- 1Institute of Ecology, Evolution and Diversity, Goethe-University Frankfurt, Frankfurt, Germany
- 2Senckenberg Biodiversity and Climate Research Centre (SBiK-F), Frankfurt, Germany
- 3LOEWE Centre for Translational Biodiversity Genomics (TBG), Frankfurt, Germany
- 4Department of Biology, University of Padova, Padua, Italy
Tree bark constitutes an ideal habitat for microbial communities, because it is a stable substrate, rich in micro-niches. Bacteria, fungi, and terrestrial microalgae together form microbial communities, which in turn support more bark-associated organisms, such as mosses, lichens, and invertebrates, thus contributing to forest biodiversity. We have a limited understanding of the diversity and biotic interactions of the bark-associated microbiome, as investigations have mainly focused on agriculturally relevant systems and on single taxonomic groups. Here we implemented a multi-kingdom metabarcoding approach to analyze diversity and community structure of the green algal, bacterial, and fungal components of the bark-associated microbial communities of beech, the most common broadleaved tree of Central European forests. We identified the most abundant taxa, hub taxa, and co-occurring taxa. We found that tree size (as a proxy for age) is an important driver of community assembly, suggesting that environmental filtering leads to less diverse fungal and algal communities over time. Conversely, forest management intensity had negligible effects on microbial communities on bark. Our study suggests the presence of undescribed, yet ecologically meaningful taxa, especially in the fungi, and highlights the importance of bark surfaces as a reservoir of microbial diversity. Our results constitute a first, essential step toward an integrated framework for understanding microbial community assembly processes on bark surfaces, an understudied habitat and neglected component of terrestrial biodiversity. Finally, we propose a cost-effective sampling strategy to study bark-associated microbial communities across large spatial or environmental scales.
Introduction
The aboveground surfaces of plants are ideal substrates for microbial colonization. The bark surface [or dermosphere; Lambais et al. (2014)], in particular, is one of such important aboveground substrates in forests. The bark provides a range of microhabitats that promote colonization of microbial communities with varied ecologies (Whitmore, 1963). On the one hand, microsites such as holes, cracks, and lenticels retain humidity and nutrients, thus constituting stable microhabitats suitable for slow-growing, stress-sensitive microbes. On the other hand, the exposed surfaces of the bark may harbor more stress-resistant microbial communities that can cope with environmental challenges (Vorholt, 2012; Aguirre-von-Wobeser et al., 2021), such as low nutrient availability, increased exposure to light, fluctuating moisture conditions and desiccation (Lindow and Brandl, 2003; Vorholt, 2012; Leff et al., 2015), and presence of compounds that are resistant to microbial degradation (e.g., suberin), or that directly inhibit microbial growth (Baldrian, 2017).
Compared to other aboveground components, such as leaves, branches or fruits, that undergo seasonal and diurnal changes (Vitulo et al., 2019), bark represents a stable, long-lived substrate that supports microbial colonization (Leff et al., 2015). Further, the bark surface is often screened from excessive precipitation- and/or UV radiation by the tree canopy and changes slowly during development over several years (Whitmore, 1963). A number of studies have investigated the bark-associated microbial diversity, especially for fungi and bacteria, in various systems, e.g., grapevine plants (Martins et al., 2013; Arrigoni et al., 2018), bark beetle-infested spruce (Strid et al., 2014), Ginkgo (Leff et al., 2015) and avocado trees (Aguirre-von-Wobeser et al., 2021). These studies report that the tree bark supports microbial communities that are often distinct from spatially-close substrates like leaves and roots (Martins et al., 2013; Leff et al., 2015; Arrigoni et al., 2018), indicating niche differentiation and a clearly structured habitat (Aguirre-von-Wobeser et al., 2021). Furthermore, the dermosphere constitutes a reservoir for microbial diversity (Arrigoni et al., 2018; Hagge et al., 2019; Kobayashi and Aoyagi, 2019), potentially harboring undiscovered specialist taxa (Aschenbrenner et al., 2017), and taxa that facilitate the colonization of other epiphytes, including lichens (Aschenbrenner et al., 2017). The microbial communities on tree bark, and the biofilm which they form, can indeed be considered the basis of a food web that supports photosynthetic epiphytes (e.g., mosses and lichens), as well as a diverse microfauna (Andre, 1985). With an estimated more than 3 trillion trees in the world (Crowther et al., 2015), bark communities could thus be particularly important reservoirs of biological diversity. However, bark is a poorly explored habitat with respect to microbial diversity and community structure, compared to other substrates such as the phyllosphere and rhizosphere.
Biological and environmental factors driving diversity and community assembly in bark-associated epiphytes have been linked to forestry management, e.g., management intensity (Boch et al., 2021), forest homogeneity (Lamit et al., 2015), deadwood abundance (Boch et al., 2021), and tree age. For the latter, higher epiphyte diversities have been linked to the availability of large, old-growth trees (Aude and Poulsen, 2000; Nascimbene et al., 2013; Boch et al., 2021), probably because of higher niche partitioning in older trees (Łubek et al., 2020). At smaller spatial scales, abiotic drivers of bark-associated diversity and community structure include ultraviolet radiation, water shortages and correlated desiccation, and poor nutrient availability (Lindow and Brandl, 2003; Vorholt, 2012; Leff et al., 2015), while biotic drivers include host traits, such as maturity of the substrate and host genotype (Arrigoni et al., 2018, 2020). Community composition is therefore, to some degree, host specific. A few studies showed that the trends observed for macroepiphytes (e.g., bryophytes, lichens) or components of the phyllosphere also appear in bark-associated microbes (e.g., Vorholt, 2012; Arrigoni et al., 2020). However, our understanding of the factors shaping the different components of the highly diverse bark-associated microbial communities is still limited. Most of the studies focus on non-natural, commercially driven ecosystems like orchards or vineyards (Martins et al., 2013; Arrigoni et al., 2018) and are often conducted over small spatial scales with small sample sizes (e.g., Leff et al., 2015). Lastly, the focus often lies on only a single group of microorganisms, with bacteria and fungi far outweighing terrestrial algae (Aschenbrenner et al., 2017; Petrolli et al., 2021). Integrative sampling of major microbial contributors over regional or potentially even global scales can help identifying not only the diversity of microorganisms but also potential cooperative and competitive interactions among them. Revealing the diversity and structure of these rather unique microbial communities is essential to predict their responses in a changing environment. Furthermore, considering the importance of fungi, bacteria and algae to ecosystem nutrient and energy budgets in terrestrial habitats, gaining information on the bark-associated microbial communities and their dynamics is essential and directly relevant for ecosystem service assessment.
In this study we present one of the first integrated investigations of the bark microbiome in temperate forests. Here we use the term microbiome following the definition by Berg et al. (2020). Specifically, we study the three main components of the bark microbiome, i.e., green algae, bacteria and fungi. We sampled bark surfaces in forests under different management regimes, ranging from highly-managed stands to relatively undisturbed sites in the core zone of a national park. We used metabarcoding to analyze microbial diversity, community structure and species interactions from the tree to the landscape level. Specifically, we asked the following questions: (i) What is the microbial diversity found on the bark of the most common broadleaved tree in central Europe (Fagus sylvatica)?, (ii) Which species co-occur and who are the main players in the identified ecological modules?, (iii) Which factors, i.e., management intensity and tree-size classes (as a proxy for tree age), affect the bark-associated microbiome, both at tree and landscape level?
The comparison of diversities among trees of different size classes within a spatially-explicit framework allowed us to test for the effects of sampling design on the estimation of microbial diversity. This information is essential for further, larger scale sampling campaigns.
Materials and Methods
Study Site and Sampling
Sampling sites are situated within the central European region of Hainich-Dün (Thuringia, Germany), one of the three regions of the Biodiversity Exploratories (Fischer et al., 2010). The Hainich-Dün region is characterized by soils stemming from calcareous bedrock, an annual rainfall between 500–800 mm, and a mean temperature of 6.5–8°C at an elevation of 285–550 m above sea level (Fischer et al., 2010).
Sample collection took place in autumn between the 13th and 15th of October 2020. We chose a subset of 16 out of the established 50 experimental plots (Fischer et al., 2010), sampling a subplot of 20 m × 20 m within the 100 m × 100 m experimental plots. These plots were chosen to represent two regimes of land-use intensity, namely a high and a low intensity forest management (eight plots each), according to the Forest Management Index (ForMI, high > 1, low < 1). This is an index combining measures of harvested stem volume, occurrence of non-natural species and deadwood stemming from harvest (Kahl and Bauhus, 2014). The plots had an average stand density of 485 trees/ha (min = 152 trees/ha, max = 1,830 trees/ha). We defined three size classes: large [i.e., > 30 cm diameter at breast height (DBH)], medium (15–30 cm DBH) and small (5–15 cm DBH). We sampled two trees per size class, resulting in a total of 96 samples. When one tree-size class was not available (three plots), we sampled more trees of the other size classes depending on which was highly abundant in the direct vicinity as judged in the field. Within each plot we recorded the spatial position of the trees relative to each other by measuring distance (m) and azimuth (degrees) from the nearest sampled tree.
We collected microbial bark surface communities using individually wrapped sterile nylon-flocked medical swabs with a 30 mm breakpoint, typically used for medical specimen collection (FLOQSwabs™, Copan, Brescia, Italy). The breakpoint mechanism minimizes the possibility of contamination when transferring the swab into the Eppendorf tube. Prior to collection the bark was moisturized with deionized water to mobilize the surface biofilms. Then the tree was swabbed at approximately 150 cm height in a 3 cm-wide band around the trunk, rolling the swab and moving it up and down while applying gentle pressure. While swabbing, we took care to include smooth surfaces as well as cracks and crevices in the bark, to ensure a good representation of micro-habitats. If present, large patches (>10 cm) of bryophytic epiphytes were excluded. Conspicuous, larger lichen thalli were not present in the swabbed areas, however, small lichen thalli/propagules were included during the swabbing. The swab head was broken off into Eppendorf tubes pre-filled with 750 μl Nucleic Acid Preservation (NAP) buffer (Camacho-Sanchez et al., 2013). Tubes were immediately placed in styrofoam boxes with ice and the samples were subsequently stored at 4°C until DNA extraction.
DNA Extraction
Prior to DNA extraction we added 750 μl ice-cold phosphate-buffered saline (PBS) into the Eppendorf tube and centrifuged the sample for 15 min at 6,000 × g as recommended by Menke et al. (2017). The supernatant was then discarded without disturbing the swab head or pellet. DNA was extracted using the Quick-DNA Fecal/Soil Microbe Microprep kit (Zymo Research Europe GmbH, Freiburg, Germany). Initial tissue lysis was achieved through mechanical disruption by bead beating, using the beads included in the extraction kit. We modified the kit protocol by directly adding the beads and bead-beating buffer into the tube containing the pellet and swab and shaking for a total of 6 min (SpeedMill PLUS, Analytik Jena, Jena, Germany). In the later steps we followed the manufacturer’s protocol, using DNAse-free water as elution buffer. We included six extraction blanks as contamination controls, that were sequenced as well. Extraction blanks consisted of one unused swab, unpacked and transferred to the NAP buffer in the field and subsequently treated in the same way as regular samples. DNA extracts were frozen at −20°C until PCR.
PCR Amplification and High-Throughput Sequencing
Algal, bacterial and fungal fractions of the extracted microbial DNA were amplified, using universal primers for the ITS2 region for fungi and algae, and the 16S hyper-variable region V3–V4 for bacteria (Table 1).
All samples were amplified in duplicate with forward and reverse primers individually tagged with octamers allowing for a double index multiplexing approach. Each duplicate contained eight PCR negative controls (i.e., master mix without sample), that were sequenced as well, meaning that a total of 110 × 2 samples were obtained after PCR. Additionally we included 16 “Multiplex Controls” (i.e., empty wells) to allow detection of potential primer jump during sequencing (Schnell et al., 2015). We set up 15 μl PCR reactions containing 5 ng of DNA, 7.5 μl of MyTaq™ HS Mix, 2x (Bioline GmbH, Luckenwalde, Germany), 0.6 μl 10 μM of each primer, and 4.3 μl DNAse free water. Cycling conditions differed in cycle number and annealing temperature among organismal groups. Conditions were as follows: an initial denaturation at 95°C for 1 min, followed by 30 (algae, bacteria) or 35 (fungi) cycles of denaturation at 95°C for 15 s, annealing a 54°C (algae), 59°C (bacteria) or 56°C (fungi) for 15 s and elongation at 72°C for 10 s, with a final extension at 72°C for 1 min. The number of PCR cycles was determined prior to sampling, using initial test PCRs with material obtained in the same manner. The algal and bacterial amplicons reached a homogenous PCR amplification across all samples after 30 cycles, while the fungal amplification required 35 cycles. Samples were randomly distributed over two 96-well plates, with both replicates following the same placement scheme.
The amplicons were individually cleaned using magnetic beads (MagSI-NGSPrep Plus, magtivio B.V., Geelen, Netherlands) and DNA concentration was quantified with fluorescence measurement using the Qubit dsDNA HS assay (Thermo Fisher Scientific, MA, United States) as specified by the manufacturer. The replicates were equimolarly pooled within the respective organismal groups, creating a total of three pools for sequencing. The pooled amplicons were send for library preparation and sequencing to Fasteris SA (Plan-les-Ouates, Switzerland). Libraries were prepared for each pool according to the Fasteris MetaFast protocol1, in order to avoid PCR for library preparation and thus minimizing additional PCR bias and chimera creation. The samples were sequenced on an Illumina MiSeq (Illumina Inc., San Diego, CA, United States) with 2 × 300 bp paired-end reads.
Bioinformatics
Adapter-trimmed reads trimmed with Trimmomatic (Bolger et al., 2014) were supplied by the sequencing provider. We demultiplexed the reads using Cutadapt v3.3 (Martin, 2011) following the demultiplexing combinatorial dual-indexes section of the manual. The error rate was set to 0.15, allowing no insertions or deletions, and discarding reads shorter than 50 bp. Commands were run a second time with the octamer tags in the reverse order to account for amplicons in mixed orientation resulting from PCR-free library preparation. The resulting files were merged to obtain one R1 and one R2 file per replicate. Reads were checked for remaining primer sequences, which were removed using Cutadapt, if present.
The demultiplexed reads were further processed with the DADA2 pipeline (Callahan et al., 2016). Filtering and trimming operations used DADA2 default parameters, except for setting a truncation length [truncLen = c(250,260)] for bacteria, but not for algae and fungi since the length of the ITS2 region can vary between taxa (Schoch et al., 2014). Furthermore, the maximum error rates were relaxed to maxEE = c(5,5) for bacteria and maxEE = c(6,6) for algae and fungi. After de-noising and sample inference, pairs were merged within each replicate, chimeras were removed and one amplicon sequence variant (ASV) table was constructed per replicate. To account for the mixed orientation of the libraries we checked the tables for reverse complement sequences, reversed them and added their counts to the respective complement sequence using DADA2s rc() function. Finally, the replicates were merged by summing up the read counts.
For taxonomic assignment the sequences were matched against publicly available databases, namely UNITE general release 8.2 (Abarenkov et al., 2020) for fungal reads and SILVA 138.1 SSU Ref NR 99 (Quast et al., 2012) for bacteria. Since no similar database is currently available for green algae we used the program SEED2 v2.1.2 (Větrovský et al., 2018) to conduct a BLASTn search against GenBank (Clark et al., 2016, last accessed 30.03.2022).
We then checked the reads for potential contamination with the decontam package (Davis et al., 2018), using the combined prevalence and frequency approach. For all organismal groups decontam only showed low numbers (algae = 0, bacteria = 8, fungi = 4) of potential contaminant ASVs, which were discarded. The decontam-filtered ASV tables were curated using the LULU algorithm (Frøslev et al., 2017) to merge highly similar ASVs and obtain more reliable diversity metrics. Taxonomic information for all ASVs can be found in Supplementary Table 1.
Diversity and Community Structure Analyses
All analyses were conducted in R (R Core Team, 2021, version 4.0.4) through RStudio (RStudio Team, 2021). ASV tables, taxonomic information and accompanying metadata were combined using the phyloseq R package (McMurdie and Holmes, 2013) to ease analyses. Figures were created with ggplot2 (Wickham, 2016) and gridExtra (Auguie, 2017). Samples were not rarefied, as recommended by McMurdie and Holmes (2014) and instead treated as compositional count data (Gloor et al., 2017). Scripts of all analyses are available on GitHub at https://github.com/LukDrey/beech_micro_communities.
Intra-Group Diversities
We calculated the Shannon Index (Shannon, 1948) as a measure of alpha diversity, using the function estimate_richness() on the full untransformed ASV table as obtained from DADA2 and LULU. Differences in Shannon diversity between tree sizes and management category were tested with an Analysis of Variance (ANOVA) with the function aov() and verified via a Tukey Honest Significant Differences test (Tukey HSD). Furthermore, we tested whether the Shannon diversity for trees within a plot was spatially autocorrelated. For this purpose, we computed Moran’s I (method from Gittleman and Kot, 1990) as a measure of spatial autocorrelation with the function Moran.I from the ape R package (Paradis and Schliep, 2019).
To create the community barplots we aggregated taxa at the order rank and subset the datasets to the 25 relatively most abundant taxa with get_top_taxa() (Teunisse, 2017). The resulting subsets were transformed to reflect their compositional nature by transform() and plotted using plot_composition(), both from the microbiome R package (Lahti and Shetty, 2017).
Inter-Group Differences
Before comparing differences in community composition of differently sized trees and management regimes, each full dataset was transformed based on centred log-ratios (CLR) with transform(). After the transformation we conducted a principal component analysis (PCA) on the clr-transformed datasets using the phyloseq function ordinate() (“RDA” method) which for clr-transformed data is the same as PCA. In the ordination plots we show the first two Principal Components (PC), with the axes scaled to the proportion of variance the PC explains, as recommended by Nguyen and Holmes (2019).
To test if groups showed similar within-group variance, we used the betadisper() function and verified the results with the accompanying permutation test permutest() from the vegan package (Oksanen et al., 2020). To test for differences in community composition between tree sizes and management intensity we performed Permutational Analysis of Variance (PERMANOVA) with a distance matrix based on Aitchison’s distance (method = “euclidean” with the phyloseq function distance() for clr-transformed data). The PERMANOVA was computed using the vegan function adonis2() examining marginal effects of tree size and management intensity together.
Species Interactions
The interaction networks were generated with the SPIEC-EASI method (Kurtz et al., 2015), a robust method for the sparse and compositional nature of microbiome datasets implemented in the R package SpiecEasi. Before network inference the ASV tables were subset to contain only ASVs contributing at least one percent of the total reads to ease both visualization and computational load. The main SPIEC-EASI algorithm was set to use the meinshausen-bühlmann’s neighborhood selection (Meinshausen and Bühlmann, 2006) and Bounded StARS model selection (Müller et al., 2016) on 50 subsamples (rep.num = 50), with lambda.min.ratio = 0.1, nlambda = 100, pulsar.select = TRUE and seed = 10010. We calculated one network per organismal group, as well as one containing all three groups together.
The obtained models were refit, turned into igraph (Csardi and Nepusz, 2006) objects and loaded in Gephi v0.9.2 (Bastian et al., 2009) for visualization. Modularity and betweenness centrality (for visualization purposes) were computed with Gephis internal algorithms (Brandes, 2001; Blondel et al., 2008). The graph layouts were constructed using the Fruchterman-Reingold algorithm (Fruchterman and Reingold, 1991). For each network, hub taxa were calculated based on vertex betweenness centrality using the igraph function betweeness() with default parameters, except setting directed = FALSE. The top five hub taxa, based on vertex betweenness centrality, were extracted.
Differential Abundance Analysis
Differential abundance analysis was conducted using ALDEx2 (Fernandes et al., 2013, 2014; Gloor et al., 2016). We compared abundances of two groups, i.e., high/low management intensity, large/small, large/medium and medium/small trees, for each organismal group. ALDEx2 generates Monte Carlo samples (N = 128), drawn from the Dirichlet distribution for each individual sample, and tests differences between specified groups through Wilcoxon rank-sum tests. ALDEx2 is a robust choice for compositional datasets because the data is clr-transformed internally. Taxa were declared differentially abundant if they showed a Benjamini-Hochberg corrected p-value < 0.05.
Results
Intra-Group Diversities
In total we obtained on average 59,324 reads per sample for algae (min = 22,913, max = 99,255), 58,259 reads for bacteria (min = 24,830, max = 124,263) and 45,403 reads for fungi (min = 18,510, max = 163,736). The extraction blanks had on average 888 reads for algae (min = 283, max = 1,679), 12,836 for bacteria (min = 462, max = 19,723), and 34,211 for fungi (min = 6171, max = 109,074), while the PCR negative controls contained on average 1,152 reads for algae (min = 272, max = 3,745), 625 reads for bacteria (min = 196, max = 2,076), and 3,241 for fungi (min = 196, max = 15,752). The negative controls were discarded from the analysis after controlling for possible contaminant sequences with decontam (Davis et al., 2018). From these reads, we retrieved 216 algal, 1,742 bacterial and 992 fungal ASVs.
Overall algae and fungi displayed similar Shannon alpha diversity, while bacteria showed a slightly higher diversity (Figure 1). Neither algae, fungi nor bacteria exhibited statistically significant differences in alpha diversity when comparing low and high management intensity plots (Figures 1A–C). Considering differences between tree sizes, smaller trees displayed higher alpha diversity for algae and fungi (Figures 1D,F). Overall, bacterial diversities were more uniform, but displayed higher median Shannon diversity values for larger trees (Figure 1E).
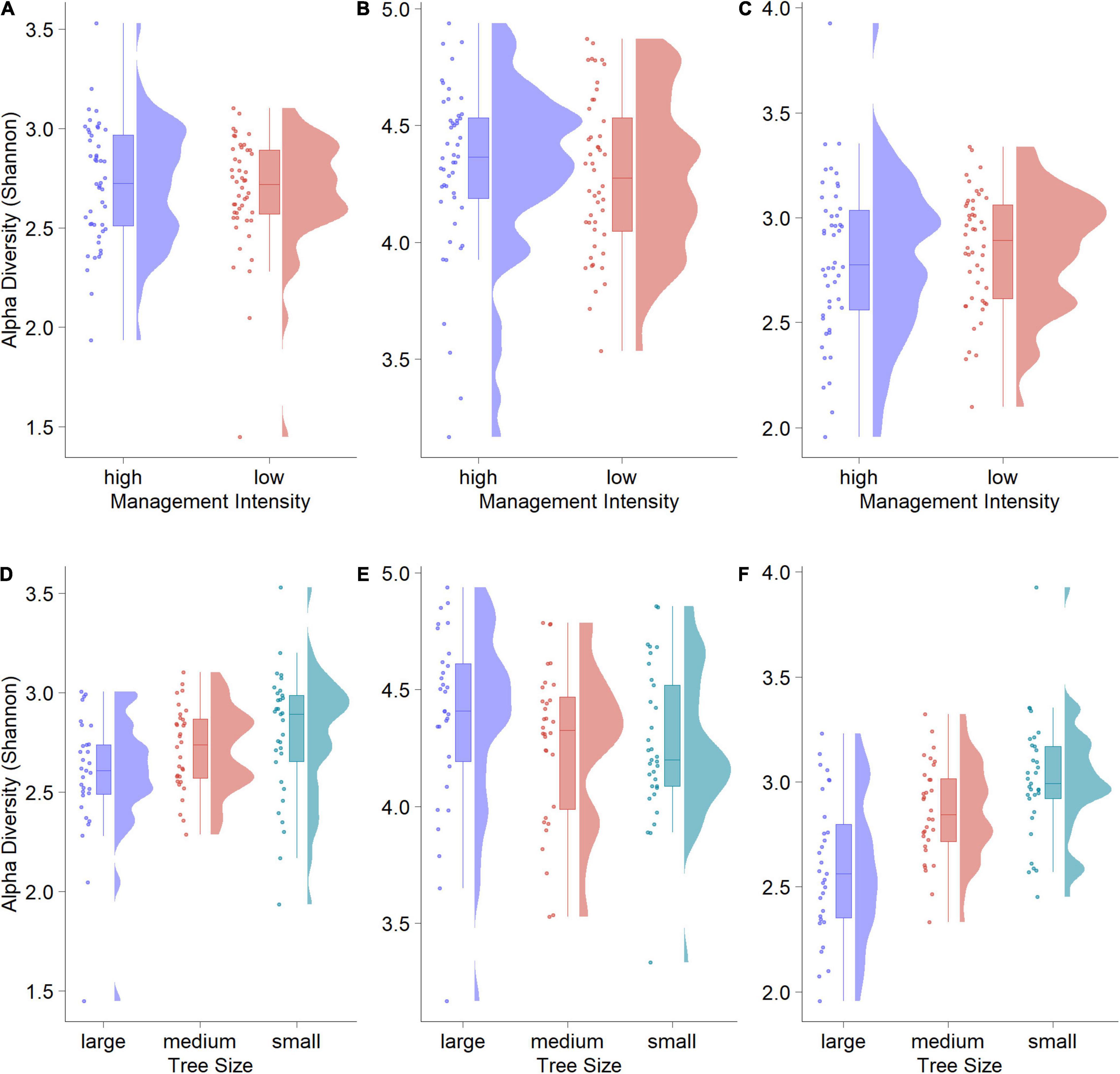
Figure 1. Rain-Cloud plots of alpha diversity (Shannon) against management regime and tree size for algae (A,D), bacteria (B,E) and fungi (C,F). Differences are visible from the boxplots, while the original data structure is visible from raw data scatters (randomly jittered) and raw data distribution.
This trend was corroborated by the results of an ANOVA comparing the three tree-size classes. For algae, we found a significant overall effect of tree size (F = 4.163, p < 0.05), that was driven by a significant difference between large and small trees (Tukey HSD p-value < 0.05). No significant differences were found between large/medium and medium/small trees. Tree size had a significant effect overall (F = 17.33, p < 0.001) in fungi, with significant differences between large and medium (p < 0.01), as well as large and small trees (p < 0.001). For bacteria we found no significant overall effects.
Spatial autocorrelation tests showed that only in four of 48 cases (three organismal groups × 16 plots) the null-hypothesis of no spatial correlation could be rejected (Table 2). This indicates that the effect of spatial autocorrelation within plots is negligible. Trees belonging to the same plot showed very similar Shannon alpha diversity values. One exception is plot HEW8 where alpha diversity was significantly spatially autocorrelated for both algae and bacteria.
Bark microbial communities were similar among trees, with only minor differences in rare orders for all three organismal groups. For algae, the predominant orders were Trebouxiales and Chlorellales, with Trebouxiales contributing more than 50% of the reads in many plots (Figure 2A). Rare orders displayed a relatively high inter-plot variability, with Prasiolales being almost absent for the plot HEW5. Compared to algae, bacteria were more homogeneous, with the same four orders—Rhizobiales, Sphingomonadales, Acetobacterales and Cytophagales—displaying comparably high relative abundances in all plots (Figure 2B). We found a higher rare-order diversity in bacteria and fungi compared to algae. Capnodiales was by far the most abundant fungal order, and dominant in all plots (Figure 2C). Compared to bacteria and algae, a higher proportion of fungal reads could not be assigned at the order rank. These unassigned reads contributed more than 25% of the total reads in some samples. The three relatively most abundant ASVs in the algal dataset belong to the genera Symbiochloris (12%), Apatoccocus (12%) and Desmococcus (8%), and in the bacterial dataset to Acidiphilium (10%), 1174-901-12 (4%) and Methylocella (4%). Only one of the three most abundant fungal ASVs could be assigned to a genus—namely Scoliciosporum (4%)—while one was assigned to Capnodiales (26%) and one only to Ascomycota (7%).
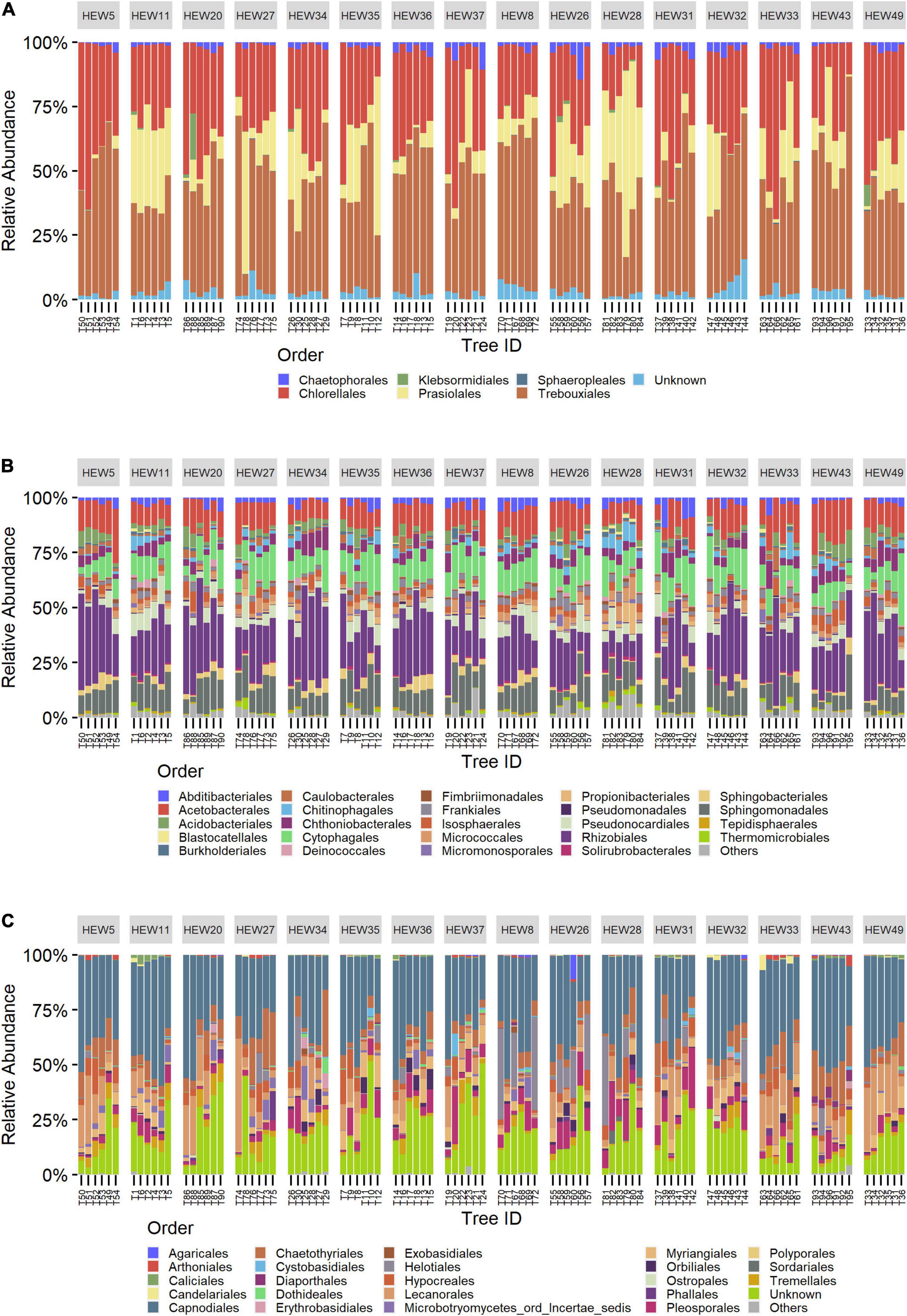
Figure 2. Community bar charts showing the relative abundance of the 25 most abundant orders split by sampling plots for algae (A), bacteria (B) and fungi (C). From left to right: the first eight plots are under a low-, and the next eight under a high-management regime. Bars within plots represent individual trees, from large to small tree-size class (2 trees each), from left to right.
Intra-Group Interactions
We inferred ASV interaction networks for all three microbial groups (Figure 3). Each ASV entering the networks contributed at least 1% of the total reads, resulting in 129 ASVs for the algae, 624 for bacteria and 289 for fungi. The algal network (Figure 3A) had a diameter of 10 (i.e., the longest shortest path between two nodes was through ten edges), an average path length of four and a modularity score of 0.575. Modularity scores > 0.4 indicate strong modularity (Newman, 2006). The diameter for the fungal network (Figure 3C) was 7, with an average path length of ∼3.2 and a modularity slightly lower than the algae at 0.434. The bacterial network (Figure 3B) was denser and more interconnected with a diameter of 5, an average path length of ∼2.7 and a modularity of 0.335.
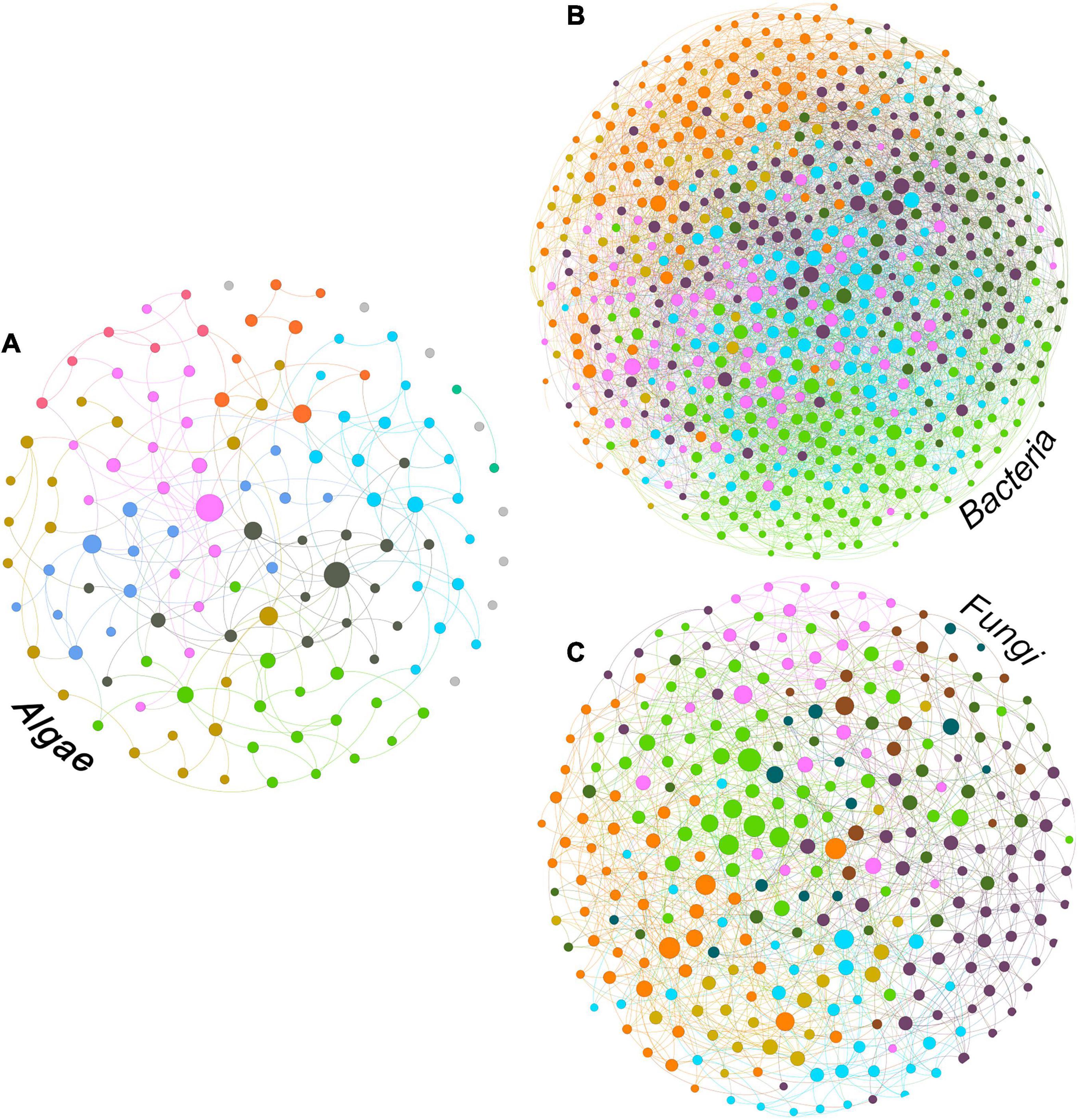
Figure 3. Intra-group ASV interaction network for algal (A), bacterial (B) and fungal (C) fractions of the bark microbiome. The size of the nodes is proportional to the value of betweenness centrality (based on Brandes (2001)) and colors correspond to modules (Blondel et al., 2008).
The algal network could be subdivided into nine different modules, five of which consisting of more than 10 ASVs (see Table 3). There were also 8 ASVs that did not interact with any other taxon in the network. The algal module with the highest number of nodes was module 2 (gold color, Figure 3A) with the most abundant ASV belonging to the genus Desmococcus (relative abundance = ∼47% in the module, Table 3).
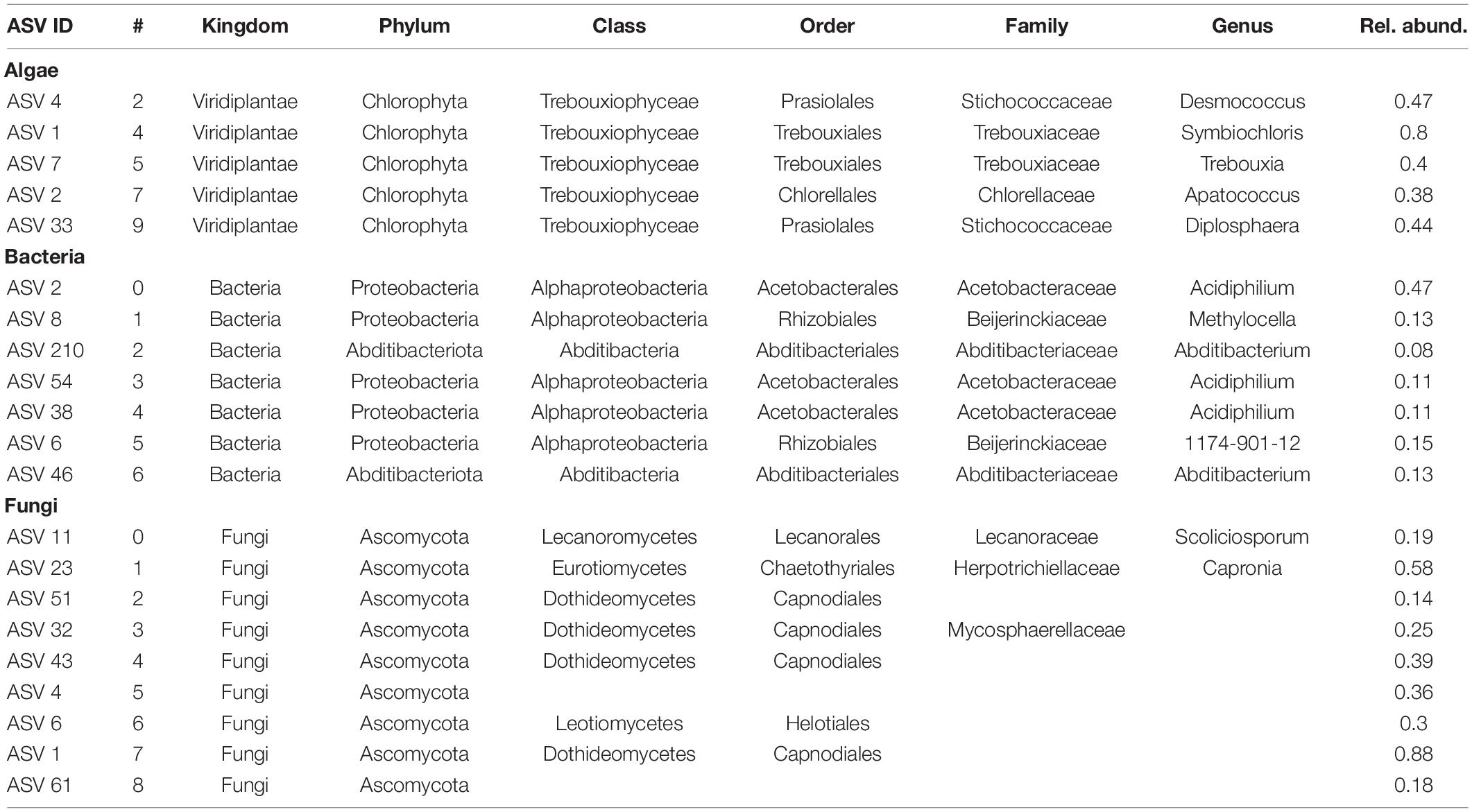
Table 3. Taxonomic assignment of the most abundant ASVs and their relative abundance for modules with more than 10 ASVs.
The bacterial network consisted of seven modules, all of which included more than 10 ASVs and no taxa with no connections. In this case, module 6 (purple color in Figure 3B) was the module with the highest count of taxa, with an ASV assigned to the genus Abditibacterium being the predominant strain (12% of reads in the module, Table 3).
For fungi, the network clustered into nine different modules, all containing more than ten ASVs and no unconnected taxa. Also in this case, module two (purple color, Figure 3C) was the module with the highest number of taxa for the fungal network, with an ASV belonging to the order Capnodiales—not assignable more specifically (Table 3)—with the highest relative abundance in the module (14%).
Network structure was examined by identifying nodes with the highest number of shortest paths going through them (betweenness centrality), indicating taxa that are important for the connectivity of the network. We defined so called hub taxa as the five taxa with the highest betweenness centrality (Table 4). In the algal network these hub taxa belonged to two orders, Chlorellales and Trebouxiales, and three different genera. Three ASVs were assigned to the genus Apatoccocus, and one to Trebouxia and Symbiochloris, respectively. Bacterial hub taxa showed a higher diversity than the algae with hub taxa belonging to five different orders. The genera include Tundrisphaera, Actinomycetospora and Oligoflexus. One of the ASVs was assigned to the group 1174-901-12, a group of uncultured bacterial strains within the order Rhizobiales and one to the family Chitinophagaceae. Many of the fungal hub taxa were not assigned at the genus rank, with the exception of two ASVs belonging to the genera Tremella and Aureobasidium. Two more ASVs were assigned to the order Capnodiales while one was only assigned at the phylum rank.
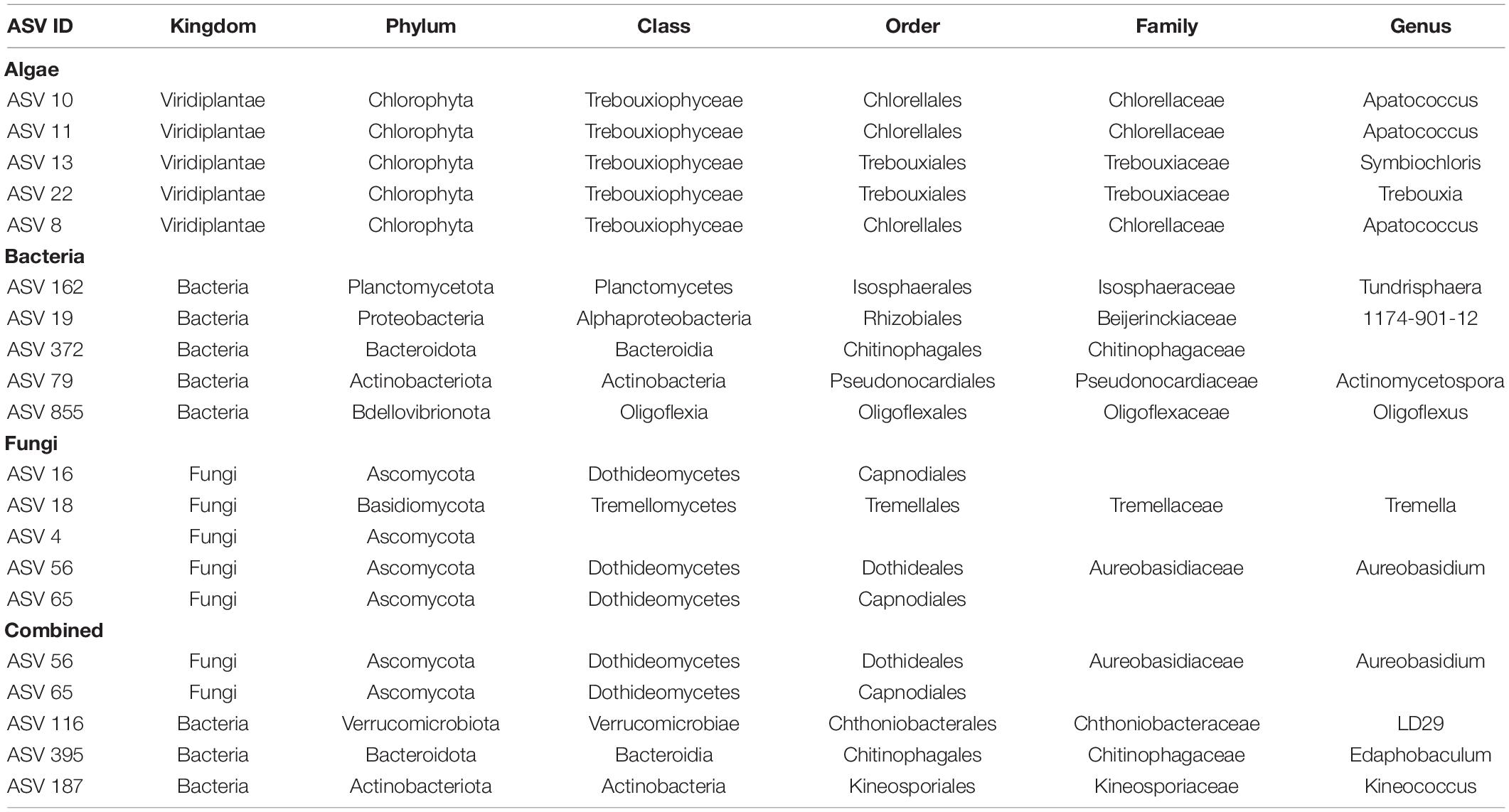
Table 4. Taxonomic information for the hub taxa of the respective network, identified based on their betweenness centrality.
Inter-Group Interactions
The combined network of algae, bacteria and fungi (Figure 4) displayed a diameter of 4, an average path length of ∼2.6 and a modularity of 0.259, making this network more densely connected than the bacterial network. Out of the eight modules, modules two (29%) and three (26%) accounted for more than half of the total reads.
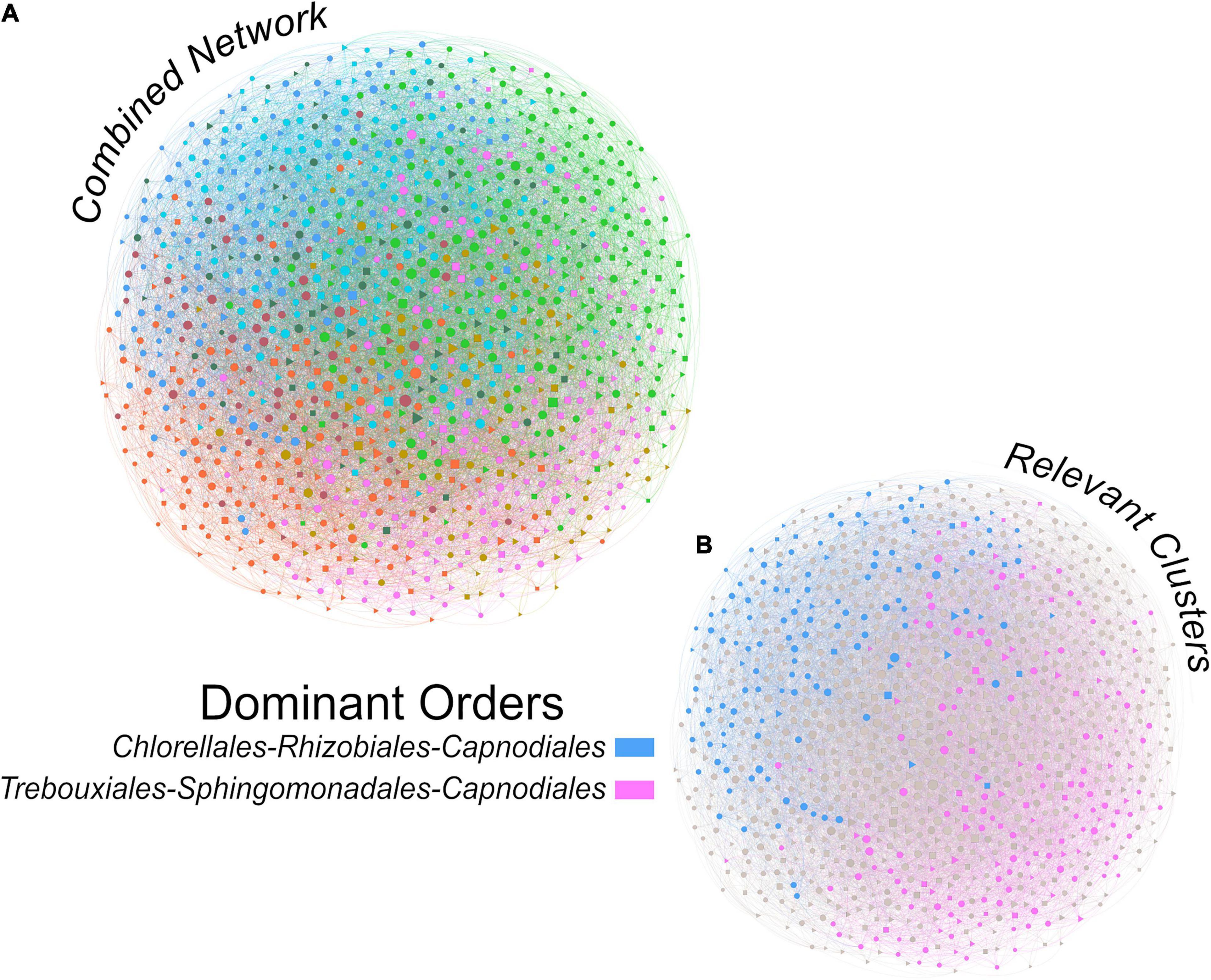
Figure 4. Inter-group ASV interaction network integrating algal, bacterial and fungal taxa for a description of the interactions within the complete bark microbiome (A). The two colored modules (B) are the two most abundant modules of the whole dataset, accounting for 29% (pink) and 26% (blue) of the total reads. The size of the nodes is proportional to the value of betweenness centrality (based on Brandes (2001)) and colors correspond to modules (Blondel et al., 2008).
The top orders for algae, bacteria and fungi in the relatively most abundant module (module 2, pink color in Figure 4B) were Trebouxiales, Sphingomonadales and Capnodiales, respectively. Algae and fungi accounted for up to 36 and 26% of the module reads, respectively, while Sphingomonadales only contributed 5% of the reads. Trebouxiales contributed 87% of the algal reads, Sphingomonadales 30% of bacterial reads, and Capnodiales 77% of the fungal reads. In total, algae contributed 42%, bacteria 19%, and fungi 34% of the reads assigned to module 2.
In the second most abundant module (module 3, blue color in Figure 4B). Chlorellales were the most important algal order, Rhizobiales the most important bacterial order, while the most important fungal order was again Capnodiales. Chlorellales contributed 18%, Rhizobiales 16% and Capnodiales only 5% of the module reads. Chlorellales accounted for 43% of the algal reads, Rhizobiales 48% of the bacterial reads, and Capnodiales 34% of the fungal reads. Overall, module three consisted of 42% algae, 34% bacteria and 10% fungi.
The hub taxa of the combined network (Table 4) contained members of two microbial groups, specifically three bacteria and two fungi. Four ASVs could be assigned down to genus rank, while one fungal ASV—a hub taxon present also in the fungal network—could only be assigned to order rank (Capnodiales). The other fungus—also a fungal hub taxon—belonged to the genus Aureobasidium, while the three bacterial ASVs belonged to the genus Edaphobaculum, the uncultured group LD29 within the order Chthoniobacterales, and the genus Kineococcus.
Drivers of Changes in Community Composition
To investigate differences in community composition we plotted the results of the PCA (Figure 5). The differences between the high and low management intensity are not readily visible by looking at the clusters of the two groups. Yet, there are significant differences between the groups as revealed by the PERMANOVA results (algae: p < 0.05, bacteria: p < 0.01, fungi: p < 0.001). The dispersion permutation test was significant (algae: p < 0.05, bacteria: p < 0.05, fungi: p < 0.05) indicating a heterogeneous dispersion within groups. If on one hand this might suggest that the results are not reliable, Anderson and Walsh (2013) showed that PERMANOVA is not sensitive against heterogeneity, compared to other analyses. Management intensity explained only ∼2% of the variance in the dataset, suggesting a subtle yet significant effect.
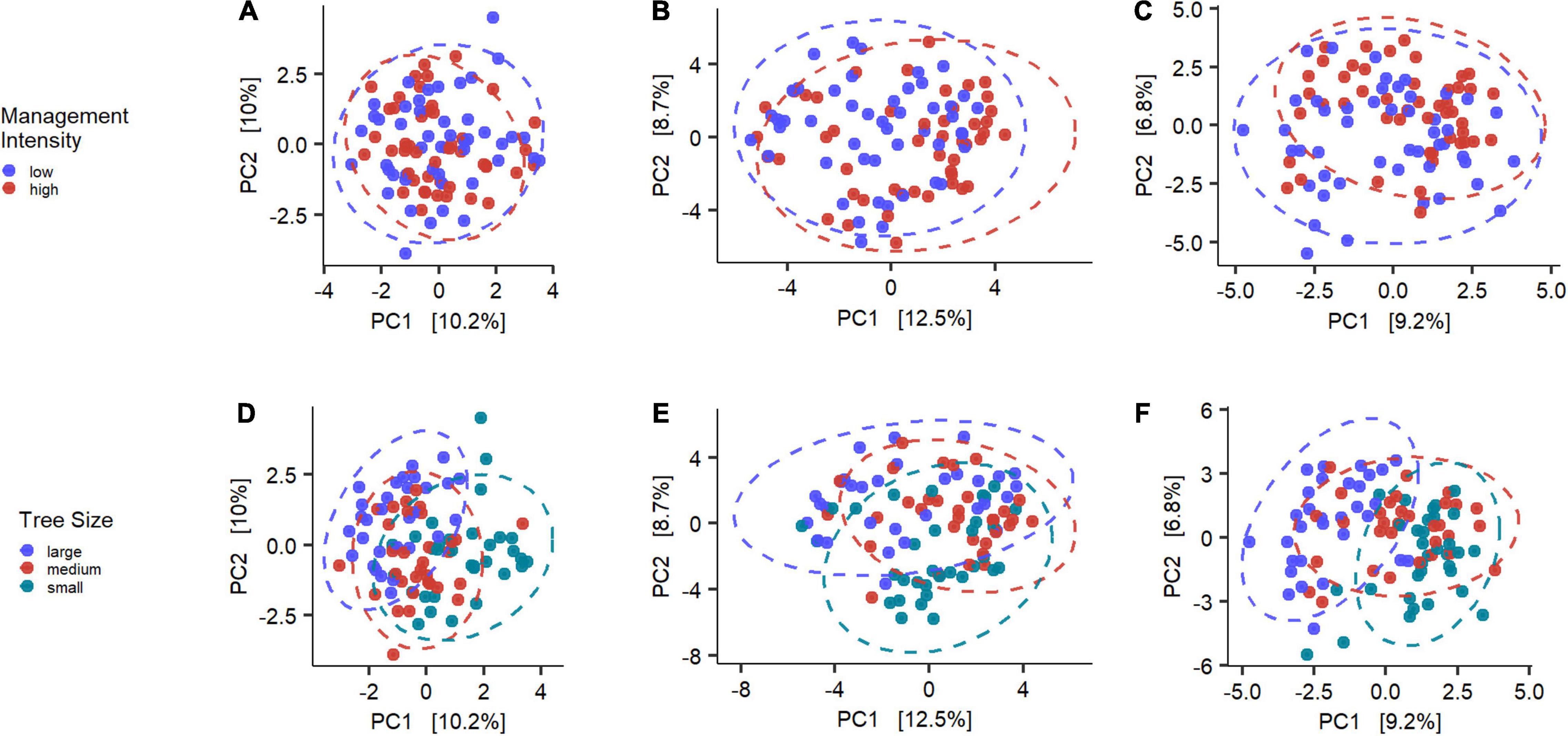
Figure 5. Overview of the Principle Component Analysis for algae (A,D), bacteria (B,E) and fungi (C,F). Colors indicate the tested groups and axes are scaled to the proportion of variance explained by the displayed Principal Component (Nguyen and Holmes, 2019).
The tree sizes clustered in a much clearer structure, with less overlap of the 95% confidence interval ellipses. The PERMANOVA analysis confirmed this observation, with highly significant results for all microbial groups (p < 0.001 for all), while the permutation test indicated homogenous dispersion within the bacteria and fungi (p > 0.05), but not the algae (p < 0.05).
Further examination of the community structure showed that the differences in community composition were also visible through significantly differentially abundant taxa (Supplementary Table 2). The difference between management intensities was little, with only four differentially abundant fungal ASVs. The tree-size classes, however, showed a different signal and confirmed the stronger differences indicated by the PERMANOVA results. Between large and medium trees we found nine algae, four bacteria and 10 fungal taxa that showed significant abundance differences. A larger difference in abundances could be seen between large and small trees, with 19 algae, 43 bacteria and 34 fungi that were differentially abundant. Almost no difference could be observed between communities of medium and small trees with only three algal and one bacterial ASVs with significant changes in abundance.
Discussion
In this study we used a multi-kingdom metabarcoding approach to investigate the microbiome (algae, bacteria, fungi) on the bark of beech from sites with different forest management intensity in the Hainich-Dün region, Thuringia, Germany. We provide a first characterization of aboveground bark-associated microbial communities in beech forests, as well as an account of microbial inter-kingdom interactions. Additionally, by testing how community diversity and structure of the different organismal groups change in relation to land-use intensity and tree size, we identify potential drivers of community assembly and provide sampling recommendations for studying the bark microbiome at broader spatial scales.
Diversity of the Bark-Associated Beech Microbiome
Our results show that the bark of beech harbors highly diverse algal, bacterial and fungal communities.
The modules and hub-taxa of the algal interaction networks are mainly represented by species of the families Trebouxiophyceae and Chlorellaceae, in particular by genera commonly found in subaerial environments and already detected in forest settings (Štifterová and Neustupa, 2015). Algae of the genus Apatococcus, a “flagship” taxon of above-ground ecosystems (Rindi, 2007), are abundant in the modules and interconnected members of the algal microbiome. Additionally, Apatoccocus is a known photobiont of Scoliciosporum (Sanders and Masumoto, 2021), a very common fungal genus in our dataset. Another abundant alga is Desmococcus sp., which typically forms visible powdery, greenish layers on the bark of trees in association with Apatococcus and other subaerial green algae (Rindi, 2007). Both are part of what is possibly the most tolerant subaerial algal community, being able to thrive even in urban areas (Barkman, 1958). Furthermore, our results confirm Symbiochloris as an important component of the dermo- and phyllosphere (Škaloud et al., 2016; Zhu et al., 2018), as well as other Trebouxiales, an order consisting of free-living as well as lichen-forming green algae (Sanders and Masumoto, 2021). One of the algal hub taxa comes from the genus Trebouxia, the most common lichen forming alga (Sanders and Masumoto, 2021).
Compared to the algae and fungi, the important taxa of the bacterial network modules are far more diverse. Similarly to Aschenbrenner et al. (2017), the bacterial community is dominated by the class Alphaproteobacteria, in particular Rhizobiales and Acetobacteriales. Among the Rhizobiales we detected Methylocella sp., a facultative methanotroph adapted to various nutrients (acetate, pyruvate, succinate, malate, and ethanol) (Dedysh and Dunfield, 2011), and 1174-901-12, previously described as an early colonizer of aerial environments (Romani et al., 2019) and a known member of the phyllosphere (Ares et al., 2021). In the case of Acetobacterales, Acidiphilium is among the taxa contributing the most to the modules. The genus consists of aerobic bacteria with photosynthetic pigments, with a pH range that matches well to the pH of beech bark (∼4.4 according to Asplund et al. (2015)) and that do not overlap in metabolic demands with Methylocella (Hiraishi and Imhoff, 2015). Another well represented class in the modules are the Abditibacteria, especially the genus Abditibacterium whose representatives are well adapted to low-nutrient conditions and have been reported on tree bark before (Tahon et al., 2018; Kobayashi and Aoyagi, 2019). Among the bacterial hub taxa we find genera from classes already reported for tree-associated microbiomes, such as Oligoflexus in bryophytes (Ma et al., 2017), Actinomycetospora and 1174-901-12 on tree bark and lichens (Yamamura et al., 2011; Ares et al., 2021), and Tundrisphaera, previously only isolated from lichen-dominated tundra soils (Kulichevskaya et al., 2017). One ASV belongs to Chitinophagaceae, a family associated with the degradation of fungal cell-walls (Carrión et al., 2019). As found for the algae, some of the most abundant genera are important components of the bacterial networks. Contrary to Aschenbrenner et al. (2017), we did not find major contributions from the genera Burkholderia and Pseudomonas, possibly indicating that these genera are specific to the bark of sycamore maple (Acer pseudoplatanus).
The investigation of the important fungal diversity was somewhat hindered by low taxonomic resolution with many ASVs that could not be assigned past phylum rank. This may result from a lack of resolution in public fungal databases combined with the presence of several unknown taxa in our dataset. Important members of the fungal networks in the beech bark community are the so-called black yeasts, e.g., Capronia and Aureobasidium, which are known to occur on tree bark and leaves (Untereiner and Malloch, 1999; Andrews et al., 2002), but also decaying wood (Cooke, 1959) and on other fungi or lichens as secondary saprobionts (Untereiner and Malloch, 1999). Other important network components belong to the genus Tremella, known mycoparasites (Zugmaier et al., 1994). Among the lichen-forming fungi, one of the most common fungi in our dataset belongs to the genus Scoliciosporum, a genus of crustose lichens that was already reported on beech bark (e.g., Dymytrova, 2011). The biggest contributors at the order rank are members of the Capnodiales (Dothideomycetes), whose species have been shown to associate with the lichen microbiome (Smith et al., 2020) and are abundant in the beech phyllosphere as well (Unterseher et al., 2016). Yet, more research into these orders is needed as they are taxonomically and ecologically highly diverse and include a large diversity of life forms, from lichenized, to mycoparasytic, epi-, ecto-, endophytic, as well as saprobiontic species. In contrast to Unterseher et al. (2016) we could not find a larger contribution of Helotiales, suggesting a specialization of this fungal order to the phyllosphere. The most abundant ASVs of two fungal modules, as well as one of the most abundant ASV overall, could not be assigned further than Ascomycota, highlighting that important components of the fungal bark microbiome remain undescribed. Sampling in different seasons may reveal an even higher—described and undescribed—fungal diversity on tree trunks (Beck et al., 2014). In conclusion, more research is needed in order to confirm the role of the bark habitat as a reservoir of novel fungal diversity. This could be done by combining genetics and culture-based approaches.
Biotic Interactions and Inter-Kingdom Synergies in the Bark Microbiome
The higher modularity scores of the fungal and especially algal networks may indicate higher specialization or niche differentiation in these groups (Augustyn et al., 2016). In contrast, bacteria are less clearly divided into ecological modules, which potentially indicates closer interactions between all taxa as there seems to be no split into specialized groups. Further analyses based on a broader dataset are needed to exclude that the observed patterns are an artifact of the overall higher diversity found in bacteria.
The results from the combined, inter-kingdom co-occurrence analysis indicate that algal and fungal specialists might be connected through a common set of bacteria. It is tempting to speculate that the interactions between Rhizobiales and Chlorellales (mostly represented by members of the genus Apatococcus) observed in the main ecological cluster in our dataset are of symbiotic nature, as Rhizobiales are well-known beneficial partners in plant-microbe interactions and common associates of lichens (Erlacher et al., 2015; Grube et al., 2015). Positive interactions among Sphingomonadales, Trebouxiales, and Capnodiales—all known occupants of bark substrates—characterize the second most important cluster. The bacterial genus Sphingomonas is very common in above-ground forests habitats (Vorholt, 2012), exhibiting facultative photosynthesis.
Finally, we identified the most highly connected taxa (hubs), i.e., taxa that are crucial for the stability of the ecological network (Banerjee et al., 2018). For bacteria, the hub taxa belong to the genera LD29 (Verrucomicrobiota), Edaphobaculum (Bacteroidetes) and Kineococcus (Actinobacteria). Little is known about their ecology, with LD29 particularly abundant in lichen thalli (Aschenbrenner et al., 2017), Edaphobaculum previously found in soils where it contributes to the creation of biofilms (Keuschnig et al., 2021), and Kineococcus isolated from soil samples as well as the rhizosphere (Normand and Benson, 2015). As for the fungi, both of the inter-kingdom hub taxa are also found as hub taxa in the fungal network. One of them belongs to the genus Aureobasidium, common on leaves of apple trees (Andrews et al., 2002) but also linked to the decay of bark (Cooke, 1959). The other could not be assigned below the order rank and is a member of the Capnodiales.
Bark Microbiome Responds to Tree Size, but Not to Intensity of Forest Management
The intensity of the forest management regime has virtually no effect on microbial community diversity and structure in our study area. This might be a result of a forest management plan that avoids clear cuts and carefully selects trees to harvest, which leads to a uniform forest structure in the study area (Schall et al., 2020). Based on a broader sampling including this and other two large forest areas in Germany, Boch et al. (2021) showed that an increase in forest management intensity is linked to reduced lichen species richness. A larger sampling effort covering a broader gradient of land-use intensity is therefore required to test whether the response of the bark-associated microbiome differs from that of the macroepiphytes.
We found significant differences in diversity and composition of the bark microbiome according to different tree-size classes. The lower microbial diversity found on larger (older) trees for algae and fungi is probably the result of environmental filtering on highly heterogeneous pioneer communities over time. This is particularly evident when comparing large and small trees, thus suggesting slow succession of these microbiomes toward final community composition. Our results from the spatial autocorrelation analysis underpin random assembly of the microbial bark community at the local (plot) level, with a high heterogeneity between trees. Lastly, a finer-scale comparison of micro-habitats, i.e., different exposures, bark crevices/cracks/holes, would be of great interest for disentangling micro-scale interactions that our approach cannot reliably identify.
Conclusions and Sampling Recommendations
In this pioneering study we provide novel insights into the diversity, spatial context, and biotic interactions that characterize the beech bark microbiome in Central European forests. We showed that there are predictable community shifts depending on tree age. These represent the first steps toward proposing a framework of community assembly on forest tree bark, a ubiquitous, ecologically relevant, yet overlooked component of terrestrial habitats.
Taken together, our results show that a single tree does not adequately characterize the bark-associated microbial community at plot level. To capture most of the microbial diversity, considering the spatial randomness shown by the spatial autocorrelation analysis, we recommend sampling using a spatially random approach with a balanced representation of the main tree-size classes present in the plot. Samples taken from multiple trees can then be combined into a composite sample. The use of composite samples ensures relatively low costs for obtaining adequate sequencing depths while maximizing the spatial range of the study and the number of plots, allowing for easy upscaling to large areas and/or environmental gradients. This data can then be used to assess the effects of factors, e.g., related to forest structure, such as stand density and canopy openness.
Data Availability Statement
The raw sequences are deposited in the NCBI SRA repository, accession numbers SRR18461106, SRR18461107, and SRR18461108. All scripts and additional data necessary to replicate the analysis are available at https://github.com/LukDrey/beech_micro_communities. The data on the Forest Management Index are available at https://www.bexis.uni-jena.de/ under Accession number 16466.
Author Contributions
FD and IS secured the funding. FD and LD devised the sampling and analytical methods, conducted the fieldwork and sampling, and wrote the manuscript with contributions from IS. LD performed the laboratory work and analysis. All authors contributed to the article and approved the submitted version.
Funding
The work has been funded through the DFG Priority Program 1374 “Biodiversity-Exploratories” (SCHM 1711/8-1 and GR 5437/4-1). Field work permits were issued by the responsible state environmental offices of Thüringen.
Conflict of Interest
The authors declare that the research was conducted in the absence of any commercial or financial relationships that could be construed as a potential conflict of interest.
Publisher’s Note
All claims expressed in this article are solely those of the authors and do not necessarily represent those of their affiliated organizations, or those of the publisher, the editors and the reviewers. Any product that may be evaluated in this article, or claim that may be made by its manufacturer, is not guaranteed or endorsed by the publisher.
Acknowledgments
We thank the managers of the Hainich-Dün Exploratory, Anna K. Franke, and all former managers for their work in maintaining the plot and project infrastructure; Victoria Grießmeier for giving support through the central office, Andreas Ostrowski for managing the central data base, and Markus Fischer, Eduard Linsenmair, Dominik Hessenmöller, Daniel Prati, Ingo Schöning, François Buscot, Ernst-Detlef Schulze, Wolfgang W. Weisser and the late Elisabeth Kalko for their role in setting up the Biodiversity Exploratories project. We thank the administration of the Hainich national park, as well as all landowners for the excellent collaboration. Additionally, we thank Ulrich Pruschitzki for his valuable help in the forest and Jürgen Otte for helpful comments on laboratory procedures and technical assistance.
Supplementary Material
The Supplementary Material for this article can be found online at: https://www.frontiersin.org/articles/10.3389/ffgc.2022.858382/full#supplementary-material
Footnotes
- ^ https://www.fasteris.com/en-us/NGS/DNA-sequencing/Metabarcoding/Metagenomics-16S-18S-ITS-or-custom-PCR-amplicons
References
Abarenkov, K., Zirk, A., Piirmann, T., Pöhönen, R., Ivanov, F., Nilsson, R. H., et al. (2020). Unite General Fasta Release For Fungi. doi: 10.15156/BIO/786368
Aguirre-von-Wobeser, E., Alonso-Sánchez, A., Méndez-Bravo, A., Villanueva Espino, L. A., and Reverchon, F. (2021). Barks from avocado trees of different geographic locations have consistent microbial communities. Arch. Microbiol. 203, 4593–4607. doi: 10.1007/s00203-021-02449-6
Anderson, M. J., and Walsh, D. C. I. (2013). PERMANOVA, ANOSIM, and the Mantel test in the face of heterogeneous dispersions: what null hypothesis are you testing? Ecol. Monogr. 83, 557–574. doi: 10.1890/12-2010.1
Andre, H. M. (1985). Associations between corticolous microarthropod communities and epiphytic cover on bark. Ecography 8, 113–119. doi: 10.1111/j.1600-0587.1985.tb01161.x
Andrews, J. H., Spear, R. N., and Nordheim, E. V. (2002). Population biology of Aureobasidium pullulans on apple leaf surfaces. Can. J. Microbiol. 48, 500–513. doi: 10.1139/w02-044
Ares, A., Pereira, J., Garcia, E., Costa, J., and Tiago, I. (2021). The leaf bacterial microbiota of female and male kiwifruit plants in distinct seasons: assessing the impact of Pseudomonas syringae pv. actinidiae. Phytobiomes J. 5, 275–287. doi: 10.1094/PBIOMES-09-20-0070-R
Arrigoni, E., Albanese, D., Longa, C. M. O., Angeli, D., Donati, C., Ioriatti, C., et al. (2020). Tissue age, orchard location and disease management influence the composition of fungal and bacterial communities present on the bark of apple trees. Environ. Microbiol. 22, 2080–2093. doi: 10.1111/1462-2920.14963
Arrigoni, E., Antonielli, L., Pindo, M., Pertot, I., and Perazzolli, M. (2018). Tissue age and plant genotype affect the microbiota of apple and pear bark. Microbiol. Res. 211, 57–68. doi: 10.1016/j.micres.2018.04.002
Aschenbrenner, I. A., Cernava, T., Erlacher, A., Berg, G., and Grube, M. (2017). Differential sharing and distinct co-occurrence networks among spatially close bacterial microbiota of bark, mosses and lichens. Mol. Ecol. 26, 2826–2838. doi: 10.1111/mec.14070
Asplund, J., Ohlson, M., and Gauslaa, Y. (2015). Tree species shape the elemental composition in the lichen Hypogymnia physodes transplanted to pairs of spruce and beech trunks. Fungal Ecol. 16, 1–5. doi: 10.1016/J.FUNECO.2015.03.006
Aude, E., and Poulsen, R. S. (2000). Influence of management on the species composition of epiphytic cryptogams in Danish Fagus forests. Appl. Veg. Sci. 3, 81–88. doi: 10.2307/1478921
Auguie, B. (2017). gridExtra: Miscellaneous Functions for “Grid” Graphics. Available online at: https://cran.r-project.org/package=gridExtra (accesed January 19, 2022).
Augustyn, W. J., Anderson, B., and Ellis, A. G. (2016). Experimental evidence for fundamental, and not realized, niche partitioning in a plant-herbivore community interaction network. J. Anim. Ecol. 85, 994–1003. doi: 10.1111/1365-2656.12536
Baldrian, P. (2017). Forest microbiome: diversity, complexity and dynamics. FEMS Microbiol. Rev. 40, 109–130. doi: 10.1093/femsre/fuw040
Banerjee, S., Schlaeppi, K., and van der Heijden, M. G. A. (2018). Keystone taxa as drivers of microbiome structure and functioning. Nat. Rev. Microbiol. 16, 567–576. doi: 10.1038/s41579-018-0024-1
Barkman, J. J. (1958). Phytosociology And Ecology Of Cryptogamic Epiphytes, Including A Taxonomic Survey And Description Of Their Vegetation Units In Europe. Assen: Van Gorcum.
Bastian, M., Heymann, S., and Jacomy, M. (2009). Gephi: An Open Source Software for Exploring and Manipulating Networks. Available online at: https://gephi.org/ (accesed January 19, 2022).
Beck, A., Peršoh, D., and Rambold, G. (2014). First evidence for seasonal fluctuations in lichen- and bark-colonising fungal communities. Folia Microbiol. (Praha) 59, 155–157. doi: 10.1007/s12223-013-0278-y
Berg, G., Rybakova, D., Fischer, D., Cernava, T., Vergès, M.-C. C., Charles, T., et al. (2020). Microbiome definition re-visited: old concepts and new challenges. Microbiome 8:103. doi: 10.1186/s40168-020-00875-0
Blondel, V. D., Guillaume, J.-L., Lambiotte, R., and Lefebvre, E. (2008). Fast unfolding of communities in large networks. J. Stat. Mech. Theory Exp. 2008:10008. doi: 10.1088/1742-5468/2008/10/p10008
Boch, S., Saiz, H., Allan, E., Schall, P., Prati, D., Schulze, E.-D., et al. (2021). Direct and indirect effects of management intensity and environmental factors on the functional diversity of lichens in central european forests. Microorganisms 9:463. doi: 10.3390/microorganisms9020463
Bolger, A. M., Lohse, M., and Usadel, B. (2014). Trimmomatic: a flexible trimmer for Illumina sequence data. Bioinformatics 30, 2114–2120. doi: 10.1093/bioinformatics/btu170
Brandes, U. (2001). A faster algorithm for betweenness centrality. J. Math. Sociol. 25, 163–177. doi: 10.1080/0022250X.2001.9990249
Callahan, B. J., McMurdie, P. J., Rosen, M. J., Han, A. W., Johnson, A. J. A., and Holmes, S. P. (2016). DADA2: high-resolution sample inference from Illumina amplicon data. Nat. Methods 13, 581–583. doi: 10.1038/nmeth.3869
Camacho-Sanchez, M., Burraco, P., Gomez-Mestre, I., and Leonard, J. A. (2013). Preservation of RNA and DNA from mammal samples under field conditions. Mol. Ecol. Resour. 13, 663–673. doi: 10.1111/1755-0998.12108
Carrión, V. J., Perez-Jaramillo, J., Cordovez, V., Tracanna, V., de Hollander, M., Ruiz-Buck, D., et al. (2019). Pathogen-induced activation of disease-suppressive functions in the endophytic root microbiome. Science 366, 606–612. doi: 10.1126/science.aaw9285
Cheng, T., Xu, C., Lei, L., Li, C., Zhang, Y., and Zhou, S. (2016). Barcoding the kingdom Plantae: new PCR primers for ITS regions of plants with improved universality and specificity. Mol. Ecol. Resour. 16, 138–149. doi: 10.1111/1755-0998.12438
Clark, K., Karsch-Mizrachi, I., Lipman, D. J., Ostell, J., and Sayers, E. W. (2016). GenBank. Nucleic Acids Res. 44, D67–D72. doi: 10.1093/nar/gkv1276
Cooke, W. B. (1959). An ecological life history of Aureobasidium pullulans (de Bary) Arnaud. Mycopathol. Mycol. Appl. 12, 1–45. doi: 10.1007/BF02118435
Crowther, T. W., Glick, H. B., Covey, K. R., Bettigole, C., Maynard, D. S., Thomas, S. M., et al. (2015). Mapping tree density at a global scale. Nature 525, 201–205. doi: 10.1038/nature14967
Csardi, G., and Nepusz, T. (2006). The Igraph Software Package For Complex Network Research. Interjournal Complex Sy, 1695. Available online at: https://igraph.org (accesed January 19, 2022).
Davis, N. M., Proctor, D. M., Holmes, S. P., Relman, D. A., and Callahan, B. J. (2018). Simple statistical identification and removal of contaminant sequences in marker-gene and metagenomics data. Microbiome 6:226. doi: 10.1186/s40168-018-0605-2
Dedysh, S. N., and Dunfield, P. F. (2011). “Facultative and obligate methanotrophs,” in Methods in Methane Metabolism, eds A. C. Rosenzweig and S. W. Ragsdale (Cambridge, MA: Elsevier/Academic Press), 31–44. doi: 10.1016/B978-0-12-386905-0.00003-6
Dymytrova, L. V. (2011). Notes on the genus Scoliciosporum (Lecanorales, Ascomycota) in Ukraine. Polish Bot. J. 56, 61–75.
Erlacher, A., Cernava, T., Cardinale, M., Soh, J., Sensen, C. W., Grube, M., et al. (2015). Rhizobiales as functional and endosymbiontic members in the lichen symbiosis of Lobaria pulmonaria L. Front. Microbiol. 6:53. doi: 10.3389/fmicb.2015.00053
Fernandes, A. D., Macklaim, J. M., Linn, T. G., Reid, G., and Gloor, G. B. (2013). ANOVA-like differential expression (ALDEx) analysis for mixed population RNA-Seq. PLoS One 8:e67019. doi: 10.1371/journal.pone.0067019
Fernandes, A. D., Reid, J. N., Macklaim, J. M., McMurrough, T. A., Edgell, D. R., and Gloor, G. B. (2014). Unifying the analysis of high-throughput sequencing datasets: characterizing RNA-seq, 16S rRNA gene sequencing and selective growth experiments by compositional data analysis. Microbiome 2:15. doi: 10.1186/2049-2618-2-15
Fischer, M., Bossdorf, O., Gockel, S., Hänsel, F., Hemp, A., Hessenmöller, D., et al. (2010). Implementing large-scale and long-term functional biodiversity research: the biodiversity exploratories. Basic Appl. Ecol. 11, 473–485. doi: 10.1016/j.baae.2010.07.009
Frøslev, T. G., Kjøller, R., Bruun, H. H., Ejrnæs, R., Brunbjerg, A. K., Pietroni, C., et al. (2017). Algorithm for post-clustering curation of DNA amplicon data yields reliable biodiversity estimates. Nat. Commun. 8:1188. doi: 10.1038/s41467-017-01312-x
Fruchterman, T. M. J., and Reingold, E. M. (1991). Graph drawing by force-directed placement. Softw. Pract. Exp. 21, 1129–1164. doi: 10.1002/spe.4380211102
Gittleman, J. L., and Kot, M. (1990). Adaptation: statistics and a null model for estimating phylogenetic effects. Syst. Zool. 39:227. doi: 10.2307/2992183
Gloor, G. B., Macklaim, J. M., and Fernandes, A. D. (2016). Displaying variation in large datasets: plotting a visual summary of effect sizes. J. Comput. Graph. Stat. 25, 971–979. doi: 10.1080/10618600.2015.1131161
Gloor, G. B., Macklaim, J. M., Pawlowsky-Glahn, V., and Egozcue, J. J. (2017). Microbiome datasets are compositional: and this is not optional. Front. Microbiol. 8:2224. doi: 10.3389/fmicb.2017.02224
Grube, M., Cernava, T., Soh, J., Fuchs, S., Aschenbrenner, I., Lassek, C., et al. (2015). Exploring functional contexts of symbiotic sustain within lichen-associated bacteria by comparative omics. ISME J. 9, 412–424. doi: 10.1038/ismej.2014.138
Hagge, J., Bässler, C., Gruppe, A., Hoppe, B., Kellner, H., Krah, F.-S., et al. (2019). Bark coverage shifts assembly processes of microbial decomposer communities in dead wood. Proc. R. Soc. B Biol. Sci. 286:20191744. doi: 10.1098/rspb.2019.1744
Herlemann, D. P., Labrenz, M., Jürgens, K., Bertilsson, S., Waniek, J. J., and Andersson, A. F. (2011). Transitions in bacterial communities along the 2000 km salinity gradient of the Baltic Sea. ISME J. 5, 1571–1579. doi: 10.1038/ismej.2011.41
Hiraishi, A., and Imhoff, J. F. (2015). “Acidiphilium,” in Bergey’s Manual of Systematics of Archaea and Bacteria, eds M. E. Trujillo, S. Dedysh, P. DeVos, B. Hedlund, P. Kämpfer, F. A. Rainey, et al. (Hoboken, NJ: Wiley), 1–14. doi: 10.1002/9781118960608.gbm00877
Ihrmark, K., Bödeker, I. T. M., Cruz-Martinez, K., Friberg, H., Kubartova, A., Schenck, J., et al. (2012). New primers to amplify the fungal ITS2 region - evaluation by 454-sequencing of artificial and natural communities. FEMS Microbiol. Ecol. 82, 666–677. doi: 10.1111/j.1574-6941.2012.01437.x
Kahl, T., and Bauhus, J. (2014). An index of forest management intensity based on assessment of harvested tree volume, tree species composition and dead wood origin. Nat. Conserv. 7, 15–27. doi: 10.3897/natureconservation.7.7281
Keuschnig, C., Martins, J. M. F., Navel, A., Simonet, P., and Larose, C. (2021). Micro-aggregation of a pristine grassland soil selects for bacterial and fungal communities and changes in nitrogen cycling potentials. bioRxiv [Preprint] bioRxiv: 2021.10.13.464334, doi: 10.1101/2021.10.13.464334
Kobayashi, K., and Aoyagi, H. (2019). Microbial community structure analysis in Acer palmatum bark and isolation of novel bacteria IAD-21 of the candidate division FBP. PeerJ 7:e7876. doi: 10.7717/peerj.7876
Kulichevskaya, I. S., Ivanova, A. A., Detkova, E. N., Rijpstra, W. I. C., Sinninghe Damsté, J. S., and Dedysh, S. N. (2017). Tundrisphaera lichenicola gen. nov., sp. nov., a psychrotolerant representative of the family Isosphaeraceae from lichen-dominated tundra soils. Int. J. Syst. Evol. Microbiol. 67, 3583–3589. doi: 10.1099/ijsem.0.002172
Kurtz, Z. D., Müller, C. L., Miraldi, E. R., Littman, D. R., Blaser, M. J., and Bonneau, R. A. (2015). Sparse and compositionally robust inference of microbial ecological networks. PLoS Comput. Biol. 11:e1004226. doi: 10.1371/journal.pcbi.1004226
Lahti, L., and Shetty, S. (2017). Microbiome – Tools For Microbiome Analysis In R. Available online at: https://github.com/microbiome/microbiome (accesed January 19, 2022).
Lambais, M. R., Lucheta, A. R., and Crowley, D. E. (2014). Bacterial community assemblages associated with the phyllosphere, dermosphere, and rhizosphere of tree species of the atlantic forest are host taxon dependent. Microb. Ecol. 68, 567–574. doi: 10.1007/s00248-014-0433-2
Lamit, L. J., Lau, M. K., Næsborg, R. R., Wojtowicz, T., Whitham, T. G., and Gehring, C. A. (2015). Genotype variation in bark texture drives lichen community assembly across multiple environments. Ecology 96, 960–971. doi: 10.1890/14-1007.1
Leff, J. W., Del Tredici, P., Friedman, W. E., and Fierer, N. (2015). Spatial structuring of bacterial communities within individual Ginkgo biloba trees. Environ. Microbiol. 17, 2352–2361. doi: 10.1111/1462-2920.12695
Lindow, S. E., and Brandl, M. T. (2003). Microbiology of the phyllosphere. Appl. Environ. Microbiol. 69, 1875–1883. doi: 10.1128/AEM.69.4.1875-1883.2003
Łubek, A., Kukwa, M., Jaroszewicz, B., and Czortek, P. (2020). Identifying mechanisms shaping lichen functional diversity in a primeval forest. For. Ecol. Manage. 475:118434. doi: 10.1016/j.foreco.2020.118434
Ma, J., Tang, J. Y., Wang, S., Chen, Z. L., Li, X. D., and Li, Y. H. (2017). Illumina sequencing of bacterial 16S rDNA and 16S rRNA reveals seasonal and species-specific variation in bacterial communities in four moss species. Appl. Microbiol. Biotechnol. 101, 6739–6753. doi: 10.1007/s00253-017-8391-5
Martin, M. (2011). Cutadapt removes adapter sequences from high-throughput sequencing reads. EMBnet J. 17:10. doi: 10.14806/ej.17.1.200
Martins, G., Lauga, B., Miot-Sertier, C., Mercier, A., Lonvaud, A., Soulas, M.-L., et al. (2013). Characterization of epiphytic bacterial communities from grapes, leaves, bark and soil of grapevine plants grown, and their relations. PLoS One 8:e73013. doi: 10.1371/journal.pone.0073013
McMurdie, P. J., and Holmes, S. (2013). phyloseq: an r package for reproducible interactive analysis and graphics of microbiome census data. PLoS One 8:e61217. doi: 10.1371/journal.pone.0061217
McMurdie, P. J., and Holmes, S. (2014). Waste Not, want not: why rarefying microbiome data is inadmissible. PLoS Comput. Biol. 10:e1003531. doi: 10.1371/journal.pcbi.1003531
Meinshausen, N., and Bühlmann, P. (2006). High-dimensional graphs and variable selection with the Lasso. Ann. Stat. 34, 1436–1462. doi: 10.1214/009053606000000281
Menke, S., Gillingham, M. A. F., Wilhelm, K., and Sommer, S. (2017). Home-made cost effective preservation buffer is a better alternative to commercial preservation methods for microbiome research. Front. Microbiol. 8:102. doi: 10.3389/fmicb.2017.00102
Müller, C. L., Bonneau, R., and Kurtz, Z. (2016). Generalized Stability Approach for Regularized Graphical Models. Available online at: http://arxiv.org/abs/1605.07072 (accesed January 19, 2022).
Muyzer, G., de Waal, E. C., and Uitterlinden, A. G. (1993). Profiling of complex microbial populations by denaturing gradient gel electrophoresis analysis of polymerase chain reaction-amplified genes coding for 16S rRNA. Appl. Environ. Microbiol. 59, 695–700. doi: 10.1128/aem.59.3.695-700.1993
Nascimbene, J., Thor, G., and Nimis, P. L. (2013). Effects of forest management on epiphytic lichens in temperate deciduous forests of Europe – a review. For. Ecol. Manage. 298, 27–38. doi: 10.1016/j.foreco.2013.03.008
Newman, M. E. J. (2006). Modularity and community structure in networks. Proc. Natl. Acad. Sci. U.S.A. 103, 8577–8582. doi: 10.1073/pnas.0601602103
Nguyen, L. H., and Holmes, S. (2019). Ten quick tips for effective dimensionality reduction. PLoS Comput. Biol. 15:e1006907. doi: 10.1371/journal.pcbi.1006907
Normand, P., and Benson, D. R. (2015). “Kineococcus,” in Bergey’s Manual of Systematics of Archaea and Bacteria, eds M. E. Trujillo, S. Dedysh, P. DeVos, B. Hedlund, P. Kämpfer, F. A. Rainey, et al. (Hoboken, NJ: Wiley), 1–5. doi: 10.1002/9781118960608.gbm00053
Oksanen, J., Blanchet, F. G., Friendly, M., Kindt, R., Legendre, P., McGlinn, D., et al. (2020). vegan: Community Ecology Package. Available online at: https://cran.r-project.org/package=vegan (accesed January 19, 2022).
Paradis, E., and Schliep, K. (2019). ape 5.0: an environment for modern phylogenetics and evolutionary analyses in R. Bioinformatics 35, 526–528. doi: 10.1093/bioinformatics/bty633
Petrolli, R., Augusto Vieira, C., Jakalski, M., Bocayuva, M. F., Vallé, C., Cruz, E. D. S., et al. (2021). A fine-scale spatial analysis of fungal communities on tropical tree bark unveils the epiphytic rhizosphere in orchids. New Phytol. 231, 2002–2014. doi: 10.1111/nph.17459
Quast, C., Pruesse, E., Yilmaz, P., Gerken, J., Schweer, T., Yarza, P., et al. (2012). The SILVA ribosomal RNA gene database project: improved data processing and web-based tools. Nucleic Acids Res. 41, D590–D596. doi: 10.1093/nar/gks1219
R Core Team (2021). R: A Language and Environment for Statistical Computing. Available online at: https://www.r-project.org/ (accesed January 19, 2022).
Rindi, F. (2007). “Diversity, distribution and ecology of green algae and cyanobacteria in urban habitats,” in Algae and Cyanobacteria in Extreme Environments, ed. J. Seckbach (Dordrecht: Springer Netherlands), 619–638. doi: 10.1007/978-1-4020-6112-7_34
Romani, M., Carrion, C., Fernandez, F., Intertaglia, L., Pecqueur, D., Lebaron, P., et al. (2019). High bacterial diversity in pioneer biofilms colonizing ceramic roof tiles. Int. Biodeterior. Biodegradation 144:104745. doi: 10.1016/j.ibiod.2019.104745
RStudio Team (2021). RStudio: Integrated Development Environment for R. Available online at: http://www.rstudio.com/ (accesed January 19, 2022).
Sanders, W. B., and Masumoto, H. (2021). Lichen algae: the photosynthetic partners in lichen symbioses. Lichenology 53, 347–393.
Schall, P., Heinrichs, S., Ammer, C., Ayasse, M., Boch, S., Buscot, F., et al. (2020). Can multi-taxa diversity in European beech forest landscapes be increased by combining different management systems? J. Appl. Ecol. 57, 1363–1375. doi: 10.1111/1365-2664.13635
Schnell, I. B., Bohmann, K., and Gilbert, M. T. P. (2015). Tag jumps illuminated - reducing sequence-to-sample misidentifications in metabarcoding studies. Mol. Ecol. Resour. 15, 1289–1303. doi: 10.1111/1755-0998.12402
Schoch, C. L., Robbertse, B., Robert, V., Vu, D., Cardinali, G., Irinyi, L., et al. (2014). Finding needles in haystacks: linking scientific names, reference specimens and molecular data for Fungi. Database 2014:bau061. doi: 10.1093/database/bau061
Shannon, C. E. (1948). A mathematical theory of communication. Bell Syst. Tech. J. 27, 379–423. doi: 10.1002/j.1538-7305.1948.tb01338.x
Škaloud, P., Friedl, T., Hallmann, C., Beck, A., and Dal Grande, F. (2016). Taxonomic revision and species delimitation of coccoid green algae currently assigned to the genus Dictyochloropsis (Trebouxiophyceae, Chlorophyta). J. Phycol. 52, 599–617. doi: 10.1111/jpy.12422
Smith, H. B. Dal Grande, F., Muggia, L., Keuler, R., Divakar, P. K., Grewe, F., et al. (2020). Metagenomic data reveal diverse fungal and algal communities associated with the lichen symbiosis. Symbiosis 82, 133–147. doi: 10.1007/s13199-020-00699-4
Štifterová, A., and Neustupa, J. (2015). Community structure of corticolous microalgae within a single forest stand: evaluating the effects of bark surface pH and tree species. Fottea 15, 113–122. doi: 10.5507/fot.2015.013
Strid, Y., Schroeder, M., Lindahl, B., Ihrmark, K., and Stenlid, J. (2014). Bark beetles have a decisive impact on fungal communities in Norway spruce stem sections. Fungal Ecol. 7, 47–58. doi: 10.1016/j.funeco.2013.09.003
Tahon, G., Tytgat, B., Lebbe, L., Carlier, A., and Willems, A. (2018). Abditibacterium utsteinense sp. nov., the first cultivated member of candidate phylum FBP, isolated from ice-free Antarctic soil samples. Syst. Appl. Microbiol. 41, 279–290. doi: 10.1016/j.syapm.2018.01.009
Teunisse, G. M. (2017). Fantaxtic – Fantaxtic Plots For Phyloseq Objects!. Available online at: https://github.com/gmteunisse/Fantaxtic (accesed January 19, 2022).
Untereiner, W. A., and Malloch, D. (1999). Patterns of substrate utilization in species of Capronia and allied black yeasts: ecological and taxonomic implications. Mycologia 91, 417–427. doi: 10.1080/00275514.1999.12061035
Unterseher, M., Siddique, A. B., Brachmann, A., and Peršoh, D. (2016). Diversity and composition of the leaf mycobiome of beech (Fagus sylvatica) are affected by local habitat conditions and leaf biochemistry. PLoS One 11:e0152878. doi: 10.1371/journal.pone.0152878
Větrovský, T., Baldrian, P., and Morais, D. (2018). SEED 2: A user-friendly platform for amplicon high-throughput sequencing data analyses. Bioinformatics 34, 2292–2294. doi: 10.1093/bioinformatics/bty071
Vieira, S., Sikorski, J., Dietz, S., Herz, K., Schrumpf, M., Bruelheide, H., et al. (2020). Drivers of the composition of active rhizosphere bacterial communities in temperate grasslands. ISME J. 14, 463–475. doi: 10.1038/s41396-019-0543-4
Vitulo, N., Lemos, W. J. F., Calgaro, M., Confalone, M., Felis, G. E., Zapparoli, G., et al. (2019). Bark and grape microbiome of vitis vinifera: influence of geographic patterns and agronomic management on bacterial diversity. Front. Microbiol. 9:3203. doi: 10.3389/fmicb.2018.03203
Vorholt, J. A. (2012). Microbial life in the phyllosphere. Nat. Rev. Microbiol. 10, 828–840. doi: 10.1038/nrmicro2910
White, T. J., Bruns, T., Lee, S., and Taylor, J. (1990). “Amplification and direct sequencing of fungal ribosomal rna genes for phylogenetics,” in PCR Protocols, eds M. Innis, D. H. Gelfland, J. J. Sninsky, and T. J. White (San Diego, CA: Academic Press), 315–322. doi: 10.1016/b978-0-12-372180-8.50042-1
Whitmore, T. C. (1963). Studies in systematic bark morphology. IV. The bark of beech, oak and sweet chestnut. New Phytol. 62, 161–169. doi: 10.1111/j.1469-8137.1963.tb06323.x
Yamamura, H., Ashizawa, H., Nakagawa, Y., Hamada, M., Ishida, Y., Otoguro, M., et al. (2011). Actinomycetospora iriomotensis sp. nov., a novel actinomycete isolated from a lichen sample. J. Antibiot. (Tokyo) 64, 289–292. doi: 10.1038/ja.2011.15
Zhu, H., Li, S., Hu, Z., and Liu, G. (2018). Molecular characterization of eukaryotic algal communities in the tropical phyllosphere based on real-time sequencing of the 18S rDNA gene. BMC Plant Biol. 18:365. doi: 10.1186/s12870-018-1588-7
Keywords: algae, bacteria, biofilm, community ecology, dermosphere, forest management, fungi, metabarcoding
Citation: Dreyling L, Schmitt I and Dal Grande F (2022) Tree Size Drives Diversity and Community Structure of Microbial Communities on the Bark of Beech (Fagus sylvatica). Front. For. Glob. Change 5:858382. doi: 10.3389/ffgc.2022.858382
Received: 19 January 2022; Accepted: 08 April 2022;
Published: 16 May 2022.
Edited by:
Paolo Giordani, University of Genoa, ItalyReviewed by:
Patricia Moya, University of Valencia, SpainAndreas Beck, Botanische Staatssammlung München, Germany
Copyright © 2022 Dreyling, Schmitt and Dal Grande. This is an open-access article distributed under the terms of the Creative Commons Attribution License (CC BY). The use, distribution or reproduction in other forums is permitted, provided the original author(s) and the copyright owner(s) are credited and that the original publication in this journal is cited, in accordance with accepted academic practice. No use, distribution or reproduction is permitted which does not comply with these terms.
*Correspondence: Lukas Dreyling, bHVrYXMuZHJleWxpbmdAc2VuY2tlbmJlcmcuZGU=; Francesco Dal Grande, ZnJhbmNlc2NvLmRhbGdyYW5kZUB1bmlwZC5pdA==