- 1Earth and Environmental Science Division, Los Alamos National Laboratory, Los Alamos, NM, United States
- 2Department of Resource and Environment, Moutai Institute, Renhuai, Guizhou, China
- 3School of Forest, Fisheries, and Geomatics Sciences, University of Florida, Gainesville, FL, United States
- 4Department of Geography and Environmental Studies, University of New Mexico, Albuquerque, NM, United States
- 5Hydrology and Remote Sensing Laboratory, Agricultural Research Service, United States Department of Agriculture, Beltsville, MD, United States
- 6School of Earth Systems and Sustainability, Southern Illinois University, Carbondale, IL, United States
- 7Illinois State Water Survey, Prairie Research Institute, University of Illinois Urbana-Champaign, Champaign, IL, United States
- 8Atmospheric Sciences and Global Change Division, Pacific Northwest National Laboratory, Richland, WA, United States
Our understanding of broad-scale forest disturbances under climatic extremes remains incomplete. Drought, as a typical extreme event, is a key driver of forest mortality but there have been no reports on continental-scale quantification of its impact on forest mortality or how it compares to other natural or anthropogenic drivers. Thus, our ability to understand and predict broad-scale carbon cycling in response to changing climate and extreme events is limited. In this study, we applied an attribution approach based on different sources of data to quantify the area and potential carbon loss/transfer in continental U.S. (CONUS) from four types of disturbance: (1) anthropogenic (especially timber harvest); (2) fire; (3) drought-associated; and (4) other from 2000 to 2014. Our results showed that anthropogenic disturbances, fire, drought-associated disturbances, and other disturbances accounted for 54.3, 10.7, 12.7, and 22.3% of total canopy area loss, respectively. Anthropogenic disturbance was the most important driver contributing to 58.1% potential carbon loss/transfer in CONUS for 2000–2014. The potential carbon loss/transfer from natural disturbances (fire, drought, and other) for the same study period accounted for approximately 41.9% of the total loss/transfer from all agents, suggesting that natural disturbances also played a very important role in forest carbon turnover. Potential carbon loss/transfer associated with drought accounted for approximately 11.6% of the total loss/transfer in CONUS, which was of similar magnitude to potential carbon loss/transfer from fire (∼11.0%). The other natural disturbance accounted for 19.3% of potential carbon loss/transfer. Our results demonstrated the importance of the impacts of various disturbances on forest carbon stocks at the continental scale, and the drought-associated carbon loss/transfer data developed here could be used for evaluating the performance of predictive models of tree mortality under droughts.
Introduction
Reports of tree mortality due to climatic extremes such as drought are increasing globally (Van Mantgem et al., 2009; Ganey and Vojta, 2011; Peng et al., 2011; Millar et al., 2012; Allen et al., 2015, 2021; Bendixsen et al., 2015; Assal et al., 2016; Gazol and Camarero, 2022). These rising extreme events, along with fires, insects, and timber harvesting, can substantially threaten forest health. For instance, tree mortality driven or triggered by these disturbances can accelerate changes in forest structure and species composition through altering the size, age, and interspecific competition of a forest (Anderegg et al., 2013; Dyderski et al., 2018). Furthermore, these mortality events can cause widespread and rapid carbon losses to the atmosphere through enhanced decomposition and combustion rates caused by the higher incidence of dead trees (Kurz et al., 2013). The consequential changes in surface albedo can alter the surface energy balance, resulting in important climate feedbacks (e.g., Maness et al., 2013). Combined, these changes can have profound impacts on the human society due to our reliance on healthy forest ecosystems to maximize related ecosystem services (e.g., food, recreations, water purification, flood control, and climate regulation). Therefore, our ability to understand and quantify such losses is of great importance.
During recent decades, many studies have been conducted to investigate forest mortality and explore the causal relationships between forest tree die off and various contributing factors. For example, tree mortality rates in the eastern and central US were found to be strongly associated with stand characteristics (e.g., age, basal area, and tree size) (Dietze and Moorcroft, 2011). Adams et al. (2017) found that widely observed hydraulic failure tends to be one of the most important underlying physiological mechanisms that causes tree death under drought stress. Moreover, there have been many studies of forest declines and tree loss driven by insect outbreaks (e.g., mountain pine beetles) (Meddens and Hicke, 2014; Hicke et al., 2020), fire (Hicke et al., 2016), drought (Klos et al., 2009; Ganey and Vojta, 2011; Yang et al., 2021), and a combination of multiple causal agents (Allen et al., 2021; Knapp et al., 2021; Yang et al., 2021).
Current studies often tend to focus on either the magnitude and intensity of tree mortality or the physiological theories underlying the death. Quantitative attribution studies of tree mortality, particularly those caused by drought, are few and have mostly been limited to relatively fine scales (e.g., plot to watershed) or species-specific responses at larger scales (e.g., Stanke et al., 2021) due to the complexity of forest ecosystem and compound effects among the underlying driving factors. Broad-scale quantitative attribution of such loss at the agent level are rarely found with a few studies focused on single agent (Tyukavina et al., 2022) or empirically attributed disturbance agencies based on forest inventory (Fitts et al., 2022). To better understand how drought impacts the large-scale carbon cycling and associated feedbacks to climate, it is important that we attribute both the area and amount of potential carbon loss to atmosphere or transfer to different products (e.g., wood products for timbers) to different types of disturbances at continental and ultimately global scales.
Currently, estimates of drought-induced mortality at large scales rely heavily on national forest inventories or forest observational networks (Klos et al., 2009; Dietze and Moorcroft, 2011); however, methods and protocols to attribute mortality rates are often inconsistent across different regions/sites or studies (e.g., the definition of drought is different for different studies) and are subjected to sampling errors as they do not have a complete spatial coverage of trees at such large scales. Benefiting from many free and low-cost moderate to high resolution remote sensing (RS) data and fast development in image processing techniques (e.g., machine learning and cloud computing) and the proliferation of data acquisition platforms (e.g., Google Earth Engine), great advancements have been achieved in broad scale monitoring of forest disturbances. For example, the long term Monitoring Trends in Burn Severity (MTBS) data set (1984–2019) provides data on fire occurrence, burn severity and extent across CONUS (Eidenshink et al., 2007), which helps attributing fire-induced tree loss. Continental scale thermal infrared RS based evapotranspiration (ET) and Evaporative Stress Index (ESI) mapping of forest canopies across the entire CONUS (Anderson et al., 2011) enables the explanation of occurrences of observed drought events under different levels of drought stress. This helps to find empirical threshold values that can consistently identify drought-associated (triggered or directly caused) disturbances. Moreover, recent developments in RS-based disturbance detection, including the first high spatial resolution (30 × 30 m) global forest disturbance database developed by Hansen et al. (2013), provide excellent opportunities to evaluate the intensity and magnitude of a variety of anthropogenic and natural disturbances at continental scales. These disturbances are not yet attributed to specific causal agents and thus making it difficult to evaluate the relative importance of different causes (McDowell et al., 2015).
In this study, we developed an attribution approach based on different sources of data to estimate the magnitude and relative contribution of four major disturbance categories to observed disturbances in the continental U.S. (CONUS) over a 15-year period (2000–2014). These disturbances are based on canopy loss estimated from 30-m resolution LANDSAT imagery developed by Hansen et al. (2013). They could represent either potential tree mortality, removal or damages. These four categories include anthropogenic, fire-caused, drought-associated, and other disturbances. Anthropogenic disturbances arise predominately from timber harvesting, land clearing and forest management. The fire-induced disturbances refer to tree mortality or damage from fire scorch or direct burning. Drought is defined based on ESI index thresholds that can potentially lead to tree mortality. The drought-associated disturbances here refer to natural disturbances that have potential linkages to droughts but are not caused by fire or humans. Mechanistically, drought could potentially lead to an increase in water stress and a reduction in carbon storage in plants, as a result of accelerated loss of water and reduction in photosynthetic carbon uptake. The resulting water stress could directly lead to tree mortality or damages through hydraulic function failure. Meanwhile, the resulting carbon starvation and water stress could also lead to reduced plant defense against insects and diseases, which could lead to an increased likelihood of tree mortality (McDowell et al., 2011). Thus, drought associated disturbances could include tree mortality or damages that are directly caused by water stress or indirectly caused by water stress through insect/diseases. The other category includes disturbances from abiotic agents, such as windthrow or frost, and biotic agents that are not related to droughts, such as pathogens or vines. The aims of this study are: (1) to design a hierarchical framework to distinguish different types of disturbances; and (2) to assess the area of disturbance and associated potential carbon stock loss/transfer at regional and CONUS scales. We expected our results to help us better understand the importance of different types of disturbances in carbon balance and the carbon sources/sinks for the forest ecosystem in the CONUS.
Materials and methods
We attributed disturbances to four disturbance categories (anthropogenic, fire, drought, and other) by combining multiple datasets from different sources with various native spatial resolutions. Continental forest disturbance areas during 2000–2014 were identified using the 30 m resolution dataset developed by Hansen et al. (2013). In view of the potential inconsistencies in disturbance detection across time in Hansen’s map (Palahí et al., 2021), we only calculated the lumped sum of areas for different types of disturbance during 2000–2014. Differentiation of disturbance agents began by identifying and removing areas of fire-caused forest loss from the MTBS database and then human-caused loss from the Nation Forest Inventory and Analysis (FIA) database (see Figure 1 for details of the workflow). For pixels that excluded fire-caused and anthropogenic disturbances, determination of climatological drought was based on the ESI drought index, which quantifies standardized anomalies in the ratio of actual to reference ET (AET/RET) and thus signals the relative transpirational flux of trees, with declining values associated with drought (Anderson et al., 2011). Finally, the amount of potential carbon loss/transfer from tree mortality or forest removal was calculated through multiplying these identified agent specific disturbance areas of loss by the per unit aboveground carbon stock estimates of canopy trees from the FIA database and RS imagery (Blackard et al., 2008). The reason why we used only the canopy trees is because Hansen’s remote-sensing-based disturbance detection has a higher level of uncertainty in quantifying the potential impact of disturbance on understory trees.
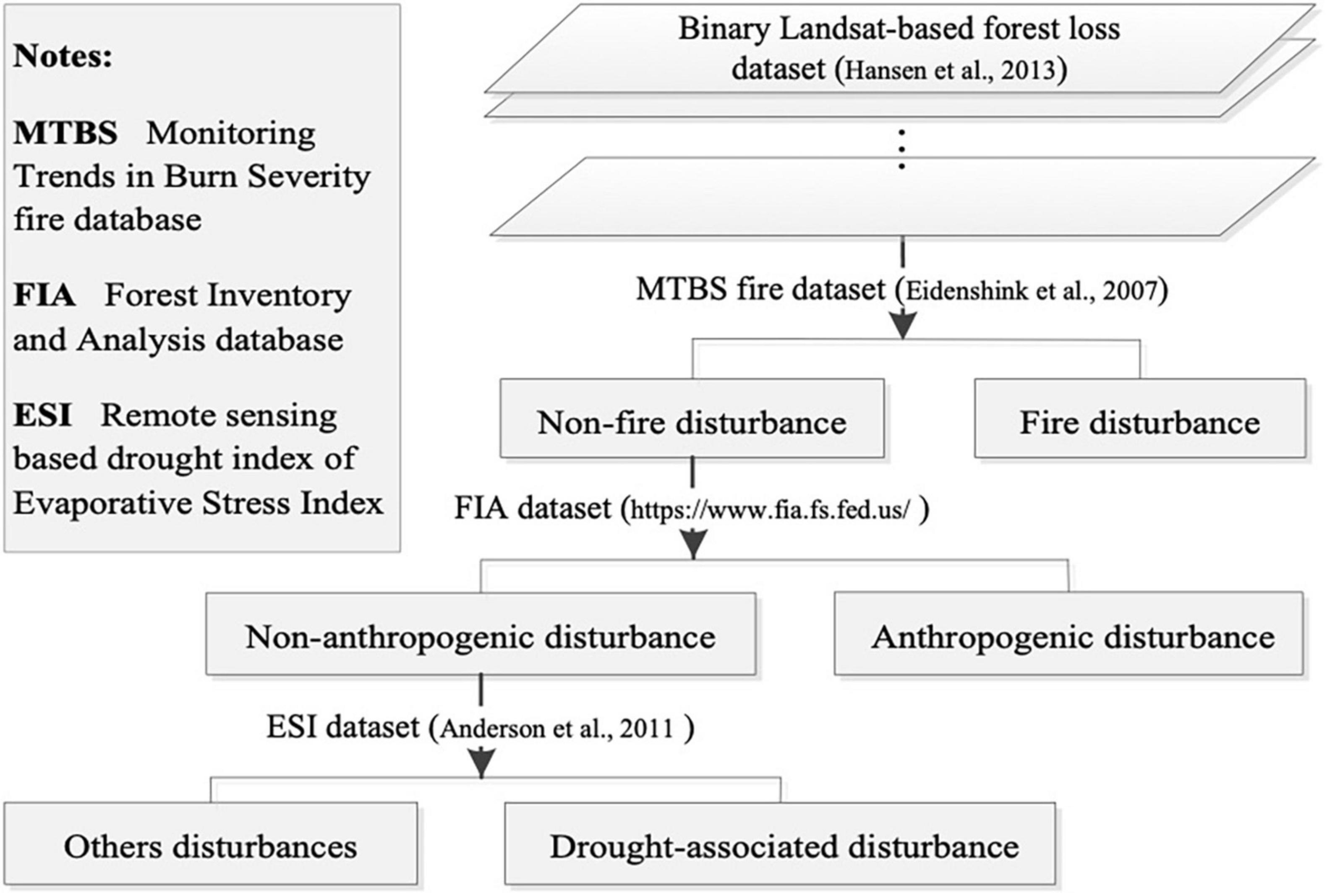
Figure 1. Workflow for the attributions of fire-caused, anthropogenic (especially timber harvest) and drought-associated disturbances.
Monitoring trends in burn severity and forest inventory and analysis datasets
The MTBS dataset is produced and administered jointly by the USDA Forest Service, US Geological Survey (USGS), and Geospatial Technology and Applications Center (GTAC) (Eidenshink et al., 2007). This is a long term (1984-current), longitudinal (allowing cross comparison), and large-scale dataset that covers the entire CONUS, Alaska, Hawaii, and Puerto Rico. The MTBS uses Landsat satellite data, including Landsat Thematic Mapper, Landsat Enhanced Thematic Mapper (ETM+), and Landsat Operational Land Imager (OLI) data, to delineate burned areas and characterize burn severity. Burn severity is classified according to increased greenness into unburned to low, low, moderate, and high classes. All fires that meet the standard of burned area greater than 500 acres in the east and 1,000 acres in the west were included. Data is available from the MTBS website.1 Since all data are generated from Landsat imagery, the MTBS raster datasets are all at 30 m spatial resolution while vector datasets (e.g., the burn area boundary) delineated from the imagery are available at a map scale of 1:24,000 to 1:50,000.
The FIA (also known as the Forest Survey at earlier time) program was established in 1928 to collect, analyze, archive, and publish information on the status, trends, and conditions of all forested lands in the U.S.2 The first official forest inventory started in 1930 and was conducted only in few states (Smith, 2002). Over time, to enhance the ability to analyze and report data of forest land, the program expanded to cover the entire U.S. and was conducted at an annual frequency. Currently, the FIA data are generated from a combination of RS imagery and field surveys, which are carried out by the USDA Forest Service and cover land of all ownerships. To streamline annual estimates of forest attributes while reducing bias, the FIA program utilized an annual panel system, and a multi-phased sampling strategy where a “pre-field phase” was used to determine forested land area based solely on remotely sensed data and the “core phase” was used to establish ground plots (Smith, 2002; Bechtold and Patterson, 2005). These plots are permanent and systematically distributed across the nation where each is measured in a continuous inventory cycle (Bechtold and Patterson, 2005; US Forest Service, 2005; Hoover et al., 2022). The cycle is approximately 5–7 years in the East with 15–20% ground plots are measured each year in the East, and 10 years with 10% ground plots per year are measured in the West. The standard sample grid is approximately one plot per 6,000 acres (Smith, 2002). Key variables including tree species, diameter, and ownership are collected on each plot (Smith, 2002). The cause of tree mortality in this dataset was created by assigning associated agents such as fire, human, and drought if those can be visually identified. If more than one agent led to tree mortality, only the agent that caused the most severe damage to the trees was recorded (US Forest Service, 2016). The collection methods are made spatially and temporally consistent to enable change analysis with the data (for more details, see US Forest Service, 2021).
Preprocessing of forest disturbance dataset
We used the global forest disturbance dataset describing forest canopy area loss from any disturbances developed by Hansen et al. (2013) with a resolution of 0.00025 degree (∼30 m). The disturbance information over CONUS during 2000–2014 was recorded as either 0 (no disturbance) or 1 (disturbance). For feasibility of data management, the disturbance dataset was originally organized by 10° windows (i.e., titles). We further divided each of the tiles into 0.5 × 0.5° windows for operational spatial analysis and aggregation of different disturbance types, resulting in 400 such windows per tile. We chose 0.5° resolution for aggregation because (1) it is one of the generally used resolutions of many global vegetation models within Earth System models; and (2) at this resolution we will get adequate number of FIA plots (>10) for statistically assessing the anthropogenic disturbances.
Identification of fire disturbances
We identified fire-caused disturbances from the forest loss database (Hansen et al., 2013) by measuring their spatial extent convergence with annual fire burned areas derived from the MTBS database (Eidenshink et al., 2007). Burned pixels with different severity levels of low, moderate, and high were shown in 30 m thematic maps derived from Landsat images. Given that low-severity fires are typically non-lethal to canopy trees and generally kill many fewer trees (<30%) than high-severity fires (>85%) (Ghimire et al., 2012), we assumed that higher fire severity led to greater tree mortality rates. Thus, we calculate three levels of potential fire-caused canopy area loss (low, middle, and high), which were calculated by using only the burned pixels with the high severity class, a combination of high and moderate classes, and all the severity classes from MTBS, respectively.
The burned pixels from MTBS dataset and canopy loss pixels from the disturbance dataset were superimposed for the same time period, and the overlapped pixels were identified as locations of tree mortality from fires (Supplementary Figure 1). We then calculated the total fire-caused area loss (Af(i), where i indicates the location of pixel i, which is also the ith half-degree window) within each window by summing all these fire disturbed pixels at 0.00025-degree (∼30 m) resolution within the window. To further identify other disturbance causes, we removed the fire-disturbance locations to create the non-fire forest canopy area loss (Anf (i) = At(i)−Af(i), where At(i) is the total disturbed area in the window) dataset.
Identification of anthropogenic disturbances
We used the FIA database (see Section “Monitoring trends in burn severity and forest inventory and analysis datasets” for detailed descriptions) to estimate the amount of anthropogenic disturbance (Ah(i)) within the half-degree window. Most of the anthropogenic disturbances are attributed to tree removal for various human purposes. The anthropogenic disturbance is identified based on the STATUSCD variable at the tree level, which tell us whether the tree was alive, dead, or removed. If trees were dead/removed, we used the AGENTCD variable to identify whether they were caused by anthropogenic disturbances (code: 80), which includes silvicultural or land clearing activity. The proportion of canopy tree basal area (BA) loss attributed to humans (Ph(i)) was calculated over 2000–2014 at 0.5° spatial resolution, based on the assumption that BAs correspond well to canopy areas across different species. The canopy/sub-canopy differentiation is based on the canopy location variable in the FIA database. Then, we estimated the overall anthropogenic disturbance (Ah(i) = At(i)Ph(i)) within each half-degree window by multiplying the proportion of canopy tree BA loss to anthropogenic causes (Ph(i)) and the total disturbed area (At(i)) within the window. We then removed the areas of anthropogenic disturbances from the non-fire forest loss (Anf (i)) dataset to create a non-fire and non-anthropogenic forest canopy area loss (Anfh(i) = At(i)−Af(i)−Ah(i)) dataset to estimate other disturbance drivers.
Identification of drought-associated disturbances
We developed an ESI threshold approach for drought-associated mortality identification to distinguish forest disturbances associated with climatological drought from dataset (Anfh(i)) that excluded mortality pixels from fire and anthropogenic drivers. In a European study, Senf et al. (2020) used -1.6 standard deviations below the local average for the climatic water balance to define the potential risk of drought; however, a universal drought threshold might be biased for different regions. Thus, in our study, determination of these drought thresholds started with a literature survey to collect the geographic locations of drought-associated tree mortality events that occurred during 2000–2014. Literature included in this survey must either have observed tree mortality with drought considered as the dominant cause or drought during the study period contributed to the mortality (if other disturbance types co-occurred). Based on this criterion, we identified 38 field studies across the CONUS and most studies have more than one qualified location (Supplementary Table 1).
To determine the geo-locations of each individual drought event, we used geographic information of observed tree mortality when reported. For studies where locations were not provided, we used a GIS-based spatial analysis method to extract geographic coordinates based on reported maps. In this way, we identified 292 locations of drought-associated tree mortality events (Figure 2A), which were then used to extract minimum monthly ESI values (Anderson et al., 2011) during the growing season for drought years determined by the survey. Here, we define the growing season for CONUS as a period from April to September. We then determined the annual minimum ESI values in two steps: (1) computing the monthly average ESI values for the growing season, resulting in six raster layers for each year of the study period; and (2) extracting the minimum ESI values from the six layers from step one on a pixel-by-pixel basis using a spatial overlay strategy, resulting in one ESI raster layer per year. Pixel values in this layer indicate the most “severe” drought condition at each location for that year. We then classified these final ESI layers into five classes including incipient-, mild-, moderate-, severe-, and extreme drought based on drought classification standards in Mu et al. (2013).
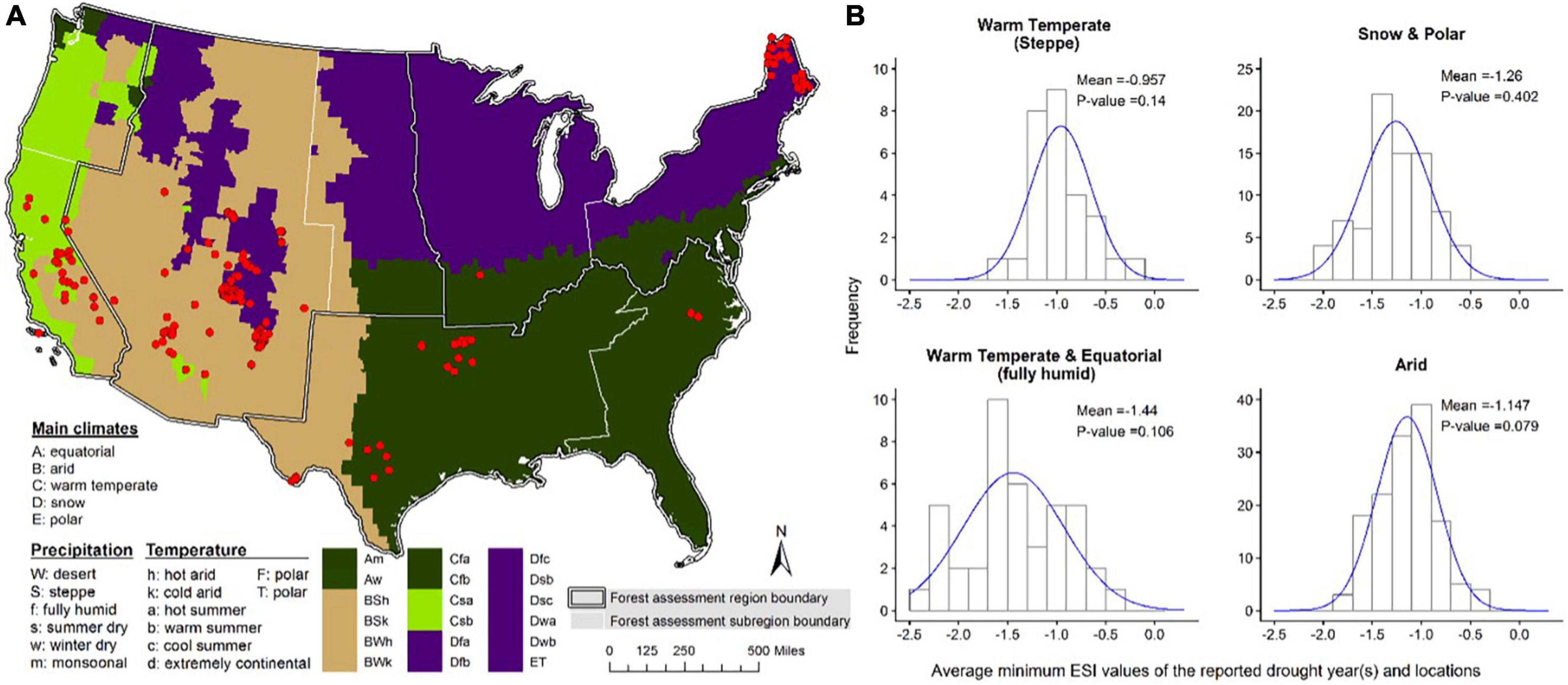
Figure 2. Drought thresholds for identifying drought-associated disturbance in each climate zone of the CONUS. (A) Major climate zones in the CONUS made by modifying Koppen–Geiger climate classification map for the U.S. counties, among which the light green represents the Warm temperate (steppe) climate, light sienna for the Arid climate, purple for the Snow and Polar climate, and darkgreen for the Warm temperate (fully humid) climate. The red dots indicate the locations of the 292 drought-induced tree mortality events identified through literature survey. (B) Normality test results of the drought indices of Evaporative Stress Index (ESI) at the locations of drought-induced tree mortality events within each climate zone.
The determination of ESI-drought mortality thresholds was based on the relationship between drought severity represented by the extracted ESI values and the occurrence of the observed mortality event at each of the 292 locations for the same drought year(s) or period(s) (Supplementary Figure 2). These thresholds were expected to vary by region; thus, we used the Koeppen–Geiger climate classification map to estimate ESI-thresholds for drought-associated disturbances. There is substantial variability in the observed minimum ESI values associated with observed mortality (Figure 2B), which challenges the selection of a suitable mortality threshold for analysis. Application of a more negative ESI threshold to a region could lead to underestimation of drought-associated mortality as it can exclude the impact of less extreme droughts, while a less negative threshold of ESI can lead to overestimation of mortality due to the potential inclusion of false impacts (e.g., insects) that are not linked to droughts. As such, we used mean drought estimates represented by ESI values as drought thresholds for each climate zone to minimize the potential for these biases (Figure 2B). Because the observed ESI thresholds of mortality for different zones approximately follows a Gaussian distribution (Figure 2B), and mean value is close to the expected value, which represents the maximum likelihood estimation of the ESI. Using the defined drought thresholds, we classified the annual ESI layers into binary maps to identify the spatial extent of annual drought and non-drought affected areas for the CONUS over the study period (Supplementary Figure 3).
Since the native ESI product used in this study is at 0.004° resolution, the spatial locations of anthropogenic disturbances at this scale are unknown within each window based on FIA data. Therefore, we first identified pixels of drought-associated disturbances () from the non-fire forest disturbances (Anf (i)) at the 0.004 degree resolution (Supplementary Figure 3). After that, we calculated the proportion of drought-associated disturbance () to the non-fire forest disturbance for each 0.5 × 0.5° analysis window. The areas of drought-associated disturbance obtained at this point might include forest loss from anthropogenic disturbance. To exclude the anthropogenic disturbance, we assumed that the probabilities of anthropogenic (Ah(i)) and non-anthropogenic disturbances (Anfh(i)) associated with droughts are equal within each window. Therefore, we recalculated drought-associated disturbance that does not include fire and human causes (Ad(i)) by multiplying the drought risk (Ld_nf (i)) and forest canopy area loss (Anfh(i)) from non-fire and non-anthropogenic disturbances.
We also considered lag effects of drought on tree mortality, in view that the legacy effect of drought can last for 3–4 years (Anderegg et al., 2015). Specifically, we considered drought-associated tree mortality events to occur with zero to three years of drought conditions. We attributed lagged disturbance using the same logic as for identifying the overall drought-associated mortality. Through tracking the coincidence of forest loss and drought-affected areas on an annual basis with zero to 3-year lags. Losses that occurred in the drought year were identified first. Then, the amount of the 1–3 years lagged losses were identified based on the loss excluding the accumulated loss from previous lagged year(s). This approach allowed evaluating the importance of temporal lags of drought stress on tree mortality.
Identification of other disturbances
The canopy area loss from “other” disturbances was estimated using the difference between the total area of canopy loss and the sum of canopy area loss from the three major disturbance types within each window. After that, we assessed the proportion of canopy area loss from each disturbance type to examine their relative contributions at each 0.5 × 0.5° analysis window.
Quantification of potential carbon loss/transfer from disturbances
We calculated the amount of potential carbon loss/transfer from live biomass to dead pool and atmosphere associated with different types of disturbance by the product of three terms: (1) area change from Hansen’s database; (2) fraction of carbon in canopy trees from the FIA database; and (3) aboveground carbon stock density from the FIA database and RS imagery (Blackard et al., 2008; Supplementary Figure 4). The reason why we used the second term is because it is difficult to know if the understory trees are killed from aerial based remote sensing imagery. The fraction is calculated using carbon stocks in the canopy trees and the total biomass based on the FIA database during 2000–2016. For pixels with missing values, we applied a regional specific mean value. It is possible that the disturbances detected by Hansen et al. (2013) can be caused by defoliation and thus not lead to actual carbon loss/transfer. Because currently there are no mature remote-sensing methodologies to differentiate defoliation versus mortality due to the fast growth of understory after disturbances, we estimated the potential carbon loss/transfer in view that severe defoliation can potentially lead to tree mortality (Davidson et al., 1999). The potential carbon loss does not account for the potentially long time period needed for decomposition or incorporation of carbon into the soil.
Method limitations
There are several potential limitations in our methodology. First, there are uncertainties in the mortalities from fire and thus we used three levels of potential fire-caused canopy area loss (low, middle, and high) to incorporate this uncertainty. Second, potential contribution from anthropogenic disturbances for the entire 0.5 × 0.5° window is assumed to have similar levels of human disturbances as represented by the FIA plots. Thus, it is likely to have biases in our estimation due to spatial scale mismatch. We tried to limit this bias by using a large analysis window so that there are a relatively larger number of FIA plots (>10) for each window. Finally, drought-induced mortality thresholds could be different at much finer resolution based on landscape location, species, and soil properties. In this study, we mainly used climate zones to differentiate the threshold values in view that we focused on a large analysis window of 0.5 × 0.5°. Future studies focused on fine spatial resolutions will need to consider these additional variabilities.
Results
Our analysis of canopy area loss from all disturbances showed that approximate 3.5 × 105 km2 forest area was disturbed for the period of 2000–2014 across CONUS. We found that anthropogenic disturbance caused the highest forest canopy area loss during 2000–2014 and accounted for more than half of all disturbed area (53.9–54.8%) in CONUS (Figure 3), especially for the South region (74.0–74.3%) (Figure 4) where short-interval commercial timber harvesting is a widespread land-use practice. Fire-caused canopy area loss during 2000–2014 represented 10.9% of all disturbed area (middle estimates) (Figure 3). The lower estimate for fire disturbances was 6.4%, which considered only high severity large fires, while the high estimate was 13.9%, which considered the sum of low, moderate and high severity fires, respectively. Regionally, large wildfires were more likely to take place in western regions (mainly west of the Rocky Mountains), thus causing the most fire-driven canopy area loss in those regions (Figure 5C). The total potential aboveground carbon loss/transfer from canopy tree mortality or removal during this period was ∼2.4 × 109 metric tons, which was ∼6.7% of the total forest carbon stock (∼3.6 × 1010 metric tons) in year 2000 and ∼58.1% of total carbon loss/transfer. The combined carbon loss/transfer from canopy trees due to natural disturbances (fire, drought, and others) was approximately 70.2–73.7% of the loss from anthropogenic disturbance, suggesting that natural disturbances play an almost equally important role in forest carbon loss/transfer.
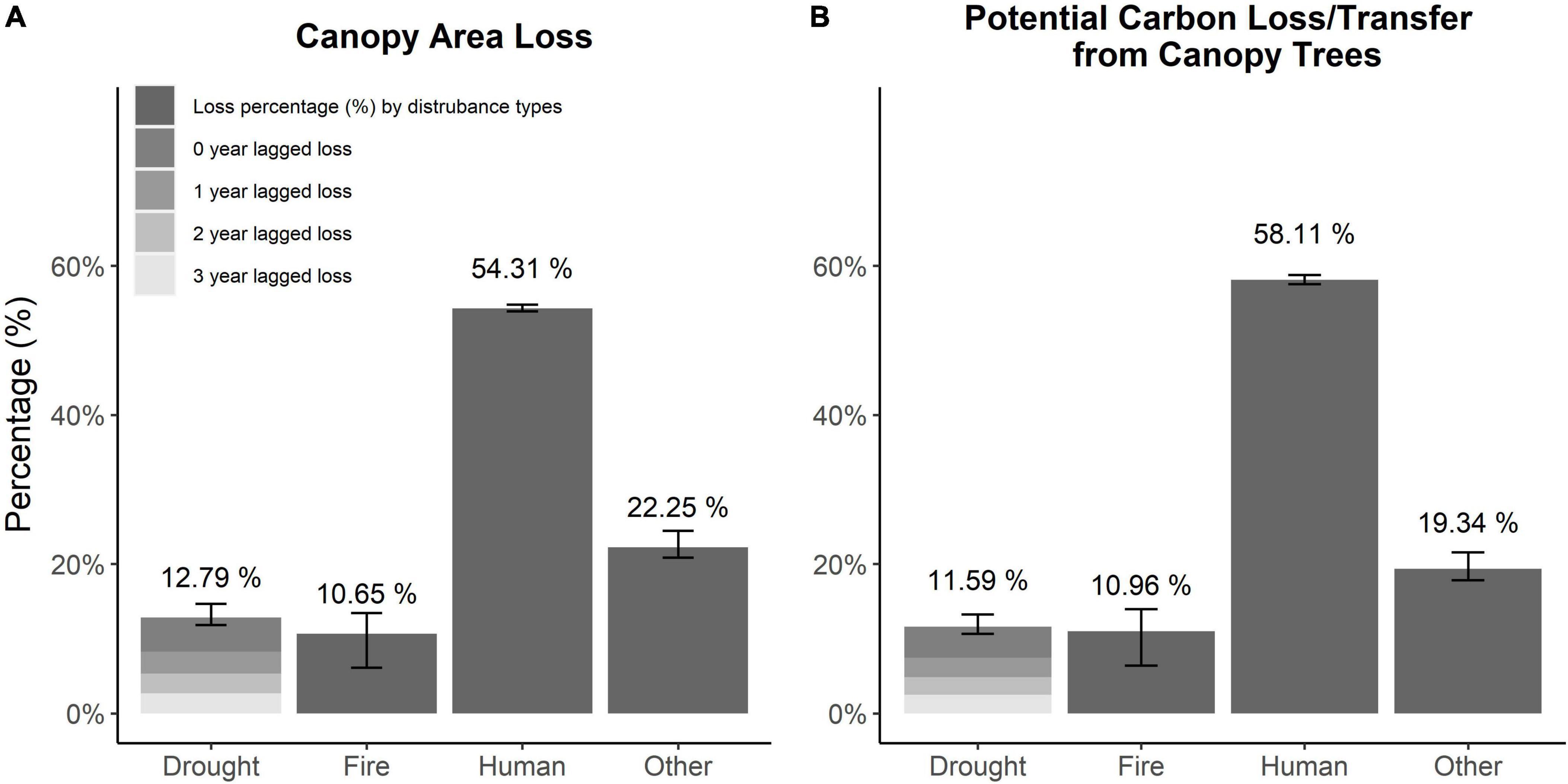
Figure 3. Forest area and potential carbon loss/transfer from forest loss in CONUS for 2000–2014. The percentage of area (A) and potential aboveground carbon stock loss/transfer from canopy trees (B) from forest loss by disturbance types, including fire, anthropogenic (especially timber harvest), drought-associated, and others. Error bars indicate the range of lower to upper estimates for each category.
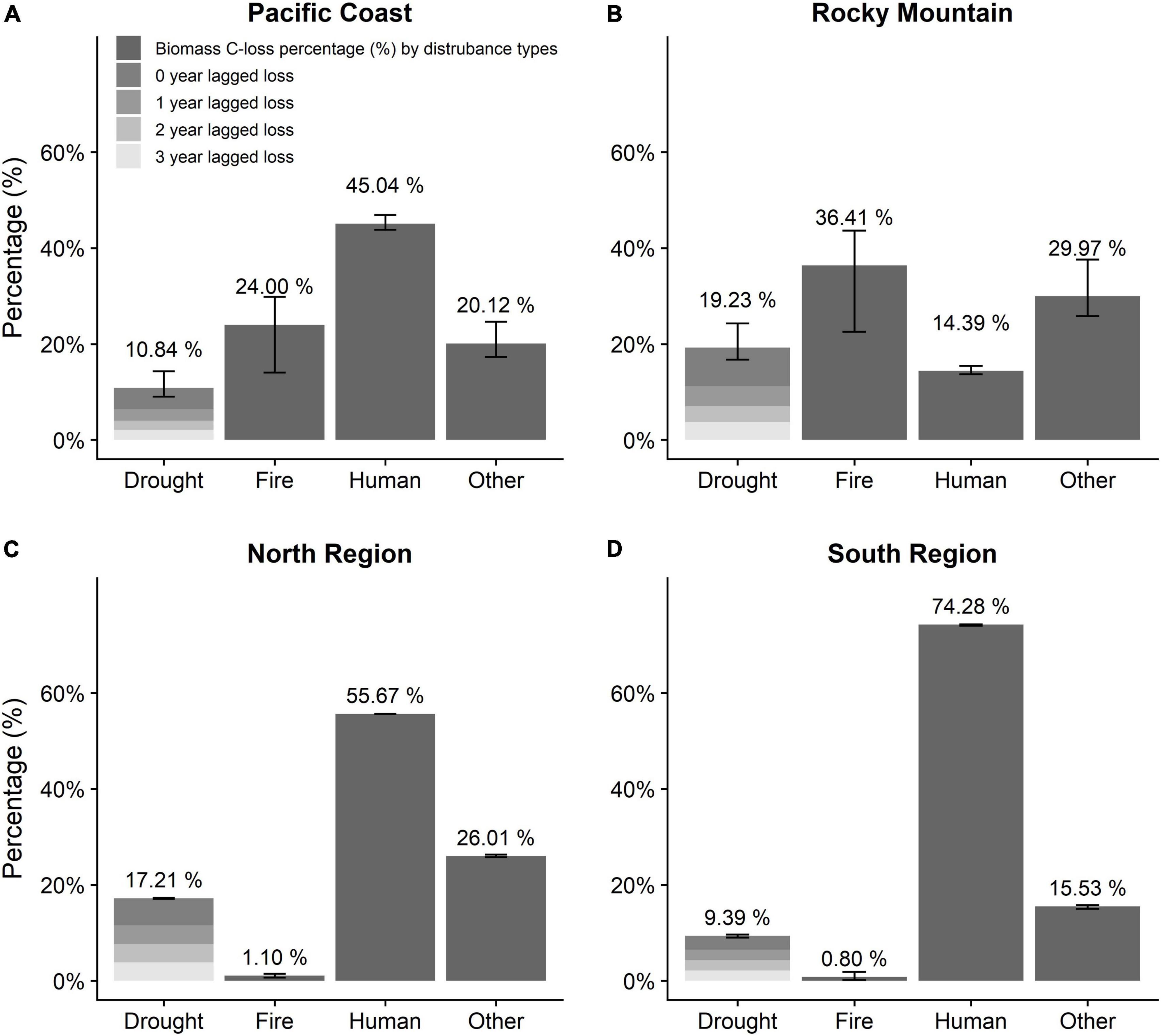
Figure 4. Variation of aboveground biomass carbon loss/transfer from canopy trees in the four major forest service regions in CONUS for 2000–2014. (A) Pacific Coast region. (B) Rocky Mountain region. (C) North Region. (D) South Region. Numbers over the gray bars are the percentage of loss to each of the major disturbances, including fire, anthropogenic (especially timber harvest), drought-associated, and others. Error bars indicate the range of lower to upper estimates for each category.
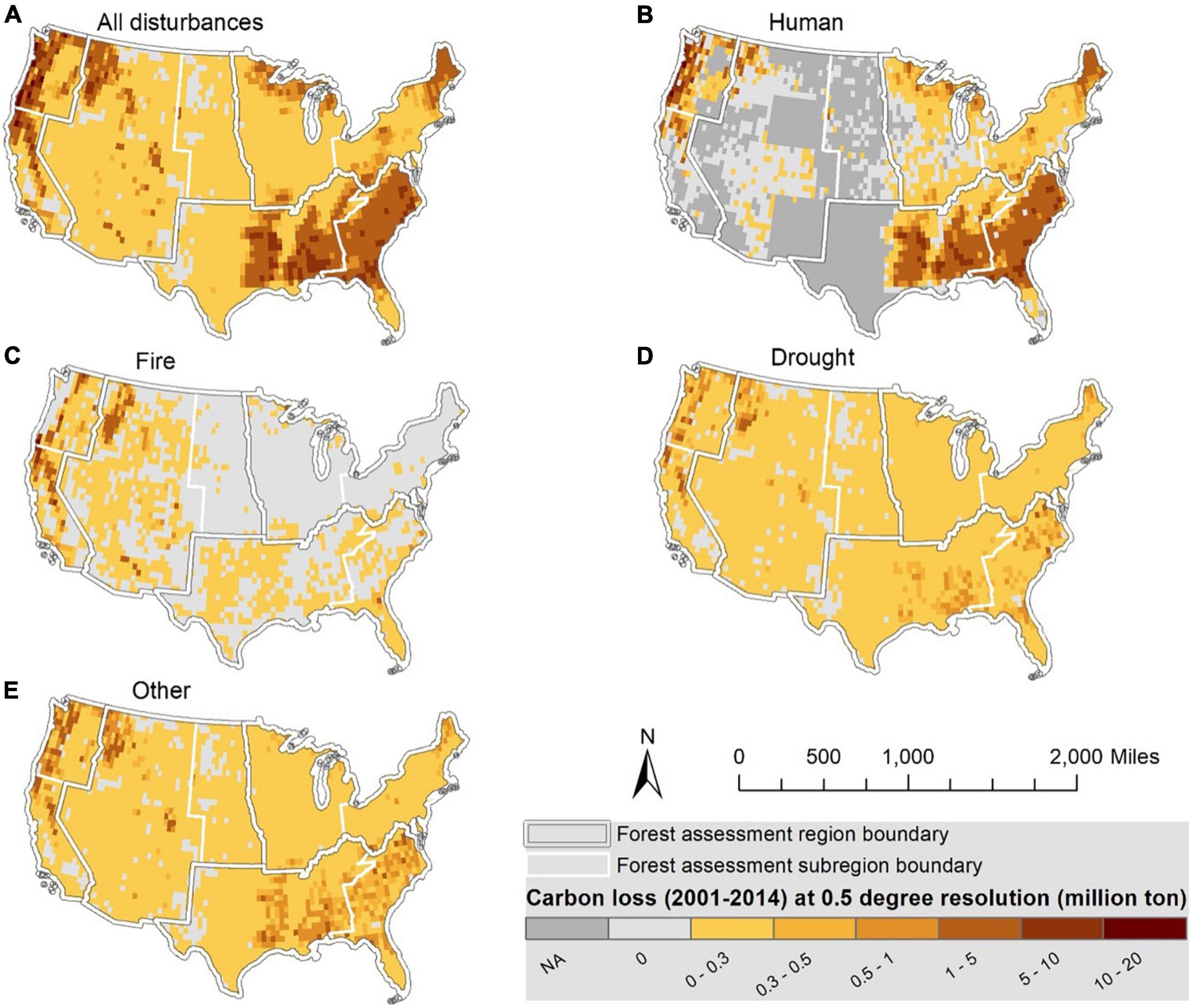
Figure 5. Distribution of potential aboveground carbon stock loss/transfer from canopy trees in CONUS for 2000–2014. A disturbances (A), anthropogenic disturbances (B), fire disturbances (C), drought associated disturbances (D), and other disturbances (E).
For the drought-associated disturbance, our literature survey indicated a positive relationship between observed tree mortality events and drought severity. Our analysis of 292 selected drought-associated mortality events in CONUS (Figure 2) and the co-located drought indices of ESI showed that the mortality events were most likely to occur due to the moderate to severe droughts (ESI < −0.9) (Supplementary Figure 2). The frequency of observed mortality events increases as drought conditions become more severe. Specifically, 22.6, 31.1, and 39.3% of all observed mortality events have occurred at moderate, severe, and extreme drought conditions, respectively. ESI thresholds used to determine pixels of drought-associated mortality were found to have large regional differences across different climate zones (Figure 2B). The thresholds are more negative in the eastern CONUS compared to those in the west, suggesting that vegetation stress leading to mortality is associated with stronger region-specific negative anomalies in the ratio of AET/RET.
By using the ESI drought thresholds for disturbed area excluded from fires and anthropogenic drivers and considering the lagged effects of droughts for 3 years, our analysis showed that drought-associated disturbance occurred extensively in many regions of CONUS during the period of study (Supplementary Figure 5c). We estimated 4.1–5.1 × 104 km2 of forest canopy area loss and 2.6–3.2 × 108 metric tons of forest carbon loss were associated with droughts from 2000 to 2014 in CONUS. The Rocky Mountain region exhibited the highest proportion of carbon loss by drought associated disturbances (16.7–25.6%), followed by North regions (16.7–17.1%) and the Pacific Coast (10.5–17.8%) (Figure 4). Continentally, drought-associated disturbance accounted for 12.8% canopy area loss and 11.6% of carbon loss/transfer, which are of similar magnitudes to those resulting from fire (10.7 and 11.0% for canopy area loss and carbon loss/transfer, respectively, Figure 3).
Our analysis also showed high regional variability in types and sizes of the loss/transfer (Figures 4, 6 and Supplementary Figure 5; see Figure 5 for maps of different regions). Geographically, most of the potential canopy carbon stock loss/transfer is concentrated in the Pacific Coast and the South (Figure 5A). The carbon loss in these regions is about four times greater than that in all other regions (Supplementary Figures 7a–d). The Southern region itself has more than half (52.2%) of the total carbon loss/transfer from all type of disturbances (Supplementary Figure 7b). Anthropogenic disturbances in this region contribute to 70.2–73.7% of the carbon loss and the drought-associated disturbances contribute to 9.0–9.6% of all carbon loss/transfer (Supplementary Figure 7d). Comparably, although the canopy area loss in the Pacific Coast region is much less compared to that in the South (Supplementary Figure 7a), this region has experienced more intensive potential carbon loss/transfer (Supplementary Figure 7b). This is especially true for the Pacific Northwest (PNW) region that experienced ∼10.1% of the total area of canopy loss (Supplementary Figure 7c) but contributed to ∼20.7% of the total potential carbon stock loss/transfer in CONUS (Supplementary Figure 7d). Such regional differences were primarily caused by the large spatial variations in carbon density. For example, compared to that in other regions, the PNW region has a much higher carbon stock density than other regions (Supplementary Figure 4).
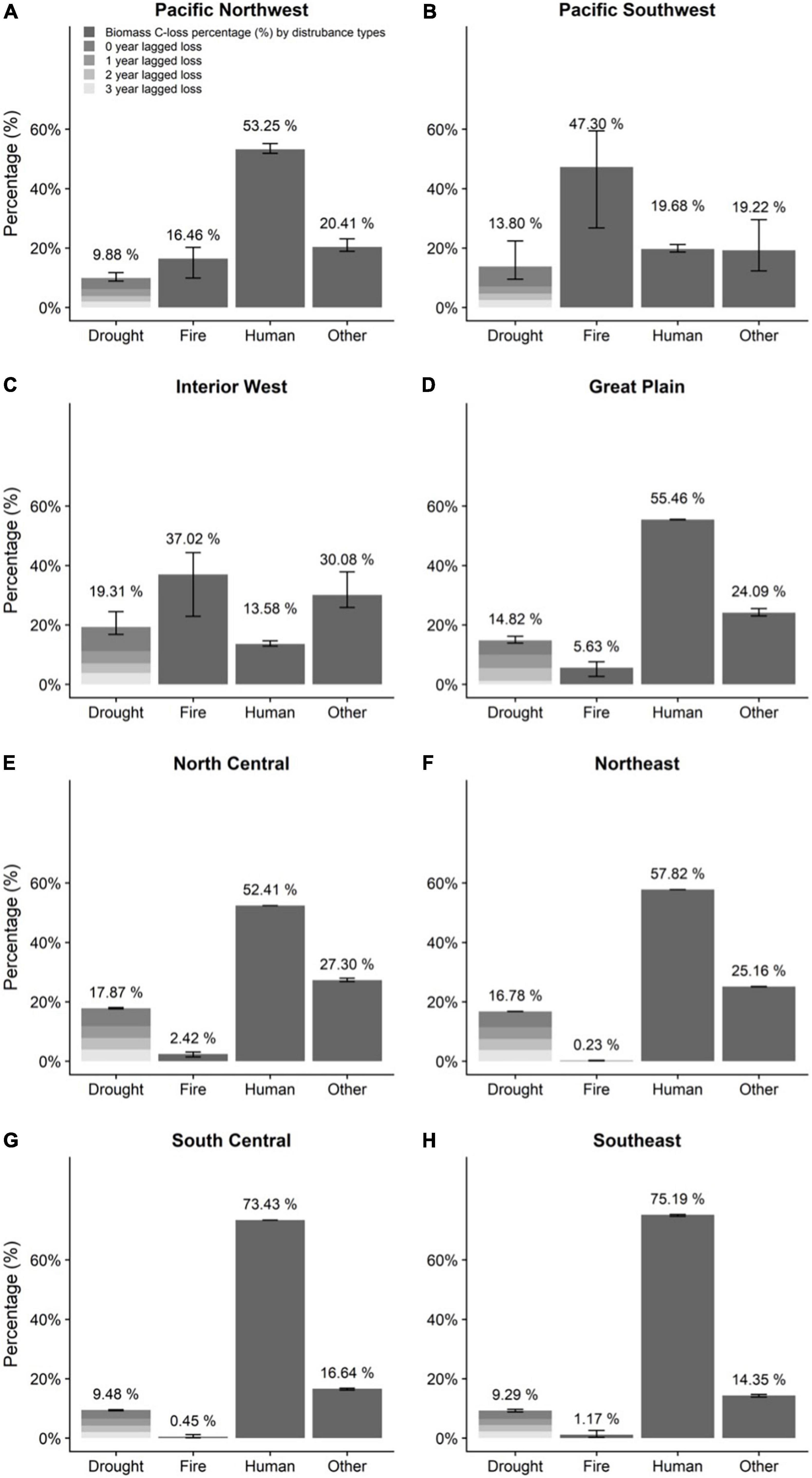
Figure 6. Variation in potential aboveground carbon loss/transfer for canopy trees in the eight forest service sub-regions in CONUS for 2000–2014. (A) Pacific Northwest. (B) Pacific Southwest. (C) Interior West. (D) Great Plains. (E) North Central. (F) Northeast. (G) South Central. (H) Southeast. Numbers over the gray bars are the percentage of loss/transfer for each of the major disturbances, including fire, anthropogenic (especially timber harvest), drought-associated, and others. Error bars indicate the range of lower to upper estimates for each category.
Discussion
In this study, we quantified the potential carbon loss/transfer in disturbed area in response to multiple agents, including anthropogenic (especially timber harvest), fire, drought, and other drivers. As far as we know, this is a pioneering study to attribute different types of disturbances including drought at the continental US scale. Our results highlight the importance of drought-associated disturbance to carbon balance in US forests, which is an important indicator for forest health under climate change. We found that the drought is associated with more potential carbon loss than fire during 2000–2014. Because drought-associated disturbances are most likely related to biotic agents such as insects and disease, we compared the disturbed area and potential carbon loss estimated from our study to previous studies focusing on biotic agents. Our result showed that drought accounts for 12.69% of disturbed area, while fire accounts for 10.65% of disturbed area. This result is consistent with a relevant study showing that carbon release from biotic disturbances is of similar magnitude to that from fire in the US during the period 1997–2015 (Kautz et al., 2018). However, we estimated that drought-associated disturbances caused ∼17.2–21.4 Mt carbon/year aboveground carbon loss from canopy trees. Our upper limit is close to reported value of ∼13.2 ± 7.3 Mt total forest carbon loss from Kautz et al. (2018). Our lower limit is higher than that from Kautz et al. (2018). This difference could result from three reasons. First, the study of Kautz et al. (2018) focused on biotic agents (e.g., insects and pathogens) while our study defines drought-associated factors to not only include drought-induced insect outbreaks, but also independent climatic droughts. Second, Kautz et al.’s (2018) used US insect and disease survey (IDS) database,3 which is derived from the airborne flight surveys without complete coverage in the domain, where as our study uses satellite imagery with a more complete land area coverage (Hansen et al., 2013). Third, the Kautz et al. (2018) study is based on a constant 10% mortality from area identified from IDS, but in reality, this number likely exhibits high spatial variability. By contrast, our study used Hansen’s disturbance dataset, which used criteria based on spectral signal change that should indicate canopy loss and varies spatially. Forest fire mortality area for western US is about 0.12–0.27 Mha/year during 1997–2012 (Hicke et al., 2016), which is similar to our reported value of 0.13–0.27 Mha for fire-induced canopy loss. Meanwhile, our study estimated an area of 0.9–0.14 Mha/year of drought associated disturbances, which is within the reported range of tree mortality area 0.03–0.38 Mha/year from bark beetles during 1997–2012. We would like to point out that our values are only for droughts and drought-associated biotic disturbance and thus this number could be smaller than those in the studies that reported tree mortality from only insects, which might include non-drought associated outbreaks.
Another recent study investigated the total excess forest canopy loss to drought-related disturbances in Europe (Senf et al., 2020). This study determined that drought caused an additional 500,000 ha of excess forest mortality between 1987 and 2016 in Europe, which accounts for 1.4% of total canopy mortality. In our study, drought accounts for about 12% of canopy disturbances. This difference could result from three possible reasons: (1) climate differences between US and Europe; (2) incorporation of 13-years of data prior to 2,000 in the European analysis that may have had a vastly different climate signature relative to the post-2,000 data; and (3) the differences in the definition of drought and its lag effect. In our studies, the drought is defined based on mean ESI for observed mortality events, which is at about one standard deviation below the mean. Senf et al. (2020) used -1.6 standard deviations below the local average for the climatic water balance to define the potential risk of drought, which would exclude more regions considered to be experiencing drought relative to our study. We have also considered 3 years of lag impact by drought in our studies, while Senf et al. (2020) did not consider multi-years of lag effects.
We want to highlight that anthropogenic disturbance is the most important driver contributing to potential carbon loss/transfer in CONUS (∼54%) during 2000–2014. This suggests that human is still the major driver of potential carbon loss/transfer in US with heavy harvesting in the Northwestern and Southeastern US. Our estimation of anthropogenic disturbance is very close to the number reported by Fitts et al. (2022) based on the proportion of trees disturbed for the silviculture category. Fitts et al. (2022) reported that more than 25% tree disturbance is caused by fire, which is much higher than the percentage of canopy area loss (10.7%) caused by fire reported by our study. This could result from the difference in our definition of fire disturbance, as we focused on the canopy tree loss from the RS perspective, while Fitts et al. (2022) used all the trees in the inventory and not only included dead trees but also partially damaged trees based on FIA tree variable: DAMAGE_AGENT_CD. Among natural disturbances, about 19.4% of potential carbon loss/transfer is in the other category, which is of similar magnitude to fire and drought. This suggests that other types of disturbances still play an important role in US carbon turnover.
There are different types of uncertainty in our analysis, including uncertainties in data sources (e.g., the sampling errors from FIA, or misclassification in satellite imagery), attribution of canopy loss based on fire severity, and drought thresholds for tree mortality. Designing future studies to reduce these uncertainties could be critical to improve our understanding of drought-induced tree mortality. There is uncertainty in estimated carbon loss induced by “other” disturbances, which still accounts for a large proportion of the total carbon loss (>19% in CONUS). These disturbances may also include fire-caused, human-made, and drought-associated disturbances that are not accurately accounted for in our analysis. It is especially true for the anthropogenic disturbance category, which is based on the FIA sampling and is more sparsely sampled than the satellite imagery, potentially leading to a bias in human-caused disturbances. We assumed that potential contribution from anthropogenic disturbances for the entire 0.5 × 0.5 window experienced similar level of human disturbances as represented by the FIA plots. If there is potential under/over-estimation as represented by FIA plots, then it is possible to have a bias. Future studies based on the temporal changes in spectral signal of different disturbance types (Kennedy et al., 2007) could be a useful approach to improve the accuracy of disturbance attribution mapping. Finally, the temporal flux of carbon loss/transfer will depend on the disturbance types. For example, the carbon release to atmosphere by severe crown fire could be instant; however, it could take decades to decompose and release carbon to atmosphere for standing dead stems caused by ground fire or drought. In our study, we mainly focused on the potential loss/transfer by different disturbances. Future modeling studies could be conducted to estimate the carbon release at a finer temporal resolution.
The key step in this study for attributing drought-associated mortality was to determine the ESI thresholds for drought-associated disturbances. As described in Section “Materials and methods,” we removed the pixels of disturbance from fire and anthropogenic disturbances and used the mean values of the ESI drought indicator as a criterion to define drought-associated disturbance on the remaining disturbed pixels. Our analysis does not distinguish abiotic disturbances such as windthrow and frost damage that have no linkage to droughts; however, these types of disturbance are substantially less frequent compared to fire, insects and harvest and therefore the bias should be relatively small. The drought-associated carbon loss could be partially linked to potential weakening of tree defense against insects and diseases during drought (Anderegg et al., 2015; Huang et al., 2020; Robbins et al., 2022). To test this hypothesis, we conducted additional analyses to assess whether drought played a role in insect/disease-caused disturbances by comparing the mean ESI values of pixels affected by as insect/disease that were defined by the US IDS database to pixels within a region. Our finding supports the hypothesis that droughts contribute to the insect/disease-caused disturbances with variabilities for different year(s) across different regions (Supplementary Figures 6a–d). Previous research suggested that there is no clear signal of drought impact on southern pine bark beetle for the southern US (Kolb et al., 2016); however, we found that in the Rocky Mountain region, insect/disease affected areas had significant lower ESI values for the year of 2003 and 2008–2011 (Supplementary Figure 6c). This indicates that trees are more stressed under droughts and insect attacks. The finding from this additional analysis highlights the difficulties of disentangling insect/disease induced mortality from drought-associated mortality, which remains a major research challenge. In cases where both disturbances coexist and interact, it is even more difficult to determine the actual cause of tree mortality and the percent contribution of each cause to the magnitude of mortality.
Regionally, we found that the Pacific Coastal and Southern regions together accounted for much more potential carbon loss/transfer due to tree canopy loss from drought-associated drivers (∼70%; Figure 5D and Supplementary Figures 8a–d) than the Rocky Mountain and Northern regions (∼30%; Supplementary Figures 8a–d). However, the Rocky Mountain region has received considerable attention in the scientific community regarding drought-associated tree mortality (Breshears et al., 2005; Huang et al., 2010). The low contributions of drought-associated potential carbon loss (∼17%) from this region could be related to two reasons. First, the Hansen et al. (2013) disturbance detection approach only focuses on forests with > 5 m height and thus omits a majority of Pinyon–Juniper woodland that has experienced substantial mortality in this region (Meddens et al., 2015). Second, the carbon stock density for a large portion of this region is relatively low compared to the Pacific region (Supplementary Figure 4). For the Northern region, the low contribution is in agreement with limited reports on drought-associated tree mortality except in the northwest part of the region (Supplementary Table 1 and Supplementary Figure 2). The relatively high contribution from the Southern region is related to high carbon-stock density and the potential linkage of drought to insect/disease-induced mortality (see the detailed analysis above and Supplementary Figure 6d).
The prediction of the terrestrial carbon cycle over the next 50 to 100 years is one of the most important sources of uncertainty in Earth system models’ prediction of global climate (Friedlingstein et al., 2014). A key component of that uncertainty lies in our poor representation of mechanisms driving climate-induced tree mortality in Earth system models (McDowell et al., 2011; Sitch et al., 2015) as tree mortality is a key driver for carbon stocks and fluxes (Massoud et al., 2019). Our data on drought-associated disturbances will provide an urgently required dataset to evaluate the performance of current models on simulating terrestrial vegetation responses to droughts, which could substantially improve our capability of predicting global carbon cycles and future climates in view of the risk of increasing drought under future global warming (Cook et al., 2015) and potential tree mortality resulting from drought (McDowell et al., 2016).
Conclusion
We applied an attribution approach based on multi-source independent datasets to quantify disturbed area and potential carbon loss/transfer from multiple forest disturbances, allowing investigation of their relative contribution to the loss/transfer. The estimates of such potential loss/transfer at the continental scale can be used to evaluate and improve dynamic vegetation models and help us understand forest health under climate changes. There are limitations to the methods developed in this study, such as heavy reliance on the accuracy of input datasets and limited scalability of ESI thresholds used to partition drought-associated losses (e.g., identified threshold values might be difficult to scale beyond US). However, our new approach enables us to differentiate disturbed area among fire, anthropogenic, drought-associated, and other disturbances. This not only provides critical information for comparing relative impacts of various disturbances on the observed tree mortality/removal, but also allows quantification of locations, areas, and associated potential carbon loss/transfer for each disturbance type. Our primary results indicated that (1) the potential loss/transfer of carbon was largely caused by anthropogenic disturbance; (2) the potential carbon loss/transfer associated with drought exceeded that from fire for 2000–2014 over the CONUS, and varied widely among regions. Our results provide a unique synthesis of continental level identification of important disturbance agents, especially drought-associated forest disturbances and quantification of potential carbon loss/transfer. This work provides critical information for more accurately predicting vegetation responses to ongoing changes in climate and disturbance regimes.
Data availability statement
Our analysis was based on open-source data for MTBS, FIA, and Remote sensing-based forest loss data. The MTBS data can be downloaded from https://www.mtbs.gov/. The FIA data can be downloaded from https://apps.fs.usda.gov/fia/datamart/datamart.html and Hansen’s data can be downloaded from: https://earthenginepartners.appspot.com/science-2013-global-forest. ESI dataset is accessed from Anderson et al. (2011).
Author contributions
CX conceived the original idea and together with NM supervised the project. CX and NM verified the analytical methods and supervised the findings of this work. MW carried out most computations and analytic calculations. DJ and GQ helped with raw data processing. MW wrote the manuscript with support from CX and NM. CX, NM, CA, MA, GW, DJ, and GQ provided critical feedback and helped to shape the data analysis and manuscript. KS helped revising the manuscript on potential carbon loss/transfer. All authors discussed the results and contributed to the final manuscript.
Funding
MW, CX, and KS acknowledged support from the Los Alamos National Laboratory (LANL), Laboratory Directed Research and Development (LDRD) program’s project titled Biotechnology for Regional Climate Resilience (#20210921DI), and Global Tree Mortality Prediction Based on Hydraulic Function Failure (20150030ER). CA acknowledged support from the U.S. Geological Survey’s Ecosystems Mission Area and the USGS Climate Research and Development Program. DJ acknowledged support by the USDA National Institute of Food and Agriculture, McIntire Stennis project (018790).
Acknowledgments
We thank Christopher Hain from NASA for providing support on ESI data.
Conflict of interest
The authors declare that the research was conducted in the absence of any commercial or financial relationships that could be construed as a potential conflict of interest.
The reviewer JH declared a shared affiliation with the author DJ to the handling editor at the time of review.
Publisher’s note
All claims expressed in this article are solely those of the authors and do not necessarily represent those of their affiliated organizations, or those of the publisher, the editors and the reviewers. Any product that may be evaluated in this article, or claim that may be made by its manufacturer, is not guaranteed or endorsed by the publisher.
Supplementary material
The Supplementary Material for this article can be found online at: https://www.frontiersin.org/articles/10.3389/ffgc.2022.693418/full#supplementary-material
Supplementary Figure 1 | Illustration of fire induced forest disturbance identification. The overlapping portions of forest fires from Monitoring Trends in Burn Severity (MTBS) database (polygons in gray) and the remotely detected forest loss (pixels in red) indicates fire caused disturbances.
Supplementary Figure 2 | Frequency of observed drought-associated tree mortality events (2000–2014) along drought intensity (blue bars) and frequency of ESI values extracted from monthly average Evaporative Stress Index (ESI) layers at 292 drought sites (7 months × 14 years × 292 drought events = 28,616 ESI values) along drought intensity for the same period (gray histogram). Numbers over bars show frequency of observed drought events under different drought stress.
Supplementary Figure 3 | Classification maps of annual drought and non-drought affected area in the CONUS for 2001–2014. This classification is based on the thresholds determined by observed drought-associated mortality events within each climate zone (Figure 2A).
Supplementary Figure 4 | Aboveground biomass carbon density (Kg C/m2) across the CONUS at 0.5-degree resolution measured in 2000.
Supplementary Figure 5 | Percentage of potential forest carbon loss/transfer from canopy trees at 0.5-degree resolution by major disturbance types [(a) anthropogenic disturbances; (b) fire disturbances; (c) drought-associated disturbances; and (d) other disturbances] in CONUS for 2000–2014.
Supplementary Figure 6 | Impact of mean Evaporative Stress Index (ESI) on insect/diseases outbreak for major forest resource assessment regions. (a) North region; (b) Pacific coast; (c) Rocky Mountain; and (d) South region. The dotted green lines indicate the selected drought threshold used for identifying drought-associated insect and disease survey (IDS) patches that recorded as insect/diseases outbreak for the four regions. ESI values that are within a defined distance (0.04 degree) of IDS patches were used in the calculation of the mean ESI. To examine the temporal impact of drought stress on the associated forest disturbance, we considered 1–3 years lagged loss.
Supplementary Figure 7 | Regional and subregional variations in canopy area and potential carbon loss/transfer from disturbances. (a–d) The percentage of area and carbon loss from disturbances in the four major forest service regions (a,b) and the eight sub-regions (c,d) of the CONUS for 2000–2014.
Supplementary Figure 8 | Regional and sub-regional variations in forest area and potential carbon loss/transfer from drought-associated forest disturbance in CONUS for 2000–2014. (a–d) The percentage of area and carbon loss from drought-associated tree mortality in the four major forest service regions (a,b) and the eight sub-regions (c,d).
Footnotes
References
Adams, H. D., Zeppel, M. J. B., Anderegg, W. R. L., Hartmann, H., Landhäusser, S. M., Tissue, D. T., et al. (2017). A multi-species synthesis of physiological mechanisms in drought-induced tree mortality. Nat. Ecol. Evol. 1, 1285–1291. doi: 10.1038/s41559-017-0248-x
Allen, C. D., Breshears, D. D., and McDowell, N. G. (2015). On underestimation of global vulnerability to tree mortality and forest die-off from hotter drought in the Anthropocene. Ecosphere 6:55. doi: 10.1890/ES15-00203.1
Allen, K. J., Verdon-Kidd, D. C., Sippo, J. Z., and Baker, P. J. (2021). Compound climate extremes driving recent sub-continental tree mortality in northern Australia have no precedent in recent centuries. Sci. Rep. 11:18337. doi: 10.1038/s41598-021-97762-x
Anderegg, W. R. L., Kane, J. M., and Anderegg, L. D. L. (2013). Consequences of widespread tree mortality triggered by drought and temperature stress. Nat. Clim. Change 3, 30–36. doi: 10.1038/nclimate1635
Anderegg, W. R. L., Schwalm, C., Biondi, F., Camarero, J. J., Koch, G., Litvak, M., et al. (2015). Pervasive drought legacies in forest ecosystems and their implications for carbon cycle models. Science 349, 528–532. doi: 10.1126/science.aab1833
Anderson, M. C., Hain, C., Wardlow, B., Pimstein, A., Mecikalski, J. R., and Kustas, W. P. (2011). Evaluation of drought indices based on Thermal remote sensing of evapotranspiration over the continental United States. J. Clim. 24, 2025–2044. doi: 10.1175/2010JCLI3812.1
Assal, T. J., Anderson, P. J., and Sibold, J. (2016). Spatial and temporal trends of drought effects in a heterogeneous semi-arid forest ecosystem. For. Ecol. Manage. 365, 137–151. doi: 10.1016/j.foreco.2016.01.017
Bechtold, W. A., and Patterson, P. L. (2005). The enhanced forest inventory and analysis program — national sampling design and estimation procedures. USDA Gen. Tech. Rep. SRS-80. Asheville, NC: U.S. Department of Agriculture, Forest Service, Southern Research Station.
Bendixsen, D. P., Hallgren, S. W., and Frazier, A. E. (2015). Stress factors associated with forest decline in xeric oak forests of south-central United States. For. Ecol. Manage. 347, 40–48. doi: 10.1016/j.foreco.2015.03.015
Blackard, J. A., Finco, M. V., Helmer, E. H., Holden, G. R., Hoppus, M. L., Jacobs, D. M., et al. (2008). Mapping U.S. forest biomass using nationwide forest inventory data and moderate resolution information. Remote Sens. Environ. 112, 1658–1677. doi: 10.1016/J.RSE.2007.08.021
Breshears, D. D., Cobb, N. S., Rich, P. M., Price, K. P., Allen, C. D., Balice, R. G., et al. (2005). Regional vegetation die-off in response to global-change-type drought. Proc. Natl. Acad. Sci. U.S.A. 102, 15144–15148. doi: 10.1073/pnas.0505734102
Cook, B. I., Ault, T. R., and Smerdon, J. E. (2015). Unprecedented 21st century drought risk in the American Southwest and Central Plains. Sci. Adv. 1:e1400082. doi: 10.1126/sciadv.1400082
Davidson, C. B., Gottschalk, K. W., and Johnson, J. E. (1999). Tree mortality following defoliation by the European gypsy moth (Lymantria dispar L.) in the United States: A review. For. Sci. 45, 74–84.
Dietze, M. C., and Moorcroft, P. R. (2011). Tree mortality in the eastern and central United States: Patterns and drivers. Glob. Change Biol. 17, 3312–3326. doi: 10.1111/j.1365-2486.2011.02477.x
Dyderski, M. K., Paź, S., Frelich, L. E., and Jagodziński, A. M. (2018). How much does climate change threaten European forest tree species distributions? Glob. Change Biol. 24, 1150–1163. doi: 10.1111/gcb.13925
Eidenshink, J., Schwind, B., Brewer, K., Zhu, Z.-L., Quayle, B., and Howard, S. (2007). A project for monitoring trends in burn severity. Fire Ecol. 3, 3–21. doi: 10.4996/fireecology.0301003
Fitts, L. A., Domke, G. M., and Russell, M. B. (2022). Comparing methods that quantify forest disturbances in the United States’ national forest inventory. Environ. Monit. Assess. 194, 1–17.
Friedlingstein, P., Meinshausen, M., Arora, V. K., Jones, C. D., Anav, A., Liddicoat, S. K., et al. (2014). Uncertainties in CMIP5 climate projections due to carbon cycle feedbacks. J. Clim. 27, 511–526. doi: 10.1175/JCLI-D-12-00579.1
Ganey, J. L., and Vojta, S. C. (2011). Tree mortality in drought-stressed mixed-conifer and ponderosa pine forests, Arizona, USA. For. Ecol. Manage. 261, 162–168. doi: 10.1016/j.foreco.2010.09.048
Gazol, A., and Camarero, J. J. (2022). Compound climate events increase tree drought mortality across European forests. Sci. Total Environ. 816:151604. doi: 10.1016/j.scitotenv.2021.151604
Ghimire, B., Williams, C. A., Collatz, G. J., and Vanderhoof, M. (2012). Fire-induced carbon emissions and regrowth uptake in western U.S. forests: Documenting variation across forest types, fire severity, and climate regions. J. Geophys. Res. Biogeosci. 117:G03036. doi: 10.1029/2011JG001935
Hansen, M. C., Potapov, P. V., Moore, R., Hancher, M., Turubanova, S. A., Tyukavina, A., et al. (2013). High-resolution global maps of 21st-century forest cover change. Science 342, 850–853. doi: 10.1126/science.1244693
Hicke, J. A., Meddens, A. J. H., and Kolden, C. A. (2016). Recent tree mortality in the Western United States from bark beetles and forest fires. For. Sci. 62, 141–153. doi: 10.5849/forsci.15-086
Hicke, J. A., Xu, B., Meddens, A. J. H., and Egan, J. M. (2020). Characterizing recent bark beetle-caused tree mortality in the western United States from aerial surveys. For. Ecol. Manage. 475:118402. doi: 10.1016/j.foreco.2020.118402
Hoover, C. M., Bartig, J. L., Bogaczyk, B., Breeden, C., Iverson, L. R., Prout, L., et al. (2022). Forest inventory and analysis data in action: Examples from eastern national forests. Trees For. People 7:100178. doi: 10.1016/J.TFP.2021.100178
Huang, C., Asner, G. P., Barger, N. N., Neff, J. C., and Floyd, M. L. (2010). Regional aboveground live carbon losses due to drought-induced tree dieback in piñon-juniper ecosystems. Remote Sens. Environ. 114, 1471–1479. doi: 10.1016/j.rse.2010.02.003
Huang, J., Kautz, M., Trowbridge, A. M., Hammerbacher, A., Raffa, K. F., Adams, H. D., et al. (2020). Tree defence and bark beetles in a drying world: Carbon partitioning, functioning and modelling. New Phytol. 225, 26–36. doi: 10.1111/nph.16173
Kautz, M., Anthoni, P., Meddens, A. J. H., Pugh, T. A. M., and Arneth, A. (2018). Simulating the recent impacts of multiple biotic disturbances on forest carbon cycling across the United States. Glob. Change Biol. 24, 2079–2092. doi: 10.1111/gcb.13974
Kennedy, R. E., Cohen, W. B., and Schroeder, T. A. (2007). Trajectory-based change detection for automated characterization of forest disturbance dynamics. Remote Sens. Environ. 110, 370–386. doi: 10.1016/j.rse.2007.03.010
Klos, R. J., Wang, G. G., Bauerle, W. L., and Rieck, J. R. (2009). Drought impact on forest growth and mortality in the southeast USA: An analysis using forest health and monitoring data. Ecol. Appl. 19, 699–708. doi: 10.1890/08-0330.1
Knapp, E. E., Bernal, A. A., Kane, J. M., Fettig, C. J., and North, M. P. (2021). Variable thinning and prescribed fire influence tree mortality and growth during and after a severe drought. For. Ecol. Manage. 479:118595. doi: 10.1016/j.foreco.2020.118595
Kolb, T. E., Fettig, C. J., Ayres, M. P., Bentz, B. J., Hicke, J. A., Mathiasen, R., et al. (2016). Observed and anticipated impacts of drought on forest insects and diseases in the United States. For. Ecol. Manage. 380, 321–334. doi: 10.1016/j.foreco.2016.04.051
Kurz, W. A., Shaw, C. H., Boisvenue, C., Stinson, G., Metsaranta, J., Leckie, D., et al. (2013). Carbon in Canada’s boreal forest-a synthesis. Environ. Rev. 21, 260–292. doi: 10.1139/er-2013-0041
Maness, H., Kushner, P. J., and Fung, I. (2013). Summertime climate response to mountain pine beetle disturbance in British Columbia. Nat. Geosci. 6, 65–70. doi: 10.1038/ngeo1642
Massoud, E. C., Xu, C., Fisher, R. A., Knox, R. G., Walker, A. P., Serbin, S. P., et al. (2019). Identification of key parameters controlling demographically structured vegetation dynamics in a land surface model: CLM4.5(FATES). Geosci. Model Dev. 12, 4133–4164. doi: 10.5194/gmd-12-4133-2019
McDowell, N. G., Beerling, D. J., Breshears, D. D., Fisher, R. A., Raffa, K. F., and Stitt, M. (2011). The interdependence of mechanisms underlying climate-driven vegetation mortality. Trends Ecol. Evol. 26, 523–532. doi: 10.1016/j.tree.2011.06.003
McDowell, N. G., Coops, N. C., Beck, P. S. A., Chambers, J. Q., Gangodagamage, C., Hicke, J. A., et al. (2015). Global satellite monitoring of climate-induced vegetation disturbances. Trends Plant Sci. 20, 114–123. doi: 10.1016/j.tplants.2014.10.008
McDowell, N. G., Williams, A. P., Xu, C., Pockman, W. T., Dickman, L. T., Sevanto, S., et al. (2016). Multi-scale predictions of massive conifer mortality due to chronic temperature rise. Nat. Clim. Change 6, 295–300. doi: 10.1038/nclimate2873
Meddens, A. J. H., and Hicke, J. A. (2014). Spatial and temporal patterns of Landsat-based detection of tree mortality caused by a mountain pine beetle outbreak in Colorado, USA. For. Ecol. Manage. 322, 78–88. doi: 10.1016/j.foreco.2014.02.037
Meddens, A. J. H., Hicke, J. A., Macalady, A. K., Buotte, P. C., Cowles, T. R., and Allen, C. D. (2015). Patterns and causes of observed piñon pine mortality in the southwestern United States. New Phytol. 206, 91–97. doi: 10.1111/nph.13193
Millar, C. I., Westfall, R. D., Delany, D. L., Bokach, M. J., Flint, A. L., and Flint, L. E. (2012). Forest mortality in high-elevation whitebark pine (Pinus albicaulis) forests of eastern California, USA; influence of environmental context, bark beetles, climatic water deficit, and warming. Can. J. For. Res. 42, 749–765. doi: 10.1139/X2012-031
Mu, Q., Zhao, M., Kimball, J. S., McDowell, N. G., and Running, S. W. (2013). A remotely sensed global terrestrial drought severity index. Bull. Am. Meteorol. Soc. 94, 83–98. doi: 10.1175/BAMS-D-11-00213.1
Palahí, M., Valbuena, R., Senf, C., Acil, N., Pugh, T. A. M., Sadler, J., et al. (2021). Concerns about reported harvests in European forests. Nature 592, E15–E17. doi: 10.1038/s41586-021-03292-x
Peng, C., Ma, Z., Lei, X., Zhu, Q., Chen, H., Wang, W., et al. (2011). A drought-induced pervasive increase in tree mortality across Canada’s boreal forests. Nat. Clim. Change 1, 467–471. doi: 10.1038/nclimate1293
Robbins, Z. J., Xu, C., Aukema, B. H., Buotte, P. C., Chitra-Tarak, R., Fettig, C. J., et al. (2022). Warming increased bark beetle-induced tree mortality by 30% during an extreme drought in California. Glob. Change Biol. 28, 509–523. doi: 10.1111/gcb.15927
Senf, C., Buras, A., Zang, C. S., Rammig, A., and Seidl, R. (2020). Excess forest mortality is consistently linked to drought across Europe. Nat. Commun. 11:6200. doi: 10.1038/s41467-020-19924-1
Sitch, S., Friedlingstein, P., Gruber, N., Jones, S. D., Murray-Tortarolo, G., Ahlström, A., et al. (2015). Recent trends and drivers of regional sources and sinks of carbon dioxide. Biogeosciences 12, 653–679. doi: 10.5194/bg-12-653-2015
Smith, W. B. (2002). Forest inventory and analysis: A national inventory and monitoring program. Environ. Pollut. 116(Suppl. 1) S233–S242.
Stanke, H., Finley, A. O., Domke, G. M., Weed, A. S., and MacFarlane, D. W. (2021). Over half of western United States’ most abundant tree species in decline. Nat. Commun. 12:451. doi: 10.1038/s41467-020-20678-z
Tyukavina, A., Potapov, P., Hansen, M. C., Pickens, A. H., Stehman, S. V., Turubanova, S., et al. (2022). Global trends of forest loss due to fire from 2001 to 2019. Front. Remote Sens. 3:825190. doi: 10.3389/frsen.2022.825190
US Forest Service (2005). Forest inventory and analysis sampling and plot design. Available online at: https://www.fia.fs.fed.us/library/fact-sheets/data-collections/Sampling and Plot Design.pdf (accessed March 10, 2022).
US Forest Service (2016). Forest inventory and analysis database user guide (Version 6.1). Available online at: https://www.fia.fs.fed.us/library/fact-sheets/datacollections/Sampling and Plot Design.pdf (accessed March 14, 2022).
US Forest Service (2021). Forest inventory and analysis national core field guide volume I: Field data collection procedures for phase 2 plots (Version 9.1). Available online at: https://www.fia.fs.fed.us/library/field-guides-methods-proc/docs/2021/core_ver9-1_9_2021_final.pdf (accessed March 10, 2022).
Van Mantgem, P. J., Stephenson, N. L., Byrne, J. C., Daniels, L. D., Franklin, J. F., Fulé, P. Z., et al. (2009). Widespread increase of tree mortality rates in the Western United States. Science 323, 521–524. doi: 10.1126/science.1165000
Keywords: forest disturbances, tree mortality, drought, fire, anthropogenic disturbances, carbon loss/transfer
Citation: Wang M, Xu C, Johnson DJ, Allen CD, Anderson M, Wang G, Qie G, Solander KC and McDowell NG (2022) Multi-scale quantification of anthropogenic, fire, and drought-associated forest disturbances across the continental U.S., 2000–2014. Front. For. Glob. Change 5:693418. doi: 10.3389/ffgc.2022.693418
Received: 10 April 2021; Accepted: 05 October 2022;
Published: 03 November 2022.
Edited by:
Manuel R. Guariguata, Center for International Forestry Research (CIFOR), IndonesiaReviewed by:
Christopher Woodall, Research and Development, Forest Service (USDA), United StatesJ. Aaron Hogan, University of Florida, United States
Bognounou Fidèle, Canadian Forest Service, Canada
Aurélie C. Shapiro, Food and Agriculture Organization of the United Nations, Italy
Copyright © 2022 Wang, Xu, Johnson, Allen, Anderson, Wang, Qie, Solander and McDowell. This is an open-access article distributed under the terms of the Creative Commons Attribution License (CC BY). The use, distribution or reproduction in other forums is permitted, provided the original author(s) and the copyright owner(s) are credited and that the original publication in this journal is cited, in accordance with accepted academic practice. No use, distribution or reproduction is permitted which does not comply with these terms.
*Correspondence: Chonggang Xu, Y3h1QGxhbmwuZ292