol. 3, 327–338. doi: 10.1111/j.2041-210X.2011.00172.x
Behera, M. D., and Kushwaha, S. P. S. (2006). “An analysis of altitudinal behavior of tree species in Subansiri district, Eastern Himalaya,” in Plant conservation and biodiversity, eds D. L. Hawksworth and A. T. Bull (Dordrecht: Springer), 277–291.
Bellard, C., Thuiller, W., Leroy, B., Genovesi, P., Bakkenes, M., and Courchamp, F. (2013). Will climate change promote future invasions? Glob. Chang. Biol. 19, 3740–3748. doi: 10.1111/gcb.12344
Benito, B. M., Cayuela, L., and Albuquerque, F. S. (2013). The impact of modelling choices in the predictive performance of richness maps derived from species-distribution models: guidelines to build better diversity models. Methods Ecol. Evol. 4, 327–335. doi: 10.1111/2041-210x.12022
Bhat, J. A., Kumar, M., Negi, A. K., Todaria, N. P., Malik, Z. A., Pala, N. A., et al. (2020). Altitudinal gradient of species diversity and community of woody vegetation in the Western Himalayas. Glob. Ecol. Conserv. 24:e01302. doi: 10.1016/j.gecco.2020.e01302
Bhattarai, K. R., and Vetaas, O. R. (2003). Variation in plant species richness of different life forms along a subtropical elevation gradient in the Himalayas, east Nepal. Glob. Ecol. Biogeogr. 12, 327–340. doi: 10.1046/j.1466-822X.2003.00044.x
Bhattarai, K. R., and Vetaas, O. R. (2006). Can Rapoport’s rule explain tree species richness along the Himalayan elevation gradient, Nepal? Divers. Distrib. 12, 373–378. doi: 10.1111/j.1366-9516.2006.00244.x
Bhattarai, K. R., Vetaas, O. R., and Grytnes, J. A. (2004). Fern species richness along a central Himalayan elevational gradient, Nepal. J. Biogeogr. 31, 389–400. doi: 10.1046/j.0305-0270.2003.01013.x
Brown, K. A., Parks, K. E., Bethell, C. A., Johnson, S. E., and Mulligan, M. (2015). Predicting plant diversity patterns in Madagascar: understanding the effects of climate and land cover change in a biodiversity hotspot. PLoS One 10:e0122721. doi: 10.1371/journal.pone.0122721
Burnett, M. R., August, P. V., Brown, J. H., and Killingbeck, K. T. (1998). The influence of geomorphological heterogeneity on biodiversity I. A patch-scale perspective. Conserv. Biol. 12, 363–370. doi: 10.1046/j.1523-1739.1998.96238.x
Cayuela, L., Golicher, D. J., Newton, A. C., Kolb, M., de Alburquerque, F. S., Arets, E. J. M. M., et al. (2009). Species distribution modeling in the tropics: problems, potentialities, and the role of biological data for effective species conservation. Trop. Conserv. Sci. 2, 319–352. doi: 10.1177/194008290900200304
Colombo, A. F., and Joly, C. A. (2010). Brazilian Atlantic Forest lato sensu: the most ancient Brazilian forest, and a biodiversity hotspot, is highly threatened by climate change. Braz. J. Biol. 70, 697–708. doi: 10.1590/S1519-69842010000400002
Connell, J. H., and Orias, E. (1964). The ecological regulation of species diversity. Am. Nat. 98, 399–414. doi: 10.1086/282335
Cramer, M. D., and Verboom, G. A. (2017). Measures of biologically relevant environmental heterogeneity improve prediction of regional plant species richness. J. Biogeogr. 44, 579–591. doi: 10.1111/jbi.12911
Currie, D. J., Mittelbach, G. G., Cornell, H. V., Field, R., Guégan, J.-F., Hawkins, B. A., et al. (2004). Predictions and tests of climate-based hypotheses of broad-scale variation in taxonomic richness. Ecol. Lett. 7, 1121–1134. doi: 10.1111/j.1461-0248.2004.00671.x
D’Amen, M., Dubuis, A., Fernandes, R. F., Pottier, J., Pellissier, L., and Guisan, A. (2015). Using species richness and functional traits predictions to constrain assemblage predictions from stacked species distribution models. J. Biogeogr. 42, 1255–1266. doi: 10.1111/jbi.12485
Dirnböck, T., Dullinger, S., and Grabherr, G. (2003). A regional impact assessment of climate and land-use change on alpine vegetation. J. Biogeogr. 30, 401–417. doi: 10.1046/j.1365-2699.2003.00839.x
Ellenberg, H., and Leuschner, C. (2010). Vegetation mitteleuropas mit den alpen: in ökologischer, dynamischer und historischer sicht. Stuttgart: Utb.
Feilhauer, H., He, K. S., and Rocchini, D. (2012). Modeling species distribution using niche-based proxies derived from composite bioclimatic variables and MODIS NDVI. Remote Sens. 4, 2057–2075. doi: 10.3390/rs4072057
Feng, J., Hu, X., Wang, J., and Wang, Y. (2016). Support for the elevational Rapoport’s rule among seed plants in Nepal depends on biogeographical affinities and boundary effects. Ecol. Evol. 6, 7246–7252. doi: 10.1002/ece3.2473
Ferrier, S., and Guisan, A. (2006). Spatial modelling of biodiversity at the community level. J. Appl. Ecol. 43, 393–404. doi: 10.1111/j.1365-2664.2006.01149.x
Fick, S. E., and Hijmans, R. J. (2017). WorldClim 2: new 1-km spatial resolution climate surfaces for global land areas. Int. J. Climatol. 37, 4302–4315. doi: 10.1002/joc.5086
Fitzpatrick, M. C., Gove, A. D., Sanders, N. J., and Dunn, R. R. (2008). Climate change, plant migration, and range collapse in a global biodiversity hotspot: the Banksia (Proteaceae) of Western Australia. Glob. Chang. Biol. 14, 1337–1352. doi: 10.1111/j.1365-2486.2008.01559.x
Gallardo-Cruz, J. A., Pérez-García, E. A., and Meave, J. A. (2009). β-diversity and vegetation structure as influenced by slope aspect and altitude in a seasonally dry tropical landscape. Landsc. Ecol. 24, 473–482. doi: 10.1007/s10980-009-9332-1
Gao, J., and Liu, Y. (2018). Climate stability is more important than water–energy variables in shaping the elevational variation in species richness. Ecol. Evol. 8, 6872–6879. doi: 10.1002/ece3.4202
Gritti, E. S., Duputie, A., Massol, F., and Chuine, I. (2013). Estimating consensus and associated uncertainty between inherently different species distribution models. Methods Ecol. Evol. 4, 442–452. doi: 10.1111/2041-210X.12032
Grytnes, J. A., and McCain, C. M. (2013). “Elevational trends in biodiversity,” in Encyclopedia of biodiversity, 2nd Edn, Vol. 3, ed. S. A. Levin (Amsterdam: Elsevier), 149–154. doi: 10.1016/B978-0-12-384719-5.00227-6
Guo, Q., Kelt, D. A., Sun, Z., Liu, H., Hu, L., Ren, H., et al. (2013). Global variation in elevational diversity patterns. Sci. Rep. 3:3007. doi: 10.1038/srep03007
Gutiérrez, A. G., and Huth, A. (2012). Successional stages of primary temperate rainforests of Chiloé Island, Chile. Perspect. Plant Ecol. Evol. Syst. 14, 243–256. doi: 10.1016/j.ppees.2012.01.004
Hawkins, B. A., Field, R., Cornell, H. V., Currie, D. J., Guégan, J.-F., Kaufman, D. M., et al. (2003). Energy, water, and broad-scale geographic patterns of species richness. Ecology 84, 3105–3117. doi: 10.1890/03-8006
Kharkwal, G., Mehrotra, P., Rawat, Y. S., and Pangtey, Y. P. S. (2005). Phytodiversity and growth form in relation to altitudinal gradient in the Central Himalayan (Kumaun) region of India. Curr. Sci. 89, 873–878.
Kluge, J., Worm, S., Lange, S., Long, D., Boehner, J., Yangzom, R., et al. (2017). Elevational seed plants richness patterns in Bhutan, Eastern Himalaya. J. Biogeogr. 44, 1711–1722. doi: 10.1111/jbi.12955
Körner, C. (2012). Treelines will be understood once the functional difference between a tree and a shrub is. Ambio 41, 197–206. doi: 10.1007/s13280-012-0313-2
Krömer, T., Acebey, A., Kluge, J., and Kessler, M. (2013). Effects of altitude and climate in determining elevational plant species richness patterns: a case study from Los Tuxtlas, Mexico. Flora Morphol. Distrib. Funct. Ecol. Plants 208, 197–210. doi: 10.1016/j.flora.2013.03.003
Leroy, B., Delsol, R., Hugueny, B., Meynard, C. N., Barhoumi, C., Barbet-Massin, M., et al. (2018). Without quality presence–absence data, discrimination metrics such as TSS can be misleading measures of model performance. J. Biogeogr. 45, 1994–2002. doi: 10.1111/jbi.13402
Lu, L., Cai, H., Yang, Y., Wang, Z., and Zeng, H. (2018). Geographic patterns and environmental determinants of gymnosperm species diversity in China. Biodivers. Sci. 26:1133. doi: 10.17520/biods.2018098
MacArthur, R. H., and MacArthur, J. W. (1961). On bird species diversity. Ecology 42, 594–598. doi: 10.2307/1932254
Malik, Z. A. (2014). Phytosociological behaviour, anthropogenic disturbances and regeneration status along an altitudinal gradient in Kedarnath Wildlife Sanctuary (KWLS) and its adjoining areas. PhD thesis. Uttarakhand: HNB Garhwal University Srinagar.
Malik, Z. A., and Nautiyal, M. C. (2016). Species richness and diversity along the altitudinal gradient in Tungnath, the Himalayan benchmark site of HIMADRI. Trop. Plant Res. 3, 396–407.
Manish, K., Pandit, M. K., Telwala, Y., Nautiyal, D. C., Koh, L. P., and Tiwari, S. (2017). Elevational plant species richness patterns and their drivers across non-endemics, endemics and growth forms in the Eastern Himalaya. J. Plant Res. 130, 829–844. doi: 10.1007/s10265-017-0946-0
Marini, L., Prosser, F., Klimek, S., and Marrs, R. H. (2008). Water-energy, land-cover and heterogeneity drivers of the distribution of plant species richness in a mountain region of the European Alps. J. Biogeogr. 35, 1826–1839. doi: 10.1111/j.1365-2699.2008.01924.x
Marmion, M., Parviainen, M., Luoto, M., Heikkinen, R. K., and Thuiller, W. (2009). Evaluation of consensus methods in predictive species distribution modelling. Divers. Distrib. 15, 59–69. doi: 10.1111/j.1472-4642.2008.00491.x
Mateo, R. G., Croat, T. B., Felicísimo, Á. M., and Munoz, J. (2010). Profile or group discriminative techniques? Generating reliable species distribution models using pseudo-absences and target-group absences from natural history collections. Divers. Distrib. 16, 84–94.
McCain, C. M. (2007). Could temperature and water availability drive elevational species richness patterns? A global case study for bats. Glob. Ecol. Biogeogr. 16, 1–13. doi: 10.1111/j.1466-8238.2006.00263.x
McCain, C. M., and Grytnes, J. A. (2010). “Elevational gradients in species richness,” in Encyclopedia of life sciences (ELS). (Chichester: John Wiley & Sons, Ltd), 1–10. doi: 10.1002/9780470015902.a0022548
Moeslund, J. E., Arge, L., Bøcher, P. K., Dalgaard, T., and Svenning, J.-C. (2013). Topography as a driver of local terrestrial vascular plant diversity patterns. Nord. J. Bot. 31, 129–144. doi: 10.1111/j.1756-1051.2013.00082.x
Mouchet, M., Levers, C., Zupan, L., Kuemmerle, T., Plutzar, C., Erb, K., et al. (2015). Testing the effectiveness of environmental variables to explain european terrestrial vertebrate species richness across biogeographical scales. PLoS One 10:e0131924. doi: 10.1371/journal.pone.0131924
Muller Dombois, D., and Ellenberg, H. (1974). Aims and methods of vegetation ecology. Hoboken, NJ: John Wiley & Sons Inc.
Murray-Smith, C., Brummitt, N. A., Oliveira-Filho, A. T., Bachman, S., Moat, J., Lughadha, E. M. N., et al. (2009). Plant diversity hotspots in the Atlantic coastal forests of Brazil. Conserv. Biol. 23, 151–163. doi: 10.1111/j.1523-1739.2008.01075.x
Myers, N. (1988). Threatened biotas:” hot spots” in tropical forests. Environmentalist 8, 187–208. doi: 10.1007/BF02240252
Naimi, B. (2015). USDM: uncertainty analysis for species distribution models. R package version 1.1–15.
Negi, V. S., Giri, L., and Sekar, K. C. (2018a). Floristic diversity, community composition and structure in Nanda Devi National Park after prohibition of human activities, Western Himalaya, India. Curr. Sci. 115, 1056–1064. doi: 10.18520/cs/v115/i6/1056-1064
Negi, V. S., Joshi, B. C., Pathak, R., Rawal, R. S., and Sekar, K. C. (2018b). Assessment of fuelwood diversity and consumption patterns in cold desert part of Indian Himalaya: implication for conservation and quality of life. J. Clean. Prod. 196, 23–31. doi: 10.1016/j.jclepro.2018.05.237
Negi, V. S., Maletha, A., Pathak, R., and Maikhuri, R. K. (2021). Expansion of a native species and its impacts on alpine ecosystems, Indian Himalaya. Biologia 76, 889–899. doi: 10.1007/s11756-021-00693-1
Negi, V. S., Pathak, R., Rawal, R. S., Bhatt, I. D., and Sharma, S. (2019). Long-term ecological monitoring on forest ecosystems in Indian Himalayan region: criteria and indicator approach. Ecol. Indic. 102, 374–381. doi: 10.1016/j.ecolind.2019.02.035
Nieto, S., Flombaum, P., and Garbulsky, M. F. (2015). Can temporal and spatial NDVI predict regional bird-species richness? Glob. Ecol. Conserv. 3, 729–735. doi: 10.1016/j.gecco.2015.03.005
O’Brien, E. (1998). Water-energy dynamics, climate, and prediction of woody plant species richness: an interim general model. J. Biogeogr. 25, 379–398. doi: 10.1046/j.1365-2699.1998.252166.x
Oli, K. P., and Zomer, R. (2011). Kailash sacred landscape conservation initiative: feasibility assessment report. Lalitpur: International Centre for Integrated Mountain Development (ICIMOD). doi: 10.53055/ICIMOD.545
Oliveira, U., Soares-Filho, B. S., Santos, A. J., Paglia, A. P., Brescovit, A. D., de Carvalho, C. J. B., et al. (2019). Modelling highly biodiverse areas in Brazil. Sci. Rep. 9:6355. doi: 10.1038/s41598-019-42881-9
Oommen, M. A., and Shanker, K. (2005). Elevational species richness patterns emerge from multiple local mechanisms in Himalayan woody plants. Ecology 86, 3039–3047. doi: 10.1890/04-1837
Pan, X., Ding, Z., Hu, Y., Liang, J., Wu, Y., Si, X., et al. (2016). Elevational pattern of bird species richness and its causes along a central Himalaya gradient, China. PeerJ 4:e2636. doi: 10.7717/peerj.2636
Panda, R. M., Behera, M. D., Roy, P. S., and Biradar, C. (2017). Energy determines broad pattern of plant distribution in Western Himalaya. Ecol. Evol. 7, 10850–10860. doi: 10.1002/ece3.3569
Pandey, B., Khatiwada, J. R., Zhang, L., Pan, K., Dakhil, M. A., Xiong, Q., et al. (2020a). Energy–water and seasonal variations in climate underlie the spatial distribution patterns of gymnosperm species richness in China. Ecol. Evol. 10, 9474–9485. doi: 10.1002/ece3.6639
Pandey, B., Nepal, N., Tripathi, S., Pan, K., Dakhil, M. A., Timilsina, A., et al. (2020b). Distribution pattern of gymnosperms’ richness in nepal: effect of environmental constrains along elevational gradients. Plants 9:625. doi: 10.3390/plants9050625
Pineda, E., and Lobo, J. M. (2009). Assessing the accuracy of species distribution models to predict amphibian species richness patterns. J. Anim. Ecol. 78, 182–190. doi: 10.1111/j.1365-2656.2008.01471.x
Pouteau, R., Gillespie, T. W., and Birnbaum, P. (2018). Predicting tropical tree species richness from Normalized Difference Vegetation Index time series: the devil is perhaps not in the detail. Remote Sens. 10:698. doi: 10.3390/rs10050698
Rahbek, C. (1995). The elevational gradient of species richness: a uniform pattern? Ecography 18, 200–205. doi: 10.1111/j.1600-0587.1995.tb00341.x
Rana, S. K., and Rawat, G. S. (2017). Database of himalayan plants based on published floras during a century. Data 2:36. doi: 10.3390/data2040036
Rana, S. K., Gross, K., and Price, T. D. (2019a). Drivers of elevational richness peaks, evaluated for trees in the east Himalaya. Ecology 100:e02548. doi: 10.1002/ecy.2548
Rana, S. K., Price, T. D., and Qian, H. (2019b). Plant species richness across the Himalaya driven by evolutionary history and current climate. Ecosphere 10:e02945. doi: 10.1002/ecs2.2945
Rana, S. K., White, A. E., and Price, T. D. (2022). Key roles for the freezing line and disturbance in driving the low plant species richness of temperate regions. Glob. Ecol. Biogeogr. 31, 280–293. doi: 10.1111/geb.13427
Rawal, R. S., Rawal, R., Rawat, B., Negi, V. S., and Pathak, R. (2018). Plant species diversity and rarity patterns along altitude range covering treeline ecotone in Uttarakhand: conservation implications. Trop. Ecol. 59, 225–239.
Rodríguez, J. P., Brotons, L., Bustamante, J., and Seoane, J. (2007). The application of predictive modelling of species distribution to biodiversity conservation. Divers. Distrib. 13, 243–251. doi: 10.1111/j.1472-4642.2007.00356.x
Rosseel, Y. (2012). lavaan: an R package for structural equation modeling. J. Stat. Softw. 48, 1–36. doi: 10.18637/jss.v048.i02
Saxena, A. K., Pandey, T., and Singh, J. S. (1985). “Altitudinal variation in the vegetation of Kumaon Himalayas,” in Perspectives in environ-mental botany, eds D. N. Rao, K. J. Ahmed, M. Yunus, and S. N. Singh (Lucknow: Print House), 43–66.
Scherrer, D., and Körner, C. (2011). Topographically controlled thermal-habitat differentiation buffers alpine plant diversity against climate warming. J. Biogeogr. 38, 406–416. doi: 10.1111/j.1365-2699.2010.02407.x
Schmidt-Lebuhn, A. N., Knerr, N. J., and González-Orozco, C. E. (2012). Distorted perception of the spatial distribution of plant diversity through uneven collecting efforts: the example of Asteraceae in Australia. J. Biogeogr. 39, 2072–2080. doi: 10.1111/j.1365-2699.2012.02756.x
Schmitt, S., Pouteau, R., Justeau, D., de Boissieu, F., and Birnbaum, P. (2017). ssdm: an r package to predict distribution of species richness and composition based on stacked species distribution models. Methods Ecol. Evol. 8, 1795–1803. doi: 10.1111/2041-210X.12841
Sharma, C. M., Mishra, A. K., Tiwari, O. P., Krishan, R., and Rana, Y. S. (2018). Regeneration patterns of tree species along an Elevational gradient in the Garhwal Himalaya. Mt. Res. Dev. 38, 211–219. doi: 10.1659/MRD-JOURNAL-D-15-00076.1
Shrestha, K. B., and Vetaas, O. R. (2009). The forest ecotone effect on species richness in an arid Trans-Himalayan landscape of Nepal. Folia Geobot. 44, 247–262. doi: 10.1007/s12224-009-9046-9
Shrestha, N., Su, X., Xu, X., and Wang, Z. (2018). The drivers of high Rhododendron diversity in south-west China: does seasonality matter? J. Biogeogr. 45, 438–447. doi: 10.1111/jbi.13136
Sinha, S., Badola, H. K., Chhetri, B., Gaira, K. S., Lepcha, J., and Dhyani, P. P. (2018). Effect of altitude and climate in shaping the forest compositions of Singalila National Park in Khangchendzonga Landscape, Eastern Himalaya, India. J. Asia Pac. Biodivers. 11, 267–275. doi: 10.1016/j.japb.2018.01.012
Stein, A., Gerstner, K., and Kreft, H. (2014). Environmental heterogeneity as a universal driver of species richness across taxa, biomes and spatial scales. Ecol. Lett. 17, 866–880. doi: 10.1111/ele.12277
Sternberg, M., and Shoshany, M. (2001). Influence of slope aspect on Mediterranean woody formations: comparison of a semiarid and an arid site in Israel. Ecol. Res. 16, 335–345. doi: 10.1046/j.1440-1703.2001.00393.x
Stevens, G. C. (1992). The elevational gradient in altitudinal range: an extension of Rapoport’s latitudinal rule to altitude. Am. Nat. 140, 893–911. doi: 10.1086/285447
Syfert, M. M., Brummitt, N. A., Coomes, D. A., Bystriakova, N., and Smith, M. J. (2018). Inferring diversity patterns along an elevation gradient from stacked SDMs: a case study on Mesoamerican ferns. Glob. Ecol. Conserv. 16:e00433. doi: 10.1016/j.gecco.2018.e00433
Testolin, R., Attorre, F., Borchardt, P., Brand, R. F., Bruelheide, H., Chytrý, M., et al. (2021). Global patterns and drivers of alpine plant species richness. Glob. Ecol. Biogeogr. 30, 1218–1231. doi: 10.1111/geb.13297
Thakur, S., Negi, V. S., Dhyani, R., Bhatt, I. D., and Yadava, A. K. (2021). Influence of environmental factors on tree species diversity and composition in the Indian western Himalaya. For. Ecol. Manage. 503:119746. doi: 10.1016/j.foreco.2021.119746
Thakur, S., Negi, V. S., Pathak, R., Dhyani, R., Durgapal, K., and Rawal, R. S. (2020). Indicator based integrated vulnerability assessment of community forests in Indian west Himalaya. For. Ecol. Manage. 457:117674. doi: 10.1016/j.foreco.2019.117674
Turner, J. R. G., Gatehouse, C. M., and Corey, C. A. (1987). Does solar energy control organic diversity? Butterflies, moths and the British climate. Oikos 48, 195–205. doi: 10.2307/3565855
Vetaas, O. R. (1992). Micro-site effects of trees and shrubs in dry Savannas. J. Veg. Sci. 3, 337–344. doi: 10.2307/3235758
Vetaas, O. R. (1993). Spatial and temporal vegetation changes along a moisture gradient in Northeastern Sudan. Biotropica 25, 164–175. doi: 10.2307/2389180
Vetaas, O. R. (2000). Comparing species temperature response curves: population density versus second-hand data. J. Veg. Sci. 11, 659–666.
Vetaas, O. R., and Grytnes, J.-A. (2002). Distribution of vascular plant species richness and endemic richness along the Himalayan elevation gradient in Nepal. Glob. Ecol. Biogeogr. 11, 291–301. doi: 10.1046/j.1466-822X.2002.00297.x
Vetaas, O. R., Paudel, K. P., and Christensen, M. (2019). Principal factors controlling biodiversity along an elevation gradient: water, energy and their interaction. J. Biogeogr. 46, 1652–1663. doi: 10.1111/jbi.13564
Williams, J. N., Seo, C., Thorne, J., Nelson, J. K., Erwin, S., O’Brien, J. M., et al. (2009). Using species distribution models to predict new occurrences for rare plants. Divers. Distrib. 15, 565–576. doi: 10.1111/j.1472-4642.2009.00567.x
Keywords: elevational gradient, environmental variables, species richness, species distribution modelling, Western Himalaya
Citation: Thakur S, Dhyani R, Negi VS and Bhatt ID (2022) Water–energy, climate, and habitat heterogeneity mutually drives spatial pattern of tree species richness in the Indian Western Himalaya. Front. For. Glob. Change 5:1022082. doi: 10.3389/ffgc.2022.1022082
Received: 18 August 2022; Accepted: 10 October 2022;
Published: 10 November 2022.
Edited by:
Yashwant Singh Rawat, Federal Technical and Vocational Education and Training Institute (FTVETI), EthiopiaReviewed by:
Mohd Tariq, Parul University, IndiaRajendra Mohan Panda, Indian Institute of Technology Kharagpur, India
Om Prakash Tiwari, Hemwati Nandan Bahuguna Garhwal University, India
Copyright © 2022 Thakur, Dhyani, Negi and Bhatt. This is an open-access article distributed under the terms of the Creative Commons Attribution License (CC BY). The use, distribution or reproduction in other forums is permitted, provided the original author(s) and the copyright owner(s) are credited and that the original publication in this journal is cited, in accordance with accepted academic practice. No use, distribution or reproduction is permitted which does not comply with these terms.
*Correspondence: Indra D. Bhatt, aWRfYmhhdHRAeWFob28uY29t