- 1AMAP, Univ. Montpellier, IRD, CNRS, CIRAD, INRAE, Montpellier, France
- 2Cirad, UPR Forêts et Sociétés, Montpellier, France
- 3Cirad-PERSYST-UPR BioWooEB, Montpellier, France
- 4Faculté des Sciences et Techniques, Université Marien Ngouabi, Brazzaville, Republic of Congo
- 5Gembloux Agro-Bio Tech, University of Liège, Gembloux, Belgium
- 6Plant Systematic and Ecology Laboratory, Department of Biology, Higher Teachers’ Training College, University of Yaoundé I, Yaoundé, Cameroon
Lianas are abundant and diverse in tropical forests and impact forest dynamics. They occupy part of the canopy, forming a layer of leaves overtopping tree crowns. Yet, their interaction with trees has been mainly studied from the ground. With the emergence of drone-based sensing, very high-resolution data may be obtained on liana distribution above canopies. Here, we assessed the relationship between common liana ground measurements and drone-determined liana leaf coverage over tree crowns, tested if this relationship is mediated by liana functional composition, and compared the signature of liana patches and tree crowns in our drone images. Using drone platforms, we acquired very high resolution RGB and multispectral images and LiDAR data over two 9-ha permanent plots located in northern Republic of Congo and delineated liana leaf coverage and individual tree crowns from these data. During a concomitant ground survey, we focused on 275 trees infested or not by lianas, for which we measured all lianas ≥ 1 cm in diameter climbing on them (n = 615) and estimated their crown occupancy index (COI). We additionally measured or recorded the wood density and climbing mechanisms of most liana taxa. Contrary to recent findings, we found significant relationships between most ground-derived metrics and the top-of-view liana leaf coverage over tree crowns. Tree crown infestation by lianas was primarily explained by the load of liana climbing on them, and negatively impacted by tree height. Liana leaf coverage over individual tree crowns was best predicted by liana basal area and negatively mediated by liana wood density, with a higher leaf area to diameter ratio for light-wooded lianas. COI scores were concordant with drone assessments, but two thirds differed from those obtained from drone measurements. Finally, liana patches had a higher light reflectance and variance of spectral responses than tree crowns in all studied spectra. However, the large overlap between them challenges the autodetection of liana patches in canopies. Overall, we illustrate that the joint use of ground and drone-based data deepen our understanding of liana-infestation pathways and of their functional and spectral diversity. We expect drone data to soon transform the field of liana ecology.
Introduction
Lianas are essential components of tropical forests. They may represent up to 20% of woody plant diversity and 40% of stem density in Neotropical forests (Dalling et al., 2012). Because they do not invest into structural support at the adult stage, often using trees to rise to the forest canopy, lianas tend to exhibit a large leaf area-stem diameter ratio compared to trees (Hegarty and Caballé, 1991; Medina-Vega et al., 2021). They can invade more than half of canopy tree crowns (Ingwell et al., 2010), forming a monolayer of leaves overtopping trees and limiting their light acquisition (Avalos et al., 1999; Visser et al., 2018). They are also suspected to better explore and capture soil resources than trees (Collins et al., 2016; Smith-Martin et al., 2019). They thus are in direct competition with trees for both below-ground and above-ground resources (Avalos et al., 1999; De Deurwaerder et al., 2018), reducing forest tree diversity, growth and carbon storage and strongly limiting forest resilience (Schnitzer and Carson, 2010; Laurance et al., 2014; van der Heijden et al., 2015; Tymen et al., 2016). Therefore, accurately estimating liana infestation is important to quantify its effects on forest functions and predict tropical forests dynamics.
Lianas have mostly been studied through ground-level observations, using common measurements such as stem diameters, following international standardized protocols (Clark and Clark, 1990; Gerwing et al., 2006; Schnitzer et al., 2008). However, while they typically represent less than 5% of woody stem biomass, they can occupy up to 30% of the forest leaf area (van der Heijden et al., 2013). To our knowledge, only one study has looked into the relationship between estimates derived from classical ground measurements (i.e., liana stem density, basal area and biomass) and the top-of-view liana leaf coverage over tree crowns, measured by a canopy crane (Cox et al., 2019). This study found no significant correlations between ground-derived estimates and the liana leaf coverage, raising important questions about the conclusions drawn from ground-based measurements on the aboveground impact of lianas on trees. Understanding the relationship between ground-based measurements and the top-of-view liana leaf coverage over tree crowns is thus important to better interpret the conclusions drawn from classical ground measurements and to better understand liana impact and proliferation in tropical forests.
One mechanism that could blur the relationship between ground-based measurements and the top-of-view liana leaf coverage on tree crowns is that lianas do not constitute a homogenous functional group. A recent study has indeed shown that liana trait variations are comparable in magnitude to tropical tree trait variations, within and across studies (Meunier et al., 2020). Indeed, lianas possess a wide range of strategies for climbing and colonizing trees, allowing them to adapt to different environments (Darwin, 1875; Hegarty, 1991; Putz and Holbrook, 1991; Rowe and Speck, 2015). For instance, physiologically, different wood densities may enable lianas to have different growth-survival strategies. As shown for trees, low wood densities tend to characterize acquisitive species, with high growth rates, large and thin leaves, high photosynthesis rates, large wood vessels and high hydraulic conductance (Van Gelder et al., 2006; Chave et al., 2009; Baraloto et al., 2010; Poorter et al., 2010; Werden et al., 2018; Buckton et al., 2019). These characteristics may in turn impact the patterns of biomass allocation between foliage and woody structures in liana species, as shown for trees where the biomass allocated to the foliage decreases with wood density (Mensah et al., 2016). Furthermore, different climbing mechanisms enable lianas to have different exploration strategies to reach the canopy (Darwin, 1875; Putz, 1984; Isnard and Silk, 2009). Active climbers, such as lianas with tendrils or twining species, exhibit a support-seeking behavior through circumnutation, whereas other climbers, for example climbing with hooks, spines or root climbing attachment, have developed entirely different mechanisms to attach and climb (Melzer et al., 2010). Attachment modes and climbing behaviors have been long known to be of key interest for understanding liana ecology (Putz, 1984). For instance, Bongers et al. (2020) recently found that climbing strategies was significantly related to the recruitment rates of lianas in Central Africa.
With the development of new emerging remote sensing tools such as unmanned aerial vehicles (hereafter referred to as drones), we can now acquire very high-resolution data in which patches of liana leaves can be visually detected on individual trees (Waite et al., 2019). These high-resolution images provide us with accurate estimations of liana leaf distribution above individual tree crowns and allow us to assess the accuracy of commonly used ground-based liana infestation estimates such as the Crown Occupancy Index (COI). This index is a semiquantitative ground-based assessment of relative liana leaf cover over trees (Clark and Clark, 1990). Some studies have looked into the accuracy of the COI to estimate liana loads through its relationship with metrics derived from common ground liana measurements (density, basal area), with positive conclusions (van der Heijden et al., 2010). As a consequence, liana-infestation has been monitored through time using the COI, as it is much less time-expensive than classical dendrometric measurements (Ingwell et al., 2010; Wright et al., 2015). However, using high resolution drone data in Malaysia, Waite et al. (2019) showed that even if a significant positive correlation between the COI and the relative liana leaves coverage over tree crowns exists, ground-based COI estimates were prone to errors, with more than 25% of trees incorrectly classified from the ground. Most of these errors occurred for low infestation levels, representing nearly half of the wrongly classified trees. Given the importance of the COI in current liana studies, the accuracy of this index should be further evaluated in different forest contexts.
One important condition for detecting liana leaves in remote sensing products is the existence of different spectral responses between liana and tree leaves. Lianas tend to have different leaf chemical properties than trees, resulting in higher reflectance across the solar spectrum, with higher differences between trees and lianas observed around 550 nm (green domain; Castro-Esau, 2004; Asner and Martin, 2012; Li et al., 2018; Chandler et al., 2021) and in the near infrared (NIR; 780–1,400 nm; Li et al., 2018) and shortwave infrared (SWIR; 1,400–3,000 nm; Chandler et al., 2021; Visser et al., 2021). Some studies have used these differences in spectral responses to detect liana infested trees and monitor infestation rates at the scale of the canopy (Marvin et al., 2016; Li et al., 2018; Chandler et al., 2021). For instance, Li et al. (2018) obtained an accurate classification of liana infested and non-infested trees using a deep self-encoding network at the scale of the canopy, using high-resolution multi-spectral [475 nm (blue) to 840 nm (NIR)] drone data. However, as most previous studies, they solely focused on the presence or absence of lianas and did not quantify the degree of infestation of trees. All studies aiming at quantifying the degree of liana infestation found reliable results only for highly infested trees (>50 or 75% of infestation by lianas) and could not accurately estimate nor detect low liana infestation rates (Marvin et al., 2016; Chandler et al., 2021). A recent study even showed that lianas and trees do not differ significantly in their spectral signatures at the leaf level (Visser et al., 2021). Nevertheless, radiative transfer modeling has suggested that lianas display a lower light absorption and greater projected leaf area, due to flatter leaf angles. This results in a higher reflectance at the canopy scale, especially in the near and shortwave infrared regions and when liana leaves are aggregated within large patches (Visser et al., 2021). Most of these studies were, however, conducted in Neotropical forests while much less is known about the spectral properties of lianas in the paleotropics. Given that lianas are present in more than 133 families of angiosperms (Gentry et al., 1991), probably generating a large range of spectral signatures as observed for trees (Féret and Asner, 2012; Rocchini et al., 2016) and that spectral signatures partly depend on local environmental conditions (Asner and Martin, 2012; Medina-Vega et al., 2021), the extent to which liana leaves can be detected from their spectral signatures should be further investigated, especially with the use of very high-resolution drone data and focusing in other tropical regions than the Neotropics. Furthermore, with the recent advances in processing Light Detection and ranging (LiDAR) data, we now can semi-automatically delineate individual tree crowns thanks to dedicated algorithms (Aubry-Kientz et al., 2019) and thus better disentangle the spectral response of tree crowns from that of liana patches.
In this study, we investigated the relationship between ground-based liana measurements and liana leaf coverage over tree crowns quantified through high-resolution drone images. More specifically, we assessed whether ground-based measurements accurately predict liana leaf coverage over tree crowns and whether these two measurements are mediated by liana functional composition. We also separated the signatures of liana patches and tree crowns both in the visible and non-visible domain to evaluate the extent to which they overlap. We hypothesize that (i) classical ground-based liana measurements are significantly correlated with drone-based liana leaf coverage estimates; (ii) liana wood density and climbing mechanisms both influence liana leaf coverage over tree crowns; (iii) the COI is not an accurate measure of liana leaf coverage for low infested trees, as found by Waite et al. (2019); and (iv) spectral responses of liana patches and tree crowns significantly differ in multispectral data, with higher reflectances of lianas both in the green and near infrared domain, as previously found in the Neotropics.
Materials and Methods
Study Site
Our study took place in Central Africa, in the north of the Republic of Congo, in the Likouala province (2°27′11.87″ N and 17°02′32.17″ E). Mean annual rainfall is 1,605 mm/year (unpublished data obtained from 2003 to 2017 using a local station at Pokola, 140 km away from our study site). The climate is characterized by two dry seasons with a short one from June to August and a long one from December to February. The Loundoungou site is located on a plateau, the geological substrate consists of limestone and alluvium from the Quaternary. Soils can be classified as Xanthic Acrisols, sandy-clay to clay-sandy, representative of the highest and lowest elevations of the plateau (Freycon, 2014). The studied landscape is generally low and flat, between 395 and 470 m asl (Freycon, 2014). The vegetation is a semi deciduous forest characterized by the abundance of trees belonging to the Fabaceae, Annonaceae, and Malvaceae families (Réjou-Méchain et al., 2021).
The experimental study site was settled in 2013–2014 in the Forest Management Unit (UFA) of Loundoungou-Toukoulaka, in a concession managed by the logging company CIB-Olam. The experimental design aims at comparing different silvicultural treatments among four 9-ha plots that were established in similar soil, topography and initial vegetation conditions (using preliminary inventories). In the present study, we focused on two 9-ha permanent plots that experienced highly selective logging operations at the end of 2018 (0.3 trees logged per ha on average). Other human activities are unlikely to have induced major disturbances in the recent decades. Inside each plot, all trees ≥ 10 cm dbh (diameter at breast height) have been identified, located, and their diameter measured each year since 2015 following international standards (Picard and Gourlet-Fleury, 2008), for a total of 6,380 trees ≥ 10 cm dbh measured in 2019.
Drone-Based Data and Pre-field Inventories Analyses
Drone-based acquisitions were performed at three different periods: in June 2018, April 2019 and in February 2020. In 2018 and 2019, Red Green Blue (RGB) images were acquired over the experimental site using an EBEE 03-907 drone, from the manufacturer SENSEFLY, operated by a private company (Sylvafrica). In 2020, we acquired three drone datasets: Red Green Blue (RGB), multispectral and LiDAR data. All flights were conducted under homogeneous sunlit conditions. RGB data were acquired with a Mavic 2 Pro drone, using an integrated three-waveband (RGB) camera (20MP Hasselblad L1D-20c gimbal). The flight trajectory was calculated and conducted with the UGCS software. The acquisitions were made in three flights covering the whole study site in less than 1-h between 7 and 8 a.m., on the 14th of February, with an overlap of 90% between flight lines. This short time of acquisition of all RGB data allowed us to obtain Digital Numbers (DN) values under homogeneous sunlight and atmospheric conditions, and were hereafter assumed as a proxy of relative radiometric responses (radiometric corrections were not possible in our study). Multispectral data were acquired in the red (660 nm ± 40 nm), green (550 nm ± 40 nm), red-edge (735 nm ± 10 nm) and near-infra red (790 nm ± 40 nm) regions using a Parrot Sequoia camera mounted on a DJI Matrice 600 drone. The flight trajectory was also calculated and conducted using UGCS. The acquisitions were made in two overlapping flights over the studied area, on the 13th of February, the first at 8 am and the second at 15 pm, with an overlap of 90% within flight lines. Data from an incident light sensor located above the drone was used for conversion of DN values into bottom-of-atmosphere (BoA) reflectance in the Pix4D software.1 However, as the Pix4D routine did not yield satisfactory results, analyses based on the BoA reflectance values of trees versus lianas were restricted to 60% of the studied area, i.e., using only the first flight to minimize atmospheric effects and avoid inter flight radiometric corrections.
All drone imagery data were processed through Pix4D to produce orthomosaics at spatial resolutions of 10 cm (2018), 5 cm (2019), 3 cm (2020 RGB), and 17 cm (2020 Multispectral). Pix4D used structure from motion algorithms, and other algorithms, to build a dense point cloud from which a digital surface model (DSM) was derived and used to produce an orthomosaic. In all cases, pixels were represented by a minimum of five overlapping images, with a total of 1095 images over 1292 ha (2018), 248 images over 80 ha (2019), 1153 images over 56 ha (2020 RGB) and 2011 images over 450 ha (2020 Multispectral). We used both the Pix4D algorithms of noise filtering, to remove outliers in the generated points cloud, and of surface smoothing (type Sharp), to flatten erroneous small bumps in the DSM.
Finally, very high-resolution LiDAR data were acquired on the 9th of February 2020 over the whole study site. A Yellowscan Surveyor Ultra sensor (combining a Velodyne Ultra Puck, 600 kHz, 905 nm wavelength LiDAR scanner and an Applanix 15 IMU) was flown mounted on the DJI Matrice 600 UAV platform at 60 m above SRTM elevation at a speed of 8 m/s, with an interline distance of 50 m. A multiple return acquisition resulted in a point density of 372 points/m2. We used a Reach RS2 GNSS base station for PPK differential positioning with a global accuracy of less than 4 cm on the X and Y axis (average accuracy of 3.7 cm on all trajectories) and less than 7 cm on the Z axis (average accuracy of 6.3 cm on all trajectories). Trajectories were post-processed in PosPac UAV v9 and point clouds were processed in LASTools (Isenburg, 2014) to derive a 1-m resolution Digital Terrain Model (DTM) using the lasground (with the wilderness option) and las2dem functions. We then normalized the point clouds (using the lasheight function) and assessed the maximum height values with a 1m-grid in the normalized point cloud (lascanopy function) to create a Canopy Height Model (CHM), representing the top-of-canopy height above the ground.
Prior to the field inventory (see next section), we conducted preliminary analyses on the 2018 and 2019 drone datasets in order to facilitate our upcoming fieldwork. Through a visual interpretation of RGB mosaics, we manually delineated what was seemingly liana leaf cover patches over individual tree crowns, using the QGIS open source software (version 3.10). We then validated the presence of lianas in these tree crowns using binoculars in the field to assess the accuracy of these preliminary delineations from the ground. This assessment also both helped our tree selection and liana delineation afterward on the 2020 drone images (see section “Liana Delineation and Spectral Signatures of Lianas”).
Field Inventories
We conducted a field campaign concomitantly with the third drone acquisition in February 2020. Using the field application of QGIS, Qfield,2 we validated and corrected (if needed) the delineation of individual tree crowns and the presence of liana leaf coverage observed on the 2019 RGB images, and recorded the corresponding tree field inventory number for each tree crown. In almost all cases the preliminary delineations of lianas were validated with the presence of liana leaf coverage patches over tree crowns, with only a few errors due to the occurrence of hemi-parasite and hemi-epiphyte plants. Because the number of liana-infested trees was much smaller than that of non-infested ones, we focused our field investigations on trees that were identified as liana-infested in our preliminary analyses and measured non-infested trees in an opportunistic way. It enabled us to optimize our time during our 3-week field mission and to ensure the measurement of numerous liana-infested trees. In total, we were able to link individual tree crowns delineated on drone images with tree ground survey numbers for a total of 275 trees, infested or not by lianas, with diameters ranging from 12.9 to 170.7 cm dbh (median of 55.7 cm dbh). All studied trees had a crown entirely visible from drone images, as estimated from the field.
For each tree, we estimated the liana leaf coverage from the ground using the widely used Crown Occupancy Index (COI; Clark and Clark, 1990; van der Heijden et al., 2010). This semi-quantitative index expresses the relative coverage of liana leaves in the tree crown using five categories: (0) no liana leaves in the crown, (1) 1–25%, (2) 26–50%, (3) 51–75%, and (4) > 75% of the tree crown covered by liana leaves. For each tree, the COI was systematically assessed by two observers, equipped with binoculars, who observed tree crowns from every visible and accessible angle, discussed their estimates and agreed on the final COI score.
All woody lianas ≥ 1 cm in diameter climbing on the studied trees, or visibly entering or leaving the tree crown, were identified by vernacular name and their diameters were measured following international protocols using a manual caliper for small lianas and a tape for large ones (Gerwing et al., 2006; Schnitzer et al., 2008). In total, we measured 615 lianas belonging to 54 vernacular taxa climbing on 104 trees. For each tree, we computed four stand level liana measurements: liana stem number (NL), liana basal area (BAL), liana maximum diameter (DmaxL), liana mean quadratic diameter (QMDL).
We measured or recorded two functional traits: the stem wood infradensity and the climbing mechanism. For stem wood infradensity, we collected stem cylinders of more than 2 cm in diameter at 1.3 m from the stem root, out of the permanent plot to avoid destructive measurements within the plots. In total, we collected stems belonging to the most abundant 20 vernacular taxa, representing 83% of the 615 liana stems inventoried. Wood samples of approximately 2 cm of length were debarked and saturated with water under pressure and the Archimedes thrust was measured following the protocol described by Birouste et al. (2014). Wood samples were first dried in an oven at 45°C for a week to avoid damaging the water saturated materials with sudden extreme temperatures. Then, we rose the temperature to 103°C for 24 h to eliminate all water residuals. Finally, the anhydrous masses of wood samples were measured, to assess the wood infradensity, hereafter named wood density.
Liana climbing mechanisms were recorded for 34 vernacular taxa, representing 92% of the 615 liana stems inventoried. Climbing mechanisms were assigned to each vernacular name following the classification proposed by Sperotto et al. (2020). We classified as “active” climbing mechanisms twining lianas, lianas with tendrils, prehensible branches and lianas with angular branches. We classified as “passive” climbing mechanisms lianas with hooks, spines, adhesive roots and simple scrambling lianas.
Data Analysis
Geospatial Analysis
Tree Crown Segmentation
We used the LiDAR data to delineate tree crowns on the two 9-ha plots. After normalizing the point cloud with the DTM, we removed the ground points and reduced randomly the density of the point cloud to 40 points/m2 to limit computing time. We then used the Adaptative Mean Shift approach (AMS3D; Ferraz et al., 2012) implemented in the open Computree software (Piboule et al., 2013). This approach composes a normalized point cloud into 3D clusters, corresponding to individual tree crowns, by finding local maxima giving a certain cylinder size. We deliberately parameterized the model to over-segment tree crowns (Figures 1A,B) because it is then easier to manually merge over-segmented crowns than to segment under-segmented crowns. After extracting the projected shapes from Computree, we manually cleaned the segmentation in QGIS, using our field observations and high resolution RGB and multispectral images (Figures 1C,D). In total, we delineated 1626 individual canopy tree crowns over the 18-ha study area. We finally assigned to each tree its maximum height using the LiDAR-derived CHM model built at 1-m resolution.
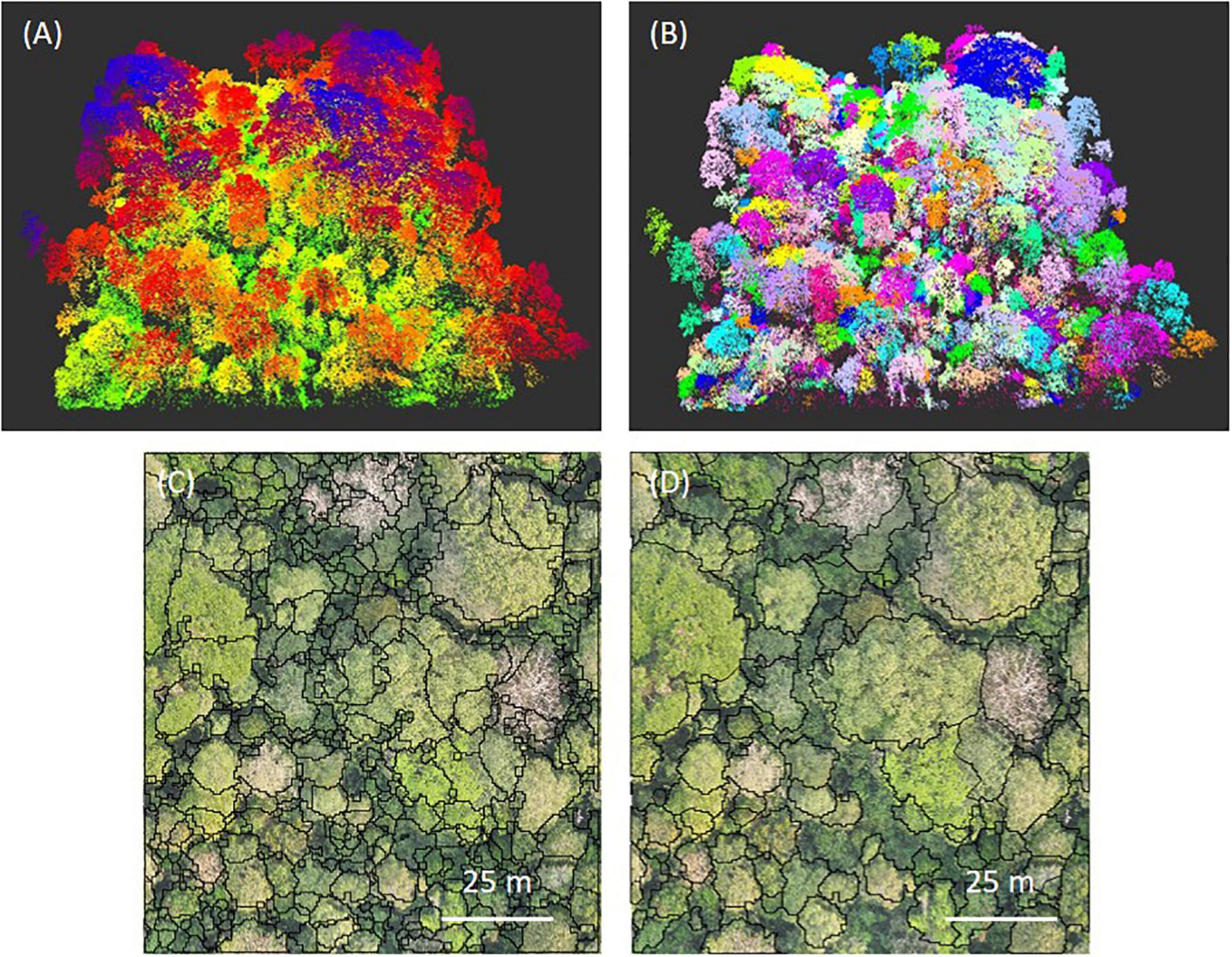
Figure 1. An example of tree crowns segmentation of a 1-ha subplot, with the AMS3D approach. (A) The point cloud before the segmentation, colored by height (green to blue) and (B) after the segmentation, colored by individual tree. The projected 2D shapes of individual tree crowns (in black) before manual cleaning in (C) and after in (D) over an RGB image.
Liana Delineation and Spectral Signatures of Lianas
In all the studied trees validated from the ground, liana leaves were clearly distinguished visually from leaves of their host trees in the visible (RGB) and/or non-visible (Red-Edge and Near Infrared) domain (Figure 2). We thus delineated all visible liana leaf coverage over the two 9-ha plots, manually from QGIS, combining our specialized field observations and a careful visual interpretation using the different 2020 spectral bands available.
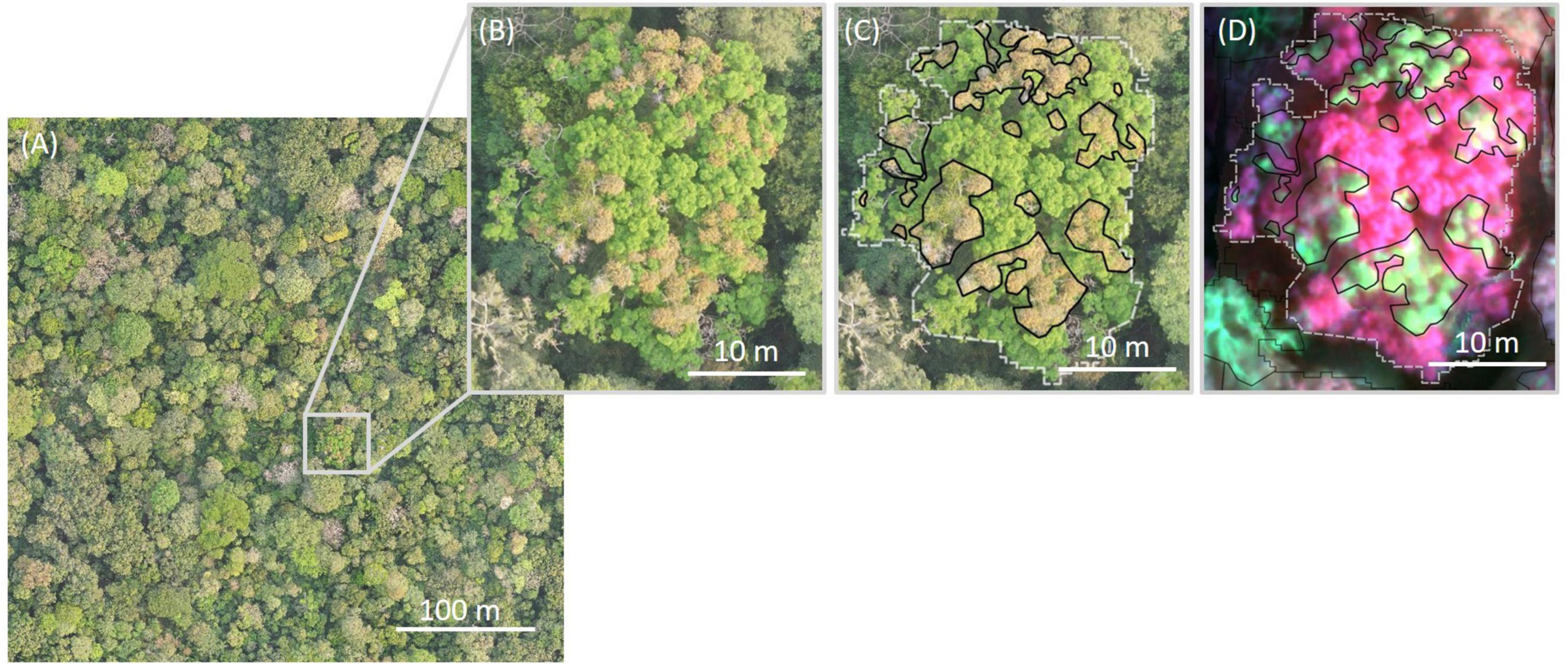
Figure 2. Example of the manual delineation of liana leaves using RGB and multispectral data. (A) RGB mosaic of one of the two 9-ha plots. A liana-infested tree crown is illustrated before (B) and after (C,D) the manual delineation of lianas (in black lines) and the LiDAR segmentation of the tree crown with the AMS3D approach (in white dotted lines) on RGB (C) and multispectral (D) images.
Using the 2020 RGB images, we then selected pixels that were likely dominated by leaves, either from trees or lianas, to quantify the photosynthetic surface of trees and lianas. To do this, we first only selected pixels belonging to a segmented tree crown (see previous section), ignoring canopy gaps and below-canopy vegetation. We then filtered out woody pixels (in our case DN values > 190 in the blue waveband) and pixels corresponding to small gaps in the tree crown (DN values ≤ 100 in the green waveband). Using this dataset, we finally estimated the relative area of liana leaves over the total canopy photosynthetic surface.
To test whether liana leaves expressed a singular spectral response, we averaged pixel DN (RGB data) and reflectance (multispectral data) values for each individual liana patch and liana-free tree crown for all bands independently to minimize the noise associated with pixel-level information. We submitted object-level averaged DN and reflectance values to a Principal Coordinate Analysis (PCA) and compared the liana and tree leaves responses along the first two PCA axes using Wilcoxon tests, to test for difference in signal average, and F-test, to test for difference in signal variance. As mentioned earlier, this analysis was conducted on only 60% of the study area to minimize atmospheric and radiometric bias in multispectral data. Considering multispectral data in DN values instead of reflectance values in the PCA led to the same results (not shown).
Statistical Analyses
Relationship Between the Crown Occupancy Index and Liana Leaf Coverage Over Tree Crowns
We used the whole dataset (275 trees) to study the relationship between the COI determined from the ground and the proportion of photosynthetic surface of lianas on tree crowns determined by drone-based data. We modeled with an ordinal probit regression the relationship between the different ground-determined COI categories (0, 1, 2, 3, or 4) and the proportion of photosynthetic surface of lianas (aka drone-determined liana leaf coverage) on tree crowns.
Relationship Between Stem Ground Measurements and Liana Leaf Coverage Over Tree Crowns
We performed all statistical analyses at the scale of individual trees. We divided our dataset in four categories by the type of infestation of the host tree:
(i) trees that were not infested by lianas. These trees did not have any visible liana climbing on them or coming from adjacent trees from the ground (COI of zero), and did not have any liana leaves visible on the crown from the drone images (n = 161 trees);
(ii) trees that were identified as infested by lianas. These trees were identified as infested either from ground measurements or/and drone observations;
(ii)-a trees that were identified as infested from both ground measurements and drone observations (n = 87 trees);
(ii)-b trees that were identified as infested only from ground measurements, with no visible liana leaves on the crown from drone images (n = 17 trees);
(ii)-c trees that were identified as infested only from drone observations, with no visible lianas climbing on them or coming from adjacent trees from the ground (n = 10 trees).
Because including category (i) may lead to an overestimation of model performance, with an inflation of zero values, we only considered infested trees of category (ii) when analysing the relationship between ground measurements and liana leaf coverage. We also discarded infested trees of the category (ii)-c because we either failed to find the corresponding lianas rooted point in the ground or the leaves most probably belonged to epiphytes (see section “Discussion”). Thus, ground measurements and liana leaf coverage comparison were done on a total of 104 trees (categories (ii)-a and (ii)-b). Our infested tree selection was the same as Cox et al. (2019) except that, here, we also included trees with lianas climbing on them without any visible leaves from above.
First, we modeled with a Bernoulli probit-regression, the probability that a tree infested from ground measurements had visible liana leaves on the crown from drone images. This model was calibrated on the 104 trees of [categories (ii)-a and (ii)-b]. Second, we modeled with a log-linear regression, the liana leaf coverage for trees infested from ground measurements and with visible liana leaves on the crown from drone images. This model was calibrated on the 87 trees of [categories (ii)-a].
For the two models, the predictors were selected among the four tree-level liana measurements calculated at the tree level (NL, BAL, DmaxL, QMDL), the LiDAR-derived total tree height (H) and the interactions between NL* QMDL and H* QMDL. We selected the predictors using a stepwise approach (backward/forward) based on the Bayesian Information Criterion (BIC; the best model has the lowest BIC).
Hence, the products of these two models could lead to a model of the liana leaf coverage for trees defined as infested from ground measurements.
Influence of Liana Functional Traits on Liana Leaf Coverage
To study the influence of growth strategies on liana leaf coverage, we analyzed the relationship between the residuals of the selected linear log-log regression model and two liana functional traits averaged at the tree level: the basal-area weighted wood density of lianas (WDL) and the climbing mechanisms of lianas (MechaL). WDL was estimated for every infested tree for which we knew the wood density of liana individuals for more than 80% of the total liana basal area (n = 57 trees) using the equation as follows:
With n the total number of liana stem climbing on the host tree, WDi the wood density for liana species i and BALi the total liana basal area for individuals belonging to the liana species i. on the host tree We calculated the climbing mechanism index (MechaL) indicating the mean liana growth strategy through the following equation:
With na the total number of liana stems with active climbing mechanisms, nbthe total number of liana stems with passive climbing mechanisms and BAia and BAib the basal area of all lianas having an active and passive climbing mechanism, respectively. We calculated MechaL for every infested tree for which we knew the climbing mechanism of more than 80% of the total liana basal area (n = 70 trees).
We did not include directly functional traits variables into the regression models but decided to look at their relationship with the residuals of the models (response minus fitted values), because we did not have trait data information for the whole dataset.
All analyses were conducted on R version 4.0.3 (R Core Team, 2020), using the libraries sf (Pebesma et al., 2018), raster (Hijmans, 2021), lidR (Roussel et al., 2020), MASS (Venables and Ripley, 2002), reshape2 (Wickham, 2007), ggplot2 (Villanueva et al., 2016), DescTools (Signorell et al., 2021), dplyr (Wickham et al., 2021), and RcmdrMisc (Fox et al., 2020).
Results
Amount of Liana Infestation Estimated From the Ground and From Drone Data
Drone- and ground-based measurements indicated that 35 and 38% of the selected 275 trees were infested by lianas, respectively. These numbers are unlikely to be representative because we deliberately sampled a higher proportion of liana-infested trees than non-infested trees. However, at the scale of the study sites (the two 9-ha plots), drone-based measurements indicated that 16.6% of canopy trees (n = 270) were infested by lianas and that liana leaves covered 6.2% of the total canopy photosynthetic surface (n = 594 patches). At the scale of the 275 trees dataset, ground-based measurements indicated that liana-infested trees were infested by an average of 5.9 lianas (range of 1–20) with a mean quadratic diameter of 3.5 cm (range of 0.7–22.8 cm) and an average basal area of 157.8 cm2 (range of 1.6–1027.5 cm2). Using herbariums, we identified a total of 54 liana taxa, although this estimate might be underestimated because some vernacular names cannot yet be assigned to a single species (work in progress). Wood density also varied markedly among liana taxa, ranging from 0.29 to 0.52 g cm–3 (mean of 0.42 g cm–3), and the basal-area weighted wood density of lianas at the scale of an individual tree, WDL, ranged from 0.37 to 0.52 g cm–3 (mean of 0.44 g cm–3). Finally, the dominant climbing mechanisms was active (64% of individuals), with 47% of the total stems belonging to twining lianas.
Link Between Drone and Ground-Based Liana Measurements
Eighty four percent of the trees hosting lianas had visible liana leaves on their tree crowns in drone images [category (ii)-a; red dots in Figure 3]. Thus, 16% of the trees observed as infested from the ground did not have any visible leaf coverage on their crowns when drone data were acquired [category (ii)-b; green dots in Figure 3]. Most of these trees consistently had a COI score of 0 (n = 5) or 1 (n = 11), except one tree that had a COI score of 3. For this tree, our field note indicated that the COI score was uncertain due to the co-existence of a hemi-epiphyte (Ficus sp.) and a mix of old and young liana leaves. Finally, we observed liana leaves in the crown of 10 trees [4%; category (ii)-c; blue dots in Figure 3] for which we failed to assign any corresponding liana stems on the ground.
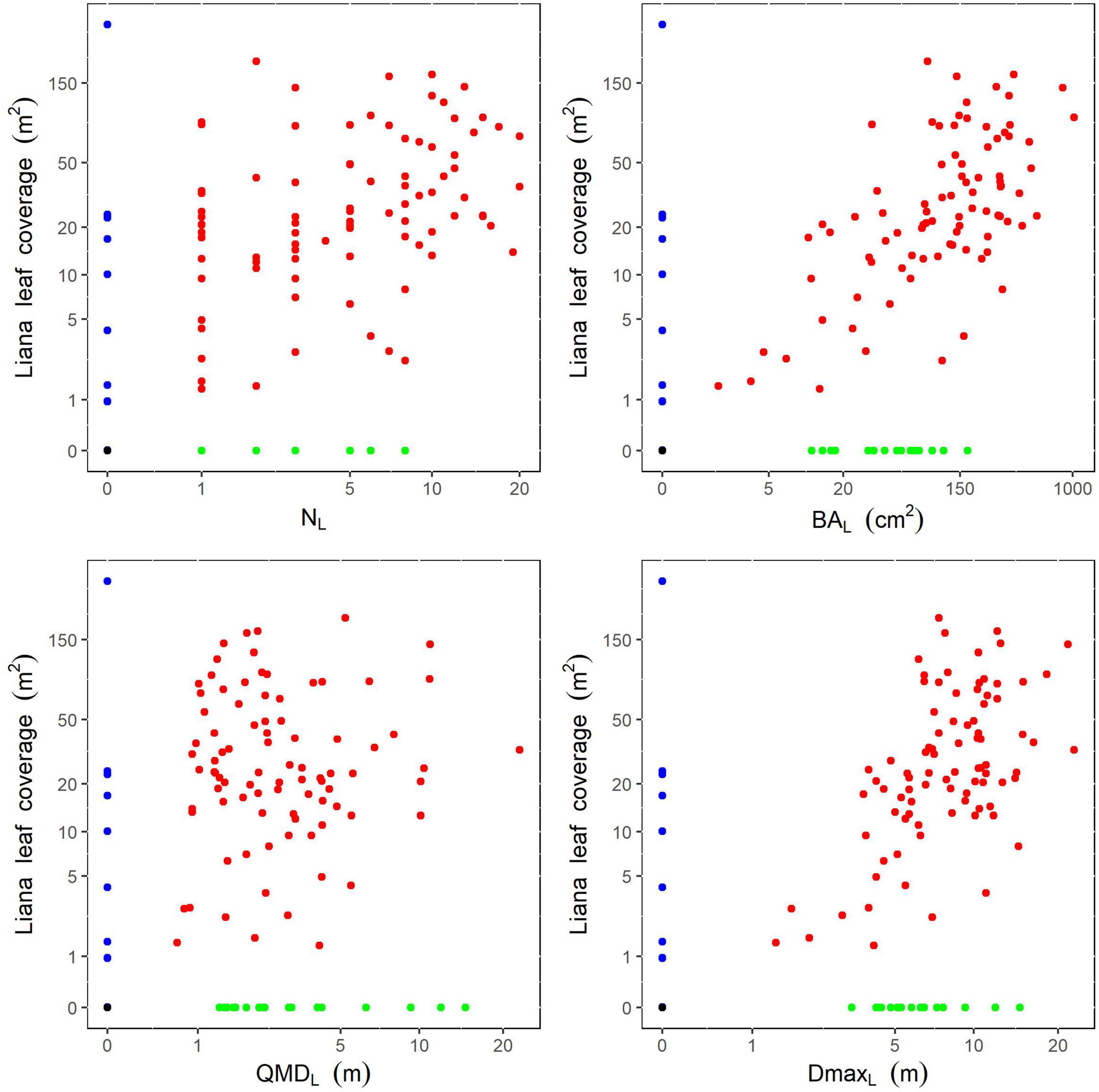
Figure 3. Relationships between liana ground measurements and liana leaf coverage estimated from drone data for the 275 studied trees. Colors illustrate the different infestation categories, black for category (i), red for (ii)-a, green for (ii)-b, and blue for (ii)-c. Axes are shown in log scale. For NL, Spearman’s ρ = 0.43, p < 0.001; for BAL, Spearman’s ρ = 0.58, p < 0.001; for QMDL, Spearman’s ρ = 0.004, p = 0.97; for DmaxL, Spearman’s ρ = 0.48, p < 0.001.
Link Between Liana Leaf Coverage and Classical Ground-Based Liana Measurements
For trees identified as infested either from the ground or drone data (category (ii)), we found that ground-derived liana stand metrics were significantly correlated with the liana leaf coverage measured on drone data (Spearman’s ρ = 0.43–0.58; p < 0.001; Figure 3), except for liana mean quadratic diameter (p = 0.97).
The infestation status of tree crowns (infested or not) was first best predicted by liana basal area and total tree height, with a predominant positive effect of liana basal area and a negative effect of tree height (Table 1). The selected model classified 86% of the 275 trees accurately but tended to overestimate the number of tree crowns infested by liana leaves using ground-based measurements as predictors (Table 2).
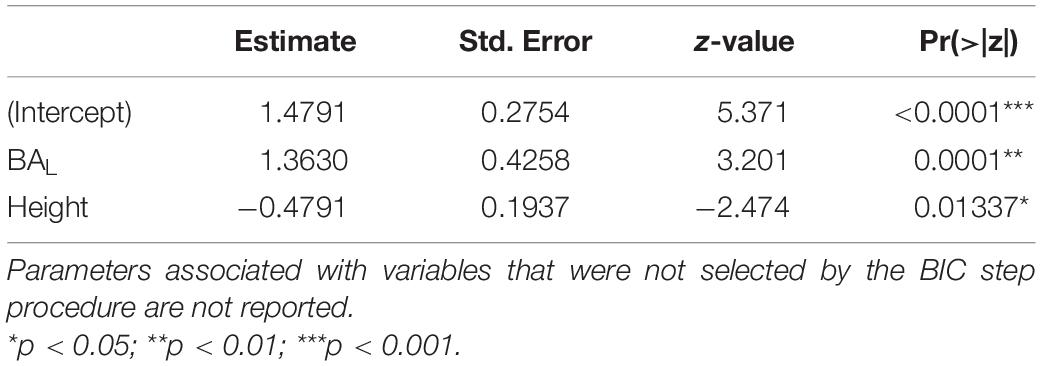
Table 1. Estimates of parameters of the model selected to predict liana infestation status (tree crowns infested or not).
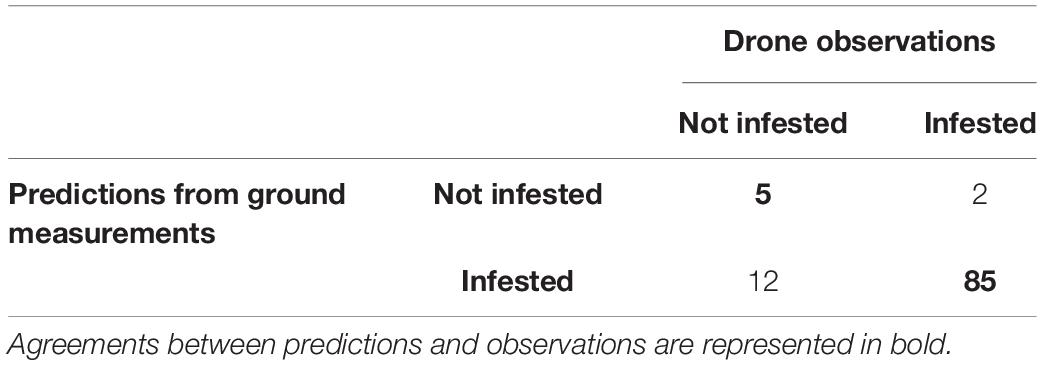
Table 2. Error matrix showing the number of tree crowns predicted to be infested by liana leaves using ground-based measurements as predictors and drone observations as reference data.
When focusing on trees being interpreted as liana-infested from both ground and drone observations [category (ii)-a; n = 87], our BIC selection procedure only retained the total liana basal area per tree as a predictor of liana leaf coverage over tree crowns, with a significant positive effect, explaining 43% of the total variance (intercept = 0.42; slope = 0.60; p < 0.001; Figure 4).
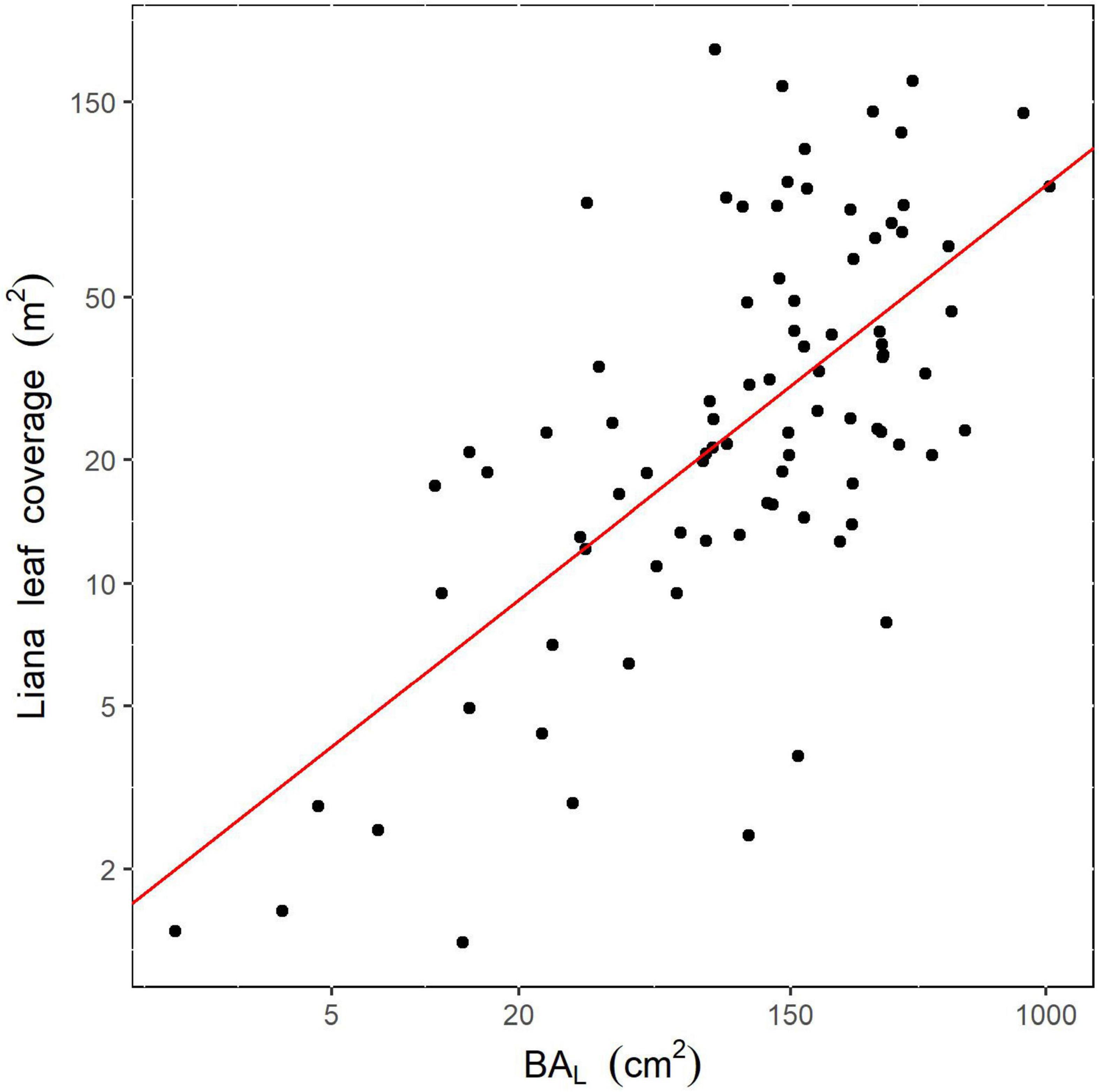
Figure 4. Relationship between total liana basal area measured from the ground and leaf coverage estimated from drone data for 87 tree crowns. The red line illustrates the predicted linear log-log model. Axes are shown in log scale.
We then found a significant, albeit weak, negative effect of liana basal area weighted wood density on the residuals of the selected model predicting liana leaf coverage (intercept = 2.18; slope = -5.10; p = 0.048; Figure 5). Trees hosting lianas with lower wood densities thus tend to have a larger liana leaf coverage over their crowns. By contrast, we did not detect any significant effect of liana climbing mechanisms on the model residuals (p = 0.96).
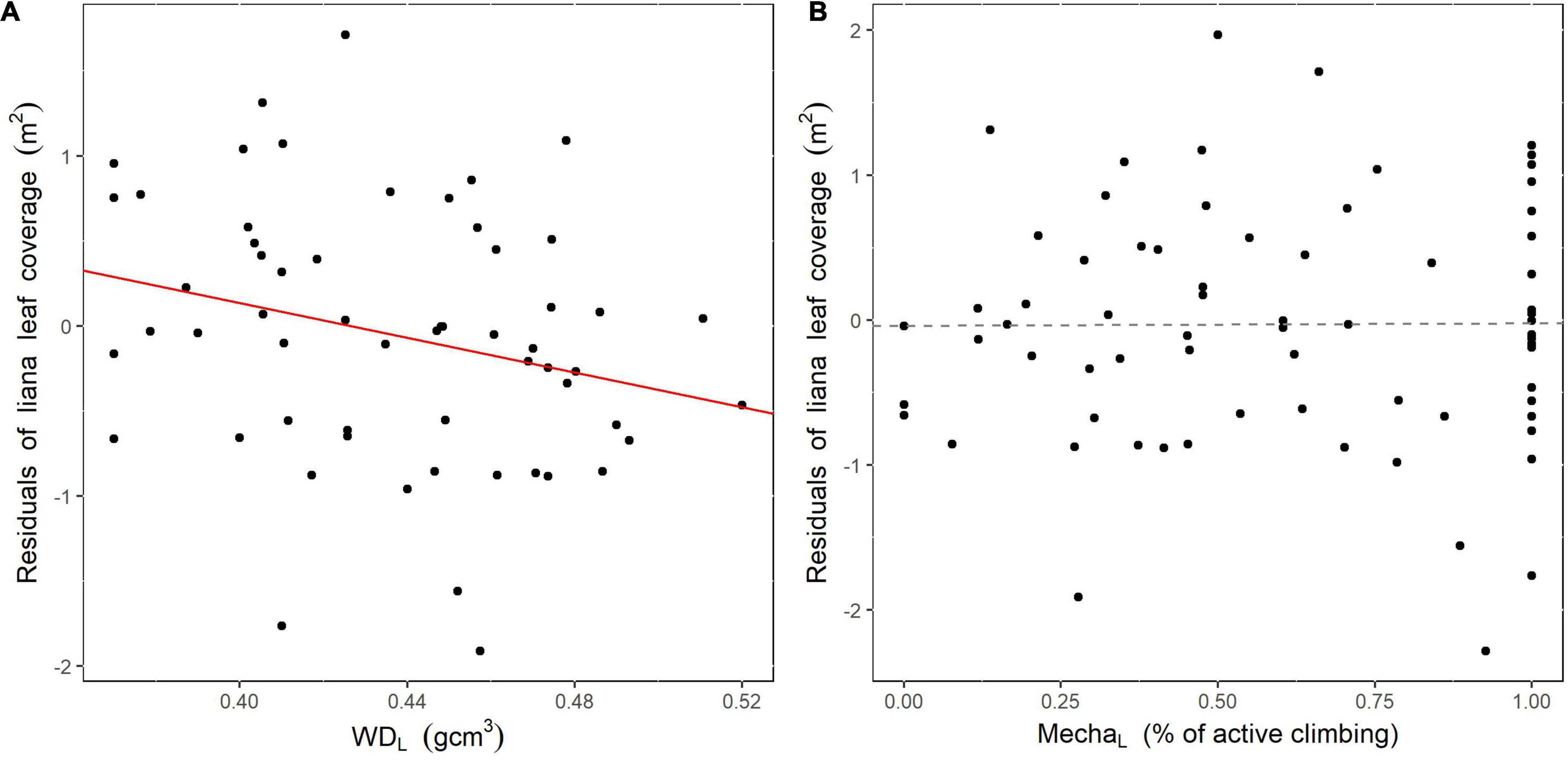
Figure 5. Relationship between the basal area weighted liana wood density (A), or the percentage of active climbing mechanism (B), and the residuals of the selected linear model predicting liana leaf coverage from total liana basal area. The red (significant slope) or dotted gray (non-significant slope) lines illustrate the predicted linear model between the two variables.
Link Between Liana Leaf Coverage and the Crown Occupancy Index
COI scores estimated from the ground and from drone data were significantly concordant (Kendall’s W = 0.94, p < 0.001, N = 275), with the same classification for 72% of the studied trees (Table 3). However, most of these agreements were due to trees identified as non-infested by the two approaches (95% of the trees identified as non-infested from the ground were also identified as non-infested by drone measurements). When the comparison was restricted to trees observed as infested from the ground, only 32% of them were assigned to the same COI category using both approaches, with an overestimation of the ground-based compared to the drone-based estimates (Table 3). For 22% of the trees, the classification varied by one class and, for 6% of the trees, it varied by two or more classes. The probit model confirmed that a large overlap exists between the probabilities of belonging to the different categories along the gradient of infestation, even if the ranks were fairly well-preserved among categories on average (Figure 6).
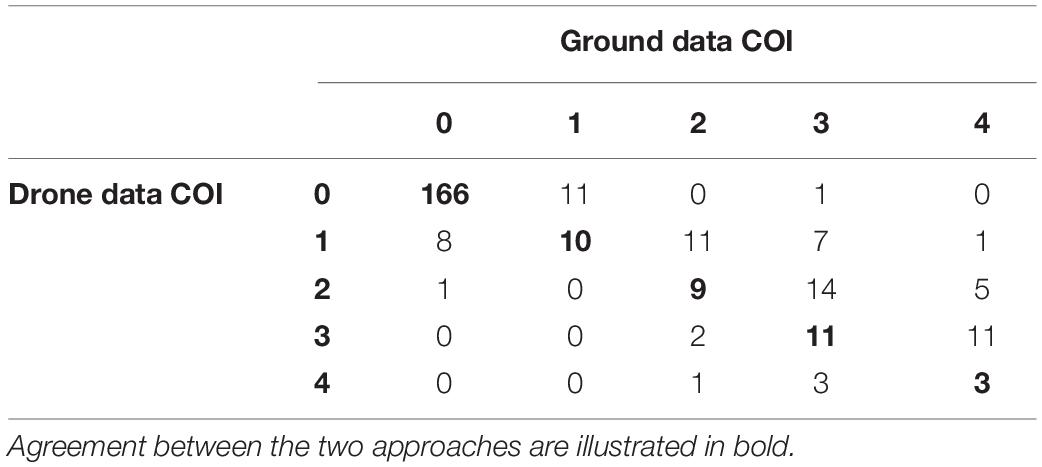
Table 3. Confusion matrix showing the number of trees classified into the five COI classes from ground and drone-based measures.
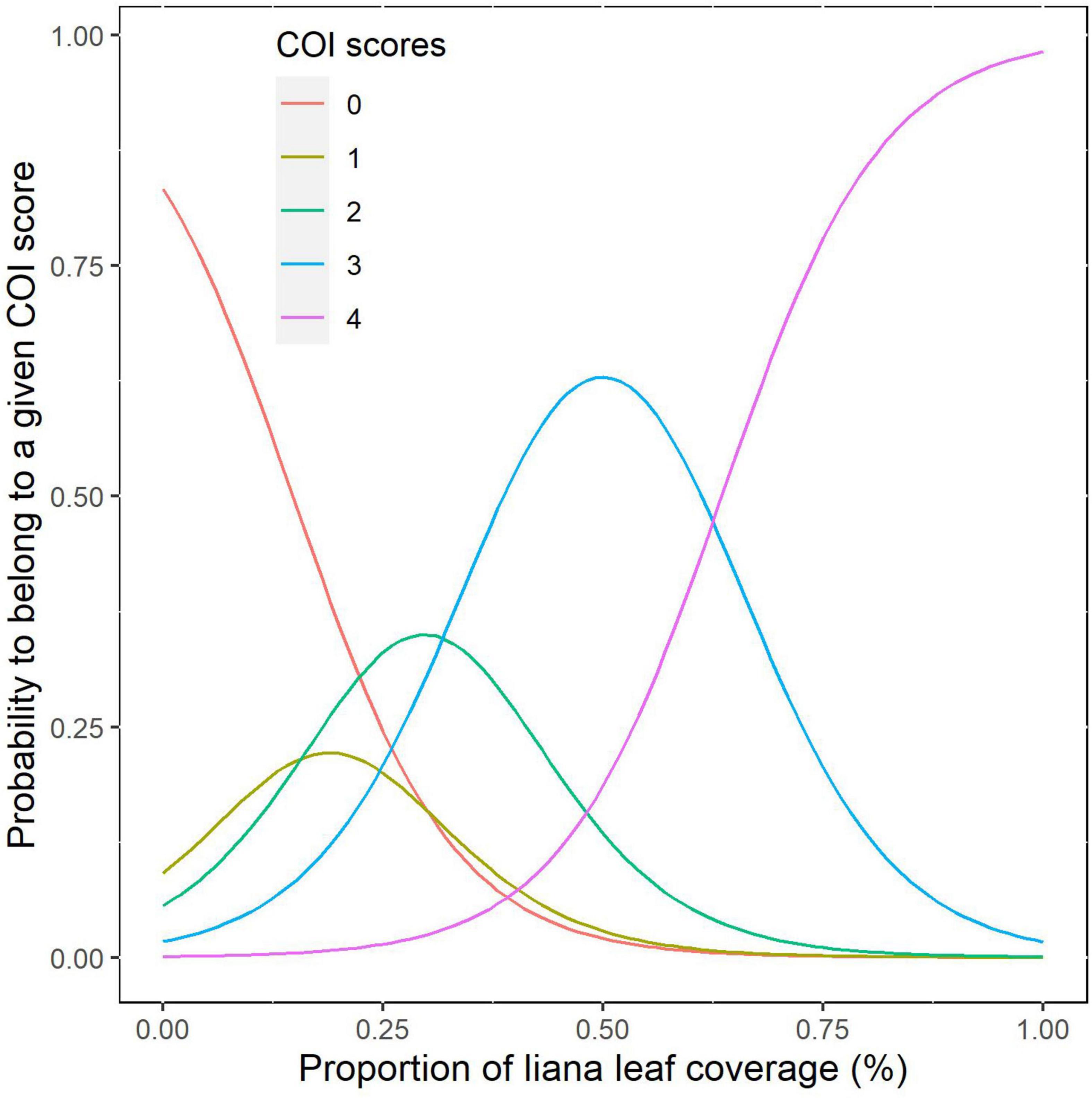
Figure 6. Probabilities that a tree belong to a given COI score determined from the ground according to the level of infestation measured with drone data.
Spectral Signatures of Lianas and Trees in Drone Images
The PCA performed on liana patches and liana-free tree crowns signal averages resulted in a first dominant axis (76% of the inertia) negatively correlated with signal intensity in all bands (Figure 7). The second axis, expressing 16% of the variance, tended to oppose invisible (near-infrared and red-edge) from visible bands. Despite a large overlap, we found that liana patches had significantly lower scores (higher DN and reflectance values) than tree crowns on the first axis (Wilcoxon’s W = 82,447, p < 0.0001) but exhibited no significant difference along the second axis (W = 141,893, p = 0.21). On the two PCA axes, the variance of the scores of individual lianas patches was significantly larger than that of trees (PCA Axis 1: F = 1.2, p = 0.05; PCA Axis 2: F = 1.6, p < 0.0001). Analyses conducted at the band level (Supplementary Figure 1) confirmed that liana patches tend to have greater DN and reflectance values than tree crowns in all the bands with a marked difference in the red and green domain compared to the other bands.
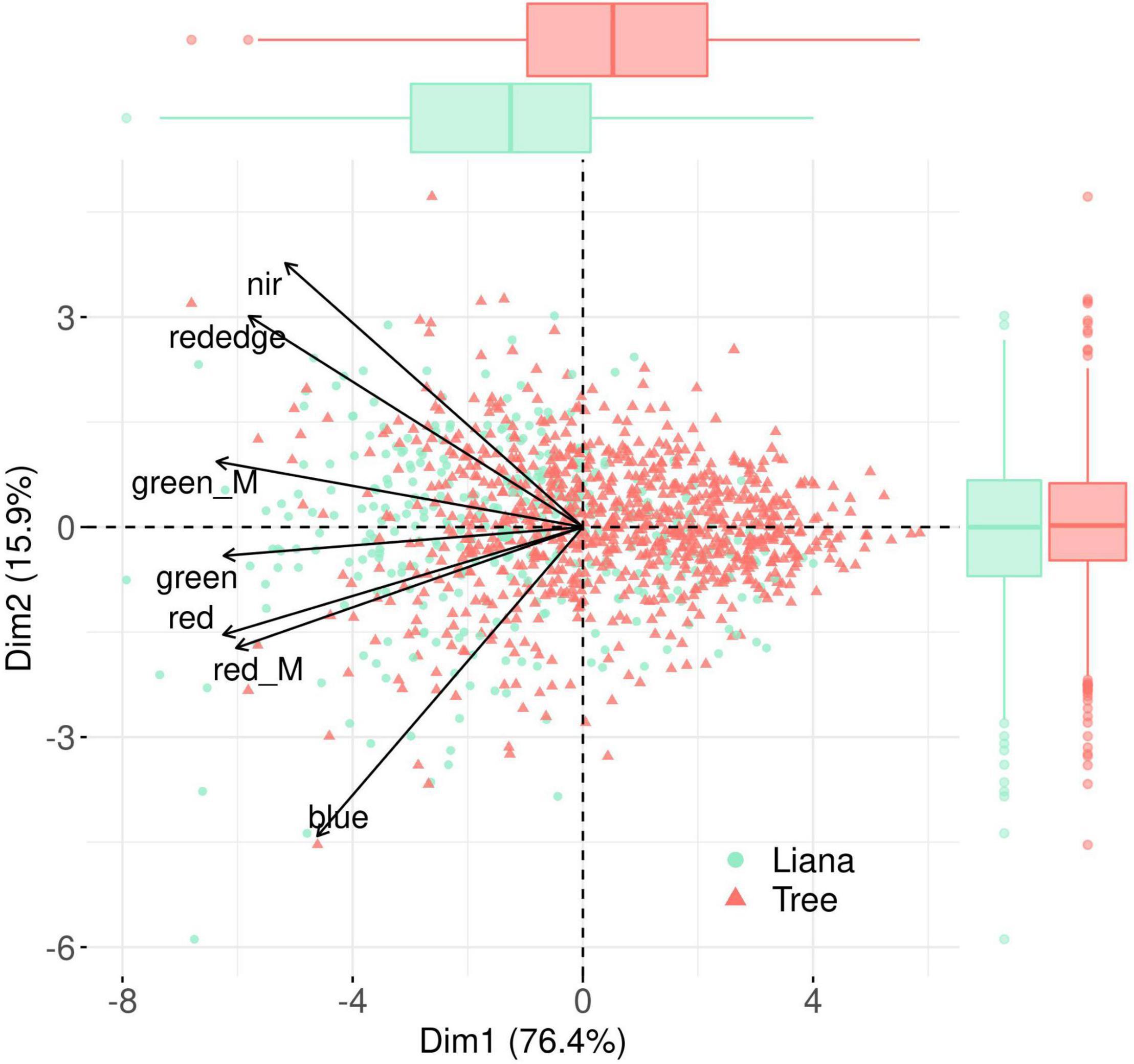
Figure 7. Plan 1–2 of a Principal Coordinate Analysis performed on RGB (red, green, blue) and multispectral (red_M, green_M, rededge, nir) bands. Tree and liana objects are represented in green and red, respectively, boxplots illustrate the differences between the scores of liana patches and tree crowns along the two first PCA axes.
Discussion
In this study, we used a combination of high-resolution drone images and field ground surveys to understand the links between below- and above-canopy liana distribution. We showed that the ability of lianas to reach the canopy depends mostly on their basal area and, to a less extent, on the total height of their host. Once the canopy is reached, lianas expand their leaves over tree crowns proportionally to their basal area, even though large variability in liana leaf cover exists for a given basal area. Some of this variability was explained here by a differential investment in leaf versus wood biomass with a larger leaf cover observed for liana species with lighter wood. We further showed that the widely used COI provides reliable information on the liana infested status but that, in our case, this index tends to provide lower estimates of infestation levels compared to drone-determined liana leaf coverage. Finally, our results suggest that liana patches display a stronger light reflectance than tree crowns in all the studied bands but that their spectral signatures largely overlap with that of trees. The mechanisms underlying these results and their implications are discussed below.
Liana Drone-Based Measurements Can Be Linked to Classical Ground Measurements, in Most Cases
Our results indicate that drone and ground data led to similar estimates of the number of liana-infested trees. The infestation status of tree crowns was indeed well predicted from ground data with 90% of the drone-based infested trees identified as such from a model using ground-derived estimates as predictors. This suggests that both methods are suited to study the number of tree crowns infested by lianas. We also found a significant correlation between classical ground measurements of lianas and drone-determined liana leaf coverage over tree crowns. This result is contradictory to that found by the only other study which has analyzed the link between above and below canopy liana measurements, using a canopy crane to determine liana leaf coverage over tree crowns (Cox et al., 2019). This study surprisingly indicated that liana leaf coverage was unrelated to liana stem count, basal area, aboveground biomass or tree diameter at breast height and the authors interpreted this negative result by the fact that lianas tended to mostly spread through neighboring tree crowns in their site. In our case, as discussed in the next section, we found strong significant correlations between the top-of-view liana leaf coverage and the ground-derived liana stem count, basal area, maximum diameter and tree height. This constitutes a reassuring result because liana infestation on trees has been extensively studied through ground measurements and estimations (Phillips et al., 2005; van der Heijden and Phillips, 2009; Ingwell et al., 2010; Wright et al., 2015). However, liana leaf cover was highly variable for a given range of ground-based measurements, keeping in mind that we studied the relationship between both measurements in log-transformed scale. This indicates that a large variance in aboveground competition between lianas and trees cannot be captured by ground measurements.
Some liana-infested trees were not identified as such from the ground [10 trees, category (ii)-c]. For one of these trees, the crown of which was heavily infested by lianas, our field notes indicated that it was impossible to follow any of the liana stems coming out or into the crown and measure them with certainty due to a poor visibility. For the other trees, a careful reanalysis of the drone images suggested that (i) five of them were infested by hemi-epiphytes or epiphytes that we did not detect during our fieldwork; (ii) four of them were trees with lianas coming from adjacent tree crowns, with probably remote rooting points of lianas that we missed from the ground. Once they reach the canopy, lianas can progress horizontally from crown to crown (Putz, 1984), sometimes up to relatively large distances (Ingwell et al., 2010), making the link between above and below-canopy views difficult to establish in some cases. Conversely, some trees identified with lianas climbing on them during the ground survey did not have liana leaf coverage over their crowns [17 trees; category (ii)-b]. Four of these trees were trees with lianas climbing on them but which did not reach the canopy either because they still were at an early stage of development or because they appeared to have fallen at the time of the inventory, due to tree branch falls. For the other trees, lianas appeared to reach the canopy from the ground but were still invisible in drone images. One potential explanation is that these lianas were either deciduous or dying at the time of the 2020 drone acquisition. However, for all trees of this category, we did not find any visible leaves on the older drone data, up to two years before (2018 and 2019; Figure 8). Even if these lianas are not currently in competition with trees for light, they still may compete with trees for below-ground resources and induce significant mechanical stress on host trees (Ingwell et al., 2010), decreasing tree growth and survival rates (Schnitzer et al., 2005). This well illustrates that if most of the drone and ground measurements can be paired with confidence, exhaustive links are unlikely to be made due to the complexity of some liana infestation pathways and that both measurements can bring complementary information on the impact of liana on forest dynamics.
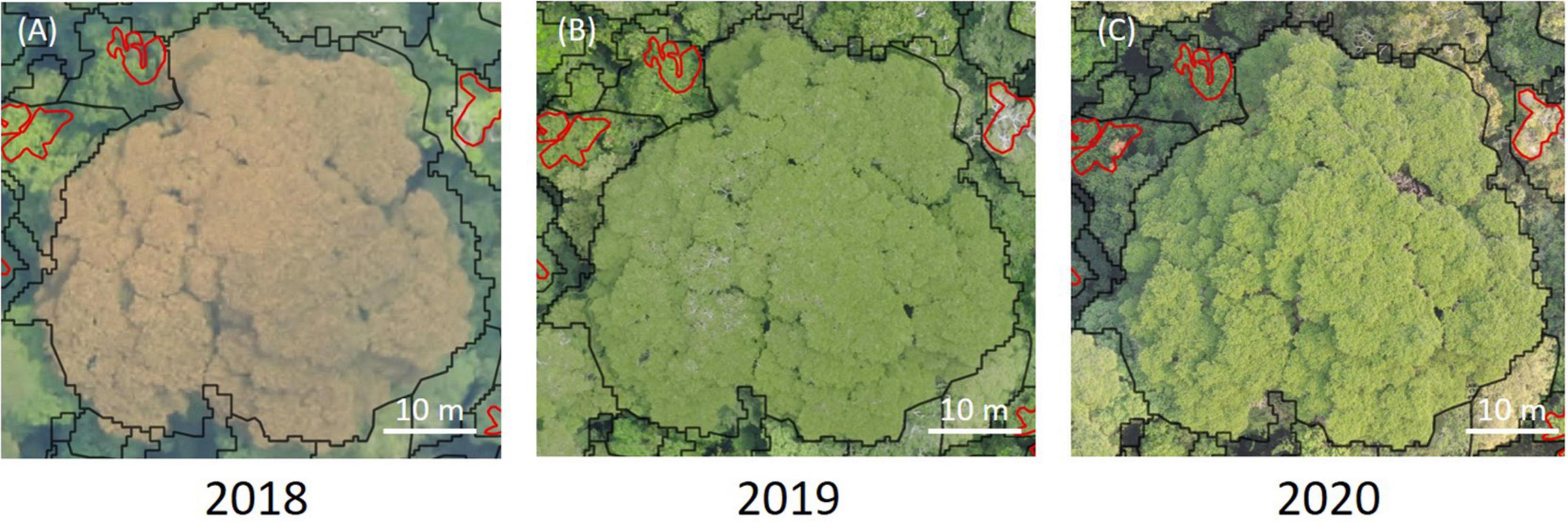
Figure 8. Example of a time series of photographs made by drone of a tree hosting a large liana of 6.3 cm dbh but that lacks any visible liana leaves in its crown. Black lines illustrate the tree crown limit and the red lines some delineated liana leaves belonging to other liana stems, as observed from the field. (A) Image at a resolution of 10 cm GSD from 2018. (B) Image at a resolution of 5 cm GSD from 2019. (C) Image at a resolution of 3 cm GSD from 2020.
The Allometry Between Liana Leaf Coverage and Ground Measurements Is Mediated by Liana Wood Density
We found that the probability of tree crowns to be infested by lianas depends strongly on the size of lianas and tree height. Larger lianas are developmentally older and have thus, on average, had more time to reach the canopy. However, the length-diameter allometry is known to be weak in lianas (Smith-Martin et al., 2019) with individuals of only 2 cm in diameter already reaching the canopy (Kurzel et al., 2006). Consistently, the total liana basal area was identified as the best predictor of tree crown infestation status (infested or not by lianas). Tree height also had a significant, yet smaller, effect on the probability of infestation of a tree crown in our statistical model. This result is consistent with previous studies where liana loads tended to be higher on smaller trees or lower canopy forests (Wright et al., 2015; Tymen et al., 2016). However, these results may originate from two non-exclusive mechanisms: lianas can more easily colonize small trees and/or liana infestation can lead to smaller tree heights by limiting their growth. By contrast, Marvin et al. (2016) found no strong associations between liana leaf coverage and canopy height but, as discussed in section “Lianas Display (Not Enough) Specific Spectral Signatures,” their remote sensing approach only enabled them to account for severe and high liana infestation levels, for which they had the lowest prediction error. Our findings also showed that if several ground-derived estimates can be successfully linked to liana leaf coverage, liana basal area was the best predictor, explaining 43% of the variance in the log-transformed space. This result is consistent with other studies that focused on the relationship between liana basal area and liana loads estimated from the ground (Ingwell et al., 2010; van der Heijden et al., 2010).
Our findings revealed that the large variability of liana leaf coverage for a given liana basal area can be partly explained by the functional composition of lianas. We indeed found a significant negative relationship between the drone-determined liana leaf coverage and mean wood density indicating that, for a given diameter, light-wooded lianas tend to invest in wider liana leaf coverages than hard-wooded lianas. As introduced earlier, variations of wood density are well-known to express different growth strategies in trees (Van Gelder et al., 2006; Chave et al., 2009; Baraloto et al., 2010; Poorter et al., 2010; Werden et al., 2018; Buckton et al., 2019) but the implication of wood density variation on liana ecology has been much less studied. Our results show that wood density significantly vary among taxa and that this variation entails ecological trade-offs among lianas, with greater biomass allocation to leaves for lianas with softer wood, as previously found for trees (Mensah et al., 2016). This pattern suggests that light-wooded lianas allocate more resources into leaves, and thus to light acquisition, at the expense of wood material, which may entail a greater mortality, as observed for trees (Chave et al., 2009). By contrast, no significant effect of liana climbing mechanism was found on liana leaf coverage. Some studies have shown that climbing mechanisms have an effect on liana recruitment and distribution across different forest structures (Dewalt et al., 2000; Bongers et al., 2020). Indeed, it is expected that climbing mechanism has a primary role in the early life of lianas and may thus constitute a central trait to understand the spatial (horizontal) liana distribution (Dias et al., 2021). For instance, Dewalt et al. (2000) showed that lianas with tendrils were more abundant in low canopy forests. The absence of an effect of climbing mechanisms on liana leaf coverage may be due to the fact that the early-life influence of climbing mechanisms on liana ecology may vanish when lianas reach the canopy. In this study, we divided our dataset between active and passive climbing mechanisms following Sperotto et al. (2020). Further investigations using different classifications, such as the one used by Dewalt et al. (2000), may provide different insights on the role of liana climbing mechanisms in liana structure and dynamics.
The Crown Occupancy Index Should Be Used Carefully
The COI is widely used to estimate liana leaf coverage over tree crowns and is now routinely used in international protocols such as in the RAINFOR network (Lopez-Gonzalez et al., 2011). We found a significant concordance between the ground-determined COI scores and the drone-determined liana leaf coverage. This supports that the COI is a quite reliable estimate of liana infestation over tree crowns, and is an alternative worth considering against time-consuming dendrometric liana measurements (van der Heijden et al., 2010), at least when substantial time is dedicated to this measurement, as done in the present study. However, we found that two thirds of the infested trees were attributed to a (generally neighboring) different infestation level from that determined by drone. This mismatch between categories raises questions about the reliability of the use of the COI to monitor liana infestation over time (Ingwell et al., 2010; Wright et al., 2015). One clear difference between the ground-determined COI and the extent of the drone-determined liana leaf coverage over tree crowns was the overestimation of infestation levels from the ground. This result is expected not to be specific to our study case because, from the ground, tree crowns are rarely entirely visible, and thus the proportion of the tree crown covered by liana leaves could easily be over-estimated (but see Waite et al. (2019)). Moreover, the results of our probit model supports the idea that dividing the COI in five infestation levels is too ambitious given the large variability of the ground estimates for a given drone-determined relative liana leaf coverage (Figure 6). Our model indeed suggests that separating, or aggregating, infestation levels in three categories, as done by Rutishauser et al. (2011), is a more conservative approach. To summarize, if the COI might be a reliable estimate of the spatial distribution of liana load on trees, it should be interpreted carefully when used to detect changes of liana infestation over time. For the latter objective, multi-temporal drone acquisition appears as a more reliable and accurate approach.
Lianas Display (Not Enough) Specific Spectral Signatures
The emergence of new remote sensing approaches and data, such as the use of high spatial and spectral resolution products, appeared as a promising way to monitor canopy liana infestation in space and time over large scales (Marvin et al., 2016; Chandler et al., 2021). A prerequisite to map liana distribution over tree crowns is that lianas should display a detectable signature over tree crowns (Visser et al., 2021). In our study site, our results suggest that liana patches exhibit significantly higher light reflectance than trees on average in all studied spectral bands, as found and predicted by Visser et al. (2021), who accounted for several parameters that were unavailable in our study, such as chemical and architectural leaf traits. Thus, the origin of these higher DN and reflectance values should be further investigated as it may be due to several non-exclusive origins, such as a higher leaf reflectance, lower gap fraction or flatter leaf angles, as shown in the neotropics by Visser et al. (2021). Note also that, in our study, we were unable to explicitly or accurately radiometrically calibrate our data due to a lack of reference data, such as those obtained with white panels or from ex situ physical measurements. To minimize this aspect, we focused on data acquired during a single flight or within 1 h, limiting the spatial extent of our analyses. Future works should thus provide more efforts to improve this calibration step to both enable the investigation of larger areas and to minimize the potential problems associated with illumination artifacts. Contrary to Chandler et al. (2021) and Visser et al. (2021), we did not find that the near-infrared region provided additional information for the discrimination of lianas versus trees (non-significant differences along the second PCA axis; Figure 7), suggesting that visible bands already contained most of the information, even if our studied bands were limited to only two invisible bands. Interestingly, the variances of the scores along the two first PCA axes were significantly higher than those of trees, suggesting a higher spectral diversity and/or a higher variability in leaf optical properties (e.g., leaf area in vertical profiles and leaf angles; see Visser et al., 2021) among lianas than among trees. Indeed, liana species have different leaf structure, pigment concentration or water content, resulting in a wide range of spectral responses (Sánchez-Azofeifa and Castro-Esau, 2006; Visser et al., 2021). Importantly, we found that the PCA scores of lianas versus trees largely overlapped, as acknowledged by previous studies (Chandler et al., 2021; Visser et al., 2021), potentially leading to a high rate of confusion in predictive models. It partly explains why reliable remote sensing predictions were obtained only for highly infested trees (>50% of infestation; Marvin et al., 2016; Chandler et al., 2021; Visser et al., 2021). If the probability of identifying liana pixels among a set of pixels is rather low, the probability that the spectral signature of a given liana does not overlap with that of its host tree is much higher, as illustrated in Figure 2. Thus, developing approaches that classify pixels based on their contrast with surrounding pixels, and accounting for tree crown segmentation information, might be more promising than adopting a pixel-based approach. The rapid development of deep learning approaches on very high-resolution images, that specifically account for texture, thus constitutes a promising avenue (Li et al., 2018). Another efficient strategy has been recently adopted by Visser et al. (2021), who combined observations, trait measurements and radiative transfer modeling to understand the promise and limitations of monitoring liana infestation from remote sensing data. Finally, with the low cost associated with drone acquisitions, it is today possible to acquire drone data at a high frequency level, typically on a monthly basis. Assuming that the leaf phenology of a given liana and its host tree are decoupled in time, repeated drone acquisitions have the potential to better discriminate liana leaf patches over tree crows using approaches accounting for intra-annual co-variability in spectral signals.
Conclusion
Combining drone-based and ground-based measurements has the potential to approximate the complex infestation pathways of lianas and their consequences on forest dynamics. Ground-based data on liana distributions and structure have been acquired for decades in tropical forests, providing key insights on liana ecology and on their impact on forest dynamics. However, as shown here, ground-based data approximate the above canopy distribution of lianas with important uncertainties, limiting our ability to understand tree-liana interactions in what is often considered as the most important forest layer for ecosystem dynamics. The emergence of easy-to-acquire drone-data can make a difference on this aspect, providing a very-high resolution view of liana distribution above the canopy, thus improving our ability to integrate explicitly light competition between lianas and trees in ecological models. Drone-based determinations also open new opportunities to study liana infestation over large extents and over time, e.g., accounting for leaf phenology when acquisitions are done with a high frequency. However, drone-data also have serious drawbacks, especially when not paired with ground data. Given that current automatic approaches are not yet fully satisfactory due to a large overlap in the spectral signatures of lianas and trees, delineating manually liana patches in drone data requires significant time and a certain level of expertise. Furthermore, as seen in this paper, the functional composition of lianas can mediate the dynamics of infestation, something that would not have been seen using only drone data. Thus, we here advocate that combining ground- and drone-based data have the potential to take a major step forward in liana ecology, especially if the dynamics of lianas through time and space are studied in relation to their diverse ecological strategies.
Data Availability Statement
The raw data supporting the conclusions of this article will be made available by the authors, without undue reservation.
Author Contributions
BK and MR-M: conceptualization and writing (original draft). BK, SP, YH, and MR-M: ground data collection. NB and EF: drone data collection and pre-processing. BK, MA-K, VR, and MR-M: formal analysis. BK and DG: wood trait measurements. All authors: writing (review and editing).
Funding
This project has received funding from the European Union’s Horizon 2020 Research and Innovation Programme under grant agreement no. 824074 in form of a prize awarded to BK. It also benefited from financial support from the French National Research Institute for Sustainable Development. The studied experimental site has been settled within the DynAfFor project (French Fund for the Global Environment; grant nos. CZZ1636.01D and CZZ1636.02D), the International Foundation for Science (grant no. D/5822-1), F.R.S-FNRS (grant no. 2017/v3/5/332 – IB/JN – 9500), Nature+ (asbl, Belgium) and the Republic of Congo (OGES-Congo). MR-M was supported by the DESSFOR ANR project (grant no. ANR-20-CE32-0010).
Conflict of Interest
The authors declare that the research was conducted in the absence of any commercial or financial relationships that could be construed as a potential conflict of interest.
Publisher’s Note
All claims expressed in this article are solely those of the authors and do not necessarily represent those of their affiliated organizations, or those of the publisher, the editors and the reviewers. Any product that may be evaluated in this article, or claim that may be made by its manufacturer, is not guaranteed or endorsed by the publisher.
Acknowledgments
We thank the CIB-Olam logging company that host and greatly facilitates the work conducted in this site. Last but not least, we are thankful to Isaac Zombo, Marien Fongo, Patchely Mbondo, Ati Ngouabi, Fred Ngouabi and Cyril Dzebou for their invaluable help during the field missions.
Supplementary Material
The Supplementary Material for this article can be found online at: https://www.frontiersin.org/articles/10.3389/ffgc.2022.803194/full#supplementary-material
Supplementary Figure S1 | Distribution of the mean DN and reflectance values per tree crown or liana patch for each RGB (red, gree, blue) and multispectral (red_M, green_M, rededge, nir) bands. Liana patches and tree crowns are represented in red and green boxplots respectively.
Footnotes
References
Asner, G. P., and Martin, R. E. (2012). Contrasting leaf chemical traits in tropical lianas and trees: implications for future forest composition. Ecol. Lett. 15, 1001–1007. doi: 10.1111/j.1461-0248.2012.01821.x
Aubry-Kientz, M., Dutrieux, R., Ferraz, A., Saatchi, S., Hamraz, H., Williams, J., et al. (2019). A comparative assessment of the performance of individual tree crowns delineation algorithms from ALS Data in tropical forests. Remote Sens. 11:1086. doi: 10.3390/rs11091086
Avalos, G., Mulkey, S. S., and Kitajima, K. (1999). Leaf optical properties of trees and lianas in the outer canopy of a tropical Dry Forest1. Biotropica 31, 517–520. doi: 10.1111/j.1744-7429.1999.tb00395.x
Baraloto, C., Timothy Paine, C. E., Poorter, L., Beauchene, J., Bonal, D., Domenach, A.-M., et al. (2010). Decoupled leaf and stem economics in rain forest trees: decoupled leaf and stem economics spectra. Ecol. Lett. 13, 1338–1347. doi: 10.1111/j.1461-0248.2010.01517.x
Birouste, M., Zamora-Ledezma, E., Bossard, C., Pérez-Ramos, I. M., and Roumet, C. (2014). Measurement of fine root tissue density: a comparison of three methods reveals the potential of root dry matter content. Plant Soil 374, 299–313.
Bongers, F., Ewango, C. E. N., Sande, M. T., and Poorter, L. (2020). Liana species decline in Congo basin contrasts with global patterns. Ecology 101:e03004. doi: 10.1002/ecy.3004
Buckton, G., Cheesman, A. W., Munksgaard, N. C., Wurster, C. M., Liddell, M. J., and Cernusak, L. A. (2019). Functional traits of lianas in an Australian lowland rainforest align with post-disturbance rather than dry season advantage. Austral Ecol. 44, 983–994. doi: 10.1111/aec.12764
Castro-Esau, K. (2004). Discrimination of lianas and trees with leaf-level hyperspectral data. Remote Sens. Environ. 90, 353–372. doi: 10.1016/j.rse.2004.01.013
Chandler, C. J., Van Der Heijden, G. M., Boyd, D. S., Cutler, M. E., Costa, H., Nilus, R., et al. (2021). Remote sensing liana infestation in an aseasonal tropical forest: addressing mismatch in spatial units of analyses. Remote Sens. Ecol. Conserv. 7, 397–410.
Chave, J., Coomes, D., Jansen, S., Lewis, S. L., Swenson, N. G., and Zanne, A. E. (2009). Towards a worldwide wood economics spectrum. Ecol. Lett. 12, 351–366. doi: 10.1111/j.1461-0248.2009.01285.x
Clark, D. B., and Clark, D. A. (1990). Distribution and effects on tree growth of Lianas and Woody Hemiepiphytes in a Costa Rican Tropical Wet Forest. J. Trop. Ecol. 6, 321–331. doi: 10.1017/s0266467400004570
Collins, C. G., Wright, S. J., and Wurzburger, N. (2016). Root and leaf traits reflect distinct resource acquisition strategies in tropical lianas and trees. Oecologia 180, 1037–1047. doi: 10.1007/s00442-015-3410-7
Cox, C. J., Edwards, W., Campbell, M. J., Laurance, W. F., and Laurance, S. G. W. (2019). Liana cover in the canopies of rainforest trees is not predicted by local ground-based measures. Austral Ecol. 44, 759–767. doi: 10.1111/aec.12746
Dalling, J. W., Schnitzer, S. A., Baldeck, C., Harms, K. E., John, R., Mangan, S. A., et al. (2012). Resource-based habitat associations in a neotropical liana community: habitat associations of lianas. J. Ecol. 100, 1174–1182. doi: 10.1111/j.1365-2745.2012.01989.x
De Deurwaerder, H., Hervé-Fernández, P., Stahl, C., Burban, B., Petronelli, P., Hoffman, B., et al. (2018). Liana and tree below-ground water competition—Evidence for water resource partitioning during the dry season. Tree Physiol. 38, 1071–1083. doi: 10.1093/treephys/tpy002
Dewalt, S. J., Schnitzer, S. A., and Denslow, J. S. (2000). Density and diversity of lianas along a chronosequence in a central Panamanian lowland forest. J. Trop. Ecol. 16, 1–19.
Dias, A. S., Oliveira, R., Martins, F., Bongers, F., Anten, N., and Sterck, F. (2021). Climbing Mechanisms as a Central Trait to Understand the Ecology of Lianas—A Global Synthesis. Available online at: https://d197for5662m48.cloudfront.net/documents/publicationstatus/62374/preprint_pdf/6068759b4d003f91e6a0bf0cfb4c9f6f.pdf (accessed September 05, 2021).
Féret, J.-B., and Asner, G. P. (2012). Tree species discrimination in tropical forests using airborne imaging spectroscopy. IEEE Trans. Geosci. Remote Sens. 51, 73–84. doi: 10.1109/tgrs.2012.2199323
Ferraz, A., Bretar, F., Jacquemoud, S., Gonçalves, G., Pereira, L., Tomé, M., et al. (2012). 3-D mapping of a multi-layered Mediterranean forest using ALS data. Remote Sens. Environ. 121, 210–223. doi: 10.1016/j.rse.2012.01.020
Fox, J., Muenchen, R., and Putler, D. (2020). RcmdrMisc: R Commander Miscellaneous Functions (2.7-0)[Computer software]. Available online at: https://cran.r-project.org/web/packages/RcmdrMisc/RcmdrMisc.pdf (accessed September 05, 2021).
Freycon, V. (2014). Caractérisation des sols de Loundoungou et de Mokabi (Congo). Montpellier: CIRAD.
Gentry, A. H., Putz, F. E., and Mooney, H. A. (1991). The Biology of Vines. Cambridge: Cambridge University Press.
Gerwing, J. J., Schnitzer, S. A., Burnham, R. J., Bongers, F., Chave, J., DeWalt, S. J., et al. (2006). A standard protocol for liana censuses 1: short communications. Biotropica 38, 256–261. doi: 10.1111/j.1744-7429.2006.00134.x
Hegarty, E. E. (1991). “Vine-host interactions,” in The Biology of Vines, eds F. E. Putz and H. A. Mooney (Cambridge: Cambridge University Press),357–375.
Hegarty, E. E., and Caballé, G. (1991). “Distribution and abundance of vines in forest communities,” in The Biology of Vines, eds F. E. Putz and H. A. Mooney (New York, NY: Cambridge University Press), 313–334.
Hijmans, R. J. (2021). Geographic Data Analysis and Modeling [R package raster version 3.4-10]. Available online at: http://cran.stat.unipd.it/web/packages/raster/ (accessed June 9, 2021).
Ingwell, L. L., Joseph Wright, S., Becklund, K. K., Hubbell, S. P., and Schnitzer, S. A. (2010). The impact of lianas on 10 years of tree growth and mortality on Barro Colorado Island, Panama: impact of lianas on 10 years of tree growth and mortality. J. Ecol. 98, 879–887. doi: 10.1111/j.1365-2745.2010.01676.x
Isenburg, M. (2014). LAStools-Efficient LiDAR Processing Software. Available online at: Lastools.Org (accessed October 10, 2017).
Isnard, S., and Silk, W. K. (2009). Moving with climbing plants from Charles Darwin’s time into the 21st century. Am. J. Bot. 96, 1205–1221. doi: 10.3732/ajb.0900045
Kurzel, B. P., Schnitzer, S. A., and Carson, W. P. (2006). Predicting liana crown location from stem diameter in three panamanian lowland forests 1. Biotropica 38, 262–266. doi: 10.1111/j.1744-7429.2006.00135.x
Laurance, W. F., Andrade, A. S., Magrach, A., Camargo, J. L. C., Valsko, J. J., Campbell, M., et al. (2014). Long-term changes in liana abundance and forest dynamics in undisturbed Amazonian forests. Ecology 95, 1604–1611. doi: 10.1890/13-1571.1
Li, W., Campos-Vargas, C., Marzahn, P., and Sanchez-Azofeifa, A. (2018). On the estimation of tree mortality and liana infestation using a deep self-encoding network. Int. J. Appl. Earth Observ. Geoinform. 73, 1–13. doi: 10.1016/j.jag.2018.05.025
Lopez-Gonzalez, G., Lewis, S. L., Burkitt, M., and Phillips, O. L. (2011). ForestPlots. Net: A Web Application and Research Tool to Manage and Analyse Tropical Forest Plot Data. New York, NY: Wiley Online Library.
Marvin, D. C., Asner, G. P., and Schnitzer, S. A. (2016). Liana canopy cover mapped throughout a tropical forest with high-fidelity imaging spectroscopy. Remote Sens. Environ. 176, 98–106. doi: 10.1016/j.rse.2015.12.028
Medina-Vega, J. A., Bongers, F., Schnitzer, S. A., and Sterck, F. J. (2021). Lianas explore the forest canopy more effectively than trees under drier conditions. Funct. Ecol. 35, 318–329.
Melzer, B., Steinbrecher, T., Seidel, R., Kraft, O., Schwaiger, R., and Speck, T. (2010). The attachment strategy of English ivy: a complex mechanism acting on several hierarchical levels. J. R. Soc. Interface 7, 1383–1389. doi: 10.1098/rsif.2010.0140
Mensah, S., Kakaï, R. G., and Seifert, T. (2016). Patterns of biomass allocation between foliage and woody structure: the effects of tree size and specific functional traits. Ann. For. Res. 59, 49–60.
Meunier, F., Krishna Moorthy, S. M., De Deurwaerder, H. P. T., Kreus, R., Van den Bulcke, J., Lehnebach, R., et al. (2020). Within-site variability of liana wood anatomical traits: a case study in Laussat, French Guiana. Forests 11:523. doi: 10.3390/f11050523
Pebesma, E., Bivand, R., Racine, E., Sumner, M., Cook, I., and Keitt, T. (2018). sf: Simple Features for R. R Package Version 0.6-1. Available online at: https://cran.r-project.org/web/packages/sf/index.html (accessed September 05, 2021).
Phillips, O. L., Vásquez Martínez, R., Monteagudo Mendoza, A., Baker, T. R., and Núñez Vargas, P. (2005). Large lianas as hyperdynamic elements of the tropical forest canopy. Ecology 86, 1250–1258.
Piboule, A., Krebs, M., Esclatine, L., and Hervé, J. C. (2013). “Computree: a collaborative platform for use of terrestrial lidar in dendrometry,” in Proceedings of the International IUFRO Conference MeMoWood, Nancy, 1–4.
Picard, N., and Gourlet-Fleury, S. (2008). Manuel de Référence Pour L’installation de Dispositifs Permanents en Forêt de Production Dans le Bassin du Congo. Yaoundé: COMIFAC.
Poorter, L., McDonald, I., Alarcón, A., Fichtler, E., Licona, J.-C., Peña-Claros, M., et al. (2010). The importance of wood traits and hydraulic conductance for the performance and life history strategies of 42 rainforest tree species. New Phytol. 185, 481–492. doi: 10.1111/j.1469-8137.2009.03092.x
Putz, F. E. (1984). The Natural History of Lianas on Barro Colorado Island, Panama. Ecology 65, 1713–1724. doi: 10.2307/1937767
Putz, F. E., and Holbrook, N. M. (1991). “Biomechanical studies of vines,” in The Biology of Vines, eds F. E. Putz and H. A. Mooney (Cambridge: Cambridge University Press), 73–97.
R Core Team (2020). R: A Language and Environment for Statistical Computing. R Package Version 4.0.3. Available online at: https://www.R-project.org/ (accessed September 05, 2021).
Réjou-Méchain, M., Mortier, F., Bastin, J.-F., Cornu, G., Barbier, N., Bayol, N., et al. (2021). Unveiling African rainforest composition and vulnerability to global change. Nature 593, 90–94. doi: 10.1038/s41586-021-03483-6
Rocchini, D., Boyd, D. S., Féret, J.-B., Foody, G. M., He, K. S., Lausch, A., et al. (2016). Satellite remote sensing to monitor species diversity: Potential and pitfalls. Remote Sens. Ecol. Conserv. 2, 25–36. doi: 10.1002/rse2.9
Roussel, J.-R., Auty, D., Coops, N. C., Tompalski, P., Goodbody, T. R., Meador, A. S., et al. (2020). LidR: an R package for analysis of Airborne Laser Scanning (ALS) data. Remote Sens. Environ. 251:112061.
Rowe, N. P., and Speck, T. (2015). “Biomechanics of lianas,” in The Ecology of Lianas, eds S. A. Schnitzer, F. Bongers, R. J. Burnham, and F. E. Putz (Chichester: John Wiley & Sons, Ltd), 323–341.
Rutishauser, E., Barthélémy, D., Blanc, L., and Eric-André, N. (2011). Crown fragmentation assessment in tropical trees: method, insights and perspectives. For. Ecol. Manage. 261, 400–407.
Sánchez-Azofeifa, G. A., and Castro-Esau, K. (2006). Canopy observations on the hyperspectral properties of a community of tropical dry forest lianas and their host trees. Int. J. Remote Sens. 27, 2101–2109. doi: 10.1080/01431160500444749
Schnitzer, S. A., and Carson, W. P. (2010). Lianas suppress tree regeneration and diversity in treefall gaps: lianas suppress tree diversity. Ecol. Lett. 13, 849–857. doi: 10.1111/j.1461-0248.2010.01480.x
Schnitzer, S. A., Kuzee, M. E., and Bongers, F. (2005). Disentangling above-and below-ground competition between lianas and trees in a tropical forest. J. Ecol. 93, 1115–1125.
Schnitzer, S. A., Rutishauser, S., and Aguilar, S. (2008). Supplemental protocol for liana censuses. For. Ecol. Manage. 255, 1044–1049. doi: 10.1016/j.foreco.2007.10.012
Signorell, A., Aho, K., Alfons, A., Anderegg, N., Aragon, T., and Arppe, A. (2021). DescTools: Tools for descriptive statistics. R Package Version 0.99.42, 28, 17. Available online at: https://cran.r-project.org/web/packages/DescTools/index.html (accessed September 05, 2021).
Smith-Martin, C. M., Bastos, C. L., Lopez, O. R., Powers, J. S., and Schnitzer, S. A. (2019). Effects of dry-season irrigation on leaf physiology and biomass allocation in tropical lianas and trees. Ecology 100:e02827. doi: 10.1002/ecy.2827
Sperotto, P., Acevedo-Rodríguez, P., Vasconcelos, T. N., and Roque, N. (2020). Towards a standardization of terminology of the climbing habit in plants. Bot. Rev. 86, 180–210.
Tymen, B., Réjou-Méchain, M., Dalling, J. W., Fauset, S., Feldpausch, T. R., Norden, N., et al. (2016). Evidence for arrested succession in a liana-infested Amazonian forest. J. Ecol. 104, 149–159. doi: 10.1111/1365-2745.12504
van der Heijden, G. M., Feldpausch, T. R., de la Fuente Herrero, A., van der Velden, N. K., and Phillips, O. L. (2010). Calibrating the liana crown occupancy index in Amazonian forests. For. Ecol. Manage. 260, 549–555. doi: 10.1016/j.foreco.2010.05.011
van der Heijden, G. M., Schnitzer, S. A., Powers, J. S., and Phillips, O. L. (2013). Liana impacts on carbon cycling, storage and sequestration in tropical forests. Biotropica 45, 682–692.
van der Heijden, G. M. F., and Phillips, O. L. (2009). Liana infestation impacts tree growth in a lowland tropical moist forest. Biogeosciences 6, 2217–2226. doi: 10.5194/bg-6-2217-2009
van der Heijden, G. M. F., Powers, J. S., and Schnitzer, S. A. (2015). Lianas reduce carbon accumulation and storage in tropical forests. Proc. Natl. Acad. Sci. U.S.A. 112, 13267–13271. doi: 10.1073/pnas.1504869112
Van Gelder, H. A., Poorter, L., and Sterck, F. J. (2006). Wood mechanics, allometry, and life-history variation in a tropical rain forest tree community. New Phytol. 171, 367–378. doi: 10.1111/j.1469-8137.2006.01757.x
Venables, W. N., and Ripley, B. D. (2002). Modern Applied Statistics with S, 4th Edn. New York, NY: Springer.
Villanueva, R. A. M., Chen, Z. J., and Wickham, H. (2016). ggplot2: Elegant Graphics for Data Analysis Using the Grammar of Graphics. New York, NY: Springer-Verlag.
Visser, M. D., Detto, M., Meunier, F., Wu, J., Bongalov, B., Coomes, D., et al. (2021). Why can we detect lianas from space? bioRxiv [Preprint]. doi: 10.1101/2021.09.30.462145
Visser, M. D., Schnitzer, S. A., Muller-Landau, H. C., Jongejans, E., de Kroon, H., Comita, L. S., et al. (2018). Tree species vary widely in their tolerance for liana infestation: a case study of differential host response to generalist parasites. J. Ecol. 106, 781–794. doi: 10.1111/1365-2745.12815
Waite, C. E., Heijden, G. M. F., Field, R., and Boyd, D. S. (2019). A view from above: unmanned aerial vehicles (UAV s) provide a new tool for assessing liana infestation in tropical forest canopies. J. Appl. Ecol. 56, 902–912. doi: 10.1111/1365-2664.13318
Werden, L. K., Waring, B. G., Smith-Martin, C. M., and Powers, J. S. (2018). Tropical dry forest trees and lianas differ in leaf economic spectrum traits but have overlapping water-use strategies. Tree Physiol. 38, 517–530. doi: 10.1093/treephys/tpx135
Wickham, H., Francois, R., Henry, L., and Müller, K. (2021). dplyr: A Grammar of Data Manipulation. R Package Version 1.0.5, 3. Available online at: https://cran.r-project.org/web/packages/dplyr/index.html (accessed September 05, 2021).
Keywords: Central Africa, woody vines, strategy, canopy, competition, UAV systems, remote sensing
Citation: Kaçamak B, Barbier N, Aubry-Kientz M, Forni E, Gourlet-Fleury S, Guibal D, Loumeto J-J, Pollet S, Rossi V, Rowe N, van Hoef Y and Réjou-Méchain M (2022) Linking Drone and Ground-Based Liana Measurements in a Congolese Forest. Front. For. Glob. Change 5:803194. doi: 10.3389/ffgc.2022.803194
Received: 27 October 2021; Accepted: 10 February 2022;
Published: 11 March 2022.
Edited by:
Félicien Meunier, Ghent University, BelgiumReviewed by:
Sruthi M. Krishna Moorthy, University of Maryland, College Park, United StatesMargaret Kalacska, McGill University, Canada
J. Antonio Guzmán Q. University of Minnesota Twin Cities, United States
Copyright © 2022 Kaçamak, Barbier, Aubry-Kientz, Forni, Gourlet-Fleury, Guibal, Loumeto, Pollet, Rossi, Rowe, van Hoef and Réjou-Méchain. This is an open-access article distributed under the terms of the Creative Commons Attribution License (CC BY). The use, distribution or reproduction in other forums is permitted, provided the original author(s) and the copyright owner(s) are credited and that the original publication in this journal is cited, in accordance with accepted academic practice. No use, distribution or reproduction is permitted which does not comply with these terms.
*Correspondence: Begüm Kaçamak, YmthY2FtYWtAZ21haWwuY29t; Maxime Réjou-Méchain, bWF4aW1lLnJlam91QGlyZC5mcg==