- 1Department of Forestry, Michigan State University, East Lansing, MI, United States
- 2Forest Carbon and Climate Program, Department of Forestry Michigan State University, East Lansing, MI, United States
- 3American Forests, Washington, DC, United States
- 4Natural Resources Canada, Canadian Forest Service, Victoria, BC, Canada
- 5Office of Sustainability and Climate, U.S. Forest Service, Washington, DC, United States
State and local governments are increasingly interested in understanding the role forests and harvested wood products play in regional carbon sinks and storage, their potential contributions to state-level greenhouse gas (GHG) reductions, and the interactions between GHG reduction goals and potential economic opportunities. We used empirically driven process-based forest carbon dynamics and harvested wood product models in a systems-based approach to project the carbon impacts of various forest management and wood utilization activities in Maryland and Pennsylvania from 2007 to 2100. To quantify state-wide forest carbon dynamics, we integrated forest inventory data, harvest and management activity data, and remotely-sensed metrics of land-use change and natural forest disturbances within a participatory modeling approach. We accounted for net GHG emissions across (1) forest ecosystems (2) harvested wood products, (3) substitution benefits from wood product utilization, and (4) leakage associated with reduced in-state harvesting activities. Based on state agency partner input, a total of 15 management scenarios were modeled for Maryland and 13 for Pennsylvania, along with two climate change impact scenarios and two bioenergy scenarios for each state. Our findings show that both strategic forest management and wood utilization can provide substantial climate change mitigation potential relative to business-as-usual practices, increasing the forest C sink by 29% in Maryland and 38% in Pennsylvania by 2030 without disrupting timber supplies. Key climate-smart forest management activities include maintaining and increasing forest extent, fostering forest resiliency and natural regeneration, encouraging sustainable harvest practices, balancing timber supply and wood utilization with tree growth, and preparing for future climate impacts. This study adds to a growing body of work that quantifies the relationships between forest growth, forest disturbance, and harvested wood product utilization, along with their collective influence on carbon stocks and fluxes, to identify pathways to enhance forest carbon sinks in support of state-level net-zero emission targets.
1. Introduction
Forests influence the earth’s climate system through complex and non-linear interactions by affecting the global carbon (C) cycle, hydrologic cycle, and energy balance (Bonan, 2008). To meet current climate goals, all sectors require greenhouse gas (GHG) emissions reductions and mitigation actions (IPCC, 2021); forests and the forest products sector provide the unique opportunity to not only help humans and society adapt to a changing climate but slow the rate of climate change by reducing or offsetting GHG emissions (Smith et al., 2014). The forestry sector and its associated forest products sector provide a cost-effective pathway to both store and sequester a substantial amount of carbon, thereby contributing to global net zero emissions goals (Griscom et al., 2017). Previous studies have estimated that forests in the United States offset approximately 14% of annual CO2 emissions and 11% for all GHG emissions (Skog, 2008; Domke et al., 2021) with significant potential for increased carbon storage and sequestration through climate-focused forest management (Fargione et al., 2018; Ontl et al., 2020; Kaarakka et al., 2021). Efforts to ensure forests in the US continue to be a net C sink relies on understanding the intersections of policy, forest and forest sector emissions, and forest management.
The United States has committed to a 50%–52% reduction in GHG emissions from 2005 levels by the year 2030 (UNFCCC, 2021). To meet this target, flexible and increasingly innovative forest management practices that support continued provisioning of essential ecosystem services and optimization of forests’ GHG mitigation potential may be valuable (Millar et al., 2007). In addition to federal actions, state and local governments have begun to enact policies that aim to curb GHG emissions (Fuhr et al., 2018). For example, the Greenhouse Gas Reduction Act (GGRA) in Maryland is an economy-wide plan that identifies reduction targets across various sectors or, in the case of forests, sequestration targets to cut annual net GHG emissions (MDE, 2021). Adopted in 2009 and amended in 2016, this legislation acknowledges the severe threat posed by climate change and outlines specific actions the forestry sector and its associated forest product sector can take to lower net GHG emissions. The GGRA recognizes the critical role forests play in mitigating climate change through carbon sequestration and storage, as well as their ability to help disadvantaged communities adapt to the negative effects of climate change, such as utilizing participatory mechanisms to protect biodiversity while addressing poverty and inequality (Menton et al., 2020). Likewise, Pennsylvania has adopted the Pennsylvania Climate Action Plan 2021 which outlines a pathway to an 80% reduction in GHG by 2050 from 2005 levels. The plan identifies 18 strategies, time frames for implementation, economic costs and benefits, and specific adaptation pathways (PDEP, 2021).
Efforts to monitor progress toward established goals rely on sound quantification of forest and forest sector emissions. Modeling complex ecosystem processes such as the C cycle at a landscape scale involves addressing interactions across spatial, temporal, and ecological scales (Geary et al., 2020). Primarily driven by land-based pools and fluxes, forest carbon flows between the atmospheric, terrestrial, and forest product carbon pools (Figure 1). To properly evaluate and account for climate change mitigation potential within forests and the forestry sector at large, a systems-based approach can help to analyze trade-offs and synergies (Nabuurs et al., 2007), as it enables understanding of interdependent systems and potential feedbacks, such as the forest ecosystem and forest products sector, concurrently (Evans et al., 2012) including trade-offs between the forest ecosystem, land-use change (LUC), harvested wood products, wood substitution benefits, and leakage associated with changes in harvest levels (Malmsheimer et al., 2008; Skog, 2008; D’Amato et al., 2011; Smith et al., 2014; Verkerk et al., 2020). Solely analyzing the impacts of specific management practices on carbon stocks within forests does not entirely account for total carbon storage as harvested wood can store carbon for decades as long-lived wood products (McKinley et al., 2011). To fully evaluate the mitigation potential of harvested wood products (HWPs), mill efficiencies, product half-lives, recycling rates, leakage, and substitution benefits should be accounted for (Malmsheimer et al., 2008; McKinley et al., 2011). Linkages to other sectors (e.g., energy and construction) at landscape or regional scales add additional insight into carbon stocks and fluxes by more reliably capturing, among other things, future product demand and fossil fuel emissions from competing sectors (Malmsheimer et al., 2008; Kurz et al., 2016). The management of multi-functional landscapes such as forests is further complicated when incorporating the financial or governance constraints of implementation (Seddon et al., 2020). Together, these environmental, economic, and political dynamics provide both opportunities and challenges for policy makers as they seek to address the impacts of forest management, forest dynamics, land-use change, and the forest products sector at varying scales (D’Amato et al., 2011; Seddon et al., 2020).
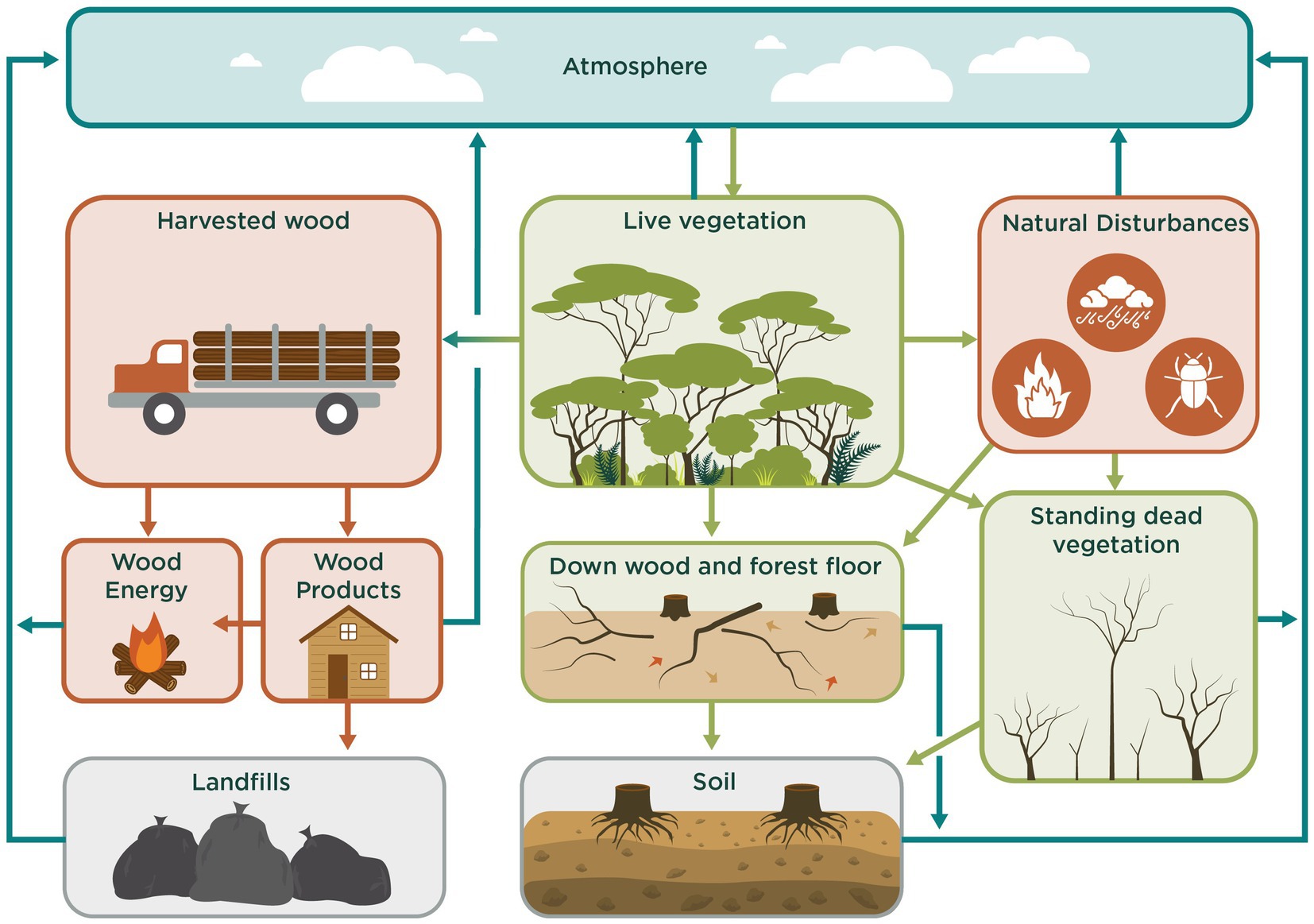
Figure 1. Systems-based framework for the forest carbon cycle with associated forest product sector (Adapted from: Heath et al., 2003).
Further complicating forest C balances is the interaction of both natural disturbances and human management activities such as harvesting or land-use change (Bellassen and Luyssaert, 2014). Interactions among harvesting levels, rate of forest recovery following disturbance, and age-dependent forest productivity are well-documented drivers of forest C sink-source strength as well as interannual C fluxes (Ryan et al., 1997; Gough et al., 2008; Peichl et al., 2010, 2022; Coursolle et al., 2012). Therefore, it is critical to understand the relationship between forest C dynamics across a heterogenous spatial mosaic of forest management activities (Vestin et al., 2020), forest recovery and successional patches (Curtis and Gough, 2018), and landscape variability (Peichl et al., 2022) to properly assess climate mitigation potential of forests. Doing so allows for furthered understanding of the possible future forest C sink-source strength, providing pivotal information to both forest managers and policy makers about the role management activities may have on forest age-class structures and therefore on forest C trajectories.
To successfully apply carbon science to meet legislative goals at broad scales, effective climate change mitigation strategies balance the maintenance or optimization of both carbon storage and sequestration while acknowledging trade-offs with ecological complexity and health (Bradford and D’Amato, 2012; Watson et al., 2018; Rogers et al., 2022). Climate-Smart Forestry (CSF) is a targeted, long-term strategy to enhance the climate benefits from forests and the forest products sector while providing sustainable resources for a growing human population (Nabuurs et al., 2018; Bowditch et al., 2020; Cooper and MacFarlane, 2023). CSF emphasizes, in part, the management of forests to continuously deliver a sustainable wood supply in both the short- and long-term without impeding the integrity and function of the forest (Bowditch et al., 2020) which can be achieved through optimizing both the age-structure and diameter distribution of forests at the landscape level (Bergeron et al., 2017) and other adaptive practices (Bowditch et al., 2020). Typically, when looking at the relationship between C storage and sequestration at landscape to stand scales, there is an inverse relationship between forest age and rates of C sequestration (Odum, 1969; Chapin et al., 2011; Stephenson et al., 2014; Curtis and Gough, 2018). Despite contrasting evidence regarding variations in forest C balances, or the difference between ecosystem C uptake and loss, forest age continually acts strongly as a predictor of forest growth regardless of initial stand characteristics such as soil fertility or hydrologic regimes (Besnard et al., 2018). Both empirical and mechanistic approaches show that following stand establishment, forest stands begin to act as a carbon sink within the first 10–20 years. However, stands do not begin to maximize mean annual increment (MAI), or the average growth in volume per year, until between 30 and 70 years in temperate eastern forests (Coomes et al., 2012; Coursolle et al., 2012; Peichl et al., 2022). However, MAI diminishes rapidly as forests continue to age toward later seral stages (>120 years) predominantly driven by increases in stand-level competition and mortality (Ryan et al., 1997; Binkley et al., 2002; Coomes et al., 2012; Chen and Luo, 2015; Yuan et al., 2019). While the strength of forest C sinks in older temperate forests (i.e., >120 years) remains inconclusive (Coursolle et al., 2012; Chen and Luo, 2015; Curtis and Gough, 2018; Yuan et al., 2019; Gundersen et al., 2021), older forests can store large amounts of carbon in both biomass and coarse woody debris, absent some level of major disturbance (Dixon et al., 1994; McGarvey et al., 2015). Additionally, older forest stands tend to maximize structural complexity, providing significant supporting and regulatory ecosystem services such as habitat creation and biodiversity (Binkley et al., 2002; Fahey et al., 2010; Ford and Keeton, 2017).
This study builds on previous work to understand how forest management and the forest product sector can contribute to achieving climate goals at the landscape scale within a participatory systems-based framework (Smyth et al., 2014; Pilli et al., 2016; Olguin et al., 2018; Dugan et al., 2018a, 2021). The specific objectives of this study are to identify climate-smart forestry practices for Maryland and Pennsylvania by applying forest ecosystem and HWP models to analyze and quantify forest carbon tradeoffs and mitigation potential among a variety of alternative management, climate, and bioenergy scenarios against a projected “business-as-usual” (BAU) simulation. We do not seek to determine how specific policies can be achieved, but instead seek to quantify the consequences of various scenarios for GHG emissions and removals. We expand upon previous research to understand the interactions between projected forest ecosystem C dynamics, HWPs, and substitution benefits, and leakage to inform policy and management decision-making regarding GHG emission reductions while developing a replicable participatory systems-based approach for future research within the US and elsewhere. Results will increase understanding of the GHG mitigation potential of forest ecosystems and the forest product sectors and can be used by state forestry practitioners and decision makers to prioritize activities and policies that best leverage forests as a solution to climate change.
2. Methodologies
2.1. Study area
Our study area encompasses all forestlands in Maryland and Pennsylvania. Maryland and Pennsylvania contain an estimated 0.99 and 6.72 million hectares of forestland that corresponds to 36.59% and 57.32% of total land area within each state, respectively (USDA Forest Service, 2019). Forestland (defined as land of at least 10% canopy cover by trees of any size and that will be naturally or artificially regenerated, Burrill et al., 2021) within both states is predominantly privately owned, with roughly one quarter managed by each state government and minor federal landholdings (Table 1; Figure 2) including a National Forest covering over 200,000 hectares in Pennsylvania. Hardwood forests dominate both states, especially the oak/forest type group in both states and maple/beech/birch forests in Pennsylvania (Table 2). However, Maryland also contains sizable areas of loblolly/shortleaf pine and oak/pine forests. Descriptions of each forest type group are listed in Appendix D of Burrill et al. (2021).
Maryland and Pennsylvania have large quantities of aging forests (i.e., reaching their commercial rotational age), with almost half of total forest area being over 80 years old (Figure 3). The current age distribution of forests in both states is a result of prior land use and management regimes throughout the early parts of the 20th century that prioritized the clearing of forested landscapes for agriculture and human settlements, forest regrowth of depleted agricultural lands, management intensification, legacy effects of diameter-limit-cuts, and the disruption to historic natural disturbance regimes (Millers et al., 1989; Otto, 1989). Presently, forests in both states ensure a steady timber supply in the near term. However, with over 63.3% and 72.1% of forestlands in Maryland and Pennsylvania, respectively, being over 60 years old and nearing their commercial rotation ages, future timber supplies are dependent upon continued successful regeneration or thinning in older stands. Together, Maryland and Pennsylvania provide a unique study area to explore the role of climate-smart forestry in quantifying the C mitigation potential of forests and HWPs.
2.2. Forest ecosystem data and modeling framework
We used the Carbon Budget Model of the Canadian Forest Sector (CBM-CFS3; Kurz et al., 2009; Kull et al., 2019), an operational scale carbon model that uses spatially referenced inventory data and empirically derived growth-yield curves to simulate forest carbon dynamics through time utilizing guidelines and carbon pools established by the Intergovernmental Panel on Climate Change (IPCC). The model incorporates both human activities and natural disturbances to simulate forest C dynamics on annual timesteps. Process-based equations simulate carbon dynamics between soil, dead organic matter, and forest processes such as litterfall, while disturbance matrices represent the impacts of specific disturbance events, through the transfer of carbon between pools, carbon transfer to the forest product sector, and carbon emissions to the atmosphere (Kurz et al., 2009). The CBM-CFS3 has had wide applications within the United States (Dugan et al., 2018b, 2021), Canada (Kurz et al., 2013), and internationally (Pilli et al., 2013, 2017, 2022; Olguin et al., 2018; FERS, 2021), with thorough ground truthing (Shaw et al., 2014) and characterization of model uncertainty (Metsaranta et al., 2017). It is also the core model of Canada’s National Forest Carbon Monitoring, Accounting and Reporting System (Kurz and Apps, 2006; Kurz et al., 2018; ECCC, 2023).
Primary data inputs to CBM-CFS3 included a detailed forest inventory, growth-yield relations to estimate forest productivity, and estimates of harvest yields and intensity, land-use change, and natural disturbances. Inventory data are categorized by a series of forest classifiers defining relevant characteristics such as spatially referenced boundaries, ownership, forest type, site productivity, or reserve status. Allometric equations are used to predict tree volume-to-biomass relationships (Boudewyn et al., 2007). For this study, forest inventory, growth-yield curves, and harvest data were estimated from the USDA Forest Service Forest Inventory and Analysis (FIA) program, which we accessed through the FIA DataMart (USDA Forest Service, 2019) using the rFIA package (Stanke et al., 2020) in the R programming environment (R Core Team, 2020). rFIA enables data exploration and user-defined spatio-temporal queries and estimation of the FIA database (FIADB). Methodologies derived from Bechtold and Patterson (2005) and Pugh et al. (2018) were used to estimate each state’s forest inventory by a predetermined list of classifiers (Supplementary Table S1). Natural disturbance history was estimated from both the FIADB and LANDFIRE (USGS, 2016) datasets to better constrain initial belowground and soil carbon parameters during what the modeling framework refers to as the model spin-up period (Kurz et al., 2009). Estimates of merchantable volume and corresponding biomass from FIADB were used to calibrate the model’s allometric volume-to-biomass assumptions to match forest type groups and growth conditions in Maryland and Pennsylvania.
To estimate empirical growth-yield curves, a Gompertz growth function (Eq. 1) was used to model plot-level relationships between merchantable timber volume and average stand age. This growth model is a common exponential function used to estimate various forest attributes while not assuming symmetry within the curve unlike other logistic functions (Fekedulegn et al., 1999). The Gompertz growth curve takes the following form:
where, is the upper asymptote, is the growth displacement, and k is the growth rate or slope at time t.
Due to limitations of using stand age as a predictor to estimate merchantable volume in uneven-aged stands following harvest events, a modified growth-yield table was derived by utilizing methods developed and validated in Pilli et al. (2013) for calculating annual growth increments of unevenly aged systems following harvests. This is derived from the assumption that younger cohorts of trees move toward canopy dominance via canopy gap dynamics inherent to uneven-aged managed forests common in this region of the US. This methodology outlines that, following the removal of a specific proportion of merchantable volume, the growth curve continues to approach the same asymptote established in the unmodified growth-yield table. Using merchantable timber volume as a function of stand age and productivity class, MAI of each productivity curve can be modified using a basic exponential function (Supplementary Equation S2) to then reapproach the original asymptote.
Harvest removals were estimated as an average annual removal of merchantable timber in cubic feet between 2007 and 2019, converted to metric tons of carbon using methodologies and specific gravities reported by Smith et al., 2006. To assign a harvest type and intensity to each record of volumetric removal, stand age at the time of removal was calculated by taking the mid-point average between time t1 and t2 (Bechtold and Patterson, 2005) where t1 is the year the unharvested stand was measured and t2 is the repeat interval year measurement post-harvest. In collaboration with state partners, harvest type and intensity were determined heuristically for each forest type based upon state-level management documentation, peer-reviewed literature, and expert input. A complete list of harvest types and intensities prescribed to each forest type group as a product of stand age can be found in Supplementary Table S3.
Longer-term averages from 2007 to 2019 were used to estimate annual area targets for all LUC and natural disturbance events including wind, fire, disease, and insects. Averaged annual LUC rates by ownership and forest type group were derived by overlaying a geospatial forestland ownership dataset (Sass et al., 2020), the Protected Areas Database of the U.S. (PAD-US), a national geodatabase of protected areas (USGS, 2018), and the National Land Cover Database (NLCD), a remotely-sensed data product used to characterize land cover and land cover change (Wickham et al., 2021). Wind disturbance events were calculated using the LANDFIRE Historic Disturbance dataset (USGS, 2016), a remotely-sensed data product provided by the USGS that estimates annual disturbance events. Annual averages for wildfire disturbances were derived from the LANDFIRE Historic Disturbance dataset (USGS, 2016) and validated through annual reports from the National Interagency Fire Center (NIFC). Annual prescribed fire acres were estimated from reports provided by the Maryland DNR Forest Service and Pennsylvania DCNR Bureau of Forestry and scaled to represent treatments on forestlands only. Annual acreages of insect and disease disturbance were derived from National Insect and Disease Detection Survey (USDA Forest Service, 2020), a spatial data product produced by USDA that collects and reports data on forests insects, diseases, and other disturbances. For more information on all input and activity data, see Supplementary material 1.2. A complete list of BAU parameters can be found in Supplementary Table S2.
2.3. Harvested wood products data and modeling framework
To calculate and assess carbon stored by and GHGs emitted from forest products, we developed separate HWP models (CBM-HWP-MD for Maryland and CBM-HWP-PA for Pennsylvania) using the Abstract Network Simulation Engine (ANSE) model framework. ANSE is a carbon estimation tool developed by the Canadian Forest Service and employed in annual reporting of Canada’s national GHG inventory. Both the CBM-HWP-MD and CBM-HWP-PA models facilitate modeling, tracking, and calculation of embodied carbon stored by and emitted from HWPs. Furthermore, both models were adapted to and parameterized with US-based data and estimates and region-specific data where available.
Specific disturbance actions implemented in the CBM-CFS3 (particularly, though not exclusively, harvest events) transfer carbon in the form of metric tons of carbon directly into the CBM-HWP-MD and CBM-HWP-PA models. This transferred carbon is then partitioned among various wood product streams (Supplementary Figure S4), based on current practices in the forest products sector. Carbon is either exported in the form of roundwood exports or produced commodities, retained for domestic commodity use, or immediate use domestically for mill residues, energy, and additional commodity production. Maryland and Pennsylvania have distinct product ratios split by wood type (Supplementary Figure S5). Each commodity stream has a corresponding half-life that determines the in-use residence time of carbon in a specific product stream before being allocated to an end-of-life path (i.e., recycled, burned for energy, or sent to a landfill). Landfills also have corresponding half-lives by landfill type and location, and from which carbon eventually decomposes and is emitted to the atmosphere as either carbon dioxide (CO2) or methane (CH4). Furthermore, each state specific model tracks inherited wood products, or products in-use, prior to model simulation starting from 1950 onwards.
When HWPs are substituted for alternative, more emissions-intensive products (e.g., concrete, steel), that change in production is assumed to have associated displaced emissions, or substitution benefit (Geng et al., 2017). For example, when additional wood products are manufactured relative to BAU, we assume those additional products will be used in place of other non-wood materials, resulting in a corresponding substitution benefit and reduction in GHG emissions (Leskinen et al., 2018). Substitution benefits are applied only to saw logs, composite panels, and bioenergy products. Inversely, a decrease in wood product manufacturing results in increased emissions (or negative substitution benefits; Myllyviita et al., 2021).
Using methods from Smyth et al. (2017), we calculated and applied state-specific displacement factors for softwood and hardwood saw logs and composite panels. The calculated displacement factors rely on LCA data for the emissions associated with the extraction, raw material transport, and manufacture of both the HWPs and the assumed alternative materials and Smyth et al. (2017) data on relative product weights in different end use products. As an additional component of the displacement factor calculations, we used state-level RPA mill residue and primary timber product data (core tables 10 and 5) and Howard et al. (2017) data on wood product market share percentages in the US to calculate weighted lumber and composite panel production and use for each state. Maryland had displacement factors of 2.045 (softwood saw logs), 2.681 (hardwood saw logs), 2.682 (softwood composite panels), and 1.972 (hardwood composite panels). Pennsylvania had displacement factors of 2.032 (softwood saw logs), 2.692 (hardwood sawlogs), 2.682 (softwood composite panels), and 1.932 (hardwood composite panels). We applied a conservative and linearly decreasing displacement factor for bioenergy for both states, starting at 0.47 in 2022 (Smyth et al., 2017) and reaching zero by 2040 to account for Maryland’s net-zero target by 2045 and the Biden Administration’s goal of reach a carbon pollution-free power sector by 2035. Calculations reflect current wood and alternative product extraction, raw material transport, and manufacture; they do not assume increased carbon efficiencies in wood (or alternative product) production throughout the modeling period, nor the adoption of carbon capture and storage, as these technologies are not currently widely adopted within the region.
For scenarios that result in lower levels of harvest relative to BAU, we apply a leakage factor to estimate an assumed increase in harvest activities occurring outside the study area compensating for a decrease in timber supply (Murray et al., 2004). We assume demand for wood products remains constant despite reductions in harvest and assume a portion of that demand will be met via imports of additional wood (i.e., leakage). Further, we assume all remaining product demand not met by wood imports will be met by an increased use of non-wood materials in place of wood. Determination of leakage rates are dependent upon the degrees of assumed regional collaboration with estimates ranging from 63.9% (Gan and McCarl, 2007) to 84.4% (Wear and Murray, 2004). In this study we assumed a leakage factor of 63.9% due to the multi-state nature of the project, meaning that the remaining 36.1% of reduced harvest rates are subject to additional emissions from non-wood materials. Leakage is only assumed to result from reduced in-state harvest whereas increased in-state harvests are assumed to result in increased wood utilization rather than reductions in out-of-state harvest.
State-specific trade and commodity data from Resource Planning Act (RPA) assessments (USDA Forest Service, 2021), US Commodity Flow Surveys (US Department of Transportation, Bureau of Transportation Statistics, US Department of Commerce, and US Census Bureau, 2020), US International Trade Commission export data (US International Trade Commission, 2021), and published peer-reviewed data (Howard and Liang, 2019) when available, or US averages from the same sources, were used to adapt and parameterize both HWP models. FAOSTAT data (FAO, 2021) were utilized to determine the commodity distributions of exported roundwood. Softwood products were parameterized and modeled separately from hardwood products, as the two wood types differ in exports and commodities produced as well as their associated product half-lives and displacement (Dymond, 2012; Howard et al., 2017). Published data were used to calculate softwood- and hardwood-specific half-lives for Maryland and Pennsylvania sawn wood and veneer products, while we relied on literature estimates for other products (Smith et al., 2006; Skog, 2008). To calculate substitution benefits, we coupled region-specific data (USDA Forest Service, 2021), US consumption rates (Howard et al., 2017), product weights (Smyth et al., 2017), and LCA data (Bala et al., 2010; Dylewski and Adamczyk, 2013; Meil and Bushi, 2013; Puettmann et al., 2016; Puettmann and Salazar, 2018, 2019; Hubbard et al., 2020; Puettmann, 2020), following methods developed by Smyth et al. (2017). Landfill CO2 and CH4 emissions rely on IPCC defaults for methane generation (k) and landfill half-lives for wet, temperate climates (Pingoud et al., 2006). See Supplementary material Appendix S1 for more details on substitution and leakage calculation methods and Appendix S2 and Appendix S3 for site-level modeling parameters.
2.4. Management and wood utilization scenarios
The management, disturbance, and wood utilization data collected as described above were used to parameterize our models for the historical period of 2007–2019. Simulations run for a total of 93 years in which the first 13 years use observed activity data and the remaining 80 years use scenario-based activity data to cover both the historical period (2007–2019) and forward-looking projections (2020–2100), providing seamless comparisons between past and future trends. Simulation projections use the most recent decadal (2007–2019) averages for harvest yields, natural disturbances, LUC, and wood use determined by the activity data above. Alternative management scenarios were then developed by changing selected parameters from the BAU scenario to represent possible alternate forest management practices or priorities. All other disturbance and climate data were assumed to be constant in all scenarios except for specific climate change scenarios. Scenarios were grouped for analysis into six broad categories representing similar management practices or objectives: (1) altered rotations; (2) tree planting; (3) maintain forest health and regeneration; (4) climate change impact; (5) no harvest activities; and (6) bioenergy (Table 3). A portfolio scenario was also constructed by combining multiple individual scenarios to represent a concurrent suite of climate-smart forestry activities.
BAU results are reported in million metric tons of C (MMT C) or million metric tons of CO2 equivalency (MMT CO2e), using 100-year global warming potentials. Annual Net Biome Productivity (NBP) is calculated as gross ecosystem productivity minus C losses from respiration, decomposition, and disturbance (including harvest and fire), where negative values represent a net carbon sink and positive values represent a net carbon source. To assess the relative gains in mitigation potential from alternative management and wood utilization practices, scenario results below are discussed in both actual values and in standardized terms relative to the BAU scenario. This standardized mitigation potential (henceforth, “mitigation”) is calculated by subtracting the annual net C balance of the BAU from the annual net C balance of each scenario, comparing net GHG emissions (CO2, CH4, CO, N2O) for each modeled scenario with net GHG emissions from the BAU simulation. This approach isolates the effect of each management practice to understand and assess the differential impact between an alternative management approach and BAU. Net emissions (or the net C balance) include (1) emissions resulting from disturbances (harvest, LUC, or natural disturbance) or decay processes in the forest ecosystem, (2) emissions resulting from HWP use, mill efficiency, and decomposition, (3) emissions results from the substitution of non-wood materials and bioenergy for other forms of energy derivation, and (4) leakage from reduced harvest rates.
A total of 15 management scenarios were modeled for Maryland and 13 management scenarios for Pennsylvania, along with two climate change impact scenarios and two bioenergy scenarios for both states (Table 3). All scenarios were developed within a participatory framework alongside state partners to represent a variety of plausible or theoretical management activities that might be implemented to respond to forest health concerns, shifting forest management priorities, or policy incentives. Partners were asked to identify scenarios of interest for all land ownership types, as well as the scale and duration of each management practice. The development of scenarios and modeling results and analysis were conducted in a close iterative process with our state partners to validate and infer any model results and parameters. More detailed descriptions of scenarios, methodologies, data acquisitions, and assumptions for each can be found in Supplementary material Appendix S1.
3. Results
3.1. Business-as-usual simulation
Our results are first partitioned between the BAU forest ecosystem C balance followed by C dynamics in the forest products sector. We then analyze the two linked assessments prior to assessing and quantifying impacts of alternative management scenarios as counterfactuals to the BAU.
3.1.1. BAU forest ecosystem C balance
Results from the BAU simulation from the forest ecosystem model suggested that forest ecosystems in Maryland will remain a net C sink (Figure 4A) from 2020 to 2100. In contrast, our results showed forest ecosystems in Pennsylvania were projected to become a net C source in 2025 (Figure 4C). From 2020 to 2100, Maryland forest ecosystems cumulatively sequestered an additional −25.96 MMT CO2e while Pennsylvania forest ecosystems cumulatively emitted +306.12 MMT CO2e. In both Maryland and Pennsylvania, the weakening forest sink was driven by LUC, a reduction in MAI due to forests aging (Supplementary Figures S10, S11), increased turnover rates from accumulation of dead organic material on the forest floor and soil pools (Supplementary Figure S9), and carbon being removed from the ecosystem through harvest delaying immediate growth potential of forests, but may ensure future growth as forests recover post-harvest.
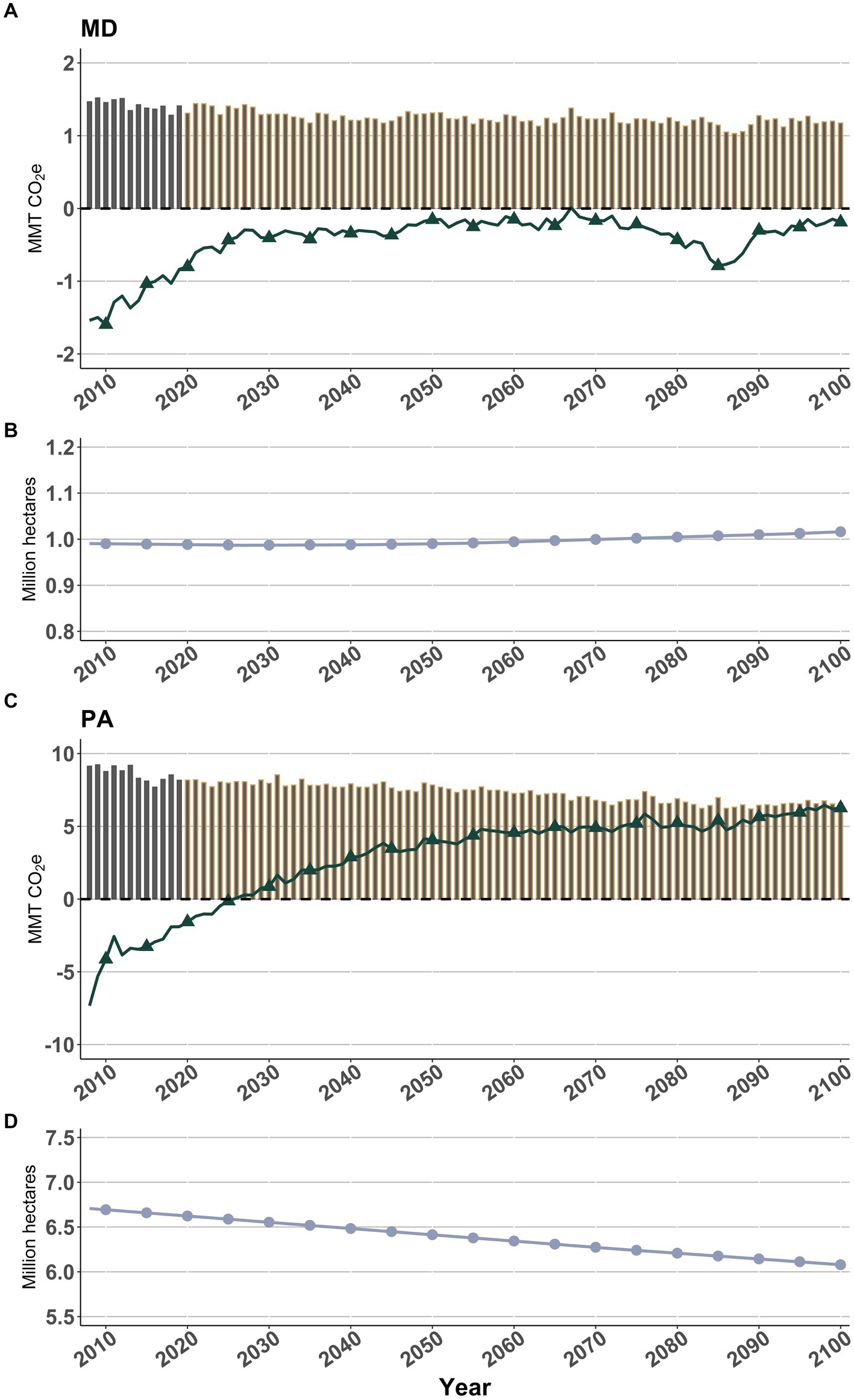
Figure 4. BAU scenario forest ecosystems results showing net biome productivity (green line), historic harvest removals (gray bars), and modeled harvest removals (dark brown bars) for (A) Maryland and (C) Pennsylvania. Annual net biome productivity (NBP) represents all growth minus respiration. Decomposition, and disturbance emissions (or transfers to the HWP sector). Panels (B) and (D) represent forest area (million hectares) across the BAU simulation for Maryland and Pennsylvania, respectively.
Timber harvest significantly impacted the C balance in both states where annually, Maryland harvested 1.22 MMT CO2e yr.−1 and Pennsylvania harvested 6.99 MMT CO2e yr.−1 on average, with cumulative removals of 198.9 MMT CO2e and 1139.9 MMT CO2e from 2020 to 2100 in Maryland and Pennsylvania, respectively. In both states, harvest rates remained relatively constant with an average of 7,516 ha yr.−1 in Maryland and 56,959 ha yr.−1 in Pennsylvania, representing 0.76% and 0.85% of the forest area in each state, though harvest rates declined slowly throughout the latter half of the century (Figure 4) as a substantial portion of forests in both states age beyond typical harvesting age thresholds (Supplementary Figures S6, S7). In isolation, harvest removals were represented as loss within the forest ecosystem results (Figures 4A,C) because the carbon in harvested wood is removed from the ecosystem; however, it is important to note that this carbon is not immediately emitted to the atmosphere but is instead transferred to the forest products sector.
As noted above, large forest areas in both states are projected to surpass typical harvesting age thresholds and reach older age classes (>130 years) where age-related patterns and processes limit forest productivity (Ryan et al., 1997; Binkley et al., 2002; Silver et al., 2013). However, older forests continue to store a substantial amount of carbon as shown in Supplementary Figures S20–S23. Area of young forests were projected to decline in both states from 2020 to 2100, driven by the dominance of aging forests on the landscape. The reduction in annual MAI caused by forest aging along with positive DOM emissions significantly weakened C sink strength in Maryland from an annual net emission of −0.45 MMT CO2e from 2020 to 2029 to an annual net emission of −0.29 MMT CO2e from 2050 to 2100, whereas Pennsylvania sequestered −0.16 MMT CO2e annually from 2020 to 2029 but emitted 5.11 MMT CO2e annually from 2050 to 2100 (Table 4, BAU).
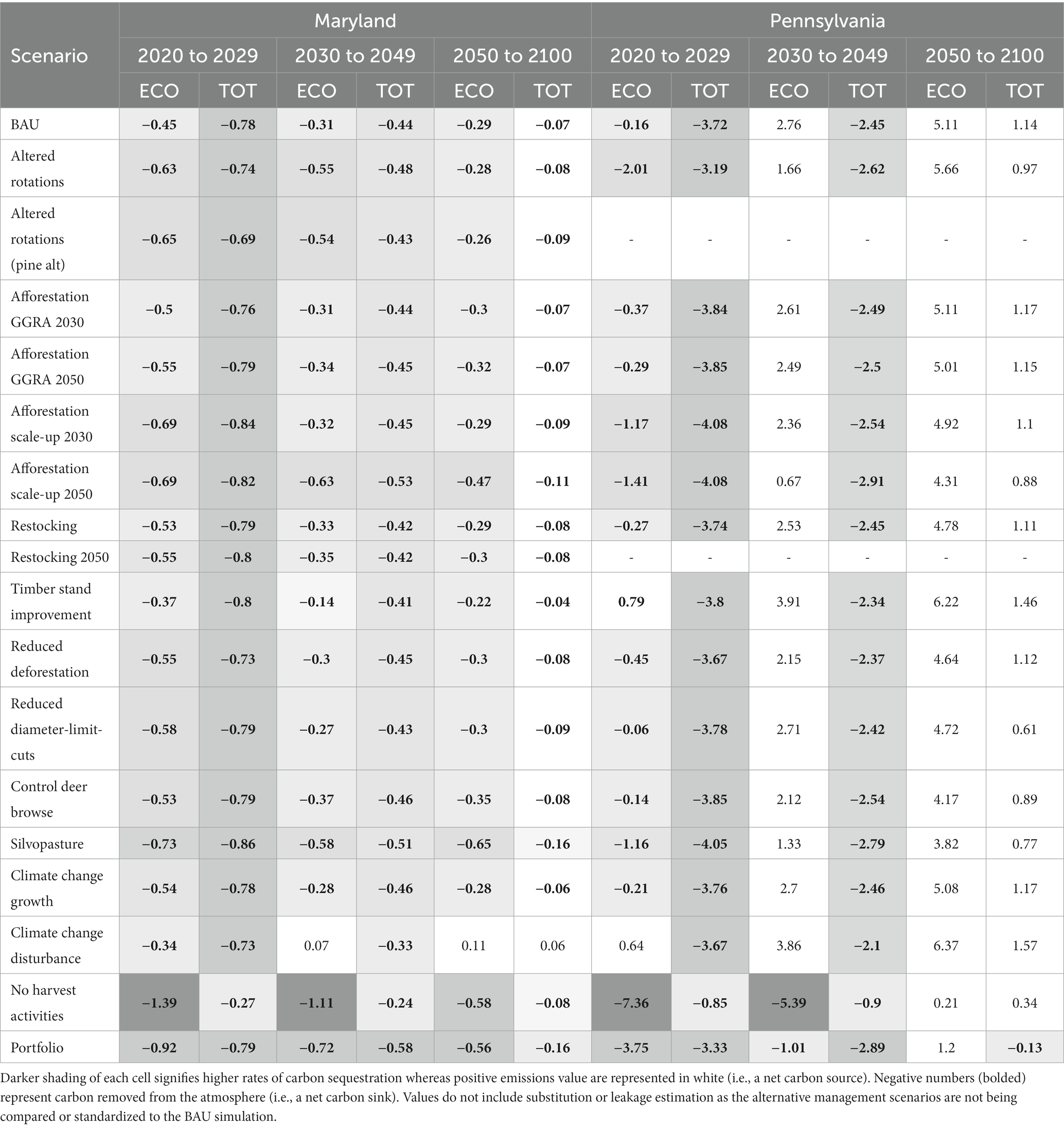
Table 4. Averaged annual net mitigation potential of BAU and each scenario for both Maryland and Pennsylvania for 2030, 2050, and 2100 (MMT CO2e-) for the forest ecosystem (ECO) and the forest ecosystem combined with HWP sector (TOT).
LUC spurred the weakening of the carbon sink from reduced future forest growth and increased post conversion DOM and soil emissions. Based on historical trends, the BAU scenario projects annual net forest loss of approximately 7,000 ha yr.−1 in Pennsylvania (0.01% of forest area) and 193 ha yr.−1 (0.02% of forest area) in Maryland (Table 3). These trends fluctuated based on future land area eligible for LUC activities, and net annual forest loss decreased in both states throughout the scenario, such that Maryland is projected to reach annual net forest gains of 694 ha yr.−1 by 2100 (Figure 4B) where the switch in net forest loss to gain was primarily driven by a lack of eligible area within the simulation to be deforested due to inputs being entirely prescriptive (i.e., afforestation rates remain constant but deforestation rates decline throughout the simulation). Forestland gradually increased in Maryland helping to maintain and stabilize the net forest C balance by the end of century. Although, LUC emitted an average of +0.12 MMT CO2e yr.−1 in Maryland and +0.49 MMT CO2e yr.−1 in Pennsylvania from 2020 to 2100.
Emissions associated with biotic and abiotic disturbances including fire, wind throw events, and disease and insect outbreaks represented an annual average loss of +0.004 MMT CO2e yr.−1 in Maryland and +0.057 MMT CO2e yr.−1 in Pennsylvania from 2020 to 2100. These emissions and their underlying causes, while significant for forest dynamics, lacked the same level of impact on net forest ecosystem C balance as LUC and harvest activities. Our BAU results did not account for fossil fuel emissions from logging operations and transportation of fiber materials. Following international reporting guidelines, all emissions from fossil fuel uses were reported outside the Land-use and forestry sector. Although, fossil fuel emissions for both wood products and other products are considered in the estimation of substitution benefits.
3.1.2. BAU harvested wood product C balance
HWPs provided additional carbon storage in both Maryland and Pennsylvania, as carbon inputs into the HWP sector (via harvest) outpaced emissions from current HWPs in use and inherited HWPs (those already in use at the start of the BAU scenario in 2007) in use or in landfills. From 2020 to 2100, Maryland stored an additional 11.57 MMT C in HWPs (Figure 5A) while Pennsylvania stored an additional 72.46 MMT C (Figure 5B). Most harvested wood was retained for domestic commodity production and use, with roundwood exports consisting of less than 2% of harvested hardwood logs in the BAU scenario (Supplementary Figure S4). Pennsylvania utilized the majority of hardwood harvest as sawlogs, while Maryland utilized hardwood for pulpwood; most softwood logs were used for pulpwood in both states. Based on average mill efficiencies, just 51% of harvested wood material in Maryland and 61% in Pennsylvania were utilized as wood product commodities; the remainder was converted to mill residue leftover from commodity production. Primarily mill residues were used for pulpwood or other composite products, though roughly 8% and 12% were used for bioenergy in Maryland and Pennsylvania, respectively.
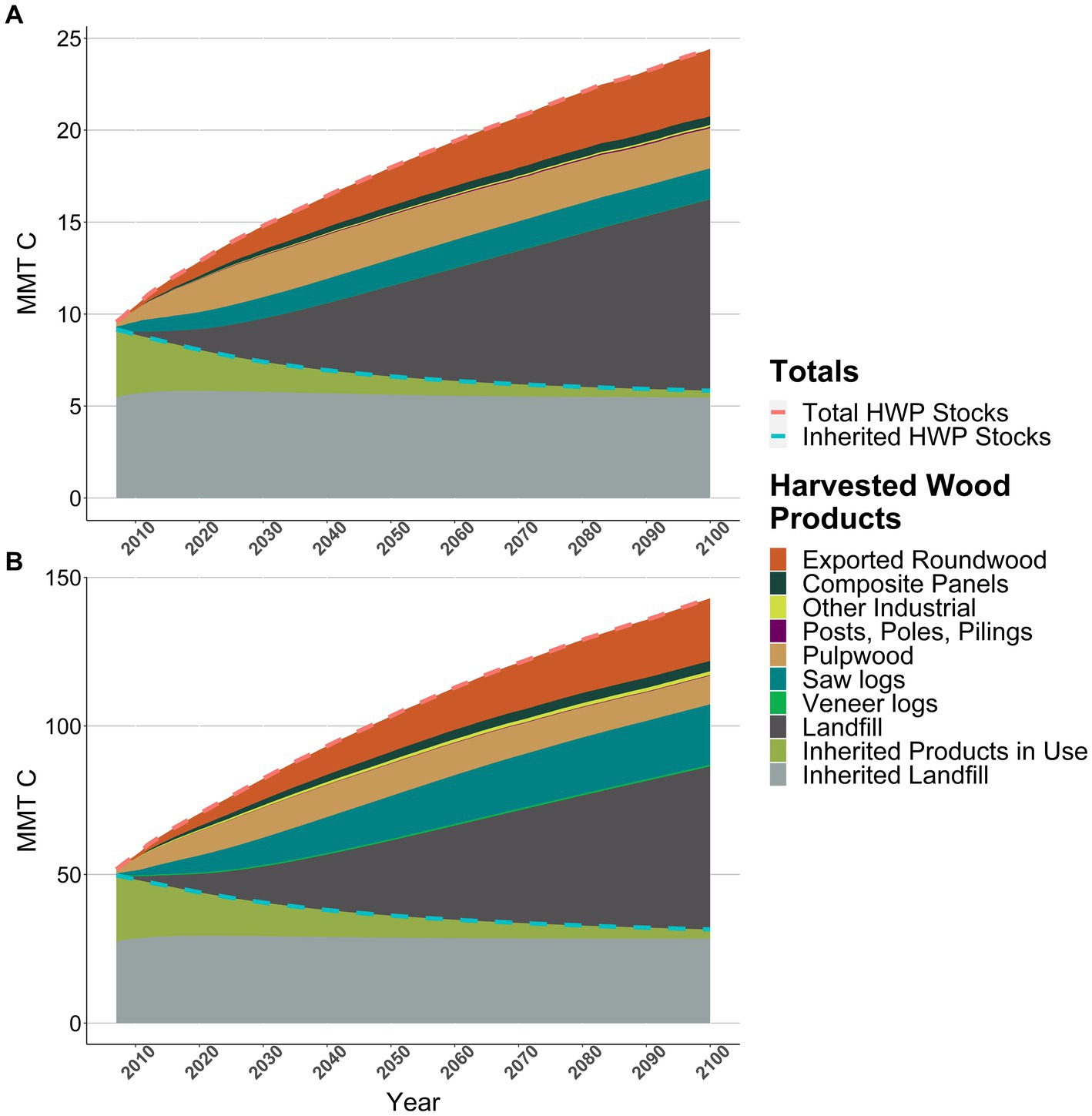
Figure 5. BAU scenario HWP carbon stocks by product stream (MMT C), 2007–2100, for (A) Maryland and (B) Pennsylvania. Inherited stocks represent stocks of products made from wood harvested already in use prior to the start of simulation runs.
Product half-lives and retirement strongly influenced the amount of C stored in or lost from HWPs. Longer-lived wood products, such as construction lumber, plywood, and panels, accounted for a significant proportion of the C stored in HWPs in current use. Products with shorter half-lives, such as pulp, paper, or bioenergy feedstocks, provided a relatively more fleeting C storage pool. Recycling of both sawlog (17.1% recycled) and pulpwood (68.2% recycled) products expanded functional lifespans and keeps C circulating in use, rather than being retired to landfills or energy recovery (Supplementary Figure S4). Landfills contained a significant pool of C from both inherited and new HWP stocks due to the slow rate of decomposition of wood within landfills and high rates of landfill HWP disposal. However, the diversion of material away from landfills represents a potentially undervalued and significant additional substitution benefit that is currently disposed of as waste in landfills. Increasingly evidence suggests that greater efficiency in wood product use by reducing material deposited in landfills can significantly boost mitigation potential through substitution (Hudiburg et al., 2019).
3.1.3. Total BAU net C balance
When assessing the net C balance of the forest ecosystem and HWP pools jointly (Figure 6), increased carbon stocks in the HWP sector enhanced the strength of the total C sink in both states. The net C balance of forests and the forest sector in Maryland reached a cumulative C sink of −38.42 MMT CO2e from 2007 to 2100 (an additional −12.46 MMT CO2e stored over the forest ecosystem alone). In the case of Pennsylvania, forest and HWPs lengthened the time the state remained a net forest C sink under BAU trends (through 2039 rather than 2025) and reduced the state’s cumulative emissions to 214.97 MMT CO2e from 2020–2100 (a − 91.15 MMT CO2e enhancement over forest ecosystem emissions alone). The gradual decline in harvest rates resulted in less C stored in HWPs, ultimately leading to an increase in net emissions because emissions from HWP remained constant while HWP inputs decreased.
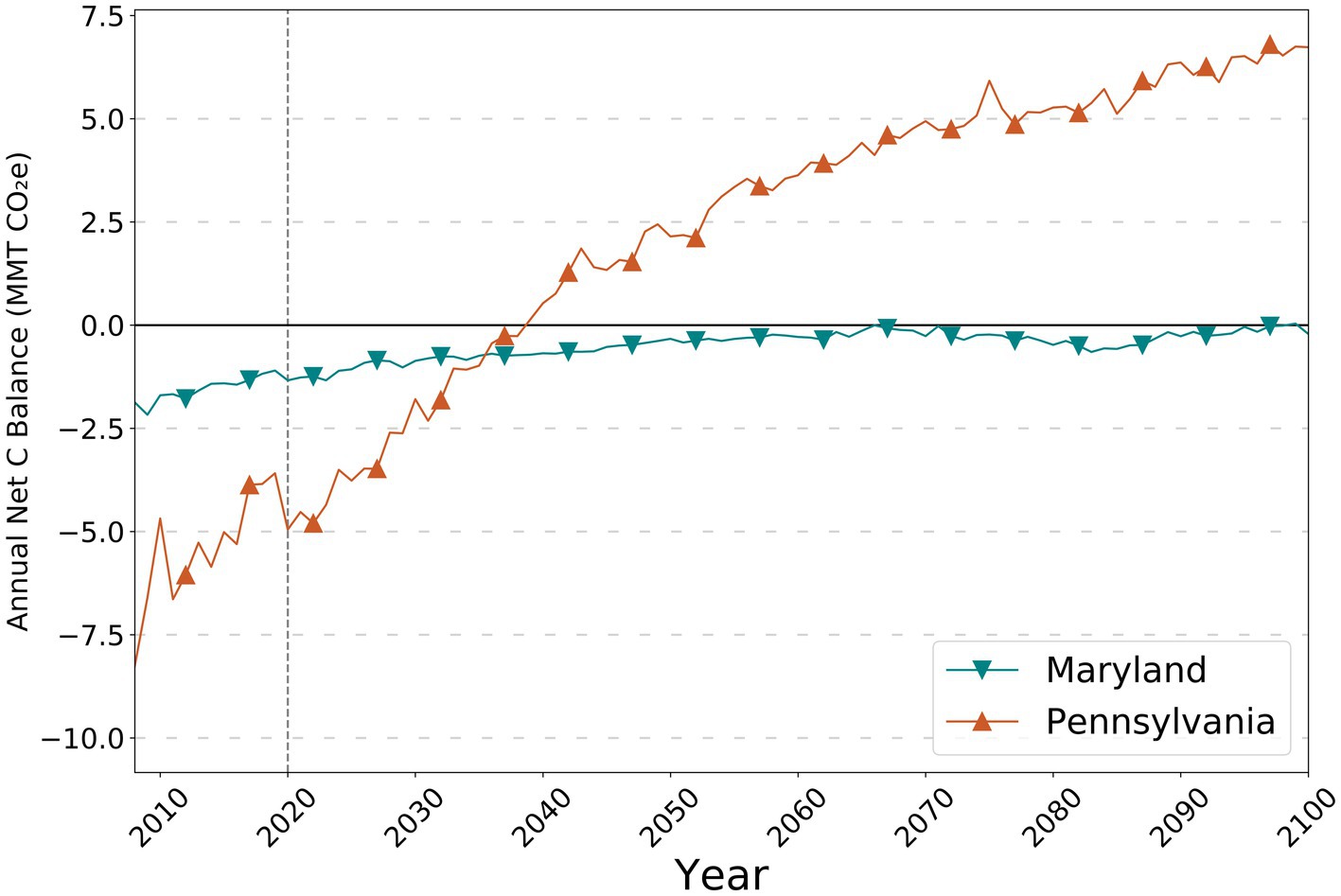
Figure 6. Annual net carbon balance for BAU simulations (MMT CO2e). Net carbon balance includes net ecosystem sequestration, carbon transfers to HWP, and emissions from wood products in use and in landfills. Negative values denote a removal of CO2e from the atmosphere (a net carbon sink).
3.2. Mitigation potential of alternative forest management and wood utilization scenarios
Several scenarios exhibited similar trends in both Maryland and Pennsylvania. The portfolio and some tree planting scenarios outperformed all other scenarios in terms of sequestering additional C (Figure 7). Most scenarios focusing on maintaining forest health, structure, and regeneration showed increased mitigation potential relative to BAU in both the forest ecosystem and HWPs. Altered rotations exhibited trade-offs in mitigation potential, increasing carbon storage on forestlands and (temporarily) decreasing carbon in HWP with a subsequent decline in substitution benefits until forest stands equalized under longer rotation lengths. Not all scenarios yielded additional mitigation potential; certain practices such as timber stand improvements treatments reduced C storage and sequestration relative to BAU as anticipated due to increased thinning and prescribed fire practices, which released C from the forest ecosystem in favor of forest health outcomes. However, the additional pulpwood removed comprised predominantly of pulp and paper products provided minimal additional substitution benefits due to the short half-life and quick retirement of such products.
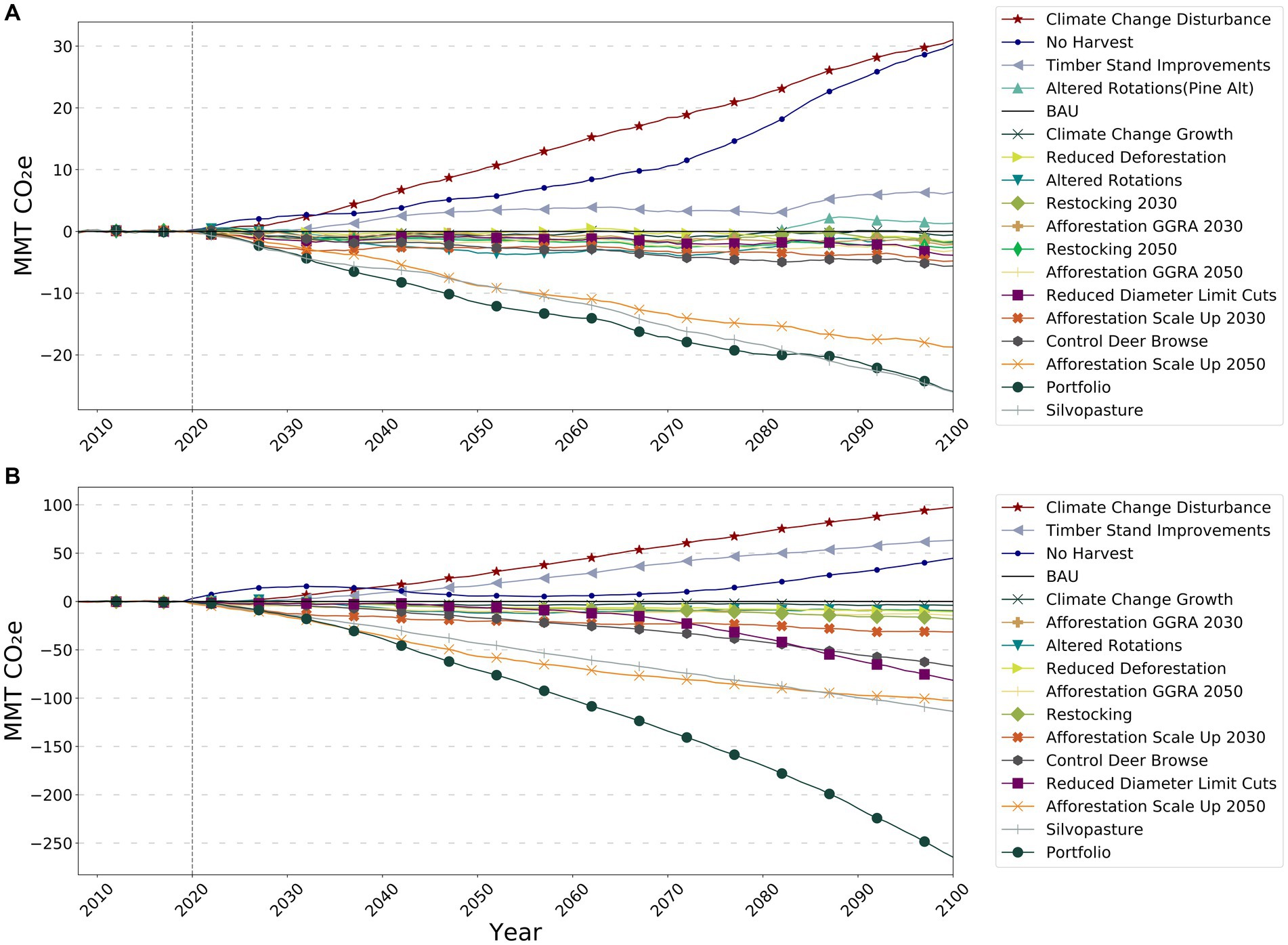
Figure 7. Cumulative carbon balance (MMT CO2e) for (A) Maryland and (B) Pennsylvania. Cumulative carbon balance includes net forest ecosystem emissions and net forest products sector emissions, including leakage and substitution benefits. Negative values denote additional carbon sequestration as compared to the BAU simulation whereas positive values denote lower rates of carbon sequestration as compared to the BAU simulation regardless of the absolute values (i.e., net carbon sink or source). Legend ordered by scenario in year 2100. For more detailed plots of cumulative carbon balance split by management practice type, see Supplementary material 1.5.
3.2.1. Altered rotations
Our results show in Table 4 that mitigation potential in the altered rotations scenarios initially increased C sequestration in the forest ecosystem and slightly decreased in HWP C. This decline in additional mitigation potential was driven by increased net HWP emissions caused by the initial deferred harvests, leakage from reduced rates of harvest, negative substitution benefits due to decreased wood availability (Figure 8), and loss of forest productivity due to age-related decline (Supplementary Figures S10, S11). Overall, results showed a net C balance of −0.74 MMT CO2e yr.−1 and −3.19 MMT CO2e yr.−1 sequestered from 2020 to 2029 in Maryland and Pennsylvania, respectively (Table 4). These increased ecosystem sequestration rates stagnated between 2030 and 2049 and returned to near BAU levels from 2050 to 2100. Cumulatively, altered rotations captured additional carbon relative to BAU at a decreasing rate, moving from −3.52 MMT CO2e additionally sequestered in 2050 to −1.68 MMT CO2e in 2100 for Maryland and from an additional −12.02 MMT CO2e sequestered in 2050 to −9.96 MMT CO2e in 2100 for Pennsylvania (Figure 7). Inversely, the altered rotation (pine alt) scenario in Maryland which increased the rotation age of loblolly pine (Pinus taeda) dominated forests an additional 20 years from the primary altered rotation scenario (now 80 years as opposed to 60 years) yielded a cumulative positive emission of +1.32 MMT CO2e as compared to BAU by 2100 (Figure 8). Driven largely by declining forest productivity and increased leakage rates. Shorter-lived pine species such as loblolly pine accrued carbon quickly during the first few decades of growth but rapidly declined in MAI soon after, limiting future additional C accrual. Overall, altered rotations increased carbon stocks within forests, but age-dependent declines in MAI dampened future sequestration potential.
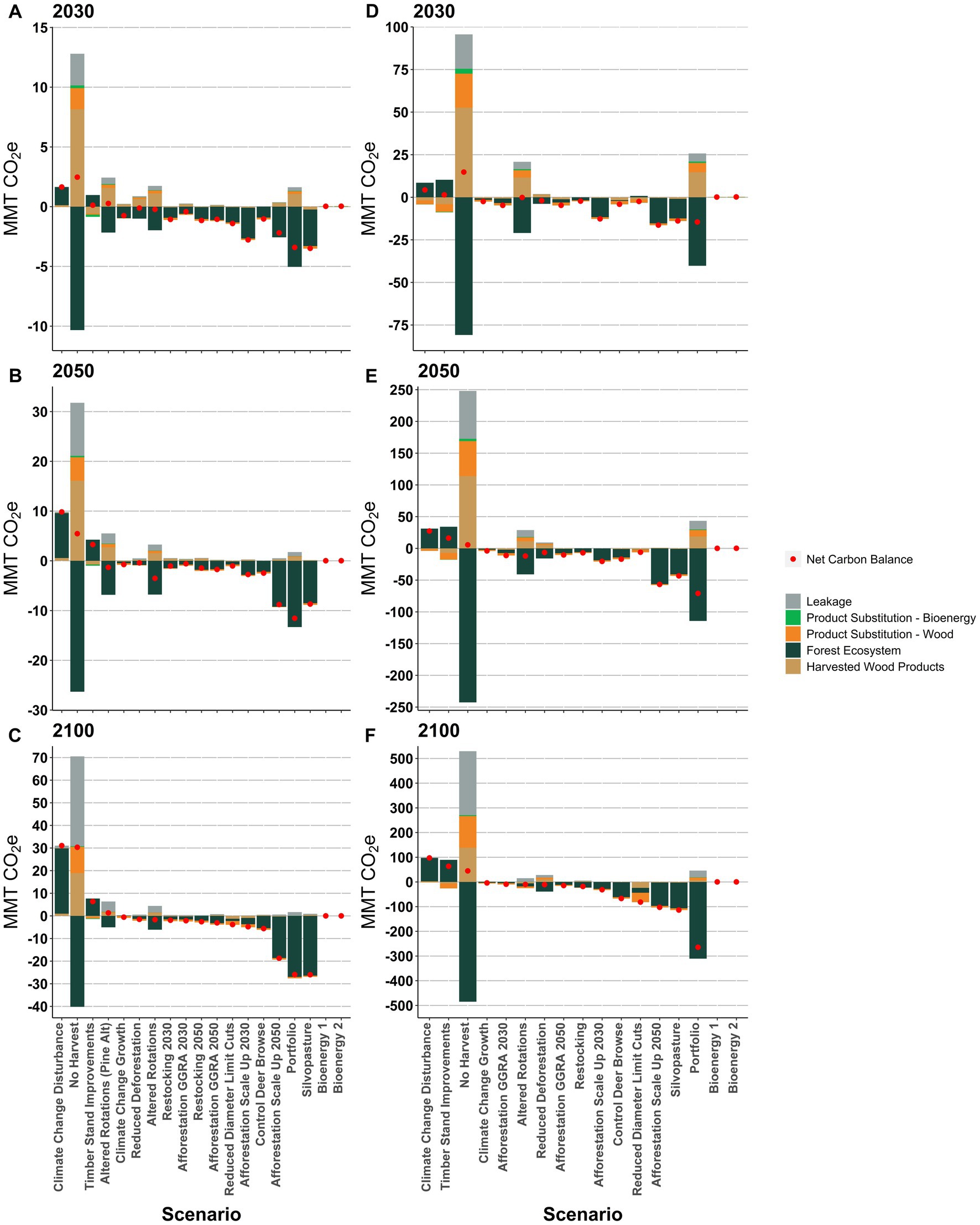
Figure 8. Cumulative mitigation potential by component in 2030, 2050, 2100 for (A–C) Maryland and (D–F) Pennsylvania. Red dots represent net carbon balance or the sum of all components for each scenario. Negative values denote additional carbon sequestered compared to the BAU simulation. Scenarios ordered by net C balance in year 2100 except for bioenergy scenarios. Y-axes across each panel are not the same.
3.2.2. Tree planting
All tree planting scenarios provided increased mitigation potential relative to BAU, proportional to the extent of area planted and the duration of planting. The afforestation GGRA 2030 scenario, the most modest tree planting scenario, provided only small gains of −2.13 MMT CO2e and −9.27 MMT CO2e additionally sequestered by 2100 in Maryland and Pennsylvania, respectively. On the other hand, the afforestation scale-up 2030 and afforestation scale-up 2050 scenarios resulted in additional mitigation potential of −4.80 MMT CO2e and −18.74 MMT CO2e, respectively, in Maryland and −31.54 MMT CO2e and −201.70 MMT CO2e, in Pennsylvania by 2100 (Figure 8). These scale-up scenarios assumed tree planting at 10x the rate of the GGRA scenarios, signaling that larger area targets and sustained timelines for tree planting realized proportionally larger C benefits. The afforestation scenarios started showing increased mitigation potential within the first 10 to 20 years. However, dependent upon the amount and duration of planting, these scenarios exhibited an increase in the rate of mitigation potential as the simulation progressed as compared to BAU driven by stands planted earlier in the simulation entering age-classes that maximized MAI. Lastly, the Silvopasture scenario, which modeled the conversion of agricultural land to low density treed systems for the entirety of the simulation showed strong mitigation potential in both states, with an additional −26.0 MMT CO2e sequestered in Maryland and an additional −113.73 MMT CO2e sequestered in Pennsylvania by 2100 (Figure 8).
3.2.3. Maintaining forest health, structure, and regeneration
All management scenarios focused on maintaining forest health, structure, and regeneration demonstrated better mitigation potential than BAU, except for the timber stand improvement (TSI) scenario (Figure 8). The loss of carbon in the TSI scenario for both Maryland and Pennsylvania resulted from higher volume of low-diameter timber being transferred to the HWP sector from increased cuttings, and larger forest ecosystem emissions from additional prescribed burns resulting in lower carbon stored in dead organic matter and deadwood C pools. However, the rate of carbon loss in this scenario was temporary as C sequestration accelerated in the latter half of the century from increased growth rates driven by thinnings. Additionally, the scenario does not quantify the reduced risk for carbon loss from catastrophic disturbance or other additional benefits provided from increasing controlled burns ultimately protecting carbon storage while promoting structural and functional diversity. The control deer browse, restocking, reduced diameter-limit-cuts (DLC) scenarios, which maintained or enhanced forest health through increased natural or artificial regeneration, provided an increase in C accumulation compared to BAU (Figure 7). In Maryland, the control deer browse, restocking, and reduced DLC scenarios cumulatively sequestered an additional −5.61 MMT CO2e, −1.9 MMT CO2e, and −3.86 MMT CO2e by 2100 relative to BAU, whereas Pennsylvania realized an additional −66.91 MMT CO2e, −18.20 MMT CO2e, and −81.56 MMT CO2e by 2100 from the same scenarios (Figure 7). The restocking 2050 scenario in Maryland cumulatively sequestered an additional −2.58 MMT CO2e suggesting that 20-year continuation restocking practices provides an additional −0.68 MMT CO2e mitigation potential. The mitigation potential of these scenarios increased through time, especially after 2070, due to newly established cohorts entering age-classes with higher growth rates (Supplementary Figures S10, S11).
3.2.4. Climate change impacts
The climate change disturbance and climate change growth scenarios explored the effects of future climate states on carbon storage and sequestration. Constructed using estimates of changes to future tree growth throughout the 21st century under RCP 8.5 (Matala et al., 2005; Duveneck and Scheller, 2015; Wang et al., 2017; Butler-Leopold et al., 2018; Supplementary Table S7), the climate change growth scenario provided minimal additional sequestration potential relative, capturing −0.59 MMT CO2e over BAU in Maryland and −3.98 MMT CO2e in Pennsylvania by 2100 (Figure 7). The climate change disturbance scenario resulted in decreased C sequestered relative to BAU, +31.05 MMT CO2e in Maryland and +97.34 MMT CO2e in Pennsylvania by 2100, caused by increased areas and intensities of natural disturbances such as fire or insect and disease outbreaks (Del Genio et al., 2007; Guyette et al., 2014; Lucash et al., 2017, 2018; Butler-Leopold et al., 2018) which led to greater direct emissions from combustion and dead organic matter decay.
3.2.5. No harvest activities
The no harvest activities scenario quantified future growth dynamics within the forest and effects on the HWP sector if all harvest and thinning activities were halted, but other management activities such as prescribed fire treatments continued. Initially, ecosystem C accumulation in the no harvest activities scenario outpaced all other scenarios since no C was removed from harvest (Table 4). However, annual productivity tapered off into the latter half of simulation period due to declines in productivity from age-related successional patterns and processes. While the forest ecosystem sequestered and stored a significant amount of additional carbon as compared to BAU, this scenario resulted in large net emissions from the HWP sector (due to continued emissions from retired products far surpassing C input), leakage, and negative substitution benefits, driven by an assumed inelastic demand for HWPs requiring material imports from other regions to satisfy continued timber demands (Figure 8). Ultimately, the sector-wide mitigation potential of no harvest activities resulted in additional emissions of +30.32 MMT CO2e in Maryland and +44.65 MMT CO2e in Pennsylvania by 2100 compared with BAU (Figure 7). This scenario highlights the influence that forest growth has on carbon sinks and the role that wood products have on sector-wide emissions. The decreased wood utilization from trees grown within Maryland and Pennsylvania in this scenario fundamentally altered carbon storage in HWPs, leading to near immediate increases in net HWP emissions; leakage and lack of substitution benefits ultimately outpaced any C benefits accumulated in the forest ecosystem.
3.2.6. Bioenergy
Both bioenergy scenarios had little to no effect on state-level carbon emissions relative to BAU (Figure 8). The additional 10% of mill residues diverted from pulpwood streams to bioenergy uses in bioenergy 1 and additional 10% of all mill residues diverted to bioenergy uses in bioenergy 2 each contributed less than −0.03 MMT CO2e in mitigation for both states by 2100. The scale at which mill residues were diverted did not yield significant differences on net emissions in either Maryland or Pennsylvania, and substitution benefits for the assumed displacement of energy coming from other fossil-fuel based energy sources were minimal (in part due to their application through 2040, to assess clean energy goals for both states). Diverting material from other primary production, secondary production, or harvest residues for bioenergy was not considered but could potentially provide substantial substitution benefits through increasing wood utilization efficiencies and from reductions to more fossil fuel intensive sources of energy such as coal or gas (Petersson et al., 2022). Additionally, substitution benefits provided from bioenergy could potentially be further boosted from incorporating carbon capture and storage technologies reducing emissions released to the atmosphere from the combustion process (Shahbaz et al., 2021).
3.2.7. Portfolio
The portfolio scenario modeled a suite of concurrent alternative management practices (Table 3) to understand the combined effect of multiple practices on forest ecosystem and HWP C balances. The portfolio provided the best mitigation potential of all scenarios, sequestering an additional −25.93 MMT CO2e in Maryland and −264.61 MMT CO2e in Pennsylvania by 2100 (Figure 7). The magnitude of additional C sequestration from the portfolio outpaced other scenarios and increased steadily relative to BAU, driven in part by management practices focused on forest health, structure, and regeneration. This scenario presents the best opportunity for minimizing forest carbon sink declines or, as in the case of Pennsylvania, prolonging the projected switch from net C sink to source. The portfolio scenario was the only scenario for Pennsylvania that remained a net C sink until 2100 (Table 4) when considering both the forest ecosystem and HWP. This effect was partly driven by mitigation potential from the HWP sector, as the forest ecosystem in Pennsylvania still ultimately became a net C source by 2100. The portfolio scenario increased the forest C sink by 29% in Maryland 38% in Pennsylvania by 2030.
4. Discussion
4.1. Forest trends and mitigation potential
Results from the BAU scenario suggest that forests in Maryland and Pennsylvania are projected to weaken as a net C sink throughout the 21st century and, in Pennsylvania, ultimately become a net C source. Several alternative management scenarios have the potential for additional mitigation benefits, including increasing or maintaining forest area, increasing the ability of forests to regenerate, encouraging sustainable harvesting practices, altering rotations, supporting sustainable wood utilization, and preparing for future climate change impacts (Table 5).
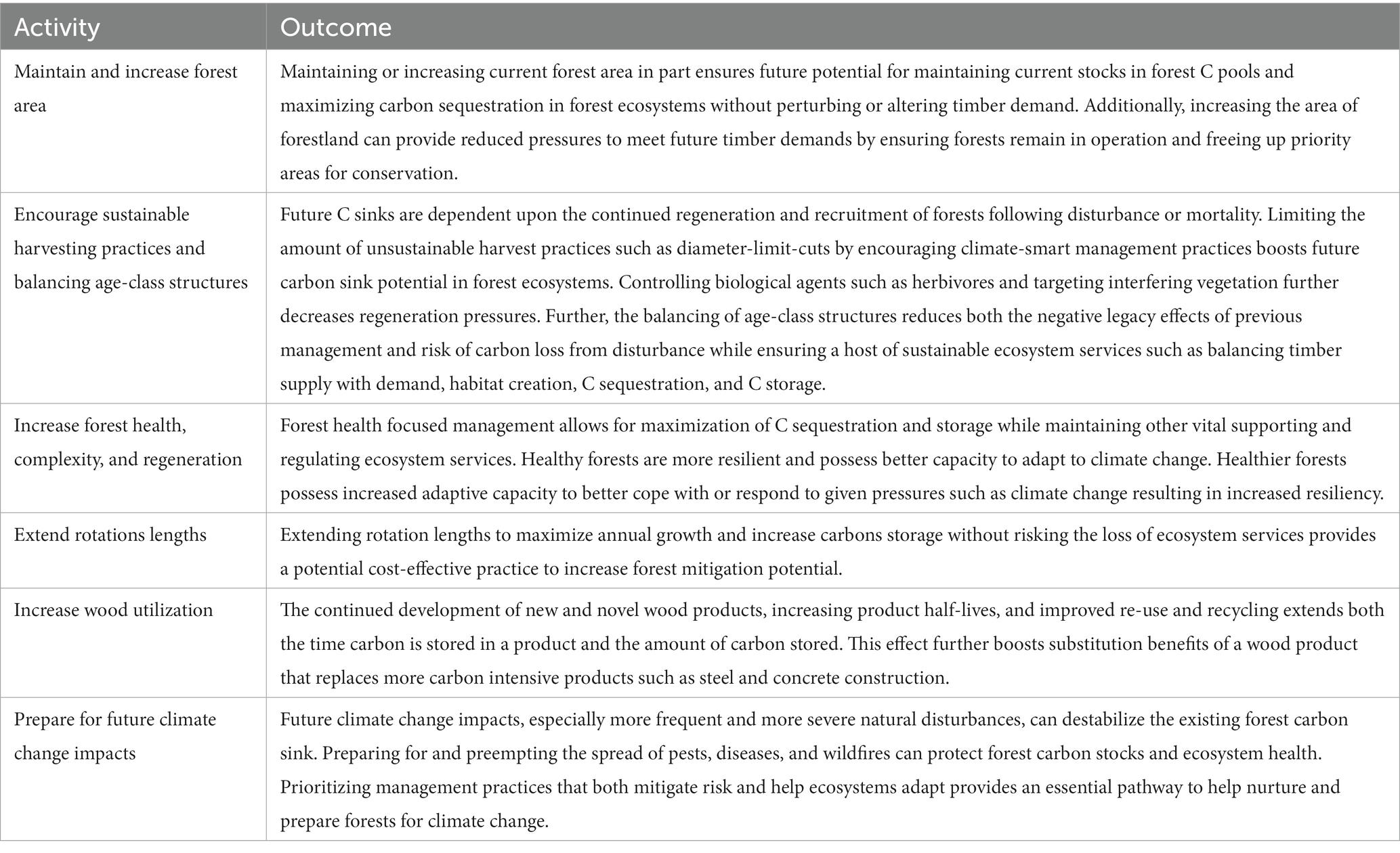
Table 5. Climate-smart forest sector pathways to enhance forest carbon sinks in support of net-zero emission goals.
Timber harvest remains the most extensive disturbance across eastern US forestlands in terms of both area and C impacts (Williams et al., 2016; Oswalt et al., 2019). Beginning in the 20th century and continued into the 21st century, the forest C sink primarily remained strong due to forest recovery or regrowth from previous harvests beginning in the mid-19th century (Birdsey et al., 2006; Woodall et al., 2015). Thus, the projected weakening trend in the forest C sink is driven in part by older forests becoming less productive with age (Ryan et al., 2010), which is well documented across US forests (Ryan et al., 1997; Binkley et al., 2002; Powers et al., 2011; Dugan et al., 2017; Curtis and Gough, 2018). However, our results strongly suggest that decisions around forest management, wood use, and policy can significantly alter and potentially improve the role of forests as C sinks in the future. Specifically, our results reinforce that forests can continue to provide a sustainable amount of wood products without hampering the mitigative potential of forests.
BAU results emphasize an important tradeoff between higher carbon stocks in older forests and diminishing rates of C sequestration as forest age (Harmon, 2001; Coomes et al., 2012; Yuan et al., 2019). However, the portfolio scenario supports that management can optimize the benefits received from a host of ecosystem services (D’Amato et al., 2011; Bradford and D’Amato, 2012; Bradford et al., 2013; Catanzaro and D’Amato, 2019; Littlefield and D’Amato, 2022) such as C sequestration in forests recovering from disturbance (Zhao et al., 2022), C storage in older forests (McGarvey et al., 2015; Curtis and Gough, 2018), and a steady stream of timber that stores additional carbon in HWP (Skog, 2008; McKinley et al., 2011) and drive the forest bioeconomy (Sohngen et al., 1999; Puddister et al., 2011; Antikainen et al., 2017). The cumulative mitigation potential realized by the portfolio scenario demonstrates the power of coordinated climate-smart forestry action, showing that the C benefits accumulated from certain practices can offset potential C losses from other management activities. The portfolio scenario results also suggest more complex interactions between management practices because contributions of some mitigation actions are not additive.
Results from the no harvest activities scenario emphasize the importance of quantifying emissions from the forest products sector. This scenario quickly increases sink strength in the forest ecosystem in both states but is accompanied by a substantial increase in emissions compared to BAU, driven by net emissions from HWPs, substitution, and leakage. Ultimately, the no harvest activities scenario performs second or third lowest in both states. This scenario signifies an important trade-off between immediate sequestration potential and future sequestration potential, ignoring the larger effects on local- to regional-economies (Antikainen et al., 2017) and potential carbon loss from increased disturbance risk associated with older forests (Kurz et al., 2008). As demonstrated with increased substitution emissions, the lack of timber supply forces other sectors (particularly construction) to increase use of non-wood materials such as concrete and steel, which have greater embodied emissions, significantly impeding GHG emission targets (Geng et al., 2017; Hildebrandt et al., 2017).
Balancing trade-offs and opportunities by managing forest landscapes as a heterogeneous patchwork provides a potential pathway to ensure forest ecosystems remain future carbon sinks (Wear and Coulston, 2015) while optimizing the co-benefits received from forests varying by age, structural complexity, and management goals (Nevins et al., 2021). These co-benefits include C storage and sequestration, habitat creation, reduced risk of disturbance, and enhanced resilience and adaptive capacity from both stand- to landscape-level management goals (Littlefield and D’Amato, 2022) as well as optimizing both short- and long-term mitigation potential (Petersson et al., 2022; Schulze et al., 2022). Specific to the context of Maryland and Pennsylvania, particular emphasis should be placed on forest regeneration following disturbances to ensure a strong future C sink, demonstrated by the scenarios in the maintaining forest health, structure, and regeneration category. The rate at which forests recover from disturbance is a strong driver of future forest C sink strength (Curtis and Gough, 2018; Peichl et al., 2022) in addition to providing valuable timber resources.
Protecting and encouraging natural regeneration is another key strategy for promoting forest diversity, in terms of age classes, species composition, and stand structure. Restocking efforts can promote a diversity of locally adapted species that are competitive under future climate conditions which, vary in mature size, shade tolerance, and canopy dominance as well as wood densities and hydrological strategies increasing forest resilience (Folke et al., 2004; Seidl et al., 2014; Anderegg et al., 2018). In addition to promising C gains in both the restocking and control deer browse scenarios, these efforts to promote increased biodiversity and structural complexity of forests also reduce disease and insect risk, further protecting future C storage and sequestration potential (Seidl et al., 2016, 2017). Ecosystem sequestration increases in the restocking scenario in the latter half of the century driven by maturing stands that have been restocked; however, the impact is not particularly significant due to a modest amount of forest stands targeted as restocking. Although control deer browse and restocking scenarios may be cost prohibitive for some, their expected C gains support these practices as a key tool for ensuring future forest productivity (Keefe et al., 2012; Buma and Wessman, 2013; Catanzaro and D’Amato, 2019; Dey et al., 2019).
The majority of forestland in the eastern US is privately held, with high potential for climate mitigation due to high percentages of working forestlands (Williams et al., 2016; Oswalt et al., 2019). Forest management planning and behavior on private lands to intentionally incorporate climate-smart management actions have strong potential for enhancing C mitigation, as demonstrated by the reduced DLC, control deer browse, and restocking results, while providing additional adaptation benefits (Köhl et al., 2010; Lafond et al., 2014; Lesser et al., 2019). However, significant uncertainties remain around forest policies that discourage harvesting or encourage lengthening rotation ages and their potential long-term effect on climate mitigation potential (Huntington et al., 2019). Ideally, policies should target not just management but the entire forest sector creating more flexibility to enhance possible forest outcomes (Sjolie et al., 2013). Potential policy effects on future investment in forest resources signals an important tradeoff where forest policy strategies could potentially incentivize continued investment in climate-smart activities or inversely, disincentive future investments (Pohjola et al., 2018). The relevancy and applications of these policies could affect both the rate and magnitude of future forest investments as well as discourage future harvests from extending rotations (Sohngen and Brown, 2011) potentially impeding future investments (Roberge et al., 2016). Where, as demonstrated by the afforestation, reduced deforestation, and silvopasture scenarios, increasing or maintaining forest extent, especially on private forestlands, remains one of the most cost effective and viable options to increasing C stocks and sequestration potential at the regional scale in the eastern US (Catanzaro and D’Amato, 2019; Doelman et al., 2020). This not only strengthens forest C sinks but has the additional benefit of reducing the timber demand on other forestlands, freeing up forests for a variety of both passive and active management approaches. Ultimately, this protects forestland by ensuring sufficient forests remain in operation to meet current and future wood demands while allowing landowners and managers to set aside areas of high priority for conservation (Pirard et al., 2016). The current decline in forest area only increases pressures on forests to meeting growing timber needs in addition to other essential supporting and regulating services (Jurgensen et al., 2014).
The allocation of forestland to various management goals and practices, such as bifurcating forests into ecological reserves and high productivity plantations based on site-specific considerations, provides a potential way to balance ecosystem service trade-offs and maximize the co-benefits humans rely on from forests (Bradford and D’Amato, 2012; Catanzaro and D’Amato, 2019). However, rarely is the delineation of forestlands into a binary paradigm of reserved lands vs. working lands easy or necessarily appropriate, considering the complex relationships between forest owner decision making, active and passive management strategies, legacies of disturbance, ecological complexity, variation in the vulnerability of sites and species to the projected impacts of climate change, and related policy implications. For example, areas of high risk to carbon loss from disturbance can be targeted for timber stand improvement practices to ensure carbon storage and stability into the future (Bachelet et al., 2015). Alternatively, areas of low forest productivity and regeneration can be targeted for restocking through enrichment plantings to boost both sequestration rates and future timber supply (Ontl et al., 2020) in part reducing timber demand of forests of higher ecological complexity and biodiversity (Köhl et al., 2020).
Balancing the trade-off between the current C storage and future C sequestration in the ecosystem relies primarily on relationships between growth, harvest removals, and recovery from disturbance, which are dependent upon both current and past legacies of management on forest structure and species composition driven by landowner goals (Seidl et al., 2014). Therefore, there is no one-size-fits-all approach to forest management for meeting carbon-related goals (Catanzaro and D’Amato, 2019). The preferred approach for any forest will vary on a case-by-case basis and be determined by climate implications, site-specific considerations including risks from natural disturbances, and forest owner values (Fahey et al., 2010; Catanzaro and D’Amato, 2019). At landscape scales, a full suite of climate-smart activities can be implemented simultaneously, as in the portfolio scenario, to optimize the mitigation potential of management activities under future climate uncertainties (Millar et al., 2007) and promote carbon and other ecosystem service benefits.
The climate change impact scenarios likely do not capture all the nuanced ways in which climate change may affect forest productivity, mortality, and demographic processes. Evidence of increased tree growth rates due to climate change is limited (Dow et al., 2022). Although the climate change growth scenario showed slight increases in growth under future climate conditions, it resulted in minimal C gains. Some studies suggest that climate change will increase drought-related mortality in forests and may ultimately decrease productivity, counteracting or exceeding any potential growth benefits observed within our prescriptive approach to future growth (Kurz et al., 2008; Allen et al., 2010, 2015). The climate change disturbance scenario emphasizes that increases in the severity and extent of natural disturbance drastically decreases ecosystem C stocks. The climate change impact scenarios suggest that increases in disturbance intensity and severity could counteract any projected increases in future growth caused by climate change resulting from carbon fertilization, warming temperatures, or longer growing seasons (Kurz et al., 2008). Additionally, evidence suggests that risk from wildfire, insect and pathogen, and drought disturbances pose a severe future threat to carbons storage (Allen et al., 2015; Jolly et al., 2015; Brando et al., 2019; Walker et al., 2019; Asaro et al., 2023). Therefore, focusing exclusively on C sequestration and storage cannot supplant other management goals such as enhancing forest health and resiliency without potential catastrophic repercussions for future ecosystem resilience. To do so would be contradictory to climate-smart forestry principles, which emphasize promoting future adaptation and resilience, and reducing catastrophic disturbance risk, while meeting current human needs. For example, the TSI scenario projected a weakening of the C sink in the near term relative to BAU due to increased removals and emissions from thinning and prescribed burns, but solely considering the C impacts does not fully account other co-benefits provided by these practices. Forest resilience treatments (which also consist of thinning and prescribed fire) can reduce future wildfire risk (Shinneman et al., 2012), increase forest restoration and health benefits (Brown et al., 2004), improve habitat creation (Tingley et al., 2018), and achieve desired species mixes or stand structural diversity (Brockway and Lewis, 1997). Resilience treatments can reduce catastrophic disturbance risk by increasing a forest’s adaptive capacity or an ecosystem’s ability to tolerate large-scale disturbances (Nicotra et al., 2015; Beever et al., 2016; Rogers et al., 2017) and future climate change induced decreases in resilience (Clark et al., 1999; Zhu et al., 2018; Hill et al., 2023). Further increases in wood utilization and efficiency of small diameter stems removed during resilience treatments can generate additional climate benefit by storing additional carbon and boosting substitution benefits (Mohammad, 2023).
Increased wood utilization has the potential co-benefit of slowing forestland conversion by supporting a more robust sustainable economy for wood products, whereas stopping harvest activities provides inverse incentives for landowners to potentially convert land (Sohngen et al., 1999). However, establishing safeguards to minimize negative externalities to forests of high biological value and biodiversity is advisable, understanding the complex interactions of multi-faceted management goals across the landscape (Clay and Cooper, 2022). Wood as a basis for a sustainable bioeconomy provides additional livelihood support for rural landowners (Puddister et al., 2011), incentivizing sustainable management in terms of C emissions and removals (Köhl et al., 2020), and a transition toward a sustainable bioeconomy supported by renewable resources instead of fossil fuels (Rockstrom et al., 2009). There is a significant trade-off between avoided GHG emissions from wood utilization and substitution and the near-term reduction in forest C sink from harvest (Soimakallio et al., 2016), but assessing net C balance within forest boundaries fails to acknowledge the key linkages between forest C dynamics, forest product-based C pools, and the important benefits of transitioning away from fossil fuel-based materials (Köhl et al., 2020).
Innovative wood utilization only serves to further boost the carbon mitigation potential of forests (McKinley et al., 2011). New technologies and uses increasing product half-lives bolster the C storage and substitution benefits of forest products, increasing displaced emissions (and so reducing embodied carbon emissions) from other more emissions-intensive materials or energy sources (Skog, 2008; McKinley et al., 2011; Xie et al., 2023). The construction industry presents a prime opportunity to maximize both the C storage and substitution benefits of HWPs, due to large emissions associated with concrete and steel production (Churkina et al., 2020; Zhang et al., 2020). Additionally, proper maintenance of landfills to ensure anaerobic conditions that slow decay of solid wood products offers additional opportunities to accumulate C stocks (Barlaz, 1998; Geng et al., 2017). Improved landfill management could involve the reduction of methane emission by diverting wood waste away from the landfill or capturing landfill methane emissions (Moreau et al., 2023). Lastly, increasing mill efficiency and effective wood recovery rates represent additional opportunities to boost the amount of C stored in forest products by utilizing more material (and the C it contains) in longer-lived products and reducing the amount of mill residue that would otherwise likely be emitted quickly (Lippke et al., 2011, 2012); over 90% of mill residue in both states goes toward short-lived pulp and fuel products, or is unused. However, to optimize both sequestration and storage benefits, forest owners and managers should consider balancing current forest growth rates with removals to not further exacerbate forest C sink declines.
To meet current ecological, economic, and climate goals, no single strategy is right for all landowners and forest types. Forest landscapes need to be managed under a variety of climate-smart approaches, tailored to site-specific conditions and management goals. This study demonstrates that a focus on existing priorities of forest health and stewardship can constitute part of an effective mitigation strategy (Millar et al., 2007), without hindering future demands on timber supply. While simultaneous increasing C sequestration potential to reduce atmospheric GHG levels in-service of 2030 emissions targets (den Elzen et al., 2022).
4.2. Management and policy opportunities
Significant mitigation opportunities exist through protecting existing forestland from permanent loss, i.e., keeping forests as forest, and planting trees in ecologically appropriate areas to increase forest acreage, two practices already aligned with policy priorities in Maryland and Pennsylvania (MDE, 2021; PDEP, 2021). Emphasis on restoration of degraded or poorly functioning forestlands along with implementing sustainable management practices would provide further C benefits through maintaining both forest health and productivity. More efficient wood utilization and creation of longer-lived wood products can increase C storage in HWP. Policies promoting bioenergy derivation from waste materials provide another potential climate mitigation strategy, especially if waste materials are diverted from landfill streams. Emerging carbon capture and storage technologies have added potential to couple with bioenergy, further increasing substitution benefits shifting production away from other more carbon intensive energy sources (Shahbaz et al., 2021; Petersson et al., 2022). Additionally, decreasing logging operation emissions, while not explicitly modeled in this study, would further enhance statewide carbon balances converging toward net-zero emissions targets. Coupling a reduction of fossil fuel use in management activities along with implementing best practices to reduce the impact of logging only further increases the potential of carbon benefits (Fahey et al., 2010). This does not preclude the impact that forest management activities focused on resiliency and adaptive capacity can have in altering net C balances. Further, the increased responsible use of forest products may result in additional climate benefits, ensuring net benefits for environment and society (Clay and Cooper, 2022).
Meeting global climate goals benefits from linking results of studies such as this to various policy levers and incentives, as well as communications and outreach. Tax programs, stewardship and management plans, voluntary offset projects, and cost-share programs have the potential to incentivize desired forest management behaviors, ensuring healthy and vigorous forests. Continued enrollment of forestland in sustainable forest management plans along with other policy vehicles may help to ensure the future viability of the forest C sink (Fahey et al., 2010). The incorporation of new data will help decisionmakers continually refine GHG emission targets and goals and better understand trade-offs, risks, and uncertainties associated with specific climate mitigation activities. Likewise, forest managers will benefit from incorporating the best possible information to inform flexible data-driven decision-making regarding forest management (Novick et al., 2022) to enhance the role of forests to meet GHG emission targets. The creation of flexible decision-making processes guided by the best available information may allow for an incorporation of refined management practices on a continual basis to adapt and mitigate climate change.
4.3. Limitations and future research
Uncertainties exist in the assumptions and simplifications necessary to model uneven-aged management through growth-yield curves, post-harvest stand dynamics, and late-stage successional dynamics (Ekholm et al., 2023). Theoretical curves of stand development may not capture multi-aged cohort dynamics of certain forests due to the complexity of forest ecosystems. Additionally, uncertainties remain about the long-term relationship between stand age and biomass represented in the form of growth-yield curves; statistical functions used to estimate the relationship between stand age and volume have strong implications for modeled results (Gustafson et al., 2020). Continued research will help to understand future forest growth, recruitment, and mortality under a changing climate with complex management interactions. With empirically driven frameworks, the chosen growth function used to model the relationship between stand-age and volume can lead to inherent biases in predicting growth, especially within older forest stands. Dependent upon the function, models may either over or under predict MAI in older stands due to increased uncertainty from underrepresentation of old trees in forest inventories. Future research will benefit from the development of improved data and modeling tools to understand tree to stand-level dynamics of volume and biomass and their relationship to stand age and other structural attributes to more accurately model forest growth within forest carbon models. Additionally, error associated with allometric equations to predict volume-to-biomass estimation remains dependent upon the sample size of trees but can oftentimes be ignored when using national inventories (Lin et al., 2023).
Modeling spatially stochastic events remains challenging but can be somewhat ameliorated through the usage of a spatially referenced model and the aggregation of forest records (Metsaranta et al., 2017). Furthermore, estimation methods for LUC can mischaracterize recently harvested forest pixels (instead classifying them as non-forest land use) and resulting in a potential overestimation of LUC rates; however, oftentimes remotely sensed inputs can be assigned an uncertainty of 5%–10%. Our analysis was designed to account for this as much as possible, but uncertainties still exist, and net C balance results are sensitive to assumed LUC trends. Capturing fine-scale management data, especially for private lands, remains challenging. However, through direct expert opinions from project stakeholders, we developed harvest regimes heuristically. Future research is needed to assess the sensitivity of future C sink-source strength to model inputs such as LUC rates, harvest rates, and growth as these inputs are prescriptive.
Further, quantifying relationships between substitution benefits, accounting of bioenergy emissions, and leakage across multiple timeframes remains challenging when the competing sectors are under pressure to actively decarbonize (Pingoud et al., 2018; Soimakallio et al., 2022). Some recent evidence suggests that substitution benefits are likely to be overestimated or oversimplified (Harmon, 2019); while this study has sought to provide reliable, state-specific substitution benefits by incorporating state- and region-specific wood use data and LCAs and allowing for the realities of leakage to dampen assumed product substitution needs, uncertainties in future product demand (which we assume to be constant) do contribute to overall substitution value uncertainties. In contrast, we did not consider substitution benefits from carbon capture emitted during woody biomass bioenergy derivation. Potential shifts in product types and half-lives of products have strong ramifications on future carbon storage (Xie et al., 2023), necessitating additional research to refine the relationship between carbon stored across HWP product streams, mill efficiencies, and the usage of both forest and mill residues. We assumed leakage rates for decreases in harvest rates; however, we did not estimate potential leakage rates associated with increases in harvest rates (i.e., reduced out-of-state harvest to compensate for increased in-state harvest); while this is how leakage is typically applied, it comes with an assumption in our case that all additional harvest goes toward additional in-state wood use, spurred by either policy or cost incentives. It is also worth noting that estimation of methane emissions from landfills, which are accounted for in this study, pose potential risks for improper accounting dependent upon assumptions which may result in smaller displacement factors (Chen et al., 2018).
Future forest C balances can be impacted in unforeseen ways with projected forest dynamics, climate change impacts, and disturbance regimes through 2100 which introduces uncertainty in our results. While some scenarios were designed to address this uncertainty prescriptively (such as the climate change scenarios), other uncertainties remain regarding fluctuations in socio-economic conditions. For example, knowledge of future rates of harvest and LUC remain highly uncertain. It is important to address socioeconomic factors driving forest management and the forest products sector when evaluating mitigation potential of scenarios due to their significant effects on both the results and feasibility of implementing carbon mitigation actions (Alig et al., 2004; Zhao et al., 2022). Additionally, our results do not incorporate changes to radiative forcings caused by a shift in surface albedo or other global teleconnection processes that may influence regional to global C balances (Swann et al., 2012).
5. Conclusion
Forest management and wood utilization can contribute substantially to regional and national climate goals. This research highlights the importance of fully accounting for GHG emissions from the forest ecosystem, land-use change, harvested wood products, and displaced emissions within a systems-based approach when evaluating the role forest management has in meeting current and future climate goals across diverse and heterogeneous forest landscapes. We examined GHG mitigation potential using a business-as-usual scenario, 15 management scenarios, as well as 2 climate and 2 bioenergy scenarios to assess how various forest sector activities affect future land surface carbon balance in both forest ecosystems and wood products. Results demonstrated that implementing a variety of climate-smart management practices can substantially increase forest C sink strength in Maryland and Pennsylvania without risking future forest resiliency. This study identifies key management activities that can significantly maintain or increase state-level forest carbon sinks, such as preserving and expanding forest area, increasing the ability of forests to regenerate, encouraging sustainable harvesting practices, extending rotations, supporting sustainable wood utilization, and preparing for future climate change impacts. Our results suggest that halting active forest management leads to counterproductive carbon outcomes when considering the forest ecosystem, harvest wood products, forest health, forest disturbances, and climate change. Concurrent implementation of best practices can increase the forest C sink by 29% in Maryland and 38% in Pennsylvania by 2030. However, the results and activities modeled in this study are not universally applicable. Designing and implementing management activities for climate benefits requires locally relevant knowledge and expertise to properly balance the trade-offs, risks, and uncertainties associated around the complex interaction of managing forests and the forest products sector.
Data availability statement
The raw data supporting the conclusions of this article will be made available by the authors, without undue reservation.
Author contributions
CP: Conceptualization, Data curation, Formal analysis, Investigation, Methodology, Software, Validation, Visualization, Writing – original draft, Writing – review & editing. KD: Conceptualization, Data curation, Formal analysis, Funding acquisition, Investigation, Methodology, Project administration, Resources, Software, Validation, Visualization, Writing – review & editing. KC: Conceptualization, Data curation, Formal analysis, Investigation, Methodology, Software, Validation, Writing – review & editing. DG-G: Conceptualization, Investigation, Methodology, Writing – review & editing. LC: Conceptualization, Funding acquisition, Project administration, Resources, Supervision, Writing – review & editing. WK: Conceptualization, Formal analysis, Funding acquisition, Investigation, Methodology, Project administration, Resources, Supervision, Validation, Writing – review & editing. MM: Conceptualization, Formal analysis, Investigation, Methodology, Resources, Software, Writing – review & editing. TO: Conceptualization, Investigation, Supervision, Writing – review & editing.
Funding
The author(s) declare financial support was received for the research, authorship, and/or publication of this article. This work was supported by the U.S Climate Alliance Technical Assistance Grant program and private foundations.
Acknowledgments
The authors thank our state partners at the Maryland DNR and Pennsylvania DCNR for providing their valuable time and expertise to accomplish this project. We thank Al Steele and others at the USFS for coordination on this project. Additionally, this study would not have been possible without the support of Eric Neilson, Scott Morken, and the entire Canadian Forest Service carbon accounting team. Lastly, we thank Eric Sprague, Brian Kittler, and Kristina Bartowitz for providing valuable guidance and feedback on this study.
Conflict of interest
The authors declare that the research was conducted in the absence of any commercial or financial relationships that could be construed as a potential conflict of interest.
Publisher’s note
All claims expressed in this article are solely those of the authors and do not necessarily represent those of their affiliated organizations, or those of the publisher, the editors and the reviewers. Any product that may be evaluated in this article, or claim that may be made by its manufacturer, is not guaranteed or endorsed by the publisher.
Supplementary material
The Supplementary material for this article can be found online at: https://www.frontiersin.org/articles/10.3389/ffgc.2023.1259010/full#supplementary-material
References
Alig, R. J., Kline, J. D., and Lichtenstein, M. (2004). Urbanization on the US landscape: looking ahead in the 21st century. Landsc. Urban Plan. 69, 219–234. doi: 10.1016/j.landurbplan.2003.07.004
Allen, C. D., Breshears, D. D., and McDowell, N. G. (2015). On underestimation of global vulnerability to tree mortality and forest die-off from hotter drought in the Anthropocene. Ecosphere 6, 1–55. doi: 10.1890/ES15-00203.1
Allen, C. D., Macalady, A. K., Chenchouni, H., Bachelet, D., McDowell, N., Vennetier, M., et al. (2010). A global overview of drought and heat-induced tree mortality reveals emerging climate change risks for forests. For. Ecol. Manage. 259, 660–684. doi: 10.1016/j.foreco.2009.09.001
Anderegg, W. R. L., Konings, A. G., Trugman, A. T., Yu, K., Bowling, D. R., Gabbitas, R., et al. (2018). Hydraulic diversity of forests regulates ecosystem resilience during drought. Nature 561, 538–541. doi: 10.1038/s41586-018-0539-7
Antikainen, R., Dalhammar, C., Hildén, M., Judl, J., Jääskeläinen, T., Kautto, P., et al. (2017). Renewal of forest based manufacturing towards a sustainable circular bioeconomy. Reports of the Finnish Environment Institute, 13, p 119.
Asaro, C., Koch, F. J., and Potter, K. M. (2023). Denser forests across the USA experience more damage from insects and pathogens. Sci. Rep. 13:3666. doi: 10.1038/s41598-023-30675-z
Bachelet, D., Ferschweiler, K., Sheehan, T. J., Sleeter, B. M., and Zhu, Z. (2015). Projected carbon stocks in the conterminous USA with land use and variable fire regimes. Glob. Chang. Biol. 21, 4548–4560. doi: 10.1111/gcb.13048
Bala, A., Raugei, M., Benveniste, G., Gazulla, C., and Fullana-I-Palmer, P. (2010). Simplified tools for global warming potential evaluation: when “good enough” is best. Int. J. Life Cycle Assess. 15, 489–498. doi: 10.1007/s11367-010-0153-x
Barlaz, M. A. (1998). Carbon storage during biodegradation of municipal solid waste components in laboratory-scale landfills. Global Biogeochem. Cycles 12, 373–380. doi: 10.1029/98GB00350
Bechtold, W. A., and Patterson, P. L. (2005). The enhanced Forest inventory and analysis program—National Sampling Design and estimation procedures. USDA general technical report SRS-80.
Beever, E. A., O’Leary, J., Mengelt, C., West, J. M., Julius, S., Green, N., et al. (2016). Improving conservation outcomes with a new paradigm for understanding species’ Fundamental and realized adaptive capacity. Conserv. Lett. 9, 131–137. doi: 10.1111/conl.12190
Bellassen, V., and Luyssaert, S. (2014). Managing forests in uncertain times. Nature 506, 153–155. doi: 10.1038/506153a
Bergeron, Y., Vijayakumar, D. B. I. P., Ouzennou, H., Raulier, F., Leduc, A., and Gauthier, S. (2017). Projections of future forest age class structure under the influence of fire and harvesting: implications for forest management in the boreal forest of eastern Canada. Forestry 90, 485–495. doi: 10.1093/forestry/cpx022
Besnard, S., Carvalhais, N., Arain, M. A., Black, A., De Bruin, S., Buchmann, N., et al. (2018). Quantifying the effect of forest age in annual net forest carbon balance. Environ. Res. Lett. 13:124018. doi: 10.1088/1748-9326/aaeaeb
Binkley, D., Stape, J. L., Ryan, M. G., Barnard, H. R., and Fownes, J. (2002). Age-related decline in forest ecosystem growth: an individual-tree, stand-structure hypothesis. Ecosystems 5, 58–67. doi: 10.1007/s10021-001-0055-7
Birdsey, R., Pregitzer, K., and Lucier, A. (2006). Forest carbon Management in the United States. J. Environ. Qual. 35, 1461–1469. doi: 10.2134/jeq2005.0162
Bonan, G. B. (2008). Forests and climate change: Forcings, feedbacks, and the climate benefits of forests. Science 320, 1444–1449. doi: 10.1126/science.1155121
Boudewyn, P., Song, X., Magnussen, S., and Gillis, M. D. (2007). Model-based, volume-to-biomass conversion for forested and vegetated land in Canada. Edmonton, Alberta: Natural Resources Canada, Canadian Forest Service, Pacific Forestry Centre, BC-X-411.
Bowditch, E., Santopuoli, G., Binder, F., del Río, M., La Porta, N., Kluvankova, T., et al. (2020). What is climate-smart forestry? A definition from a multinational collaborative process focused on mountain regions of Europe. Ecosyst. Serv. 43:101113. doi: 10.1016/j.ecoser.2020.101113
Bradford, J. B., and D’Amato, A. W. (2012). Recognizing trade-offs in multi-objective land management. Front. Ecol. Environ. 10, 210–216. doi: 10.1890/110031
Bradford, J. B., Jensen, N. R., Domke, G. M., and D’Amato, A. W. (2013). Forest ecology and management potential increases in natural disturbance rates could offset forest management impacts on ecosystem carbon stocks. For. Ecol. Manage. 308, 178–187. doi: 10.1016/j.foreco.2013.07.042
Brando, P. M., Paolucci, L., Ummenhofer, C. C., Ordway, E. M., Hartmann, H., Cattau, M. E., et al. (2019). Droughts, wildfires, and Forest carbon cycling: a pantropical synthesis. Annu. Rev. Earth Planet. Sci. 47, 555–581. doi: 10.1146/annurev-earth-082517-010235
Brockway, D. G., and Lewis, C. E. (1997). Long-term effects of variable frequency fire on plant community diversity, structure and productivity in a longleaf pine wiregrass Flatwoods ecosystem. For. Ecol. Manage. 96, 167–183. doi: 10.1016/S0378-1127(96)03939-4
Brown, R. T., Agee, J. K., and Franklin, J. F. (2004). Forest restoration and fire: principles in the context of place. Conserv. Biol. 18, 903–912. doi: 10.1111/j.1523-1739.2004.521_1.x
Buma, B., and Wessman, C. A. (2013). Forest resilience, climate change, and opportunities for adaptation: a specific case of a general problem. For. Ecol. Manage. 306, 216–225. doi: 10.1016/j.foreco.2013.06.044
Burrill, E. A., DiTommaso, A. M., Turner, J. A., Pugh, S. A., Menlove, J., Christensen, G., et al. (2021). The Forest inventory and Analaysis database: Database description and user guide version 9.0.1 for phase 2. Available at: http://www.fia.fs.fed.us/library/database-documentation/
Butler-Leopold, P. R., Iverson, L. R., Thompson, F. R., Brandt, L. A., Handler, S. D., Janowiak, M. K., et al. (2018). Mid-Atlantic Forest ecosystem vulnerability assessment and synthesis: A report for the mid-Atlantic climate change response framework project. General Technical Report NRS-181, US Forest Service, Northern Research Station.
Catanzaro, P., and D’Amato, A. (2019). Forest carbon: An essential natural solution for climate change. University of Massachusetts, Amherst, MA: Cooperative Extension Landowner Outreach Pamphlet, 28.
Chapin, F. S., Matson, P. A., and Vitousek, P. (2011). Principles of terrestrial ecosystem ecology. 2nd Edn. New York: Springer.
Chen, H. Y. H., and Luo, Y. (2015). Net aboveground biomass declines of four major forest types with forest ageing and climate change in western Canada’s boreal forest. Glob. Chang. Biol. 21, 3675–3684. doi: 10.1111/gcb.12994
Chen, J., Ter-Mikaelian, M. T., Yang, H., and Colombo, S. J. (2018). Assessing the greenhouse gas effects of harvested wood products manufactured from managed forests in Canada. Forestry 91, 193–205. doi: 10.1093/forestry/cpx056
Churkina, G., Organschi, A., Reyer, C. P. O., Ruff, A., Vinke, K., Liu, Z., et al. (2020). Buildings as a global carbon sink. Nat Sustain 3, 269–276. doi: 10.1038/s41893-019-0462-4
Clark, J. S., Beckage, B., Camill, P., Cleveland, B., HilleRisLambers, J., Lichter, J., et al. (1999). Interpreting recruitment limitation in forests. Am. J. Bot. 86, 1–16. doi: 10.2307/2656950
Clay, K., and Cooper, L. (2022). Safeguarding against harm in a climate-smart Forest economy: definitions, challenges, and solutions. Sustainability 14:4209. doi: 10.3390/su14074209
Coomes, D. A., Holdaway, R. J., Kobe, R. K., Lines, E. R., and Allen, R. B. (2012). A general integrative framework for modelling woody biomass production and carbon sequestration rates in forests. J. Ecol. 100, 42–64. doi: 10.1111/j.1365-2745.2011.01920.x
Cooper, L., and MacFarlane, D. (2023). Climate-smart forestry: promise and risks for forests, society, and climate. PLOS Climate 2:e0000212. doi: 10.1371/journal.pclm.0000212
Coursolle, C., Margolis, H. A., Giasson, M. A., Bernier, P. Y., Amiro, B. D., Arain, M. A., et al. (2012). Influence of stand age on the magnitude and seasonality of carbon fluxes in Canadian forests. Agric. For. Meteorol. 165, 136–148. doi: 10.1016/j.agrformet.2012.06.011
Curtis, P. S., and Gough, C. M. (2018). Forest aging, disturbance and the carbon cycle. New Phytol. 219, 1188–1193. doi: 10.1111/nph.15227
D’Amato, A. W., Bradford, J. B., Fraver, S., and Palik, B. J. (2011). Forest management for mitigation and adaptation to climate change: insights from long-term silviculture experiments. For. Ecol. Manage. 262, 803–816. doi: 10.1016/j.foreco.2011.05.014
Del Genio, A. D., Yao, M. S., and Jonas, J. (2007). Will moist convection be stronger in a warmer climate? Geophys. Res. Lett. 34:16. doi: 10.1029/2007GL030525
den Elzen, M. G. J., Dafnomilis, I., Forsell, N., Fragkos, P., Fragkiadakis, K., Höhne, N., et al. (2022). Updated nationally determined contributions collectively raise ambition levels but need strengthening further to keep Paris goals within reach. Mitig. Adapt. Strat. Glob. Chang. 27:33. doi: 10.1007/s11027-022-10008-7
Dey, D. C., Knapp, B. O., Battaglia, M. A., Deal, R. L., Hart, J. L., O’Hara, K. L., et al. (2019). Barriers to natural regeneration in temperate forests across the USA. New For. 50, 11–40. doi: 10.1007/s11056-018-09694-6
Dixon, R., Brown, S., Houghton, R., Solomon, A., Trexler, M., and Wisniewski, J. (1994). Carbon pools and flux of dynamic Forest ecosystems. Science 263, 185–191.
Doelman, J. C., Stehfest, E., van Vuuren, D. P., Tabeau, A., Hof, A. F., Braakhekke, M. C., et al. (2020). Afforestation for climate change mitigation: potentials, risks and trade-offs. Glob. Chang. Biol. 26, 1576–1591. doi: 10.1111/gcb.14887
Domke, G. M., Walters, B. F., Nowak, D. J., Smith, J., Ogle, S. M., and Coulston, J. W. (2021). “Greenhouse gas emissions and removals from forest land and urban trees in the United States, 1990-2019,” in iResource update FS-307. Madison, WI: USDA Forest Service, Northern Research Station. 5 p. [plus 2 appendixes].
Dow, C., Kim, A. Y., D’Orangeville, L., Gonzalez-Akre, E. B., Helcoski, R., Herrmann, V., et al. (2022). Warm springs alter timing but not total growth of temperate deciduous trees. Nature 608, 552–557. doi: 10.1038/s41586-022-05092-3
Dugan, A. J., Birdsey, R., Healey, S. P., Pan, Y., Zhang, F., Mo, G., et al. (2017). Forest sector carbon analyses support land management planning and projects: assessing the influence of anthropogenic and natural factors. Clim. Change 144, 207–220. doi: 10.1007/s10584-017-2038-5
Dugan, A. J., Birdsey, R., Mascorro, V. S., Magnan, M., Smyth, C. E., Olguin, M., et al. (2018a). A systems approach to assess climate change mitigation options in landscapes of the United States forest sector. Carbon Balance Manag. 13:13. doi: 10.1186/s13021-018-0100-x
Dugan, A. J., Lichstein, J. W., Steele, A., Metsaranta, J. M., Bick, S., and Hollinger, D. Y. (2021). Opportunities for forest sector emissions reductions: a state-level analysis. Ecol. Appl. 31, 1–15. doi: 10.1002/eap.2327
Dugan, A. J., Steele, A., Hollinger, D., Birdsey, R., and Lichstein, J. (2018b). Assessment of Forest sector carbon stocks and mitigation potential for the state forests of Pennsylvania. A report for the Pennsylvania Department of Conservation and Natural Resources. USDA Forest Service. Available at: https://www.climatehubs.usda.gov/sites/default/files/PA_ForestCarbon_MainReport.pdf
Duveneck, M. J., and Scheller, R. M. (2015). Climate-suitable planting as a strategy for maintaining forest productivity and functional diversity. Ecol. Appl. 25, 1653–1668. doi: 10.1890/14-0738.1
Dylewski, R., and Adamczyk, J. (2013). “Life cycle assessment (LCA) of building thermal insulation materials” in Eco-efficient construction and building materials: Life cycle assessment (LCA), eco-labelling and case studies (Cambridge, UK: Woodhead Publishing Limited), 267–286.
Dymond, C. C. (2012). Forest carbon in North America: annual storage and emissions from British Columbia’s harvest, 1965-2065. Carbon Balance Manag. 7:8. doi: 10.1186/1750-0680-7-8
Ekholm, A., Lundqvist, L., Petter Axelsson, E., Egnell, G., Hjältén, J., Lundmark, T., et al. (2023). Long-term yield and biodiversity in stands managed with the selection system and the rotation forestry system: a qualitative review. For. Ecol. Manage. 537:120920. doi: 10.1016/j.foreco.2023.120920
Evans, M. R., Norris, K. J., and Benton, T. G. (2012). Predictive ecology: systems approaches. Philos. Trans. R. Soc. B 367, 163–169. doi: 10.1098/rstb.2011.0191
Fahey, T. J., Woodbury, P. B., Battles, J. J., Goodale, C. L., Hamburg, S. P., Ollinger, S. V., et al. (2010). Forest carbon storage: ecology, management, and policy. Front. Ecol. Environ. 8, 245–252. doi: 10.1890/080169
FAO (2021). FAOSTAT. License: CC BY-NC-SA 3.0 IGO FAOSTAT, Rome. Accessible at: https://www.fao.org/faostat/.
Fargione, J. E., Bassett, S., Boucher, T., Bridgham, S. D., Conant, R. T., Cook-Patton, S. C., et al. (2018). Natural climate solutions for the United States. Sci. Adv. 4, 1–15. doi: 10.1126/sciadv.aat1869
Fekedulegn, D., Mac Siurtain, M. P., and Colbert, J. J. (1999). Parameter estimation of nonlinear growth models in forestry. Silva Fennica 33, 327–336. doi: 10.14214/sf.653
FERS (2021). Ireland’s National Forestry Accounting Plan 2021–2025. Forest, Environmental Research & Services Limited. Department of Agriculture, Food and the Marine. Dublin, p 84.
Folke, C., Carpenter, S., Walker, B., Scheffer, M., Elmqvist, T., Gunderson, L., et al. (2004). Regime shifts, resilience, and biodiversity in ecosystem management. Annu. Rev. Ecol. Evol. Syst. 35, 557–581. doi: 10.1146/annurev.ecolsys.35.021103.105711
Ford, S. E., and Keeton, W. S. (2017). Enhanced carbon storage through management for old-growth characteristics in northern hardwood-conifer forests. Ecosphere 8:e01721. doi: 10.1002/ecs2.1721
Fuhr, H., Hickmann, T., and Kern, K. (2018). The role of cities in multi-level climate governance: local climate policies and the 1.5 °C target. Curr. Opin. Environ. Sustain. 30, 1–6. doi: 10.1016/j.cosust.2017.10.006
Gan, J., and McCarl, B. A. (2007). Measuring transnational leakage of forest conservation. Ecol. Econ. 64, 423–432. doi: 10.1016/j.ecolecon.2007.02.032
Geary, W. L., Bode, M., Doherty, T. S., Fulton, E. A., Nimmo, D. G., Tulloch, A. I. T., et al. (2020). A guide to ecosystem models and their environmental applications. Nat Ecol Evol 4, 1459–1471. doi: 10.1038/s41559-020-01298-8
Geng, A., Yang, H., Chen, J., and Hong, Y. (2017). Review of carbon storage function of harvested wood products and the potential of wood substitution in greenhouse gas mitigation. Forest Policy Econ. 85, 192–200. doi: 10.1016/j.forpol.2017.08.007
Gough, C. M., Vogel, C. S., Schmid, H. P., and Curtis, P. S. (2008). Controls on annual Forest carbon storage: lessons from the past and predictions for the. Bioscience 58, 609–622. doi: 10.1641/B580708
Griscom, B. W., Adams, J., Ellis, P. W., Houghton, R. A., Lomax, G., Miteva, D. A., et al. (2017). Natural climate solutions. Proc. Natl. Acad. Sci. U. S. A. 114, 11645–11650. doi: 10.1073/pnas.1710465114
Gundersen, P., Thybring, E. E., Nord-Larsen, T., Versterdal, L., Nadelhoffer, K. J., and Johannsen, V. K. (2021). Old-growth forest carbon sinks overestimated. Nature 591, E21–E23. doi: 10.1038/s41586-021-03266-z
Gustafson, E. J., Kern, C. C., Miranda, B. R., Sturtevant, B. R., Bronson, D. R., and Kabrick, J. M. (2020). Climate adaptive silviculture strategies: how do they impact growth, yield, diversity and value in forested landscapes? For. Ecol. Manage. 470–471:118208. doi: 10.1016/j.foreco.2020.118208
Guyette, R. P., Thompson, F. R., Whittier, J., Stambaugh, M. C., and Dey, D. C. (2014). Future fire probability modeling with climate change data and physical chemistry. For. Sci. 60, 862–870. doi: 10.5849/forsci.13-108
Harmon, M. E. (2001). Carbon sequestration in forests: addressing the scale question. J. For. 99, 24–29. doi: 10.1093/jof/99.4.24
Harmon, M. E. (2019). Have product substitution carbon benefits been overestimated? A sensitivity analysis of key assumptions. Environ. Res. Lett. 14:065008. doi: 10.1088/1748-9326/ab1e95
Heath, L. S., Smith, J. E., and Birdsey, R. A. (2003). “Carbon trends in U.S. forestlands: A context for the role of soils in forest carbon sequestration,” in The potential of U.S. forest soils to sequester carbon and mitigate the greenhouse effect. eds. J. M. Kimble, L. S. Heath, R. A. Birdsey, and R. Lal (CRC Press), 35–45.
Hildebrandt, J., Hagemann, N., and Thrän, D. (2017). The contribution of wood-based construction materials for leveraging a low carbon building sector in europe. Sustain. Cities Soc. 34, 405–418. doi: 10.1016/j.scs.2017.06.013
Hill, A. P., Nolan, C. J., Hemes, K. S., Cambron, T. W., and Field, C. B. (2023). Low-elevation conifers in California’s Sierra Nevada are out of equilibrium with climate. PNAS Nexus 2, 1–9. doi: 10.1093/pnasnexus/pgad004
Howard, J. L., and Liang, S. (2019). U.S. timber production, trade, consumption, and Price statistics, 1965–2017 (Research Paper FPL–RP–701). Available at: http://www.ascr.usda.
Howard, J. L., McKeever, D. B., and Liang, S. (2017). U.S. Forest products annual market review and prospects, 2013–2017. In U.S. Forest products annual market review and prospects (Research Note; FPL–RN–0348). Available at: http://www.ascr.usda/gov/complaint_filing_cust.html
Hubbard, S. S., Bergman, R. D., Sahoo, K., and Bowe, S. A. (2020). A life cycle assessment of hardwood lumber production in the northeast and north Central United States. CORRIM Report. 45 p.
Hudiburg, T. W., Law, B. E., Moomaw, W. R., Harmon, M. E., and Stenzel, J. E. (2019). Meeting GHG reduction targets requires accounting for all forest sector emissions. Environ. Res. Lett. 14:095005. doi: 10.1088/1748-9326/ab28bb
Huntington, H. G., Barrios, J. J., and Arora, V. (2019). Review of key international demand elasticities for major industrializing economies. Energy Policy 133:110878. doi: 10.1016/j.enpol.2019.110878
IPCC (2021). “Summary for policy makers” in Climate change 2021: The physical science basis. Contribution of working group 1 to the sixth assessment report of the intergovernmental panel on climate change. eds. V. Masson-Delmotte, P. Zhai, A. Pirani, S. L. Connors, C. Pean, and S. Berger, et al. (Cambridge, UK, and New York, NY, USA: Cambridge University Press), 42.
Jolly, W. M., Cochrane, M. A., Freeborn, P. H., Holden, Z. A., Brown, T. J., Williamson, G. J., et al. (2015). Climate-induced variations in global wildfire danger from 1979 to 2013. Nat. Commun. 6:8537. doi: 10.1038/ncomms8537
Jurgensen, C., Kollert, W., and Lebedys, A. (2014). Assessment of industrial Roundwood production from planted forests (FP/48/E; Issue Planted Forests and trees Working Paper Series No. 48).
Kaarakka, L., Cornett, M., Domke, G., Ontl, T., and Dee, L. E. (2021). Improved forest management as a natural climate solution: a review. Ecol Solut Evid 2, 1–10. doi: 10.1002/2688-8319.12090
Keefe, K., Alavalapati, J. A. A., and Pinheiro, C. (2012). Is enrichment planting worth its costs? A financial cost-benefit analysis. Forest Policy Econ. 23, 10–16. doi: 10.1016/j.forpol.2012.07.004
Köhl, M., Ehrhart, H. P., Knauf, M., and Neupane, P. R. (2020). A viable indicator approach for assessing sustainable forest management in terms of carbon emissions and removals. Ecol. Indic. 111:106057. doi: 10.1016/j.ecolind.2019.106057
Köhl, M., Hildebrandt, R., Olschofksy, K., Köhler, R., Rötzer, T., Mette, T., et al. (2010). Combating the effects of climatic change on forests by mitigation strategies. Carbon Balance Manag. 5:8. doi: 10.1186/1750-0680-5-8
Kull, S. J., Rampley, G. J., Morken, S., Metsaranta, J., Neilson, E. T., and Kurz, W. A. (2019). Operational-scale carbon budget model of the Canadian Forest sector (CBM-CFS3) version 1.2: User’s guide. Natural Resources Canada, Canadian Forest Service, Northern Forestry Centre. Available at: http://cfs.nrcan.gc.ca/publications?id=36556
Kurz, W. A., and Apps, M. J. (2006). Developing Canada’s national forest carbon monitoring, accounting and reporting system to meet the reporting requirements of the Kyoto protocol. Mitig. Adapt. Strat. Glob. Chang. 11, 33–43. doi: 10.1007/s11027-006-1006-6
Kurz, W. A., Birdsey, R. A., Mascorro, V. S., Greenberg, D., Dai, Z., Olguín, M., et al. (2016). Integrated modeling and assessment of north American Forest carbon dynamics technical report: tools for monitoring, reporting and projecting forest greenhouse gas emissions and removals. (Summary Report) Montreal, Canada: Commission for Environmental Cooperation.
Kurz, W. A., Dymond, C. C., White, T. M., Stinson, G., Shaw, C. H., Rampley, G. J., et al. (2009). CBM-CFS3: a model of carbon-dynamics in forestry and land-use change implementing IPCC standards. Ecol. Model. 220, 480–504. doi: 10.1016/j.ecolmodel.2008.10.018
Kurz, W. A., Hayne, S., Fellows, M., Macdonald, J. D., Metsaranta, J. M., Hafer, M., et al. (2018). Quantifying the impacts of human activities on reported greenhouse gas emissions and removals in Canada’s managed forest: conceptual framework and implementation. Can. J. For. Res. 48, 1227–1240. doi: 10.1139/cjfr-2018-0176
Kurz, W. A., Shaw, C. H., Boisvenue, C., Stinson, G., Metsaranta, J., Leckie, D., et al. (2013). Carbon in Canada’s boreal forest — a synthesis. Environ. Rev. 21, 260–292. doi: 10.1139/er-2013-0041
Kurz, W. A., Stinson, G., and Rampley, G. (2008). Could increased boreal forest ecosystem productivity offset carbon losses from increased disturbances? Philosoph Trans R Soc B Biol Sci 363, 2261–2269. doi: 10.1098/rstb.2007.2198
Lafond, V., Lagarrigues, G., Cordonnier, T., and Courbaud, B. (2014). Uneven-aged management options to promote forest resilience for climate change adaptation: effects of group selection and harvesting intensity. Ann. For. Sci. 71, 173–186. doi: 10.1007/s13595-013-0291-y
Leskinen, P., Cardellini, G., González-García, S., Hurmekoski, E., Sathre, R., Seppälä, J., et al. (2018). “Substitution effects of wood-based products in climate change mitigation” in From Science to Policy 7 (European Forest Institute), 28.
Lesser, M. R., Dovciak, M., Wheat, R., Curtis, P., Smallidge, P., Hurst, J., et al. (2019). Modelling white-tailed deer impacts on forest regeneration to inform deer management options at landscape scales. For. Ecol. Manage. 448, 395–408. doi: 10.1016/j.foreco.2019.06.013
Lin, J., Gamarra, J. G. P., Drake, J. E., Cuchietti, A., and Yanai, R. D. (2023). Scaling up uncertainties in allometric models: how to see the forest, not the trees. For. Ecol. Manage. 537:120943. doi: 10.1016/j.foreco.2023.120943
Lippke, B., Gustafson, R., Venditti, R., Steele, P., Volk, T. A., Oneil, E., et al. (2012). Comparing life-cycle carbon and energy impacts for biofuel, wood product, and forest management alternatives. For. Prod. J. 62, 247–257. doi: 10.13073/FPJ-D-12-00017.1
Lippke, B., Oneil, E., Harrison, R., Skog, K., Gustavsson, L., and Sathre, R. (2011). Life cycle impacts of forest management and wood utilization on carbon mitigation: knowns and unknowns. Carbon Manage 2, 303–333. doi: 10.4155/cmt.11.24
Littlefield, C. E., and D’Amato, A. W. (2022). Identifying trade-offs and opportunities for forest carbon and wildlife using a climate change adaptation lens. Conserv Sci Pract 4:12631. doi: 10.1111/csp2.12631
Lucash, M. S., Scheller, R. M., Gustafson, E. J., and Sturtevant, B. R. (2017). Spatial resilience of forested landscapes under climate change and management. Landsc. Ecol. 32, 953–969. doi: 10.1007/s10980-017-0501-3
Lucash, M. S., Scheller, R. M., Sturtevant, B. R., Gustafson, E. J., Kretchum, A. M., and Foster, J. R. (2018). More than the sum of its parts: how disturbance interactions shape forest dynamics under climate change. Ecosphere 9:2293. doi: 10.1002/ecs2.2293
Malmsheimer, R. W., Heffernan, P., Brink, S., Crandall, D., Deneke, F., Galik, C., et al. (2008). Forest management solutions for mitigating climate change in the United States. J For. 106, 115–171. doi: 10.1093/jof/106.3.115
Matala, J., Ojansuu, R., Peltola, H., Sievanen, R., and Kellomaki, S. (2005). Introducing effects of temperature and CO2 elevation on tree growth into a statistical growth and yield model. Ecol. Model. 181, 173–190. doi: 10.1016/j.ecolmodel.2004.06.030
McGarvey, J. C., Thompson, J. R., Epstein, H. E., and Shugart, H. H. (2015). Carbon storage in old-growth forests of the mid-Atlantic: toward better understanding the eastern forest carbon sink. Ecology 96, 311–317. doi: 10.1890/14-1154.1
McKinley, D. C., Ryan, M. G., Birdsey, R. A., Giardina, C. P., Harmon, M. E., Heath, L. S., et al. (2011). A synthesis of current knowledge on forests and carbon storage in the United States. Ecol. Appl. 21, 1902–1924. doi: 10.1890/10-0697.1
MDE (2021). The greenhouse gas emissions reduction act: 2030 GGRA plan. Maryland Department of the Environment. Annapolis, MD. Available at: https://mde.maryland.gov/programs/Air/ClimateChange/Pages/Greenhouse-Gas-Emissions-Reduction-Act-(GGRA)-Plan.aspx
Meil, J., and Bushi, L. (2013). Life cycle assessment of MBMA primary and secondary structural steel and wall and roof panel products: Final report. The Athena Sustainable Materials Institute. Available at: http://www.athenasmi.org/wp-content/uploads/2016/09/MBMA_FInal_Report_April_2013_with_addenda.pdf
Menton, M., Larrea, C., Latorre, S., Martinez-Alier, J., Peck, M., Temper, L., et al. (2020). Environmental justice and the SDGs: from synergies to gaps and contradictions. Sustain. Sci. 15, 1621–1636. doi: 10.1007/s11625-020-00789-8
Metsaranta, J. M., Shaw, C. H., Kurz, W. A., Boisvenue, C., and Morken, S. (2017). Uncertainty of inventory-based estimates of the carbon dynamics of Canada’s managed forest (1990–2014). Can. J. For. Res. 47, 1082–1094. doi: 10.1139/cjfr-2017-0088
Millar, C. I., Stephenson, N. L., and Stephens, S. L. (2007). Climate change and forests of the future: managing in the face of uncertainty. Ecol. Appl. 17, 2145–2151. doi: 10.1890/06-1715.1
Millers, I., Shriner, D. S., and Rizzo, D. (1989). History of hardwood decline in the eastern United States. GTR NE-126. Broomall, PA: USDA Forest Service, Northeastern Research Station. 75.
Mohammad, N. A. B. (2023). “Concern on Wood waste utilization: environment and economic evaluation” in Wood waste management and products. eds. S. N. Sarmin, M. Jawaid, and R. Elias (Singapore: Springer Nature Singapore Pte Ltd.), 23–32.
Moreau, L., Thiffault, E., Kurz, W. A., and Beauregard, R. (2023). Under what circumstances can the forest sector contribute to 2050 climate change mitigation targets? A study from forest ecosystems to landfill methane emissions for the province of Quebec, Canada. GCB Bioenergy 15, 1119–1139. doi: 10.1111/gcbb.13081
Murray, B. C., Mccarl, B. A., and Lee, H.-C. (2004). Estimating Leakage from Forest carbon sequestration programs. Land Econ. 80, 109–124. doi: 10.2307/3147147
Myllyviita, T., Soimakallio, S., Judl, J., and Seppälä, J. (2021). Wood substitution potential in greenhouse gas emission reduction–review on current state and application of displacement factors. For Ecosyst 8:42. doi: 10.1186/s40663-021-00326-8
Nabuurs, G. J., Masera, O., Andrasko, K., Benitez-Ponce, P., Boer, R., Dutschke, M., et al. (2007). “Forestry” in Climate change 2007: Mitigation. Contribution of working group III to the fourth assessment report of the intergovernmental panel on climate change. eds. B. Metz, O. R. Davidson, P. R. Bosch, R. Dave, and L. A. Meyer (Cambridge, UK, and New York, NY, USA: Cambridge University Press), 389–394.
Nabuurs, G.-J., Verkerk, P. J., Schelhaas, M.-J., González Olabarria, J. R., Trasobares, A., and Cienciala, E. (2018). Climate-smart forestry: Mitigation impacts in three European regions. From Science to Policy 6. Available at: https://efi.int/sites/default/files/files/publication-bank/2018/efi_fstp_6_2018.pdf
Nevins, M. T., D’Amato, A. W., and Foster, J. R. (2021). Future forest composition under a changing climate and adaptive forest management in southeastern Vermont, USA. For. Ecol. Manage. 479:118527. doi: 10.1016/j.foreco.2020.118527
Nicotra, A. B., Beever, E. A., Robertson, A. L., Hofmann, G. E., and O’Leary, J. (2015). Assessing the components of adaptive capacity to improve conservation and management efforts under global change. Conserv. Biol. 29, 1268–1278. doi: 10.1111/cobi.12522
Novick, K. A., Metzger, S., Annderegg, W. R. L., Barnes, M., Cala, D. S., Guan, K., et al. (2022). Informing nature-based climate solutions for the United States with the best-available science. Glob. Chang. Biol. 28, 3778–3794. doi: 10.1111/gcb.16156
Odum, E. P. (1969). The strategy of ecosystem development: an understanding of ecological succession provides a basis for resolving man’s conflict with nature. Science 164, 262–270. doi: 10.1126/science.164.3877.262
Olguin, M., Wayson, C., Fellows, M., Birdsey, R., Smyth, C. E., Magnan, M., et al. (2018). Applying a systems approach to assess carbon emission reductions from climate change mitigation in Mexico’s forest sector. Environ. Res. Lett. 13:aaaa03. doi: 10.1088/1748-9326/aaaa03
Ontl, T. A., Janowiak, M. K., Swanston, C. W., Daley, J., Handler, S., Cornett, M., et al. (2020). Forest Management for Carbon Sequestration and Climate Adaptation. J. For. 86–101:62. doi: 10.1093/jofore/fvz062
Oswalt, S. N., Smith, W. B., Miles, P. D., and Pugh, S. A. (2019). Forest resources of the United States, 2017: A technical document supporting the Forest Service 2020 RPA assessment. GTR WO-97, USDA Forest Service, Southern Research Station.
Otto, J. S. (1989). Forest fallowing in the southern Appalachian Mountains: a problem in comparative agricultural history. Proc. Am. Philos. Soc. 133, 51–63.
PDEP (2021). Pennsylvania climate action plan 2021: Strategies for government, business, agriculture, and community leaders—and all Pennsylvanians. Pennsylvania Department of Environment Protection. Harrisburg, PA. Available at: www.dep.pa.gov/climate
Peichl, M., Arain, M. A., and Brodeur, J. J. (2010). Agricultural and Forest meteorology age effects on carbon fluxes in temperate pine forests. Agric. For. Meteorol. 150, 1090–1101. doi: 10.1016/j.agrformet.2010.04.008
Peichl, M., Martínez-García, E., Fransson, J. E. S., Wallerman, J., Laudon, H., Lundmark, T., et al. (2022). Landscape-variability of the carbon balance across managed boreal forests. Glob. Chang. Biol. 29, 1119–1132. doi: 10.1111/gcb.16534
Petersson, H., Ellison, D., Mensah, A. P., Berndes, G., Egnell, G., Lundblad, M., et al. (2022). On the role of forests and the forest sector for climate change mitigation in Sweden. GCB-Bioenergy 14, 793–813. doi: 10.1111/gcbb.12943
Pilli, R., Alkama, R., Cescatti, A., Kurz, W. A., and Grassi, G. (2022). The European forest carbon budget under future climate conditions and current management practices. Biogeosciences 13, 3263–3284. doi: 10.5194/bg-19-3263-2022
Pilli, R., Grassi, G., Kurz, W. A., Fiorese, G., and Cescatti, A. (2017). The European forest sector: past and future carbon budget and fluxes under different management scenarios. Biogeosciences 14, 2387–2405. doi: 10.5194/bg-14-2387-2017
Pilli, R., Grassi, G., Kurz, W. A., Moris, J. V., and Viñas, R. A. (2016). Modelling forest carbon stock changes as affected by harvest and natural disturbances. II. EU-level analysis. Carbon Balance Manag. 11:4. doi: 10.1186/s13021-016-0059-4
Pilli, R., Grassi, G., Kurz, W. A., Smyth, C. E., and Blujdea, V. (2013). Application of the CBM-CFS3 model to estimate Italy’s forest carbon budget, 1995-2020. Ecol. Model. 266, 144–171. doi: 10.1016/j.ecolmodel.2013.07.007
Pingoud, K., Ekholm, T., Sievänen, R., Huuskonen, S., and Hynynen, J. (2018). Trade-offs between forest carbon stocks and harvests in a steady state – a multi-criteria analysis. J. Environ. Manage. 210, 96–103. doi: 10.1016/j.jenvman.2017.12.076
Pingoud, K., Skog, K. E., Martino, D. L., Tonosaki, M., and Xiaoquan, Z. (2006). Chapter 12: Harvested Wood products (Vol. 4). Available at: https://www.ipcc-nggip.iges.or.jp/public/2006gl/pdf/4_Volume4/V4_12_Ch12_HWP.pdf
Pirard, R., Dal, L., and Warman, R. (2016). Do timber plantations contribute to forest conservation? Environ. Sci. Policy 57, 122–130. doi: 10.1016/j.envsci.2015.12.010
Pohjola, J., Laturi, J., Lintunen, J., and Uusivuori, J. (2018). Immediate and long-run impacts of a forest carbon policy – a market-level assessment with heterogeneous forest owners. J. For. Econ. 32, 94–105. doi: 10.1016/j.jfe.2018.03.001
Powers, M., Kolka, R., Palik, B., McDonald, R., and Jurgensen, M. (2011). Long-term management impacts on carbon storage in Lake states forests. For. Ecol. Manage. 262, 424–431. doi: 10.1016/j.foreco.2011.04.008
Puddister, D., Dominy, S. W. J., Baker, J. A., Morris, D. M., Maure, J., Rice, J. A., et al. (2011). Opportunities and challenges for Ontario’s forest bioeconomy. For. Chron. 87:45. doi: 10.5558/tfc2011-045
Puettmann, M. (2020). CORRIM report: Life cycle assessment for the production of northeast-northcentral softwood lumber. Available at: https://corrim.org/wp-content/uploads/2020/06/CORRIM-AWC-NENC-Lumber.pdf
Puettmann, M., Kaestner, D., and Taylor, A. (2016). CORRIM REPORT: Life cycle assessment of oriented Strandboard (OSB) production.” Available at: https://corrim.org/wp-content/uploads/2020/12/CORRIM-AWC-OSB-Final.pdf
Puettmann, M., and Salazar, J. (2018). Cradle to gate life cycle assessment of north American particleboard production. Available at: https://corrim.org/wp-content/uploads/2019/03/LCA-Particleboard.pdf
Puettmann, M., and Salazar, J. (2019). Cradle to gate life cycle assessment of north American medium density fiberboard production. Available at: https://corrim.org/wp-content/uploads/2019/06/LCA-MDF-20190314.pdf
Pugh, S. A., Turner, J. A., Burrill, E. A., and David, W. (2018). The Forest inventory and analysis database: Population estimation user guide (edition: November, 2018). USDA Forest Service. 166 p. [Online]. Available at web address: http://www.fia.fs.fed.us/library/database-documentation.
R Core Team (2020). R: A language and environment for statistical computing. Vienna, Austria: R Foundation for Statistical Computing.
Roberge, J. M., Laudon, H., Bjorkman, C., Ranius, T., Sandstrom, C., Felton, A., et al. (2016). Socio-ecological implications of modifying rotation lengths in forestry. Ambio 45, 109–123. doi: 10.1007/s13280-015-0747-4
Rockstrom, J., Steffen, W., Noone, K., Persson, A., Chapin, F. S., Lambin, E. F., et al. (2009). A safe operating space for humanity. Nature 461, 472–475. doi: 10.1038/461472a
Rogers, N. S., D’Amato, A. W., Kern, C. C., and Bèdard, S. (2022). Northern hardwood silviculture at a crossroads: sustaining a valuable resource under future change. For. Ecol. Manage. 512:120139. doi: 10.1016/j.foreco.2022.120139
Rogers, B. M., Jantz, P., and Goetz, S. J. (2017). Vulnerability of eastern US tree species to climate change. Glob. Chang. Biol. 23, 3302–3320. doi: 10.1111/gcb.13585
Ryan, M. G., Binkley, D., and Fownes, J. H. (1997). Age-related decline in Forst productivity: pattern and process. Adv. Ecol. Res. 27, 263–266. doi: 10.1016/s0065-2504(08)60010-0
Ryan, M. G., Harmon, M. E., Birdsey, R. A., Giardina, C. P., Heath, L. S., Houghton, R. A., et al. (2010). “A synthesis of the science on forests and carbon for U.S. forests” in Issues in Ecology
Sass, E. M., Butler, B. J., and Markowski-Lindsay, M. (2020). Distribution of Forest ownerships across the conterminous United States, 2017. Res. Map NRS-11. [Scale 1: 10000000, 1: 80000000]
Schulze, E. D., Bouriaud, O., Irslinger, R., and Valentini, R. (2022). The role of wood harvest from sustainably managed forests in the carbon cycle. Ann. For. Sci. 79:17. doi: 10.1186/s13595-022-01127-x
Seddon, N., Chausson, A., Berry, P., Girardin, C. A. J., Smith, A., and Turner, B. (2020). Understanding the value and limits of nature-based solutions to climate change and other global challenges. Philos. Trans. R. Soc. B 375:120. doi: 10.1098/rstb.2019.0120
Seidl, R., Rammer, W., and Spies, T. A. (2014). Disturbance legacies increase the resilience of forest ecosystem structure, composition, and functioning. Ecol. Appl. 24, 2063–2077. doi: 10.1890/14-0255.1
Seidl, R., Spies, T. A., Peterson, D. L., Stephens, S. L., and Hicke, J. A. (2016). Searching for resilience: addressing the impacts of changing disturbance regimes on forest ecosystem services. J. Appl. Ecol. 53, 120–129. doi: 10.1111/1365-2664.12511
Seidl, R., Thom, D., Kautz, M., Martin-Benito, D., Peltoniemi, M., Vacchiano, G., et al. (2017). Forest disturbances under climate change. Nat. Clim. Chang. 7, 395–402. doi: 10.1038/nclimate3303
Shahbaz, M., AlNouss, A., Mckay, G., Mackey, H., Elkhalifa, S., and Al-Ansari, T. (2021). A comprehensive review of biomass based thermochemical conversion technologies integrated with CO2 capture and utilization within BECCS networks. Resour. Conserv. Recycl. 174:105734. doi: 10.1016/j.resconrec.2021.105734
Shaw, C. H., Hilger, A. B., Metsaranta, J., Kurz, W. A., Russo, G., Eichel, F., et al. (2014). Evaluation of simulated estimates of forest ecosystem carbon stocks using ground plot data from Canada’s National Forest Inventory. Ecol. Model. 272, 323–347. doi: 10.1016/j.ecolmodel.2013.10.005
Shinneman, D. J., Palik, B. J., and Cornett, M. W. (2012). Can landscape-level ecological restoration influence fire risk? A spatially-explicit assessment of a northern temperate-southern boreal forest landscape. For. Ecol. Manage. 274, 126–135. doi: 10.1016/j.foreco.2012.02.030
Silver, E. J., D’Amato, A. W., Fraver, S., Palik, B. J., and Bradford, J. B. (2013). Structure and development of old-growth, unmanaged second-growth, and extended rotation Pinus resinosa forests in Minnesota, USA. For. Ecol. Manage. 291, 110–118. doi: 10.1016/j.foreco.2012.11.033
Sjolie, H. K., Latta, G. S., and Solberg, B. (2013). Potentials and costs of climate change mitigation in the Norwegian forest sector – does choice of policy matter? Can. J. For. Res. 43:457. doi: 10.1139/cjfr-2012-0457
Skog, K. E. (2008). Sequestration of carbon in harvested wood products for the United States. For. Prod. J. 58, 56–72.
Smith, P., Bustamante, M., Ahammad, H., Clark, H., Dong, H., Elsiddig, E. A., et al. (2014). “Agriculture, forestry and other land use (AFOLU)” in Climate change 2014 mitigation of climate change. Contribution of working group III to the fifth assessment report of the intergovernmental panel on climate change. eds. O. Edenhofer, R. Pichs-Madruga, Y. Sokona, E. Farahani, S. Kander, and K. Seyboth, et al. (Cambridge, United Kingdom and New York, NY, USA: Cambridge University Press), 811–922.
Smith, J. E., Heath, L. S., Skog, K. E., and Birdsey, R. A. (2006). Methods for calculating Forest ecosystem and harvested carbon with standard estimates for Forest types of the United States. GTR NE-343. Newtown Square, PA: USDA Forest Service, Northeastern Research Station. Available at: https://www.fs.usda.gov/treesearch/pubs/22954
Smyth, C., Rampley, G., Lemprière, T. C., Schwab, O., and Kurz, W. A. (2017). Estimating product and energy substitution benefits in national-scale mitigation analyses for Canada. GCB Bioenergy 9, 1071–1084. doi: 10.1111/gcbb.12389
Smyth, C. E., Stinson, G., Neilson, E., Lemprière, T. C., Hafer, M., Rampley, G. J., et al. (2014). Quantifying the biophysical climate change mitigation potential of Canada’s forest sector. Biogeosciences 11, 3515–3529. doi: 10.5194/bg-11-3515-2014
Sohngen, B., and Brown, S. (2011). Extending timber rotations: carbon and cost implications. Clim. Pol. 8, 435–351. doi: 10.3763/cpol.2007.0396
Sohngen, B., Mendelsohn, R., and Sedjo, R. (1999). Forest management, conservation, and global timber markets. Americ. J. Agr. Econ 81, 1–13. doi: 10.2307/1244446
Soimakallio, S., Böttcher, H., Niemi, J., Mosley, F., Turunen, S., Hennenberg, K. J., et al. (2022). Closing an open balance: the impact of increased tree harvest on forest carbon. GCB Bioenergy 14, 989–1000. doi: 10.1111/gcbb.12981
Soimakallio, S., Saikku, L., Valsta, L., and Pingoud, K. (2016). Climate change mitigation challenge for Wood utilization-the case of Finland. Environ. Sci. Technol. 50, 5127–5134. doi: 10.1021/acs.est.6b00122
Stanke, H., Finley, A. O., Weed, A. S., Walters, B. F., and Domke, G. M. (2020). rFIA: an R package for estimation of forest attributes with the US Forest inventory and analysis database. Environ Model Softw. 127:104664. doi: 10.1016/j.envsoft.2020.104664
Stephenson, N. L., Das, A. J., Condit, R., Russo, S. E., Baker, P. J., Beckman, N. G., et al. (2014). Rate of tree carbon accumulation increases continuously with tree size. Nature 507, 90–93. doi: 10.1038/nature12914
Swann, A. L. S., Fung, I. Y., and Chiang, J. C. H. (2012). Mid-latitude afforestation shifts general circulation and tropical precipitation. PNAS 109, 712–716. doi: 10.1073/pnas.1116706108/-/DCSupplemental
Tingley, M. W., Stillman, A. N., Wilkerson, R. L., Howell, C. A., Sawyer, S. C., and Siegel, R. B. (2018). Cross-scale occupancy dynamics of a postfire specialist in response to variation across a fire regime. J. Anim. Ecol. 87, 1484–1496. doi: 10.1111/1365-2656.12851
UNFCCC (2021). The United States of America - nationally determined contributions: Reducing greenhouse gases in the United States: A 2030 target. Bonn, Germany: United Nations Framework Convention on Climate Change.
US Department of Transportation, Bureau of Transportation Statistics, US Department of Commerce, and US Census Bureau (2020). 2017 commodity flow survey (CFS) public use file (PUF) data users guide (Technical Documentation). Available at: https://www2.census.gov/programs-surveys/cfs/datasets/2017/cfs_2017_puf_users_guide.pdf
US International Trade Commission (2021). US International Trade Commission Trade & Tariff Database. Available at: https://dataweb.usitc.gov/tariff
USDA Forest Service (2019). Forest inventory and analysis program. Available at: https://www.fia.fs.usda.gov/
USDA Forest Service (2020). U.S. insects and diseases survey. Available at: https://www.fs.fed.us/foresthealth/applied-sciences/mapping-reporting/detection-surveys.shtml
USDA Forest Service (2021). Timber product output studies. Available at: https://www.fia.fs.usda.gov/program-features/tpo/
USGS (2016). LANDFIRE: Historical disturbance (HDist). U.S. Geological Survey data release. Available at: https://landfire.gov/hdist.php (Accessed November, 2020).
USGS (2018). U.S. Geological Survey (USGS) GAP analysis project (GAP), 2018, protected areas database of the United States (PAD-US). U.S. Geological Survey data release.
Verkerk, P. J., Costanza, R., Hetemäki, L., Kubiszewski, I., Leskinen, P., Nabuurs, G. J., et al. (2020). Climate-smart forestry: the missing link. Forest Policy Econ. 115:102164. doi: 10.1016/j.forpol.2020.102164
Vestin, P., Mölder, M., Kljun, N., and Cai, Z. (2020). Impacts of clear-cutting of a boreal Forest on carbon dioxide, methane and nitrous oxide fluxes. Forests 11, 1–28. doi: 10.3390/f11090961
Walker, X. J., Baltzer, J. L., Cumming, S. G., Day, N. J., Ebert, C., Goetz, S., et al. (2019). Increasing wildfires threaten historic carbon sink of boreal forest soils. Nature 572, 520–523. doi: 10.1038/s41586-019-1474-y
Wang, W. J., He, H. S., Thompson, F. R. III, Fraser, J. S., and Dijak, W. D. (2017). Changes in forest biomass and tree species distribution under climate change in the northeastern United States. Landsc. Ecol. 32, 1399–1413. doi: 10.1007/s10980-016-0429-z
Watson, J. E. M., Evans, T., Venter, O., Williams, B., Tulloch, A., Stewart, C., et al. (2018). The exceptional value of intact forest ecosystems. Nat Ecol Evol 2, 599–610. doi: 10.1038/s41559-018-0490-x
Wear, D. N., and Coulston, J. W. (2015). From sink to source: regional variation in U.S. forest carbon futures. Sci. Rep. 5:16518. doi: 10.1038/srep16518
Wear, D. N., and Murray, B. C. (2004). Federal timber restrictions, interregional spillovers, and the impact on US softwood markets. J. Environ. Econ. Manag. 47, 307–330. doi: 10.1016/S0095-0696(03)00081-0
Wickham, J., Stehman, S. V., Sorenson, D. G., Gass, L., and Dewitz, J. A. (2021). Thematic accuracy assessment of the NLCD 2016 land cover for the conterminous United States. Remote Sens. Environ. 257:112357. doi: 10.1016/j.rse.2021.112357
Williams, C. A., Gu, H., Maclean, R., Masek, J. G., and Collatz, G. J. (2016). Disturbance and the carbon balance of US forests: a quantitative review of impacts from harvests, fires, insects, and droughts. Global Planet. Change 143, 66–80. doi: 10.1016/j.gloplacha.2016.06.002
Woodall, C. W., Walters, B. F., Coulston, J. W., D’Amato, A. W., Domke, G. M., Russell, M. B., et al. (2015). Monitoring network confirms land use change is a substantial component of the Forest carbon sink in the eastern United States. Sci. Rep. 5, 1–9. doi: 10.1038/srep17028
Xie, S. H., Kurz, W. A., and McFarlane, P. N. (2023). Substitution benefits of British Columbia’s mitigation strategies in the bioeconomy. Mitig. Adapt. Strat. Glob. Chang. 28:8. doi: 10.1007/s11027-023-10055-8
Yuan, Z., Ali, A., Jucker, T., Ruiz-Benito, P., Wang, S., Jiang, L., et al. (2019). Multiple abiotic and biotic pathways shape biomass demographic processes in temperate forests. Ecology 100:e02650. doi: 10.1002/ecy.2650
Zhang, X., Chen, J., Dias, A. C., and Yang, H. (2020). Improving carbon stock estimates for in-use harvested Wood products by linking production and consumption—a global case study. Environ. Sci. Technol. 54, 2565–2574. doi: 10.1021/acs.est.9b05721
Zhao, J., Daigneault, A., and Weiskittel, A. (2022). Estimating regional timber supply and forest carbon sequestration under shared socioeconomic pathways: a case study of Maine, USA. PLOS Climate 1, 1–20. doi: 10.1371/journal.pclm.0000018
Keywords: climate change mitigation, carbon cycling, forest carbon, climate-smart forestry, harvested wood products, greenhouse gas emissions, CBM-CFS3
Citation: Papa CC, DeLyser K, Clay K, Gadoth-Goodman D, Cooper L, Kurz WA, Magnan M and Ontl T (2023) Modeling climate-smart forest management and wood use for climate mitigation potential in Maryland and Pennsylvania. Front. For. Glob. Change. 6:1259010. doi: 10.3389/ffgc.2023.1259010
Edited by:
Mohammad Ibrahim Khalil, University College Dublin, IrelandReviewed by:
David Ellison, University of Bern, SwitzerlandEmil Cienciala, Institute of Forest Ecosystem Research, Czechia
Copyright © 2023 Papa, DeLyser, Clay, Gadoth-Goodman, Cooper, Kurz, Magnan and Ontl. This is an open-access article distributed under the terms of the Creative Commons Attribution License (CC BY). The use, distribution or reproduction in other forums is permitted, provided the original author(s) and the copyright owner(s) are credited and that the original publication in this journal is cited, in accordance with accepted academic practice. No use, distribution or reproduction is permitted which does not comply with these terms.
*Correspondence: Chad C. Papa, cGFwYWNoYWRAbXN1LmVkdQ==; Kendall DeLyser, a2RlbHlzZXJAYW1lcmljYW5mb3Jlc3RzLm9yZw==