- 1Centre Nutrition, Santé et Société (NUTRISS), Institut sur la Nutrition et les Aliments Fonctionnels (INAF), Université Laval, Québec City, QC, Canada
- 2School of Nutrition, Université Laval, Québec City, QC, Canada
- 3Mass General Brigham, Boston, MA, United States
- 4Ariadne Labs, Boston, MA, United States
- 5Harvard Medical School, Boston, MA, United States
- 6The Broad Institute, Boston, MA, United States
- 7Library, Université Laval, Québec City, QC, Canada
- 8Human Longevity, Inc., San Diego, CA, United States
Background: There is a significant lack of consistency used to determine the scientific validity of nutrigenetic research. The aims of this study were to examine existing frameworks used for determining scientific validity in nutrition and/or genetics and to determine which framework would be most appropriate to evaluate scientific validity in nutrigenetics in the future.
Methods: A systematic review (PROSPERO registration: CRD42021261948) was conducted up until July 2021 using Medline, Embase, and Web of Science, with articles screened in duplicate. Gray literature searches were also conducted (June-July 2021), and reference lists of two relevant review articles were screened. Included articles provided the complete methods for a framework that has been used to evaluate scientific validity in nutrition and/or genetics. Articles were excluded if they provided a framework for evaluating health services/systems more broadly. Citing articles of the included articles were then screened in Google Scholar to determine if the framework had been used in nutrition or genetics, or both; frameworks that had not were excluded. Summary tables were piloted in duplicate and revised accordingly prior to synthesizing all included articles. Frameworks were critically appraised for their applicability to nutrigenetic scientific validity assessment using a predetermined categorization matrix, which included key factors deemed important by an expert panel for assessing scientific validity in nutrigenetics.
Results: Upon screening 3,931 articles, a total of 49 articles representing 41 total frameworks, were included in the final analysis (19 used in genetics, 9 used in nutrition, and 13 used in both). Factors deemed important for evaluating nutrigenetic evidence related to study design and quality, generalizability, directness, consistency, precision, confounding, effect size, biological plausibility, publication/funding bias, allele and nutrient dose-response, and summary levels of evidence. Frameworks varied in the components of their scientific validity assessment, with most assessing study quality. Consideration of biological plausibility was more common in frameworks used in genetics. Dose-response effects were rarely considered. Two included frameworks incorporated all but one predetermined key factor important for nutrigenetic scientific validity assessment.
Discussion/Conclusions: A single existing framework was highlighted as optimal for the rigorous evaluation of scientific validity in nutritional genomics, and minor modifications are proposed to strengthen it further.
Systematic Review Registration: https://www.crd.york.ac.uk/prospero/display_record.php?RecordID=261948, PROSPERO [CRD42021261948].
Background
It has been suggested for decades that optimal strategies for managing health and disease should be personalized. In 1903, Dr. William Osler stated that “variability is the law of life and no two individuals react alike and behave alike under the abnormal conditions which we know as disease. The good [healthcare provider] treats the disease, the great one treats the patient (1).” In modern times, we are experiencing a new level of personalized healthcare, with the consideration of genetic variation for treating, managing and preventing various diseases and conditions. In the field of nutrition, the use of genetic information to personalize dietary recommendations is a potentially powerful tool emerging as a result of the developing field of nutritional genomics. This is a field that explores the interplay between genetics and nutrition, and their subsequent impact on health-related outcomes (2). While this science is applied to clinical practice and more broad guidance for clinicians has been published (3, 4), there is considerable debate about whether the use of nutrigenetic tests have sufficient scientific validity to be supported (5, 6). In order to resolve this debate, there is a clear need to determine the quality of evidence (scientific validity) of the various nutrigenetic tests and their clinical claims. In addition to scientific validity, various factors should be considered when determining the suitability of a (nutri)genetic test for clinical practice. Analytic validity, clinical utility, ethical/legal implications, and risk vs. benefit are some of these factors; these are considered in the development of comprehensive clinical practice guidelines (CPGs) (7, 8). Scientific validity is one key factor evaluated prior to developing CPGs, and is considered a component of clinical validity, alongside clinical test performance (9). Scientific validity in genetics is about determining whether a gene-disease association is substantiated in the scientific literature (9). Assessing the strength and quality of evidence is a key component of determining scientific validity.
Several methods exist for evaluating the scientific validity in the fields of nutrition (10–13) and genetics (14–18) individually. However, nutritional genomics has unique considerations; as such, guidelines for evaluating genetic research, or for evaluating nutrition research are likely insufficient for properly evaluating the distinctive field of nutrigenetics. This poses concerns given the importance of selecting frameworks for evaluating scientific validity that match the research question and the type of evidence available (19).
Therefore, the aim of this study was to first provide a systematic overview of evidence evaluation frameworks used to assess scientific validity in the fields of both nutrition and genetics. We further aimed to determine if a framework exists that is appropriate for evaluating scientific validity in nutritional genomics. Lastly, we aimed to propose a framework (either an existing framework, modified version or novel framework) for use specifically in determining scientific validity in nutritional genomics. The proposed framework is intended to stimulate discussions in the scientific community and form the foundation for future refinement.
Methods
This systematic review was registered with PROSPERO (CRD42021261948) and the protocol can be accessed at https://www.crd.york.ac.uk/prospero/display_record.php?RecordID=261948. The review process was guided by previously established methods for conducting rigorous systematic reviews (20, 21).
Literature Search and Inclusion Criteria
First, a systematic search was undertaken by two investigators using three search engines: Embase, Web of Science, and Medline. The search terms are outlined in Supplementary Table 1, and databases were searched up until July 1, 2021. The PRISMA flow diagram (Figure 1) was used to guide the search strategy, moving from abstract to full-text screening (22). Article screening and selection were completed independently by two authors (JK and VG). First, titles and abstracts were screened in duplicate using Covidence software in which articles that clearly did not meet the eligibility criteria were excluded. Next, full-text articles were screened and the final list of included articles was developed. Reference lists of two relevant articles were also reviewed to search for eligible studies (18, 19). During full-text screening, JK and VG further noted any potentially relevant frameworks mentioned in the full-texts and additionally reviewed these to determine if they met the inclusion criteria. If an article met all inclusion criteria, cited articles were then searched using Google Scholar, to determine if the framework had been used for evaluating scientific validity of the body of evidence in a topic specific to nutrition and/or genetics. An additional gray literature search was conducted in June-July 2021 using the Google search engine using various combinations of key terms: evaluating; evaluation; evidence-based; evidence synthesis; scientific validity; nutrition; genetics; genomics; medicine; tool; approach; guide; manual; method; framework; levels of evidence. Included articles consisted of those that provided the complete methods for an evidence grading framework used to evaluate the quality of scientific evidence (i.e., scientific validity) in nutrition and/or genetics. Articles related to assessing the quality of genetic tests more broadly (i.e., those that considered various aspects of quality such as clinical utility, clinical validity, analytic validity, etc.) were included with the condition that a component of the methodology related to assessing scientific validity; the article summary then focused exclusively on the scientific validity assessment from the framework. Articles related to evidence evaluation frameworks specific to health services/systems more broadly such as those that related to public health program assessment, but not scientific validity in nutrition and/or genetics, were excluded.
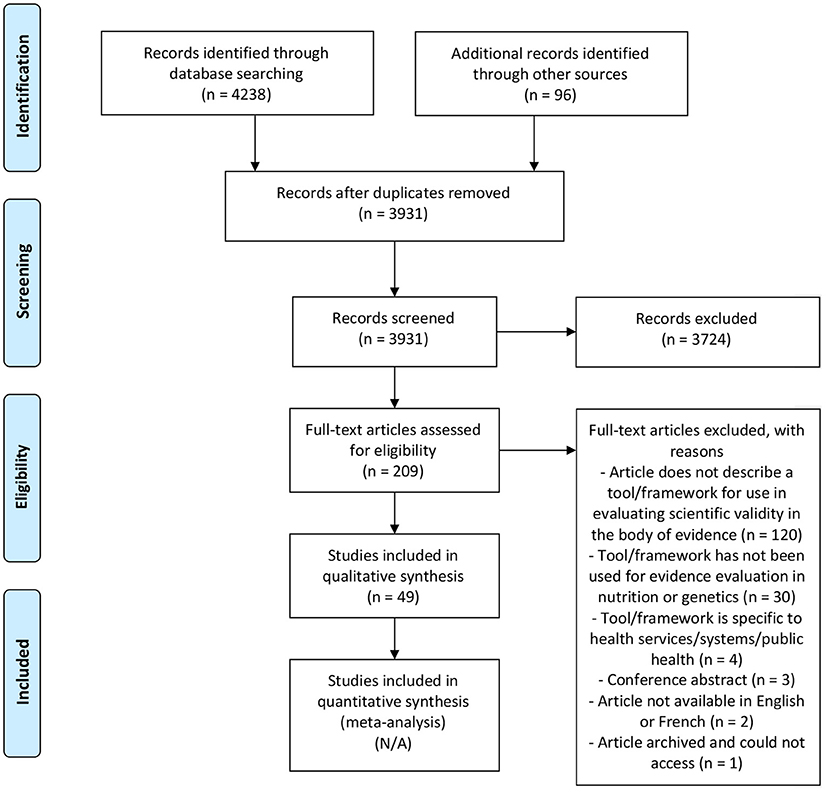
Figure 1. PRISMA 2009 flow diagram (22).
Data Extraction
Data extraction templates were piloted in duplicate on 10 included articles, with one author completing the remaining data extraction (JK). The final data extraction template included the following headings: first author name and year, framework title, included study designs for evidence evaluation, main scientific validity evaluation components described in the tool, categories for levels of evidence, a brief description of the framework and whether the framework has been used in nutrition and/or genetics. Data was also extracted for completion of the categorization matrix, further described below.
Categorization Matrix and Appraisal of Frameworks
The included frameworks were then critically appraised for their appropriateness for evaluating the quality of nutrigenetic research. A categorization matrix was used to guide the appraisal process; matrix headings were developed within the context of key factors to consider in determining levels of evidence for nutrigenetic study findings. These factors (matrix headings) were drafted collaboratively by the expert working group (JK, VG, M-CV, RG). The first draft was developed based on a review of the literature on standard items included in evidence evaluation tools, critiques of existing evidence evaluation tools and special considerations for evidence evaluation of nutrigenetic research. Following this, an external panel of experts in nutritional genomics and/or knowledge translation (either in nutrition or genetics) reviewed/revised the draft categorization matrix and provided comments. The external panel is listed in the acknowledgments section. Following revisions, all authors and members of the external panel approved the final categorization matrix.
Each included framework was then added to the categorization matrix, and data were extracted to evaluate the suitability of the framework for evaluating evidence in nutrigenetics. Checkmarks indicated that a factor was considered in the framework, whereas an “X” indicated that the factor was not considered in that particular framework. For example, frameworks that incorporated evidence of biological plausibility (as defined in Table 1) as a factor contributing the strength of the evidence received a checkmark in the categorization matrix whereas those that did not consider evidence of biological plausibility received an X. It should be noted that frameworks with more X's are not necessarily less robust, but rather they were deemed less appropriate for nutrigenetic evidence evaluation specifically. Based on the results of the categorization matrix, a framework was recommended, with minor revisions, for use in analyzing the scientific validity of gene-diet interactions/associations.
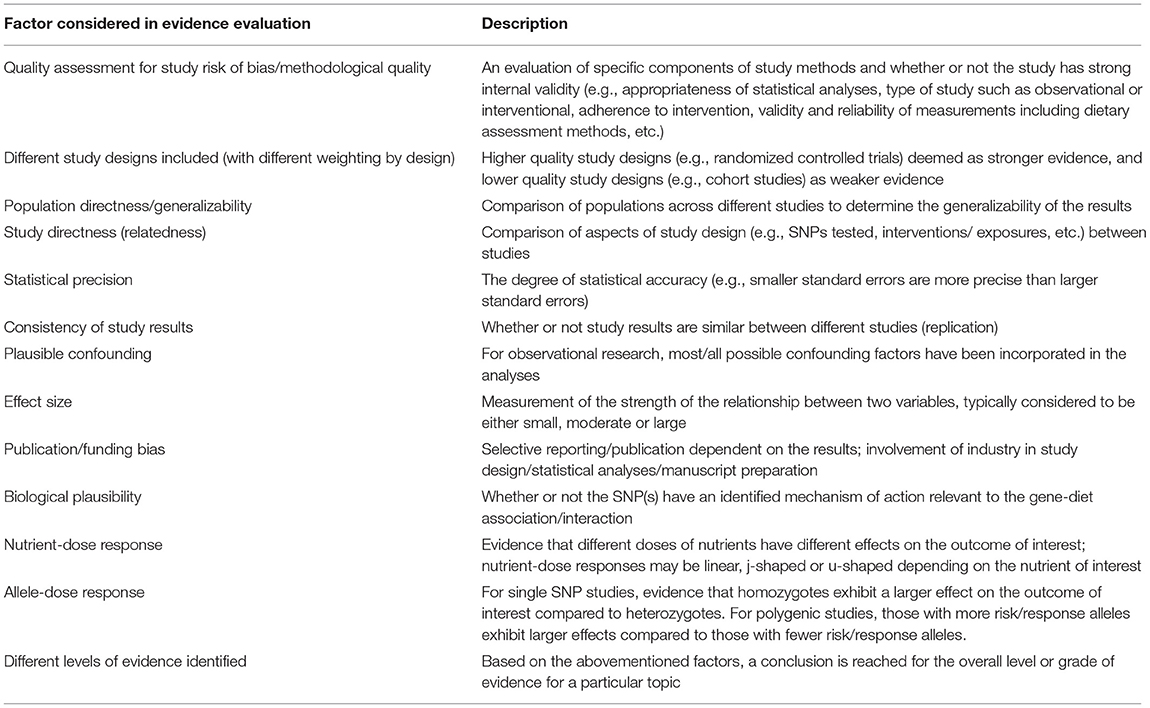
Table 1. Description of factors deemed important to consider for evaluating the body of evidence in nutritional genomics.
Results
Systematic Review of Evidence Evaluation Frameworks
Upon screening 3,931 articles, a total of 49 articles were included in this systematic review (Figure 1) representing 41 unique frameworks (n = 19 used in genetics; n = 9 used in nutrition; n = 13 used in both genetics and nutrition). One framework that has been used for evidence evaluation in both nutrition and genetics has further been used for evidence evaluation in nutritional genomics (23). The main reasons for excluding studies were if an article did not include an overview of a specific framework that was intended for use in evaluating scientific validity of the body of evidence and not exclusively single studies/articles [e.g., (24–26)] or if the framework had not been used for scientific validity assessment in nutrition or genetics [e.g., (20, 27, 28)].
There was a wide variability in the factors considered when determining scientific validity in the existing frameworks (Tables 2, 3). Frameworks commonly considered study design and quality for evidence evaluation. Consideration of biological plausibility was more commonly considered in frameworks used in genetics than in nutrition. Effect size, statistical precision, and dose-response effects were rarely included as key components of evidence evaluation in both the fields of nutrition and genetics. For frameworks used in genetics, the scientific validity assessment was often part of a larger framework for evaluating genetic tests. For example, in addition to scientific validity assessment (a component of clinical validity), the ACCE framework provides guidance for evaluating analytical validity, clinical utility and ethical/legal/social implications (8). Typically, the evaluation of scientific validity was summarized into 3–4 categories indicating the overall level of evidence, such as “high,” “moderate,” or “low” quality, or “Grade A/B/C/D.”

Table 2. Summary of included evidence evaluation frameworks for determining scientific validity in nutrition and/or genetics.
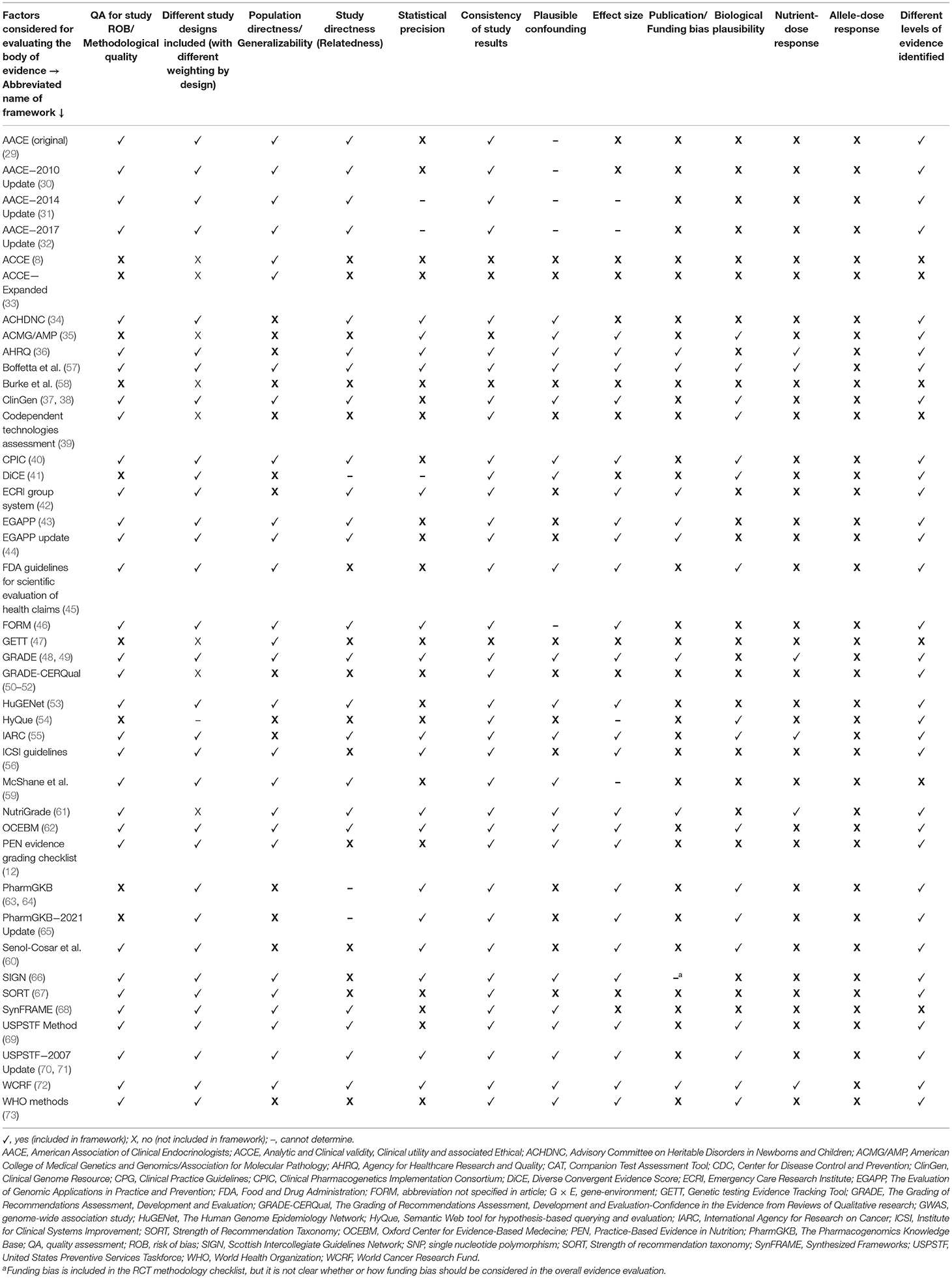
Table 3. Categorization matrix to determine the appropriateness of existing tools for evaluating the scientific validity of nutrigenetic interactions.
Categorization Matrix Development
Important factors (matrix headings) identified by the expert working group and external expert panel for evaluating nutrigenetic evidence related to: study design and quality, generalizability, directness, consistency, precision, confounding, effect size, biological plausibility, publication/funding bias, allele and nutrient dose-response, as well as summary levels of evidence. The categorization matrix is detailed in Table 3 and a description of each key factor (column heading) is described in Table 1.
It should be noted that only brief descriptions are provided in Table 1 and many of the factors incorporate consideration of several sub-factors. For example, in a risk of bias assessment of individual studies, the quality of data collection methods, statistical analyses, and sample size would be taken into consideration, among other factors (74). The factors were identified through expert consultation as well as the literature search. For example, it has been suggested in the literature that there is stronger evidence of clinical validity when a gene-disease relationship is biologically plausible, or a mechanism of action is well-understood (14, 37, 75). Similar views have been highlighted in the field of nutrigenetics (20) and medicine (76). Unknown confounding factors can result in erroneous correlations, but if a causal mechanism can provide a plausible explanation for the correlation, we increase our confidence that the correlation could be causal (76). Moreover, evidence of biological plausibility can lead to improved generalizability (77); if a function is altered, ethnicity is less likely to influence this function. However, epistasis is plausible (78), and another SNP that may be more prevalent in certain ethnicities could alter the effects of a functional SNP; epistasis is a factor to consider when evaluating the broader topic of biological plausibility. For example, a 4-SNP haplotype in leukotriene A4 hydrolase can modify the risk of myocardial infarction dependent on ethnicity (79). This factor was then presented to the expert group for feedback, and was well-received. Therefore, evidence of biological plausibility (functional SNPs) and epistasis were included in the categorization matrix. Ethnicity was also included in the matrix, falling within the category titled “Population Directness/Generalizability.” Related, evidence of allele-dose response was also included in the matrix given that it is expected for homozygotes to have an enhanced response compared to heterozygotes for a specific allele, or in the case of polygenic studies, an increasing number of risk/response alleles would result in a greater risk/response [e.g., (80–82)]. Some critiques of existing evidence evaluation frameworks were also considered when developing the categorization matrix including: limited criteria on validity (including concurrent and external validity), reliability, inadequacy addressing non-RCTs, as well as balancing simplicity and comprehensiveness/clarity (19, 83).
Appropriateness of Existing Frameworks for Nutrigenetic Evidence Evaluation
The categorization matrix was used to determine which, if any, of the existing might be most appropriate for comprehensive nutrigenetic evidence evaluation. Significantly, this systematic review did not identify any frameworks that considered all the factors deemed important by the expert group in evidence evaluation for nutritional genomics. However, there were two identified tools that considered all but one key factor relevant to evidence evaluation in nutritional genomics—the methods used by the World Cancer Research Fund (WCRF) and the gene-environment interaction framework developed by Boffetta et al. (57, 72). The factor missing from both of these methods was consideration of evidence of allele-dose responses when determining levels of evidence (57, 72). It is interesting to note that both frameworks were developed specifically for use in evidence evaluation related to cancer outcomes (57, 72). Boffetta et al.'s framework is similar to the WCRF, and the WCRF authors suggest that WCRF systematic reviews should be used (when possible) to score the strength of the evidence using this framework (57). The framework of Boffetta et al. was unique from others identified in this review as it was specific to gene-environment interactions, including gene-diet interactions. Additionally, while it was developed specifically for cancer outcomes, the authors note that it can also be applied to different health outcomes (57). Given its direct relevance to nutrigenetics, its previous use in this field (23), and applicability to broader health outcomes, this framework (57) was deemed more appropriate for nutrigenetic evidence evaluation compared to the WCRF methods (72).
We propose the use of Boffetta et al.'s framework (57), with some minor modifications, for use in broad (i.e., beyond cancer outcomes) nutrigenetics evidence evaluation. The use of this framework would result in the classification of nutrigenetic evidence into three categories: strong, moderate, or weak.
Proposed Modifications to Boffetta et al.'s Framework
Four minor modifications to this framework are suggested. First, (i) evidence of allele-dose responses should increase our confidence in a gene-diet interaction effect. This could be integrated into Step 4 of the framework as it is considered an “additional support for [the] interaction” (57). Additionally (ii), it would be beneficial to provide guidance on how individual studies can be used for evidence evaluation when systematic reviews do not exist. Further (iii), it should also be evident that this framework can be used for evaluation of topics beyond cancer occurrence, and how the guidelines may change when evaluating evidence for other outcomes (e.g., identifying resources beyond the WRCF systematic reviews and IARC monographs). Last (iv), it could be beneficial to provide a title for this framework for ease of reference. As the authors suggest, these guidelines are considered interim recommendations and their performance should be further tested in outcomes beyond cancer occurrence (57).
Discussion
This review provides an overview of one key aspect to consider when evaluating the suitability of nutrigenetic tests for clinical practice—scientific validity. While we propose that Boffetta et al. (57) have developed the most appropriate framework for determining the scientific validity of gene-diet associations and interactions, an important next step is to consider how we should then use summaries of scientific validity to develop clinical best practice guidelines. Further components of genetic testing evaluation may include clinical utility, analytic validity, ethical/legal/social implications, and others (18). In nutrition, dietary interventions may also be evaluated in the context of patient views/preferences, facilitators and barriers to their application, resource implications, and others (13). Future research should seek to explore these additional evaluation components and determine how nutrigenetic interventions should be evaluated within these contexts. It should be noted that while genetic variability is one key aspect of precision nutrition, multiple other environmental, and personal factors can influence health and disease risk (84). Examples include physical activity levels, the gut microbiome, and the metabolome (84). As such, systems biology framework is necessary for both nutrigenetic research and when developing clinical best practice guidelines in precision nutrition. Furthermore, if a genetic test lacks clinical (including scientific) validity, there is typically little need to proceed with assessing other aspects of utility/validity as there is insufficient evidence to support the particular test for clinical use (44). In addition, a care map, which outlines step-by-step directions for the appropriate integration of nutrigenetic testing into clinical practice, has been developed (3). This provides a broad overview of factors to consider to promote ethical and evidence-based practice in the field (3).
The framework developed by Boffetta et al. has been used to evaluate the evidence for nutritional genomics and breast cancer, lung cancer, prostate cancer, colorectal cancer, and stomach cancer outcomes (23). This previously conducted review identified one gene-diet interaction with moderately strong evidence (Grade BBB)—the interaction between the 10p14 locus and processed meat consumption on colorectal cancer risk (23, 85). CPG developers should now use these results to determine the possible suitability (or not) for testing this gene-diet interaction in clinical practice setting, using established CPG methods (7, 13). Additionally, the Boffetta et al. framework should now be used to determine levels of evidence for nutrigenetic outcomes beyond those related to cancer, while incorporating the modified methods described herein. This framework could be used on nutrigenetic topics deemed priorities, perhaps using a similar strategy for topic selection as that employed by the Evaluation of Genomic Application in Practice and Prevention (EGAPP) group, further discussed below (44). Some example topics for consideration may include the impact of caffeine on anxiety or other psychological conditions, based on genetic variation (86–89), or the impact of FTO genetic variation on weight and body composition responses to dietary protein intake (90, 91).
Researchers have highlighted the need for evidence syntheses and critical appraisal of evidence for years, in order to guide evidence-based nutrigenetic testing (92). Despite this, systematic reviews with evidence grading of gene-diet associations are limited, and there are varying opinions about the scientific validity of certain available nutrigenetic tests (5, 6). It is important to note that searches conducted for identifying articles relevant to a particular topic must be systematic and comprehensive in order to avoid any biases in the included studies for a particular review. It is worth highlighting that current practice guidelines for MTHFR genetic testing were not based on a systematic search, qualitative synthesis or evidence grading method (93); this could lead to biases in the guidelines. Given the extensive list of possible topics for evidence synthesis and evaluation, prioritization of nutrigenetic topics is needed. The EGAPP group prioritizes systematic review topics based on topic nomination by EGAPP members, stakeholder groups, steering committees, external consultants, Office of Public Health Genomics staff, and outside stakeholders (44). EGAPP then uses the results of these systematic reviews to make practice recommendations (either “recommendation for,” “recommendation against,” or “insufficient evidence” to make a recommendation) (44). A similar approach could work for evidence evaluation and clinical practice recommendations in nutritional genomics.
Overall, this review provided several novel insights for implementation science in nutrition, genetics, and nutrigenetics. Frameworks were variable in their consideration of biological plausibility, with more genetic frameworks considering this factor compared to nutrition frameworks. In the field of nutrigenetics, biological plausibility was deemed a key factor that should be integrated in evidence evaluation during the development of the categorization matrix. In genetics, the term “functional SNP” is used to describe SNPs with known physiological mechanisms of action (or those with a biologically plausible gene-environment function) (20, 94). The Clinical Genome Resource (ClinGen) Framework suggests that evidence of a functional variant contributes to an increasing strength of evidence (37). More specifically the strength of evidence increases depending on the evidence type. Evidence of a biochemical function, protein interaction, or expression is considered the lowest level of evidence, followed by evidence from cells of affected individuals or engineered cells as a higher level of evidence, and animal models, cell culture model systems, rescue in animal model, or rescue in engineered equivalent as the highest level of evidence (37). In this framework, evidence of function is scored, with a maximum possible score of six (37). Other frameworks and commentaries have also suggested that biological plausibility increases confidence in the evidence (14, 37, 75). Such evidence can additionally enhance the generalizability of research results; if a function is altered, ethnicity should not influence this function. However, it should also be noted that epistasis is plausible (78), and another SNP that may be more prevalent in certain ethnicities could alter the effects of a functional SNP. Overall, while biological plausibility was often not considered in existing frameworks, it was deemed important for nutrigenetic evidence evaluation.
There are some limitations and strengths of the present review. While we systematically searched the evidence, we cannot preclude the possibility that some evidence evaluation frameworks were missed. This is a possible limitation. In addition, while our methods were sound for developing the categories within the matrix, this is only a first iteration, and it is possible that future iterations may include different categories. For example, researchers have pointed out that genetic architecture matters in terms of the effect size of SNPs; ancestry-informative markers can provide a more detailed characterization of populations (79). Thus, consideration of how SNPs act within a particular genetic architecture may also be important to include in nutrigenetic evidence evaluation. Moreover, our search was limited to frameworks that have been previously used to evaluate evidence in nutrition and/or genetics, but other frameworks specific to genetics and nutrigenetics have been developed (20, 95). Future research should now seek to use these frameworks in order to pilot test their use for evidence evaluation in genetics and nutrigenetics and troubleshoot any issues. In addition, to our knowledge two frameworks exist that have been specifically developed for evaluating levels of evidence for gene-diet associations and interactions (20, 57). Only one of these frameworks met the inclusion criteria for the present review (57). The framework from Grimaldi et al. (20) was excluded given that it has not been used in a subsequent systematic review of nutrigenetic interactions for evidence evaluation thus far. This could be due to limitations of its use. Furthermore, the categorization matrix was developed while prioritizing comprehensiveness over simplicity, to determine the most thorough method for nutrigenetic evidence evaluation. This is considered a strength of the present work. More complex systems tend to be viewed as more rigorous. For example, evidence evaluation assessors have perceived GRADE as a more complex but highly rigorous system (96). It should be noted that frameworks which did not incorporate the key criteria deemed important by the expert panel, may still be highly rigorous for use in other fields, beyond nutritional genomics.
Overall, we have provided a synthesis of 49 articles representing 41 total frameworks that have been used to evaluate scientific validity in nutrition and genetics. Based on our evaluation of existing frameworks, it appears that Boffetta et al. have developed the most suitable and rigorous framework for use in evaluating the scientific validity of various gene-diet associations and interactions (57). Some minor modifications may help strengthen this framework further.
Data Availability Statement
The original contributions presented in the study are included in the article/Supplementary Material, further inquiries can be directed to the corresponding author/s.
Author Contributions
M-CV, RG, and JK conceptualized the review and developed the methods. M-CV and RG provided supervision for the project. DZ-M took primary responsibility on developing the search strategy, with guidance from JK. JK and VG were responsible for article screening and selection, as well as summarizing included articles and completing the categorization matrix, with assistance from EG and JM-B. JK wrote the first article draft. RH, DZ-M, VG, M-CV, RG, and JK revised the manuscript. All authors approved the final manuscript.
Funding
JK received postdoctoral fellowships from the Canadian Institutes of Health Research, INAF, and NUTRISS. M-CV holds a Tier 1 Canada Research Chair in Nutrition Applied to Genetics and Metabolic Health.
Conflict of Interest
RH was employed by company Human Longevity, Inc. RG has received compensation for advising the following companies: AIA, Embryome, Genomic Life, Grail, Humanity, Kneed Media, OptumLabs, Plumcare, Verily; and is co-founder of Genome Medical, Inc, a technology and services company providing genetics expertise to patients, providers, employers and care systems.
The remaining authors declare that the research was conducted in the absence of any commercial or financial relationships that could be construed as a potential conflict of interest.
Publisher's Note
All claims expressed in this article are solely those of the authors and do not necessarily represent those of their affiliated organizations, or those of the publisher, the editors and the reviewers. Any product that may be evaluated in this article, or claim that may be made by its manufacturer, is not guaranteed or endorsed by the publisher.
Acknowledgments
The authors would like to acknowledge the following members of the external review panel for their contribution to the development of categorization matrices: Dr. Sophie Desroches, Dr. Keith Grimaldi, Dr. Matthew Lebo, and Dr. David M. Mutch.
Supplementary Material
The Supplementary Material for this article can be found online at: https://www.frontiersin.org/articles/10.3389/fnut.2021.789215/full#supplementary-material
References
1. Osler W. On the educational value of the medical society. Boston Med Surg J. (1903) 148:275–9. doi: 10.1056/NEJM190303121481101
2. Sales NMR, Pelegrini PB, Goersch MC. Nutrigenomics: definitions and advances of this new science. J Nutr Metab. (2014) 2014:1–6. doi: 10.1155/2014/202759
3. Horne J, Nielsen D, Madill J, Robitaille J, Vohl M-C, Mutch D. Guiding global best practice in personalized nutrition based on genetics: the development of a nutrigenomics care map. J Acad Nutr Diet. (2021). doi: 10.1016/j.jand.2021.02.008. [Epub ahead of print].
4. Rozga M, Latulippe ME, Steiber A. Advancements in personalized nutrition technologies: guiding principles for registered dietitian nutritionists. J Acad Nutr Diet. (2020) 120:1074–85. doi: 10.1016/j.jand.2020.01.020
5. Görman U, Mathers JC, Grimaldi KA, Ahlgren J, Nordström K. Do we know enough? A scientific and ethical analysis of the basis for genetic-based personalized nutrition. Genes Nutr. (2013) 8:373–81. doi: 10.1007/s12263-013-0338-6
6. Loos RJF. From nutrigenomics to personalizing diets: are we ready for precision medicine? Am J Clin Nutr. (2019) 109:1–2. doi: 10.1093/ajcn/nqy364
7. Andrews J, Guyatt G, Oxman AD, Alderson P, Dahm P, Falck-Ytter Y, et al. GRADE guidelines: 14. going from evidence to recommendations: the significance and presentation of recommendations. J Clin Epidemiol. (2013) 66:719–25. doi: 10.1016/j.jclinepi.2012.03.013
8. CDC. ACCE Model Process for Evaluating Genetic Tests. (2021). Available online at: https://www.cdc.gov/genomics/gtesting/acce/index.htm (accessed February 15, 2021)
9. Zimmern RL. Testing challenges: evaluation of novel diagnostics and molecular biomarkers. Clin Med. (2009) 9:68–73. doi: 10.7861/clinmedicine.9-1-68
10. GRADE Handbook. Available online at: https://gdt.gradepro.org/app/handbook/handbook.html (accessed September 29, 2020)
11. Cochrane Collaboration. Our Evidence. Available online at: https://www.cochrane.org/evidence (accessed November 20, 2020)
12. PEN. PEN Evidence Grading Checklist. (2010). Available online at: http://www.pennutrition.com/pdf/PEN_Evidence_Grading_Dec_2010.pdf (accessed September 5, 2021).
13. Brouwers MC, Kho ME, Browman GP, Burgers JS, Cluzeau F, Feder G, et al. AGREE II: advancing guideline development, reporting and evaluation in health care. Can Med Assoc J. (2010) 182:E839–42. doi: 10.1503/cmaj.090449
14. National Academies of Sciences, Engineering, and Medicine, Health and Medicine Division, Board Board on Health Care Services, Board Board on the Health of Select Populations, Committee Committee on the Evidence Base for Genetic Testing. An Evidence Framework for Genetic Testing. An Evidence Framework for Genetic Testing. Washington, DC: National Academies Press (US) (2017). Available online at: http://www.ncbi.nlm.nih.gov/books/NBK425803/ (accessed November 20, 2020).
15. Sun F, Bruening W, Erinoff E, Schoelles KM. Addressing Challenges in Genetic Test Evaluation: Evaluation Frameworks and Assessment of Analytic Validity. Rockville, MD: Agency for Healthcare Research Quality (US) (2011). (AHRQ Methods for Effective Health Care). Available online at: http://www.ncbi.nlm.nih.gov/books/NBK56750/ (accessed November 20, 2020)
16. Goldman JS, Hahn SE, Catania JW, Larusse-Eckert S, Butson MB, Rumbaugh M, et al. Genetic counseling and testing for Alzheimer disease: joint practice guidelines of the American College of Medical Genetics and the National Society of Genetic Counselors. Genet Med. (2011) 13:597–605. doi: 10.1097/GIM.0b013e31821d69b8
17. Waggoner D. Yield of additional genetic testing after chromosomal microarray for diagnosis of neurodevelopmental disability and congenital anomalies: a clinical practice resource of the American College of Medical Genetics and Genomics (ACMG). Genet Med. (2018) 20:9. doi: 10.1038/s41436-018-0040-6
18. Pitini E, De Vito C, Marzuillo C, D'Andrea E, Rosso A, Federici A, et al. How is genetic testing evaluated? A systematic review of the literature. Europ J Hum Genet. (2018) 26:605–15. doi: 10.1038/s41431-018-0095-5
19. Irving M, Eramudugolla R, Cherbuin N, Anstey KJ. A critical review of grading systems: implications for public health policy. Eval Health Prof. (2017) 40:244–62. doi: 10.1177/0163278716645161
20. Grimaldi KA, van Ommen B, Ordovas JM, Parnell LD, Mathers JC, Bendik I, et al. Proposed guidelines to evaluate scientific validity and evidence for genotype-based dietary advice. Genes Nutr. (2017) 12:35. doi: 10.1186/s12263-017-0584-0
21. Khan KS, Kunz R, Kleijnen J, Antes G. Five steps to conducting a systematic review. J R Soc Med. (2003) 96:118–21. doi: 10.1258/jrsm.96.3.118
22. Moher D, Liberati A, Tetzlaff J, Altman D, The PRISMA Group. Preferred reporting items for systematic reviews and meta-analyses: the PRISMA statement. PLoS Med. (2009) 6:e1000097. doi: 10.1371/journal.pmed.1000097
23. Theodoratou E, Timofeeva M, Li X, Meng X, Ioannidis JPA. Nature, nurture, and cancer risks: genetic and nutritional contributions to cancer. Annu Rev Nutr. (2017) 37:293–320. doi: 10.1146/annurev-nutr-071715-051004
24. Shea BJ, Reeves BC, Wells G, Thuku M, Hamel C, Moran J, et al. AMSTAR 2: a critical appraisal tool for systematic reviews that include randomised or non-randomised studies of healthcare interventions, or both. BMJ. (2017) 358:j4008. doi: 10.1136/bmj.j4008
25. National Institute for Healthcare Excellence. Developing NICE guidelines: the manual. (2014). Retrieved from: https://www.nice.org.uk/process/pmg20/resources/developing-nice-guidelines-the-manual-pdf-72286708700869 (accesesed October 15, 2020).
26. Aromataris E, Munn Z, (editors.). JBI Manual for Evidence Synthesis. JBI (2020). Available online at: https://wiki.jbi.global/display/MANUAL doi: 10.46658/JBIMES-20-01 (accessed May 11, 2021)
27. Iowa Model Collaborative, Buckwalter KC, Cullen L, Hanrahan K, Kleiber C, McCarthy AM, et al. Iowa model of evidence-based practice: revisions and validation: iowa model-revised. Worldviews Evid Based Nurs. (2017) 14:175–82. doi: 10.1111/wvn.12223
28. Rich K. Clinical guidelines: grading the evidence behind the practice. J Vasc Nurs. (2012) 30:132–3. doi: 10.1016/j.jvn.2012.09.001
29. Mechanick J, Bergman D, Braithwaite S, Palumbo P. American association of clinical endocrinologists protocol for standardized production of clinical practice guidelines. Endocrine Pract. (2004) 10:353–61. doi: 10.4158/EP.10.4.353
30. Mechanick JI, Camacho PM, Cobin RH, Garber AJ, Garber JR, Gharib H, et al. American Association of clinical endocrinologists protocol for standardized production of clinical practice guidelines−2010 update. Endocrine Pract. (2010) 16:270–83. doi: 10.4158/EP.16.2.270
31. Mechanick JI, Camacho PM, Garber AJ, Garber JR, Pessah-Pollack R, Petak SM, et al. American association of clinical endocrinologists and american college of endocrinology protocol for standardized production of clinical practice guidelines, algorithms, and checklists - 2014. update and the AACE G4G program. Endocrine Pract. (2014) 20:692–702. doi: 10.4158/EP14166.PS
32. Mechanick JI, Pessah-Pollack R, Camacho P, Correa R, Figaro MK, Garber JR, et al. American association of clinical endocrinologists and american college of endocrinology protocol for standardized production of clinical practice guidelines, algorithms, and checklists – (2017). update Univ S C Dep music. Endocrine Pract. (2017) 23:1006–21. doi: 10.4158/EP171866.GL
33. Burke W, Zimmern R. Moving beyond ACCE: An Expanded Framework for Genetic Test Evaluation. PHG Foundation (2007). Available online at: https://www.phgfoundation.org/documents/369_1409657043.pdf (accessed February 15, 2021)
34. Calonge N, Green NS, Rinaldo P, Lloyd-Puryear M, Dougherty D, Boyle C, et al. Committee report: method for evaluating conditions nominated for population-based screening of newborns and children. Genet Med. (2010) 12:153–9. doi: 10.1097/GIM.0b013e3181d2af04
35. on behalf of the ACMG Laboratory Quality Assurance Committee, Richards S, Aziz N, Bale S, Bick D, Das S, et al. Standards and guidelines for the interpretation of sequence variants: a joint consensus recommendation of the American College of Medical Genetics and Genomics and the Association for Molecular Pathology. Genet Med. (2015) 17:405–23. doi: 10.1038/gim.2015.30
36. Owens DK, Lohr KN, Atkins D, Treadwell JR, Reston JT, Bass EB, et al. AHRQ Series Paper 5: grading the strength of a body of evidence when comparing medical interventions—Agency for Healthcare Research and Quality and the Effective Health-Care Program. J Clin Epidemiol. (2010) 63:513–23. doi: 10.1016/j.jclinepi.2009.03.009
37. Strande NT. Evaluating the clinical validity of gene-disease associations: an evidence-based framework developed by the clinical genome resource. Am J Hum Genet. (2017) 100:895–906. doi: 10.1016/j.ajhg.2017.04.015
38. Actionability – ClinGen. Clinical Genome Resource. Available online at: https://www.clinicalgenome.org/working-groups/actionability/ (accessed May 26, 2021)
39. Merlin T, Farah C, Schubert C, Mitchell A, Hiller JE, Ryan P. Assessing personalized medicines in Australia: a national framework for reviewing codependent technologies. Med Decis Making. (2013) 33:333–42. doi: 10.1177/0272989X12452341
40. Caudle K, Klein T, Hoffman J, Muller D, Whirl-Carrillo M, Gong L, et al. Incorporation of pharmacogenomics into routine clinical practice: the Clinical Pharmacogenetics Implementation Consortium (CPIC) guideline development process. CDM. (2014) 15:209–17. doi: 10.2174/1389200215666140130124910
41. Ciesielski TH, Pendergrass SA, White MJ, Kodaman N, Sobota RS, Huang M, et al. Diverse convergent evidence in the genetic analysis of complex disease: coordinating omic, informatic, and experimental evidence to better identify and validate risk factors. BioData Min. (2014) 7:10. doi: 10.1186/1756-0381-7-10
42. Treadwell JR, Tregear SJ, Reston JT, Turkelson CM. A system for rating the stability and strength of medical evidence. BMC Med Res Methodol. (2006) 6:52. doi: 10.1186/1471-2288-6-52
43. Teutsch SM, Bradley LA, Palomaki GE, Haddow JE, Piper M, Calonge N, et al. The Evaluation of Genomic Applications in Practice and Prevention (EGAPP) initiative: methods of the EGAPP working group. Genet Med. (2009) 11:12. doi: 10.1097/GIM.0b013e318184137c
44. Veenstra DL, Piper M, Haddow JE, Pauker SG, Klein R, Richards CS, et al. Improving the efficiency and relevance of evidence-based recommendations in the era of whole-genome sequencing: an EGAPP methods update. Genet Med. (2013) 15:14–24. doi: 10.1038/gim.2012.106
45. Food and Drug Administration. Guidance for Industry: Evidence-Based Review System for the Scientific Evaluation of Health Claims. (2009). Available online at: https://www.fda.gov/regulatory-information/search-fda-guidance-documents/guidance-industry-evidence-based-review-system-scientific-evaluation-health-claims#system (accessed September 5, 2021).
46. Hillier S, Grimmer-Somers K, Merlin T, Middleton P, Salisbury J, Tooher R, et al. FORM: an Australian method for formulating and grading recommendations in evidence-based clinical guidelines. BMC Med Res Methodol. (2011) 11:23. doi: 10.1186/1471-2288-11-23
47. Rousseau F, Lindsay C, Charland M, Labelle Y, Bergeron J, Blancquaert I, et al. Development and description of GETT: a genetic testing evidence tracking tool. Clin Chem Lab Med. (2010) 48:1397–407. doi: 10.1515/CCLM.2010.291
48. Guyatt G, Oxman AD, Akl EA, Kunz R, Vist G, Brozek J, et al. GRADE guidelines: 1. introduction—GRADE evidence profiles and summary of findings tables. J Clin Epidemiol. (2011) 64:383–94. doi: 10.1016/j.jclinepi.2010.04.026
49. Guyatt GH, Oxman AD, Schünemann HJ, Tugwell P, Knottnerus A. GRADE guidelines: a new series of articles in the Journal of Clinical Epidemiology. J Clin Epidemiol. (2011) 64:380–2. doi: 10.1016/j.jclinepi.2010.09.011
50. Lewin S, Booth A, Glenton C, Munthe-Kaas H, Rashidian A, Wainwright M, et al. Applying GRADE-CERQual to qualitative evidence synthesis findings: introduction to the series. Implementation Sci. (2018) 13:2, s13012–017–0688–3. doi: 10.1186/s13012-017-0689-2
51. Munthe-Kaas H, Bohren MA, Glenton C, Lewin S, Noyes J, Tunçalp Ö, et al. Applying GRADE-CERQual to qualitative evidence synthesis findings—paper 3: how to assess methodological limitations. Implementation Sci. (2018) 13:9. doi: 10.1186/s13012-017-0690-9
52. Colvin CJ, Garside R, Wainwright M, Munthe-Kaas H, Glenton C, Bohren MA, et al. Applying GRADE-CERQual to qualitative evidence synthesis findings—paper 4: how to assess coherence. Implementation Sci. (2018) 13:13. doi: 10.1186/s13012-017-0691-8
53. Ioannidis JP, Boffetta P, Little J, O'Brien TR, Uitterlinden AG, Vineis P, et al. Assessment of cumulative evidence on genetic associations: interim guidelines. Int J Epidemiol. (2008) 37:120–32. doi: 10.1093/ije/dym159
54. Callahan A, Cifuentes JJ, Dumontier M. An evidence-based approach to identify aging-related genes in Caenorhabditis elegans. BMC Bioinformatics. (2015) 16:40. doi: 10.1186/s12859-015-0469-4
55. WHO. IARC Monographs on the Evaluation of Carcinogenic Risks to Humans. (2006). Available online at: https://monographs.iarc.who.int/wp-content/uploads/2018/06/CurrentPreamble.pdf (accessed September 5, 2021).
56. Greer N, Mosser G, Logan G, Halaas G. A practical approach to evidence grading. Jt Comm J Qual Improv. (2000) 26:700–12. doi: 10.1016/S1070-3241(00)26059-8
57. Boffetta P, Winn DM, Ioannidis JP, Thomas DC, Little J, Smith GD, et al. Recommendations and proposed guidelines for assessing the cumulative evidence on joint effects of genes and environments on cancer occurrence in humans. Int J Epidemiol. (2012) 41:686–704. doi: 10.1093/ije/dys010
58. Burke W, Coughlin SS, Lee NC, Weed DL, Khoury MJ. Application of population screening principles to genetic screening for adult-onset conditions. Genet Test. (2001) 5:201–11. doi: 10.1089/10906570152742245
59. McShane LM, Cavenagh MM, Lively TG, Eberhard DA, Bigbee WL, Williams PM, et al. Criteria for the use of omics-based predictors in clinical trials: explanation and elaboration. BMC Med. (2013) 11:220. doi: 10.1186/1741-7015-11-220
60. Senol-Cosar O, Schmidt RJ, Qian E, Hoskinson D, Mason-Suares H, Funke B, et al. Considerations for clinical curation, classification, and reporting of low-penetrance and low effect size variants associated with disease risk. Genet Med. (2019) 21:2765–73. doi: 10.1038/s41436-019-0560-8
61. Schwingshackl L, Knüppel S, Schwedhelm C, Hoffmann G, Missbach B, Stelmach-Mardas M, et al. Perspective: NutriGrade: a scoring system to assess and judge the meta-evidence of randomized controlled trials and cohort studies in nutrition research. Adv Nutr. (2016) 7:994–1004. doi: 10.3945/an.116.013052
62. OCEBM Levels of Evidence. Centre for Evidence-Based Medicine (CEBM), University of Oxford. Available online at: https://www.cebm.ox.ac.uk/resources/levels-of-evidence/ocebm-levels-of-evidence (accessed March 10, 2021)
63. PharmGKB. Clinical Annotation Levels of Evidence. PharmGKB (2021). Available online at: https://www.pharmgkb.org/page/clinAnnLevels (accessed July 12, 2021)
64. Whirl-Carrillo M, McDonagh EM, Hebert JM, Gong L, Sangkuhl K, Thorn CF, et al. Pharmacogenomics knowledge for personalized medicine. Clin Pharmacol Ther. (2012) 92:414–7. doi: 10.1038/clpt.2012.96
65. Whirl-Carrillo M, Huddart R, Gong L, Sangkuhl K, Thorn CF, Whaley R, et al. An evidence-based framework for evaluating pharmacogenomics knowledge for personalized medicine. Clin Pharmacol Therap. (2021) 110:563–72. doi: 10.1002/cpt.2350
66. Harbour R, Miller J. A new system for grading recommendations in evidence based guidelines. BMJ. (2001) 323:334–6. doi: 10.1136/bmj.323.7308.334
67. Ebell MH, Siwek J, Weiss BD, Woolf SH, Susman J, Ewigman B, et al. Strength of recommendation taxonomy (SORT): a patient-centered approach to grading evidence in the medical literature. Am Fam Physician. (2004) 69:548–56.
68. Hornberger J, Doberne J, Chien R. Laboratory-Developed Test—SynFRAME: an approach for assessing laboratory-developed tests synthesized from prior appraisal frameworks. Genet Test Mol Biomarkers. (2012) 16:605–14. doi: 10.1089/gtmb.2011.0177
69. Harris RP, Helfand M, Woolf SH, Lohr KN, Mulrow CD, Teutsch SM, et al. Current methods of the U.S. Preventive Services Task Force. Am J Prev Med. (2001) 20:21–35. doi: 10.1016/S0749-3797(01)00261-6
70. Guirguis-Blake J, Calonge N, Miller T, Siu A, Teutsch S, Whitlock E, et al. Current processes of the U.S. Preventive Services Task Force: refining evidence-based recommendation development. Ann Intern Med. (2007) 147:117–22. doi: 10.7326/0003-4819-147-2-200707170-00170
71. U.S. Preventive Services Task Force. Grade Definitions. (2018). Available online at: https://www.uspreventiveservicestaskforce.org/uspstf/about-uspstf/methods-and-processes/grade-definitions (accessed July 12, 2021)
72. World Cancer Research Fund. Diet, Nutrition, Physical Activity and Cancer: A Global Perspective. (2018). Available online at: https://www.wcrf.org/wp-content/uploads/2021/02/Summary-of-Third-Expert-Report-2018.pdf (accessed September 5, 2021).
73. FAO, (editor.). Diet, Nutrition, and the Prevention of Chronic Diseases: Report of a WHO-FAO Expert Consultation. Geneva: World Health Organization (2003).
74. Study Quality Assessment Tools. NHLBI, NIH. Available online at: https://www.nhlbi.nih.gov/health-topics/study-quality-assessment-tools (accessed July 27, 2021)
75. Edwards SL, Beesley J, French JD, Dunning AM. Beyond GWASs: illuminating the dark road from association to function. Am J Hum Genet. (2013) 93:779–97. doi: 10.1016/j.ajhg.2013.10.012
76. Clarke B, Gillies D, Illari P, Russo F, Williamson J. The evidence that evidence-based medicine omits. Prevent Med. (2013) 57:745–7. doi: 10.1016/j.ypmed.2012.10.020
77. Dekkers OM, Elm E von, Algra A, Romijn JA, Vandenbroucke JP. How to assess the external validity of therapeutic trials: a conceptual approach. Int J Epidemiol. (2010) 39:89–94. doi: 10.1093/ije/dyp174
78. Yip DK-S, Chan LL, Pang IK, Jiang W, Tang NLS, Yu W, et al. A network approach to exploring the functional basis of gene–gene epistatic interactions in disease susceptibility. Stegle O, editor. Bioinformatics. (2018) 34:1741–9. doi: 10.1093/bioinformatics/bty005
79. Helgadottir A, Manolescu A, Helgason A, Thorleifsson G, Thorsteinsdottir U, Gudbjartsson DF, et al. A variant of the gene encoding leukotriene A4 hydrolase confers ethnicity-specific risk of myocardial infarction. Nat Genet. (2006) 38:68–74. doi: 10.1038/ng1692
80. Dong Q, Zhang W, Wu J, Li B, Schron EH, McMahon T, et al. Applying surface-based hippocampal morphometry to study APOE-E4 allele dose effects in cognitively unimpaired subjects. NeuroImage: Clin. (2019) 22:101744. doi: 10.1016/j.nicl.2019.101744
81. Vallée Marcotte B, Guénard F, Lemieux S, Couture P, Rudkowska I, Calder PC, et al. Fine mapping of genome-wide association study signals to identify genetic markers of the plasma triglyceride response to an omega-3 fatty acid supplementation. Am J Clin Nutr. (2019) 109:176–85. doi: 10.1093/ajcn/nqy298
82. Teare MD, Knevel R, Morgan MD, Kleszcz A, Emery P, Moore DJ, et al. Allele-dose association of the C5orf30 rs26232 Variant with joint damage in rheumatoid arthritis. Arthritis Rheumat. (2013) 65:2555–61. doi: 10.1002/art.38064
83. The GRADE Working Group, Atkins D, Eccles M, Flottorp S, Guyatt GH, Henry D, et al. Systems for grading the quality of evidence and the strength of recommendations I: critical appraisal of existing approaches the GRADE Working Group. BMC Health Serv Res. (2004) 4:38. doi: 10.1186/1472-6963-4-38
84. de Toro-Martín J, Arsenault BJ, Després J-P, Vohl M-C. Precision nutrition: a review of personalized nutritional approaches for the prevention and management of metabolic syndrome. Nutrients. (2017) 9:913. doi: 10.3390/nu9080913
85. Figueiredo JC, Hsu L, Hutter CM, Lin Y, Campbell PT, Baron JA, et al. Genome-wide diet-gene interaction analyses for risk of colorectal cancer. PLoS Genet. (2014) 10:e1004228. doi: 10.1371/journal.pgen.1004228
86. Rogers PJ, Hohoff C, Heatherley SV, Mullings EL, Maxfield PJ, Evershed RP, et al. Association of the anxiogenic and alerting effects of caffeine with ADORA2A and ADORA1 polymorphisms and habitual level of caffeine consumption. Neuropsychopharmacology. (2010) 35:1973–83. doi: 10.1038/npp.2010.71
87. Renda G, Committeri G, Zimarino M, Di Nicola M, Tatasciore A, Ruggieri B, et al. Genetic determinants of cognitive responses to caffeine drinking identified from a double-blind, randomized, controlled trial. Eur Neuropsychopharmacol. (2015) 25:798–807. doi: 10.1016/j.euroneuro.2015.03.001
88. Childs E, Hohoff C, Deckert J, Xu K, Badner J, de Wit H. Association between ADORA2A and DRD2 Polymorphisms and caffeine-induced anxiety. Neuropsychopharmacology. (2008) 33:2791–800. doi: 10.1038/npp.2008.17
89. Alsene K, Deckert J, Sand P, de Wit H. Association between A 2a receptor gene polymorphisms and caffeine-induced anxiety. Neuropsychopharmacology. (2003) 28:1694–702. doi: 10.1038/sj.npp.1300232
90. Merritt DC, Jamnik J, El-Sohemy A. FTO genotype, dietary protein intake, and body weight in a multiethnic population of young adults: a cross-sectional study. Genes Nutr. (2018) 13:4. doi: 10.1186/s12263-018-0593-7
91. Zhang X, Qi Q, Zhang C, Smith SR, Hu FB, Sacks FM, et al. FTO genotype and 2-year change in body composition and fat distribution in response to weight-loss diets: the POUNDS LOST trial. Diabetes. (2012) 61:3005–11. doi: 10.2337/db11-1799
92. Pavlidis C, Nebel J-C, Katsila T, Patrinos GP. Nutrigenomics 2.0: the need for ongoing and independent evaluation and synthesis of commercial nutrigenomics tests' scientific knowledge base for responsible innovation. OMICS. (2016) 20:65–8. doi: 10.1089/omi.2015.0170
93. Hickey SE, Curry CJ, Toriello HV. ACMG practice guideline: lack of evidence for MTHFR polymorphism testing. Genet Med. (2013) 15:153–6. doi: 10.1038/gim.2012.165
94. Buckland PR. The importance and identification of regulatory polymorphisms and their mechanisms of action. Biochim Biophys Acta. (2006) 1762:17–28. doi: 10.1016/j.bbadis.2005.10.004
95. Lin JS, Thompson M, Goddard KA, Piper MA, Heneghan C, Whitlock EP. Evaluating genomic tests from bench to bedside: a practical framework. BMC Med Inform Decis Mak. (2012) 12:117. doi: 10.1186/1472-6947-12-117
Keywords: nutrition, genetics, nutrigenetics/nutrigenomics, nutritional genomics, systematic review, clinical practice, scientific validity, frameworks
Citation: Keathley J, Garneau V, Zavala-Mora D, Heister RR, Gauthier E, Morin-Bernier J, Green R and Vohl M-C (2021) A Systematic Review and Recommendations Around Frameworks for Evaluating Scientific Validity in Nutritional Genomics. Front. Nutr. 8:789215. doi: 10.3389/fnut.2021.789215
Received: 04 October 2021; Accepted: 26 November 2021;
Published: 14 December 2021.
Edited by:
Joanne B. Cole, Massachusetts General Hospital and Harvard Medical School, United StatesReviewed by:
Jim Kaput, Independent Researcher, Lausanne, SwitzerlandKeith Anthony Grimaldi, Institute of Communication and Computer Systems, Greece
Copyright © 2021 Keathley, Garneau, Zavala-Mora, Heister, Gauthier, Morin-Bernier, Green and Vohl. This is an open-access article distributed under the terms of the Creative Commons Attribution License (CC BY). The use, distribution or reproduction in other forums is permitted, provided the original author(s) and the copyright owner(s) are credited and that the original publication in this journal is cited, in accordance with accepted academic practice. No use, distribution or reproduction is permitted which does not comply with these terms.
*Correspondence: Marie-Claude Vohl, bWFyaWUtY2xhdWRlLnZvaGxAZnNhYS51bGF2YWwuY2E=