Integrated Analysis of Human Milk Microbiota With Oligosaccharides and Fatty Acids in the CHILD Cohort
- 1Department of Medical Microbiology and Infectious Diseases, University of Manitoba, Winnipeg, MB, Canada
- 2Children's Hospital Research Institute of Manitoba, Winnipeg, MB, Canada
- 3Developmental Origins of Chronic Diseases in Children Network (DEVOTION), Winnipeg, MB, Canada
- 4Digestive Oncology Research Center, Digestive Disease Research Institute, Tehran University of Medical Sciences, Tehran, Iran
- 5Department of Pediatrics and Child Health, University of Manitoba, Winnipeg, MB, Canada
- 6Department of Pediatrics and Larson-Rosenquist Foundation Mother-Milk-Infant Center of Research Excellence, University of California, San Diego, San Diego, CA, United States
- 7Department of Biomedical and Molecular Sciences, Queen's University, Kingston, ON, Canada
- 8School of Computing, Queen's University, Kingston, ON, Canada
- 9Department of Pediatrics, University of Alberta, Edmonton, AB, Canada
- 10Department of Pediatrics, University of British Columbia, Vancouver, BC, Canada
- 11Division of Respiratory Medicine, Department of Pediatrics, Hospital for Sick Children, University of Toronto, Toronto, ON, Canada
- 12Department of Medicine, McMaster University, Hamilton, ON, Canada
- 13Department of Physiology, University of Toronto, Toronto, ON, Canada
- 14Department of Agricultural Food, and Nutritional Science, University of Alberta, Edmonton, AB, Canada
- 15Department of Animal Science, University of Manitoba, Winnipeg, MB, Canada
Background: Human milk contains many bioactive components that are typically studied in isolation, including bacteria. We performed an integrated analysis of human milk oligosaccharides and fatty acids to explore their associations with milk microbiota.
Methods: We studied a sub-sample of 393 mothers in the CHILD birth cohort. Milk was collected at 3–4 months postpartum. Microbiota was analyzed by 16S rRNA gene V4 sequencing. Oligosaccharides and fatty acids were analyzed by rapid high-throughput high performance and gas liquid chromatography, respectively. Dimension reduction was performed with principal component analysis for oligosaccharides and fatty acids. Center log-ratio transformation was applied to all three components. Associations between components were assessed using Spearman rank correlation, network visualization, multivariable linear regression, redundancy analysis, and structural equation modeling. P-values were adjusted for multiple comparisons. Key covariates were considered, including fucosyltransferase-2 (FUT2) secretor status of mother and infant, method of feeding (direct breastfeeding or pumped breast milk), and maternal fish oil supplement use.
Results: Overall, correlations were strongest between milk components of the same type. For example, FUT2-dependent HMOs were positively correlated with each other, and Staphylococcus was negatively correlated with other core taxa. Some associations were also observed between components of different types. Using redundancy analysis and structural equation modeling, the overall milk fatty acid profile was significantly associated with milk microbiota composition. In addition, some individual fatty acids [22:6n3 (docosahexaenoic acid), 22:5n3, 20:5n3, 17:0, 18:0] and oligosaccharides (fucosyl-lacto-N-hexaose, lacto-N-hexaose, lacto-N-fucopentaose I) were associated with microbiota α diversity, while others (C18:0, 3′-sialyllactose, disialyl-lacto-N-tetraose) were associated with overall microbiota composition. Only a few significant associations between individual HMOs and microbiota were observed; notably, among mothers using breast pumps, Bifidobacterium prevalence was associated with lower abundances of disialyl-lacto-N-hexaose. Additionally, among non-secretor mothers, Staphylococcus was positively correlated with sialylated HMOs.
Conclusion: Using multiple approaches to integrate and analyse milk microbiota, oligosaccharides, and fatty acids, we observed several associations between different milk components and microbiota, some of which were modified by secretor status and/or breastfeeding practices. Additional research is needed to further validate and mechanistically characterize these associations and determine their relevance to infant gut and respiratory microbiota development and health.
Introduction
Milk microbiota refers to the microbial community in the milk that the infant receives through breastfeeding. Although intramammary colonization has not been definitively proven (1), several culture-dependent and -independent studies have confirmed the presence of bacteria as well as fungi in human milk (2, 3). Milk serves as a niche for these microbes, and has likely evolved to select and enrich beneficial bacteria for delivery to the infant. Compared to the growing body of evidence identifying modifiable extrinsic factors shaping the milk microbiota (4, 5), the influence of the intrinsic milk environment and its major components is relatively unexplored. We previously modeled the milk environment as an unobservable variable using summary measures (first principal component axes) of human milk oligosaccharides (HMOs) and fatty acids (4). Although we did not find significant associations with the overall milk microbiota composition using these summary metrics, it remains possible that interactions occur between individual bacteria, HMOs, and fatty acids.
HMOs constitute 20% of milk carbohydrates (6). Most studies to-date have focused on HMOs and the infant gut microbiota, especially Bifidobacterium and Bacteroides (7–10). However, it is less clear whether milk microbiota in general or milk Bifidobacterium specifically could also utilize HMOs. A few observational studies have detected positive correlations between total HMO concentration and milk bacteria including Bifidobacterium spp. and Staphylococcus (11–13). On the other hand, some individual HMOs including lacto-N-fucopentaose (LNFP) I, 2′-fucosyllactose (2′FL), 3′-sialyllactose (3′SL), 6′-sialyllactose (6′SL), and lacto-N-tetraose (LNT) were negatively correlated with Actinobacteria (including bifidobacteria), Staphylococcus, and other taxa in milk. Some HMOs have also been positively correlated with potential pathogens such as Enterobacter/Klebsiella (13–15). In a small metagenomic study of human milk, HMO metabolism genes were not detected, which was attributed to the absence of Bifidobacterium spp. (16). However, other reports observed differences in the prevalence of Bifidobacterium according to the maternal secretor status (17), which is a major determinant of HMO synthesis. Notably, none of these studies accounted for the mode of breastfeeding (directly at the breast or pumped and bottled) which was a key determinant of milk microbiota composition in our previous study (4). Overall, these divergent results from relatively small studies highlight the need to examine HMOs and milk microbiota in larger studies while accounting for secretor status, mode of breastfeeding and other potential confounding factors.
Milk lipids are the main source of energy for the infant and primarily consist of triglycerides (6), which are fatty acids bound to glycerol. While the relative amounts of some milk fatty acids are fairly consistent across studies, geographies and individual women (18), others such as docosahexaenoic acid (DHA) are strongly influenced by maternal diet (19). In vitro studies have shown that polyunsaturated fatty acids (PUFAs) can have inhibitory effects on bacteria, including Lactobacillus, in a dose-dependent manner (20) while 12:0, cis-9/trans-11 and trans-10/cis-12 conjugated linoleic acid (CLA) can inhibit growth of Staphylococcus aureus in vitro (21). PUFAs and saturated fatty acids (SFAs) have also been shown to influence the microbiota composition and diversity in the gut (22–24), but their impact on milk microbiota has not been widely studied. Some correlations have been observed between milk fatty acids and milk microbiota (14). Specifically, negative correlations were reported for milk monounsaturated fatty acids (MUFAs) and PUFAs with Proteobacteria, Streptococcus, Acinetobacter, Lactobacillus, and Bifidobacterium while SFAs were positively correlated with Pseudomonas (25). However, these correlations have not been examined while controlling for maternal fish oil supplement use, which modifies milk n3-PUFA concentrations (26) and might also influence microbiota (27).
Given the suggestive evidence noted above and the potential roles of HMOs and fatty acids in promoting or inhibiting bacterial growth in experimental settings (23, 28), we hypothesized that these different milk components could shape the milk microbiota composition and could be influenced by maternal secretor status, fish oil supplement use, and breastfeeding practices. Therefore, the objective of this study was to perform an integrative analysis of milk microbiota with HMOs and fatty acids while taking into account key factors that impact these milk components (Figure 1).
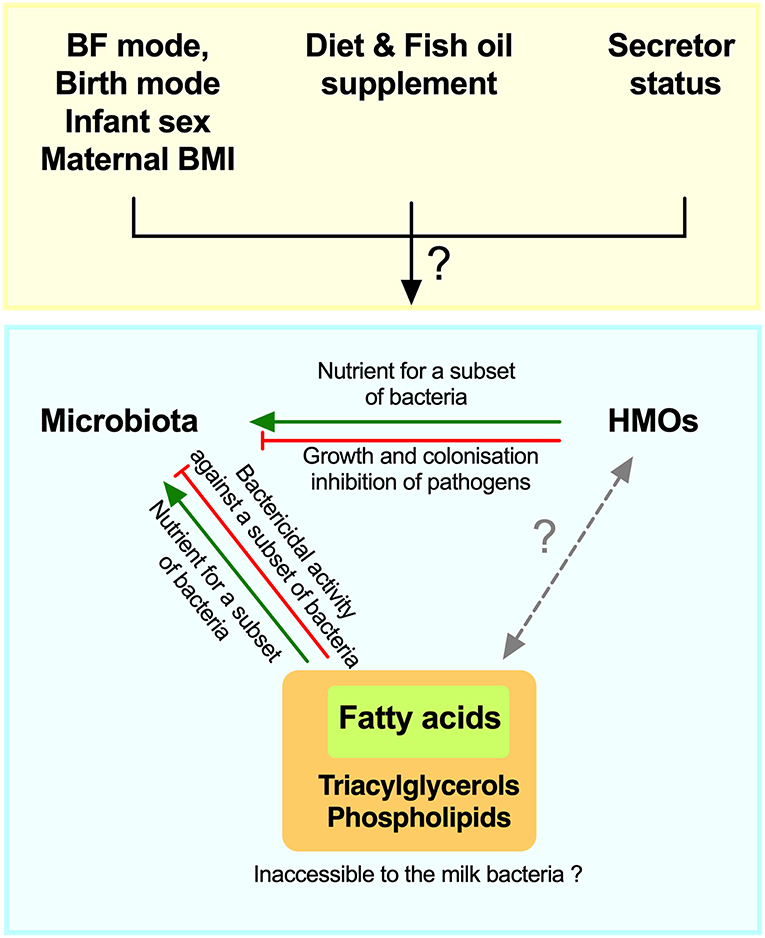
Figure 1. Schematic of hypothesized integrative associations of human milk oligosaccharides (HMOs) and fatty acids, with milk microbiota, and factors that could modify these associations. BF, breastfeeding; BMI, body mass index.
Materials and Methods
Study Design
We studied a representative subset of 393 mothers participating in the Canadian Healthy Infant Longitudinal Development (CHILD) birth cohort (29). Women with singleton pregnancies were enrolled between 2008 and 2012 and remained eligible if they delivered a healthy infant >35 weeks gestation (n = 3,455). We selected a representative subset of mother-infant dyads with available milk samples (N = 432), ensuring equal representation across the four study sites, excluding dyads missing key maternal (e.g., diet, BMI) or infant (1y clinical) data, and then randomly selecting among the rest (4). For the current study, samples with sufficient depth of sequencing for microbiota analysis (N = 393) were included (Supplementary Methods). All participants gave written informed consent in accordance with the Declaration of Helsinki. The protocol was approved by the Human Research Ethics Boards at McMaster University, the Hospital for Sick Children, and the Universities of Manitoba, Alberta, and British Columbia.
Milk Sample Collection
Each mother provided one sample of milk at 3–4 months postpartum [mean (SD) 17 (5) weeks postpartum] in a sterile milk container provided by the CHILD study. To control for differences in the milk composition of fore- and hindmilk (30) as well as the diurnal variation (31), a mix of foremilk and hindmilk from multiple feeds during a 24-h period was collected. Hand expression was recommended, but pumping was also acceptable. The sample was not collected aseptically. Samples were refrigerated at home for up to 24 h before being collected and processed by study staff (32). Samples were stored at −80°C until analysis.
DNA Extraction and Microbiota Analysis
Milk microbiota was assessed by 16SrRNA gene sequencing of V4 hypervariable region with modified F515/R806 primers (33) on a MiSeq platform (Illumina, San Diego, CA, USA) and processed using dada2 (34) as previously described (4) (Supplementary Methods). Potential reagent contaminants were identified and excluded using decontam package (35). Demultiplexed sequencing data was deposited into the Sequence Read Archive (SRA) of NCBI and can be accessed via accession number SRP153543.
HMO Analysis
Human milk oligosaccharides (HMOs) were previously measured in the same milk samples by high performance liquid chromatography (36). The following 19 HMOs were detected on the basis of retention time comparison with commercial standard oligosaccharides and mass spectrometry analysis: 2′FL, 3-fucosyllactose (3FL), 3′SL, 6′SL, LNT, lacto-N-neotetraose (LNnT), LNFPI, LNFPII, LNFPIII, sialyl-LNT (LST) b, LSTc, difucosyl-LNT (DFLNT), disialyl-LNT (DSLNT), fucosyl-lacto-N-hexaose (FLNH), difucosyl-lacto-N-hexaose (DFLNH), fucosyl-disialyl-lacto-N-hexaose (FDSLNH), and disialyl-lacto-N-hexaose (DSLNH), difucosyllactose (DFLac), and lacto-N-hexaose (LNH) and were quantified based on response factors. These 19 HMOs typically account for >90% of total HMO content; their concentrations were summed to estimate total HMO concentration. The relative abundance of each HMO was calculated. The amount of HMO-bound fucose (Fuc) and HMO-bound sialic acid (Sia) were calculated. HMOs were also grouped according to common structural elements as previously described (37) (Table S1). Maternal secretor status was defined by the presence or near absence of 2′FL or LNFP I. HMOs were studied as both absolute concentrations (μg/mL) and relative proportions.
Fatty Acids Analysis
Fatty acids were also previously analyzed by gas liquid chromatography at University of Alberta as previously described (38). The following 29 fatty acids were detected: capric acid (10:0), lauric acid (12:0), myristic acid (14:0), physeteric acid (14:1n9), pentadecylic acid (15:0), palmitic acid (16:0), 7-hexadecenoic acid (16:1n9), margaric acid (17:0), stearic acid (18:0), trans vaccenic acid (TVA, 18:1t11), oleic acid (18:1n9), cis-vaccenic acid (18:1c11), linoleic acid (LA, 18:2n6), arachidic acid (20:0), γ-linoleic acid (GLA, 18:3n6), α-linolenic acid (ALA, 18:3n3), eicosadienoic acid (20:2n6), dihomo-γ-linolenic acid (DHGLA, 20:3n6), arachidonic acid (AA, 20:4n6), conjugated linoleic acid (CLA, family of isomers of linoleic acid), eicosapentanoic acid (EPA, 20:5n3), nervonic acid (24:1n9), docosahexaenoic acid (DHA, 22:6n3), lignoceric acid (24:0), osbond Acid (22:5n6), docosapentaenoic acid (DPA, 22:5n3), eicosatetraenoic acid (20:4n3), adrenic acid (C22:4n6). Fatty acids were grouped into SFAs, MUFAs, and PUFAs. Additionally, they were classified as synthesized de novo in the mammary gland, or acquired from the diet, liver synthesis, or body fat storage. PUFAs were also divided into n-3 and n-6 series (Table S1).
Covariates
The mode of breastmilk feeding was reported for breastfed infants at the time of breastmilk collection and classified as “direct breastfeeding only” (feeding at the breast only, with no feeding of pumped milk), or “some pumped milk” (at least one serving of pumped milk in the past 2 weeks) (39). Maternal age, infant sex, birth weight, gestational age, method of birth, and parity were documented from hospital records. Method of birth was categorized as normal vaginal delivery, emergency, or elective Cesarean section. Maternal ethnicity and fish oil supplement use during pregnancy was self-reported by standardized questionnaire.
Infant Secretor Status
Infant DNA was isolated from peripheral blood samples. Genotyping of single nucleotide polymorphisms (SNPs) was performed using the HumanCoreExome BeadChip (Illumina, San Diego, CA, USA) comprising >240 K tagSNPs and >240 K exome-focused markers. A stringent process of quality control at infant and SNP levels was applied using PLINK v.1.9 (http://pngu.mgh.harvard.edu/purcell/plink/href) (40). Samples were excluded if demonstrated low genotype call rates (missingness >0.10), discrepancies with reported sex, were ethnic outliers based on PCA, or were related as determined by identity by descent. SNPs were excluded with missingness >0.10, had significant departures from Hardy-Weinberg equilibrium (p <10−6), or had a minor allele frequency <0.01. IMPUTE2 software (41) was used to impute genotypes based on the 1000 Genomes Project reference panel (42), which resulted in >23,000,000 single nucleotide variants of high quality (>0.7). Using this imputed dataset, infant secretor status was defined based on FUT2 rs601338 or rs1047781 SNPs.
Statistical Analysis
Data analysis was conducted in R (43). α diversity was assessed by the observed OTUs (richness) and inverse Simpson index (diversity). To control for the compositional nature of the data, microbiota, fatty acids, and HMO relative abundances were center log-ratio transformed following zero-replacement (44, 45). After this transformation, correlations of taxa relative abundances with fatty acids and HMOs were compared at phylum, family, and genus levels by Spearman rank correlation. P-values were corrected with Benjamini–Hochberg's false discovery rate (FDR) method. Analyses of HMOs and fatty acids were stratified by maternal secretor status and fish oil supplement use, respectively. Additionally, partial correlations were assessed using Spearman rank correlation and visualized as networks using qgraph package (46). The association of HMOs and fatty acids composition profiles with milk microbiota composition was assessed by redundancy analysis (RDA) with 1,000 permutations using the vegan package (47). Structural equation modeling (SEM) was performed using the lavaan package (48). Model fit was assessed by χ2 test, the comparative fix index (CFI), and the standardized root mean residuals (SRMR). Non-significant χ2 test, CFI ≥ 0.9, RMSEA < 0.05, and SRMR < 0.08 were considered as indications of good model fit (49). Association of HMOs Z normalized relative abundances with Bifidobacterium presence was assessed using logistic regression stratified by mode of breastfeeding and adjusted for maternal secretor status. Covariate selections for different statistical tests were informed by our previous report of the association of maternal, infant, and early life factors with milk microbiota composition (4). P-values of less than 0.05 were considered significant.
Results
The majority of our representative subset of 393 mothers were Caucasian (74%) and the mean ± SD maternal age was 33 ± 4 years. About half (54%) were primiparous and a quarter (24%) delivered by Cesarean section. The mean ± SD lactation stage at sample collection was 17 ± 5 weeks postpartum and 58% of mothers were providing some pumped milk to their infant. Seventy-two percent of mothers were secretors and 21% used fish oil (Table S2).
Milk microbiota and HMO profiles in the CHILD cohort have been previously described (4, 36, 50). Overall, Streptococcus, Ralstonia, and Acidovorax were the most abundant genera (Figure 2A) and Proteobacteria (mean 67%) and Firmicutes (mean 26%) were the most abundant phyla (Figure 2B). 2′FL (among secretor mothers), LNFP II, and LNT were the most abundant HMOs (Figure 2C) and fucosylated/non-sialylated was the most abundant HMO group (Figure 2D). Fatty acids oleic acid (18:1n9), palmitic acid (16:0), and linoleic acid (18:2n6) were the most abundant while the majority had very low relative abundances (<1%) (Figure 2E). SFAs (41%) and MUFAs (39%) were the most abundant fatty acids followed by PUFAs (17%) (Figure 2F).
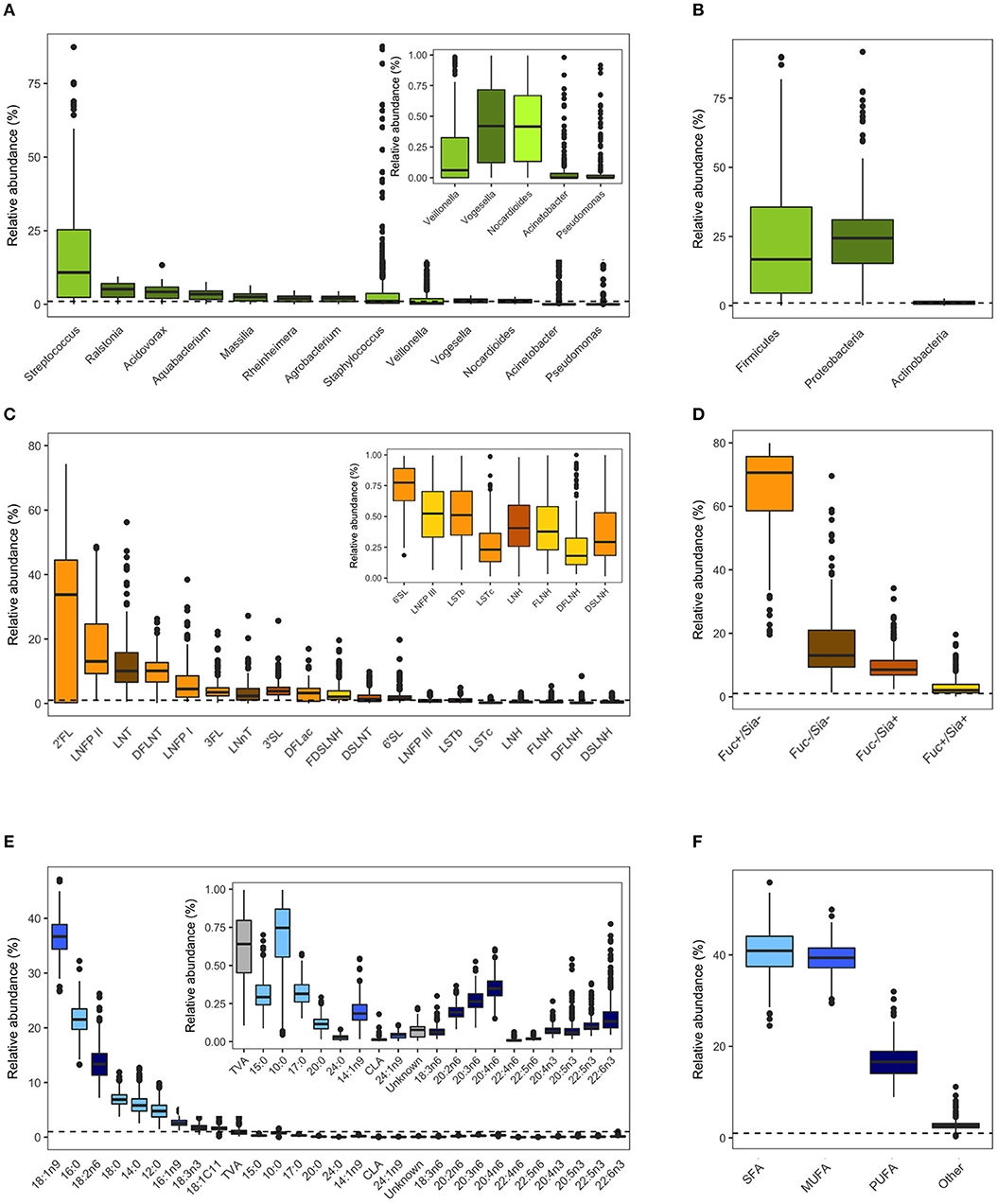
Figure 2. Milk microbiota, human milk oligosaccharides (HMOs), and fatty acids relative abundances. (A) Abundant bacteria genera (>0.01% mean relative abundance), (B) aggregated relative abundances at the phylum level, (C) HMOs, (D) HMO groups based on fucosylation and sialyation, (E) fatty acids, (F) fatty acids categories. Dashed horizontal lines mark 1% relative abundance; components with means <1% are shown in figure insets. Figures are adapted from results originally reported in Moossavi et al. (4), Azad et al. (36), and Miliku et al. (50).
Correlations Are Stronger and More Frequent Within Than Between Different Types of Milk Components
Integrated correlations of different components were visualized in a heatmap. The strongest correlations were observed within each group (Figure 3A). For example, Staphylococcus was strongly negatively correlated with nearly every other core taxa (r-values <-0.60, p < 0.05). Strong positive correlations were also found between LNFP II/FDSLNH, C12:0/C14:0, 22:4n6/24:1n9 (all r > 0.4, p < 0.05). Next, to isolate and visualize the most significant associations, we assessed pairwise partial correlations between members of milk microbiota, HMOs, and fatty acids using Spearman correlation. Correlations with p-value of < 0.05 after Benjamini–Hochberg's correction were visualized as a network (Figure 3B). Consistent with the conventional heatmap, the network shows that the number and strength of intra-group correlations were higher than inter-group correlations, which could be partly attributed to the compositional nature of all three components. Partial correlations between core microbiota and fatty acids included Agrobacterium/18:1n9 (positive), and Ralstonia/18:1c11 (negative). Correlations between microbiota and HMOs included Vogesella/DSLNT (negative) and Ralstonia/6'SL (negative) (Figure 3B).
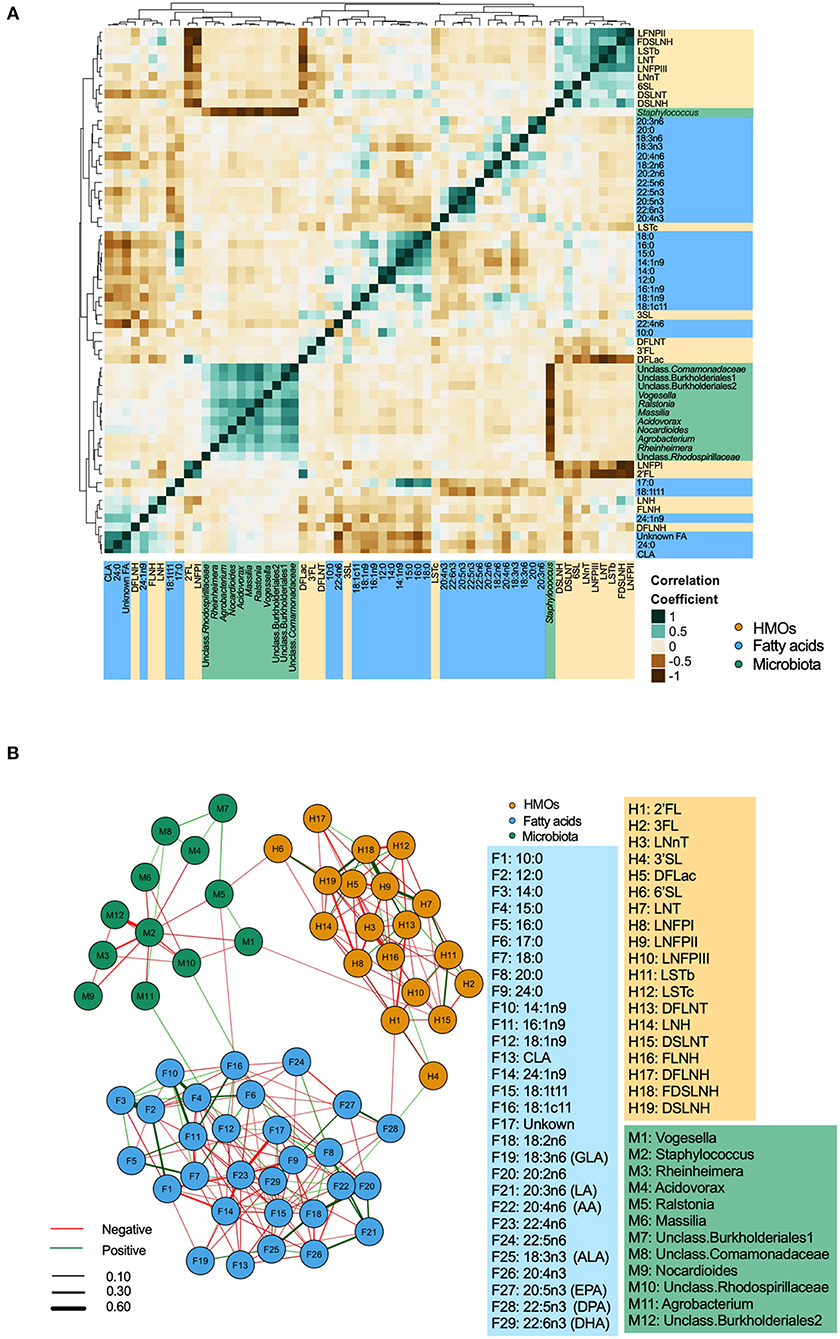
Figure 3. Inter and intra-correlations of milk microbiota, human milk oligosaccharides (HMOs), and fatty acids. (A) Clustering heatmap based on Spearman rank correlation, (B) Network visualization based on partial Spearman rank correlations. Line color represents the direction of correlation: green (positive), red (negative). Line width is proportional to the correlation coefficient.
Some HMOs and Fatty Acids Are Associated With Milk Microbiota α Diversity and Overall Composition
We previously identified mode of breastfeeding, infant sex, parity, and birth mode to be associated with microbiota richness and diversity, but found no association between milk microbiota diversity and the first principal component axes (PC1) for either HMOs or fatty acids (4). However, when examining individual HMOs and fatty acids (expressed as relative proportions) in the current analysis, some associations were observed. Controlling for the above-mentioned covariates, FLNH was associated with microbiota richness [β = 5.90, 95%CI (0.04–11.8) per SD increase in FLNH, p = 0.04) while LNH (β = 1.32, 95%CI (0.04–2.60), p = 0.04] and LNFP I (β = −1.04, 95%CI (−2.03 to −0.05), p = 0.03] were associated with microbiota diversity (Figure 4A). These opposite effects of different HMOs could explain the apparent lack of association of the overall HMO profile and microbiota diversity. Other HMOs were not associated with milk microbiota richness and diversity, and we did not detect any significant patterns of association when grouping HMOs according to fucosylation and sialyation (Figure 4A) or other common structural elements (37) (not shown).
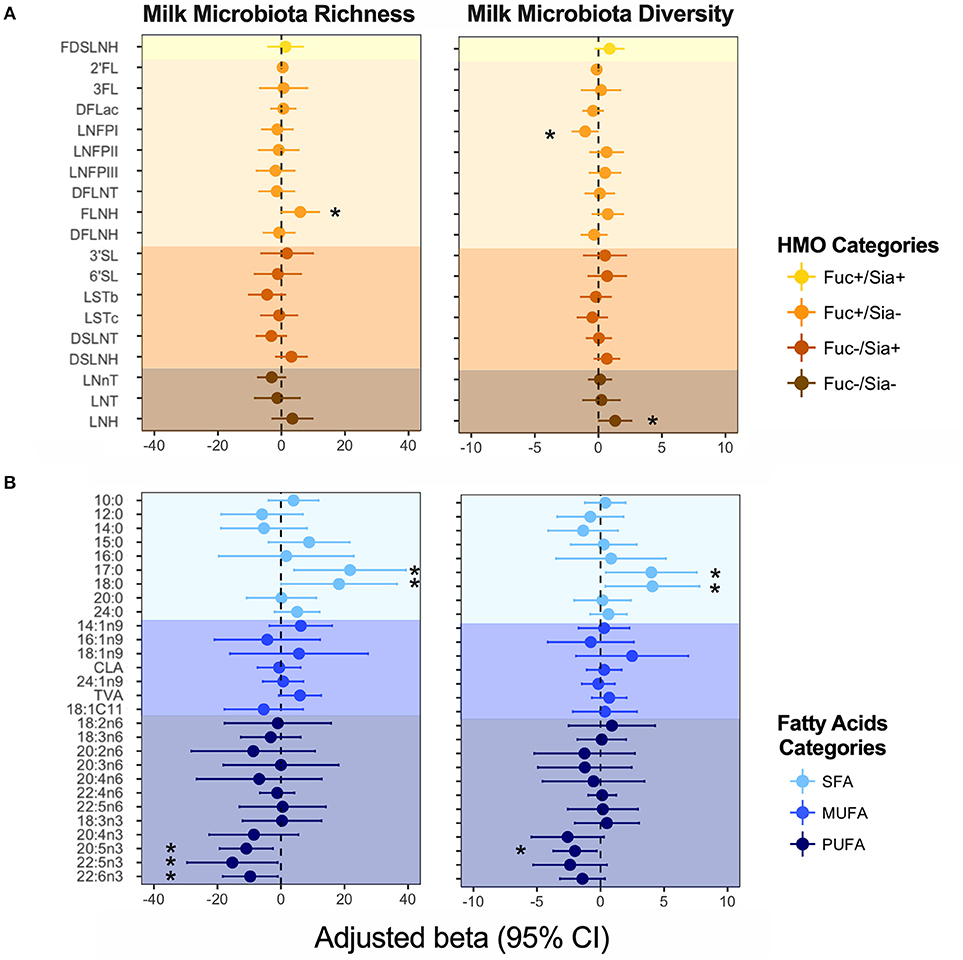
Figure 4. Association of (A) human milk oligosaccharides (HMOs) and (B) fatty acids with milk microbiota richness (observed ASVs) and diversity (inverse Simpson index). Adjusted for mode of breastfeeding, infant sex, parity, and birth mode. Fatty acids and HMOs are colored according to their categories. *denotes significant associations (p < 0.05).
Fatty acids DHA, DPA, EPA (all n3-PUFAs) were negatively associated with milk microbiota richness [e.g., DHA: β = −9.67, 95%CI (−18.30 to −1.031), p = 0.028], while SFAs 17:0 and 18:0 were positively associated with these metrics (Figure 4B). We did not detect any associations for MUFAs (Figure 4B), nor for fatty acids grouped based on their source/origin (i.e., synthesized de novo in the mammary gland, or acquired from the diet, liver synthesis, or body fat storage) (Figure S1).
Using redundancy analysis, overall HMO and fatty acid compositions were not associated with the overall composition of the milk microbiota (4.82% variation explained, p = 0.30 for HMOs and 7.60%, p = 0.13 for fatty acids; Table 1), as previously observed (4). Consistent with this finding, milk microbiota diversity and taxonomic clusters were not associated with the overall composition of HMOs or fatty acids (Figure S2). However, a few significant associations were observed when examining individual HMOs and fatty acids. Specifically, HMO 3′SL (0.58%, p = 0.01) and DSLNT (0.45%, p = 0.04) and fatty acid 18:0 (0.55%, p = 0.01) were significantly associated with the overall composition of the milk microbiota (Table 1).
Some HMOs and Fatty Acids Are Weakly Associated With Specific Milk Bacteria Relative Abundances
Next, we assessed the correlation of individual HMOs and fatty acids with milk microbiota at phylum, family, and genus taxonomic levels (all taxa with >0.01% mean relative abundance). Only a few weak (r < |0.15|) associations between HMOs and microbiota were identified. These associations were mostly between DFLac, type 1 HMOs (consisting of LNT, LNFP I, LNFP II, LSTb, and DSLNT), and non-fucosylated/non-sialylated HMOs (LNnT, LNT, LNH) with Bacteroidetes and Prevotellaceae (Figure S3). When stratified by maternal secretor status, only sialylated/non-fucosylated HMOs remained significantly correlated with Prevotella (r = 0.22, p = 0.028). We did not detect any significant associations with HMOs expressed as absolute quantities (not shown).
Similarly, we identified a few weak correlations (r <|0.20|) between fatty acids and milk bacteria including negative correlations between C18:1c11/Actinobacteria and CLA/Porphyromonadaceae, and positive correlations between C20:4n6/Bacteroidetes, C22:5n3/Fusobacteria, and 22:4n6/Prevotellaceae. No correlations with any of the fatty acid categories (SFAs, MUFAs, or PUFAs) were observed (not shown).
When focusing on the core milk microbiota [amplicon sequence variants (ASVs) present in at least 95% of samples with more than 1% mean relative abundance], some additional patterns emerged. Overall, core microbiota was weakly correlated with fatty acids and HMOs (Figure 5). Nocardioides was significantly associated with several fatty acids including 12:0, 14:0, 16:0, 24:0 (SFAs, correlations with r > |0.20| and p < 0.05) (Figure 5A). The strongest individual correlations were observed for DSLNT with Nocardioides, Massilia, and unclassified Comamonadaceae (negative correlations with r <−0.23 and p < 0.05) (Figure 5B). In general, the directions of correlation of core ASVs with HMOs were opposite for fucosylated vs. sialylated oligosaccharides. These correlations were directionally consistent across most taxa, with the notable exception of Staphylococcus: while most ASVs were positively correlated with fucosylated HMOs and negatively correlated with sialylated HMOs, these relationships were inversed for Staphylococcus (Figure 5B). Interestingly, when stratified by secretor status, these associations were only observed in non-secretor mothers (Figure 5C). Additionally, MUFAs were significantly associated with Staphylococcus in the absence of fish oil supplementation (Figure 5D).
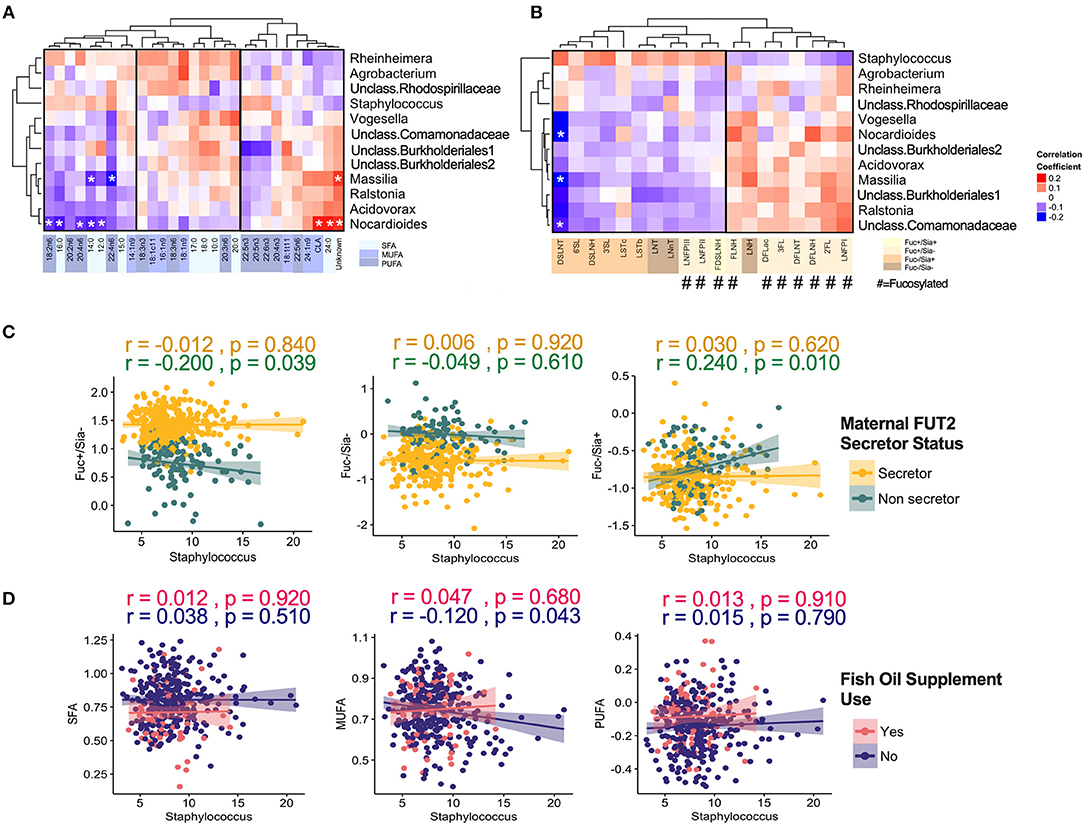
Figure 5. Correlation of core milk microbiota (>1% relative abundance) with milk components. (A) Fatty acids, (B) human milk oligosaccharides (HMOs). Correlation of core Staphylococcus ASV with (C) HMOs stratified by maternal secretor status and (D) Fatty acids groups stratified by maternal fish oil supplement.
Maternal and Infant Secretor Status Are Not Strongly Associated With Overall Milk Microbiota Composition
As previously reported (36), maternal FUT2-secretor status was a clear determinant of HMO composition (Figure S4). We also assessed infant secretor status because this genotypic trait could influence the infant oral microbiota, which could potentially inoculate the mother's milk. We did not identify major differences in the milk microbiota structure or α diversity based on maternal or infant secretor status (Figure 6). However, Bacteroidetes relative abundance was significantly higher in milk produced by secretor mothers (β = 2.15, 95% CI 0.56–3.73 for secretor vs. non-secretor mothers, p < 0.01, independent of mode of breastfeeding and other relevant covariates) (Figure 6A). This difference was driven by enrichment of Prevotella (0.5 ± 1.7 vs. 0.2 ± 0.7, p < 0.05). There was also a trend in the association of infant secretor status with milk Actinobacteria diversity (β = 0.37, 95% CI −0.031 to 0.775, p = 0.07 for secretor vs. non-secretor infants). Maternal and infant secretor statuses were not associated with Bifidobacterium relative abundance or prevalence (not shown), or milk microbiota β diversity (Figure S4A). Total HMO concentration was not associated with milk microbiota structure (not shown).
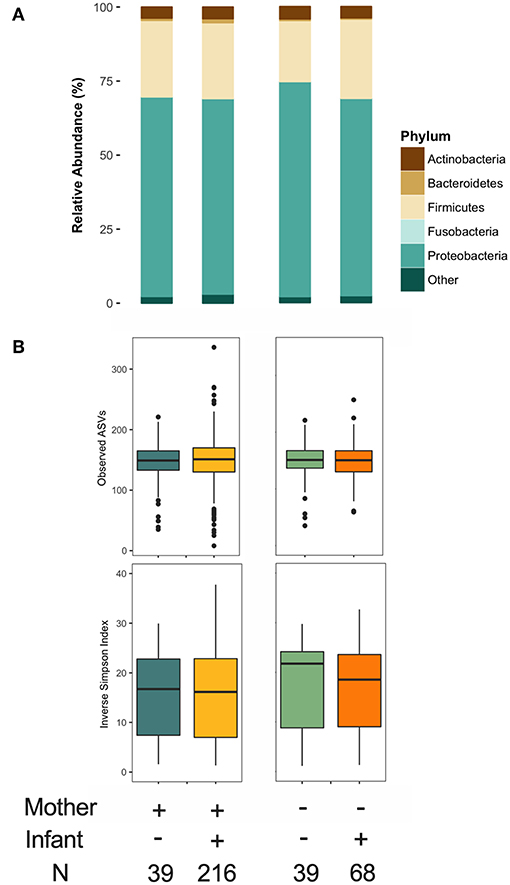
Figure 6. Association of maternal and infant secretor status with milk microbiota. (A) Taxonomy at phylum level and (B) α diversity.
HMOs Are Associated With Milk Bifidobacterium Prevalence and Abundance
Given the established ability of bifidobacteria to consume HMOs, we specifically explored the association of HMOs and bifidobacteria in milk. Bifidobacterium was present in 154 (39%) samples with mean relative abundance of 0.25 ± 0.98%. Overall, we did not observe significant associations for total or individual HMO concentrations or relative abundances (not shown); however, patterns emerged after stratification on feeding method. We undertook stratification because we have previously shown that Bifidobacterium is more prevalent in milk microbiota of mothers feeding exclusively at the breast (4). Among these mothers, Bifidobacterium relative abundance was negatively associated with type 1 HMOs (r = −0.29, p < 0.001) (Figure 7A). Among mothers providing some pumped breast milk, Bifidobacterium was less likely to be present in milk with higher levels of DSLNH (OR = 0.65, 95%CI 0.43–0.93, p = 0.03 per 1 SD increase in DSLNH, Figure 7B).
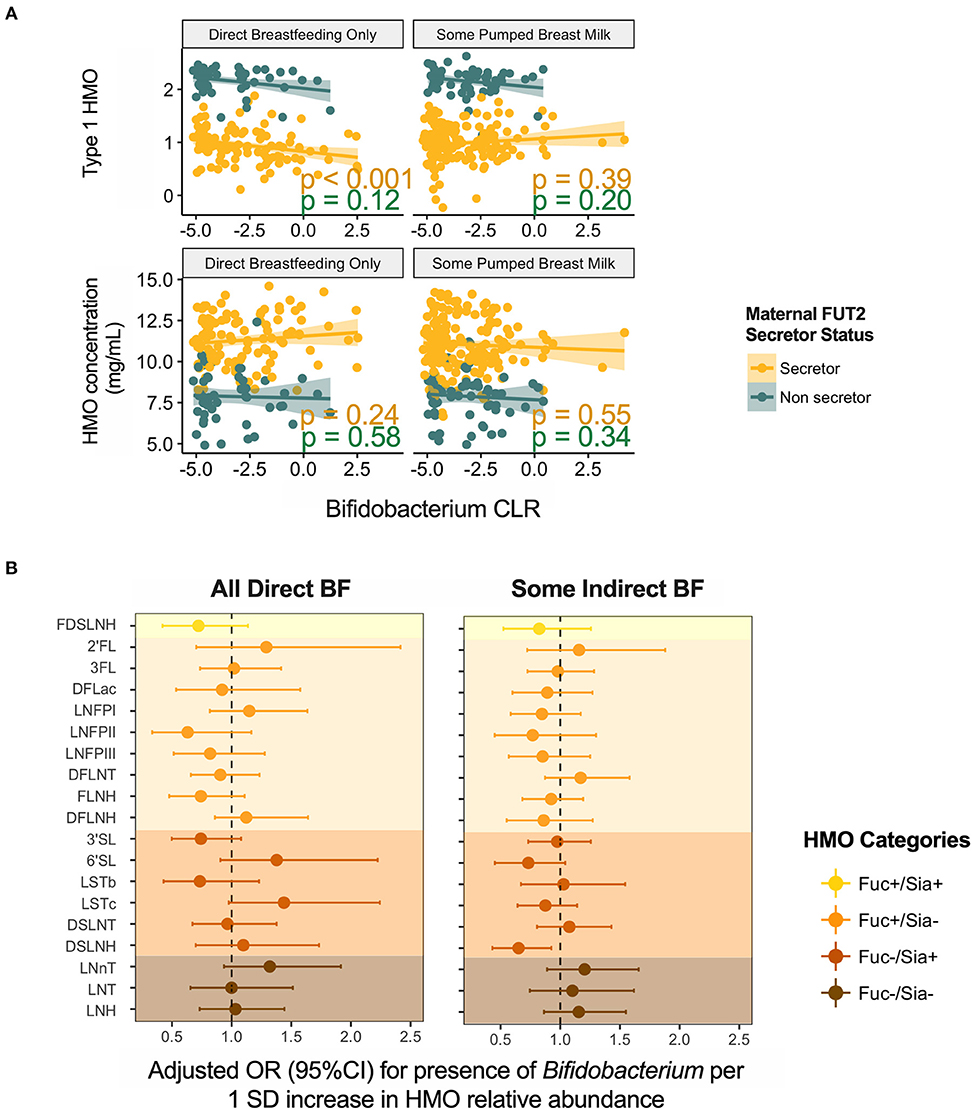
Figure 7. Association of Bifidobacterium (A) relative abundance and (B) prevalence with human milk oligosaccharides. BF, breastfeeding.
Milk Fatty Acid Profile Is Associated With Milk Microbiota Composition When Controlling for Relevant Confounding Factors
Finally, we used SEM to assess the associations between milk components using summary statistics (first principal component axes; PC1) while controlling for key covariates (Figure 8). Fatty acids PC1 was significantly associated with milk microbiota composition (PC1) when controlling for fish oil supplement use (a key dietary source of n3-PUFA) and maternal BMI (a key factor influencing fatty acids originating from liver synthesis or body storage), maternal secretor status, mode of breastfeeding, infant sex, and birth mode (β = 0.14, p < 0.01). HMO PC1 was not significantly associated with milk microbiota composition in this model. Thus, consistent with results from redundancy analysis, the SEM results suggest that fatty acids, more so than HMOs, are associated with milk microbiota composition while controlling for relevant factors (Figure 4).
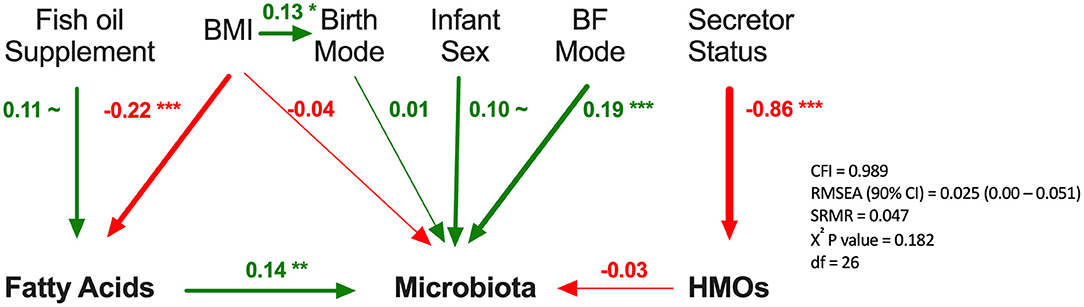
Figure 8. Structural equation modeling of the association of human milk oligosaccharides (HMOs) and fatty acids with microbiota. Standardized β coefficients are reported. BF, breastfeeding; BMI, maternal body mass index; CFI, comparative fix index; CI, confidence interval; RSMEA, root mean square error of approximation; SRMR, standardized root mean residuals; ~p < 0.1, *p < 0.05, **p < 0.01, ***p < 0.001, Green (positive), red (negative).
Discussion
To our knowledge, this is the first integrative analysis of human milk microbiota with HMOs and milk fatty acids. We applied multiple statistical approaches to combine and simultaneously analyse the association of these milk components with milk microbiota while controlling for important covariates reflecting maternal genetics, diet, and breastfeeding practices. Overall, the correlations between milk components within each group were stronger and more frequent than correlations between components from different groups. Still, a few weak associations were observed between individual HMOs or fatty acids and microbial taxa, including Bifidobacterium. Interestingly, some of these associations were modified by maternal secretor status, fish oil supplementation, or method of breastfeeding. In structural equation models controlling for these and other key factors influencing milk composition, the overall fatty acid profile (but not the overall HMO profile) remained directly associated with milk microbiota composition.
Our study contributes new evidence on the potential interactions between HMOs and milk bacteria. Some bacterial genera are known to either utilize HMOs (e.g., Bifidobacterium, Bacteroides, and Lactobacillus) or benefit from their growth-promoting effect without direct utilization (e.g., Staphylococcus) (12, 28, 51). We identified correlations between certain HMOs and Bacteroidetes, Bifidobacterium, and Staphylococcus. The correlations were relatively weak and occurred in both positive and negative directions, in agreement with the different HMO consumption characteristics of these bacteria (12, 28). However, these correlations were different from previous small-scale q-PCR-based (11, 17) and sequencing-based studies (13, 14, 17). Notably, Bacteroidetes relative abundance was higher in milk from secretor mothers suggesting preferential utilization of α-1,2 HMOs. Lack of major shifts in the mature milk microbiota by maternal secretor status was in accordance with previous studies (15, 17). Interestingly, while nearly all core milk microbiota demonstrated specific and inverse correlation patterns with fucosylated and sialylated HMOs (which could indicate utilization or inhibition), these relationships were inversed for Staphylococcus. Although Staphylococcus does not utilize HMOs, it could still be influenced by the presence of HMOs in the microenvironment (12). Finally, HMO 3′SL and DSLNT (α2-3-sialylated HMOs) were significantly associated with the overall composition of the milk microbiota (52), suggesting that some HMOs might indeed shape milk microbiota. Interestingly, some Bifidobacterium spp. found in milk have sialidase enzymes with activity on α2-3 linkages, enabling them to release free sialic acid from these particular HMOs (52). However, whether these HMOs are metabolized by the majority of milk bacteria is not known. Given the large abundance of lactose as a more accessible carbon source in milk, it is also not known if HMOs are a major source of energy for milk bacteria.
We specifically investigated Bifidobacterium because this species is predominant in the gut of breastfed infants, yet the source of this bacterium is unclear. Interestingly, only 39% of human milk samples in our study contained Bifidobacterium, which is curious in light of the current consensus that Bifidobacterium is vertically transferred via breastmilk to “seed” the infant gut. Notably, our samples were collected at 3–4 months postpartum, so it remains possible that bifidobacteria are more ubiquitously present earlier in lactation. Previous studies examining strains of Bifidobacterium in infant gut, mother's milk, and maternal gut have concluded that they could be transferred from mother to infant through milk (53, 54). If this is true, HMOs could provide a mechanism for selection and enrichment of Bifidobacterium spp. in human milk as it is being produced in the mammary gland. Indeed, we found significant associations between a few HMOs or HMO types and the presence or relative abundance of Bifidobacterium in milk, suggesting that certain HMO profiles could perhaps generate a favorable niche for this bacterium in milk by providing a preferential substrate for specific Bifidobacterium species. However, this hypothesis requires further investigation since Bifidobacterium and HMO metabolism pathways were not detected in a small-scale shotgun metagenomic study of the milk microbiota (16), and to our knowledge, there is currently no evidence that bacteria actively metabolize HMOs in the mammary gland.
We also provide evidence for a potential association between milk fatty acids and microbiota. The majority of studies examining fatty acids and microbial communities have focused on microbial production of short chain fatty acids in the gastrointestinal tract (55), whereas we explored associations between medium and long chain fatty acids and microbiota in milk. We observed a significant association of the fatty acids profile with the overall microbiota composition. However, this pattern emerged only when examining the summary metric of fatty acids profile (i.e., PC1) and controlling for HMO profile and confounding variables, potentially indicating a subtle impact of the fatty acids on microbiota. We also identified a few specific fatty acids to be associated with microbiota α diversity or overall composition. Stearic acid (18:0) was the fourth most abundant fatty acid in our study and was significantly associated with the overall composition of the milk microbiota independently of confounding factors.
The causative mechanism for the associations between milk fatty acids and milk microbiota is unclear since some fatty acids have antibacterial properties (20, 21) while others are produced or consumed by the microbial community. Pathways are described for recognition and uptake of exogenous fatty acids by bacteria. Internalized fatty acids will be metabolized and utilized as transcriptional regulators, source of energy, or membrane phospholipids (56). Moreover, some fatty acids are considered as potential prebiotics (57). Even though we did not observe many correlations of milk fatty acids with milk microbiota, it is possible that fatty acids alter bacterial physiology and virulence (58–61) rather than growth kinetics. Alternatively, milk fatty acids could modulate milk immune cell concentrations (13) and hence indirectly affect milk microbiota. Generally, it is unclear if milk fatty acids, which are largely bound to glycerol, are readily accessible to milk bacteria. It is possible that some fatty acids are released in the infant oral cavity by lingual lipases (62) and thus either locally affect the oral bacteria before retrograde inoculation of the mammary gland, or alternatively get transferred to the intramammary region with the retrograde milk flow during breastfeeding.
Strengths and Limitations
The main strength of this study is our integrative, multi-variable approach to assessing the associations between HMOs, fatty acids, and milk microbiota, using the rich data and large sample size afforded by the CHILD cohort. In this study, we were able to control for maternal intake of fish oil supplement and secretor status as key factors influencing fatty acids and HMOs, respectively. Additionally, informed by our previous analysis (4), we controlled for other factors that may influence the microbiota. A limitation of our study is that milk samples were pooled from multiple feeds and not collected aseptically, in order to reflect the “average” composition of milk fed to each infant. Thus, diurnal variability and differences in foremilk and hindmilk could not be studied. Information on mastitis, which could be associated with shifts in milk microbiota and fatty acid compositions (63, 64), was not available in our study, and absolute concentrations of fatty acids were not available. Other milk components such as antimicrobial peptides and antibodies, which could confound the association of HMOs and fatty acids with milk microbiota, were not available for our samples. Finally, as with all culture-independent microbiota studies, we could not quantify bacterial load nor confirm the viability of bacteria identified in our samples (65).
Conclusion and Future Direction
Overall, using multiple approaches for data integration to analyse three major components of human milk (fatty acids, microbiota, and HMOs), we identified strong correlations within each group, but relatively few correlations between groups. Fatty acid composition, more so than HMO profile, was associated with milk microbiota composition when controlling for relevant confounders. While the cumulative effect of HMOs on the entire microbial community appears to be small, our findings suggest that individual HMOs might promote or inhibit growth of specific milk bacteria, potentially providing a selection mechanism for vertical mother-offspring transmission of microbiota. Further research is warranted to explore these possibilities, and to determine how these and other milk components collectively modulate the infant gut microbiota and influence the risk of developing microbiota-mediated childhood diseases.
Data Availability
The datasets generated for this study can be found in Sequence Read Archive, SRP153543.
Ethics Statement
All participants gave written informed consent in accordance with the Declaration of Helsinki. The protocol was approved by the Human Research Ethics Boards at McMaster University, the Hospital for Sick Children, and the Universities of Manitoba, Alberta, and British Columbia.
Author Contributions
SM and MA contributed to conceptualization. SM, EK, CF, and LB contributed to methodology. SM, FA and, KM contributed to investigation. SM, CF, LB, and MA contributed to writing the original draft. FA, KM, SS, BR, QD, AB, PM, ST, TM, DL, MS, PS, and EK contributed to writing the review and to editing. MA contributed to funding acquisition and supervision. AB, PM, ST, DL, and MS contributed to PS resources.
Funding
The Canadian Institutes of Health Research (CIHR) and the Allergy, Genes and Environment Network of Centres of Excellence (AllerGen NCE) provided core support for the CHILD Study. This research was specifically funded by the Heart and Stroke Foundation and Canadian Lung Association, in partnership with the Canadian Respiratory Research Network and AllerGen NCE. Additional support was provided by the Natural Science and Engineering Research Council of Canada (NSERC) Discovery Program and internal grants from the University of Manitoba. Infrastructure at the Gut Microbiome Laboratory was supported by grants from the Canadian Foundation for Innovation. This research was supported, in part, by the Canada Research Chairs program. LB is the Larsson-Rosenquist Foundation Endowed Chair in Collaborative Human Milk Research, which is endowed by the Family Larsson-Rosenquist Foundation. SM holds a Research Manitoba Doctoral Studentship. These entities had no role in the design and conduct of the study; collection, management, analysis, and interpretation of the data; and preparation, review, or approval of the manuscript.
Conflict of Interest Statement
The authors declare that the research was conducted in the absence of any commercial or financial relationships that could be construed as a potential conflict of interest.
Acknowledgments
We are grateful to all the families who took part in this study, and the whole CHILD team, which includes interviewers, nurses, computer and laboratory technicians, clerical workers, research scientists, volunteers, managers, and receptionists. The full list of CHILD study investigators is listed separately. We acknowledge Sonia Anand and Guillaume Pare (McMaster University) for generating the genetic data. We thank Susan Goruk (University of Alberta) for technical assistance with fatty acids measurements. We also thank Lorena Vehling (University of Manitoba) for creating the breastfeeding variables.
Supplementary Material
The Supplementary Material for this article can be found online at: https://www.frontiersin.org/articles/10.3389/fnut.2019.00058/full#supplementary-material
Abbreviations
HMO, human milk oligosaccharide; MUFA, monounsaturated fatty acid; PUFA, polyunsaturated fatty acid; SFA, saturated fatty acid; 2′FL, 2′-fucosyllactose; 3FL, 3-fucosyllactose; 3′SL, 3′-sialyllactose; 6′SL, 6′-sialyllactose; LNT, Lacto-N-tetraose; LNnT, Lacto-N-neotetraose; LNFP, Lacto-N-fucopentaose; LST, Sialyl-LNT; DFLNT, Difucosyl-LNT; DSLNT, Disialyl-LNT; FLNH, Fucosyl-lacto-N-hexaose; DFLNH, Difucosyl-lacto-N-hexaose; FDSLNH, Fucosyl-disialyl-lacto-N-hexaose; DSLNH, Disialyl-lacto-N-hexaose; DFLac, Difucosyllactose; LNH, Lacto-N-hexaose; TVA, Trans vaccenic acid; LA, Linoleic acid; GLA, γ-linoleic acid; ALA, α-linolenic acid; DHGLA, Dihomo-γ-linolenic acid; AA, Arachidonic acid; CLA, Conjugated linoleic acid; EPA, Eicosapentanoic acid; DHA, docosahexaenoic acid; DPA, docosapentaenoic acid.
References
1. Rainard P. Mammary microbiota of dairy ruminants: fact or fiction? Vet Res. (2017) 48:25. doi: 10.1186/s13567-017-0429-2
2. Fitzstevens JL, Smith KC, Hagadorn JI, Caimano MJ, Matson AP, Brownell EA. Systematic review of the human milk microbiota. Nutr Clin Pract. (2016) 32:354–64. doi: 10.1177/0884533616670150
3. Boix-Amoros A, Martinez-Costa C, Querol A, Collado MC, Mira A. Multiple approaches detect the presence of fungi in human breastmilk samples from healthy mothers. Sci Rep. (2017) 7:13016. doi: 10.1038/s41598-017-13270-x
4. Moossavi S, Sepehri S, Robertson B, Bode L, Goruk S, Field CJ, et al. Composition and variation of the human milk microbiome is influenced by maternal and early life factors. Cell Host Microbe. (2019) 25:324–35. doi: 10.1016/j.chom.2019.01.011
5. Bode L, McGuire M, Rodriguez JM, Geddes DT, Hassiotou F, Hartmann PE, et al. It's alive: microbes and cells in human milk and their potential benefits to mother and infant. Adv Nutr. (2014) 5:571–3. doi: 10.3945/an.114.006643
6. Garwolinska D, Namieśnik J, Kot-Wasik A, Hewelt-Belka W. Chemistry of human breast milk – a comprehensive review of the composition and role of milk metabolites in child development. J Agric Food Chem. (2018) 66:11881–96. doi: 10.1021/acs.jafc.8b04031
7. Bender JM, Li F, Martelly S, Byrt E, Rouzier V, Leo M, et al. Maternal HIV infection influences the microbiome of HIV-uninfected infants. Sci Transl Med. (2016) 8:351er6. doi: 10.1126/scitranslmed.aah7122
8. Kirmiz N, Robinson RC, Shah IM, Barile D, Mills DA. Milk glycans and their interaction with the infant-gut microbiota. Annu Rev Food Sci Technol. (2018) 9:429–50. doi: 10.1146/annurev-food-030216-030207
9. Korpela K, Salonen A, Hickman B, Kunz C, Sprenger N, Kukkonen K, et al. Fucosylated oligosaccharides in mother's milk alleviate the effects of caesarean birth on infant gut microbiota. Sci Rep. (2018) 8:13757. doi: 10.1038/s41598-018-32037-6.
10. Asakuma S, Hatakeyama E, Urashima T, Yoshida E, Katayama T, Yamamoto K, et al. Physiology of consumption of human milk oligosaccharides by infant gut-associated bifidobacteria. J Biol Chem. (2011) 286:34583–92. doi: 10.1074/jbc.M111.248138
11. Aakko J, Kumar H, Rautava S, Wise A, Autran C, Bode L, et al. Human milk oligosaccharide categories define the microbiota composition in human colostrum. Benef Microbes. (2017) 8:563–7. doi: 10.3920/BM2016.0185
12. Hunt KM, Preuss J, Nissan C, Davlin CA, Williams JE, Shafii B, et al. Human milk oligosaccharides promote the growth of staphylococci. Appl Environ Microbiol. (2012) 78:4763–70. doi: 10.1128/AEM.00477-12
13. Williams JE, Price WJ, Shafii B, Yahvah KM, Bode L, McGuire MA, et al. Relationships among microbial communities, maternal cells, oligosaccharides, and macronutrients in human milk. J Hum Lact. (2017) 33:540–51. doi: 10.1177/0890334417709433
14. Gómez-Gallego C, Morales J, Monleón D, du Toit E, Kumar H, Linderborg K, et al. Human breast milk NMR metabolomic profile across specific geographical locations and its association with the milk microbiota. Nutrients. (2018) 10:1355. doi: 10.3390/nu10101355.
15. Ramani S, Stewart CJ, Laucirica DR, Ajami NJ, Robertson B, Autran CA, et al. Human milk oligosaccharides, milk microbiome and infant gut microbiome modulate neonatal rotavirus infection. Nat Commun. (2018) 9:5010. doi: 10.1038/s41467-018-07476-4
16. Ward TL, Hosid S, Ioshikhes I, Altosaar I. Human milk metagenome- a functional capacity analysis. BMC Microbiol. (2013) 13:116. doi: 10.1186/471-2180-13-116.
17. Cabrera-Rubio R, Kunz C, Rudloff S, Garcia-Mantrana I, Crehua-Gaudiza E, Martinez-Costa C, et al. Association of maternal secretor status and human milk oligosaccharides with milk microbiota: an observational pilot study. J Pediatr Gastroenterol Nutr. (2018) 68:256–63. doi: 10.1097/MPG.0000000000002216
18. Koletzko B, Rodriguez-Palmero M, Demmelmair H, Fidler N, Jensen R, Sauerwald T. Physiological aspects of human milk lipids. Early Hum Dev. (2001) 65:S3–18. doi: 10.1016/S0378-3782(01)00204-3
19. Urwin HJ, Miles EA, Noakes PS, Kremmyda LS, Vlachava M, Diaper ND, et al. Salmon consumption during pregnancy alters fatty acid composition and secretory IgA concentration in human breast milk. J Nutr. (2012) 142:1603–10. doi: 10.3945/jn.112.160804
20. Kankaanpää PE, Salminen SJ, Isolauri E, Lee YK. The influence of polyunsaturated fatty acids on probiotic growth and adhesion. FEMS Microbiol Lett. (2001) 194:149–53. doi: 10.1111/j.1574-6968.2001.tb09460.x
21. Kelsey JA, Bayles KW, Shafii B, McGuire MA. Fatty acids and monoacylglycerols inhibit growth of Staphylococcus aureus. Lipids. (2006) 41:951–61. doi: 10.1007/s11745-006-5048-z
22. Kabara JJ, Swieczkowski DM, Conley AJ, Truant JP. Fatty acids and derivatives as antimicrobial agents. Antimicrob Agents Chemother. (1972) 2:23–8.
23. Churchward CP, Alany RG, Snyder LAS. Alternative antimicrobials: the properties of fatty acids and monoglycerides. Crit Rev Microbiol. (2018) 44:561–70. doi: 10.1080/1040841x.2018.1467875
24. Menni C, Zierer J, Pallister T, Jackson MA, Long T, Mohney RP, et al. Omega-3 fatty acids correlate with gut microbiome diversity and production of N-carbamylglutamate in middle aged and elderly women. Sci Rep. (2017) 7:11079. doi: 10.1038/s41598-017-10382-2
25. Kumar H, du Toit E, Kulkarni A, Aakko J, Linderborg KM, Zhang Y, et al. Distinct patterns in human milk microbiota and fatty acid profiles across specific geographic locations. Front Microbiol. (2016) 7:1619. doi: 10.3389/fmicb.2016.01619
26. Dunstan JA, Roper J, Mitoulas L, Hartmann PE, Simmer K, Prescott SL. The effect of supplementation with fish oil during pregnancy on breast milk immunoglobulin A, soluble CD14, cytokine levels and fatty acid composition. Clin Exp Allergy. (2004) 34:1237–42. doi: 10.1111/j.1365-2222.2004.02028.x
27. Caesar R, Tremaroli V, Kovatcheva-Datchary P, Cani PD, Backhed F. Crosstalk between gut microbiota and dietary lipids aggravates WAT inflammation through TLR Signaling. Cell Metab. (2015) 22:658–68. doi: 10.1016/j.cmet.2015.07.026
28. Craft KM, Townsend SD. The human milk glycome as a defense against infectious diseases: rationale, challenges, and opportunities. ACS Infect Dis. (2017) 4:77–83. doi: 10.1021/acsinfecdis.7b00209
29. Subbarao P, Anand SS, Becker AB, Befus AD, Brauer M, Brook JR, et al. The Canadian Healthy Infant Longitudinal Development (CHILD) Study: examining developmental origins of allergy and asthma. Thorax. (2015) 70:998–1000. doi: 10.1136/thoraxjnl-2015-207246
31. Nozad S, Ramin AG, Asri Rezaie S. Diurnal variations in milk macro-mineral concentrations in Holstein dairy cows in Urmia, Iran. Vet Res Forum. (2012) 3:281–5. doi: 10.2298/AVB1101003N
32. Moraes TJ, Lefebvre DL, Chooniedass R, Becker AB, Brook JR, Denburg J, et al. The Canadian healthy infant longitudinal development birth cohort study: biological samples and biobanking. Paediatr Perinat Epidemiol. (2015) 29:84–92. doi: 10.1111/ppe.12161
33. Caporaso JG, Lauber CL, Walters WA, Berg-Lyons D, Huntley J, Fierer N, et al. Ultra-high-throughput microbial community analysis on the Illumina HiSeq and MiSeq platforms. ISME J. (2012) 6:1621–4. doi: 10.1038/ismej.2012.8
34. Callahan BJ, McMurdie PJ, Rosen MJ, Han AW, Johnson AJ, Holmes SP. DADA2: High-resolution sample inference from Illumina amplicon data. Nat Methods. (2016) 13:581–3. doi: 10.1038/nmeth.3869
35. Davis NM, Proctor D, Holmes SP, Relman DA, Callahan BJ. Simple statistical identification and removal of contaminant sequences in marker-gene and metagenomics data. Microbiome. (2018) 6:226. doi: 10.1186/s40168-018-0605-2
36. Azad MB, Robertson B, Atakora F, Becker AB, Subbarao P, Moraes TJ, et al. Human milk oligosaccharide concentrations are associated with multiple fixed and modifiable maternal characteristics, environmental factors, and feeding practices. J Nutr. (2018) 148:1733–42. doi: 10.1093/jn/nxy175
37. McGuire MK, Meehan CL, McGuire MA, Williams JE, Foster J, Sellen DW, et al. What's normal? Oligosaccharide concentrations and profiles in milk produced by healthy women vary geographically. Am J Clin Nutr. (2017) 105:1086–100. doi: 10.3945/ajcn.116.139980
38. Cruz-Hernandez C, Goeuriot S, Giuffrida F, Thakkar SK, Destaillats F. Direct quantification of fatty acids in human milk by gas chromatography. J Chromatogr A. (2013) 1284:174–9. doi: 10.1016/j.chroma.2013.01.094
39. Klopp A, Vehling L, Becker AB, Subbarao P, Mandhane PJ, Turvey SE, et al. Modes of infant feeding and the risk of childhood asthma: a prospective birth Cohort study. J Pediatr. (2017) 190:192–9. doi: 10.1016/j.jpeds.2017.07.012
40. Purcell S, Neale B, Todd-Brown K, Thomas L, Ferreira MA, Bender D, et al. PLINK: a tool set for whole-genome association and population-based linkage analyses. Am J Hum Genet. (2007) 81:559–75. doi: 10.1086/519795
41. Marchini J, Howie B, Myers S, McVean G, Donnelly P. A new multipoint method for genome-wide association studies by imputation of genotypes. Nat Genet. (2007) 39:906–13. doi: 10.1038/ng2088
42. Genomes Project C, Auton A, Brooks LD, Durbin RM, Garrison EP, Kang HM, et al. A global reference for human genetic variation. Nature. (2015) 526:68–74. doi: 10.1038/nature15393
43. R Core Team. R: A Language and Environment for Statistical Computing. Vienna: R Foundation for Statistical Computing (2017).
44. Palarea-Albaladejo J, Martin-Fernandez JA. zCompositions – R package for multivariate imputation of left-censored data under a compositional approach. Chemom Intell Lab Syst. (2015) 143:85–96. doi: 10.1016/j.chemolab.2015.02.019
45. Gloor GB, Reid G. Compositional analysis: a valid approach to analyze microbiome high-throughput sequencing data. Can J Microbiol. (2016) 62:692–703. doi: 10.1139/cjm-2015-0821
46. Epskamp S, Cramer AOJ, Waldorp LJ, Schmittmann VD, Borsboom D. qgraph: network visualizations of relationships in psychometric data. J Stat Softw. (2012) 48:1–18. doi: 10.18637/jss.v048.i04
47. Oksanen J, Blanchet FG, Friendly M, Kindt R, Legendre P, McGlinn D, et al. Vegan: Community Ecology Package. R package version 2.4-3. (2017).
48. Rosseel Y. lavaan: An R package for structural equation modeling. J Stat Softw. (2012) 48:1–36. doi: 10.18637/jss.v048.i02
49. Kline RB. Principles and Practices of Structural Equation Modeling. 4th ed. New York, NY: The Guilford Press (2016).
50. Miliku K, Becker AB, Goruk S, Subbarao P, Mandhane PJ, Turvey SE, et al. Human milk fatty acids and asthma development in preschool children. Curr Dev Nutr. (2018) 2:nzy040. doi: 10.1093/cdn/nzy040
51. Zúñiga M, Monedero V, Yebra MJ. Utilization of host-derived glycans by intestinal Lactobacillus and Bifidobacterium species. Front Microbiol. (2018) 9:1917. doi: 10.3389/fmicb.2018.01917
52. Juge N, Tailford L, Owen CD. Sialidases from gut bacteria: a mini-review. Biochem Soc Trans. (2016) 44:166–75. doi: 10.1042/BST20150226
53. Asnicar F, Manara S, Zolfo M, Truong DT, Scholz M, Armanini F, et al. Studying vertical microbiome transmission from mothers to infants by strain-level metagenomic profiling. mSystems. (2017) 2:e00164–16. doi: 10.1128/mSystems.00164-16
54. Biagi E, Quercia S, Aceti A, Beghetti I, Rampelli S, Turroni S, et al. The bacterial ecosystem of mother's milk and infant's mouth and gut. Front Microbiol. (2017) 8:1214. doi: 10.3389/fmicb.2017.01214
55. McNabney S, Henagan T. Short chain fatty acids in the colon and peripheral tissues: a focus on butyrate, colon cancer, obesity and insulin resistance. Nutrients. (2017) 9:1348. doi: 10.3390/nu9121348
56. Fujita Y, Matsuoka H, Hirooka K. Regulation of fatty acid metabolism in bacteria. Mol Microbiol. (2007) 66:829–39. doi: 10.1111/j.1365-2958.2007.05947.x
57. Gibson GR, Hutkins R, Sanders ME, Prescott SL, Reimer RA, Salminen SJ, et al. Expert consensus document: The International Scientific Association for Probiotics and Prebiotics (ISAPP) consensus statement on the definition and scope of prebiotics. Nat Rev Gastroenterol Hepatol. (2017) 14:491–502. doi: 10.1038/nrgastro.2017.75
58. Baker LY, Hobby CR, Siv AW, Bible WC, Glennon MS, Anderson DM, et al. Pseudomonas aeruginosa responds to exogenous polyunsaturated fatty acids (PUFAs) by modifying phospholipid composition, membrane permeability, and phenotypes associated with virulence. BMC Microbiol. (2018) 18:117. doi: 10.1186/s12866-018-1259-8
59. Moravec AR, Siv AW, Hobby CR, Lindsay EN, Norbash LV, Shults DJ, et al. Exogenous polyunsaturated fatty acids impact membrane remodeling and affect virulence phenotypes among pathogenic vibrio species. Appl Environ Microbiol. (2017) 83:e01415–17. doi: 10.1128/AEM.01415-17
60. Eder AE, Munir SA, Hobby CR, Anderson DM, Herndon JL, Siv AW, et al. Exogenous polyunsaturated fatty acids (PUFAs) alter phospholipid composition, membrane permeability, biofilm formation and motility in Acinetobacter baumannii. Microbiology. (2017) 163:1626–36. doi: 10.1099/mic.0.000556
61. Hobby CR, Herndon JL, Morrow CA, Peters RE, Symes SJK, Giles DK. Exogenous fatty acids alter phospholipid composition, membrane permeability, capacity for biofilm formation, and antimicrobial peptide susceptibility in Klebsiella pneumoniae. Microbiologyopen. (2019) 8:e00635. doi: 10.1002/mbo3.635
62. Fredrikzon B, Hernell O, Bläckberg L. Lingual lipase. Its role in lipid digestion in infants with low birthweight and/or pancreatic insufficiency. Acta Paediatr Scand Suppl. (1982) 296:75–80.
63. Hunt KM, Williams JE, Shafii B, Hunt MK, Behre R, Ting R, et al. Mastitis is associated with increased free fatty acids, somatic cell count, and interleukin-8 concentrations in human milk. Breastfeed Med. (2013) 8:105–10. doi: 10.1089/bfm.2011.0141
64. Patel SH, Vaidya YH, Patel RJ, Pandit RJ, Joshi CG, Kunjadiya AP. Culture independent assessment of human milk microbial community in lactational mastitis. Sci Rep. (2017) 7:7804. doi: 10.1038/s41598-017-08451-7
Keywords: Bifidobacterium, breastmilk, microbiota, human milk oligosaccharide, fatty acids, mode of breastfeeding, CHILD cohort
Citation: Moossavi S, Atakora F, Miliku K, Sepehri S, Robertson B, Duan QL, Becker AB, Mandhane PJ, Turvey SE, Moraes TJ, Lefebvre DL, Sears MR, Subbarao P, Field CJ, Bode L, Khafipour E and Azad MB (2019) Integrated Analysis of Human Milk Microbiota With Oligosaccharides and Fatty Acids in the CHILD Cohort. Front. Nutr. 6:58. doi: 10.3389/fnut.2019.00058
Received: 27 February 2019; Accepted: 15 April 2019;
Published: 16 May 2019.
Edited by:
Juan M. Rodríguez, Complutense University of Madrid, SpainReviewed by:
Maria Jesus Yebra, Instituto de Agroquímica y Tecnología de Alimentos (IATA), SpainGiuseppe Conte, University of Pisa, Italy
Copyright © 2019 Moossavi, Atakora, Miliku, Sepehri, Robertson, Duan, Becker, Mandhane, Turvey, Moraes, Lefebvre, Sears, Subbarao, Field, Bode, Khafipour and Azad. This is an open-access article distributed under the terms of the Creative Commons Attribution License (CC BY). The use, distribution or reproduction in other forums is permitted, provided the original author(s) and the copyright owner(s) are credited and that the original publication in this journal is cited, in accordance with accepted academic practice. No use, distribution or reproduction is permitted which does not comply with these terms.
*Correspondence: Meghan B. Azad, meghan.azad@umanitoba.ca