- 1Laboratoire Evolution et Diversité Biologique (EDB, UMR 5174) CNRS, IRD, Université Toulouse III, Toulouse, France
- 2Laboratoire Interactions Sol Plante Atmosphère (UMR 1391 ISPA), Bâtiment C2, INRAE Centre de Bordeaux-Aquitaine, Villenave, France
- 3Institut Européen De La Forêt Cultivée, Cestas, France
- 4AMAP, Université de Montpellier, CIRAD, CNRS, INRAE, IRD, Montpellier, France
- 5UMR SILVA INRAE, AgroParisTech, Université de Lorraine, Nancy, France
- 6Department of Natural Resources and Environmental Studies, National Dong Hwa University, Hualien City, Taiwan
Extreme wind blowdown events can significantly modify the structure and composition of forests, and the predicted shift in tropical cyclone regimes due to climate change could strongly impact forests across the tropics. In this study, we coupled an individual-based and spatially-explicit forest dynamics model (TROLL) with a mechanistic model estimating wind damage as a function of tree size, traits, and allometry (ForestGALES). We assimilated floristic trait data and climate data from a subtropical forest site in Taiwan to explore the effect of wind regimes on forest properties. We found that the average canopy height and biomass stocks decreased as wind disturbance strength increased, but biomass stocks showed a nonlinear response. Above a wind intensity threshold, both canopy height and biomass drastically decreased to near-zero, exhibiting a transition to a non-forest state. Wind intensity strongly regulated wind impact, but varying wind frequency did not cause discernible effects. The implementation of within-stand topographic heterogeneity led to weak effects on within-stand forest structure heterogeneity at the study site. In conclusion, the intensity of wind disturbances can potentially greatly impact forest structure by modifying mortality. Individual-based modeling provides a framework in which to investigate the impact of wind regimes on mortality, other factors influencing wind-induced tree mortality, as well as interaction between wind and other forms of forest disturbance and human land use legacy.
Introduction
Natural disturbances shape forest structure, composition and dynamics, and play a substantial role in controlling the global carbon cycle (Reichstein et al., 2013; Pugh et al., 2019). Wind is a major source of natural disturbances and an important driver of tree mortality (Mitchell, 2013; Gardiner et al., 2016): while wind can cause partial tree damage such as branch snapping and defoliation, extreme wind events can lead to tree death, mainly through stem breakage and tree uprooting. In particular, extreme wind disturbances generated by tropical cyclones in regions such as the Caribbean (Lugo et al., 2000) and the northwestern Pacific (Lin et al., 2011) have been shown to control forest structure at a regional scale (Hogan et al., 2018; Ibanez et al., 2020), with one notable effect being the reduction of canopy height with increased cyclone frequency and intensity (Ibanez et al., 2019). Cyclone regimes are projected to change in the future (Lin et al., 2020), with a general increase in cyclone intensity and interregional differences in the trend of cyclone frequency (Knutson et al., 2020). In order to anticipate how such changes will affect community- and ecosystem-scale properties, it is essential to deepen our understanding of the mechanisms through which cyclonic winds regulate tree mortality, which remains one of the demographic processes that are less well understood in forest ecosystems (Bugmann et al., 2019; Koch et al., 2021).
However, it remains intrinsically difficult to reach general conclusions on factors driving wind-induced tree mortality based on empirical studies: these studies rely on observations of forest damage after individual cyclone events, which are both limited in their temporal extent, and driven by contingent factors such as cyclone trajectory and force (Lugo, 2008) and human land use legacy (Kulakowski et al., 2011; Schwartz et al., 2017). In various studies, a higher probability and level of damage has been found to be correlated to tree characteristics such as larger stem diameter (Ostertag et al., 2005; Halder et al., 2021), larger height (Dunham and Cameron, 2000; Vandecar et al., 2011), or lower wood density (Curran et al., 2008; Webb et al., 2014). However, the size dependence of damage can vary with tree species (Xi, 2015) and damage type (Everham and Brokaw, 1996), and some studies even did not observe any size effect on tree damage (Bellingham et al., 1996). In addition, wind impact on trees can vary at fine scales within a given forest and for a given species, as wind speed interacts in complex ways with terrain topography, often accelerating at hilltops and mountain ridges, and decelerating in leeward valleys (Ruel et al., 1998; Belcher et al., 2011). As a result, windward, exposed terrains can experience heavier wind damage than leeward regions and valleys (McEwan et al., 2011; Yap et al., 2016) (but see de Toledo et al., 2012).
Recently, a number of studies have contributed to a more mechanistic understanding of wind-tree interactions at a finer scale. To do so, they modelled the response of trees or tree-like structures when exposed to wind drag using the finite-element method (Moore and Maguire, 2008; Jackson et al., 2019b,c), and used continuously collected field data to relate tree motion to architectural properties (Jackson et al., 2019a,2021). These studies provide important insights into the mechanisms underlying wind-tree interaction. However, the response of trees to wind depends not only on individual characteristics, but also on the tree neighborhood, and more specifically on the presence and the relative size of neighboring trees, which influence wind sheltering: as an illustration, wind typically has a disproportionate impact on emergent, unsheltered trees (Hale et al., 2012; Seidl et al., 2014; Duperat et al., 2021). Hence, the impact of wind on a forest stand is more than the sum of the impacts on single trees: the heterogeneity of forest structure and composition must be accounted for when modeling the impacts of wind, especially for mixed sized forests.
In light of these challenges, dynamic forest models that are both spatially explicit and individual-based could be useful since they link external environmental conditions to community- and ecosystem-scale properties, through individual-level processes such as mortality, while explicitly accounting for spatiotemporal heterogeneity of forests (Seidl et al., 2014; Fischer et al., 2016). By including extreme wind disturbances in the climate forcing of these models, it is possible to represent mortality in a more mechanistic fashion, and generate stand-level predictions about forest response to wind disturbances, which could then be compared with forest inventory or remote sensing data. Several individual-based models (Ancelin et al., 2004; Schelhaas et al., 2007; Uriarte et al., 2009; Seidl et al., 2014; Kamimura et al., 2019) and land surface models (Chen et al., 2018) have incorporated windthrow or storm damage models. However, these models are heavily skewed toward temperate forests with relatively homogeneous stand structure. To the best of our knowledge, there is currently no forest dynamics model that simulates the effects of extreme wind disturbance at species-rich, structurally heterogeneous forests in the subtropics and the tropics, where tropical cyclones and downburst winds can be important disturbance factors.
In this study, we explored the community-wide consequences of wind disturbances, using a model-based approach. We coupled a wind disturbance module to an individual-based forest model, and created forcing conditions from tropical cyclone records and wind speed data. We parameterized this model for the Fushan Forest in Taiwan, located in a cyclone-prone region, and explored how extreme wind events impact tree mortality and forest structure and dynamics. Specifically, we addressed the following questions:
(1) How does wind-induced tree mortality impact forest structure and dynamics? We expect that the presence of wind-induced tree mortality would cause a reduction in average canopy height due to higher vulnerability of tall trees to wind damage. This could in turn increase light availability in the understory and facilitate tree establishment, leading to an increase in stem density. We also expect that total mortality as well as the fraction of mortality due to treefall would increase.
(2) How do changes in frequency and intensity of extreme wind events lead to shifts in forest dynamics and structure? We expect that higher wind frequency and intensity would result in more wind-induced mortality for large trees, which in turn would increase the extent of canopy height reduction and stem density increase.
(3) How does topographical heterogeneity within a forest stand influence wind damage patterns? We expect that the inclusion of topography would lead to a higher heterogeneity in forest structure, with lower canopy height and higher stem density in more exposed locations.
Materials and Methods
Description of the TROLL Model
The TROLL model is a spatially explicit individual-based model that divides the aboveground space of a forest stand into 3D cells of size 1 m3 (voxels). Solar irradiance inside each voxel is computed as the irradiance fraction transmitted from immediately above the focal voxel (Chave, 1999; Maréchaux and Chave, 2017). No more than one individual tree can establish in each 1 × 1 m pixel at any given time, and only self-standing stems ≥ 1 cm in DBH (diameter at breast height, measured at 1.3 m above ground, or 20 cm above deformities or buttresses if present) are explicitly modeled (herbaceous plants and lianas are not included). Seeds and seedlings < 1 cm in DBH are not directly modeled, but represented as part of a seed/seedling pool. Each modeled tree is characterized by its DBH, height, crown size (radius and depth), total leaf surface area, and age. Trees are assigned species-specific traits, which control photosynthesis, growth as well as other physiological and demographic processes. At each monthly time step, trees grow depending on the balance between carbon acquisition based on a C3 photosynthesis model (Farquhar et al., 1980) and respiration, with assimilated carbon allocated into wood or leaf production. Height-DBH allometric relations are prescribed at the species level, while the DBH-crown size allometry is assumed to be species-independent. Tree mortality in TROLL is represented by several processes: (i) stochastic tree death, here assumed to be negatively dependent on species-specific wood density (WD) (Wright et al., 2010): meff=m−α×WD, (α is positive, so m is the maximal possible value of the mortality rate), (ii) carbon starvation, when net assimilated carbon is negative over a consecutive period exceeding leaf lifespan, so that old leaves have all died while no new leaves could be produced, (iii) stochastic treefall, dependent on a species-specific tree height threshold related to maximum realized tree height, and (iv) secondary treefall, when a tree is affected by a falling neighboring tree. To more explicitly link tree mortality to exogenous factors such as wind disturbance, we updated the treefall module for this study to simulate treefall more mechanistically (cf. below).
Inputs for a typical run of TROLL (v3.0) include (1) monthly mean climate forcing data for the simulated location, (2) average trajectory of daily climatic variation, (3) species-specific plant traits for the simulated forest, and (4) species-independent general parameters, including those related to the stochastic tree death and treefall. The source code of TROLL (v3.0) is written in C++ and is available on https://github.com/troll-code/troll. On a computing cluster, each simulation of 200 × 200 m and 500 years uses around 15 min of computer processing unit (CPU) time.
Modeling Tree-Top Wind Speeds in a Mixed-Sized Forest
Horizontal wind speed decreases as one approaches the canopy top from above the canopy. This is modeled by the aerodynamic momentum transfer model above a vegetation canopy (Monteith and Unsworth, 2008, p310). The horizontal wind speed profile with height is represented with the following equation:
where u(z) (m s–1) is the horizontal wind speed at height z (m) above ground or a displacement surface, k = 0.41 is the von Kármán constant, and u* (m s–1) is the friction velocity. Parameter d is called the zero-plane displacement height, and z0the aerodynamic roughness. Both d and z0 are dependent on canopy height H (m), and much research has explored the variation in both parameters d and z0 over forest vegetation (Shaw and Pereira, 1982; Dorman and Sellers, 1989; Shuttleworth et al., 1989; Raupach et al., 1991). In general, d ranges from 0.7 H to 0.9 H, and z0 from 0.04 H to 0.08 H for tropical forests. For this study, we chose the intermediate values of d = 0.8 H and z0 = 0.06 H. These values are consistent with dense and tall evergreen forest vegetation as simulated in the SiB2 model (Sellers et al., 1996).
The aerodynamic momentum transfer model (1a) is only applicable above the canopy. In this study, we decided to apply an additional model to represent wind within the canopy (Inoue, 1963):
where α=H/Ls≈3; empirical values of Ls are reported in Table 1 of Raupach et al. (1996). With this parameterization, horizontal wind speed u(z) at a height of d+z0=0.86H and within the canopy at H/2 is 66% and 22% of u(H), respectively. To reduce computational burden, we assumed that trees whose height is lower than H/2 were not directly affected by wind. To account for canopy height heterogeneity and neighbor tree sheltering, we calculated canopy height H for every 20 × 20 m quadrat by taking the arithmetic mean of the top leaf-containing voxel layer of each pixel within the quadrat.
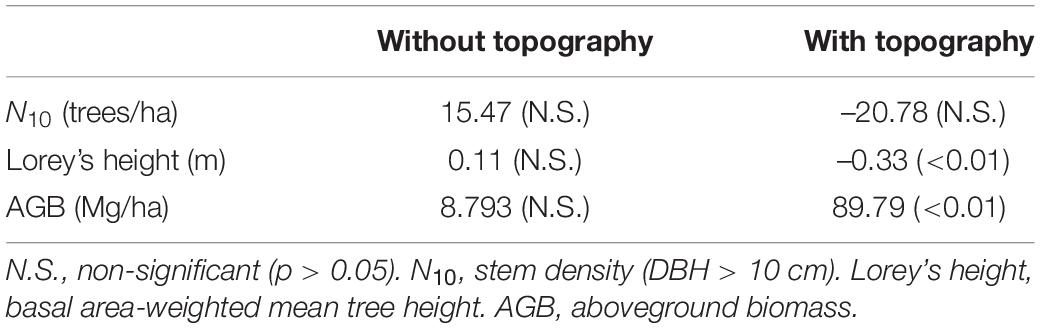
Table 1. Slope estimates of linear models of each simulated quadrat-level forest structure metrics to the wind speed correction factor, with p-values in parentheses.
From the CRU-NCEP reanalysis dataset, we obtained empirical atmospheric wind speed values (cf. the “Climate forcing” section below), which are conventionally measured at 10 m above ground or above a displacement surface. In order to obtain the horizontal wind speed experienced by each tree, we applied the following equation, derived from eq. (1a), to convert horizontal atmospheric wind speed at d + 10, u(d + 10), into top-canopy wind speed u(H) within each 20 × 20 m quadrat:
Finally, for each tree with height h > H/2, we computed u(h) using either Eqs. (1a) or (1b).
Modeling Wind-Induced Tree Death: Critical Wind Speed
To simulate the impact of extreme wind disturbance, we assumed that wind-induced treefall is a stochastic process whose probability depends on the difference between the tree-top wind speed u(h) exerted on a tree of height h (m), and a tree-specific critical wind speed (CWS). The higher u(h) is relative to CWS, the more likely the tree is to fall and die. In this study, we used a logistic model to relate the wind-induced tree death probability to (u(h) – CWS) (Valinger and Fridman, 1999; Hale et al., 2015):
For the estimation of CWS, we followed the approach of ForestGALES, a wind damage risk model originally developed for even-sized and even-spaced plantation forests (Gardiner et al., 2000, 2008) but then adapted for use with individual trees in more complex forest structures (Hale et al., 2012, 2015; Duperat et al., 2021; Quine et al., 2021). ForestGALES models the biomechanical conditions under which the tree, simplified as an anchored vertical object, will break (causing stem breakage) or lose root anchorage (causing uprooting) when subject to a turning moment caused by wind loading.
Importantly, the formulation of Eq. (3) departs from that of ForestGALES, where the control of wind speed on mortality is defined to be u(H), the top-canopy wind speed. To account for the reduced impact of wind within forest canopies, the CWS in ForestGALES can be modulated by a competition index, so that trees within the canopy are less exposed to wind damage than top-canopy trees (Hale et al., 2012; Duperat et al., 2021; Quine et al., 2021). In the present formulation, CWS does not depend on the tree neighborhood, but the probability of wind-induced tree death depends on wind speed at tree height, as computed from Eqs. (1a) and (1b).
At our study site, field observations indicate that the proportion of tree uprooting is low compared to that of stem breakage (Supplementary Appendix A). We therefore focused on wind-induced stem breakage. The critical wind speed (CWS, m s–1) inducing stem breakage and treefall is calculated by the following equation (Hale et al., 2015):
where DBH (m) is the tree diameter at breast height, and MOR (Pa, kg m–1 s–2) is the fresh-wood modulus of rupture. We estimated MOR from oven-dry wood density (WD, g cm–3) through the following exponential relationship (Green et al., 1999, see Supplementary Appendix B):
TC is the turning moment coefficient (kg), which relates the square of mean wind speed (m2 s–2) to the maximum turning moment (kg m2 s–2) experienced by an individual tree. We used the following empirical equation for TC (Hale et al., 2012):
where the constant τ (kg m–3) = 111.7 (Hale et al., 2015). A higher TC value means larger turning moment for a given wind speed.
The wind damage parameter P (unitless) is stand-specific and determines the overall susceptibility of a forest stand to wind-induced tree death: the smaller P value is, the lower the critical wind speed is for any given tree under the same condition, meaning that the forest is overall more susceptible to wind-induced tree death. It encapsulates multiple constants and corrective factors, as well as factors in the original equation (see Hale et al., 2015, Eqs. 11 and 12; also see Supplementary Appendix C) that are difficult to estimate, and whose interpretation is beyond the scope of this study. The value of P was thus tuned here by means of a sensitivity analysis (see below).
Climatic Forcing
The TROLL model requires the following monthly mean climatic variables: daytime and nighttime mean temperature, daytime mean irradiance, and daytime mean vapor pressure deficit (VPD). We used the CRU-NCEP dataset to provide the monthly climate forcing, a global gridded (0.5° × 0.5°) sub-daily (6-hourly) climate product spanning the 1901--2016 period (version 8; version 7 archived1) (Viovy, 2018). It was constructed by combining observation-based CRU TS 3.2 data (Harris et al., 2014) and model-based NCEP-NCAR data (Kalnay et al., 1996). We used CRU-NCEP variables for the 1980–2016 period, for which the most observations are available (Kistler et al., 2001).
We estimated the monthly average frequency of cyclones occurring within a sufficiently close distance to the study site. For this, we used the IBTrACS dataset (International Best Track Archive for Climate Stewardship database; v04r00, archived2), which contains records of global tropical cyclones occurring since 1945 (Knapp et al., 2010). A common measure of the spatial extent of tropical cyclones is the mean radius of gale-force winds, by convention defined as 17.5 m s–1. Based on the range of reported values in the literature, we assumed the mean radius of gale-force winds (R17, km) to be 150 km (Chan and Chan, 2012; Weber et al., 2014; Lu et al., 2017). Thus, for a given site, we calculated the monthly mean frequency of recorded tropical cyclones occurring within a 150-km distance from the site over the period of 1987–2020. At Fushan, cyclone occurrence frequency was highest from July to September (∼ 0.5 cyclones per month); on average, total annual cyclone frequency was around 1.84 cyclones per year.
For the selected tropical cyclone records, we calculated on-site cyclonic wind speed (Vsite, m s–1) using the empirical function that relates the radial variation of the tangential wind speed beyond the radius of maximum sustained wind in mature tropical cyclones (Anthes, 1982; Hsu and Babin, 2005):, where d (km) is the distance between the site and the cyclone center. Since d < R17 by definition, it follows that Vsite > 17.5 m s–1. We then fitted the on-site cyclonic wind speed values of each month to a Weibull distribution, using the function fitdistr in the R package MASS (Venables and Ripley, 2002), and used the scale and shape parameters as input climate forcing variables. At Fushan, the year-round Vsite distribution is right-skewed, with 1st quartile = 18.85 m s–1, median = 20.60 m s–1, and 3rd quartile = 25.18 m s–1.
The coupling of TROLL to wind disturbances was performed as follows. At each time step, the occurrence of an extreme wind event was drawn from a cyclone occurrence probability, assuming that one extreme wind event at most can occur per time step. If an extreme wind event occurred, we drew a random wind speed value from the input wind speed distribution. We accounted for canopy heterogeneity and neighbor tree sheltering by only considering trees with height > H/2 as being exposed to wind disturbance, where H represents quadrat-wide average top canopy height, calculated by the mean value of the top leaf-containing voxel height of each pixel within the quadrat. For each exposed tree, we converted atmospheric wind speed, u(d + 10), to its tree-top wind speed, u(h) (Eqs. 1a, 1b, 2), and calculated its critical wind speed (Eq. 4). We then compared the two wind speed values, and determined if wind-induced tree death happens through a stochastic process, dependent on a logistic function of the difference between the two wind speeds (Eq. 3). Secondary treefall was modeled by assuming that when each tree dies, it falls in a random direction and increases the death rate in the impacted pixels.
Study Site and Parameterization
We parameterized the TROLL model with wind-induced tree mortality for a forest site in Taiwan. The Fushan Forest Dynamics Plot (FDP) is a 25-hectare (500 m × 500 m) plot in a moist broadleaf forest in the northeastern region of Taiwan (Su et al., 2007), part of ForestGEO (Forest Global Earth Observatory) (Condit, 1998; Anderson-Teixeira et al., 2015). Fushan is under strong influence of the northeasterly monsoon in winter and frequent typhoon visits in summer and autumn, with mean annual precipitation around 4200 mm, mean annual temperature around 18°C, and a mean relative humidity around 95%. Plot elevation ranges from 600 m to 733 m (Su et al., 2007). It was established in 2004 and censused every 5 years since then: all self-standing stems with a DBH ≥ 1 cm were identified, measured, tagged and mapped, with a total of 110 recorded tree species in the plot (Su et al., 2007).
Species-specific plant functional traits that TROLL requires as input parameters include leaf mass per area (LMA, g m–2), nitrogen and phosphorus content (Nmass and Pmass, mg g–1), wood density (WD, g cm–3), maximum DBH (cm), DBH-height allometric parameters and regional relative abundance. These traits were measured at Fushan according to ForestGEO protocol (Iida et al., 2014) for 94 species, covering ca. 90% of the censused trees. Climatic variables were extracted from the CRU-NCEP dataset at the geographic coordinates closest to Fushan FDP (24° 45′ N, 121° 32′ E).
Stem density and aboveground biomass (AGB) estimations were available at Fushan from census data. In order to estimate on-site tree mortality, we used data from the annual tree mortality survey, which has been conducted at Fushan following ForestGEO protocol since 2017. The mortality survey records the number of tree deaths and cause of death (standing, uprooted, or broken) of a subset of censused trees (Arellano et al., 2021). Using the mortality data at Fushan spanning 2017 to 2020, we calculated the mean annual mortality rate for all trees DBH > 10 cm, as well as the proportion of mortality attributed to treefall. For the detailed protocol of attribution of mortality factors, see Supplementary Appendix A.
Unless otherwise specified, for all simulations, we simulated forest regeneration from bare soil for a reference plot area of 4 hectares (200 m × 200 m) for a duration of 500 years with a monthly timestep. Since we aimed to examine the effects of extreme wind on mature forest, the wind-induced tree mortality sub-model was activated after a 100-year spin up phase.
Sensitivity Analysis
The wind-induced tree mortality sub-model includes a single parameter P (unitless). To investigate model responses to its value, we conducted a sensitivity analysis by varying the value of P across a range of [0.005, 1], with a varying step of 0.005: for each value, we performed five replicates of TROLL simulations (a total of 1000 simulations).
We calculated the steady-state values (mean over the last 100 years of the simulation) of three structure metrics: stem density (DBH > 10 cm; N10, tree ha–1), Lorey’s height (basal area-weighted mean tree height, m) (Pourrahmati et al., 2018), and aboveground biomass (AGB, Mg ha–1). For trees with DBH > 10 cm, we also calculated two mortality statistics: mean annual mortality and fraction of mortality due to treefall (%Mtreefall). We reported mortality statistics at the onset of wind disturbance (year 101–200, i.e., first 100 years after wind submodule activation) and after reaching the steady state (year 401–500, i.e., last 100 years of simulation). We qualitatively described trends and sensitivity of these statistics in response to variation of parameter value.
Effects of Wind Frequency and Intensity
To examine how the frequency and intensity of extreme wind events influence forests, we performed two series of simulations: (1) we varied cyclone occurrence frequency from 0.1 to 2 times the empirical frequency (obtained from cyclone best-track data), with a varying step of 0.1, while maintaining wind intensity; (2) we varied the scale parameter of the wind speed distribution (parameter controlling mean and median of the Weibull distribution), from 0.1 to 10 times the empirical values (estimated from the cyclone best-track wind speed distribution), with a varying step of 0.1, while maintaining empirical frequency. Five replicates were performed for each condition (in total, 100 simulations for frequency and 500 simulations for intensity were performed). We set P = 0.7 based on the results of the sensitivity analysis, where simulation results are close to field observations and not near the forest tipping point (P < 0.3). As in previous steps, we calculated the steady-state values (mean over the last 100 years of the simulation) of stem density (DBH > 10 cm) (tree ha–1), Lorey’s height (m), aboveground biomass (AGB, Mg ha–1), as well as mean annual mortality and fraction of mortality due to treefall (%Mtreefall) for trees with DBH > 10 cm. We qualitatively described trends and sensitivity of these statistics in response to variation of wind frequency and intensity.
Effects of Topography on Wind Disturbances
In the original TROLL model, the effect of topography was not taken into account. Based on the knowledge that wind speed is altered over an uneven topography, we implemented quadrat-scale wind speed correction factors in the model that account for these topographical effects. For this, we used the Global Wind Atlas (GWA) data, which are produced through downscaling using the WAsP program (Mortensen et al., 2000), incorporating surface elevation and aerodynamic roughness lengths (Badger et al., 2015). We obtained 250 m × 250 m GWA pixels that fall in the area covered by the 1° × 1° CRU-NCEP pixel where the Fushan site is located: this represents a grid of 200 × 200 GWA pixels. We normalized the GWA wind speed values of the selected pixels so that the mean GWA wind speed is equal to the mean CRU-NCEP wind speed. We then resampled the GWA pixels to the 20m × 20m quadrat scale using bilinear interpolation with the resample function in the raster package (Hijmans, 2022), and selected the resampled pixels falling within the area of the Fushan plot: this represents a grid of 25 × 25 = 625 resampled pixels. We used the GWA wind speed values of these resampled pixels, normalized by the plot-wide mean, as the wind speed correction factor for each quadrat.
The wind speed correction factor ranged from 0.27 to 1.96, and was used as a proxy for topographic heterogeneity: if the topographic effect is implemented, the wind speed experienced at each quadrat is the plot-wide wind speed (randomly drawn from the input wind speed distribution) multiplied by this correction factor: a value above 1 means that the wind speed at that quadrat is considered to speed up (due to exposed terrain), and considered to slow down when the value is below 1.
We performed simulations with and without topographical effect at the Fushan site for a plot area of 25 hectares (500 m × 500 m), and examined the relationship between quadrat-level steady-state values (mean over the last 100 years of the simulation) of stem density (DBH > 10 cm) (tree ha–1), Lorey’s height (m), aboveground biomass (AGB, Mg ha–1) and the topographic effect by performing linear regressions for each statistics as a function of quadrat-level wind speed correction factor.
In order to compare simulated results with field observation, we also calculated field-measured quadrat-level stem density (DBH > 10 cm), Lorey’s height (m) and aboveground biomass. We plotted them against quadrat-level mean elevation (m), which ranged from 616 to 730 m, and performed linear regressions for each statistic as a function of the quadrat-level mean elevation.
Data Analysis
Data processing, statistical analysis and visualization were performed in R 3.3.0 (R Core Team, 2021). Apart from those already mentioned elsewhere, R packages ggplot2, cowplot, ncdf4, BIOMASS, geosphere, sp, and tidyr were used for this study (Pebesma and Bivand, 2005; Wickham, 2016; Réjou-Méchain et al., 2017; Wilke, 2020; Hijmans, 2021; Pierce, 2021; Wickham and Girlich, 2022).
Results
Sensitivity Analysis
As the P parameter value decreased (stronger wind-induced tree mortality), both average canopy height (Lorey’s height) and aboveground biomass (AGB) decreased, although AGB showed a hump-shaped pattern (Figure 1): at low P-values (P < 0.3), canopy height and biomass decreased to extremely low levels, suggesting a transition from forest to non-forest state. Stem density (N10) only slightly decreased, but showed an abrupt increase around P = 0.15, where average canopy height and AGB reached extremely low levels, before decreasing again at lower P-values.
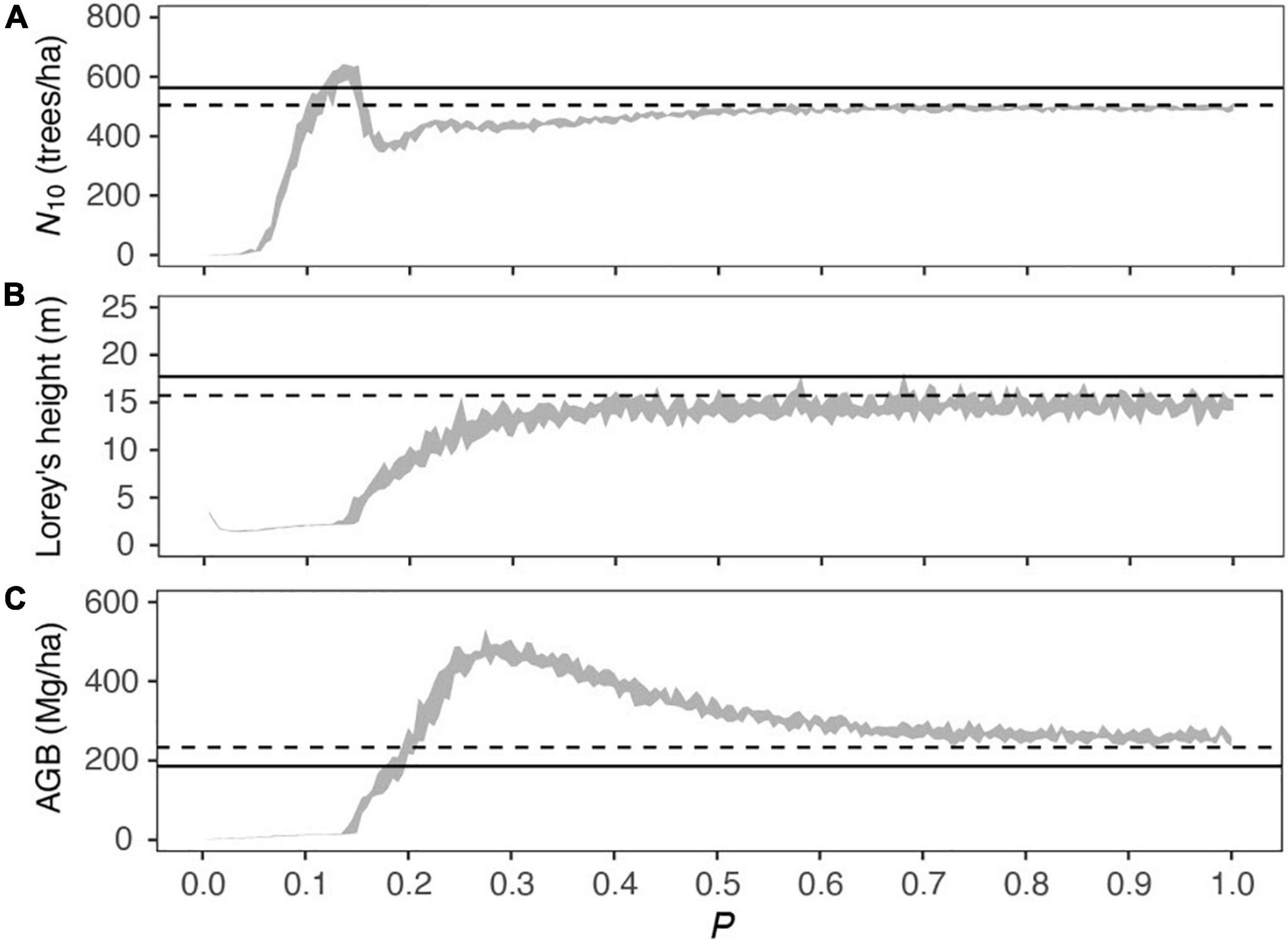
Figure 1. Summary statistics of the simulated forests, in relation to the critical wind speed parameter P (smaller P means stronger effect): (A) N10, density of stems with DBH > 10 cm (trees ha–1); (B) Lorey’s height, basal area-weighted mean tree height (m); (C) AGB, aboveground biomass (Mg ha–1). Shaded areas represent the value range of five replicates for each simulation condition. Dashed lines represent simulation value with no wind disturbance, and Solid lines represent field observations.
Mean annual mortality and fraction of mortality due to treefalls (%Mtreefall) increased as P decreased, although %Mtreefall exhibited an erratic nonlinear response. Both statistics were markedly lower at the end of the simulation (after the steady state has been reached) than immediately after the onset of wind forcing (Figure 2). The fraction of mortality due to treefalls (%Mtreefall) was overall around 75%, substantially higher than field observations (Figure 2, horizontal black line; Supplementary Appendix A).
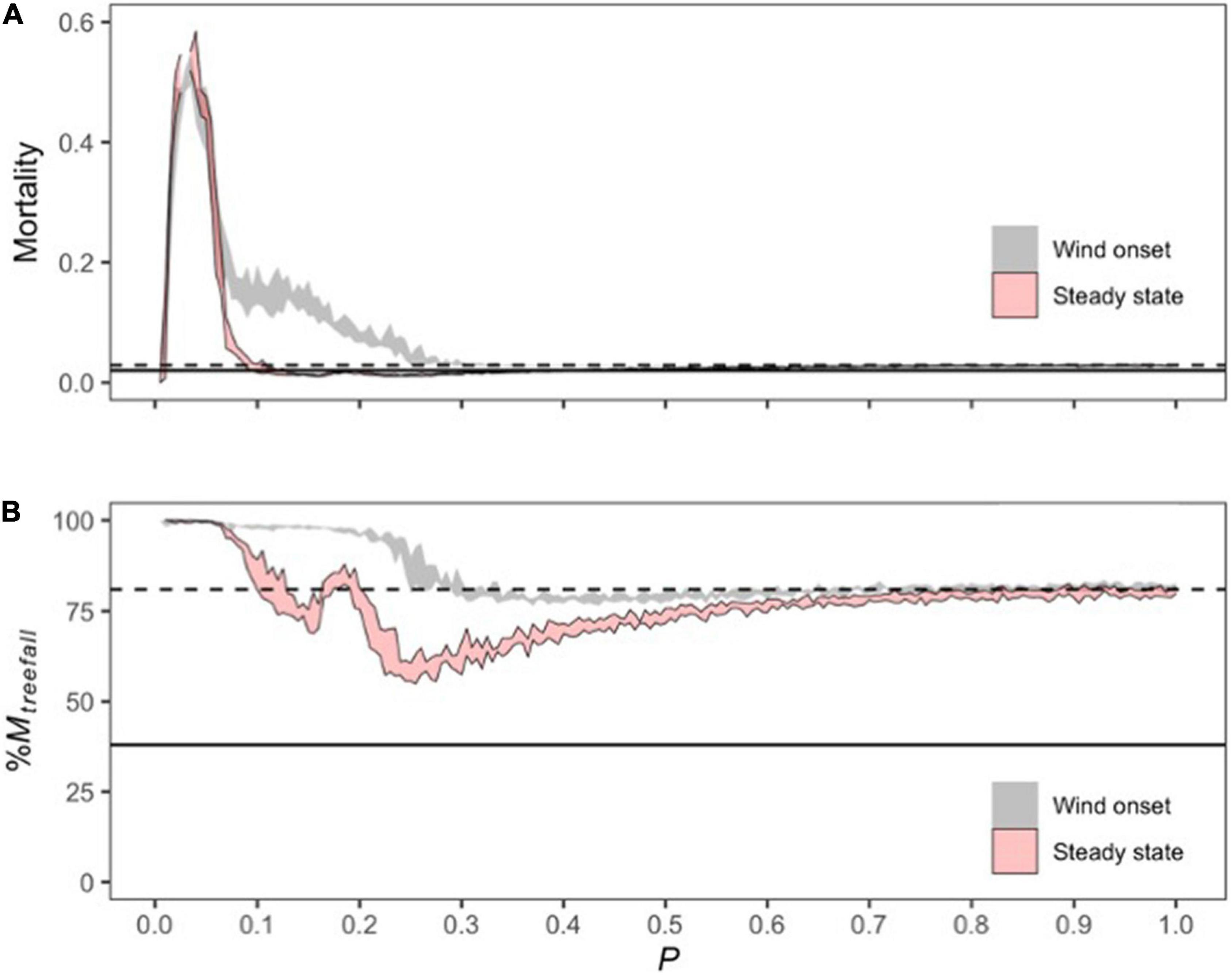
Figure 2. (A) Mean annual mortality rate and (B) fraction of mortality attributed to treefalls (%Mtreefall) for trees with DBH > 10 cm, in relation to the critical wind speed parameter P (smaller P means stronger effect). Shaded areas represent the value range of five replicates for each simulation condition. Black without border: mean values of the first 100 years after wind onset (year 101–200). Red with border: mean values of the last 100 years of simulation (year 401–500). Dashed lines represent simulation value with no wind disturbance, and solid lines represent field observations.
Effects of Wind Frequency and Intensity
None of the forest structure and mortality statistics showed significant change with varying wind frequency. On the other hand, as wind intensity increased, stem density (N10), average canopy height (Lorey’s height) and aboveground biomass (AGB) all decreased (with a hump-shaped response for aboveground biomass), and mean mortality and the fraction of mortality due to treefalls (%Mtreefall) increased, especially at higher wind intensity, where the near-zero level canopy height and biomass suggested a transition from forest to non-forest state as observed during the sensitivity test (Figure 3).
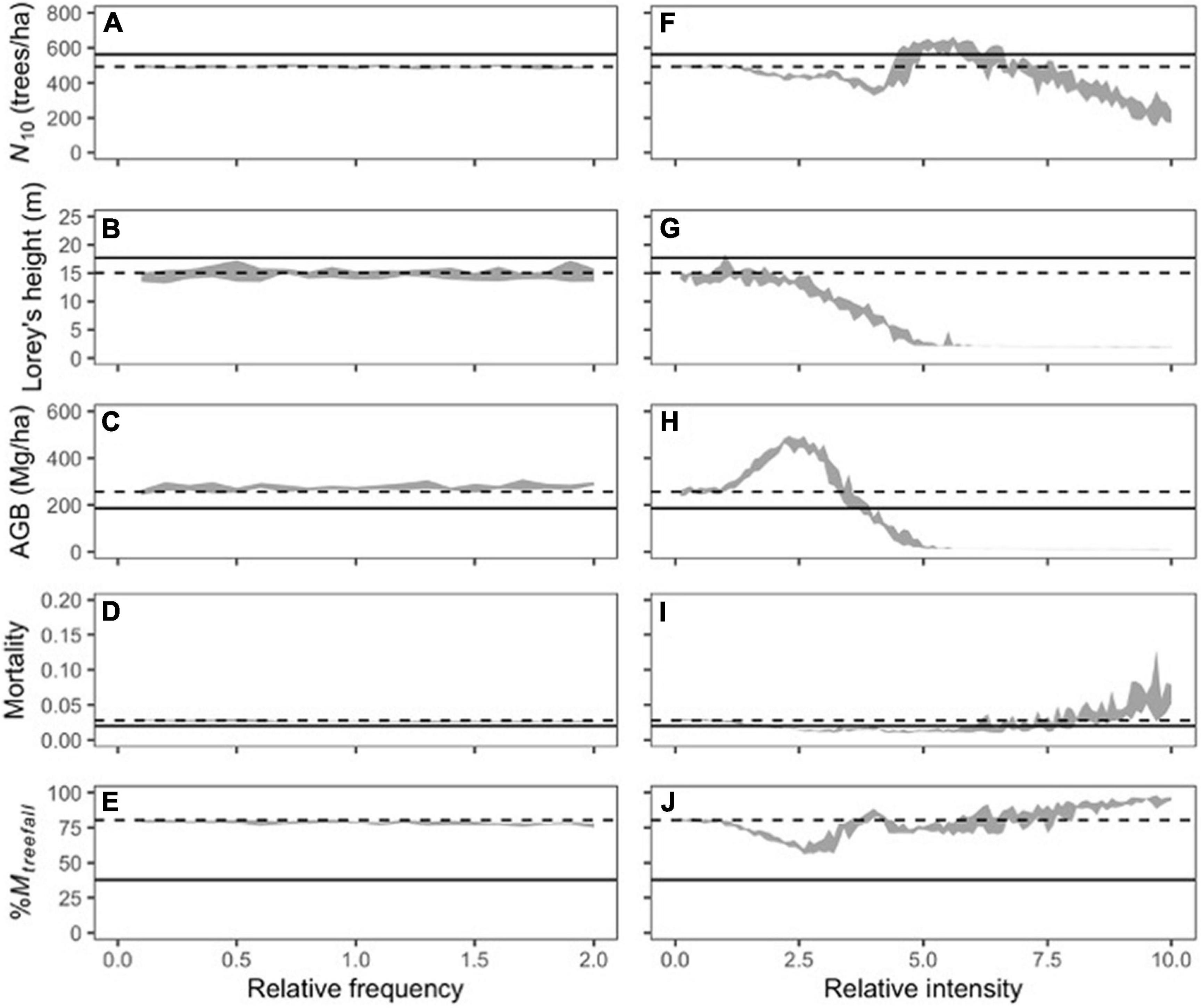
Figure 3. Simulated forest structure in relation to extreme wind frequency (A–E) and intensity (F–J), relative to empirical values from cyclone best-track data. For simulations with varying frequency, empirical wind intensity were used, and vice versa. Shaded areas represent the value ranges of five replicates for each simulation condition. (A,F) N10, density of stems with DBH > 10 cm (trees ha–1). (B,G) Lorey’s height, basal area-weighted mean tree height (m). (C,H) AGB, aboveground biomass (Mg ha–1). (D,I) mean annual mortality rate. (E,J) %Mtreefall, fraction of mortality due to treefalls. Dashed lines represent simulation value with no wind disturbance, and solid lines represent field observations.
Effects of Topography on Wind Disturbances
Implementing the topographic effect, we found weak but significant relationships between topographic wind speed correction and forest structure. Results of linear regressions showed that stem density (DBH > 10 cm, N10) was not significantly related to wind speed correction (p = 0.09), but average canopy height (Lorey’s height) decreased and aboveground biomass (AGB) increased significantly at quadrats with higher wind speed correction factors (representing more exposed terrain) (Figure 4 and Table 1). In contrast, the field-observed relationship between quadrat-scale forest structure statistics and elevation at Fushan was stronger and exhibited a different pattern: higher stem density and lower average canopy height were observed at more exposed quadrats, but biomass was not found to vary as a function of quadrat elevation (Figure 5 and Table 2).
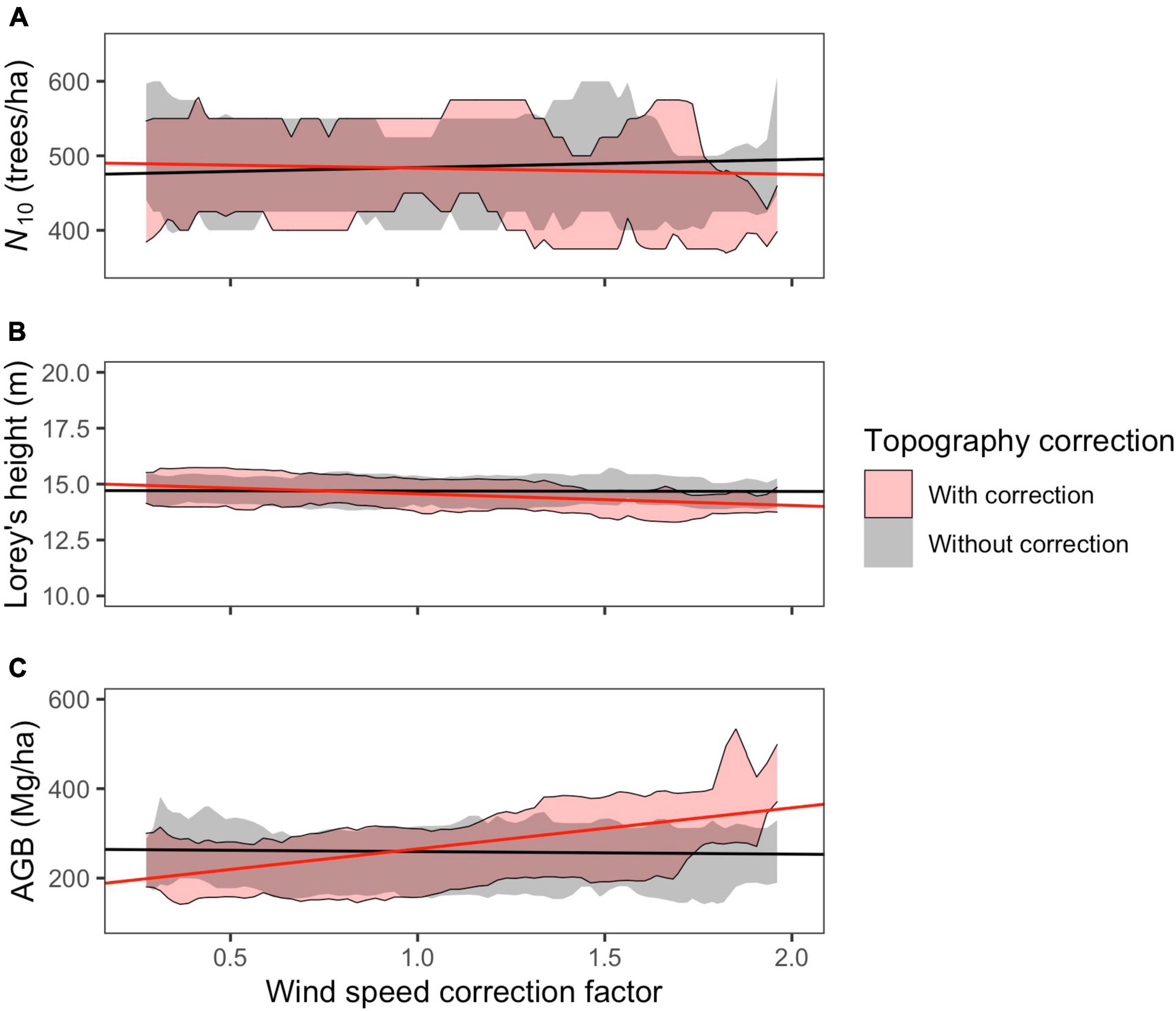
Figure 4. Simulated quadrat-level forest structure statistics as a function of the topography-related wind speed correction factor of each quadrat. A correction factor > 1 means that the quadrat-level wind speed is accelerated,, and a correction factor < 1 means that the quadrat-level wind speed is decelerated. Shaded areas represent interquartile ranges (IQR), calculated within a moving window frame (0.025) across the whole x-axis value range and then linearly interpolated to the x-axis value at each quadrat. Black without border: without topography correction. Red with border: with topography correction. Solid lines represent the linear regression curve. (A) N10, density of stems with DBH > 10 cm (trees ha–1). (B) Lorey’s height, basal area-weighted mean tree height (m). (C) AGB, aboveground biomass (Mg ha–1). For all structure statistics, the quadrat-wide values are converted to the corresponding hectare-wide values.
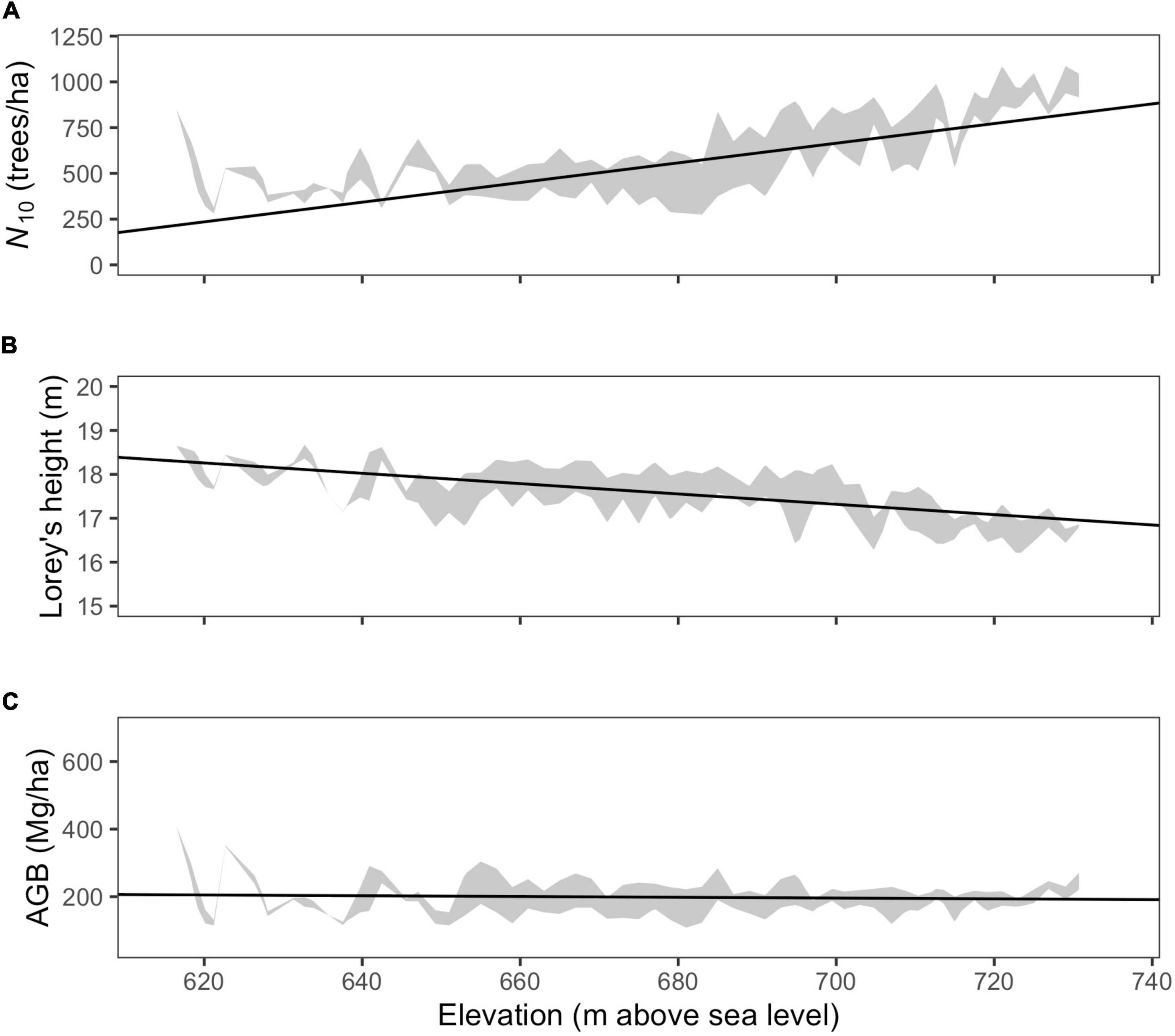
Figure 5. Quadrat-wide (20 m × 20 m) forest summary statistics at the Fushan site, as a function of the quadrat elevation above sea level. Shaded areas represent interquartile ranges (IQR), calculated within a moving window frame (2 m) across the whole x-axis value range and then linearly interpolated to the x-axis value at each quadrat. Solid lines represent the linear regression curve. (A) N10, density of stems with DBH > 10 cm (trees ha–1). (B) Lorey’s height, basal area-weighted mean tree height (m). (C) AGB, aboveground biomass (Mg ha–1). The quadrat-wide values were converted to corresponding hectare-wide values.
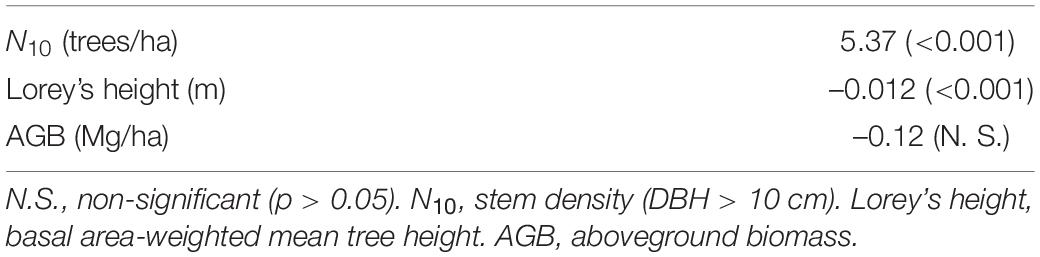
Table 2. Slope estimates of linear models of each quadrat-level forest structure metrics to the wind speed correction factor, with p-Values in parentheses.
Discussion
In this study, we included a model of wind-induced tree mortality in an individual-based forest model, and simulated how wind-induced tree mortality affects the structure and dynamics of a subtropical forest. We found that wind-induced tree mortality had a large negative impact on canopy height and a more complex influence on stem density and biomass. The comparison of tree mortality at the onset of wind disturbance and at the steady state reveals forest adaptation to repeated wind disturbances. Wind intensity was found to exert a strong control on wind impact, while wind frequency did not. Implementation of topographic heterogeneity showed a weak but significant effect on within-stand canopy height and biomass at the Fushan site: this implementation could serve as a basis to incorporate more complex wind-terrain interactions in individual-based models.
Effects of Wind-Induced Tree Death
In response to increasing susceptibility to wind-induced tree death, canopy height was found to decrease sharply. According to the wind damage model, TC is proportional to DBH2 × H (Eq. 6), and CWSstembreak is proportional to (DBH3 / TC)1/2 (Eq. 4): it thus follows that CWSstembreak is proportional to (DBH / H)1/2. Hence, wind damage risk can be expected to be higher for trees with a smaller diameter-to-height ratio (Cremer et al., 1982), and the observed reduction of canopy height may be caused by the fact that taller trees are in general more exposed and less protected by the sheltering of neighboring trees, meaning that they experience higher tree-top wind speed and higher wind damage risk (Hale et al., 2012; Duperat et al., 2021). A corollary of this hypothesis is that tree height in the canopy may become more homogenous as the effect of wind-induced tree mortality strengthens (Van Bloem et al., 2007; Chi et al., 2015). The hump-shaped response of biomass, although unexpected, might be the result of the joint effect of the selection of trees with denser wood and the reduction of larger trees, which contribute the most to total forest biomass.
A transition from forest to non-forest state around P = 0.3 was apparent, where stem density increased abruptly as canopy height and biomass decreased to near-zero. This increase in stem density likely reflects a light condition that favors more smaller trees to establish as the forest canopy opens up. As the effect of wind disturbances grew even stronger, even these small-height trees started to be affected due to the absence of sheltering from taller trees, causing stem density to decrease as well.
Steady-state total mortality increased with disturbance intensity, but only starting from around the transition point. Steady-state fraction of mortality due to treefall decreased as disturbance intensity increased until after the transition point, where it increased drastically. In addition, both mortality statistics were markedly lower at the end of the simulation than at the onset of wind disturbance. These observations suggest that long-term exposure to wind disturbances results in modification to the forest structure through the higher survival of more resistant species, such as those with larger diameter-to-height ratio or higher wood density. This forest structural change could account for the low immediate mortality after a cyclone passage observed in some empirical studies (Walker, 1991; Bellingham et al., 1992).
In addition to changes in structure and species composition, plastic acclimation could also regulate forest response to wind disturbances, as shown by a modeling study (Kamimura et al., 2019). In this study, plastic acclimation was not taken into account as the model did not allow traits to vary plastically over the lifetime of an individual, and trait variations were not inheritable. In the future, it would be highly interesting to explore the eco-evolutionary dynamics that results from temporally plastic trait variability and the inheritance of more wind-resistant traits (Telewski and Moore, 2016).
Effects of Wind Frequency and Intensity
Increasing wind intensity negatively affects forest stature and biomass stocks, consistent with results of global-scale studies that showed a clear empirical relationship between higher cyclone intensity and lower overall forest stature (Ibanez et al., 2019); however, varying wind frequency did not cause any effect on forest structure. Forest response to disturbance must be considered by placing the return period of the disturbance events in perspective with the life span of the affected organism (Turner and Dale, 1998): as trees can live for decades or even centuries in a forest, they can be exposed to centennial disturbance events. Therefore, a forest could be capable of adapting to frequent cyclone visits without suffering catastrophic loss, as long as their intensity is within the tolerance range of the forest. On the other hand, extremely intense cyclones, even when occurring on a centennial basis, could be sufficient to cause severe consequences on forest structure and potentially even threaten the very persistence of the forest ecosystem.
Some global-scale comparative studies have demonstrated a relationship between structural or functional composition of forests and frequency of cyclone events (Hogan et al., 2018; Ibanez et al., 2019). Since in real life the frequency of cyclone events and the average experienced cyclone intensity are highly correlated, this observed relationship could be partially due to variation in intensity as well. On the other hand, if we take into account the phenomenon of delayed mortality due to wind-induced partial tree damage, there could potentially be a genuine effect of cyclone frequency. Finally, in this study, we tested the model at a forest site which has experienced high cyclone frequency in the past: it is possible that a forest site which has experienced low cyclone frequency in the past could respond more strongly to an increase in cyclone frequency. In light of predictions on future increase in tropical cyclone intensity (Knutson et al., 2020), this result suggests that forest ecosystems in cyclone-prone areas may risk undergoing profound structural modifications or even state shifts, and more intensive and preemptive monitoring would be needed to anticipate the consequences (Newman, 2019). Modeling scenarios of simultaneous modifications of wind frequency and intensity, as well as gradual changes of the wind regime over the course of the simulation (non-stationary extreme winds) should also be explored, in order to improve the realism of disturbance forcing.
Effect of Topography
Topography alters the impact of extreme wind on forests in complex ways. Many studies have found that exposed locations tended to be subject to more severe wind damage than sheltered locations (Ruel et al., 1998; Magnabosco Marra et al., 2014). In this study, we introduced a simple correction factor to model the effect of quadrat-wide topographic heterogeneity, based on the assumption that wind speed accelerates locally on exposed terrain such as hilltop and mountain ridges, and decelerates locally in sheltered terrain such as valleys (Belcher et al., 2011; Mitchell, 2013). The simulation results showed a weak but significant effect of topographic heterogeneity on canopy height and biomass stocks within the forest plot at Fushan, with higher average canopy height and aboveground biomass at more exposed quadrats. The increase of aboveground biomass at more exposed quadrats was consistent with the hump-shaped response of biomass as wind disturbance strength increased (Figure 1). However, field observations were not consistent with the prediction, showing higher stem density and lower average canopy height at higher quadrats, and no significant relationship between biomass and quadrat elevation (Figure 5 and Table 2).
The discrepancy between the predicted pattern and field-observed pattern strongly suggests that topographic effect on wind-induced mortality is not a simple function of elevation, and that it will be necessary to refine the model representation of wind-topography interaction. This could be improved, for example, by taking into account canopy surface model and wind direction, as well as tree acclimation to the local wind environment through resource reallocation and modification of allometry (Eloy et al., 2017; Dèfossez et al., 2021).
In the current study, wind speed correction was based on Global Wind Atlas data generated by the WasP model, which is the most applied model for predicting topographic effects on the local wind climate, in particular for the wind energy industry (Finnigan et al., 2020). The model is based on an analytical solution of wind flow over hills originally formulated by Jackson and Hunt (1975). It works best in regions with moderate slopes (≤1:10), which is the case at the Fushan site. Future work will require evaluation of the suitability of GWA data to represent spatial variation of wind speed at forest sites of various steepness and aerodynamic roughness.
Nevertheless, this preliminary exploration demonstrated how spatial variation of wind speed could be coupled to wind-induced tree mortality in an individual-based model, and could serve as a framework under which to further investigate how topography mediates the effect of wind disturbances.
Challenges of Model Representation of Wind-Induced Tree Mortality
The first and foremost challenge when simulating wind disturbance in a mixed-sized forest is the description of the wind profile: since the individual-based model TROLL does not prescribe fixed stand-level characteristics (notably top canopy height), many factors that control wind profile dynamically change across time and space. Wind speeds above the tree canopy are commonly modeled by a logarithmic profile (Raupach et al., 1991), which is the approach taken in the ForestGALES model. The aerodynamic parameters used in the logarithmic profile have been shown to depend on the plant area index, and as such, they are expected to vary seasonally. A detailed parameterization has recently been proposed based on remote-sensing LAI and canopy height products, which could help account for this variability and enhance realism of wind profile modeling (Liu et al., 2021).
In this study, we further assumed that the wind speed experienced by trees within the canopy follows an exponentially decreasing profile (Inoue, 1963), and that trees well below the displacement height in effect do not experience wind disturbance; other equations have been derived to describe the decreasing wind speed profile within the canopy (Raupach and Thom, 1981; Ancelin et al., 2004), and multiple measurements have also been made in different canopies (Raupach et al., 1996). Significant variations in wind profile could exist due to the heterogeneity of forest structural characteristics, such as leaf area index, stem density and spacing (Lalic et al., 2003; Moon et al., 2016; de Santana et al., 2017). In addition, the interaction of horizontal winds with the canopy structure creates turbulent eddy structures, whose effects have not yet been fully explored in the current model (Raupach et al., 1996; de Langre, 2008). For example, the gust structure of the wind could be accounted for by including a gust factor, as in the original ForestGALES model (Quine et al., 2021).
The original ForestGALES model estimated critical wind speed for both types of wind-induced damage, tree uprooting and stem breakage. In a preliminary test, we simulated both processes, but found that few tree uprooting occurred compared to stem breakage (Supplementary Appendix C). This is consistent with the empirical mortality survey at Fushan, where many more dead trees exhibit stem breakage than uprooting (Supplementary Appendix C). This has motivated our decision to simplify model representation and focus on stem breakage. One further motivation for ignoring tree uprooting is that it is largely controlled by root anchoring, which is currently still difficult to represent mechanistically and to parameterize for all tree species, due to our limited understanding on root traits and the physics underlying root-soil interaction. In the future, efforts should be made to devote more attention to the process of root anchoring.
Lastly, although we parameterized species-specific wind susceptibility using plant traits to the extent possible, and assumed that the free parameter P was identical for all the trees in a forest stand, P may in reality still be species-specific due to factors such as wood deformities, stem taper, relative allocation of total biomass to stem weight, as well as the capacity for defoliation that reduces wind loading. These factors could all contribute to model uncertainty, and further investigation is needed to better constrain them in future model developments.
Field Mortality Survey Data
In this study, we retrieved data from the annual mortality surveys conducted in ForestGEO sites, in the hope of calibrating the model using empirical mortality data as a complement to inventory data. The survey data could inform us on the number of tree deaths and treefall that occur within the forest plot, and simulated mortality rates showed good correspondence with observed values calculated from the survey data, but inferring the cause of tree death from the observed damage modes of the dead trees has proven to be difficult. A tree may experience multiple damage successively or simultaneously, which would all contribute to causing treefall, or it may experience damage after its death: these different scenarios could not be distinguished by post hoc records of observed damage on dead trees. In addition, a large proportion of stem breakage and uprooting in the understory are likely to be caused by treefall events of neighboring trees, and not directly by wind.
As a consequence, it is possible that the field observed numbers of tree deaths with uprooting or stem breakage were an overestimation of the wind-induced uprooting or stem breakage events in reality. However, the observed fraction of tree deaths due to treefalls were considerably lower than the simulated values, suggesting that there might be additional acclimation and adaptation phenomena limiting treefalls, which were not accounted for in the current model. Nevertheless, the annual mortality survey data still contain the most detailed fine-scale records on tree mortality in species-rich natural forests available to date, and the observed patterns of damage modes, while not sufficient to calibrate the model, still served as a heuristic basis that provide information on patterns of tree death.
Perspectives
In a mixed-sized natural forest, natural thinning and gap dynamics frequently modify tree density and local stand height, causing changes in the level of physical sheltering from neighboring trees: the wind loading and damage risk of a tree may change considerably as its sheltering status changes even when its size does not (Schelhaas et al., 2007; Seidl et al., 2014; Quine et al., 2021). In the current implementation of the wind damage model, local sheltering is accounted for by considering a tree’s height with respect to local canopy top height, simulating within-canopy wind attenuation, and assigning a height threshold under which a tree is not considered to experience wind disturbances. A finer representation of the effect of local sheltering could be achieved by including a “competition index,” calculated based on a tree’s relative size to neighboring trees, in the formulation of the tree’s turning moment (TC), so that local sheltering is represented as a continuous variation and not as a single cutoff point (Hale et al., 2012; Duperat et al., 2021), even though the choice of competition index is not trivial and requires careful consideration. In addition, the TC equation is currently empirically fitted to a limited number of temperate plantation forest sites, and its transferability to the tropics and to mixed-sized natural forests needs to be examined in more detail: recent works of fine-scale measurement of tree movement in response to wind in natural forests represent the first step to overcoming this challenge (Jackson et al., 2021).
The current model could be further refined by including other aspects of wind impact on trees, such as successive damage that does not immediately cause death but increases delayed mortality (Walker, 1995; Tanner et al., 2014), coping mechanisms such as defoliation (reduction of wind drag at the cost of temporary lower productivity) and re-sprouting (allowing survival even after stem breakage). Other factors influencing tree mortality, such as preexisting stem rot or deformities, interactions with other forms of disturbance such as insect attacks, fire and drought (Seidl et al., 2011; Reichstein et al., 2013; Newman, 2019). Human land use legacy and fragmentation (Laurance and Curran, 2008; Uriarte et al., 2009; McGroddy et al., 2013; Schwartz et al., 2017) could also be considered conjointly with the wind disturbance model: this could potentially be useful for forest conservation and management, by evaluating how forest response to disturbance is modulated by different resource use and management strategies (Hogan et al., 2020). Parameter calibration and model validation could also be improved with the help of high-resolution satellite data that monitor wind gap formation and dynamics (Hayashi et al., 2015; Kislov and Korznikov, 2020; Ballère et al., 2021).
Finally, although the current study focuses on the impacts of wind on a cyclone-prone forest, the model we developed could also be applied to explore wind vulnerability of forests that are less accustomed to wind disturbances, as well as the effects of localized wind blowdown events, which are thought to shape the structure and dynamics of Amazonian forest (Magnabosco Marra et al., 2018; Negrón-Juárez et al., 2018; Peterson et al., 2019). The exploration of more forest settings and wind regimes could help us go beyond single forest plots and take into account landscape- and regional-level heterogeneity in the model (Seidl et al., 2014; Peereman et al., 2020), in order to explore the consequences of wind disturbance at a landscape or even regional level.
Conclusion
In this study, we explored the long-term effects of wind-induced tree mortality to a subtropical forest. The results indicate that wind disturbance could have strong negative effects on forest structure when intensity is high, which has important implications given the projected increase in tropical cyclone intensity. This modeling framework of wind-induced tree mortality, including the preliminary implementation of topographic effects, could serve as the basis for improving representation of the mortality processes in vegetation models, deepen our mechanistic understanding of how wind disturbance acts on forests over a large spatial scale in conjunction with other types of disturbance, as well as generate predictions on the future of natural forests in response to the changing wind regime.
Data Availability Statement
The original contributions presented in the study are included in the article/Supplementary Material, further inquiries can be directed to the corresponding author/s.
Author Contributions
EPR, BG, FF, IM, and JC contributed to conception and design of the study. EJ processed and provided climate forcing datasets. I-FS provided Fushan field census data. EPR performed the model simulations and wrote the first draft of the manuscript. EPR, BG, and JC wrote sections of the manuscript. All authors contributed to manuscript revision, read, and approved the submitted version.
Funding
This work was supported by the “Investissement d’Avenir” grants managed by the Agence Nationale de la Recherche (CEBA, ref. ANR-10-LABX-25-01; TULIP, ref. ANR-10-LABX-0041; ANAEE-France: ANR-11-INBS-0001). This work was also funded by the administrative region of Occitanie (France) and Centre National d’Études Spatiales (CNES).
Conflict of Interest
The authors declare that the research was conducted in the absence of any commercial or financial relationships that could be construed as a potential conflict of interest.
Publisher’s Note
All claims expressed in this article are solely those of the authors and do not necessarily represent those of their affiliated organizations, or those of the publisher, the editors and the reviewers. Any product that may be evaluated in this article, or claim that may be made by its manufacturer, is not guaranteed or endorsed by the publisher.
Acknowledgments
Simulations were run on the OLYMPE cluster at CALMIP, Toulouse. We thank Dr. Chia-Hao Chang-Yang at the National Sun Yat-sen University for helping to provide us with the mortality survey data at Fushan FDP. The data at Fushan FDP results from long-term fieldwork and research supported by the Taiwan Forestry Bureau, the Taiwan Forestry Research Institute and the Ministry of Science and Technology of Taiwan. We would like to express our gratitude to all field technicians and students who helped with the implementation and recensus of Fushan Forest Dynamics plots. We also thank the staff at Fushan Research Center for providing logistic support.
Supplementary Material
The Supplementary Material for this article can be found online at: https://www.frontiersin.org/articles/10.3389/ffgc.2022.753100/full#supplementary-material
Footnotes
- ^ https://rda.ucar.edu/datasets/ds314.3/
- ^ https://www.ncdc.noaa.gov/ibtracs/index.php?name=ib-v4-access
References
Ancelin, P., Courbaud, B., and Fourcaud, T. (2004). Development of an individual tree-based mechanical model to predict wind damage within forest stands. Ecol. Manage. 203, 101–121. doi: 10.1016/j.foreco.2004.07.067
Anderson-Teixeira, K. J., Davies, S. J., Bennett, A. C., Gonzalez-Akre, E. B., Muller-Landau, H. C., Wright, S. J., et al. (2015). CTFS-ForestGEO: A worldwide network monitoring forests in an era of global change. Glob. Chang. Biol. 21, 528–549. doi: 10.1111/gcb.12712
Anthes, R. A. (1982). Tropical Cyclones: Their Evolution, Structure And Effects. Ephrata, PA: American Meteorological Society, 20.
Arellano, G., Zuleta, D., and Davies, S. J. (2021). Tree death and damage: A standardized protocol for frequent surveys in tropical forests. J. Veg. Sci. 32:981. doi: 10.1111/jvs.12981
Badger, J., Hahmann, A., Larsén, X. G., Badger, M., Kelly, M., Olsen, B. T., et al. (2015). The Global Wind Atlas: An EUDP Project Carried out by DTU Wind Energy.
Ballère, M., Bouvet, A., Mermoz, S., Le Toan, T., Koleck, T., Bedeau, C., et al. (2021). SAR data for tropical forest disturbance alerts in French Guiana: benefit over optical imagery. Remote Sens. Environ. 252:159. doi: 10.1016/j.rse.2020.112159
Belcher, S. E., Harman, I. N., and Finnigan, J. J. (2011). The wind in the willows: Flows in forest canopies in complex terrain. Annu. Rev. Fluid. Mech. 44, 479–504. doi: 10.1146/annurev-fluid-120710-101036
Bellingham, P. J., Kapos, V., Varty, N., Healey, J. R., Tanner, E. V. J., Kelly, D. L., et al. (1992). Hurricanes need not causes high mortality: the effects of Hurricane Gilbert on forest in Jamaica. J. Trop. Ecol. 8, 217–223. doi: 10.1017/S0266467400006386
Bellingham, P. J., Kohyama, T. S., and Aiba, S. (1996). The effects of a typhoon on Japanese warm temperate rainforests. Ecol. Res. 11, 229–247. doi: 10.1007/BF02347781
Bugmann, H., Seidl, R., Hartig, F., Bohn, F., Bruna, J., Cailleret, M., et al. (2019). Tree mortality submodels drive simulated long-term forest dynamics: assessing 15 models from the stand to global scale. Ecosphere 10:2. doi: 10.1002/ecs2.2616
Chan, K. T. F., and Chan, J. C. L. (2012). Size and strength of tropical cyclones as inferred from QuikSCAT data. Mon. Weath. Rev. 140, 811–824. doi: 10.1175/MWR-D-10-05062.1
Chave, J. (1999). Study of structural, successional and spatial patterns in tropical rain forests using TROLL, a spatially explicit forest model. Ecol. Modell. 124, 233–254. doi: 10.1016/S0304-3800(99)00171-4
Chen, Y.-Y., Gardiner, B. A., Pasztor, F., Blennow, K., Ryder, J., Valade, A., et al. (2018). Simulating damage for wind storms in the land surface model ORCHIDEE-CAN (revision 4262). Geosci. Model. Dev. 11, 771–791. doi: 10.5194/gmd-11-771-2018
Chi, C.-H., McEwan, R. W., Chang, C.-T., Zheng, C., Yang, Z., Chiang, J.-M., et al. (2015). Typhoon disturbance mediates elevational patterns of forest structure, but not species diversity, in humid monsoon asia. Ecosystems 18, 1410–1423. doi: 10.1007/s10021-015-9908-3
Cremer, K. W., Borough, C. J., McKinnell, F. H., and Carter, P. R. (1982). Effects of stocking and thinning on wind damage in plantations. New Zeal. J. Sci. 12, 224–268.
Curran, T. J., Brown, R. L., Edwards, E., Hopkins, K., Kelley, C., McCarthy, E., et al. (2008). Plant functional traits explain interspecific differences in immediate cyclone damage to trees of an endangered rainforest community in north Queensland. Austral. Ecol. 33, 451–461. doi: 10.1111/j.1442-9993.2008.01900.x
de Langre, E. (2008). Effects of Wind on Plants. Annu. Rev. Fluid. Mech. 40, 141–168. doi: 10.1146/annurev.fluid.40.111406.102135
de Santana, R. A. S., Dias-Júnior, C. Q., do Vale, R. S., Tóta, J., and Fitzjarrald, D. R. (2017). Observing and modeling the vertical wind profile at multiple sites in and above the amazon rain forest canopy. Adv. Meteorol. 2017:157. doi: 10.1155/2017/5436157
de Toledo, J. J., Magnusson, W. E., Castilho, C. V., and Nascimento, H. E. M. (2012). Tree mode of death in Central Amazonia: Effects of soil and topography on tree mortality associated with storm disturbances. Ecol. Manage. 263, 253–261. doi: 10.1016/j.foreco.2011.09.017
Dèfossez, P., Rajaonalison, F., and Bosca, A. (2021). How wind acclimation impacts Pinus pinaster growth in comparison to resource availability. Int. J. For. Res. 95, 118–129. doi: 10.1093/forestry/cpab028
Dorman, J. L., and Sellers, P. J. (1989). A global climatology of albedo, roughness length and stomatal resistance for atmospheric general circulation models as represented by the Simple Biosphere Model (SiB). J. Appl. Meteorol. 28, 833–855. doi: 10.1175/1520-0450(1989)028<0833:agcoar>2.0.co;2
Dunham, R. A., and Cameron, A. D. (2000). Crown, stem and wood properties of wind-damaged and undamaged Sitka spruce. For. Ecol. Manage. 135, 73–81. doi: 10.1016/S0378-1127(00)00299-1
Duperat, M., Gardiner, B., and Ruel, J.-C. (2021). Testing an individual tree wind damage risk model in a naturally regenerated balsam fir stand: Potential impact of thinning on the level of risk. Forestry 94, 141–150. doi: 10.1093/forestry/cpaa023
Eloy, C., Fournier, M., Lacointe, A., and Moulia, B. (2017). Wind loads and competition for light sculpt trees into self-similar structures. Nat. Commun. 8, 1–12.
Everham, E. M., and Brokaw, N. V. L. (1996). Forest damage and recovery from catastrophic wind. Bot. Rev. 62, 113–185. doi: 10.1007/BF02857920
Farquhar, G. D., von Caemmerer, S., and Berry, J. A. (1980). A biochemical model of photosynthetic CO2 assimilation in leaves of C3 species. Planta 149, 78–90. doi: 10.1007/BF00386231
Finnigan, J., Ayotte, K., Harman, I., Katul, G., Oldroyd, H., Patton, E., et al. (2020). Boundary-layer flow over complex topography. Bound. Layer Meteorol. 177, 247–313. doi: 10.1007/s10546-020-00564-3
Fischer, R., Bohn, F., Dantas, K., de Paula, M., Dislich, C., Groeneveld, J., et al. (2016). Lessons learned from applying a forest gap model to understand ecosystem and carbon dynamics of complex tropical forests. Ecol. Modell. 326, 124–133. doi: 10.1016/j.ecolmodel.2015.11.018
Gardiner, B. A., Berry, P., and Moulia, B. (2016). Review: wind impacts on plant growth, mechanics and damage. Plant Sci. 245, 94–118. doi: 10.1016/j.plantsci.2016.01.006
Gardiner, B. A., Byrne, K., Hale, S., Kamimura, K., Mitchell, S. J., Peltola, H., et al. (2008). A review of mechanistic modelling of wind damage risk to forests. Forestry 81, 447–463. doi: 10.1093/forestry/cpn022
Gardiner, B. A., Peltola, H., and Kellomäki, S. (2000). Comparison of two models for predicting the critical wind speeds required to damage coniferous trees. Ecol. Modell. 129, 1–23. doi: 10.1016/S0304-3800(00)00220-9
Green, D. W., Winandy, J. E., and Kretschmann, D. E. (1999). Mechanical properties of wood” in Wood handbook—Wood as an engineering material. Madison, WI: USDA Forest Service, Forest Products Laboratory.
Halder, N. K., Merchant, A., Misbahuzzaman, K., Wagner, S., and Mukul, S. A. (2021). Why some trees are more vulnerable during catastrophic cyclone events in the Sundarbans mangrove forest of Bangladesh? For. Ecol. Manage. 490:119117. doi: 10.1016/j.foreco.2021.119117
Hale, S. E., Gardiner, B. A., Peace, A., Nicoll, B., Taylor, P., and Pizzirani, S. (2015). Comparison and validation of three versions of a forest wind risk model. Environ. Model. Softw. 68, 27–41. doi: 10.1016/j.envsoft.2015.01.016
Hale, S. E., Gardiner, B. A., Wellpott, A., Nicoll, B. C., and Achim, A. (2012). Wind loading of trees: influence of tree size and competition. Eur. J. For. Res. 131, 203–217. doi: 10.1007/s10342-010-0448-2
Harris, I., Jones, P. D., Osborn, T. J., and Lister, D. H. (2014). Updated high-resolution grids of monthly climatic observations - the CRU TS3.10 Dataset. Int. J. Climatol. 34, 623–642. doi: 10.1002/joc.3711
Hayashi, M., Saigusa, N., Oguma, H., Yamagata, Y., and Takao, G. (2015). Quantitative assessment of the impact of typhoon disturbance on a Japanese forest using satellite laser altimetry. Remote Sens. Environ. 156, 216–225. doi: 10.1016/j.rse.2014.09.028
Hogan, J. A., Feagin, R. A., Starr, G., Ross, M., Lin, T.-C., O’connell, C., et al. (2020). A Research framework to integrate cross-ecosystem responses to tropical cyclones. BioScience 70, 477–489. doi: 10.1093/biosci/biaa034
Hogan, J. A., Zimmerman, J. K., Thompson, J., Uriarte, M., Swenson, N. G., Condit, R., et al. (2018). The frequency of cyclonic wind storms shapes tropical forest dynamism and functional trait dispersion. Forests 9:404. doi: 10.3390/f9070404
Hsu, S. A., and Babin, A. (2005). Estimating the radius of maximum wind via satellite during Hurricane Lili (2002) over the Gulf of Mexico. Natl. Weather Assoc. Electron. 6, 1–6.
Ibanez, T., Keppel, G., Baider, C., Birkinshaw, C., Florens, F. B. V., Laidlaw, M., et al. (2020). Tropical cyclones and island area shape species abundance distributions of local tree communities. Oikos 129, 1–11. doi: 10.1111/oik.07501
Ibanez, T., Keppel, G., Menkes, C., Gillespie, T. W., Lengaigne, M., Mangeas, M., et al. (2019). Globally consistent impact of tropical cyclones on the structure of tropical and subtropical forests. J. Ecol. 107, 279–292. doi: 10.1111/1365-2745.13039
Iida, Y., Kohyama, T. S., Swenson, N. G., Su, S.-H., Chen, C.-T., Chiang, J.-M., et al. (2014). Linking functional traits and demographic rates in a subtropical tree community: The importance of size dependency. J. Ecol. 102, 641–650. doi: 10.1111/1365-2745.12221
Inoue, E. (1963). On the Turbulent Structure of Airflow within. J. Meteorol. Soc. Japan Ser. II 41, 317–326. doi: 10.2151/jmsj1923.41.6_317
Jackson, P. S., and Hunt, J. C. R. (1975). Turbulent wind flow over a low hill. Q. J. R. Meteorol. 101, 929–955. doi: 10.1002/qj.49710143015
Jackson, T. D., Sethi, S., Dellwik, E., Angelou, N., and Bunce, A. (2021). The motion of trees in the wind: a data synthesis. Biogeosciences 18, 4059–4072. doi: 10.5194/bg-18-4059-2021
Jackson, T., Shenkin, A., Kalyan, B., Zionts, J., Calders, K., Origo, N., et al. (2019a). A new architectural perspective on wind damage in a natural forest. Front. For. Glob. Chang. 1, 1–10. doi: 10.3389/ffgc.2018.00013
Jackson, T., Shenkin, A., Moore, J., Bunce, A., van Emmerik, T., Kane, B., et al. (2019b). An architectural understanding of natural sway frequencies in trees. J. R. Soc. Interf. 16:116. doi: 10.1098/rsif.2019.0116
Jackson, T., Shenkin, A., Wellpott, A., Calders, K., Origo, N., Disney, M., et al. (2019c). Finite element analysis of trees in the wind based on terrestrial laser scanning data. Agric. For. Meteorol. 265, 137–144. doi: 10.1016/j.agrformet.2018.11.014
Kalnay, E., Kanamitsu, M., Kistler, R., Collins, W., Deaven, D., Gandin, L., et al. (1996). The NCEP/NCAR 40-year Reanalysis Project. Bull. Am. Meteorol. Soc. 77, 437–471.
Kamimura, K., Gardiner, B., Dupont, S., and Finnigan, J. (2019). Agent-based modelling of wind damage processes and patterns in forests. Agric. For. Meteorol. 268, 279–288. doi: 10.1016/j.agrformet.2019.01.020
Kislov, D. E., and Korznikov, K. A. (2020). Automatic windthrow detection using very-high-resolution satellite imagery and deep learning. Remote Sens. 12:1145. doi: 10.3390/rs12071145
Kistler, R., Kalnay, E., Collins, W., Saha, S., White, G., Woollen, J., et al. (2001). The NCEP-NCAR 50-year reanalysis: monthly means CD-ROM and documentation. Bull. Am. Meteorol. Soc. 82, 247–268. doi: 10.1175/1520-0477(2001)082<0247:tnnyrm>2.3.co;2
Knapp, K. R., Kruk, M. C., Levinson, D. H., Diamond, H. J., and Neumann, C. J. (2010). The International Best Track Archive for Climate Stewardship (IBTrACS) Unifying Tropical Cyclone Data. Bull. Am. Meteorol. Soc. 91, 363–376. doi: 10.1175/2009BAMS2755.1
Knutson, T., Camargo, S. J., Chan, J. C. L., Ho, C.-H., Kossin, J., Mohapatra, M., et al. (2020). Tropical cyclones and climate change assessment: Part II: Projected Response to Anthropogenic Warming. Bull. Am. Meteorol. Soc. 101, E303–E322. doi: 10.1175/BAMS-D-18-0194.1
Koch, A., Hubau, W., and Lewis, S. L. (2021). Earth system models are not capturing present-day tropical forest carbon dynamics. Earth Fut. 9, 1–19. doi: 10.1029/2020EF001874
Kulakowski, D., Bebi, P., and Rixen, C. (2011). The interacting effects of land use change, climate change and suppression of natural disturbances on landscape forest structure in the Swiss Alps. Oikos 120, 216–225. doi: 10.1111/j.1600-0706.2010.18726.x
Lalic, B., Mihailovic, D. T., Rajkovic, B., Arsenic, J. D., and Radlovic, D. (2003). Wind profile within the forest canopy and in the transition layer above it. Environ. Model. Softw. 18, 943–950. doi: 10.1016/S1364-8152(03)00068-9
Laurance, W. F., and Curran, T. J. (2008). Impacts of wind disturbance on fragmented tropical forests: a review and synthesis. Austral. Ecol. 33, 399–408. doi: 10.1111/j.1442-9993.2008.01895.x
Lin, T.-C., Hamburg, S. P., Lin, K.-C., Wang, L.-J., Chang, C.-T., Hsia, Y.-J., et al. (2011). Typhoon disturbance and forest dynamics: lessons from a northwest pacific subtropical forest. Ecosystems 14, 127–143. doi: 10.1007/s10021-010-9399-1
Lin, T.-C., Hogan, J. A., and Chang, C.-T. (2020). Tropical cyclone ecology: a scale-link perspective. Trends Ecol. Evol. 35, 594–604. doi: 10.1016/j.tree.2020.02.012
Liu, Y., Guo, W., Huang, H., Ge, J., and Qiu, B. (2021). Estimating global aerodynamic parameters in 1982–2017 using remote-sensing data and a turbulent transfer model. Remote Sens. Environ. 260:112428. doi: 10.1016/j.rse.2021.112428
Lu, X., Yu, H., Yang, X., and Li, X. (2017). Estimating tropical cyclone size in the Northwestern Pacific from geostationary satellite infrared images. Remote Sens. 9:728. doi: 10.3390/rs9070728
Lugo, A. E. (2008). Visible and invisible effects of hurricanes on forest ecosystems: an international review. Austral. Ecol. 33, 368–398. doi: 10.1111/j.1442-9993.2008.01894.x
Lugo, A. E., Rogers, C. S., and Nixon, S. W. (2000). Hurricanes, coral reefs and rainforests: resistance, ruin and recovery in the caribbean. AMBIO J. Hum. Environ. 29, 106–114. doi: 10.1579/0044-7447-29.2.106
Magnabosco Marra, D., Chambers, J. Q., Higuchi, N., Trumbore, S. E., Ribeiro, G. H. P. M., dos Santos, J., et al. (2014). Large-scale wind disturbances promote tree diversity in a Central Amazon forest. PLoS One 9:e114769. doi: 10.1371/journal.pone.0114769
Magnabosco Marra, D., Trumbore, S. E., Higuchi, N., Ribeiro, G. H. P. M., Negrón-Juárez, R. I., Holzwarth, F., et al. (2018). Windthrows control biomass patterns and functional composition of Amazon forests. Glob. Chang. Biol. 24, 5867–5881. doi: 10.1111/gcb.14457
Maréchaux, I., and Chave, J. (2017). An individual-based forest model to jointly simulate carbon and tree diversity in Amazonia: description and applications. Ecol. Monogr. 87, 632–664. doi: 10.1002/ecm.1271
McEwan, R. W., Lin, Y.-C., Sun, I.-F., Hsieh, C.-F., Chang, S.-H., Song, L.-W., et al. (2011). Topographic and biotic regulation of aboveground carbon storage in subtropical broad-leaved forests of Taiwan. For. Ecol. Manage. 262, 1817–1825. doi: 10.1016/j.foreco.2011.07.028
McGroddy, M., Lawrence, D., Schneider, L., Rogan, J., Zager, I., and Schmook, B. (2013). Damage patterns after hurricane dean in the southern yucatan: has human activity resulted in more resilient forests? For. Ecol. Manage. 310, 812–820. doi: 10.1016/j.foreco.2013.09.027
Mitchell, S. J. (2013). Wind as a natural disturbance agent in forests: a synthesis. Forestry 86, 147–157. doi: 10.1093/forestry/cps058
Monteith, J., and Unsworth, M. (2008). Principles of environmental physics: plants, animals, and the atmosphere. Cambridge: Academic Press, doi: 10.1016/C2010-0-66393-0
Moon, K., Duff, T. J., and Tolhurst, K. G. (2016). Sub-canopy forest winds?: understanding wind profiles for fire behaviour simulation. Fire Saf. J. 105, 320–329. doi: 10.1016/j.firesaf.2016.02.005
Moore, J. R., and Maguire, D. A. (2008). Simulating the dynamic behavior of Douglas-fir trees under applied loads by the finite element method. Tree Physiol. 28, 75–83. doi: 10.1093/treephys/28.1.75
Mortensen, N. G., Heathfield, D. N., Landberg, L., Rathmann, O., Troen, I., and Petersen, E. L. (2000). Wind Atlas Analysis and Application Program: WAsP 7. Version 7.1: Help Facility. Roskilde: Risa National Laboratory.
Negrón-Juárez, R. I., Holm, J. A., Magnabosco Marra, D., Rifai, S. W., Riley, J. A., Chamber, J. Q., et al. (2018). Vulnerability of Amazon forests to storm-driven tree mortality. Environ. Res. Lett. 13:54021. doi: 10.1088/1748-9326/aabe9f
Newman, E. A. (2019). Disturbance Ecology in the Anthropocene. Front. Ecol. Evol. 7:147. doi: 10.3389/fevo.2019.00147
Ostertag, R., Silver, W. L., and Lugo, A. E. (2005). Factors affecting mortality and resistance to damage following hurricanes in a rehabilitated subtropical moist forest. Biotropica 37, 16–24. doi: 10.1111/j.1744-7429.2005.04052.x
Pebesma, E. J., and Bivand, R. S. (2005). Classes and methods for spatial data in R. R News 5, 9–13.
Peereman, J., Hogan, J. A., and Lin, T. C. (2020). Landscape representation by a permanent forest plot and alternative plot designs in a Typhoon Hotspot, Fushan, Taiwan. Remote Sens. 12:660. doi: 10.3390/rs12040660
Peterson, C. J., de Mello Ribeiro, G. H. P., Negrón-Juárez, R., Magnabosco Marra, D., Chambers, J. Q., Higuchi, N., et al. (2019). Critical wind speeds suggest wind could be an important disturbance agent in Amazonian forests. For. An Int. J. For. Res. 92, 444–459. doi: 10.1093/forestry/cpz025
Pierce, D. (2021). ncdf4: Interface to Unidata netCDF (Version 4 or Earlier) Format Data Files. R package version 1.19.
Pourrahmati, M. R., Baghdadi, N., Darvishsefat, A. A., Namiranian, M., Gond, V., Bailly, J.-S., et al. (2018). Mapping lorey’s height over Hyrcanian forests of Iran using synergy of ICESat/GLAS and optical images. Eur. J. Remote Sens. 51, 100–115. doi: 10.1080/22797254.2017.1405717
Pugh, T. A. M., Arneth, A., Kautz, M., Poulter, B., and Smith, B. (2019). Important role of forest disturbances in the global biomass turnover and carbon sinks. Nat. Geosci. 12, 730–735. doi: 10.1038/s41561-019-0427-2
Quine, C., Gardiner, B., and Moore, J. R. (2021). “Wind disturbance in forests: The process of wind created gaps, tree overturning, and stem breakage,” in Plant Disturbance Ecology 2e, eds E. A. Johnson and K. Miyanishi (Cambridge: Academic Press), 117–184. doi: 10.1016/b978-0-12-818813-2.00004-6
R Core Team (2021). R: A language and environment for statistical computing. Vienna: R Foundation for Statistical Computing.
Raupach, M. R., and Thom, A. S. (1981). Turbulence in and above plant canopies. Annu. Rev. Fluid. Mech. 13, 97–129. doi: 10.1146/annurev.fl.13.010181.000525
Raupach, M. R., Antonia, R. A., and Rajagopalan, S. (1991). Rough-wall turbulent boundary layers. Appl. Mech. Rev. 44, 1–25. doi: 10.1115/1.3119492
Raupach, M. R., Finnigan, J. J., and Brunet, Y. (1996). Coherent eddies and turbulence in vegetation canopies: the mixing-layer analogy. Bound. Layer Meteorol. 78, 351–382. doi: 10.1007/BF00120941
Reichstein, M., Bahn, M., Ciais, P., Frank, D., Mahecha, M. D., Seneviratne, S. I., et al. (2013). Climate extremes and the carbon cycle. Nature 500, 287–295. doi: 10.1038/nature12350
Réjou-Méchain, M., Tanguy, A., Piponiot, C., Chave, J., and Hérault, B. (2017). BIOMASS: an R package for estimating above-ground biomass and its uncertainty in tropical forests. Methods Ecol. Evol. 8:9. doi: 10.1111/2041-210X.12753
Ruel, J.-C., Pin, D., and Cooper, K. (1998). Effect of topography on wind behaviour in a complex terrain. Forestry 71, 261–265. doi: 10.1093/forestry/71.3.261
Schelhaas, M. J., Kramer, K., Peltola, H., van der Werf, D. C., and Wijdeven, S. M. J. (2007). Introducing tree interactions in wind damage simulation. Ecol. Modell. 207, 197–209. doi: 10.1016/j.ecolmodel.2007.04.025
Schwartz, N. B., Uriarte, M., Defries, R., Bedka, K. M., Fernandes, K., Gutiérrez-Vélez, V., et al. (2017). Fragmentation increases wind disturbance impacts on forest structure and carbon stocks in a western Amazonian landscape. Ecol. Appl. 27, 1901–1915. doi: 10.1002/eap.1576
Seidl, R., Fernandes, P. M., Fonseca, T. F., Gillet, F., Jönsson, A. M., Merganičová, K., et al. (2011). Modelling natural disturbances in forest ecosystems: a review. Ecol. Modell. 222, 903–924. doi: 10.1016/j.ecolmodel.2010.09.040
Seidl, R., Rammer, W., and Blennow, K. (2014). Simulating wind disturbance impacts on forest landscapes: Tree-level heterogeneity matters. Environ. Model Softw. 51, 1–11. doi: 10.1016/j.envsoft.2013.09.018
Sellers, P. J., Tucker, C. J., Collatz, G. J., Los, S. O., Justice, C. O., Dazlich, D. A., et al. (1996). A revised land surface parameterization (SiB2) for atmospheric GCMs. Part II: The generation of global fields of terrestrial biophysical parameters from satellite data. J. Clim. 9, 706–737. doi: 10.1175/1520-0442(1996)009<0706:arlspf>2.0.co;2
Shaw, R. H., and Pereira, A. R. (1982). Aerodynamic roughness of a plant canopy: A numerical experiment. Agric. Meteorol. 26, 51–65. doi: 10.1016/0002-1571(82)90057-7
Shuttleworth, W. J., Leuning, R., Black, T. A., Grace, J., Jarvis, P. G., Roberts, J., et al. (1989). Micrometeorology of temperate and tropical forest [and discussion]. Phil. Trans. Roy. Soc. Lond. B 324, 299–334. doi: 10.1098/rstb.1989.0050
Su, S.-H., Chang-Yang, C.-H., Lu, C.-L., Tsui, C.-C., Lin, T.-T., Lin, C.-L., et al. (2007). Fushan subtropical forest dynamics plot: tree species characteristics and distribution patterns. Taipei: Taiwan Forestry Research Institute.
Tanner, E. V. J., Rodriguez-Sanchez, F., Healey, J. R., Holdaway, R. J., and Bellingham, P. J. (2014). Long-term hurricane damage effects on tropical forest tree growth and mortality. Ecology 95, 2974–2983. doi: 10.1890/13-1801.1
Telewski, F. W., and Moore, J. (2016). Trait selection to improve windfirmness in trees. CAB Rev.: Perspect. Agric. Vet. Sci. Nutr. Nat. Resour. 11, 1–10. doi: 10.1079/PAVSNNR201611050
Turner, M. G., and Dale, V. H. (1998). Comparing large, infrequent disturbances: what have we learned? Ecosystems 1, 493–496. doi: 10.1007/s100219900045
Uriarte, M., Canham, C. D., Thompson, J., Zimmerman, J. K., Murphy, L., Sabat, A. M., et al. (2009). Natural disturbance and human land use as determinants of tropical forest dynamics: Results from a forest simulator. Ecol. Monogr. 79, 423–443. doi: 10.1890/08-0707.1
Valinger, E., and Fridman, J. (1999). Models to assess the risk of snow and wind damage in pine, spruce, and birch forests in Sweden. Environ. Manage. 24, 209–217. doi: 10.1007/s002679900227
Van Bloem, S. J., Murphy, P. G., and Lugo, A. E. (2007). A link between hurricane-induced tree sprouting, high stem density and short canopy in tropical dry forest. Tree Physiol. 27, 475–480. doi: 10.1093/treephys/27.3.475
Vandecar, K. L., Lawrence, D., Richards, D., Schneider, L., Rogan, J., Schmook, B., et al. (2011). High mortality for rare species following hurricane disturbance in the southern yucatán. Biotropica 43, 676–684. doi: 10.1111/j.1744-7429.2011.00756.x
Venables, W. N., and Ripley, B. D. (2002). Modern Applied Statistics with S, Fourth Edition. New York, NY: Springer.
Viovy, N. (2018). CRUNCEP Version 7 - Atmospheric Forcing Data for the Community Land Model. Research Data Archive at the National Center for Atmospheric Research, Computational and Information Systems Laboratory. Available online at: http://rda.ucar.edu/datasets/ds314.3/. [accessed May 4, 2017]
Walker, L. R. (1991). Tree damage and recovery from hurricane hugo in luquillo experimental forest, puerto rico. Biotropica 23, 379–385. doi: 10.2307/2388255
Walker, L. R. (1995). Timing of post-hurricane tree mortality in puerto rico. J. Trop. Ecol. 11, 315–320. doi: 10.1017/S0266467400008786
Webb, E. L., van de Bult, M., Fa’aumu, S., Webb, R. C., Tualaulelei, A., and Carrasco, L. R. (2014). Factors affecting tropical tree damage and survival after catastrophic wind disturbance. Biotropica 46, 32–41. doi: 10.1111/btp.12067
Weber, H. C., Lok, C. C. F., Davidson, N. E., and Xiao, Y. (2014). Objective estimation of the radius of the outermost closed isobar in tropical cyclones. Trop. Cyclone Res. Rev. 3, 1–21. doi: 10.6057/2014TCRR01.01
Wilke, C. O. (2020). cowplot: Streamlined Plot Theme and Plot Annotations for ‘ggplot2’. R package version 1.1.1.
Wright, S. J., Kitajima, K., Kraft, N. J. B., Reich, P. B., Wright, I. J., Bunker, D. E., et al. (2010). Functional traits and the growth-mortality trade-off in tropical trees. Ecology 91, 3664–3674. doi: 10.1890/09-2335.1
Xi, W. (2015). Synergistic effects of tropical cyclones on forest ecosystems: a global synthesis. J. For. Res. 26, 1–21. doi: 10.1007/s11676-015-0018-z
Keywords: disturbance regime, windthrow, tree mortality, topography, forest recovery, ecosystem resilience
Citation: Rau EP, Gardiner BA, Fischer FJ, Maréchaux I, Joetzjer E, Sun I-F and Chave J (2022) Wind Speed Controls Forest Structure in a Subtropical Forest Exposed to Cyclones: A Case Study Using an Individual-Based Model. Front. For. Glob. Change 5:753100. doi: 10.3389/ffgc.2022.753100
Received: 04 August 2021; Accepted: 07 April 2022;
Published: 03 May 2022.
Edited by:
Jess K. Zimmerman, University of Puerto Rico, Puerto RicoReviewed by:
Cara Rockwell, Florida International University, United StatesTobias Jackson, University of Cambridge, United Kingdom
Copyright © 2022 Rau, Gardiner, Fischer, Maréchaux, Joetzjer, Sun and Chave. This is an open-access article distributed under the terms of the Creative Commons Attribution License (CC BY). The use, distribution or reproduction in other forums is permitted, provided the original author(s) and the copyright owner(s) are credited and that the original publication in this journal is cited, in accordance with accepted academic practice. No use, distribution or reproduction is permitted which does not comply with these terms.
*Correspondence: Jérôme Chave, amVyb21lLmNoYXZlQHVuaXYtdGxzZTMuZnI=