- 1Geography, College of Life and Environmental Sciences, University of Exeter, Exeter, United Kingdom
- 2School of Geographical Sciences, University of Bristol, Bristol, United Kingdom
Quantifying resilience of socio-ecological systems (SES) can be invaluable to delineate management strategies of natural resources and aid the resolution of socio-environmental conflicts. However, resilience is difficult to quantify and the factors contributing to it are often unknown. We provide a theoretical and conceptual framework to quantify resilience in a long-term context. Our approach uses elements from interdisciplinarity and network perspectives to establish links and causalities between social and ecological variables and resilience attributes. The evaluation and modeling of SES structure and function are established from the analysis of dynamic Bayesian networks (DBN). DBN models allow quantifying resilience through probabilities and offer a platform of interdisciplinary dialogue and an adaptive framework to address questions on ecosystem monitoring and management. The proposed DBN is tested in Monquentiva, a SES located in the high Andes of Colombia. We determined historical socio-ecological resilience from paleoecological evidence (palynological diversity, forest cover, fires, and precipitation) and social-economic factors (governance, social organization, and connectivity) between 1920 and 2019. We find that transformation processes in Monquentiva are mainly related to social change (e.g., social organization) and increased ecological diversity that in turn have fostered SES resilience between 1980 and 2019. The ability to predict the SES response over time and under cumulative, non-linear interactions across a complex ecosystem highlights the utility of DBNs for decision support and environmental management. We conclude with a series of management and policy-relevant applications of the DBN approach for SES resilience assessment.
Introduction
The resilience of socio-ecological systems (SES) has become a focal concept for the management of natural resources and resolution of socio-environmental conflicts (Folke et al., 2004). Studies investigating the patterns and drivers of ecosystem resilience extend from ecology and natural resource management to food security, community planning, and disaster management (Folke et al., 2010; Allen et al., 2016; Eason et al., 2016). Efforts to apply resilience concepts within these fields have stimulated an interest in assessing and measuring resilience from multiple disciplinary perspectives (Allen et al., 2016; Quinlan et al., 2016). The application and measurement of resilience are varied and often only indirectly linked to theoretical frameworks. Resilience assessments that are adaptive and quantitative, and integrate interdisciplinary and network perspectives, are needed to account for the dynamic nature of SES under environmental and social change.
Resilience theory provides a framework for integrating ecosystem dynamics and environmental change to inform conservation and management of SES. Two aspects of resilience described in the literature are: (i) Ecological resilience, referring to the capacity of an ecosystem to absorb disturbance without changing structure and function (Gunderson, 2000) and (ii) engineering resilience—defined as the capacity of an ecosystem to recover from a disturbance that causes change in structure and function (Holling, 1973). However, resilience is more than being persistent or robust to disturbance. Resilience emerges from cumulative effects, feedback loops, and the dynamic systems that characterize a SES (Angeler and Allen, 2016). Resilience also relates to opportunities that disturbances provide for renewal and recombination of processes and the emergence of new trajectories in a system (Berkes et al., 2008). As such, resilience also involves three main system capacities: absorption, adaptation, and transformation (Carpenter et al., 2001; Elmqvist et al., 2019), which should all be incorporated into resilience assessments of SES.
Assessing SES resilience can be challenging and requires integrating approaches, knowledge, and theories from different disciplines (Ostrom, 2009; Quinlan et al., 2016). In practice, SES resilience assessment is often developed from the perspective of a single discipline and tends to oversimplify ecological and social subsystems (Cote and Nightingale, 2012; Schulter et al., 2014). However, SES resilience assessments should integrate the knowledge produced across disciplines including qualitative indicators and variables (Linstadter et al., 2016). Interdisciplinarity refers to integrative research that ties the principles from multiple disciplines together into one cohesive body of work, where the whole is more than the sum of its parts (Davidson, 2015). In our experience, interdisciplinarity is a site-specific but intensive dialogue between social and ecological experts that aims at a cross-disciplinary appreciation of socio-ecological resilience. An interdisciplinary framework needs to be holistic and embrace complexity. Complex systems research has addressed how decentralized local interactions of social and ecological agents give rise to collective outcomes (Crawford et al., 2005), such as a cooperation action between resource users.
A key conceptual challenge discussed in the social sciences is the view of resilience as a normative property, as if resilience is always a value-free, desirable end-state. Normative connotations of resilience risk introducing fallacies inherent in the unequal power relations created by resilience discourse, which is to say, presenting as necessary and good for communities “resilient” policy decisions that have been made for economic or political reasons (Cote and Nightingale, 2012; Elmqvist et al., 2019). Resilient systems, however, are not necessarily sustainable, nor are sustainable systems inherently resilient to disturbance and change. Ideally, sustainable systems are resilient in a desired state and have a high likelihood to maintain that desired state over time (Allen et al., 2014). A further challenge is to delimitate and specify interdependencies of subsystems within a SES (Berkes et al., 2008). The latter is also a prerequisite for understanding how cross-scale interactions affect the coupling between social and ecological subsystems (Allen et al., 2014; Quinlan et al., 2016), and the implications for overall SES resilience. Research on SES resilience often involves a limited consideration of temporal scales (Quinlan et al., 2016). However, SES resilience assessment inherently requires evaluation of disturbance response over long temporal frameworks (Schulter et al., 2014). Resilience also needs to be evaluated against a baseline or a non-static desirable state, that can only be established by looking at long-term system behavior.
Any approach aiming to assess and quantify SES resilience requires the integration of ideas from social (Callon, 1984; Latour, 2005) and environmental (Wu and David, 2002) sciences and focus on human-ecosystem frameworks. For example, a network perspective that has gained strength within contemporary social sciences, and that is relevant to this interdisciplinary endeavor, is the actor-network theory (ANT) proposed by Bruno Latour and Michel Callon. ANT delineates the interaction of networks based on the experiences of the actors in a SES, without reproducing the disciplinary division between social and natural sciences (Latour, 2005). Inspired by ANT, we see the resilience to be measured as the result of place-based processes, in particular, of the experiences and responses of actors facing social and/or ecological disturbances. Actors then respond by absorbing, adapting to, or transforming the disturbances. The notion of resilience is a “bridging concept” in this sense, between the natural and social sciences (Davoudi et al., 2012).
We present an overview of the state of the art on socio-ecological resilience concepts and modeling, and Bayesian networks (BN) to assess SES resilience overtime. First, we review the definitions of SES resilience in the literature and discuss the challenges of integrating long-term social and ecological information. Second, we present definitions of SES resilience and measures from the literature, including qualitative and quantitative assessments and their application to SES resilience. Third, we compile information on network theory and address advantages and disadvantages of network approaches. We review how BN and dynamic Bayesian networks (DBN) operate and their application to model and assess SES resilience using long-term information. We also discuss a dynamic Bayesian network approach to build temporally robust models of SES resilience. This dynamic approach takes the long-term perspective from a plurality of data sources and an array of interdisciplinary approaches linked to paleoecology, archeology, anthropology, and history. Finally, we present an application of this approach to a SES in the Colombian Andes.
Social and ecological information in socio-ecological systems resilience assessments
SES are complex, integrated systems in which humans are part of nature (Berkes et al., 2008) and where nature is also composed of human and non-human elements (Latour, 2005). Resilience in SES depends on the strength of feedbacks arising from interactions between the social and ecological elements of a system e.g., biophysical processes, abiotic structure, species composition, human actors, and socio-economic processes (Figure 1; Berkes et al., 2000). Long-term ecological records can describe the conditions and dynamics of ecosystems and natural environments (Willis and MacDonald, 2011) and the changes a SES has undergone during the past. Records of forest and fire dynamics, climate change, and human-landscape interactions are important for understanding ecological processes within SES. Such data are normally obtained from both paleoecological and archeological records and span centuries to millennia (Figure 2). On the other hand, long-term socio-cultural information can include archeological, anthropological, and historical records documenting past social structures, organizational practices, economic factors, or adaptive strategies (Grier et al., 2017). In timescales of centuries to millennia, archeology is the primary source of socio-cultural data. Historical information from archives and ethnohistories can also offer a long-term perspective of these changes (Figure 2). An approach that combines questions, theory, and analysis of social and biophysical information to quantify resilience in a long-term context still needs to be fully developed. This is partially due to poor understanding of how each data source operates in the long term, and the challenges behind their integration around resilience theory frameworks (Dearing et al., 2015) and the disciplinary infrastructures of each information source (Redman et al., 2003; Butzer, 2005).
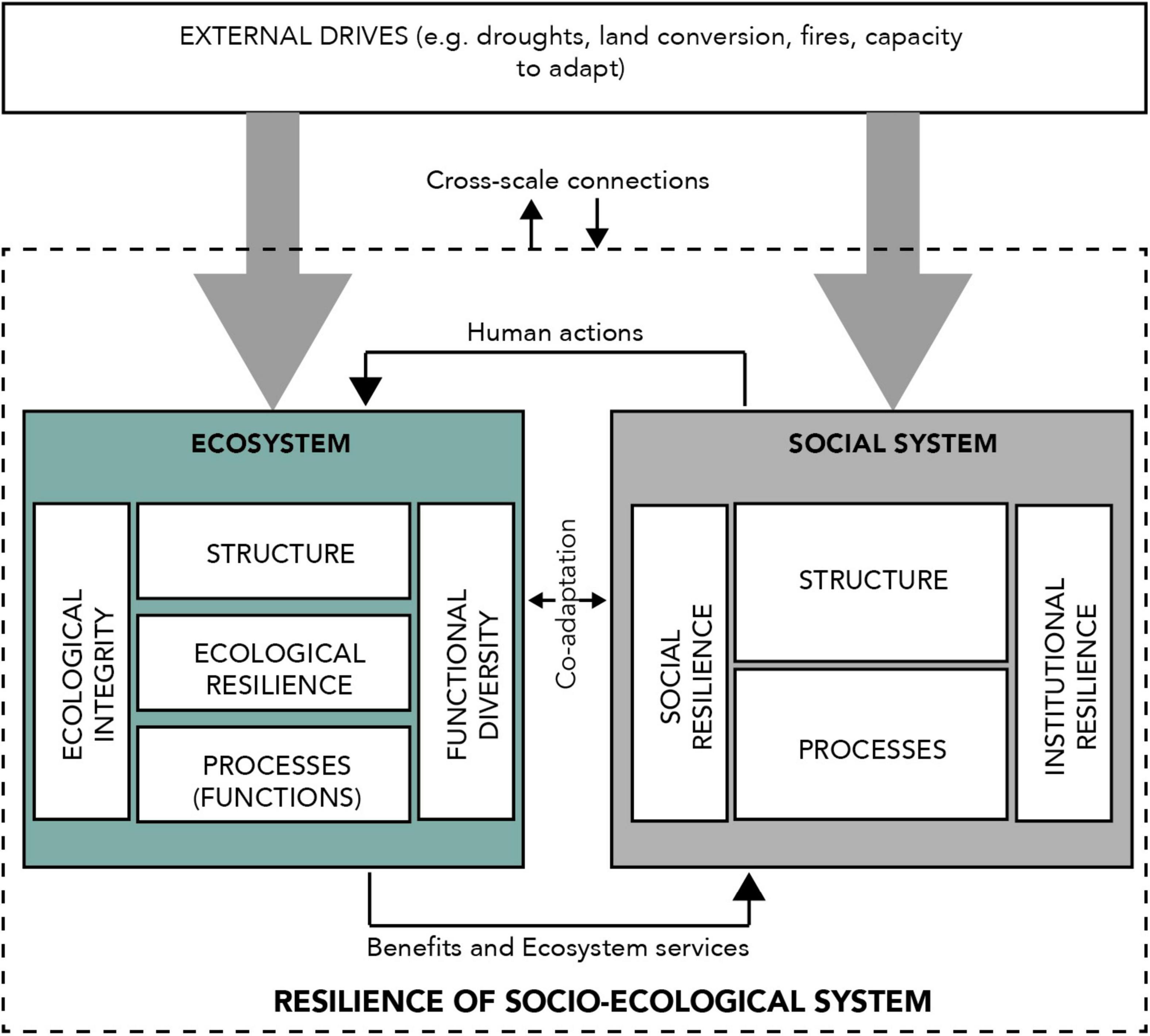
Figure 1. The conceptual framework for a resilience assessment of socio-ecological systems [adapted and modified from Berkes et al. (2000)].
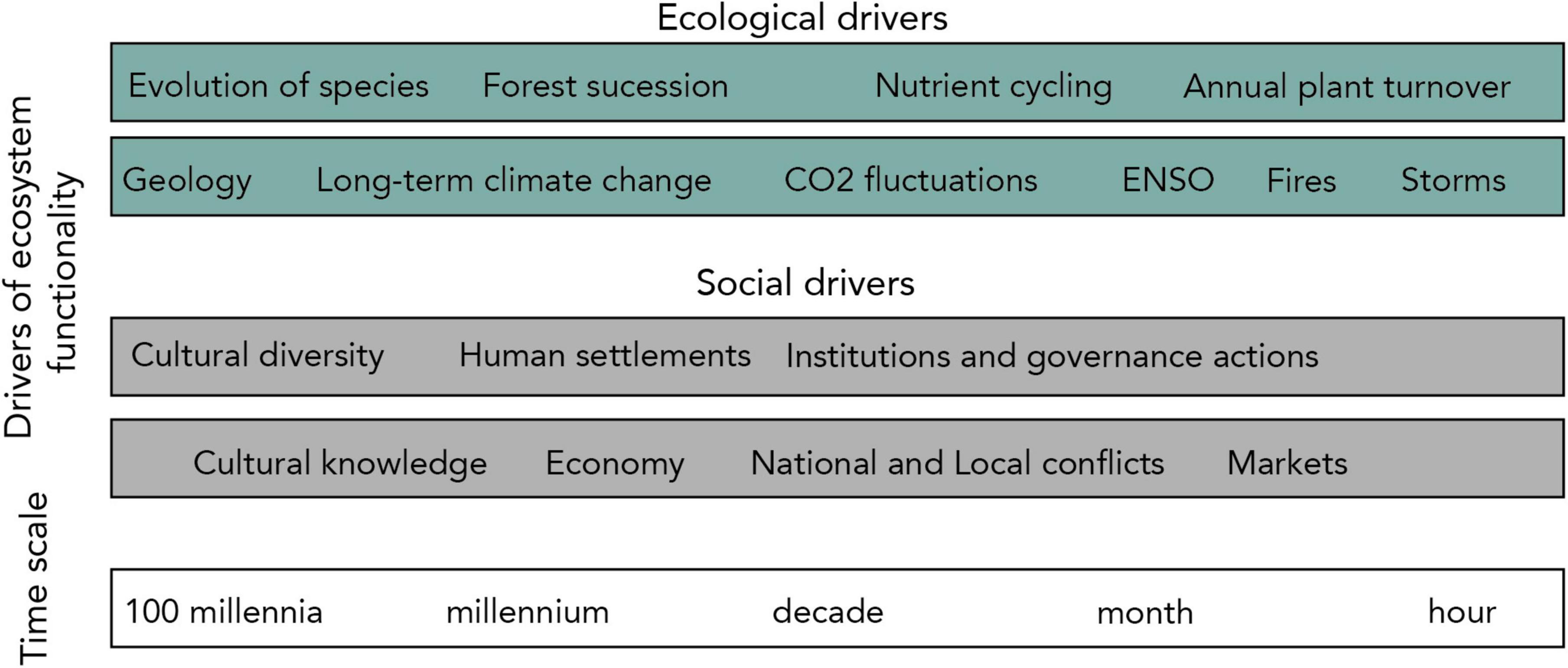
Figure 2. Multi-temporal variation of ecological (green bars) and social drivers (gray bars) of change in a socio-ecological system.
An integrated approach to assess socio-ecological systems resilience
Defining socio-ecological systems resilience and measures
To assess long-term resilience effectively, we need to adopt a definition of resilience for SES that clearly describes relationships between both systems: social and ecological. One key aspect of socio-ecological resilience is the capacity of the system to absorb disturbance and reorganize while the same core structure and function are retained over time (Carpenter et al., 2001; Folke, 2006). Absorption capacity relies on strategies or actions to cope with change rather than instigating a significant change to reduce or avoid future disturbances (Béné et al., 2016). Resilience in SES also includes two other aspects: adaptive and transformative capacities (Carpenter et al., 2001). The adaptive capacity refers to the ability of the system to learn, combine experience and knowledge, adjust responses to disturbances, and continue operating (Berkes et al., 2008). The transformative capacity corresponds to reaching a new system state when ecological or social structures are reorganized in more fundamental ways, such as by changing key institutional arrangements (Walker et al., 2004; Figure 3). An essential aspect of this conceptualization is the recognition that resilience results from the combination of the three capacities: (1) absorptive capacity leading to persistence, (2) adaptive capacity leading to incremental adjustments/changes and adaptation, and (3) transformative capacity leading to transformational responses (Carpenter et al., 2001; Béné et al., 2016).
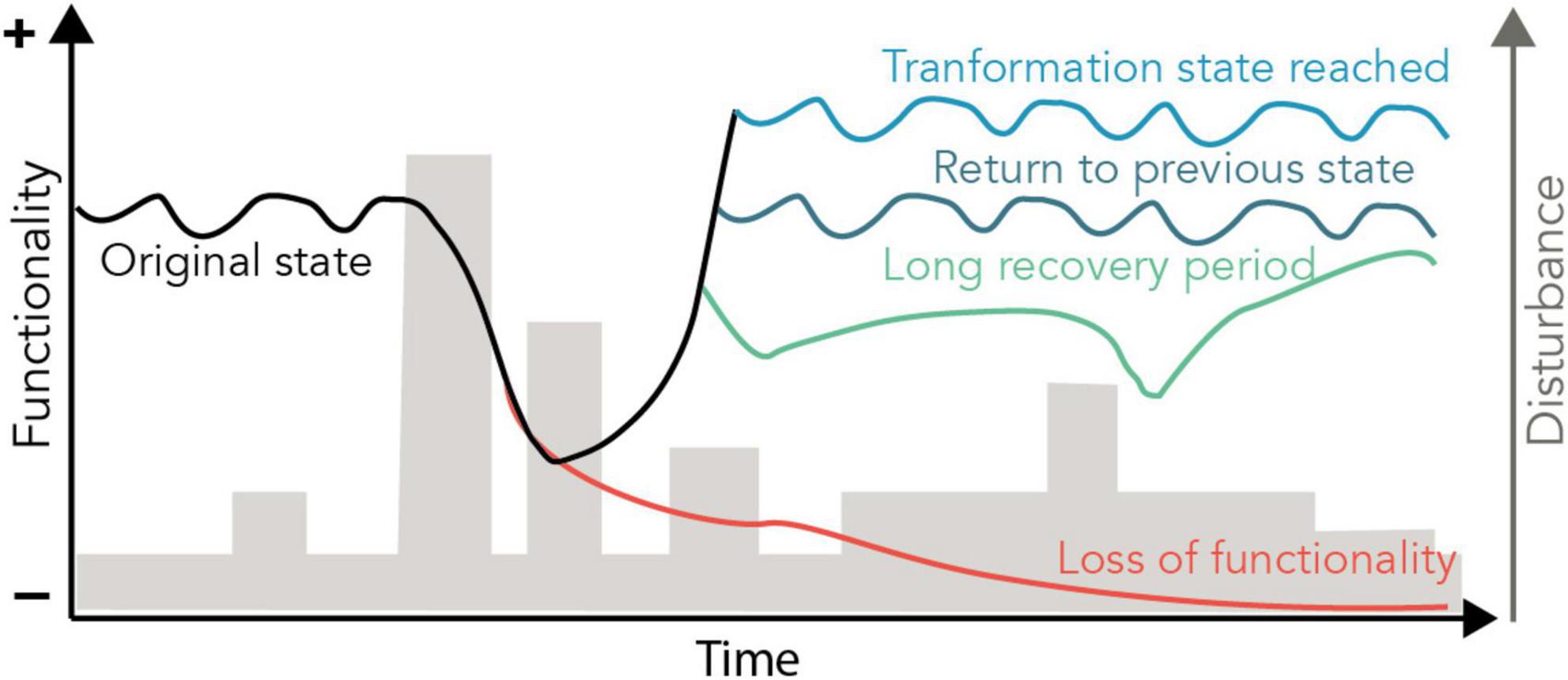
Figure 3. A graphic depiction of resilience in terms of functionality illustrating possible ways in which a socio-ecological system may respond to disturbances. The gray silhouette represents the frequency of disturbance over time.
Resilience is also connected to functionality. Functionality in a SES is closely linked to service provision and can be conceptualized based on system function in ecological and social terms (Angeler and Allen, 2016; Nash et al., 2016). If SES functionality does not persist following a disturbance, the system cannot absorb, adapt, or recover its core structure. As such, the state of the system’s functionality after disturbance is a crucial factor determining its resilience (Figure 3). Capacities of absorption, adaptation, and transformation of SES can be conceptualized in terms of functionality to generate resilience measures (Renschler et al., 2010). However, resilience measures based on functionality and resilience capacities are challenging as non-linearity emerges from the cumulative interactions of disturbances and environmental, ecological, economic, and socio-cultural processes over time (Holling, 2001). Functionality can serve as a proxy of resilience and its assessment should involve uncertainties associated with biological systems, social complexities, and our current understanding of the SES dynamics. Thus, heuristic models and integrative methods are needed to capture system uncertainties and dynamics linked to SES resilience.
Resilience assessments in the literature involve qualitative and quantitative approaches (Angeler and Allen, 2016; Quinlan et al., 2016). Examples of qualitative resilience assessments involve interviews and participatory methods with stakeholders (Wilson and Wilson, 2019; Saja et al., 2021). Participatory methods from the social sciences can incorporate social and ecological variables under the umbrella of methods such as ethnography, which involve collecting data through immersing the researcher in the daily life of socio-ecological communities, so as to produce detailed and “thick descriptions” of socio-environmental relationships. Participatory methods can also involve the collection of qualitative data through methods such as oral histories, interviews, focus groups, and social cartographies, with the participatory element allowing researcher experience to ground these specific insights into everyday practice or experience in broader contexts, including wider biophysical processes that have historically influenced the territory (Hastrup, 2013). These methods are relevant to interdisciplinary processes as they tend to increase awareness of resilience and its drivers but provide limited metrics and long-term dynamics of SES. Meanwhile, quantitative methods identify underlying patterns and causal explanations of SES change and have been divided into two types: metric-based and model-based approaches (Angeler and Allen, 2016). Metric-based approaches use measures of individual properties to assess overall system performance, whereas model-based approaches use system configuration modeling to predict the system evolution (Linkov and Kott, 2019). Several efforts have focused on developing metrics applicable to SES (Kerner and Thomas, 2014; Cushman and McGarigal, 2019), including long-term perspectives (Dearing, 2008). However, the lack of universally applicable resilience metrics and the inability to formalize these metrics have been barriers to implementing consistent approaches to SES resilience (Linkov and Kott, 2019) that can inform management and policy. Model-based approaches focus on representing the real world and defining resilience using mathematical and physical concepts applied to SES (Linkov and Kott, 2019). Modeling the SES performance is information-demanding and requires a detailed understanding of the social and ecological aspects of a system to simulate resilience capacities and, consequently, system functionality. In the following sections, we focus on BN to model SES resilience. This approach combines an assessment of resilience capacities and functionality through network structures and modeling.
A network perspective for socio-ecological systems resilience
Network perspectives, which provide an effective tool for analyzing and visualizing complex interactions, can be used to model past social and ecological interactions within a SES (Moore et al., 2016; Sayles et al., 2019), and to characterize the flowing of material, energy, and information between the SES constituents (Cote and Nightingale, 2012). A network perspective can thus be effective for understanding the structure and function of SES—specifically, by incorporating graph theory and statistics with descriptions of social and ecological dimensions, depicted in terms of nodes and links (Newman, 2004). A network can represent a given system in terms of its localized variables (i.e., nodes) and the relations between those variables (i.e., links) (Figure 4A). The links in a network are usually directed (i.e., one-way or two-way connections/arrows), and they can depict any relations between linked nodes. The nature of connections can be entirely social, entirely ecological, or a mixture of both components. For instance, a socio-ecological relationship may consist of resource extraction by peasants from their environment. Human activities can create a socio-ecological network by linking ecological nodes, where two independent ecological systems can become connected by human practices (e.g., ancient communities in the tropical Andes have developed their productive activities at various altitudes, and as a result, previously unconnected high Andean vegetation has become interconnected (Langebaek, 1987). Such a graphic exercise linking components and their relations allows to produce a network perspective that can serve as a basis to develop functionality and resilience measures for the SES.
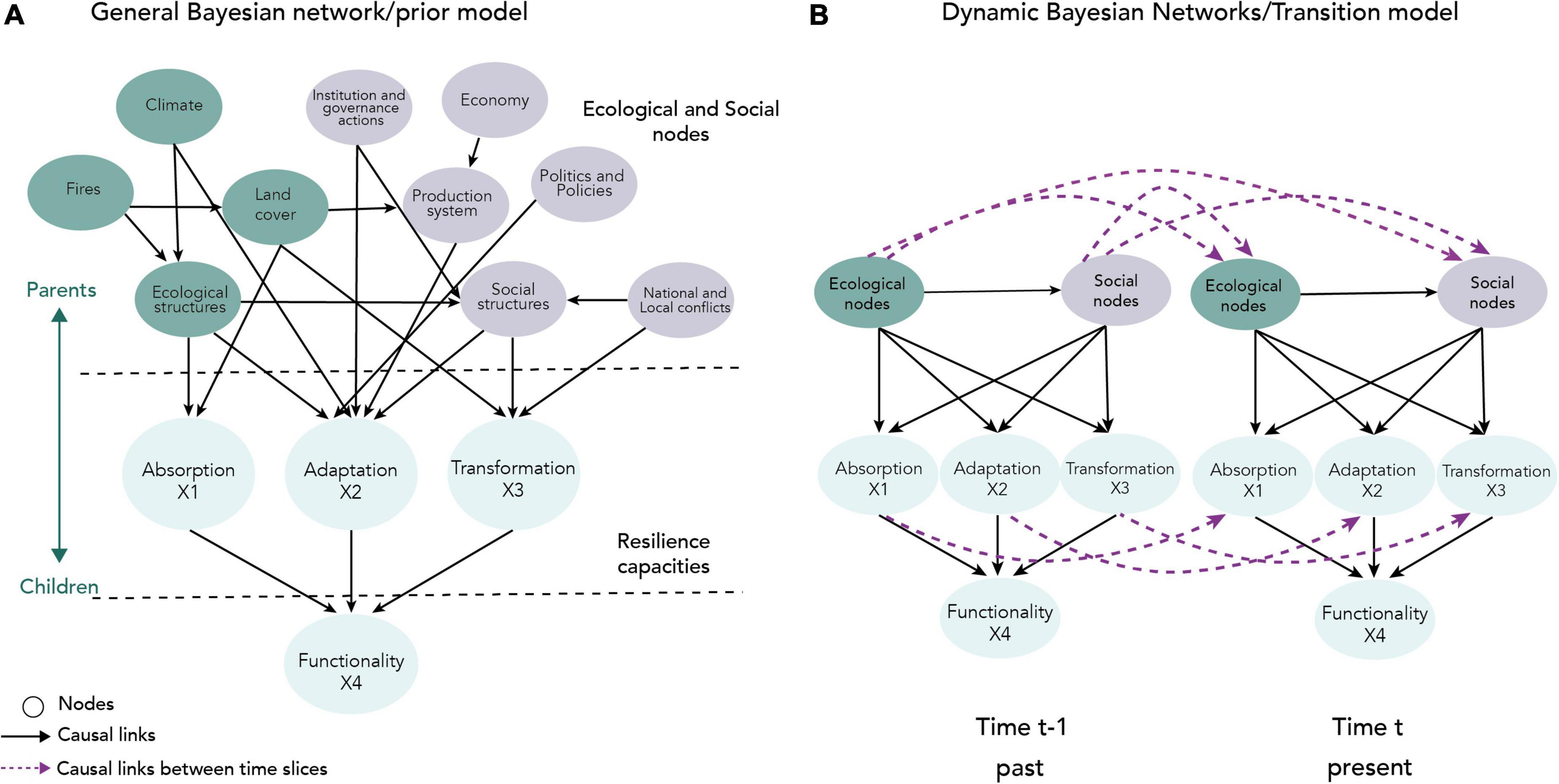
Figure 4. An example of general and dynamic Bayesian networks representing the influences of social and ecological drivers on SES functionality. (A) The prior model or general Bayesian network represents interactions that are instantaneous, (B) the transition model or dynamic Bayesian network represents interactions that are both instantaneous and temporal. Green circles highlight the ecological nodes and gray circles highlight social nodes that may be considered in the assessment of SES resilience.
A clear advantage of a network approach is that structural properties can be revealed and associated with SES resilience (Moore et al., 2016). Networks elucidate the structure of interactions between SES components and how this structure affects the performance and functions of the system (Janssen et al., 2006). The breakdown of network interactions over time can be related to the loss of functionality and resilience within the SES. Also, a socio-ecological network perspective in modeling resilience can provide a common ground where interdisciplinary and integrative approaches are explored (e.g., Quinlan et al., 2016). An expert consensus and stakeholder participation often provide valuable knowledge about the SES, and involving these stakeholders in the modeling process can facilitate communication and learning (Ruckelshaus et al., 2015). Participatory processes are also relevant to find the typological structure and links of networks. A collective building of networks allows an integrative resilience assessment and identifies reliable interactions that can support decision-making (Jakeman et al., 2006). As a result, SES resilience can be represented as interconnected networks composed of social and ecological nodes developed through interdisciplinary processes and stakeholder dialogues.
Bayesian networks to quantify socio-ecological system resilience
BN deal with the challenges of modeling complex networks where variables are linked through conditional probabilities (Marcot and Penman, 2019). Their ability to model associations from observational data sets has drawn attention from many disciplines, including biology and engineering (Aguilera et al., 2011; Wu et al., 2018). Key advantages of BN include integrating qualitative and quantitative information, incorporating uncertainty, and their graphical structure (Uusitalo, 2007). The BN graphical structure represents causalities in a modeled system, increasing modeling transparency (Jensen, 1996), and facilitating interdisciplinary communication with other disciplines and stakeholders (Voinov and Bousquet, 2010). For example, BN that integrate goals, interests, and opinions from stakeholders and experts have been used to address water availability (Henriksen et al., 2007), agricultural (Salliou et al., 2017), and forest (Gonzalez-Redin et al., 2016) systems. Usually, participatory dialogues with stakeholders and experts lay the conceptual and theoretical bases for the selection of nodes and understandings of their links in the BN (Angeon and Lardon, 2008). These participatory processes are the basis of the BN analysis and have the potential to deliver SES resilience assessments that are most relevant for management. The involvement of stakeholders also reduces the likelihood that policy or management strategies derived from the assessment result in socio-environmental conflicts.
Graphically, a BN is a snapshot of a system represented by a directed acyclic graph, consisting of nodes and links (Figure 4A). The BN graph is acyclic (i.e., without cycles), because link directions prevent a parent node from being its own child (i.e., without self-loops or parallel edges). The nodes represent variables, which can be states, processes, outcomes or drivers of specific components of the system. Each node contains the probability of occurrence of an event or state. Nodes are classified into parent or child, a parent node is the predecessor of a child node or successor in the BN. The links denote the causal relationships between nodes. For example, in Figure 4A, ecological and social nodes are predecessors of absorption (X1), adaptation (X2), and transformation (X3), which are the children of both sets of nodes. Likewise, nodes X1, X2, and X3 are the parents of the node functionality (X4).
BN operate by calculating the conditional probability distributions that measure the relationships between variables or nodes. Equation 1 represents the joint probability distribution of a BN consisting of n random variables or nodes (X). Each node Xi has a conditional probability distribution P[xi | pa(xi)] attached, where pa(xi) represents the parents of each node Xi in the graph. An advantage of BN is that the network development can help to identify relevant (or irrelevant) variables for one node of interest and relationships between variables. This provides structure to the interaction networks but also simplifies the joint probability distribution of variables necessary to represent the resilience model. The BN provides a compact representation of probabilities of all variables, defined as the product of the conditional probability related to each node:
Where X = x1…. xn, and pa(xi) is the set of parents of variable xi.
BN are able to provide reliable uncertainty estimates when explicitly included in the conditional probability distributions of nodes in the network. Building a prior BN gives structure to the interaction network and simplifies the joint probability distribution of variables necessary to represent the model. BN is particularly appropriate for dealing with inherent uncertainty, which tends to be a key issue in SES. To assess uncertainties in the probabilities of a BN, a sensitivity analysis is often performed. This allows identifying the most important and impactful variables and collecting the most relevant data for those variables (Kleijnen, 1995). As discussed in section “ Social and ecological information in SES resilience assessments,” assessing SES resilience requires long-term ecological and social information to quantify the cumulative effects of disturbance. Incorporating a temporal dimension to the modeling of SES is achieved by using an extension of BN known as the DBN. An example of a DBN for a SES representing the influence between socio-economic drivers, environmental pressures, and ecosystem function is shown in Figure 4. In DBN, nodes represent variables at points in time (Tucker and Liu, 2004). Therefore, the joint probability distribution of t = 0 is the same as Equation 1, whereas the joint probability distribution of the following time slices (t > 0) is:
Where xi(t) is the node i at time t and pa(xi(t)) are the parents of xi(t) in the graph. DBN incorporates a prior and a transition model, unrolling a single BN over time (Figure 4B). A prior model specifies the initial conditions of the Bayesian network (Figure 4A), while the transitional model defines how variables change over time (Figure 4B). The prior model represents the nodes and their connections that regulate SES functionality (Figure 4A). Based on the structure and causal relationships of the prior model, the transition model represents the relationships between drivers and functionality along two-time slides, past (t-1) and present (t) (Figure 4B), similar to Markov models (Tucker and Liu, 2004). In this context, DBN is an applicable method that assesses the resilience of SES through functionality by estimating the joint probability of all nodes or variables at the current time and all preceding times. For example, the conditional probability of the system to retain functionality (X4), in time t, is represented by P(Xt4| Xt1, Xt2, Xt3, Xt–14). Note that the temporal nature of the last example represents changes in functionality over time, where the causal knowledge of social and ecological drivers of change influences SES functionality. For this step, interdisciplinary understandings are essential to structure the prior model and provide the basis for assessing SES resilience.
An assumption underlying DBN is stationarity, because the structure and parameters of DBN are fixed over time. However, this assumption can be too restrictive and may not hold for social and ecological systems. There have been several efforts to relax the stationary assumption by extending each hidden node of Hidden Markov Model (HMM) into a DBN, which properly works the underlying time-evolving networks (Zhu and Wang, 2015). Similarly, this hybrid methodology could explore possible feedback effects of functionality at time t on social or ecological nodes at time t + 1. Quantifying resilience requires a temporal component, making management attempts based on static reference conditions insufficient. Combining DBN and HMM approaches can adopt a long-term perspective to model the probability that a SES maintains structure and functionality after disturbances. DBN allows quantification of probabilities that the SES switches from one functionality state to another and probabilities of function losses or gains over time.
A socio-ecological resilience assessment based on dynamic Bayesian networks and integrating long-term social and ecological data
The Bayesian network approach to analyze SES resilience focuses on the time-sensitive causal relationships between ecological and social drivers of change and three capacities of resilience to quantify ecosystem functionality. We propose the following six steps based on interdisciplinarity to build socio-ecological networks, and DBN for modeling and assessing SES resilience (Figure 5).
Step 1. Identifying social and ecological drivers of change in SES and nodes for the DBN. Given the complexity of SES, identifying these drivers is crucial to understanding SES dynamics and its responses to disturbance and change. This analysis should consider drivers and interaction effects that may not be readily apparent from the perspective of a single discipline. For example, drivers such as climate change, governance, economy, technology, policies, and global market change and their interactions can drive changes on SES (Johnson et al., 2021). Under our network approach, experts choose a priori the drivers of change which will constitute the nodes in the network. In this step of the assessment, it is crucial to establish causal links based on participatory approaches and interdisciplinary discussions.
Step 2. Identify and characterize the sources of disturbance. This step refers to specifying the disturbance regime to which the SES is subject, e.g., the type of disturbance, their frequency and intensity (White and Jentsch, 2001). Disturbances may include both human (e.g., fires, local conflict, and habitat fragmentation) and natural (e.g., fires, recurrent droughts, and floods). Socio-economic data could inform the identification and characterization of natural and anthropogenic disturbances at discrete points in time, while paleoecology can inform the characterization of those disturbances over time (e.g., Davies et al., 2018). For example, fire is one of the most common disturbance factors reconstructed in paleoecology (Vegas-Vilarrubia et al., 2011). However, a complete characterization of fires requires understanding the underlying causes, which can be explained by merging social and ecological information. In this case, the underlying causes of fires in the Colombian Amazon during the last decades are related to several socioeconomic conflicts including land tenure, land allocation and armed conflict (Armenteras et al., 2019).
Step 3. Resilience decomposition and functionality. The three capacities of resilience in the BN become bridging nodes between social and ecological nodes and the system’s functionality nodes (Dee et al., 2017). In this step, absorption, adaptation, and transformation represent the three capacities of resilience and help to quantify the system’s functionality. Considering our example of the SES network (Figure 4), drivers associated with absorption may be the physical structure of the ecosystem or information on land use conversion. Adaptation as capacity may involve parameters such as institutional and governance actions referred to preventive measures that reduce the risk of collapse and enable SES to continue functioning during and after the disturbance. Transformation may consider social structures such as communities or corporations and national and local conflicts that may have pushed the SES to a new state after disturbance. Resilience capacities and functionality can be measured independently through expert knowledge (Sensier et al., 2016) or by using predictive models where functionality is the dependent variable (Youn et al., 2011). Alternatively, all three resilience capacities can be quantified together by Composite Indicators (Burgass et al., 2017), which offer a means of aggregating multiple indicators to track and communicate complex systems.
Step 4. Develop conditional probability tables (CPTs). Conditional probability of discrete variables is usually represented by a conditional probability table in which local probability is listed when a child node adopts each feasible value for each combination of values of its parent node (Jensen, 1996). The joint probability distribution of variables can be determined by local conditional probability tables that include unknown parameters to be estimated based on expert knowledge and automated learning algorithms (Jensen and Nielsen, 2007). As for the parent nodes of absorption, adaptation, and transformation, consisting of the different combinations of social and ecological nodes, the conditional probabilities are assigned based on the expert judgment and the learning algorithm for each combination of states of the parent nodes.
Step 5. A resilience assessment over time (modeling). The resilience levels are modeled through DBNs by calculating joint probabilities of the nodes and linkages, obtained in Steps 1, 2, and 3. The result of each BN is a performance point. The collection of the performance points results in a function that parameterizes system functionality and its changes, starting from a stable state or performance point and ending with the possible pathways in which the SES may respond to disturbances (Figure 3). The resulting time series is used to interpret changes in functionality over time and be directly linked to SES resilience. For example, monitoring how forests and their inhabitants recover from a series of fires becomes an ideal resilience metric (e.g., Davies et al., 2018; Ibáñez et al., 2019). The flexibility of the approach allows measuring not just the impact of disturbances on SES, but also identifying and characterizing the main social and ecological processes that make the system resilient.
Step 6. Model validation and application. The resilience evaluation can be directly assessed by verifying the accuracy of the DBN Model. Here we recommend a validation model based on interdisciplinary expertise and stakeholder evaluations. The experimental validation for DBN-based resilience includes a sensitivity analysis, which examines how small probability changes affect other parameters over time (Kleijnen, 1995). The results of a sensitivity analysis can be used to validate the model, but also to warn of unrealistic model behavior, point out important assumptions and help to formulate model structure. A comprehensive framework to validate expert-based models has been developed (Pitchforth and Mengersen, 2013) and accounts for the difficulty of validating expert decisions and that is applicable to our DBN models. The framework includes seven types of validity based on psychometrics: nomological, face, content, concurrent, convergent, discriminant, and predictive validity. For each validity aspect, questions ranging from the network structure to their implementation are suggested to test the validity of the DBN. For example, a question relevant to a SES resilience assessment and within the content validity is: Do the states of the nodes reflect all the known possibilities from expert knowledge and domain literature? Once a validation and a sensitivity analysis is conducted, participatory activities with stakeholders can further evaluate how the model is reflecting SES and changes in its functionality over time.
A study case for Monquentiva, Colombian Andes
We apply the DBN approach to model and quantify resilience of the Monquentiva socio-ecological system located in a biodiversity hotspot in the high Andes of Colombia. The village of Monquentiva is part of the municipality of Guatavita, northeast of the city of Bogotá (Figure 6). The Monquentiva community has high social cohesion and a considerable level of organization that aims to sustainable use and manage natural resources (Amador-Jiménez, 2020). The Monquentiva community runs a cheese and dairy cooperative since 1990 and rates of deforestation have decreased in the area even when deforestation has intensified in Colombia (Amador-Jiménez and Millner, 2021). Today the relationship between the social and ecological subsystems at Monquentiva is therefore thought to be stable and harmonious, and if so, the Monquentiva SES could be an example of a resilient socio-ecological system in the 2nd most diverse country on earth (Hernández, 2016). However, a careful assessment of SES resilience in Monquentiva is yet to be done. Here we model long-term functionality of the Monquentiva SES and apply the DBN network approach to test how the SES resilience has varied over time.
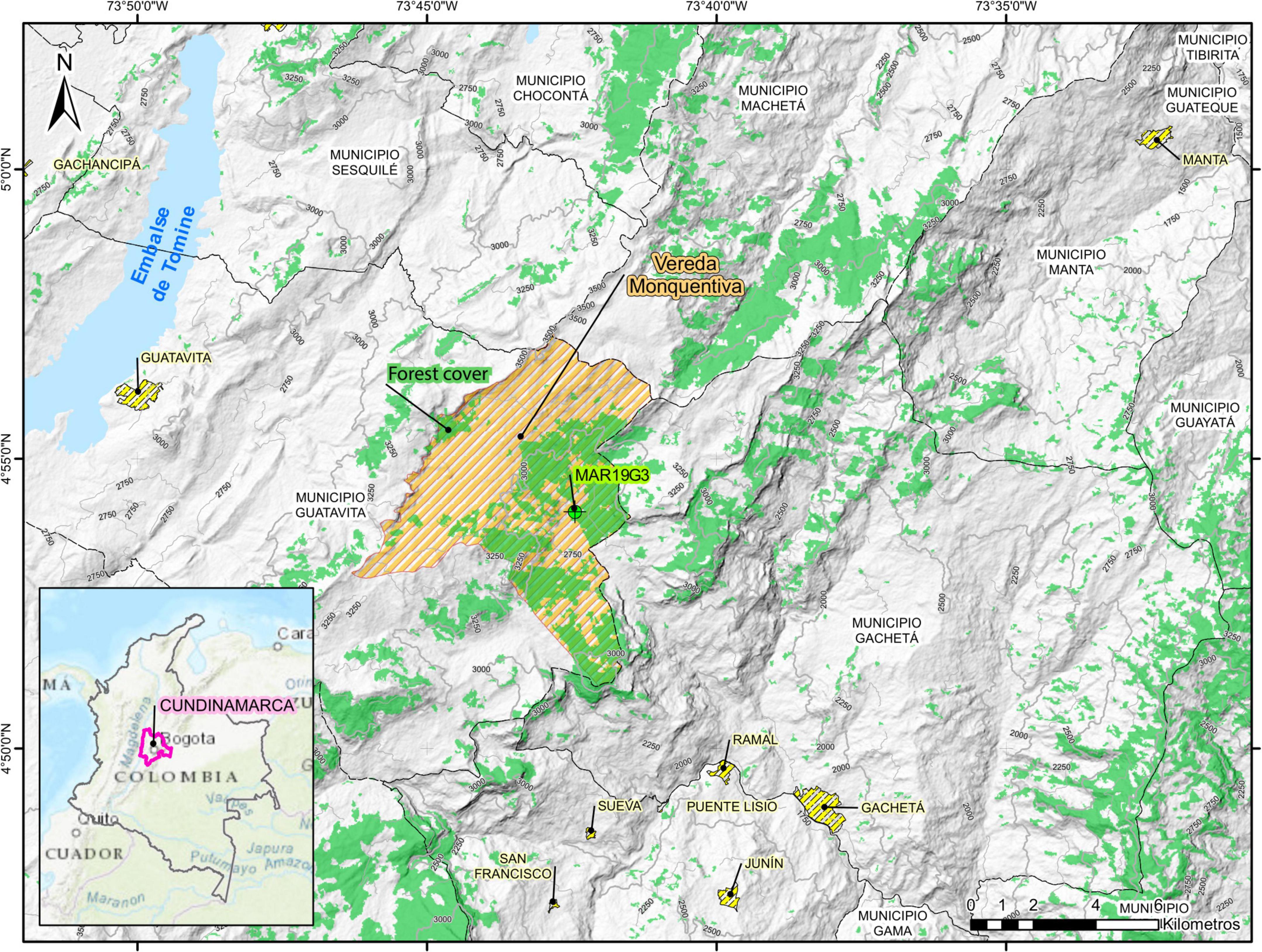
Figure 6. Map showing the location of Monquentiva in the high Andes of Colombia. The green dot indicates the location of the coring site for MAR19-G3. Orange hatching shows the Vereda (Village) of Monquentiva and green patches show the current distribution of High Andean Forest.
Materials and methods
We selected socio-economic and ecological nodes through discussions among experts from the social and ecological sciences and stakeholders. Scientific experts had worked in Monquentiva for over 3 years and included anthropologists, archeologists, historians, cultural geographers, ecologists, and paleoecologists. The selection of stakeholders, mainly policymakers and officials, was relatively simple since the experts in the area are a limited group (n = 15), including forest engineers, conservation professionals, environmental officials, and researchers in ecology and environmental anthropology. In this research, we approached 9 of the 15 stakeholders identified for the area, among them men and women. We organized interviews, meetings, and focus groups: with a (1) official from the Office of the Environment of the Guatavita Mayor’s Office, two (2) CAR (Corporación Autónoma Regional [CAR], 2019) officials in charge of the jurisdiction of the Monquentiva, two (2) researchers from Conservation International (international environmental NGO that operates in Monquentiva), two (2) environmental engineers and ecologists from the District University of Colombia, a (1) Colombian official from IDEAM (Institute of Hydrology, Meteorology, and Environmental Studies), an (1) official from the Chingaza National Park in the area near Monquentiva. The participation of the Monquentiva community (n = 30) was given by generational groups (grandparents, adults, and young people) and by groups of practices and professions among the peasants: dairy farming, a forest plantation, environmental conservation, farm caretakers, and leaders in a peasant association. We held eight meetings and two workshops with local communities, stakeholders, and experts, and the interdisciplinary discussions occurred throughout the data collection phase. The research team was responsible for guiding the experts through the tasks, encouraging discussion, and presenting results and analysis back to the experts. In addition, information from these discussions and paleoenvironmental data gathered through analysis of sediment sequences was used to structure the Monquentiva DBN. The socio-economic variables used in the DBN were population density, social organization, connectivity, and economic autonomy (Supplementary Table 1). The ecological variables included precipitation, forest cover, diversity, and fire activity (Supplementary Figure 1). The long-term behavior of ecological variables was derived from pollen and charcoal records from the sediment core labeled MAR19-GIII and meteorological data, while the socio-economic information was gathered through ethnographic work and oral history at Monquentiva (Amador-Jiménez, 2020). Ecological variables were discretized (Jensen, 1996) at equal intervals, converting continuous indicators into ordinal categories. For example, forest cover was converted into high, moderate, and low. Social variables were converted into zero, deficient, low, moderate, high, and very high by ranking methods (Supplementary Table 1).
The mathematical model was designed from a database of 12 variables and 96 observations per time window (Supplementary Table 2). The choices for the prior model and periods were determined by the time-scale of interest and social and ecological knowledge of the territory. For Monquentiva, we chose the period 1920–1960 as our prior model based on the availability of historical records for the socio-economic variables. We chose two subsequent periods 1960–1980 and 1980–2022 because they represented contrasting socio-environmental periods for this specific SES. Each variable was plotted as a node in a prior Bayesian network corresponding to the period 1920–1960 (time = t–1) and links were established with the three resilience capacities: absorption, adaptation, and transformation—probabilities quantified and assigned based on expert discussions (Figure 7A, left). A second BN for 1960–1980 (time = t) plotted the relationships between ecological and socio-economic nodes, the three resilience capacities, as well as the legacy of node changes from the prior BN at t–1. For fire activity for instance, we drew links between the node fires and transformation at t–1, and a link between fires at t–1 and fires at t (Figure 7). A third BN was plotted for the period 1980–2022 (time = t + 1). The next stage involves the compositional elements of the BN, which are typically in the form of conditional probability tables (CPT). The conditional probabilities were determined using Expectation-Maximization (EM) learning (Jensen and Nielsen, 2007). Thus, we used Equation 1 to calculate the probability of each resilience capacity given the interactions between socio-economic and ecological variables or nodes, and Equation 2 to model functionality over time incorporating the three resilience capacities. The model did not implement a linear regression so there were no assumptions made on the errors. However, the best score in iteration 0 from the DBN was –573.663 and EM Log Likelihood was –147.162.
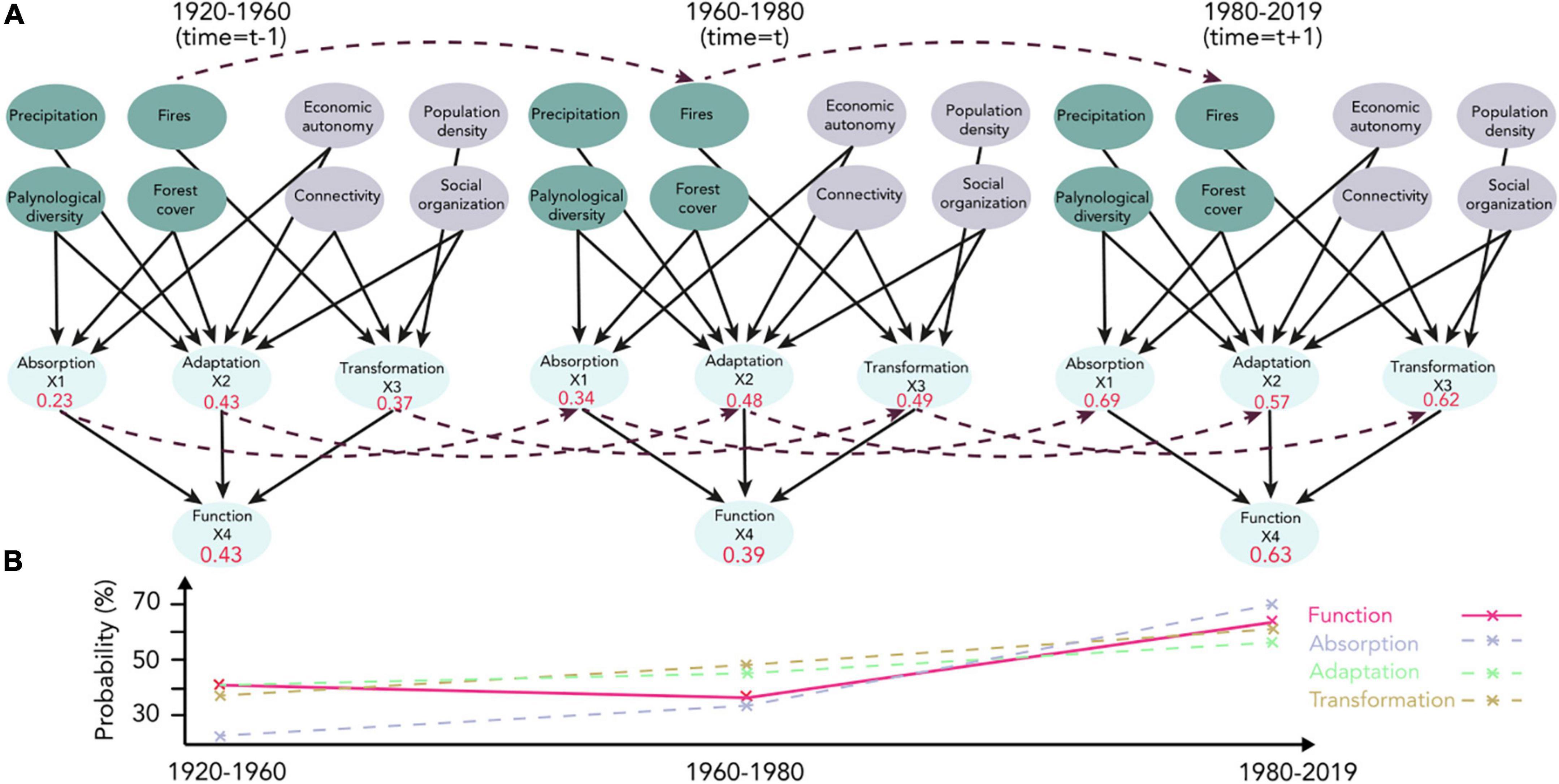
Figure 7. (A) The topology of the Dynamic Bayesian network for the Monquentiva SES and the conditional probabilities for three resilience capacities over three time slices 1920–1960 (Left), 1960–1980 (Center) and 1980–2021 (Right). (B) Historical changes in probability of resilience capacities and functionality in Monquentiva.
A sensitivity analysis was used to measure the degree to which the modeling variables influence the output of the model (Supplementary Figure 2). In this case, the focus is on studying the parameters which influence the target variable functionality. The sensitivity analysis applies the algorithm proposed by Kjærulff and van der Gaag (2000), to calculate the complete posterior probability distribution of selected nodes over all numerical parameters of the Bayesian network. The sensitivity analysis allowed the identification of important variables within the network and helped to verify the behavior (i.e., the relationship between nodes) of the developed model. In the modeling process, we used the GeNIe environment (Druzdzel, 1999) developed by the Decision Systems Laboratory of the University of Pittsburgh1 to build the network structure, calculate conditional probabilities tables, and validate and verify the model (Supplementary File 1).
Results and discussion
We observed that system functionality increased in the Monquentiva SES from 1920 to 2022 (Figure 7B). The functionality probability reached 0.63 in the most recent timeslice indicating the highest probability of maintaining functionality and resilience in the Monquentiva SES over the last 40 years. We also observed increases in the probabilities for absorption, adaptation and transformation over time, which in turned contributed to the accumulation of resilience probabilities. The pollen-reconstructed forest cover and diversity increased over the last 40 years, as well as social organization which together have likely led to a more functional and resilient system. The sensitivity analysis showed that the number of sensitive parameters increases toward 2022 (Supplementary Figure 2). Between 1920 and 1960 (time = t–1), a low probability of functionality is associated with less absorption and low levels of forest cover, diversity, economic autonomy, and social organization. Between 1960 and 1980 (time = t), the low functionality probability in the Monquentiva SES is related to poor absorption and low levels of forest cover, diversity, and social organization. Contrastingly, between 1980 and 2022 (time = t + 1) the increase in functionality probability relates to increased absorption, adaptation, and transformation in the SES, and resulted from high levels of diversity, forest cover, economic autonomy, and moderate levels of connectivity and social organization (Supplementary Figure 2).
Approaches for understanding environmental resilience need an integration of social and biophysical sciences (Morehouse et al., 2008). But this integration represents a challenge in modern research, even more incorporating ancestral knowledge and daily practices in the restoration and assessment of ecological resilience (Reyes-García et al., 2019). Consequently, successful knowledge integration lies in intertwining this interdisciplinarity to understand the past and in implementation in the present, which usually does not happen. Our approach and application in Monquentiva are an effort in that direction, emerging from a commitment to interdisciplinarity and allowing a methodology that incorporates local knowledge.
SES resilience assessments based on DBN can reveal strategic opportunities and arguments to help guide governance transformation and management because it is an adaptive, quantitative, interdisciplinary and participatory approach. The DBN can adapt as new knowledge about the system becomes available. As such management recommendations can also evolve rather than stalling on past or static assessments. DBN naturally focuses on the relationship between actions, knowledge, uncertainty, and causality and can be supplemented with variables encoding management decisions that in turn affect social and ecological nodes (Jensen, 1996). The consequences of various management decisions can be studied not only from the perspective of expected outcomes, but also concerning the risks of highly undesirable outcomes (Kjærulff and van der Gaag, 2000). The DBN is also quantitative and can help managers understand and measure SES resilience while integrating qualitative social resilience research. Because the DBN resilience assessment focuses on multiple human and non-human factors and is based on interdisciplinary and participatory dialogues, it can consequently guide management actions that preserve desired functional socio-ecological networks and that explicitly recognize the priorities and needs of human actors in the SES (Salliou et al., 2017). For Monquentiva, we found that SES functionality and resilience were maximum with high levels of economic autonomy and moderate levels of social organization. Several studies have revealed the contribution made by social organizations and agricultural cooperatives to sustainability (Galdeano-Gómez et al., 2015; Giagnocavo et al., 2018). This suggests that current management of Monquentiva by the local community is optimal and should be sustained. Future work could use the DBN to assess whether maintaining current management practices could continue to enhance the SES functionality and resilience.
Another area where DBN resilience assessments can inform management is the long-term and dynamic nature of the DBN. If the management goal for Monquentiva is to preserve the ecosystems embedded by the community, essential functions linked to ecosystem services should be maintained. The long-term knowledge embedded in the DBN could provide information on stable ecosystem states and how far the SES may have drifted from historical states or baselines in response to management (Willis and MacDonald, 2011). This long-term perspective can help identify conditions under which ecosystem function is maintained or not, and therefore can inform managers about the level of intervention needed (Willis and Birks, 2006). In the determination of baselines and initial states, paleoecology as a discipline has to offer a reference point, for example, to restore a habitat (Froyd and Willis, 2008). The initial states of the DBN could inform ecosystem restoration for Monquentiva after incorporating multi-millennial ecological information that represents the system before human activities began in the region (Espinoza et al., 2022). In South America, no SES management initiatives have been reported where the long-term ecological baselines are considered. Differently, in the United States and Europe long-term baselines are pillars of conservation ecology and have been widely used in ecosystem restoration (Swetnam et al., 1999; Willis and Birks, 2006). Through the use of a DBN, management and restoration in Monquentiva could be oriented to restoration to baseline resilience states.
The correlation between levels of quantified SES resilience and political aspects of the SES (Béné et al., 2016) could be relevant for policymakers. For instance, questions that can be explored through the DBN are: Do strong social cohesion and good governance explain high levels of SES resilience? For instance, we were able to show in our Monquentiva case study that social organization was a strong element enhancing resilience in the SES. Alternatively, does social disintegration or absence of governability affect the SES ability to maintain resilience? These questions are fundamental to the resilience-building narrative and relevant to NGOs and development agencies that aim to promote or implement sustainable management strategies. A DBN resilience assessment can help prevent functionality losses in SES, highlight new challenges linked to global environmental change, and help to simulate the effect of policy changes or management strategies on the SES resilience (Schulter et al., 2014; Salliou et al., 2017).
The application of DBN resilience assessment is, however, not challenge free. Some practical limitations could include the absence of funding that allows interdisciplinary work. In the case of Monquentiva, we had the rare opportunity to have both the socio-cultural and long-term ecology components working alongside from the beginning of the project. More often, the socio-cultural research is added as a supplement at the end of the ecological investigation. It is also common to see purely socio-cultural projects that stand alone without the contribution of ecological sciences. In this exercise, the interdisciplinary funding allowed co-production of knowledge on the Monquentiva SES and enriching discussions that resulted in the present analysis and other joint initiatives. This highlights the importance of resources for truly interdisciplinary projects where SES are concerned. Another challenge of the DBN for SES resilience assessment is that its structure is assumed to be stationary and constant over time. In other words, the DBN does not allow for transformation of relationships between components of the SES between time steps. This could be circumvented by combining the DBN approach with Hidden Markov Model (HMM). This combination extends each hidden node of the traditional HMM into a hidden DBN and develops the transition between nodes to describe the transition between network structures (Zhu and Wang, 2015).
Conclusion
Resilience theory has rapidly developed across multiple scientific spheres. The development and application of the concept to environmental change problems has seen little integration of social and ecological sciences, despite the significant role that people can play in sustaining or depleting ecosystem resilience. For this work, we chose to adopt the definition of socio-ecological resilience that considers humans embedded in nature and broken down into three main capacities: absorption, adaptation, and transformation. Also, we identify the importance of integrating the social sciences to improve the conceptualization and investigation of ecological resilience. Interdisciplinary approaches are more likely to generate resilience assessments that recognize the power relations in a SES. In this sense, we should not only think about the resilience of what and for what, also, for whom.
Our approach to quantify resilience is based on networks and interdisciplinary frameworks and considers elements from past studies on resilience conceptualization and quantification (Gunderson, 2000; Cote and Nightingale, 2012; Angeler and Allen, 2016). The innovation is the recognition that SES resilience should be assessed through a probabilistic rather than deterministic approach. A DBN serves this purpose by integrating social and ecological factors and focusing on changes in functionality over time. Also, the underlying principle of our framework is that a more resilient system is more capable of retaining ecological and social functionality after disturbance. Based on this principle, SES resilience can be measured and monitored simultaneously at different levels, different points in time, and in different socio-environmental contexts.
We explore the potential of integrated methods as DBN modeling to quantify the resilience of SES. Although DBN models have not been used in SES resilience assessments, we have shown that they can provide information on resilience structure and function. The model has a rigorous probabilistic foundation which provides substantial benefits to resilience assessment. In particular, the development of the model structure and validation serves as a platform of interdisciplinary dialogue where experts from social and ecological sciences can discuss their respective understanding of the mechanisms and processes leading to resilience of the SES. DBN also offer a flexible framework to address many questions in ecosystem monitoring and management. We anticipate that networks-based approaches will become essential for handling multidimensional observations and indispensable for assessing the dynamics of SES resilience. Further research into these techniques, including for instance long-term ecological records combined with historical information and socio-cultural contexts are likely to extend the scope of DBNs applications for SES resilience assessments.
Our assessment of the Monquentiva SES resilience showed that functionality can be quantitatively assessed over time through interdisciplinary work and the application of DBN. Studies that aim to assess SES in longer timescales would require the development of an increased number of time slices and BN, however, changes in SES resilience can be identified even in a simplified network like that developed for Monquentiva. The DBN-based framework could be further extended to root-cause nodes to identify other fundamental causes of resilience building or losses in the SES. For Monquentiva, our resilience assessment suggests that the enhanced social organization, connectivity and increased economic autonomy may have resulted in increased biodiversity and forest cover which in turn has promoted SES resilience.
Author contributions
FF-G and DU conceived the research and approach and conducted the dynamic Bayesian networks analyses. DU and NM acquired the funding. FF-G, MA-J, and CD collected and curated the data for the study case. MA-J and NM contributed to the resilience conceptualization and social aspects of the modeling process. All authors contributed to the writing of the manuscript and approved it for publication.
Funding
This work was conducted within the research project “BioResilience: Biodiversity resilience and ecosystem services in post-conflict socio-ecological systems in Colombia”, funded by the NERC-AHRC, United Kingdom (grant number NE/R017980/1).
Acknowledgments
We thank the community of Monquentiva for welcoming us and supporting the research, and Alcaldía de Guatavita and Corporacion Autonoma Regional de Cundinamarca CAR for facilitating research activities. We also thank the BioResilience team and the laboratory of tropical palaeoecology of the University of Exeter for internal discussions on interdisciplinarity and socio-ecological resilience. We are particularly grateful to MC and three reviewers whose comments and suggestions improved the final version of this manuscript.
Conflict of interest
The authors declare that the research was conducted in the absence of any commercial or financial relationships that could be construed as a potential conflict of interest.
Publisher’s note
All claims expressed in this article are solely those of the authors and do not necessarily represent those of their affiliated organizations, or those of the publisher, the editors and the reviewers. Any product that may be evaluated in this article, or claim that may be made by its manufacturer, is not guaranteed or endorsed by the publisher.
Supplementary material
The Supplementary Material for this article can be found online at: https://www.frontiersin.org/articles/10.3389/ffgc.2022.889274/full#supplementary-material
Footnotes
References
Aguilera, P. A., Fernández, A., Fernández, R., Rumí, R., and Salmerón, A. (2011). Bayesian networks in environmental modelling. Environ. Model. Softw. 26, 1376–1388. doi: 10.1016/j.envsoft.2011.06.004
Allen, C. R., Angeler, D. G., Cumming, G. S., Folke, C., Twidwell, D., and Uden, D. R. (2016). Quantifying spatial resilience. J. Appl. Ecol. 53, 625–635. doi: 10.1111/1365-2664.12634
Allen, C. R., Angeler, D. G., Garmestani, A. S., Gunderson, L. H., and Holling, C. S. (2014). Panarchy: Theory and application. Ecosystems 17, 578–589. doi: 10.1007/s10021-013-9744-2
Amador-Jimé,nez, M. (2020). Commoning to face COVID19, the experience of the Monquentiva Páramo Community. Exeter: Blog BioResilience.
Amador-Jiménez, M., and Millner, N. (2021). Militarisation under COVID-19: Understanding the differential impact of lockdown on the forests of Colombia. Front. Hum. Dyn. 3:769365. doi: 10.3389/fhumd.2021.769365
Angeler, D. G., and Allen, C. R. (2016). Quantifying resilience. J. Appl. Ecol. 53, 617–624. doi: 10.1111/1365-2664.12649
Angeon, V., and Lardon, S. (2008). Participation and governance in territorial development projects: The’territory game’as a local project leadership system. Int. J. Sustain. Dev. 11, 262–281. doi: 10.1504/IJSD.2008.026505
Armenteras, D., Schneider, L., and Dávalos, L. M. (2019). Fires in protected areas reveal unforeseen costs of Colombian peace. Nat. Ecol. Evol. 3, 20–23. doi: 10.1038/s41559-018-0727-8
Béné, C., Headey, D., Haddad, L., and von Grebmer, K. (2016). Is resilience a useful concept in the context of food security and nutrition programmes? Some conceptual and practical considerations. Food Secur. 8, 123–138. doi: 10.1007/s12571-015-0526-x
Berkes, F., Colding, J., and Folke, C. (2000). Rediscovery of traditional ecological knowledge as adaptive management. Ecol. Appl. 10, 1251–1262. doi: 10.2307/2641280
Berkes, F., Colding, J., and Folke, C. (2008). Navigating Social-Ecological Systems: Building Resilience for Complexity and Change. Cambridge: Cambridge University Press.
Burgass, M. J., Halpern, B. S., Nicholson, E., and Milner-Gulland, E. J. (2017). Navigating uncertainty in environmental composite indicators. Ecol. Indicat. 75, 268–278. doi: 10.1016/j.ecolind.2016.12.034
Butzer, K. W. (2005). Environmental history in the mediterranean world: Cross-disciplinary investigation of cause-and-effect for degradation and soil erosion. J. Archaeol. Sci. 32, 1773–1800. doi: 10.1016/j.jas.2005.06.001
Callon, M. (1984). Some elements of a sociology of translation: Domestication of the scallops and the fishermen of st brieuc bay. Sociol. Rev. 32, 196–233. doi: 10.1111/j.1467-954X.1984.tb00113.x
Carpenter, S., Walker, B., Anderies, J. M., and Abel, N. (2001). From metaphor to measurement: Resilience of what to what? Ecosystems 4, 765–781. doi: 10.1007/s10021-001-0045-9
Corporación Autónoma regional de Cundinamarca [CAR] (2019). Histórico de Series Hidrometeorológicas. Bogotá, CO: Corporación Autónoma regional de Cundinamarca.
Cote, M., and Nightingale, A. J. (2012). Resilience thinking meets social theory: Situating social change in socio-ecological systems (SES) research. Prog. Hum. Geogr. 36, 475–489. doi: 10.1177/0309132511425708
Crawford, T., Messina, J., Manson, S., and O’Sullivan, D. (2005). Complexity science, complex systems, and land-use research. Environ. Plann. B 32, 792–798. doi: 10.1068/b3206ed
Cushman, S. A., and McGarigal, K. (2019). Metrics and models for quantifying ecological resilience at landscape scales. Front. Ecol. Evol. 7:440. doi: 10.3389/fevo.2019.00440
Davidson, R. A. (2015). Integrating disciplinary contributions to achieve community resilience to natural disasters. Civil Eng. Environ. Syst. 32, 55–67. doi: 10.1080/10286608.2015.1011627
Davies, A. L., Streeter, R., Lawson, I. T., Roucoux, K. H., and Hiles, W. (2018). The application of resilience concepts in palaeoecology. Holocene 28, 1523–1534. doi: 10.1177/0959683618777077
Davoudi, S., Shaw, K., Haider, L., Quinlan, A., Peterson, G., Wilkinson, C., et al. (2012). Resilience: A bridging concept or a dead end?“Reframing” resilience: Challenges for planning theory and practice interacting traps: Resilience assessment of a pasture management system in northern afghanistan urban resilience: What does it mean in planning practice? Resilience as a useful concept for climate change adaptation? the politics of resilience for planning: A cautionary note. Plann. Theory Pract. 13, 299–333. doi: 10.1080/14649357.2012.677124
Dearing, J., Acma, B., Bub, S., Chambers, F., Chen, X., Cooper, J., et al. (2015). Social-ecological systems in the Anthropocene: The need for integrating social and biophysical records at regional scales. Anthropocene Rev. 2, 220–246. doi: 10.1177/2053019615579128
Dearing, J. A. (2008). Landscape change and resilience theory: A palaeoenvironmental assessment from Yunnan, SW China. Holocene 18, 117–127. doi: 10.1177/0959683607085601
Dee, L. E., Allesina, S., Bonn, A., Eklöf, A., Gaines, S. D., Hines, J., et al. (2017). Operationalizing network theory for ecosystem service assessments. Trends Ecol. Evol. 32, 118–130. doi: 10.1016/j.tree.2016.10.011
Druzdzel, M. (1999). “SMILE: Structural Modeling, Inference, and Learning Engine and GeNIE: A Development Environment for Graphical Decision-Theoretic Models,” in Proceedings of the Sixteenth National Conference on Artificial Intelligence and Eleventh Conference on Innovative Applications of Artificial Intelligence, (Florida).
Eason, T., Garmestani, A. S., Stow, C. A., Rojo, C., Alvarez-Cobelas, M., and Cabezas, H. (2016). Managing for resilience: An information theory-based approach to assessing ecosystems. J. Appl. Ecol. 53, 656–665. doi: 10.1111/1365-2664.12597
Elmqvist, T., Andersson, E., Frantzeskaki, N., McPhearson, T., Olsson, P., Gaffney, O., et al. (2019). Sustainability and resilience for transformation in the urban century. Nat. Sustain. 2, 267–273. doi: 10.1038/s41893-019-0250-1
Espinoza, I. G., Franco-Gaviria, F., Castañeda, I., Robinson, C., Room, A., Berrío, J. C., et al. (2022). Holocene fires and ecological novelty in the high colombian cordillera oriental. Front. Ecol. Evol. 10:895152. doi: 10.3389/fevo.2022.895152
Folke, C. (2006). Resilience: The emergence of a perspective for social–ecological systems analyses. Glob. Environ. Change 16, 253–267. doi: 10.1016/j.gloenvcha.2006.04.002
Folke, C., Carpenter, S., Walker, B., Scheffer, M., Elmqvist, T., Gunderson, L., et al. (2004). Regime shifts, resilience, and biodiversity in ecosystem management. Annu. Rev. Ecol. Evol. Syst. 35, 557–581. doi: 10.1146/annurev.ecolsys.35.021103.105711
Folke, C., Carpenter, S. R., Walker, B., Scheffer, M., Chapin, T., and Rockstrom, J. (2010). Resilience thinking: Integrating resilience, adaptability and transformability. Ecol. Soc. 15:20. doi: 10.5751/ES-03610-150420
Froyd, C. A., and Willis, K. J. (2008). Emerging issues in biodiversity & conservation management: The need for a palaeoecological perspective. Quat. Sci. Rev. 27, 1723–1732. doi: 10.1016/j.quascirev.2008.06.006
Galdeano-Gómez, E., Pérez-Mesa, J. C., and Godoy-Durán, Á (2015). The social dimension as a driver of sustainable development: The case of family farms in southeast Spain. Sustain. Sci. 11, 349–362. doi: 10.1007/s11625-015-0318-4
Giagnocavo, C., Galdeano-Gómez, E., and Pérez-Mesa, J. C. (2018). Cooperative longevity and sustainable development in a family farming system. Sustainability 10:2198. doi: 10.3390/su10072198
Gonzalez-Redin, J., Luque, S., Poggio, L., Smith, R., and Gimona, A. (2016). Spatial Bayesian belief networks as a planning decision tool for mapping ecosystem services trade-offs on forested landscapes. Environ. Res. 144, 15–26. doi: 10.1016/j.envres.2015.11.009
Grier, C., Alessa, L., and Kliskey, A. (2017). Looking to the past to shape the future: Addressing social-ecological change and adaptive trade-offs. Reg. Environ. Change 17, 1205–1215. doi: 10.1007/s10113-016-1096-y
Gunderson, L. H. (2000). Ecological resilience—in theory and application. Ann. Rev. Ecol. Syst. 31, 425–439. doi: 10.1146/annurev.ecolsys.31.1.425
Hastrup, K. (2013). Water and the configuration of social worlds: An anthropological perspective. J. Water Resour. Protect. 05, 59–66. doi: 10.4236/jwarp.2013.54A009
Henriksen, H. J., Rasmussen, P., Brandt, G., von Bülow, D., and Jensen, F. V. (2007). Public participation modelling using Bayesian networks in management of groundwater contamination. Environ. Model. Softw. 22, 1101–1113. doi: 10.1016/j.envsoft.2006.01.008
Hernández, A. M. (2016). “Conexión vital para el desarrollo sostenible del país,” in Biodiversidad 2015. Estado y tendencias de la biodiversidad continental de Colombia., eds M. F. Gómez, L. A. Moreno, G. I. Andrade, and C. y Rueda (Bogotá: Instituto Alexander von Humboldt).
Holling, C. S. (1973). Resilience and Stability of Ecological Systems. Ann. Rev. Ecol. System. 4, 1–23. doi: 10.1146/annurev.es.04.110173.000245
Holling, C. S. (2001). Understanding the complexity of economic, ecological, and social systems. Ecosystems 4, 390–405. doi: 10.1007/s10021-001-0101-5
Ibáñez, I., Acharya, K., Juno, E., Karounos, C., Lee, B. R., McCollum, C., et al. (2019). Forest resilience under global environmental change: Do we have the information we need? A systematic review. PLoS One 14:e0222207. doi: 10.1371/journal.pone.0222207
Jakeman, A. J., Letcher, R. A., and Norton, J. P. (2006). Ten iterative steps in development and evaluation of environmental models. Environ. Model. Softw. 21, 602–614. doi: 10.1016/j.envsoft.2006.01.004
Janssen, M. A., Bodin, Ö, Anderies, J. M., Elmqvist, T., Ernstson, H., McAllister, R. R. J., et al. (2006). Toward a network perspective of the study of resilience in social-ecological systems. Ecol. Soc. 11:15. doi: 10.5751/ES-01462-110115
Jensen, F. V., and Nielsen, T. D. (2007). Bayesian Networks and Decision Graphs. New York, NY: Springer. doi: 10.1007/978-0-387-68282-2
Johnson, L. R., Johnson, M. L., Aronson, M. F., Campbell, L. K., Carr, M. E., Clarke, M., et al. (2021). Conceptualizing social-ecological drivers of change in urban forest patches. Urban Ecosyst. 24, 633–648. doi: 10.1007/s11252-020-00977-5
Kerner, D. A., and Thomas, J. S. (2014). Resilience attributes of social-ecological systems: Framing metrics for management. Resources 3, 672–702. doi: 10.3390/resources3040672
Kjærulff, U., and van der Gaag, L. C. (2000). “Making Sensitivity Analysis Computationally Efficient,” in Proceedings of the 16th Conference on Uncertainty in Artificial Intelligence, (San Francisco, CA: Morgan Kaufmann).
Kleijnen, J. P. (1995). Verification and validation of simulation models. Eur. J. Operat. Res. 82, 145–162. doi: 10.1016/0377-2217(94)00016-6
Langebaek, C. H. (1987). Mercados, Poblamiento e Integración Étnica Entre Los Muiscas: Siglo XVI. Bogotá, CO: Banco de la República.
Latour, B. (2005). Reassembling the Social: An Introduction to Actor-network-theory. Oxford: Oxford University Press.
Linkov, I., and Kott, A. (2019). “Fundamental concepts of cyber resilience: Introduction and overview,” in Cyber Resilience of Systems and Networks, eds A. Kott and I. Linkov (Berlin: Springer), 1–25. doi: 10.1007/978-3-319-77492-3_1
Linstadter, A., Kuhn, A., Naumann, C., Rasch, S., Sandhage-Hofmann, A., Amelung, W., et al. (2016). Assessing the resilience of a real-world social-ecological system: Lessons from a multidisciplinary evaluation of a South African pastoral system. Ecol. Soc. 21:35. doi: 10.5751/ES-08737-210335
Marcot, B. G., and Penman, T. D. (2019). Advances in Bayesian network modelling: Integration of modelling technologies. Environ. Model. Softw. 111, 386–393. doi: 10.1016/j.envsoft.2018.09.016
Moore, C., Grewar, J., and Cumming, G. S. (2016). Quantifying network resilience: Comparison before and after a major perturbation shows strengths and limitations of network metrics. J. Appl. Ecol. 53, 636–645. doi: 10.1111/1365-2664.12486
Morehouse, B. J., Ferguson, D. B., Owen, G., Browning-Aiken, A., Wong-Gonzalez, P., Pineda, N., et al. (2008). Science and socio-ecological resilience: Examples from the arizona-sonora border. Environ. Sci. Policy 11, 272–284. doi: 10.1016/j.envsci.2007.07.007
Nash, K. L., Graham, N. A. J., Jennings, S., Wilson, S. K., and Bellwood, D. R. (2016). Herbivore cross-scale redundancy supports response diversity and promotes coral reef resilience. J. Appl. Ecol. 53, 646–655. doi: 10.1111/1365-2664.12430
Newman, M. E. J. (2004). Detecting community structure in networks. Eur. Phys. J. B 38, 321–330. doi: 10.1140/epjb/e2004-00124-y
Ostrom, E. (2009). A General framework for analyzing sustainability of social-ecological systems. Science 325:419. doi: 10.1126/science.1172133
Pitchforth, J., and Mengersen, K. (2013). A proposed validation framework for expert elicited bayesian networks. Expert Syst. Appl. 40, 162–167. doi: 10.1016/j.eswa.2012.07.026
Quinlan, A. E., Berbés-Blázquez, M., Haider, L. J., and Peterson, G. D. (2016). Measuring and assessing resilience: Broadening understanding through multiple disciplinary perspectives. J. Appl. Ecol. 53, 677–687. doi: 10.1111/1365-2664.12550
Redman, C., Grove, J., and Kuby, L. (2003). Integrating social science into the long-term ecological research (LTER) network: Social dimensions of ecological change and ecological dimensions of social change. Ecosystems 7, 161–171. doi: 10.1007/s10021-003-0215-z
Renschler, C. S., Frazier, A. E., Arendt, L. A., Cimellaro, G. P., Reinhorn, A. M., and Bruneau, M. (2010). A Framework for Defining and Measuring Resilience at the Community Scale: the Peoples Resilience Framework. Buffalo, NY: MCEER Buffalo.
Reyes-García, V., Fernaìndez, L. A. ì, McElwee, P., Molnaìr, Z., Öllerer, K., Wilson, S. J., et al. (2019). The contributions of indigenous peoples and local communities to ecological restoration: Indigenous peoples for ecological restoration. Restor. Ecol. 27, 3–8. doi: 10.1111/rec.12894
Ruckelshaus, M., McKenzie, E., Tallis, H., Guerry, A., Daily, G., Kareiva, P., et al. (2015). Notes from the field: Lessons learned from using ecosystem service approaches to inform real-world decisions. Ecol. Econ. 115, 11–21. doi: 10.1016/j.ecolecon.2013.07.009
Saja, A. M. A., Teo, M., Goonetilleke, A., and Ziyath, A. M. (2021). Assessing social resilience in disaster management. Int. J. Disaster Risk Reduct. 52:101957. doi: 10.1016/j.ijdrr.2020.101957
Salliou, N., Barnaud, C., Vialatte, A., and Monteil, C. (2017). A participatory bayesian belief network approach to explore ambiguity among stakeholders about socio-ecological systems. Environ. Model. Softw. 96, 199–209. doi: 10.1016/j.envsoft.2017.06.050
Sayles, J., García, M. M., Hamilton, M., Alexander, S., Baggio, J., Fischer, A. P., et al. (2019). Social-ecological network analysis for sustainability sciences: A systematic review and innovative research agenda for the future. Environ. Res. Lett. 14:093003. doi: 10.1088/1748-9326/ab2619
Schulter, M., Hinkel, J., Bots, P. W. G., and Arlinghaus, R. (2014). Application of the SES framework for model-based analysis of the dynamics of social-ecological systems. Ecol. Soc. 19:36. doi: 10.5751/ES-05782-190136
Sensier, M., Bristow, G., and Healy, A. (2016). Measuring regional economic resilience across Europe: operationalizing a complex concept. Spat. Econ. Anal. 11, 128–151. doi: 10.1080/17421772.2016.1129435
Swetnam, T. W., Allen, C. D., and Betancourt, J. L. (1999). Applied historical ecology: Using the past to manage for the future. Ecol. Appl. 9, 1189–1206. doi: 10.1890/1051-0761(1999)009[1189:AHEUTP]2.0.CO;2
Tucker, A., and Liu, X. (2004). A Bayesian network approach to explaining time series with changing structure. Intell. Data Anal. 8, 469–480. doi: 10.3233/IDA-2004-8504
Uusitalo, L. (2007). Advantages and challenges of bayesian networks in environmental modelling. Ecol. Model. 203, 312–318. doi: 10.1016/j.ecolmodel.2006.11.033
Vegas-Vilarrubia, T., Rull, V., Montoya, E., and Safont, E. (2011). Quaternary palaeoecology and nature conservation: A general review with examples from the neotropics. Quater. Sci. Rev. 30, 2361–2388. doi: 10.1016/j.quascirev.2011.05.006
Voinov, A., and Bousquet, F. (2010). Modelling with stakeholders. Environ. Model. Softw 25, 1268–1281. doi: 10.1016/j.envsoft.2010.03.007
Walker, B., Hollin, C. S., Carpenter, S. R., and Kinzig, A. (2004). Resilience, adaptability and transformability in social-ecological systems. Ecol. Soc. 9:5. doi: 10.5751/ES-00650-090205
White, P. S., and Jentsch, A. (2001). “The Search for Generality in Studies of Disturbance and Ecosystem Dynamics,” in Progress in Botany: Genetics Physiology Systematics Ecology, eds K. Esser, U. Lüttge, J. W. Kadereit, and W. Beyschlag (Berlin: Springer), 399–450.
Willis, K. J., and Birks, H. J. B. (2006). What is natural? The need for a long-term perspective in biodiversity conservation. Science 314, 1261–1265. doi: 10.1126/science.1122667
Willis, K. J., and MacDonald, G. M. (2011). Long-Term ecological records and their relevance to climate change predictions for a warmer world. Ann. Rev. Ecol. Evol. System. 42, 267–287. doi: 10.1146/annurev-ecolsys-102209-144704
Wilson, G. A., and Wilson, O. J. (2019). Assessing the resilience of human systems: A critical evaluation of universal and contextual resilience variables. Resilience 7, 126–148. doi: 10.1080/21693293.2018.1539205
Wu, J., and David, J. L. (2002). A spatially explicit hierarchical approach to modeling complex ecological systems: Theory and applications. Ecol. Model. 153, 7–26. doi: 10.1016/S0304-3800(01)00499-9
Wu, P. P.-Y., McMahon, K., Rasheed, M. A., Kendrick, G. A., York, P. H., Chartrand, K., et al. (2018). Managing seagrass resilience under cumulative dredging affecting light: Predicting risk using dynamic bayesian networks. J. Appl. Ecol. 55, 1339–1350. doi: 10.1111/1365-2664.13037
Youn, B. D., Hu, C., and Wang, P. (2011). Resilience-Driven system design of complex engineered systems. J. Mech. Design 133:101011. doi: 10.1115/1.4004981
Keywords: dynamic Bayesian networks, functionality, interdisciplinarity, participatory approach, resilience modeling, socio-ecological systems, disturbance
Citation: Franco-Gaviria F, Amador-Jiménez M, Millner N, Durden C and Urrego DH (2022) Quantifying resilience of socio-ecological systems through dynamic Bayesian networks. Front. For. Glob. Change 5:889274. doi: 10.3389/ffgc.2022.889274
Received: 04 March 2022; Accepted: 03 October 2022;
Published: 20 October 2022.
Edited by:
Marco Cervellini, University of Camerino, ItalyReviewed by:
Ana Stritih, Technical University of Munich, GermanyFederica Onori, European Commission Headquarters, Belgium
Matthew Grainger, Norwegian Institute for Nature Research (NINA), Norway
Copyright © 2022 Franco-Gaviria, Amador-Jiménez, Millner, Durden and Urrego. This is an open-access article distributed under the terms of the Creative Commons Attribution License (CC BY). The use, distribution or reproduction in other forums is permitted, provided the original author(s) and the copyright owner(s) are credited and that the original publication in this journal is cited, in accordance with accepted academic practice. No use, distribution or reproduction is permitted which does not comply with these terms.
*Correspondence: Felipe Franco-Gaviria, ai5mcmFuY28tZ2F2aXJpYUBleGV0ZXIuYWMudWs=