- 1Department of Plant, Soil and Microbial Sciences, Michigan State University, East Lansing, MI, United States
- 2Ecology, Evolution, and Behavior, Michigan State University, East Lansing, MI, United States
- 3Department of Biological Sciences, Western Michigan University, Kalamazoo, MI, United States
Wildfire and burn severity influence soil microbial communities during post-fire recovery. If post-fire differences in microbial communities affect soil carbon (C) pool dynamics, altered microbial communities could influence the transition of forests from C sources to C sinks during ecosystem recovery. For example, fire may change the abundance of copiotrophic and oligotrophic bacteria, influencing the kinetic rates of soil C pools due to differences in C-acquisition strategies and nutrient requirements. We assessed differences in soil bacterial communities and soil C pool kinetics 1 year after a wildfire in a mixed-conifer forest in northern California, United States. We determined whether differences in bacterial communities and soil C pools were related to copiotrophic versus oligotrophic life history strategies. Specifically, we assessed bacterial community oligotrophy versus copiotrophy based on phyla relative abundances and predicted 16S gene copy numbers. We then determined whether these life-histories were correlated with C pool kinetic rates. We further determined whether C degradation metabolic pathways predicted using PICRUSt2 were related to C pool kinetics. We found that copiotrophic bacteria exhibited greater abundance in burned areas than unburned areas, evidenced by increases in 16S rRNA gene copy number and by taxonomic classifications. Furthermore, the abundance of predicted metabolic pathways associated with fast-cycling C compounds increased with severity, including carbohydrate, alcohol, and amine degradation pathways, suggesting increased copiotrophic metabolic strategies. In contrast, the abundance of metabolic pathways of slow-cycling aromatic C compounds did not change, indicating oligotrophic metabolic strategies did not increase. The kinetic rate of the active C pool was positively related to the copiotrophic metabolic pathway of alcohol degradation, and negatively related to oligotrophic pathways like aromatic compound degradation. The links between C pool kinetics and phylum-level life-strategy classifications were ambiguous. Overall, our results suggest that metabolic life-strategies are related to soil C pool kinetics and could have short- and long-term impacts on soil C persistence during post-fire recovery. In the short-term, increased copiotrophy could increase soil C efflux via rapid cycling of labile C pools. However, over the longer-term lower prevalence of oligotrophic strategies could allow aromatic compounds associated with pyrogenic C to accumulate, increasing stable soil C stocks.
Introduction
Wildfire frequency and severity have increased in the forests of the western United States over the past several decades (Westerling, 2006; Dennison et al., 2014), a trend that is predicted to continue (Flannigan et al., 2009; Kitzberger et al., 2017; Podschwit et al., 2018). Increased burn severity influences soil carbon (C) cycling for years to decades post-fire by altering soil C pool structure, efflux rates, and decomposition (Holden et al., 2016; Adkins et al., 2019; Dove et al., 2020). Understanding drivers of post-fire soil C cycling is important because the balance between soil C efflux and photosynthetic C gains governs the transition of ecosystems from C sources to C sinks during ecosystem recovery (Kashian et al., 2006). Because soil microbes drive the soil C cycle, it is important to understand how microbial communities are affected by wildfire.
The amount of C respired versus retained in soils is dependent on the structure and stability of soil C pools (Trumbore, 2000; Kuzyakov, 2011; Torn et al., 2013). Soil C can be conceptualized as distinct C pools with variable turnover times: an active C pool (Ca) with a mean residence time of days to months and a non-active pool (Cs) with a mean residence time of years to decades (Paul et al., 2006). The sizes of the active and non-active C pools and their associated kinetic rates (ka and ks, respectively) can be quantified by measuring soil C respiration over long-term lab incubations (Collins et al., 2000; Kuzyakov, 2011). In this approach, C pools are a function of biological processes rather than intrinsic chemical characteristics (see Schmidt et al., 2011). Microbial activity therefore dictates soil C pool kinetics, and microbial community structure has been shown to be predictive of C pool kinetics in some ecosystems (e.g., Alaskan tundra soils) (Hale et al., 2019). However, whether differences in microbial community structure influence the kinetics of the active and non-active C pools during post-fire recovery of temperate forests is unknown.
Disturbance-induced changes to microbial communities could alter ecosystem processes if the microbial community is not resistant or resilient to disturbance or not functionally redundant with the pre-disturbance community (Allison and Martiny, 2008; Treseder et al., 2012). In the case of fires, microbial community composition in forest soil is often not resistant or resilient over the short- to intermediate-term, as soil microbiomes exhibit decreases in diversity and changes in community composition that persist from months to years post-fire (Weber et al., 2014; Rodríguez et al., 2017, 2018; Whitman et al., 2019; Adkins et al., 2020; Sáenz de Miera et al., 2020). Post-fire soil microbial communities appear to be functionally distinct, becoming predominated by more copiotrophic bacterial taxa compared to oligotrophic taxa in a variety of ecosystems across the globe, including evergreen shrublands, eucalypt forests, temperate mixed-conifer forests, and boreal conifer forests in both North America and Eurasia (Pérez-Valera et al., 2019; Whitman et al., 2019; Adkins et al., 2020; Ling et al., 2021; Ammitzboll et al., 2022). Further increased post-fire predominance of copiotrophs appears to scale with burn severity (Ferrenberg et al., 2013; Adkins et al., 2020), although the impacts of severity on bacterial life strategy has not been extensively studied.
The classification of bacterial taxa as copiotrophic or oligotrophic represents a trait-based framework for describing bacterial community structure (Fierer, 2017), and these taxa may differ in their effects on the soil C cycle. Copiotrophs rely on labile C for efficient growth, have high nutrient requirements, and exhibit high growth rates. In contrast, oligotrophs exhibit slow growth rates, but have high substrate affinity and may outcompete copiotrophs when nutrient content and/or organic matter quality is low (Fierer et al., 2007; Ramirez et al., 2012). These ecological strategies are reflected at the gene level. For example, high numbers of 16S rRNA gene copies harbored within a bacteria species is reflective of copiotrophic traits like high growth rate and low C use efficiency (Roller et al., 2016; Romillac and Santorufo, 2021). Furthermore, metagenomic and metabolic approaches have revealed that soils abundant in copiotrophs harbor more genes associated with carbohydrate utilization and protein degradation, and fewer genes and enzymes associated with recalcitrant C degradation, organic N decomposition, and P scavenging (Fierer et al., 2012; Ramirez et al., 2012; Hartman et al., 2017). The combined influences of oligotroph vs. copiotroph abundance, nutrient content, and organic matter quality may thus alter soil C pool kinetics. The links between microbial life strategy and preferred C substrates may be particularly relevant in burned ecosystems because fire can increase soil pyrogenic C (PyC), a spectrum of fire affected C that is often enriched in aromatic rings that likely require oligotrophic microbes for decomposition (Bird et al., 2015).
Consistent patterns have emerged in post-fire differences in bacterial community composition among many ecosystem types, and these differences are often reflected by divergent responses of oligotrophic and copiotrophic taxa to fire and burn severity. The putative copiotrophic phyla Bacteroidetes, Actinobacteria, and Firmicutes tend to exhibit higher abundance post-fire, whereas the putative oligotrophic phylum Acidobacteria exhibits lower abundance (Weber et al., 2014; Xiang et al., 2014; Pérez-Valera et al., 2019; Whitman et al., 2019). Changes in bacterial phyla abundance are likely due to a combination of direct and indirect effects of fire on soils. For example, spore-forming, heat resistant taxa such as Actinobacteria and Firmicutes are more likely to survive soil heating events (Prendergast-Miller et al., 2017). These and other copiotrophic phyla (e.g., Bacteroidetes, Proteobacteria) may also positively respond to the fire-induced increases in total inorganic nitrogen (TIN) and dissolved organic C (Fernández et al., 1997; Wan et al., 2001; Wang et al., 2012). Changes to both microbial communities and nutrient availability have been demonstrated to scale with burn severity in mixed-conifer forests (Adkins et al., 2019, 2020); thus, C-cycling processes that are driven by microbial community structure can be expected to vary with burn severity. Although a few studies have accounted for the role of burn severity in shaping microbial communities (Weber et al., 2014; Whitman et al., 2019; Adkins et al., 2020; Sáenz de Miera et al., 2020), most have not, and identifying the influence of severity on post-fire microbial communities has been identified as a key information need in fire ecology research (Hart et al., 2005; Pressler et al., 2018). Understanding the relationship between severity, bacterial life strategy, and soil C pools may be of particular importance because over short to intermediate timescales post-fire, copiotrophic bacteria increase with severity (Adkins et al., 2020), while the slow cycling soil C pool increases and the fast-cycling (labile) soil C pool decreases or remains unchanged (Adkins et al., 2019). Thus, fire may cause community-scale bacterial life strategy to become uncoupled from the C pools most suitable for supporting that strategy, an effect may increase with severity and result in changes to the soil C cycle.
The numerous interacting direct and indirect effects of fire and burn severity on soil and bacterial properties highlights the importance of employing a systems approach to understanding disturbance effects on the soil C cycle. Understanding the relationships between soil characteristics and the C cycle will improve predictions of the impacts of changing fire regimes on the C sink strength of forests, and understanding the influence of bacterial communities on soil functions is necessary for improving ecosystem models (Schimel and Schaeffer, 2012; Treseder et al., 2012; Graham et al., 2016). Here, our overarching objectives are to (1) determine how C pool structure and kinetics are related to soil properties and bacterial communities 1-year post-fire, and (2) how the oligotroph-copiotroph framework can explain post-fire differences in soil bacterial communities. In service of these objectives, we addressed four hypotheses. We hypothesized that (1) changes in C pool structure and kinetics across a burn severity gradient can be explained by accounting for soil properties; (2) bacterial OTUs that have previously been identified as positive fire responders will be positively correlated with burn severity; (3) copiotrophic bacteria abundance will be positively correlated with burn severity and therefore positively related to nutrient availability and labile C availability; and (4) copiotrophic bacterial life strategies will be positively associated with ka, whereas oligotrophic strategies will be positively associated with ks.
Materials and Methods
Site Description
Our study was conducted in mixed-conifer forest in the Klamath National Forest, located in northern California, United States. The forest type is a California mixed-conifer forest (Ruefenacht et al., 2008), which consists of Pinus ponderosa Lawson & C. Lawson, P. lambertiana Douglas, P. jeffreyi Balf., Abies concolor (Gord. & Glend.) Lindl. ex Hildebr., Pseudotsuga menziesii (Mirb.) Franco, Calocedrus decurrens (Torr.) Florin, Arbutus menziesii Pursh., and Quercus kelloggii Newberry. Soils of our study area belong to the Skalan series and its associates (Soil Survey Staff et al., 2015); Skalan is a loamy-skeletal, isotic, mesic Vitrandic Haploxeralf that forms in weathered gneiss residuum and is slightly acidic (Soil Survey Staff et al., 2016). The mean annual precipitation is 1,290 mm and mean annual temperature is 9.0°C (NCEI-NOAA, 2017). Our study focuses on areas affected by the Beaver Fire (Lat: 41.88993, Long: −122.87056; Supplementary Figure 1), a wildfire that burned ∼13,000 ha between July 30, 2014 to August 31, 2014. Burn severity estimates based on the Differenced Normalized Burn Ratio (dNBR) indicate that the Beaver Fire resulted in ∼4,800 ha of burned area classified as high severity, ∼4,600 ha classified as moderate severity, ∼3,700 ha classified as low severity, and ∼750 ha within the fire perimeter was unburned (MTBS, 2017). dNBR severity estimates are based on Landsat reflectance images collected in the growing seasons immediately before and after fire occurrence, and reflect post- versus pre-fire changes in vegetation and soil exposure (Parsons et al., 2010), resulting in continuous dNBR values assigned to both burned and unburned plots. dNBR values for unburned plots are typically < 100, whereas the upper limit for burned plots can exceed 1,000.
Field Methods
Between August 3, 2015 and August 10, 2015 (i.e., 1-year post-fire), we sampled 10 plots (4 unburned, 6 burned; dNBR 0–863). At each plot, we determined live and dead tree basal area and sampled for forest floor and mineral soil. We measured tree diameters at breast height (DBH) for all live and dead trees > 10 cm DBH within an 11.3 m sampling radius, and we used these values to calculate live and dead tree basal area at the plot level. We sampled forest floor and mineral soil 15 m from the plot center at azimuths of 0°, 90°, 180° and 270°, for a total of 40 forest floor and 40 mineral soil samples collected. The forest floor includes the plant litter and duff layers, and is equivalent to the combined Oi, Oe, and Oa horizons in the USDA Soil Taxonomy classification system (Perry et al., 2008). We collected all forest floor material from within a 15 cm radius circular sampling frame. We inserted a 5 cm radius metal cylinder into the mineral soil to 5 cm and collected mineral soil using a stainless-steel scoop. We collected one additional volumetric mineral soil sample from the center of each plot to estimate bulk density using the same sampling method. Forest floor samples were stored at ambient temperature for ∼14 days prior to being transported to the lab. Mineral soil samples were stored under refrigeration for 2–7 days and shipped to the lab on ice. Upon arrival at the lab, the forest floor samples were air-dried, and mineral soils were sieved (2 mm) and sub-sampled for DNA analysis. Sub-samples for DNA analysis were stored at −80°C, and the remainder of the mineral soils were refrigerated at ∼4°C until analyses.
Lab Methods
Soil Processing and Chemical Analyses
We processed the forest floor in a blender to pass a 2 mm mesh screen and then pulverized a subsample of the blended material in a ball mill (SPEX Sample Prep LLC, Metuchen, NJ, United States). We oven-dried the pulverized forest floor material at 65°C prior to determination of total C and N. We used the sieved mineral soil for determination of total C, N, pyrogenic-C (PyC), NO3-N, NH4-N, extractable P, and pH. We oven-dried the mineral soil sample to be used for C and N analysis at 105°C and then pulverized the subsamples as described above. We analyzed one forest floor sample and one mineral soil sample from each subplot for total C and N concentrations on a dry combustion elemental analyzer (Costech Analytical Technologies Inc., Valencia, CA, United States), using atropine as the quantification standard. We quantified mineral soil PyC concentrations by digesting 0.5 g mineral soil from each subplot in 10 mL 1.0 M HNO3 + 20 mL 30% H2O2 at 100°C for 16 h (Kurth et al., 2006) and analyzing post-digested soils for residual C and N.
We extracted a 10 g sample of fresh mineral soil from each subplot for NH4+-N and NO3–-N in 50 mL 2.0 M KCl for 1 h. We filtered the resulting soil extracts through 2.5 μm pore-size filter paper (GE Healthcare UK Limited, Little Chalfont, Buckinghamshire, United Kingdom). We determined the extract NH4+ concentrations spectrophotometrically by reacting with ammonia salicylate and ammonia cyanurate (Sinsabaugh et al., 2000), and measuring absorbance at 595 nm (BioTek Elx800, BioTek Instruments Inc., Winooski, VT, United States). We determined the concentrations of NO3– in the extracts spectrophotometrically by reacting the extracts with vanadium (III) chloride, sulfanilamide, and N-(1-naphthyl)-ethylenediamine dihydrochloride and measuring absorbance at 540 nm (Doane and Horwáth, 2003). We extracted 2.5 g subsamples of fresh mineral soils for phosphorus (P) in 40 mL 0.5 M NaHCO3 and determined P concentration using the Olsen method (Olsen et al., 1954). We measured mineral soil pH of a 1:2 (w:v) soil slurry with a benchtop pH meter (Oakton pH 700, Oakton Instruments, Vernon Hills, IL, United States).
We isolated the light particulate organic matter (LPOM) fraction from mineral soil to determine SOM composition via diffuse reflectance Fourier transform infrared (DRIFT) spectroscopy. To collect LPOM, we first dispersed mineral soil in DI water via sonication. We then passed the soil solution through a 53 μm sieve, followed by isolation of LPOM via flotation in 1.7 g cm–3 sodium iodide solution. We then pulverized the LPOM fraction using mortar and pestle. We scanned undiluted LPOM samples in aluminum 96 well-plates in diffuse reflectance mode using a mid-infrared spectrometer (Vertex 70, Bruker Scientific LLC, Billerica, MD, United States). We scanned from 4,000 to 400 cm–1 with a resolution of 4 cm–1 and 64 scans per spectrum. Background spectra from empty wells were collected with every plate. We background-subtracted and baseline corrected the resulting spectra in OPUS 7.5 (Bruker Scientific) and calculated the relative areas of peaks indicative of aliphatic (2,930–2,870 cm–1), carbohydrate (1,160–1,040 cm–1), aromatic (1,590–1,570 cm–1, 1,550–1,500 cm–1, 975–700 cm–1), and amide (1,450–1,400 cm–1, 1,320–1,220 cm–1) compounds (Calderón et al., 2011). We considered the sum of aliphatic and carbohydrate peak areas as a proxy for labile C.
Determination of Carbon Pool Structure and Kinetics
We incubated soils to determine the size and kinetic rates of the Ca and Cs pools and potential soil C flux rates (Paul et al., 2001). We adjusted a 30 g sample of fresh mineral soil from each subplot to 40% water filled pore space (WFPS) in 120 mL specimen cups. The specimen cups were placed in 1 L glass jars, and the soils were incubated in the dark for 300 days at ambient temperature (∼23°C) with biweekly adjustments of soil moisture to 40% WFPS. We measured CO2 evolution on days 10, 14, 28, 42, 58, 90, and every 30 days thereafter until day 300. Prior to each measurement event, we flushed the jars to ambient CO2 concentrations, then tightly sealed the jars for 24–48 h before sampling a 1 mL gas aliquot through septa fitted to the jar lids. We measured CO2 concentration of the aliquot using an infrared gas analyzer (LI-COR Inc., Lincoln, NE, United States), and calculated CO2-C efflux as the difference in CO2-C concentrations between the soil containing jars and blank jars that contained only a specimen cup and DI water.
DNA Extraction and Bioinformatic Analysis
We extracted DNA from 0.25 g of mineral soil from each of the 40 subplots using the MoBIO PowerSoil DNA isolation kit (MoBIO laboratories, Carlsbad, CA, United States), according to the manufacturer’s instructions. The extracted DNA was amplified using PCR with barcoded 515f/806r universal primers of the V4 region of the 16S-rRNA gene (Caporaso et al., 2010). Samples were sequenced on the Illumina MiSeq platform using the v2 500 cycle Reagent Kit at the Michigan State University Genomics Core. We processed DNA sequences using the QIIME2 bioinformatics pipeline (Bolyen et al., 2019). We denoised, merged forward and reverse reads, and removed chimeras using the q2-DADA2 plugin (Callahan et al., 2016). After sequence processing there were 20,815–67,314 sequences per sample (mean = 36,766). We did not rarefy our samples because rarefying decreases statistical power and is not recommended when library sizes vary by less than 10-fold (McMurdie and Holmes, 2014; Weiss et al., 2017). We inferred phylogenetic trees by applying MAFFT multiple sequence alignment (Katoh and Standley, 2013) and FastTree 2 (Price et al., 2010) using the q2-phylogeny plugin. With the q2-diversity plugin, we used the phylogenetic tree to calculate OTU richness, Faith’s phylogenetic diversity (Faith, 1992), and a weighted UniFrac distance matrix (Lozupone and Knight, 2005). We used the q2-feature-classifier plugin (Bokulich et al., 2018) with the classify_sklearn action (Pedregosa et al., 2011) to classify taxonomic composition of our samples employing a Naïve Bayes classifier trained on the SILVA SSURef database version 132 using a 99% similarity threshold (Quast et al., 2013). We filtered OTUs associated with Archaea, Eukaryotes, mitochondria, and chloroplasts. We calculated oligotroph-to-copiotroph ratio at the phylum level as the ratio of the sum of relative abundances of all taxa classified within the phyla Acidobacteria and Verrucomicrobia to the sum of the relative abundances of all taxa classified within the phyla Actinobacteria, Firmicutes, and Bacteroidetes (Fierer et al., 2007, 2012; Ramirez et al., 2012). We predicted 16S rRNA gene copy numbers using the ribosomal RNA operon database (rrnDB) (Stoddard et al., 2015). For each OTU, we assigned 16S gene copy number as the genus mean; if a genus was not represented in rrnDB we assigned the family mean and so on up taxonomic levels until all OTUs were assigned a 16S gene copy number value. We then calculated the abundance-weighted mean 16S gene copy number for each bacterial community as another indicator of community-scale copiotrophy (Nemergut et al., 2016). This approach of predicting 16S gene copy numbers based on databases has frequently been used as an indicator of community-scale life-strategy (Nemergut et al., 2016; Roller et al., 2016; Kurm et al., 2017; Wu et al., 2017; Li et al., 2021), but these estimates can be biased based on reference genomes in the database and should thus be interpreted with caution (Louca et al., 2018).
We predicted metagenomic functional potential of soil bacterial communities using the PICRUSt2 (Phylogenetic Investigation of Communities by Reconstruction of Unobserved States) software (Douglas et al., 2019). PICRUSt2 uses HMMER software (hmmer.org) to perform multiple sequence alignment via hidden Markov models, places reads in a reference tree using EPA-ng (Barbera et al., 2018), and outputs a tree file with Gappa (Czech et al., 2020). PICRUSt2 then performs hidden-state prediction of gene families (Louca and Doebeli, 2017), and uses MinPath (Ye and Doak, 2009) to infer the relative abundance of MetaCyc metabolic pathways (Caspi et al., 2018). MetaCyc is a metabolic pathway database in which pathways are hierarchically grouped according to metabolic function. We focused our analysis on degradation pathway groups related to C cycling, including carbohydrate degradation, alcohol degradation, amine degradation, amino acid degradation, aromatic compound degradation, carboxylate degradation, fatty acid and lipid degradation, nucleoside and nucleotide degradation, and secondary metabolite degradation pathways. PICRUSt2 calculates weighted nearest sequenced taxon index (NSTI), which is an indicator of the average phylogenetic distance of bacteria within an OTU table compared to genomes in the reference database, and is correlated with accuracy of metagenome predictions (Langille et al., 2013). The mean weighted NSTI for our samples was 0.12 (SD = 0.04), which is similar or better to that in other soil studies (Canarini et al., 2021; Toole et al., 2021; Wang et al., 2021), and indicates that metagenomic functional profiles can be accurately predicted in our dataset.
Statistical Analysis
Fire and Severity Effects on Soil Properties and Tree Basal Area
We performed all statistical analysis in the R statistical computing environment (R Core Team, 2019). We used linear mixed models (R Package: nlme; Pinheiro et al., 2019) to determine the impacts of wildfire occurrence and burn severity (dNBR) on live and dead tree basal area, total C and N, C:N ratio, PyC, NH4+-N, NO3–-N, TIN, extractable P, pH, gravimetric soil moisture, forest floor mass, and relative DRIFT peak areas. To account for potential legacy effects of soil properties related to topography, all models initially included elevation, slope, and aspect as covariates. Topographic variables that were not significant at α < 0.05 were sequentially removed from the models. All models included plot-identifier as a random effect, and the NH4+-N, NO3–-N, and TIN models included total N as a covariate. We determined that inorganic N variables did not meet assumptions of normality, so we normalized these variables using Box-Cox transformations (Box and Cox, 1964). We determined whether wildfire occurrence or dNBR was a better predictor of differences by fitting separate models that included either fire occurrence (burned or unburned) or burn severity as an explanatory variable and comparing model AIC values. We selected fire occurrence or severity as the better predictor if the model containing that variable was ≥ 2.0 AIC points lower than the alternative model.
Fire and Severity Effects on Soil Carbon Pools and Fluxes
We assessed the impacts of fire and severity on soil C flux rates and cumulative soil C flux over our soil incubation using the following fixed effects portion of linear mixed models:
where βi represents the model-fitted intercept or slope coefficient and fire variable is either fire occurrence or burn severity. Each model also initially included all topographic variables and a plot-identifier random effect. Non-significant topographic variables were sequentially removed, and selection of fire occurrence or severity as a more suitable predictor was performed using AIC values as described above.
We assessed the size and mineralization rates of the Ca and Cs pools using non-linear mixed models. We assessed the sizes of the Ca and Cs pools and their mineralization rate constants using the following fixed effects portion a two-compartment first-order kinetics model (Kuzyakov, 2011):
where RateCO2 is the soil CO2 efflux rate at each measurement event, Ca is the size of the active C pool, ka is its mineralization coefficient, Cs is the size of the non-active C pool and ks is its mineralization rate coefficient. In this model, Cs is constrained to be CSOC – Crma, where CSOC is total soil organic C content.
We determined whether fire occurrence or severity affected soil C pools by first fitting a global non-linear model that included all 40 incubation replicates. We performed model selection procedures for random effects in non-linear models as described by Pinheiro and Bates (2000), which resulted in the inclusion of a plot identifier as a random effect associated with Ca and ks, and no random effect associated with ka. We assessed how wildfire impacted the size or kinetic rates of the Ca and Cs pools by adding fire occurrence or severity as a covariate to the non-linear models. Selection of fire occurrence or severity as a more suitable predictor variable was performed using AIC values as previously described. In addition to the global model, we fit separate non-linear models for each incubation replicate to obtain independent estimates of Ca, ka, and ks for use in elastic net regularization analysis and structural equation modeling. Five incubation replicates (two replicates from separate unburned plots, and one each from plots with dNBR values of 108, 350, and 478) did not converge during non-linear regression due to consistently high respiration rates throughout the incubation and were excluded from further analysis. After exclusion, each of the ten plots still had C pool parameter estimates from at least three subplots.
Fire and Severity Effects on Soil Bacterial Communities and Imputed Metagenomes
We assessed the impacts of wildfire and burn severity on Faith’s phylogenetic diversity, OTU richness, Shannon’s diversity, Pielou’s evenness, O:C, weighed 16S rRNA gene copy number, and the relative abundance of the ten most abundant bacterial phyla using linear mixed models and AIC model-selection as described above. The ten most abundant bacterial phyla represented all phyla that exhibited > 0.5% relative abundance and 94.1% of the total abundance. We used multivariate statistical approaches (R Package: vegan; Oksanen et al., 2019) to determine the impacts of fire occurrence, burn severity, and soil properties on bacterial community structure. Using a weighted UniFrac distance matrix of the bacterial community, we employed principle coordinates analysis (PCoA) to determine the relationships between plant and soil characteristics and bacterial communities. Additionally, we performed PCoA on a Bray-Curtis distance matrix of imputed MetaCyc metabolic pathways, hierarchically grouped into common C-degradation pathways.
We identified positive and negative fire responsive bacterial OTUs using species indicator analysis with fire occurrence as the explanatory variable, relative abundance of OTUs as response variables, and point biserial correlation as the output statistic (R Package: indicspecies; De Caceres and Legendre, 2009). We assessed which positive fire-responder OTUs were also positively related to severity by performing linear mixed-model analysis on the relative abundance of each fire-responsive OTU with dNBR as the explanatory variable and unburned plots excluded from the analysis.
Links Between Ecosystem Characteristics and Soil Carbon Pools
We determined properties linked to the size and kinetic rates of soil C pools using structural equation modeling. We constructed an initial SEM meta-model composed of linear mixed models that included live tree basal area, dead tree basal area, forest floor mass, total C, PyC, total N, TIN, extractable P, pH, and soil moisture as response variables (R Package: piecewiseSEM; Lefcheck, 2016). We assessed relationships of soil properties, live and dead tree basal area, and topography with C pools using separate SEMs for each C pool parameter (i.e., Ca, ka, ks). Additional methodological details and results of our SEMs are provided in Supplementary Note 1.
Relationships Between Bacteria, Carbon Pools, and Soil Nutrients
We used correlation analyses to assess relationships between log2 bacterial abundance at the levels of phylum and genus with C pools, soil TIN and P, pH, and relative DRIFT peak areas associated aromatic and labile compounds in the LPOM fraction. We selected these soil variables because they indicate soil nutrient availability and the amount of labile vs. recalcitrant C, and are therefore associated with bacterial copiotrophic versus oligotrophic life-strategies. We performed univariate correlations between all bacterial phyla and the selected soil variables. At the genus level, we performed correlations within phyla that our linear mixed-models indicated were related to burn severity and that previous research indicates exhibit either copiotrophic or oligotrophic life strategy (i.e., Acidobacteria, Actinobacteria, Bacteroidetes, Proteobacteria, and Verrucomicrobia). All correlations were performed using Spearman’s rank correlation to account for non-normality of bacterial abundance data and we considered correlations significant at α = 0.05.
We performed elastic net regularization (R Package: glmnet; Friedman et al., 2010) to identify bacteria phyla that are predictive of C pool parameters. Elastic net regularization selects the best set of predictors for a given response by using LASSO and ridge regression, but does not assign significance values to selected predictors; elastic net regularization is frequently used with sparse datasets when the number of potential predictors is larger than the number of observations, as is often the case for genomic data (Friedman et al., 2010; Wagg et al., 2019). We used linear mixed-models to determine relationships between C degradation metabolic pathways and C pool parameters.
Results
Relationships Between Fire, Tree Basal Area, and Soil Properties
Linear Mixed Models
Our AIC-based model selection indicated that fire occurrence had more explanatory power for models assessing total soil C, NH4+-N and TIN, whereas severity had more explanatory power for dead tree basal area, forest floor mass, total N, pH, and soil moisture (Table 1). Neither explanatory variable had more power for live tree basal area, C:N, NO3–-N, extractable P, or relative DRIFT peak areas.
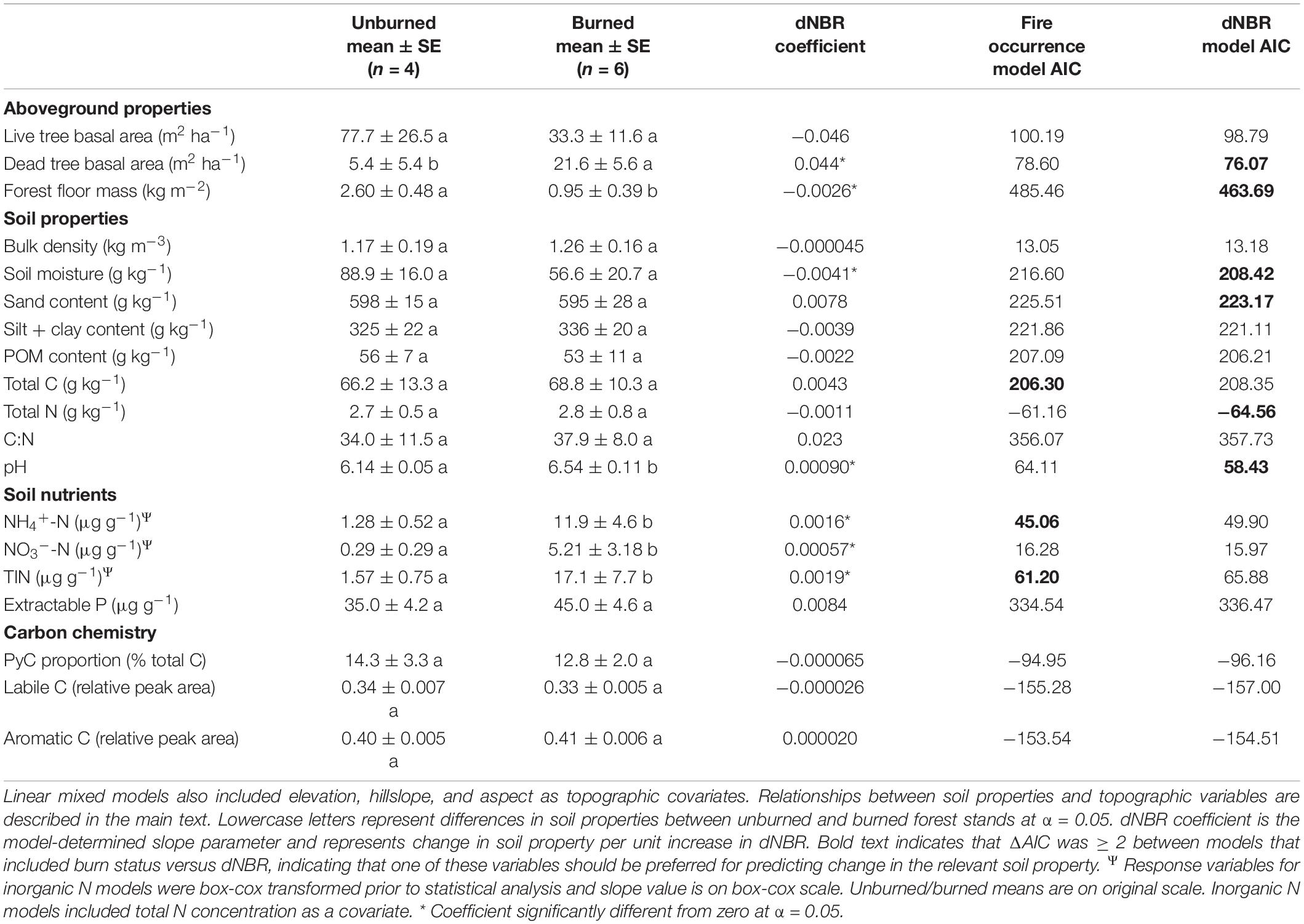
Table 1. Relationship of fire occurrence and burn severity (dNBR) to soil properties as determined via linear mixed models.
Concentrations of NH4+-N, NO3–-N, and TIN were higher in burned stands than unburned stands (Table 1). Additionally, total N was a significant covariate for NH4+-N, NO3–-N, and TIN, all of which were all positively related to total N (p < 0.001, p = 0.041, and p < 0.001, respectively). Dead tree basal area increased with severity (p = 0.005), whereas live tree basal area was not related to severity. Forest floor mass was negatively associated with severity (p = 0.002). Mineral soil gravimetric moisture was negatively related to severity (p = 0.004). Soil pH increased in association with severity (p = 0.007). Mineral soil total C and N, C:N, PyC, extractable P, and relative DRIFT peak areas were not significantly affected by fire occurrence or burn severity. Our SEM meta-model revealed relationships between severity and soil properties that were not captured by linear mixed-models (Supplementary Note 1 and Supplementary Figure 2).
Relationship of Wildfire and Burn Severity to Soil Carbon Pools and Fluxes
AIC-based comparisons of mixed-models assessing soil CO2 efflux rate over our 300-day incubation did not suggest a preference for fire occurrence versus severity-based models (Figure 1). However, AIC values indicated fire occurrence had more explanatory power when assessing the same data as cumulative CO2-C flux (Supplementary Figure 3). There was a negative main effect of fire occurrence on flux rate (p = 0.01) and a positive interaction effect of fire occurrence × ln(day) (p = 0.007). Together, these effects indicate that initial flux rates were lower in soils in burned areas, and, over the course of the incubation, flux rates decreased more slowly in burned soils compared to unburned soils. Flux rates were positively related to elevation, but, when the data was assessed as cumulative CO2-C flux, elevation was not a significant predictor.
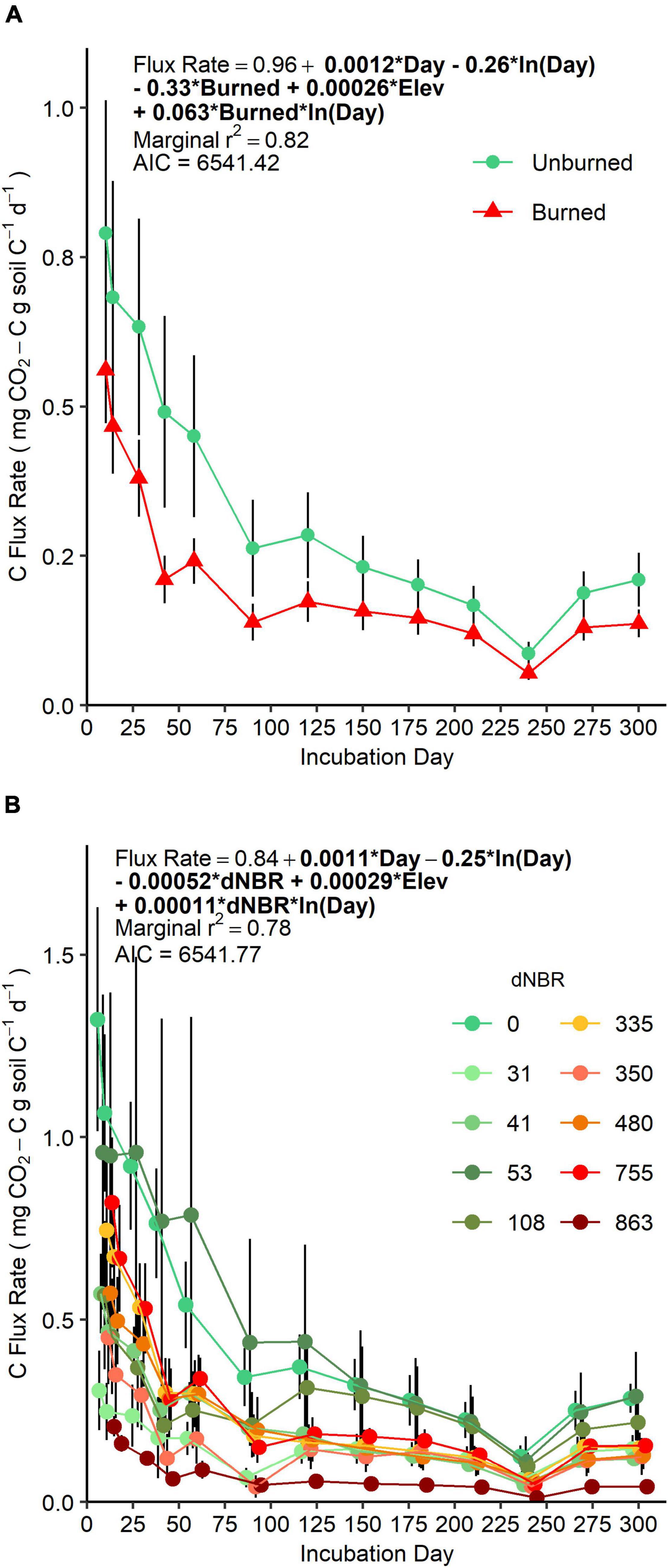
Figure 1. Mean (±SE) CO2-C efflux rate (points) over a 300-day laboratory incubation of mineral soils (0–5 cm) grouped by fire-occurrence (A) and severity (B). Colored lines represent change in CO2-C efflux rates between sampling days and vertical bars represent standard errors. (A) SE is based on n = 4 unburned plots and n = 6 burned plots. (B) SE is based on n = 3 or 4 subplots per plot. For each sampling day, sample points are offset on the horizontal axis to aid in interpretation, but gas sampling for all microcosm jars were performed on the incubation day.
AIC-based model selection of our global non-linear mixed models quantifying soil C pool structure and kinetics indicated that a fire occurrence model had more explanatory power than a severity model (ΔAIC = 3.08). ks decreased from 0.00017 in unburned soils to 0.000070 in burned soils (p = 0.002). This is equivalent to Cs mean residence time increasing from 16.1 years to 39.2 years. The sizes of the Ca and Cs pools and ka were not significantly different between burned and unburned soils. Modeled Ca was 1220 ± 210 mg kg soil–1 (2.08 ± 0.37% of total C), and ka was 0.028 ± 0.002 (mean residence time = 35.7 days). The SEMs indicated that Ca, ka, and ks were all directly or indirectly linked to severity (Table 2; Supplementary Note 1).
Relationships Between Wildfire, Bacterial Communities, and Imputed Metabolic Pathways
Bacterial Diversity and Life History Strategy
Fire occurrence and severity negatively impacted Faith’s phylogenetic diversity, with AIC values indicating no preference for fire occurrence or severity-based models (Figures 2A,B). AIC values indicated that the severity model better explained OTU richness, which was negatively correlated with severity (Figures 2C,D). Fire occurrence had more explanatory power for changes in phyla-level oligotroph-to-copiotroph ratios, which decreased from 1.08 in unburned soils to 0.48 in burned soils (Figures 2E,F). Fire occurrence and severity were positively associated with 16S rRNA gene copy number, with AIC values indicating no preference for either explanatory variable (Figures 2G,H).
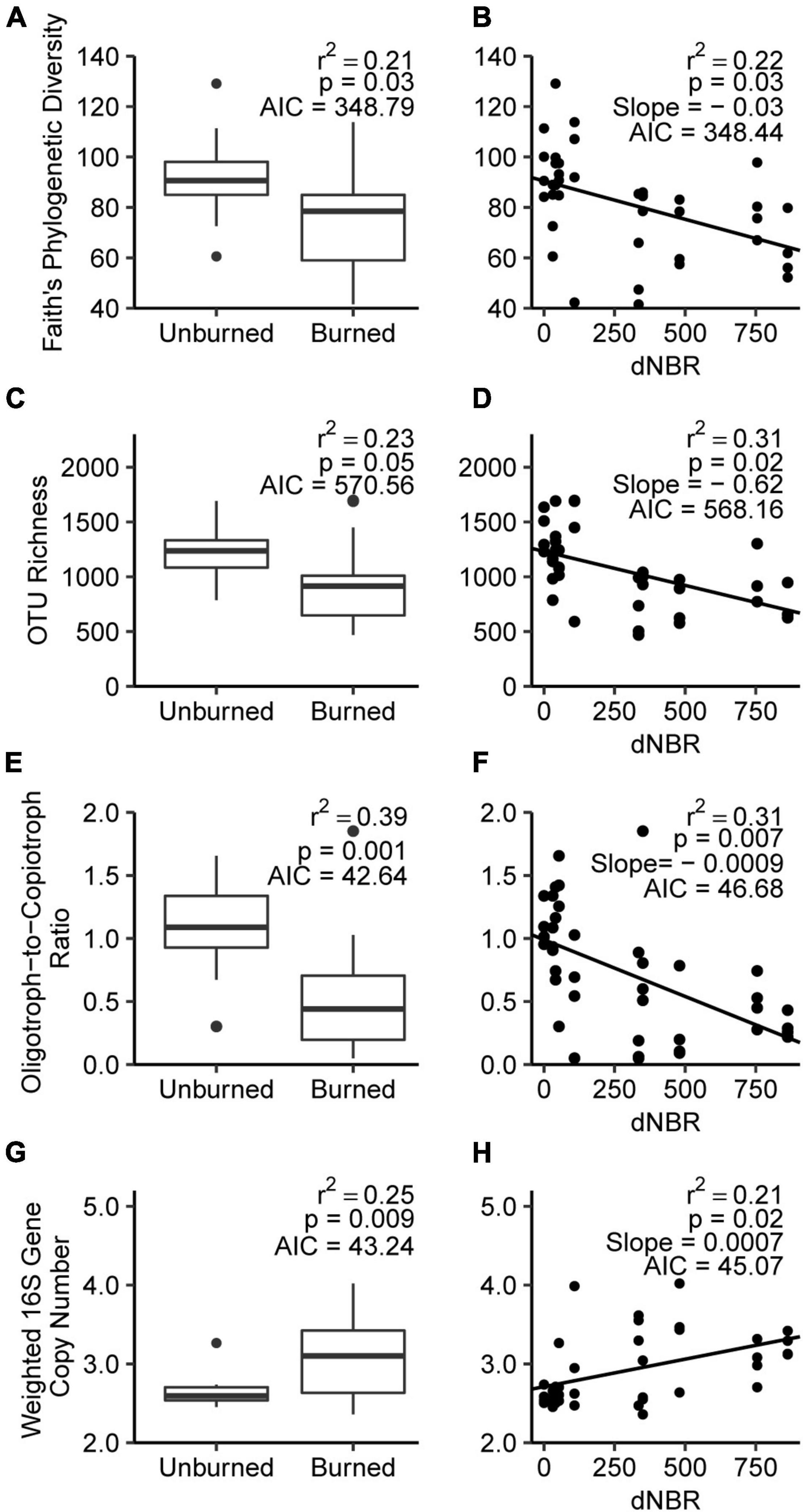
Figure 2. Relationship between fire occurrence and severity for selected microbial community characteristics, including Faith’s phylogenetic diversity (A,B), OTU richness (C,D), oligotrophic-to-copiotrophic taxa ratio (E,F), and weight 16S gene copy number (G,H).
Bacterial Community Structure
We performed PCoA on a weighted UniFrac distance matrix and found that the first axis explained 54.9% of variation, and the second axis explained an additional 8.7% (Figure 3). Fire occurrence (r2 = 0.24, p < 0.001) and severity (r2 = 0.57, p < 0.001) were both significantly correlated with the PCoA ordination. Several soil properties were also significantly correlated with the ordination, including NH4-N concentration (p = 0.002), TIN concentration (p = 0.008), P concentration (p = 0.009), pH (p < 0.001), soil moisture (p = 0.010), forest floor mass (p = 0.003), and labile C peak areas (p = 0.020) and aromatic C peak areas (p = 0.024) in the LPOM fraction.
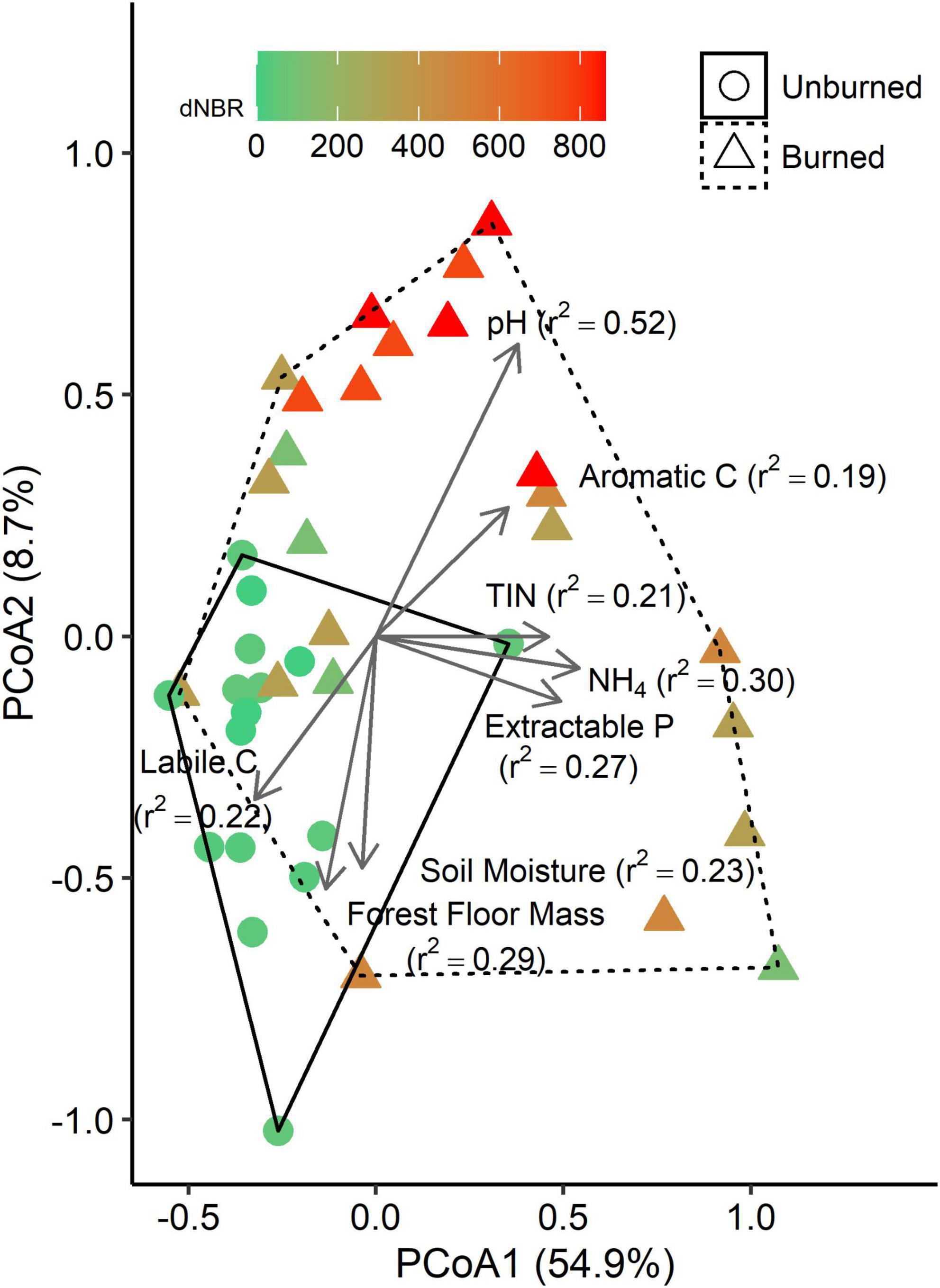
Figure 3. Principle coordinates analysis (PCoA) plots based on a weighted UniFrac distance matrix of bacterial communities in mineral soils (0–5 cm). Each point represents the bacterial community from a single subplot. Vectors represent variables that are significantly correlated with one of the PCoA axes, and vector lengths are scaled based on r2 values. Solid and dashed hulls depict the ordination space that encompasses all burned and unburned samples, respectively.
We assessed the relationship between wildfire occurrence and severity and the relative abundances of the ten most abundant bacterial groups using univariate linear mixed models. AIC values indicated that the fire occurrence models had more explanatory power for assessing differences in Bacteroidetes, Acidobacteria, Verrucomicrobia, and Planctomycetes relative abundance. The relative abundances of Bacteroidetes, Actinobacteria, and Firmicutes were higher in burned areas than unburned areas (Figure 4). The relative abundances of Acidobacteria, Verrucomicrobia, and Planctomycetes relative abundance were lower in burned areas than unburned areas. There was no significant effect of fire occurrence or burn severity on α-Proteobacteria,γ-Proteobacteria, δ-proteobacteria or Gemmatimonadetes relative abundance.
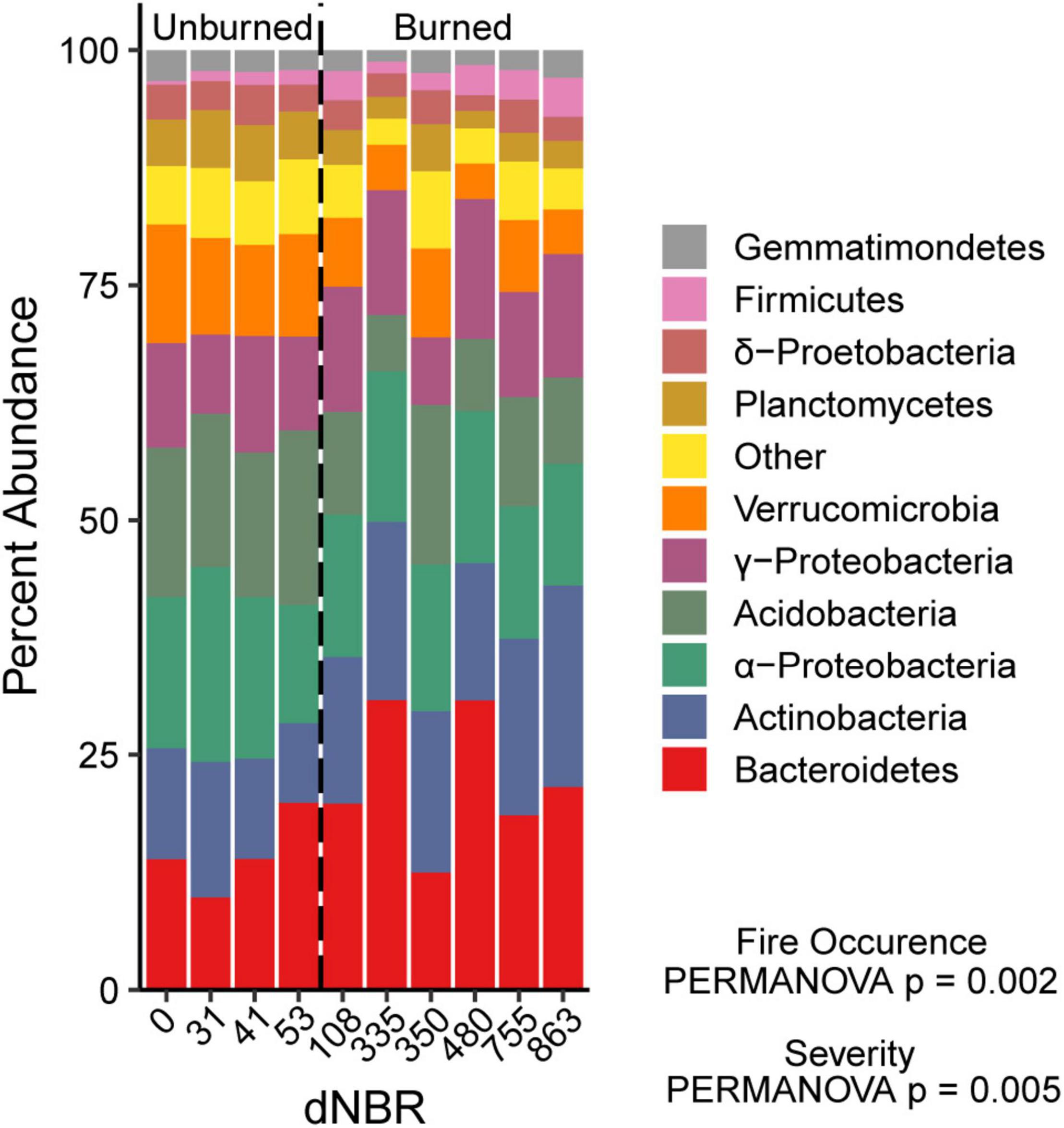
Figure 4. Relative abundance of the ten most abundant bacterial phyla across a gradient of burn severity in mineral soils (0–5 cm). The ten most abundant phyla accounted for ∼94% of the total bacterial community. Abundances at each dNBR level are the means of four subplots.
Bacterial OTUs
Indicator species analysis identified 53 OTUs as indicators of burned soils (i.e., positive fire responders) and 74 OTUs as indicators of unburned soils (i.e., negative fire responders) (Supplementary Tables 1, 2). The positive fire responders most commonly belonged to the Actinobacteria and Bacteroidetes phyla, as well as to the α-proteobacteria class, which, respectively, accounted for 28.6, 23.2, and 26.8% of the positive responder OTUs. The seven OTUs that exhibited the strongest positive response to fire (point biserial correlation > 0.60) came from the genera Massilia (γ-proteobacteria), Roseomonas (α-proteobacteria), Segetibacter (Bacteroidetes; 2 OTUs), Blastococcus (Actinobacteria), unclassified Micrococcaceae genus (Actinobacteria), and unclassified Burkholderiaceae genus (γ-proteobacteria). The negative fire responders most frequently belonged to the Planctomycetes phylum, which accounted for 14.7% of these responders, and to the α-proteobacteria and γ-proteobacteria classes, which accounted for 18.7 and 17.3%, respectively. The five OTUs that exhibited the strongest negative response came from the genera Cytophaga (Bacteroidetes), IS-44 (γ-proteobacteria), Mycobacterium (Actinobacteria), uncultured Elsteraceae genus (α-proteobacteria), and uncultured Gemmataceae genus (Planctomycetes). OTUs identified as positive fire responders had a significantly higher mean 16S gene copy number than negative fire responders (3.41 vs. 2.64; p = 0.020).
We identified 15 OTUs as positively associated with severity based on linear mixed models (Supplementary Figure 4). Of the 15 OTUs, six were from the Bacteroidetes phylum, two were from Actinobacteria, and one was from Verrucomicrobia. Four severity responders were from the α-proteobacteria class, one was from the γ-proteobacteria class, and one was from the δ-proteobacteria class. The abundances of all of the severity-associated OTUs were positively correlated with either TIN or P (data not shown).
Carbon Degradation Metabolic Pathways
Using PICRUSt2, we identified 135 MetaCyc metabolic pathways associated with C-degradation functions. PCoA on a Bray-Curtis matrix of the MetaCyc pathways grouped into common C-degradation functions indicated that the first axis explained 45.1% of variation and the second axis explained 22.1% (Figure 5). Fire occurrence (r2 = 0.16, p < 0.001) and severity (r2 = 0.41, p < 0.001) were significantly correlated with the ordination. Additionally, several soil properties were significantly correlated with the ordination, including total C (p = 0.009), C:N ratio (p = 0.044), NH4-N (p = 0.008), TIN (p = 0.047), extractable P (p = 0.002), pH (p = 0.010), and forest floor mass (p = 0.007).
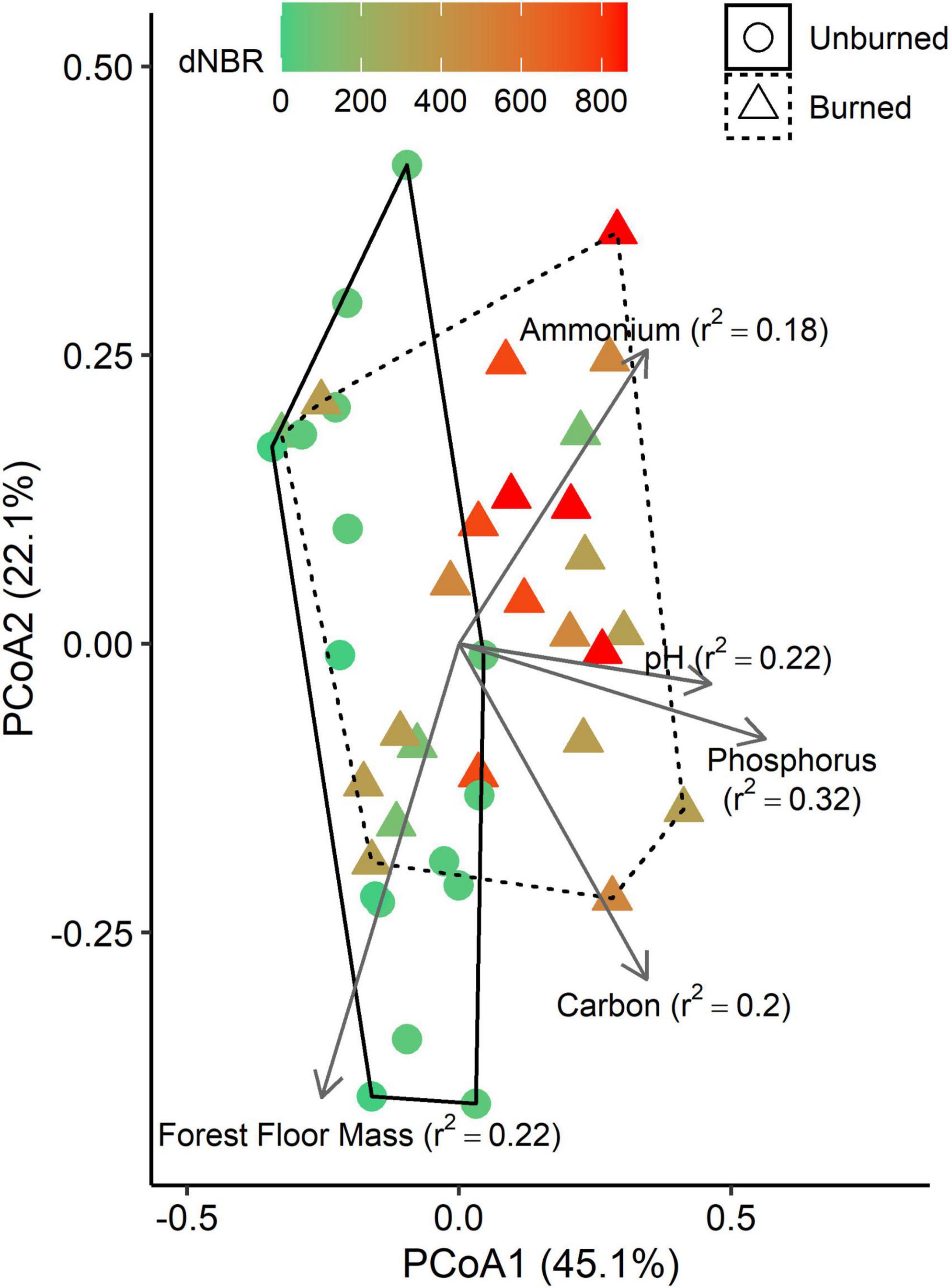
Figure 5. Principle coordinates analysis (PCoA) plots based on a Bray-Curtis distance matrix of imputed MetaCyc pathways grouped into common C-degradation functions. Vectors represent variables that are significantly correlated with one of the PCoA axes, and vector lengths are scaled based on r2 values. Solid and dashed hulls depict the ordination space that encompasses all burned and unburned samples, respectively.
AIC values indicated that severity had more explanatory power for differences in carbohydrate degradation, alcohol degradation, amine and polyamine degradation, carboxylate degradation, and nucleotide and nucleoside degradation. Severity positively impacted the relative abundance of imputed pathways associated with carbohydrate degradation, alcohol degradation, amine and polyamine degradation, carboxylate degradation, and secondary metabolite degradation (Figure 6). The relative abundance of amino acid degradation pathways was higher in burned areas than unburned areas (p = 0.021). The relative abundances of aromatic compound degradation pathways and fatty acid and lipid degradation pathways were not related to fire occurrence or severity.
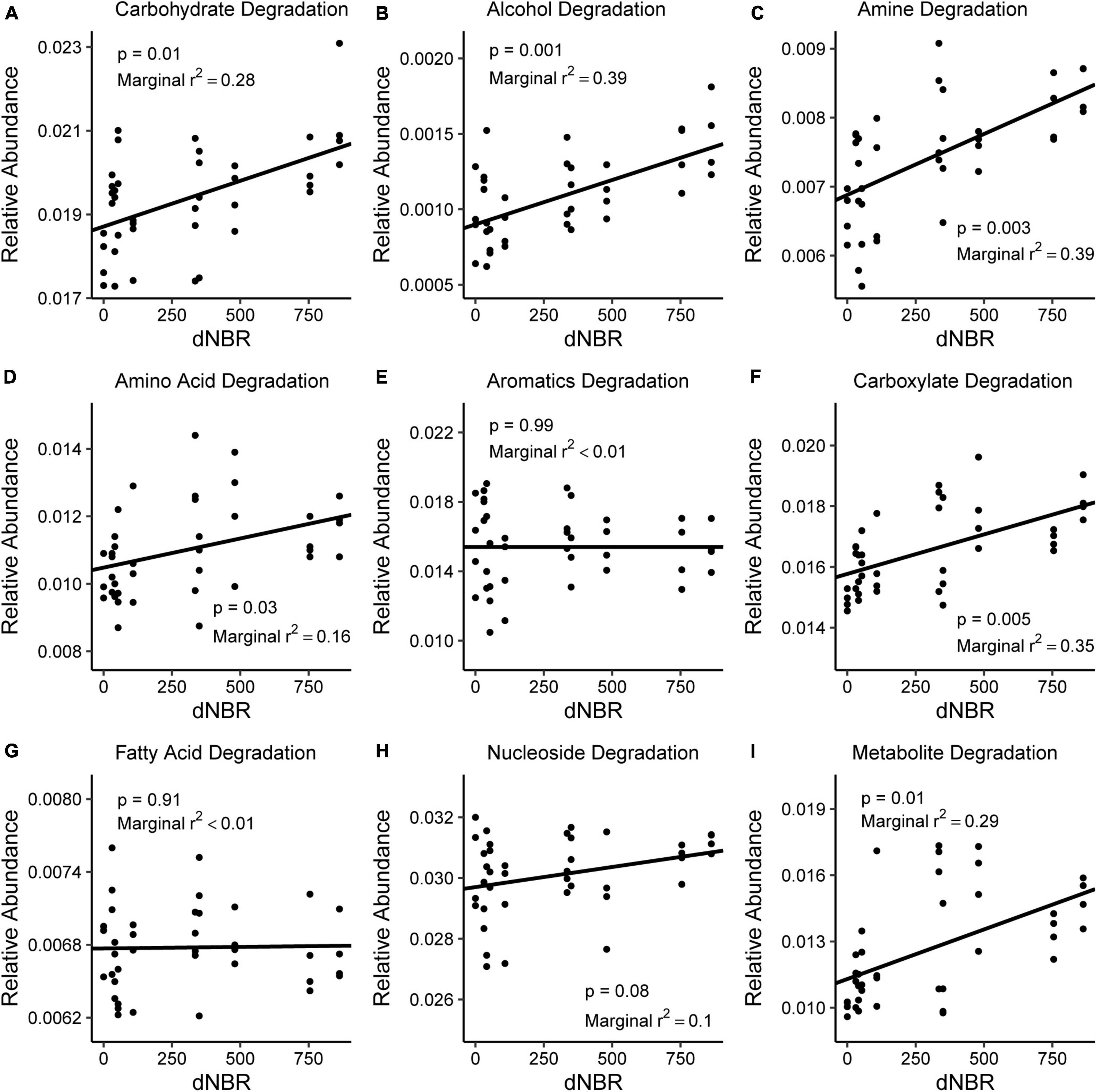
Figure 6. Relationships between burn severity and the relative abundance of imputed C-degradation pathways including (A) carbohydrate, (B) alcohol, (C) amine, (D) amino acid, (E) aromatic compound, (F) carboxylate, (G) fatty acid, (H) nucleoside, and (I) secondary metabolite degradation pathways. The relative abundance of all C-degradation pathways was estimated using PICRUSt2.
Relationships Between Bacterial Life History Traits, Soil Carbon Pools and Kinetics, and Soil Nutrients
Weighted mean 16S gene copy numbers were positively associated with soil TIN (p = 0.003) and pH (p = 0.006) and also tended to increase with soil P (p = 0.061). 16S copy numbers were not associated with soil C pool parameters determined via lab incubation or with DRIFT spectroscopy. The relationships between bacterial taxa at the phylum and genus level with soil C pools and soil nutrients are discussed in detail in Supplementary Note 2. Briefly, phyla typically classified as copiotrophs were frequently positively correlated with soil nutrients, and, in some cases, with Ca. Firmicutes, a putative copiotrophic phylum, was identified as positively associated with ka via simple correlation analysis and elastic net regression (Figure 7 and Supplementary Table 3). Phyla typically classified as oligotrophs were generally negatively correlated with soil nutrients, but none were associated with ks. When considered at the genus level, taxa harbored within phyla typically classified as copiotrophs or oligotrophs exhibited a wide variety of relationships between soil nutrients and soil C pools.
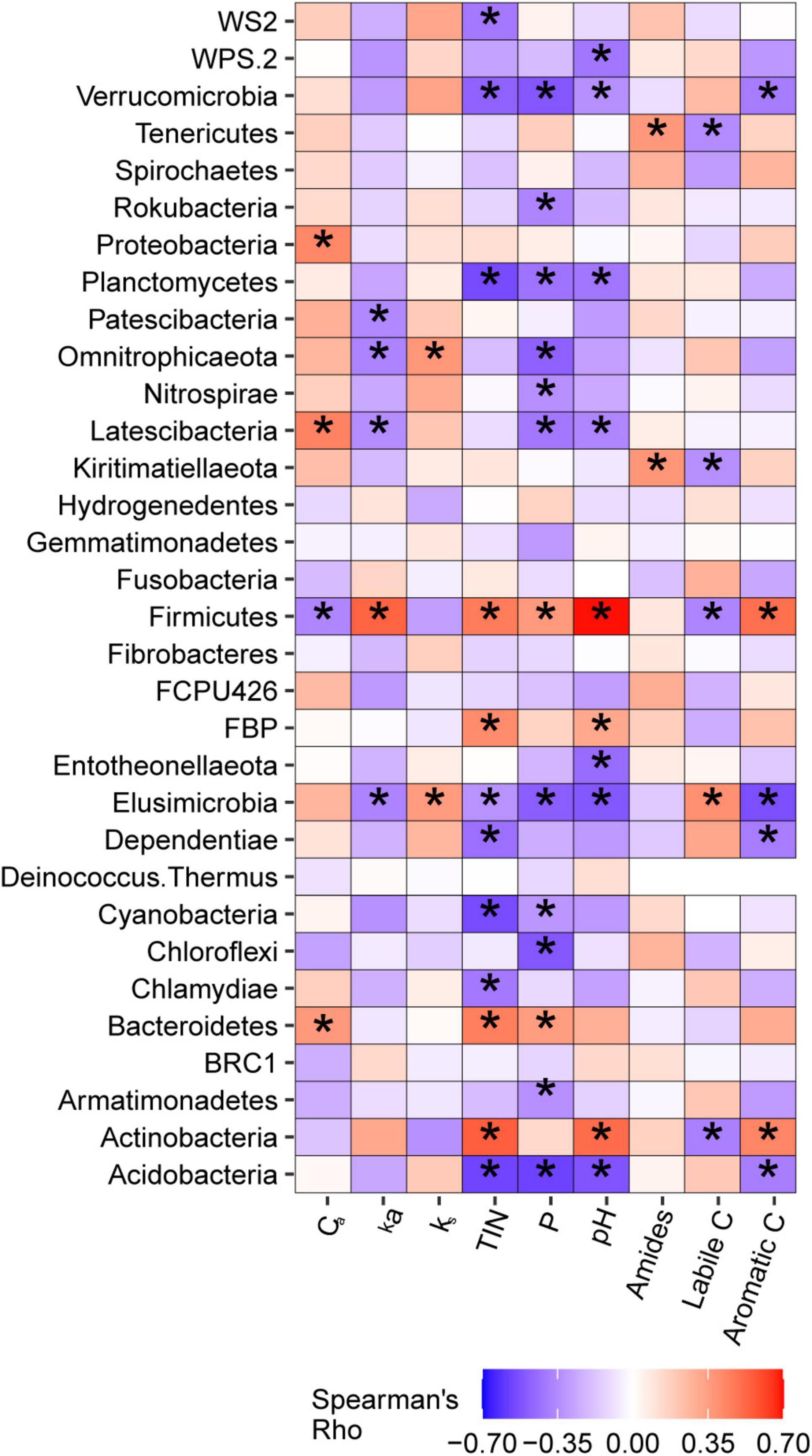
Figure 7. Correlations betweenbacterial phyla and active carbon pool size (Ca) and kinetic rate (ka), non-active carbon pool kinetic rate (ks), total inorganic nitrogen (TIN), phosphorus (P), pH, and amide, labile C, and aromatic C regions of MIR spectra of light particulate soil organic matter. *Indicate correlations that were significant at α = 0.05.
Linear mixed models indicated that ka was positively associated with metabolic pathways of alcohol degradation (p = 0.004) and fatty acid and lipid degradation (p = 0.003), and negatively associated with pathways of aromatic compound degradation (p = 0.005) and nucleoside and nucleotide degradation (p = 0.009). Ca pool size was negatively associated with alcohol degradation pathways (p = 0.040) and positively associated with aromatic compound degradation (p = 0.015). ks was not related to any metabolic pathways.
Discussion
Hypothesis 1: Carbon Pools Are Related to Differences in Soil Properties Across a Burn Severity Gradient
Our SEMs indicate that soil properties including forest floor mass, total N, pH, and organic matter chemistry were related to C pools, but live and dead tree basal area also played an important role in explaining differences in C pools (Table 2). Dead tree basal area was related to several soil properties 1-year post-fire, exhibiting direct links to total N and soil moisture and an indirect link to TIN (Supplementary Figure 2). We also observed a positive relationship between dead tree basal area and total N in a previous study (Adkins et al., 2020), an effect that could be caused by decomposition of dead tree roots leading to increased soil N inputs (Fahey et al., 1988). Root dynamics could also explain the direct negative link between dead tree basal area and Ca (Table 2), potentially a result of decreased root exudation (Boddy et al., 2007; de Graaff et al., 2010). Along with forest floor mass and total N, dead tree basal area offset the direct positive link between severity and Ca, suggesting vegetation dynamics play an important role in mediating the response of soil C stability to fire. We provide additional discussion on the relationships between soil properties and C pools captured by our SEMs in Supplementary Note 1.
Hypothesis 2: Bacteria Previously Identified as Fire Responders Are Positively Associated With Burn Severity
In support of our hypothesis, some of the genera harboring the severity-responsive OTUs (Supplementary Figure 4) have previously been identified as fire-responsive taxa (e.g., Adhaeribacter, Roseomonas, and Flavisolibacter) (Weber et al., 2014; Whitman et al., 2019). All of the genera harboring the severity-responsive OTUs were positively correlated with either TIN or P, suggesting copiotrophic life-strategies. To the best of our knowledge, other OTUs we identified as severity-responders have not previously been characterized as fire-responders. For example, Segetibacter accounted for two severity-responsive OTUs (and three positive fire-responsive OTUs) but has not been identified as fire-responsive in previous studies. However, Segetibacter was identified as responding positively to PyC additions in a lab incubation study, suggesting post-fire affinity (Woolet and Whitman, 2020). We identified several genera as positive fire-responders that other studies have also identified, including Aeromicrobium, Blastococcus, Massilia, Phenylobacterium, and Devosia (Weber et al., 2014; Whitman et al., 2016; Huffman and Madritch, 2018). Our identification of unique severity responsive OTUs suggests that high burn severity may cause soil function to become dissimilar from pre-fire conditions. This is further evidenced by the positive associations of several imputed C-metabolism pathways with severity (Figure 6).
Hypothesis 3: Copiotrophic Bacteria Abundance Is Positively Related to Burn Severity
Numerous lines of evidence suggested that burned areas had greater abundance of copiotrophic bacteria compared to unburned areas, and this effect increased with severity. Our finding of increased copiotroph abundance post-fire agrees with other research from ecosystems across the globe (Pérez-Valera et al., 2019; Whitman et al., 2019; Ling et al., 2021; Ammitzboll et al., 2022). Some researchers found that the increased copiotrophic predominance dissipated by 1-year post-fire (Pérez-Valera et al., 2019; Ammitzboll et al., 2022), whereas here we found copiotrophic abundance remained higher than in unburned areas at 1-year post-fire, and in previous research we found higher copiotrophy 3-years post-fire in a Sierra Nevada mixed-conifer forest (Adkins et al., 2020). The differences in timeframes at which copiotrophic abundance returns to pre-fire levels may be explained by the positive relationship between copiotrophs and severity. The larger magnitude of the effect of high burn severity on copiotroph abundance may require more time to return to pre-fire levels, but burn severity is often not incorporated into studies on fire’s effects on microbial communities. Along with a few other studies that have considered burn severity (Whitman et al., 2019; Adkins et al., 2020), our findings suggest that researchers should consistently account for severity when investigating the influence of fire on microbial communities.
Increased copiotrophic bacterial abundance was driven by nutrient dynamics rather than C dynamics. For instance, the higher mean weighted 16S gene copy number in burned areas was positively associated with TIN and pH, but not with labile or aromatic C pools. Additionally, our findings that positive fire responders have more 16S gene copies than negative fire responders, and that all severity-responsive OTUs were positively correlated TIN and/or P suggest that fire-induced increases in soil nutrient concentrations promote copiotrophic bacteria. This is further supported by the positive relationship of the copiotrophic phyla Bacteroidetes with TIN. Although Bacteroidetes abundance was also positively associated with Ca – an indicator of labile C availability – Ca did not differ between burned and unburned areas and thus cannot explain the greater Bacteroidetes abundance in burned areas. This suggests that copiotrophic bacteria do not necessarily respond to labile C and nutrient availability simultaneously and that higher TIN concentrations maintain the greater copiotroph populations in burned areas despite limited supply of preferred C substrates.
The mismatches between life history traits and C availability could influence soil C cycling during ecosystem recovery. In fact, imputed C degradation pathways suggest that copiotrophic metabolic strategies (i.e., carbohydrate, alcohol, and amine decomposition) increased with fire and severity, while pathways associated with decomposition of recalcitrant substances (i.e., aromatics) did not change (Figure 6). Although metabolic functions predicted by PICRUSt2 are not as reliable as direct metagenome approaches, studies comparing predicted metabolic profiles with direct metagenomics have found robust agreement between the approaches (Hartman et al., 2017; Douglas et al., 2020; Toole et al., 2021). Additionally, there have been very few metagenomic studies performed on post-fire soils (but see Taş et al., 2014; Johnston et al., 2016), so our results here represent valuable initial evidence of the influence of fire and burn severity on metabolic pathways that can be used to drive future research.
Over the short term, increases in labile C metabolic pathways could increase C cycling rates as microbes quickly utilize labile C sources. However, if aboveground biomass loss leads to decreased labile C inputs, this effect may be short-lived. This notion is supported by soil enzyme studies performed in Mediterranean shrublands and pine forests that have found elevated activities of carbohydrate-targeting enzymes within 1-month post-fire (Rodríguez et al., 2018; Pérez-Valera et al., 2019). In contrast, measurements performed 1–3 years post-fire have found carbohydrate-targeting enzymes activities that are depressed or no different than unburned controls (Rodríguez et al., 2017, 2018). Although we did not find greater amounts of PyC or aromatic compounds in burned compared to unburned areas (Table 1), inputs of recalcitrant C compounds to soil are often high after fire due to the formation of pyrogenic organic matter and wood deposition resulting from tree mortality (Miesel et al., 2015; Maestrini et al., 2017). If the input of this recalcitrant C is not accompanied by increases in the metabolic pathways associated with its degradation, recalcitrant C could accumulate in soil, leading to larger Cs pools and soil C stocks over the long-term.
Hypothesis 4: Bacterial Life Strategies Are Associated With Carbon Pool Kinetic Rates
Our hypothesis that ka would be positively associated with copiotrophic life strategy is supported by the positive relationship between ka with the copiotrophic-associated metabolic pathways of alcohol degradation. Further, ka decreased when there was a greater abundance of the oligotrophic metabolic pathway of aromatic compound degradation. Additionally, Crm a size was negatively associated with alcohol degradation pathways and positively associated with aromatic compound degradation pathways, indicating the size of the labile C pool is influenced by relative investment in copiotrophic vs. oligotrophic metabolic strategies. Support for links between copiotrophy and ka was more limited when considered from a taxonomic perspective. Firmicutes was the only phylum that was positively associated with ka, and, although Firmicutes is often considered copiotrophic (Ramirez et al., 2012), we found that it exhibited both copiotrophic and oligotrophic characteristics. For example, positive associations between Firmicutes with inorganic nutrients suggest copiotrophy, whereas correlations between Firmicutes and C pools suggest oligotrophy (Figure 7). Whether ks is associated with a specific bacterial life strategy is similarly ambiguous. We found that ks was positively associated with Elusimicrobia abundance. Although Elusimicrobia has not been classified as either copiotrophic or oligotrophic, previous research has identified oligotrophic tendencies with regard to C use for this phylum. For example, Elusimicrobia have been found to preferentially utilize recalcitrant forms of C as substrate (Chávez-Romero et al., 2016), and have been identified as lignin degraders (Wilhelm, 2016). However, we found Elusimicrobia was negatively correlated aromatic C and positively correlated with labile C, suggesting copiotrophy with regards to C use. This contrasts with the negative association of Elusimicrobia with TIN and P, which suggest oligotrophy. Overall, life-history strategy appears to influence C pool kinetics, but metabolic pathways may be a more useful indicator of this relationship than bacterial taxa.
Conclusion
Copiotrophic bacteria abundance was greater in burned areas than unburned areas, and copiotrophic metabolic pathways increased with burn severity. Because copiotrophic metabolic pathways were positively related to ka, these changes could lead to short-term increases in post-fire soil C cycling. However, metabolic pathways associated with cycling of aromatic C substrates did not differ between burned and unburned areas, despite the fact that aromatic compounds often increase after fire due to the formation of PyC. An increase in aromatic C without a corresponding increase in pathways associated with its degradation could allow soil C to accumulate during post-fire recovery, contributing to the stable C pool. In fact, our results suggest that soil C is more persistent in burned than unburned areas 1 year after fire (as indicated by lower ks). The increase in C persistence – and potential accumulation of aromatic C – may partially offset ecosystem C losses from biomass combustion while vegetation recovers.
Overall, our results indicate that bacterial communities and life history strategy are associated with C pools and kinetics, primarily attributable to changes in C degradation pathways. Future research could confirm these findings by employing direct metagenomic or metabolomic approaches. Such information could be incorporated into global ecosystem models to help anticipate the effects of fire regime change on the global C cycle. In contrast to clear links between metabolic pathways and C kinetics, links between taxonomic-based life-history classifications and C pool kinetics were somewhat ambiguous. Differences in bacterial community structure between burned and unburned areas could be explained by the copiotroph-oligotroph life-history framework when considered at the phylum level or with 16S gene copy number, but was less effective in explaining differences at the genus level. This suggests that coarse life-history classifications fail to capture the metabolic breadth of bacterial taxa and may therefore limit the ability to predict the influence of microbial communities on ecosystem function during post-fire recovery. Future research could incorporate isotopic tracing techniques to further elucidate which bacterial taxa drive differences in C cycling during post-fire recovery and whether life-history strategy explains these differences.
Data Availability Statement
The datasets presented in this study can be found in online repositories. The names of the repository/repository and accession number(s) can be found below: SRA, PRJNA816726. Mendeley Data, http://dx.doi.org/10.17632/fjn7shxfk7.1.
Author Contributions
JA: formal analysis, investigation, writing – original draft, and visualization. KD: methodology, formal analysis, investigation, resources, writing, review, and editing. JM: conceptualization, resources, writing, review, editing, and supervision. All authors contributed to the article and approved the submitted version.
Funding
This work was supported by the USDA National Institute of Food and Agriculture, McIntire–Stennis Project 1006839 and by Michigan State University. JA was also supported by an NSF Graduate Research Fellowship Program award.
Conflict of Interest
The authors declare that the research was conducted in the absence of any commercial or financial relationships that could be construed as a potential conflict of interest.
Publisher’s Note
All claims expressed in this article are solely those of the authors and do not necessarily represent those of their affiliated organizations, or those of the publisher, the editors and the reviewers. Any product that may be evaluated in this article, or claim that may be made by its manufacturer, is not guaranteed or endorsed by the publisher.
Acknowledgments
We thank Bernardo Maestrini and Joshua James for field assistance, and Katherine Walker for laboratory assistance. We also thank USDA Forest Service staff from the Klamath National Forest for help in obtaining sampling permits and facilitating sampling logistics.
Supplementary Material
The Supplementary Material for this article can be found online at: https://www.frontiersin.org/articles/10.3389/ffgc.2022.873527/full#supplementary-material
References
Adkins, J., Docherty, K. M., Gutknecht, J. L. M., and Miesel, J. R. (2020). How do soil microbial communities respond to fire in the intermediate term? Investigating direct and indirect effects associated with fire occurrence and burn severity. Sci. Total Environ. 745:140957. doi: 10.1016/j.scitotenv.2020.140957
Adkins, J., Sanderman, J., and Miesel, J. (2019). Soil carbon pools and fluxes vary across a burn severity gradient three years after wildfire in Sierra Nevada mixed-conifer forest. Geoderma 333, 10–22. doi: 10.1016/j.geoderma.2018.07.009
Allison, S. D., and Martiny, J. B. H. (2008). Colloquium paper: resistance, resilience, and redundancy in microbial communities. Proc. Natl. Acad. Sci. U. S. A. 105, 11512–11519. doi: 10.1073/pnas.0801925105
Ammitzboll, H., Jordan, G. J., Baker, S. C., Freeman, J., and Bissett, A. (2022). Contrasting successional responses of soil bacteria and fungi to post-logging burn severity. For. Ecol. Manage. 508:120059. doi: 10.1016/j.foreco.2022.120059
Barbera, P., Kozlov, A. M., Czech, L., Morel, B., Darriba, D., Flouri, T., et al. (2018). EPA-ng: massively Parallel Evolutionary Placement of Genetic Sequences. Syst. Biol. 68, 365–369. doi: 10.1093/sysbio/syy054
Bird, M. I., Wynn, J. G., Saiz, G., Wurster, C. M., and McBeath, A. (2015). The pyrogenic carbon cycle. Annu. Rev. Earth Planet. Sci. 43, 273–298. doi: 10.1146/annurev-earth-060614-105038
Boddy, E., Hill, P. W., Farrar, J., and Jones, D. L. (2007). Fast turnover of low molecular weight components of the dissolved organic carbon pool of temperate grassland field soils. Soil Biol. Biochem. 39, 827–835. doi: 10.1016/j.soilbio.2006.09.030
Bokulich, N. A., Kaehler, B. D., Rideout, J. R., Dillon, M., Bolyen, E., Knight, R., et al. (2018). Optimizing taxonomic classification of marker-gene amplicon sequences with QIIME 2’s q2-feature-classifier plugin. Microbiome 6:90. doi: 10.1186/s40168-018-0470-z
Bolyen, E., Rideout, J. R., Dillon, M. R., Bokulich, N. A., Abnet, C. C., Al-Ghalith, G. A., et al. (2019). Reproducible, interactive, scalable and extensible microbiome data science using QIIME 2. Nat. Biotechnol. 37, 852–857. doi: 10.1038/s41587-019-0209-9
Box, G. E. P., and Cox, D. R. (1964). An Analysis of Transformations. J. R. Stat. Soc. Ser. B 26, 211–243. doi: 10.1111/j.2517-6161.1964.tb00553.x
Calderón, F. J., Reeves, J. B., Collins, H. P., and Paul, E. A. (2011). Chemical Differences in Soil Organic Matter Fractions Determined by Diffuse-Reflectance Mid-Infrared Spectroscopy. Soil Sci. Soc. Am. J. 75, 568–579. doi: 10.2136/sssaj2009.0375
Callahan, B. J., McMurdie, P. J., Rosen, M. J., Han, A. W., Johnson, A. J. A., and Holmes, S. P. (2016). DADA2: high-resolution sample inference from Illumina amplicon data. Nat. Methods 13, 581–583. doi: 10.1038/nmeth.3869
Canarini, A., Schmidt, H., Fuchslueger, L., Martin, V., Herbold, C. W., Zezula, D., et al. (2021). Ecological memory of recurrent drought modifies soil processes via changes in soil microbial community. Nat. Commun. 12, 1–14. doi: 10.1038/s41467-021-25675-4
Caporaso, J. G., Kuczynski, J., Stombaugh, J., Bittinger, K., Bushman, F. D., Costello, E. K., et al. (2010). QIIME allows analysis of high- throughput community sequencing data Intensity normalization improves color calling in SOLiD sequencing. Nat. Publ. Gr. 7, 335–336. doi: 10.1038/nmeth0510-335
Caspi, R., Billington, R., Fulcher, C. A., Keseler, I. M., Kothari, A., Krummenacker, M., et al. (2018). The MetaCyc database of metabolic pathways and enzymes. Nucleic Acids Res. 46, D633–D639. doi: 10.1093/nar/gkx935
Chávez-Romero, Y., Navarro-Noya, Y. E., Reynoso-Martínez, S. C., Sarria-Guzmán, Y., Govaerts, B., Verhulst, N., et al. (2016). 16S metagenomics reveals changes in the soil bacterial community driven by soil organic C, N-fertilizer and tillage-crop residue management. Soil Tillage Res. 159, 1–8. doi: 10.1016/j.still.2016.01.007
Collins, H. P., Elliott, E. T., Paustian, K., Bundy, L. G., Dick, W. A., Huggins, D. R., et al. (2000). Soil carbon pools and fluxes in long-term Corn Belt agroecosystems. Soil Biol. Biochem. 32, 157–168. doi: 10.1016/S0038-0717(99)00136-4
Czech, L., Barbera, P., and Stamatakis, A. (2020). Genesis and Gappa: processing, analyzing and visualizing phylogenetic (placement) data. Bioinformatics 36, 3263–3265. doi: 10.1093/bioinformatics/btaa070
De Caceres, M., and Legendre, P. (2009). Associations between species and groups of sites: indices and statistical inference. Ecology 90, 3566–3574. doi: 10.1890/08-1823.1
de Graaff, M.-A., Classen, A. T., Castro, H. F., and Schadt, C. W. (2010). Labile soil carbon inputs mediate the soil microbial community composition and plant residue decomposition rates. New Phytol. 188, 1055–1064. doi: 10.1111/j.1469-8137.2010.03427.x
Dennison, P. E., Brewer, S. C., Arnold, J. D., and Moritz, M. A. (2014). Large wildfire trends in the western United States, 1984-2011. Geophys. Res. Lett. 41, 2928–2933. doi: 10.1002/2014GL059576
Doane, T. A., and Horwáth, W. R. (2003). Spectrophotometric determination of nitrate with a single reagent. Anal. Lett. 36, 2713–2722. doi: 10.1081/AL-120024647
Douglas, G. M., Maffei, V. J., Zaneveld, J., Yurgel, S. N., Brown, J. R., Taylor, C. M., et al. (2019). PICRUSt2: an improved and extensible approach for metagenome inference. bioRxiv [Preprint]. doi: 10.1101/672295
Douglas, G. M., Maffei, V. J., Zaneveld, J. R., Yurgel, S. N., Brown, J. R., Taylor, C. M., et al. (2020). PICRUSt2 for prediction of metagenome functions. Nat. Biotechnol. 38, 685–688. doi: 10.1038/s41587-020-0548-6
Dove, N. C., Safford, H. D., Bohlman, G. N., Estes, B. L., and Hart, S. C. (2020). High-severity wildfire leads to multi-decadal impacts on soil biogeochemistry in mixed-conifer forests. Ecol. Appl. 30:e02072. doi: 10.1002/eap.2072
Fahey, T. J., Hughes, J. W., Pu, M., and Arthur, M. A. (1988). Root decomposition and nutrient flux following whole-tree harvest of northern hardwood forest. For. Sci. 34, 744–768. doi: 10.1093/forestscience/34.3.744
Faith, D. P. (1992). Conservation evaluation and phylogentic diversity. Biol. Conserv. 61, 1–10. doi: 10.1186/2046-9063-10-10
Fernández, I., Cabaneiro, A., and Carballas, T. (1997). Organic matter changes immediately after a wildfire in an atlantic forest soil and comparison with laboratory soil heating. Soil Biol. Biochem. 29, 1–11. doi: 10.1016/S0038-0717(96)00289-1
Ferrenberg, S., O’neill, S. P., Knelman, J. E., Todd, B., Duggan, S., Bradley, D., et al. (2013). Changes in assembly processes in soil bacterial communities following a wildfire disturbance. ISME J. 7, 1102–1111. doi: 10.1038/ismej.2013.11
Fierer, N. (2017). Embracing the unknown: disentangling the complexities of the soil microbiome. Nat. Rev. Microbiol. 15, 579–590. doi: 10.1038/nrmicro.2017.87
Fierer, N., Bradford, M. A., and Jackson, R. B. (2007). Toward an ecological classification of soil bacteria. Ecology 88, 1354–1364. doi: 10.1890/05-1839
Fierer, N., Lauber, C. L., Ramirez, K. S., Zaneveld, J., Bradford, M. A., and Knight, R. (2012). Comparative metagenomic, phylogenetic and physiological analyses of soil microbial communities across nitrogen gradients. ISME J. 6, 1007–1017. doi: 10.1038/ismej.2011.159
Flannigan, M. D., Krawchuk, M. A., de Groot, W. J., Wotton, B. M., and Gowman, L. M. (2009). Implications of changing climate for global wildland fire. Int. J. Wildl. Fire 18, 483–507. doi: 10.1071/WF08187
Friedman, J., Hastie, T., and Tibshirani, R. (2010). Regularizaton paths for generalized linear models via coordinate descent. J. Stat. Softw. 33, 1–22.
Graham, E. B., Knelman, J. E., Schindlbacher, A., Siciliano, S., Breulmann, M., Yannarell, A., et al. (2016). Microbes as engines of ecosystem function: when does community structure enhance predictions of ecosystem processes? Front. Microbiol. 7:214. doi: 10.3389/fmicb.2016.00214
Hale, L., Feng, W., Yin, H., Guo, X., Zhou, X., Bracho, R., et al. (2019). Tundra microbial community taxa and traits predict decomposition parameters of stable, old soil organic carbon. ISME J. 13, 2901–2915. doi: 10.1038/s41396-019-0485-x
Hart, S. C., DeLuca, T. H., Newman, G. S., MacKenzie, M. D., and Boyle, S. I. (2005). Post-fire vegetative dynamics as drivers of microbial community structure and function in forest soils. For. Ecol. Manage. 220, 166–184. doi: 10.1016/j.foreco.2005.08.012
Hartman, W. H., Ye, R., Horwath, W. R., and Tringe, S. G. (2017). A genomic perspective on stoichiometric regulation of soil carbon cycling. ISME J. 11, 2652–2665. doi: 10.1038/ismej.2017.115
Holden, S. R., Rogers, B. M., Treseder, K. K., and Randerson, J. T. (2016). Fire severity influences the response of soil microbes to a boreal forest fire. Environ. Res. Lett. 11:035004. doi: 10.1088/1748-9326/11/3/035004
Huffman, M. S., and Madritch, M. D. (2018). Soil microbial response following wildfires in thermic oak-pine forests. Biol. Fertil. Soils 54, 985–997. doi: 10.1007/s00374-018-1322-5
Johnston, E. R., Rodriguez-R, L. M., Luo, C., Yuan, M. M., Wu, L., He, Z., et al. (2016). Metagenomics reveals pervasive bacterial populations and reduced community diversity across the Alaska tundra ecosystem. Front. Microbiol. 7:579. doi: 10.3389/fmicb.2016.00579
Kashian, D. M., Romme, W. H., Tinker, D. B., Turner, M. G., and Ryan, M. G. (2006). Carbon storage on landscapes with stand-replacing fires. Bioscience 56, 598–606. doi: 10.1641/0006-3568(2006)56[598:csolws]2.0.co;2
Katoh, K., and Standley, D. M. (2013). MAFFT multiple sequence alignment software version 7: improvements in performance and usability. Mol. Biol. Evol. 30, 772–780. doi: 10.1093/molbev/mst010
Kitzberger, T., Falk, D. A., Westerling, A. L., and Swetnam, T. W. (2017). Direct and indirect climate controls predict heterogeneous early-mid 21st century wildfire burned area across western and boreal North America. PLoS One 12:e0188486. doi: 10.1371/journal.pone.0188486
Kurm, V., Van Der Putten, W. H., De Boer, W., Naus-Wiezer, S., and Gera Hol, W. H. (2017). Low abundant soil bacteria can be metabolically versatile and fast growing. Ecology 98, 555–564. doi: 10.1002/ecy.1670
Kurth, V. J., MacKenzie, M. D., and DeLuca, T. H. (2006). Estimating charcoal content in forest mineral soils. Geoderma 137, 135–139. doi: 10.1016/j.geoderma.2006.08.003
Kuzyakov, Y. (2011). How to link soil C pools with CO2 fluxes? Biogeosciences 8, 1523–1537. doi: 10.5194/bg-8-1523-2011
Langille, M. G. I., Zaneveld, J., Caporaso, J. G., McDonald, D., Knights, D., Reyes, J. A., et al. (2013). Predictive functional profiling of microbial communities using 16S rRNA marker gene sequences. Nat. Biotechnol. 31, 814–821. doi: 10.1038/nbt.2676
Lefcheck, J. S. (2016). piecewiseSEM: piecewise structural equation modelling in r for ecology, evolution, and systematics. Methods Ecol. Evol. 7, 573–579. doi: 10.1111/2041-210X.12512
Li, H., Yang, S., Semenov, M. V., Yao, F., Ye, J., Bu, R., et al. (2021). Temperature sensitivity of SOM decomposition is linked with a K-selected microbial community. Glob. Chang. Biol. 27, 2763–2779. doi: 10.1111/gcb.15593
Ling, L., Fu, Y., Jeewani, P. H., Tang, C., Pan, S., Reid, B. J., et al. (2021). Organic matter chemistry and bacterial community structure regulate decomposition processes in post-fire forest soils. Soil Biol. Biochem. 160:108311. doi: 10.1016/j.soilbio.2021.108311
Louca, S., and Doebeli, M. (2017). Efficient comparative phylogenetics on large trees. Bioinformatics 34, 1053–1055. doi: 10.1093/bioinformatics/btx701
Louca, S., Doebeli, M., and Parfrey, L. W. (2018). Correcting for 16S rRNA gene copy numbers in microbiome surveys remains an unsolved problem. Microbiome 6, 1–12. doi: 10.1186/s40168-018-0420-9
Lozupone, C., and Knight, R. (2005). UniFrac : a New Phylogenetic Method for Comparing Microbial Communities UniFrac : a New Phylogenetic Method for Comparing Microbial Communities. Appl. Environ. Microbiol. 71, 8228–8235. doi: 10.1128/AEM.71.12.8228
Maestrini, B., Alvey, E. C., Hurteau, M. D., Safford, H., and Miesel, J. R. (2017). Fire severity alters the distribution of pyrogenic carbon stocks across ecosystem pools in a Californian mixed-conifer forest. J. Geophys. Res. Biogeosci. 122, 2338–2355. doi: 10.1002/2017JG003832
McMurdie, P. J., and Holmes, S. (2014). Waste Not, Want Not: why Rarefying Microbiome Data Is Inadmissible. PLoS Comput. Biol. 10:e1003531. doi: 10.1371/journal.pcbi.1003531
Miesel, J. R., Hockaday, W. C., Kolka, R. K., and Townsend, P. A. (2015). Soil organic matter composition and quality across fire severity gradients in coniferous and deciduous forests of the southern boreal region. J. Geophys. Res. Biogeosci. 120, 1124–1141. doi: 10.1002/2015JG002959
MTBS (2017). Monitoring trends in burn severity. Available Online at: https://www.mtbs.gov (accessed December 15, 2017).
NCEI-NOAA (2017). National centers for environmental information. Available Online at: https://www.ncei.noaa.gov (accessed August 4, 2020).
Nemergut, D. R., Knelman, J. E., Ferrenberg, S., Bilinski, T., Melbourne, B., Jiang, L., et al. (2016). Decreases in average bacterial community rRNA operon copy number during succession. ISME J. 10, 1147–1156. doi: 10.1038/ismej.2015.191
Oksanen, J., Blanchet, F. G., Friendly, M., Kindt, R., Legendre, P., McGlinn, D., et al. (2019). vegan: Community Ecology Package. Version: 2.5-7.
Olsen, S. R., Cole, C. V., Watanable, F. S., and Dean, L. A. (1954). Estimation of available phosphorus in soils by extraction with sodium bicarbonate. Washington, D.C: U.S. Dept. of Agriculture.
Parsons, A., Robichaud, P. R., Lewis, S. A., Napper, C., Clark, J. T., and Jain, T. B. (2010). Field guide for mapping post-fire soil burn severity. Gen. Tech. Rep. RMRS-GTR-243. Fort Collins, CO: U.S. Department of Agriculture, Forest Service, Rocky Mountain Research Station.
Paul, E. A., Morris, S. J., and Bohm, S. (2001). “The determination of soil C pool sizes and turnover rates: Biophysical fractionation and tracers,” in Assessment Methods for Soil Carbon, eds R. Lal, J. M. Kimble, R. F. Follett, and B. A. Stewart (Boca Raton, Florida: CRC Press LLC), 193–206.
Paul, E. A., Morris, S. J., Conant, R. T., and Plante, A. F. (2006). Does the acid hydrolysis-incubation method measure meaningful soil organic carbon pools? Soil Sci. Soc. Am. J. 70, 1023–1035. doi: 10.2136/sssaj2005.0103
Pedregosa, F., Varoquaux, G., Gramfort, A., Michel, V., Thirion, B., Grisel, O., et al. (2011). Scikit-learn: machine learning in Python. J. Mach. Learn. Res. 12, 2825–2830. doi: 10.1080/13696998.2019.1666854
Pérez-Valera, E., Goberna, M., and Verdú, M. (2019). Fire modulates ecosystem functioning through the phylogenetic structure of soil bacterial communities. Soil Biol. Biochem. 129, 80–89. doi: 10.1016/j.soilbio.2018.11.007
Perry, D. A., Oren, R., and Hart, S. C. (2008). Forest Ecosystems. Baltimore: Johns Hopkins University Press.
Pinheiro, J., Bates, D., Debroy, S., and Sarkar, D. (2019). nlme: Linear and nonlinear mixed effects models. Version 3.1-157.
Pinheiro, J. C., and Bates, D. M. (2000). Mixed-effects models in S and S-Plus. New York: Springer-Verlag.
Podschwit, H. R., Larkin, N. K., Steel, E. A., Cullen, A., and Alvarado, E. (2018). Multi-model forecasts of very-large fire occurences during the end of the 21st century. Climate 6, 1–21. doi: 10.3390/cli6040100
Prendergast-Miller, M. T., de Menezes, A. B., Macdonald, L. M., Toscas, P., Bissett, A., Baker, G., et al. (2017). Wildfire impact: natural experiment reveals differential short-term changes in soil microbial communities. Soil Biol. Biochem. 109, 1–13. doi: 10.1016/j.soilbio.2017.01.027
Pressler, Y., Moore, J. C., and Cotrufo, M. F. (2018). Belowground community responses to fire: meta-analysis reveals contrasting responses of soil microorganisms and mesofauna. Oikos 128, 1–19. doi: 10.1111/oik.05738
Price, M. N., Dehal, P. S., and Arkin, A. P. (2010). FastTree 2 - Approximately maximum-likelihood trees for large alignments. PLoS One 5:e9490. doi: 10.1371/journal.pone.0009490
Quast, C., Pruesse, E., Yilmaz, P., Gerken, J., Schweer, T., Yarza, P., et al. (2013). The SILVA ribosomal RNA gene database project: improved data processing and web-based tools. Nucleic Acids Res. 41, 590–596. doi: 10.1093/nar/gks1219
R Core Team (2019). R: A language and environment for statistical computing. Vienna, Austria: R Foundation for Statistical Computing.
Ramirez, K. S., Craine, J. M., and Fierer, N. (2012). Consistent effects of nitrogen amendments on soil microbial communities and processes across biomes. Glob. Chang. Biol. 18, 1918–1927. doi: 10.1111/j.1365-2486.2012.02639.x
Rodríguez, J., González-Pérez, J. A., Turmero, A., Hernández, M., Ball, A. S., González-Vila, F. J., et al. (2017). Wildfire effects on the microbial activity and diversity in a Mediterranean forest soil. Catena 158, 82–88. doi: 10.1016/j.catena.2017.06.018
Rodríguez, J., González-Pérez, J. A., Turmero, A., Hernández, M., Ball, A. S., González-Vila, F. J., et al. (2018). Physico-chemical and microbial perturbations of Andalusian pine forest soils following a wildfire. Sci. Total Environ. 634, 650–660. doi: 10.1016/j.scitotenv.2018.04.028
Roller, B. R. K., Stoddard, S. F., and Schmidt, T. M. (2016). Exploiting rRNA operon copy number to investigate bacterial reproductive strategies. Nat. Microbiol. 1, 1–7. doi: 10.1038/nmicrobiol.2016.160
Romillac, N., and Santorufo, L. (2021). Transferring concepts from plant to microbial ecology: a framework proposal to identify relevant bacterial functional traits. Soil Biol. Biochem. 162:108415. doi: 10.1016/j.soilbio.2021.108415
Ruefenacht, B., Finco, M. V., Nelson, M. D., Czaplewski, R., Helmer, E. H., Blackard, J. A., et al. (2008). Conterminous U.S. and Alaska Forest Type Mapping Using Forest Inventory and Analysis Data. Photogramm. Eng. Remote Sens. 74, 1379–1388. doi: 10.14358/PERS.74.11.1379
Sáenz de Miera, L. E., Pinto, R., Gutierrez-Gonzalez, J. J., Calvo, L., and Ansola, G. (2020). Wildfire effects on diversity and composition in soil bacterial communities. Sci. Total Environ. 726:138636. doi: 10.1016/j.scitotenv.2020.138636
Schimel, J. P., and Schaeffer, S. M. (2012). Microbial control over carbon cycling in soil. Front. Microbiol. 3:348. doi: 10.3389/fmicb.2012.00348
Schmidt, M. W. I., Torn, M. S., Abiven, S., Dittmar, T., Guggenberger, G., Janssens, I. A., et al. (2011). Persistence of soil organic matter as an ecosystem property. Nature 478, 49–56. doi: 10.1038/nature10386
Sinsabaugh, R. L., Reynolds, H., and Long, T. M. (2000). Rapid assay for amidohydrolase (urease) activity in environmental samples. Soil Biol. Biochem. 32, 2095–2097. doi: 10.1016/S0038-0717(00)00102-4
Soil Survey Staff, Natural Resources Conservation Service [NRCS], and United States Department of Agriculture [USDA] (2015). Official soil series descriptions. Available online at: www.nrcs.usda.gov (accessed January 25, 2016).
Soil Survey Staff, Natural Resources Conservation Service [NRCS], and United States Department of Agriculture [USDA] (2016). Web Soil Survey. Available online at: http://websoilsurvey.sc.egov.usda.gov/ (accessed August 4, 2020).
Stoddard, S. F., Smith, B. J., Hein, R., Roller, B. R. K., and Schmidt, T. M. (2015). rrnDB: improved tools for interpreting rRNA gene abundance in bacteria and archaea and a new foundation for future development. Nucleic Acids Res. 43, D593–D598. doi: 10.1093/nar/gku1201
Taş, N., Prestat, E., McFarland, J. W., Wickland, K. P., Knight, R., Berhe, A. A., et al. (2014). Impact of fire on active layer and permafrost microbial communities and metagenomes in an upland Alaskan boreal forest. ISME J. 8, 1904–1919. doi: 10.1038/ismej.2014.36
Toole, D. R., Zhao, J., Martens-Habbena, W., and Strauss, S. L. (2021). Bacterial functional prediction tools detect but underestimate metabolic diversity compared to shotgun metagenomics in southwest Florida soils. Appl. Soil Ecol. 168:104129. doi: 10.1016/j.apsoil.2021.104129
Torn, M. S., Kleber, M., Zavaleta, E. S., Zhu, B., Field, C. B., and Trumbore, S. E. (2013). A dual isotope approach to isolate soil carbon pools of different turnover times. Biogeosciences 10, 8067–8081. doi: 10.5194/bg-10-8067-2013
Treseder, K. K., Balser, T. C., Bradford, M. A., Brodie, E. L., Dubinsky, E. A., Eviner, V. T., et al. (2012). Integrating microbial ecology into ecosystem models: challenges and priorities. Biogeochemistry 109, 7–18. doi: 10.1007/s10533-011-9636-5
Trumbore, S. (2000). Age of soil organic matter and soil respiration: radiocarbon constraints of belowground C dynamics. Ecol. Appl. 10, 399–411. doi: 10.1890/1051-0761(2000)010[0399:aosoma]2.0.co;2
Wagg, C., Schlaeppi, K., Banerjee, S., Kuramae, E. E., and van der Heijden, M. G. A. (2019). Fungal-bacterial diversity and microbiome complexity predict ecosystem functioning. Nat. Commun. 10, 1–10. doi: 10.1038/s41467-019-12798-y
Wan, S., Hui, D., and Luo, Y. (2001). Fire effects on nitrogen pools and dynamics in terrestrial ecosystems: a meta-analysis. Ecol. Appl. 11, 1349–1365. doi: 10.1890/1051-0761(2001)011[1349:feonpa]2.0.co;2
Wang, J., Wu, Y., Li, J., He, Q., and Bing, H. (2021). Soil enzyme stoichiometry is tightly linked to microbial community composition in successional ecosystems after glacier retreat. Soil Biol. Biochem. 162:108429. doi: 10.1016/j.soilbio.2021.108429
Wang, Q., Zhong, M., and Wang, S. (2012). A meta-analysis on the response of microbial biomass, dissolved organic matter, respiration, and N mineralization in mineral soil to fire in forest ecosystems. For. Ecol. Manage. 271, 91–97. doi: 10.1016/j.foreco.2012.02.006
Weber, C. F., Lockhart, J. S., Charaska, E., Aho, K., and Lohse, K. A. (2014). Bacterial composition of soils in ponderosa pine and mixed conifer forests exposed to different wildfire burn severity. Soil Biol. Biochem. 69, 242–250. doi: 10.1016/j.soilbio.2013.11.010
Weiss, S., Xu, Z. Z., Peddada, S., Amir, A., Bittinger, K., Gonzalez, A., et al. (2017). Normalization and microbial differential abundance strategies depend upon data characteristics. Microbiome 5, 1–18. doi: 10.1186/s40168-017-0237-y
Westerling, A. L. (2006). Warming and Earlier Spring Increase Western U.S. Forest Wildfire Activity. Science 313, 940–943. doi: 10.1126/science.1128834
Whitman, T., Pepe-Ranney, C., Enders, A., Koechli, C., Campbell, A., Buckley, D. H., et al. (2016). Dynamics of microbial community composition and soil organic carbon mineralization in soil following addition of pyrogenic and fresh organic matter. ISME J. 10, 2918–2930. doi: 10.1038/ismej.2016.68
Whitman, T., Whitman, E., Woolet, J., Flannigan, M. D., Thompson, D. K., and Parisien, M.-A. (2019). Soil bacterial and fungal response to wildfires in the Canadian boreal forest across a burn severity gradient. Soil Biol. Biochem. 138:107571. doi: 10.1016/j.soilbio.2019.107571
Wilhelm, R. C. (2016). Deciphering decomposition and the effects of disturbance in forest soil microbial communities with metagenomics and stable isotope probing. Ph.D. thesis. Vancouver: University of British Columbia Library. doi: 10.14288/1.0319123
Woolet, J., and Whitman, T. (2020). Pyrogenic organic matter effects on soil bacterial community composition. Soil Biol. Biochem. 141:107678. doi: 10.1016/j.soilbio.2019.107678
Wu, L., Yang, Y., Chen, S., Jason Shi, Z., Zhao, M., Zhu, Z., et al. (2017). Microbial functional trait of rRNA operon copy numbers increases with organic levels in anaerobic digesters. ISME J. 11, 2874–2878. doi: 10.1038/ismej.2017.135
Xiang, X., Shi, Y., Yang, J., Kong, J., Lin, X., Zhang, H., et al. (2014). Rapid recovery of soil bacterial communities after wildfire in a Chinese boreal forest. Sci. Rep. 4, 1–8. doi: 10.1038/srep03829
Keywords: microbial metabolism, microbial community composition, fire severity, soil carbon stability, soil carbon pools
Citation: Adkins J, Docherty KM and Miesel JR (2022) Copiotrophic Bacterial Traits Increase With Burn Severity One Year After a Wildfire. Front. For. Glob. Change 5:873527. doi: 10.3389/ffgc.2022.873527
Received: 10 February 2022; Accepted: 08 April 2022;
Published: 11 May 2022.
Edited by:
Nicasio T. Jiménez-Morillo, University of Évora, PortugalReviewed by:
Caroline Poyntner, University of Innsbruck, AustriaEvgeny Abakumov, Saint Petersburg State University, Russia
Copyright © 2022 Adkins, Docherty and Miesel. This is an open-access article distributed under the terms of the Creative Commons Attribution License (CC BY). The use, distribution or reproduction in other forums is permitted, provided the original author(s) and the copyright owner(s) are credited and that the original publication in this journal is cited, in accordance with accepted academic practice. No use, distribution or reproduction is permitted which does not comply with these terms.
*Correspondence: Jaron Adkins, amFyb24uYWRraW5zQHVzdS5lZHU=
†Present address: Jaron Adkins, Department of Watershed Sciences, Utah State University, Logan, UT, United States