- 1Department of Human Health and Nutritional Sciences, University of Guelph, Guelph, ON, Canada
- 2Department of Mathematics and Statistics, University of Guelph, Guelph, ON, Canada
- 3Department of Family Relations and Applied Nutrition, University of Guelph, Guelph, ON, Canada
Adopting a healthy diet remains central for the prevention of obesity. In adults, higher intake of ultra-processed food is associated with a greater risk of overweight and obesity. However, little is known about the degree of food processing and its association with anthropometric measures in families with preschool-aged children, a critical period for the development of dietary patterns. This cross-sectional study included preschool-aged children (n = 267) between 1.5 and 5 years of age and their parents (n = 365) from 242 families enrolled in the Guelph Family Health Study. Dietary assessment was completed using ASA24-Canada-2016. Foods and beverages were classified based on their degree of food processing using the NOVA Classification (unprocessed or minimally processed foods, processed culinary ingredients, processed foods, and ultra-processed foods). Associations between the energy contribution (% kcal) of each NOVA category and anthropometric measures were examined using linear regression models with generalized estimating equations, adjusted for sociodemographic variables. The energy contribution of ultra-processed foods was the highest relative to the other NOVA categories among parents (44.3%) and children (41.3%). The energy contribution of unprocessed or minimally processed foods was 29.1% for parents and 35.3% for children, processed foods was 24.0% for parents and 21.3% for children, and processed culinary ingredients was 2.6% for parents and 2.1% for children. Ultra-processed foods (% kcal) were positively associated with BMI (β = 0.04, 95% CI: 0.01–0.07, P = 0.02), waist circumference (β = 0.11, 95% CI: 0.03–0.18, P = 0.008) and body weight (β = 0.13, 95% CI: 0.03–0.22, P = 0.01) in parents, but not children. Unprocessed foods (% kcal) were negatively associated with waist circumference in parents (β = −0.09, 95% CI: 0.18–0.01, P = 0.03) and children (β = −0.03, 95% CI: 0.05–0.01, P = 0.01), as well as body weight (β = −0.12, 95% CI: 0.23–0.00, P = 0.04) in parents. The degree of food processing primarily influenced anthropometric outcomes in parents. Nevertheless, diets of children were similar, suggesting that such exposure in families may eventually lead to outcomes observed in parents.
Introduction
Obesity is a public health problem and the increased prevalence of obesity worldwide is driven in part by changes in the global food system, replacing dietary patterns based on home-prepared foods with industrially processed and pre-packaged foods (1). Evidence suggests that a greater intake of ultra-processed foods is associated with obesity and related cardiometabolic outcomes (2, 3). Ultra-processed foods are defined by the NOVA food classification system as industrial formulations of ingredients derived from additives and food substances (4). The NOVA system is a diet classification tool that considers the nature, purpose and extent of food processing when classifying foods and beverages into four categories that range from least to most processed and include: unprocessed or minimally processed foods (e.g., whole foods, fruits, vegetables, eggs, and milk); processed culinary ingredients (e.g., sugar, salt, butter, and cooking oil); processed foods (e.g., salted nuts, simple breads, and cheese); ultra-processed foods (e.g., pre-packaged meals and breads, sugary drinks and sweetened or salty snacks) (4). Ultra-processed foods are typically energy-dense and characterized as having poor nutritional content, including higher levels of sodium, free and added sugars and saturated fats, compared to their unprocessed or minimally processed counterparts (4).
Data from household food purchases reveal an increase in ultra-processed food sales globally (5). This rise in household availability of ultra-processed foods parallels the increased prevalence of overweight and obesity (5, 6). Clinical and observational studies have also identified a potential association between ultra-processed food consumption and increased obesity risk. For example, results of a randomized controlled trial demonstrated that an ultra-processed diet caused weight gain in adults relative to an unprocessed diet despite being matched for calories (7). Cross-sectional studies reported similar findings linking higher intakes of ultra-processed foods to increased prevalence of obesity in both adults and children (8–12). Similar associations between ultra-processed food intakes and anthropometric measures or related risk markers have been identified in longitudinal studies in adults (13–17) and children (18–20). Recent systematic reviews and meta-analyses also confirm the overarching finding that ultra-processed foods are positively associated with excess body weight and obesity in adolescents and adults (21, 22) and additional factors related to cardiometabolic risk in children and adults (23). However, the role of the different degrees of food processing (ranging from unprocessed to ultra-processed) on indicators of obesity in children remains unknown.
Further, although children are the leading consumers of ultra-processed foods (24–26), few studies have explored the association between the degree of food processing and obesity risk in preschool-aged children. The focus on young children is important as early dietary behaviors may track into adulthood, potentially playing a role in the development of chronic diseases later in life (27). The home food environment also influences the development of early dietary patterns, underscoring the importance of research within the family unit (27–29). Thus, assessing dietary intake in families, including both parents and children may provide unique perspectives of the role of the family environment in shaping children’s food choices and dietary habits. Since the most effective programs for addressing and preventing childhood obesity are family based, insights into the dietary intakes of parents and children may provide the basis of future diet and weight-related behavior change interventions (30). Therefore, the aim of this study was to investigate the associations between food intake according to the degree of food processing and anthropometric indicators of obesity in Canadian preschool-aged children and their parents.
Materials and methods
Study design and participants
The Guelph Family Health Study (GFHS) is a longitudinal health promotion study investigating early life risk factors for obesity and chronic diseases in families with young children. This cross-sectional study collected data from families participating in the GFHS between April 2017 and March 2020. Families were recruited through the local Family Health Team, Community Health Centre, and Ontario Early Years Centres if they had at least one child between the ages 1.5–5 years, resided in Guelph-Wellington in Ontario, Canada with no plans of relocating within next year, and could respond to questionnaires in English. Parents provided written informed consent and the University of Guelph Research Ethics Board approved the study (REB #17-07-003).
A total of 246 families (749 participants; 427 parents, 322 children) were enrolled in the GFHS. Of these, 117 participants were excluded from the current analyses due to the following: missing dietary data (37 parents, 28 children), implausible energy intakes (>1.5 times the interquartile range below the 25th or above the 75th percentiles; nine parents, 17 children), pregnancy or breastfeeding (16 mothers), breastfed (nine children), as intake amounts could not be verified, and illness (one child). The final sample for this study included n = 365 parents and n = 267 children from 242 families.
Dietary assessment
Parents reported dietary intake for themselves and parent 1 (defined as the first parent enrolled in the study, 90% mothers) reported dietary intake on behalf of their participating child(ren). Dietary intake data was evaluated for energy intakes using the National Cancer Institute’s Automated Self-Administered 24-h (ASA24) Dietary Assessment Tool, version 2016 adapted for use in the Canadian population. The ASA24 is a self-administered, web-based 24-h dietary recall program that has been validated for use among adults (31) and preschool-aged children (32). The ASA24 was derived from the USDA’s Automated Multiple-Pass Method (AMPM), providing a modified approach to traditional interviewer-administered 24-h recalls. The ASA24 uses food images to assist respondents with portion size estimation and provides nutrient content for foods, beverages, and supplements.
Classification of foods by the degree of processing
Foods reported in the 24-h recall were manually classified according to the degree of processing according to the four NOVA classification system categories: unprocessed or minimally processed foods, including naturally present “fresh” or “whole” foods altered by methods that do not require the addition of substances such as salts, sugars, oils or fats (e.g., fresh, frozen or dry fruits and vegetables, packaged grains, legumes, fresh or frozen meat and fish, eggs and plain milk); processed culinary ingredients, found in home kitchens to cook/season foods and make dishes palatable (e.g., starch, table sugar, salt, lard, butter, and oils); processed foods, described as products made by adding processed culinary ingredients such as salt, sugars and/or oils to unprocessed or minimally processed foods (e.g., artisan breads and cheeses, canned fish, salted meat, fruit preserves and vegetables in brine); ultra-processed foods, defined as ready-to-eat food products made with industrial formulations of ingredients and additives, containing minimal or no whole foods (e.g., soft drinks, sweetened or salted pre-packaged snacks, sweetened breakfast cereals, mass-produced breads, processed meats and ready-to-eat frozen or shelf-stable meals) (4, 33, 34). This work was completed by one trained researcher using a pre-constructed standard operating procedure that was independently reviewed by multiple researchers.
Automated Self-Administered 24-h “Food Description” and “Food Source” variables were used to identify the degree of food processing by providing information about the preservation processes (e.g., fresh, frozen, dried or canned in own juice, oil, water or syrup), production methods (e.g., home, bakery, or industrially prepared), addition of ingredients (e.g., sweetened, salted, or unsalted) and source of foods (e.g., fast food or grocery store). In cases of ambiguity, food items were coded under the lesser processed category. Zero-calorie foods (e.g., water) were not classified and excluded from the analyses. Since the reporting of recipes was not required in ASA24, home-made mixed dishes were classified as “un-disaggregated home-made dish” under the unprocessed or processed foods categories, depending on the processing level of the core ingredients (35). Energy intake from foods was quantified and reported in either absolute (kcal/day) and/or relative values (% kcal/day).
Anthropometric indicators of obesity
Anthropometric measures, including body weight, height and waist circumference were obtained at the University of Guelph Body Composition Laboratory. The measurements were performed by trained research staff and under standard conditions, with participants standing and either barefoot or in socks. Body weight (kg) was measured to the nearest 0.001 kg using a calibrated electronic weighing scale (BOD POD™, COSMED USA Inc., Concord, CA, USA). Height (cm) was measured to the nearest 0.1 cm using a calibrated wall-mounted stadiometer (Seca Model 222, Mount Pleasant, SC, USA) for parents and older children or a ShorrBoard pediatric measuring board (ShorrBoard®, Weigh and Measure LLC., Olney, MD, USA) for younger children. Waist circumference (cm) was measured to the nearest 0.1 cm at the top of the right iliac crest using a Gulick II measuring tape (Gulick II, Country Technology Inc., Gay Mills, WI, USA). Two measures were taken for height and waist circumference; if the difference between the values was greater than 0.5 cm, a third measure was taken and the mean of the nearest two values was reported as the final value. BMI [weight (kg)/height (m)2] was calculated for the parents. BMI z-scores, measures of relative weight adjusted for child age and sex, were calculated for children based on the WHO Child Growth Standards using the R package “zscorer” version 0.3.1 statistical software (36).
Percent fat mass was measured by trained research staff using the BOD POD™ digital scale (COSMED USA Inc., Concord, CA, USA) for parents or during bioelectrical impedance analysis (BIA) using a Quantum IV BIA Analyzer System (RJL Systems, Clinton Township, MI, USA) for children. Participants were instructed to avoid food and drink and vigorous physical activity for 2 h (parents) or 30 min (children) prior to the assessment. Two BIA measurements were taken; if the difference between the two resistance values was greater than 5%, a third measurement was taken. Percent fat mass from the BIA assessment for children was estimated using total body water calculation by Kushner et al. (37) and hydration constants by Fomon et al. (38).
BMI or BMI z-scores for 36 participants (n = 11 parents and n = 25 children), waist circumference data for 38 participants (n = 8 parents and n = 30 children), body weight for 16 participants (n = 11 parents and n = 4 children) and percent fat mass data for 205 participants (n = 141 parents and n = 64 children) were missing and excluded from the regression analyses.
Statistical analysis
Data were analyzed using SAS University Edition version 3.6 (SAS Institute Inc., Cary, NC, USA) (39). Linear regression models with generalized estimating equations were fitted to estimate the associations between food intake according to the degree of food processing and obesity indicators (BMI or BMI z-scores, waist circumference, body weight and percent fat mass). Generalized estimating equations were used to obtain coefficient estimates (β), 95% confidence intervals (CIs), and P-values that account for dependence among participants within the same family (40). Anthropometric measures (BMI or BMI z-scores, waist circumference, body weight and percent fat mass) of parents and children were independently regressed onto each processed food category, expressed as percent of total energy. Analyses were conducted separately for parents and children. Models were adjusted for variables that were identified as potential confounders including age (years), sex, annual household income (<$50,000; $50,000–$99,999; $100,000–$149,999; $150,000 or more; Did not disclose), ethnicity (White; Other, including Black, Chinese, Japanese, Korean, Latin American, Mixed ethnicity, South Asian, Southeast Asian, and West Asian; or Did not disclose) and education for parent models (no postsecondary degree; postsecondary graduate; postgraduate training), or highest level of parental education for child models.
Results
Participant characteristics and energy intake
Participant characteristics are reported in Table 1. Among the total sample of 365 parents and 267 children, 59% were mothers (n = 216) and 52% were girls (n = 138). The mean age was 35.7 (SD = 4.7) years among parents and 3.6 (SD = 1.2) years among children. Approximately 80% of parents and 75% of children identified as White. A total of 49% of parents (n = 179) reported an annual household income of $100,000 or greater and about 35% (n = 127) obtained postgraduate training or degrees. The mean BMI or BMI z-score value for parents and children was 26.8 (SD = 6.1) and 0.5 (SD = 0.8), respectively. The mean waist circumference was 92.8 (SD = 15.1) for parents and 51.1 (SD = 3.3) for children. The mean percent fat mass values were 29.4 for both parents (SD = 9.9) and children (SD = 5.5). The mean daily energy intake was 2211.9 (SD = 859.5) kcal for parents and 1408.9 (SD = 381.2) kcal for children.
Distribution of energy intakes according to the degree of food processing
The dietary contribution of ultra-processed foods to total energy intake was the highest among the processed food categories, for both parents and children (Table 2). Ultraprocessed foods represented 44.3 and 41.3% of total energy intake among parents and children, respectively. Collectively, ready-to-eat meals, breads, and sweet desserts and baked goods accounted for almost half (20.6% of 44.3%) of the energy from ultra-processed foods in the parents’ diets. Breads (6.7%), sweet snacks (5.6%), and sweetened milk-based products (5.3%) were the greatest contributors of energy from ultra-processed foods in the children’s diets.
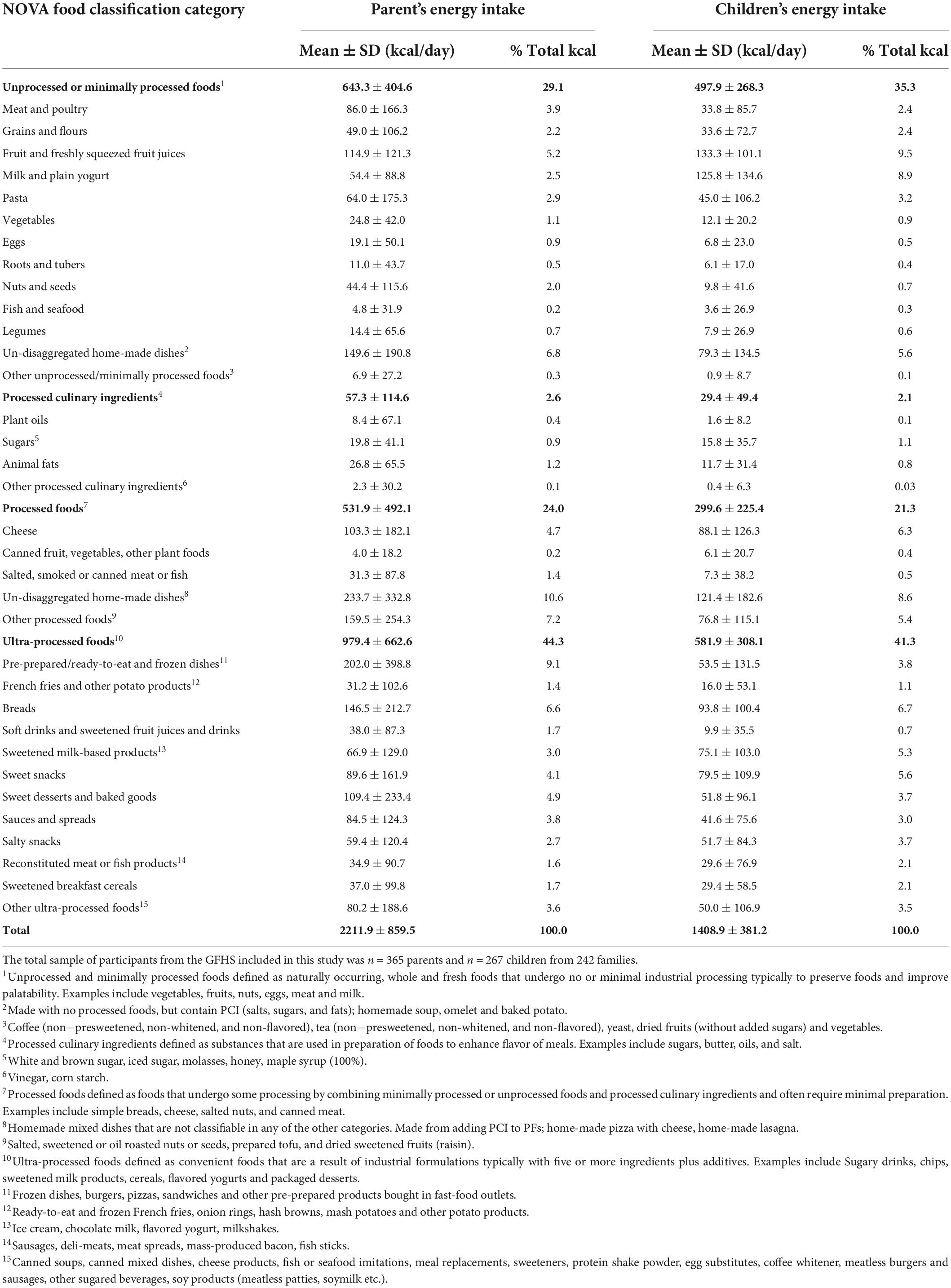
Table 2. Distribution of energy intake among NOVA food classification categories among participants in the Guelph Family Health Study (n = 365 parents and n = 267 children from 242 families).
Unprocessed or minimally processed foods represented 29.1% of total energy intake in parents’ diets and 35.3% in the children’s diets. Home-made dishes (6.8%) and fruit and freshly squeezed fruit juices (5.2%) in the parents’ diets, and fruit and freshly squeezed fruit juices (9.5%) and milk and plain yogurt (8.9%) in the children’s diets provided the greatest energy from unprocessed or minimally processed foods.
Processed foods provided 24.0 and 21.3% of total energy in the parents’ and children’s diets, respectively, with processed home-made dishes contributing the greatest source of energy for both parents (10.6%) and children (8.6%).
Processed culinary ingredients accounted for 2.6% of energy intake in the parents’ diets and 2.1% of energy intake in the children’s diets. Animal fats (1.2%) and sugars (1.1%) were the main contributors of processed culinary ingredients in the diets of parents and children, respectively.
Associations between the degree of food processing and anthropometric indicators of obesity
For parents, ultra-processed foods (% kcal) were positively associated with BMI (β = 0.04, 95% CI: 0.01–0.07, P = 0.02), waist circumference (β = 0.11, 95% CI: 0.03–0.18, P = 0.008) and body weight (β = 0.13, 95% CI: 0.03–0.22, P = 0.01), with a borderline result seen for percent fat mass (β = 0.05, 95% CI: 0.00–0.11, P = 0.054) (Table 3). No significant associations were seen between ultra-processed food intake and anthropometric measures in children (Table 4). However, processed foods (%) were positively associated with BMI z-scores (β = 0.008, 95% CI: 0.001–0.015, P = 0.04) in children, but not BMI in parents. In contrast, unprocessed or minimally processed foods (% kcal) were negatively associated with waist circumference in parents (β = −0.09, 95% CI: 0.18–0.01, P = 0.03) and children (β = −0.03, 95% CI: 0.05–0.01, P = 0.01). Unprocessed or minimally processed foods (% kcal) were also negatively associated with body weight in parents (β = −0.12, 95% CI: 0.23–0.00, P = 0.04). No additional significant associations between processed foods or processed culinary ingredients and anthropometric measures were seen in parents or children.
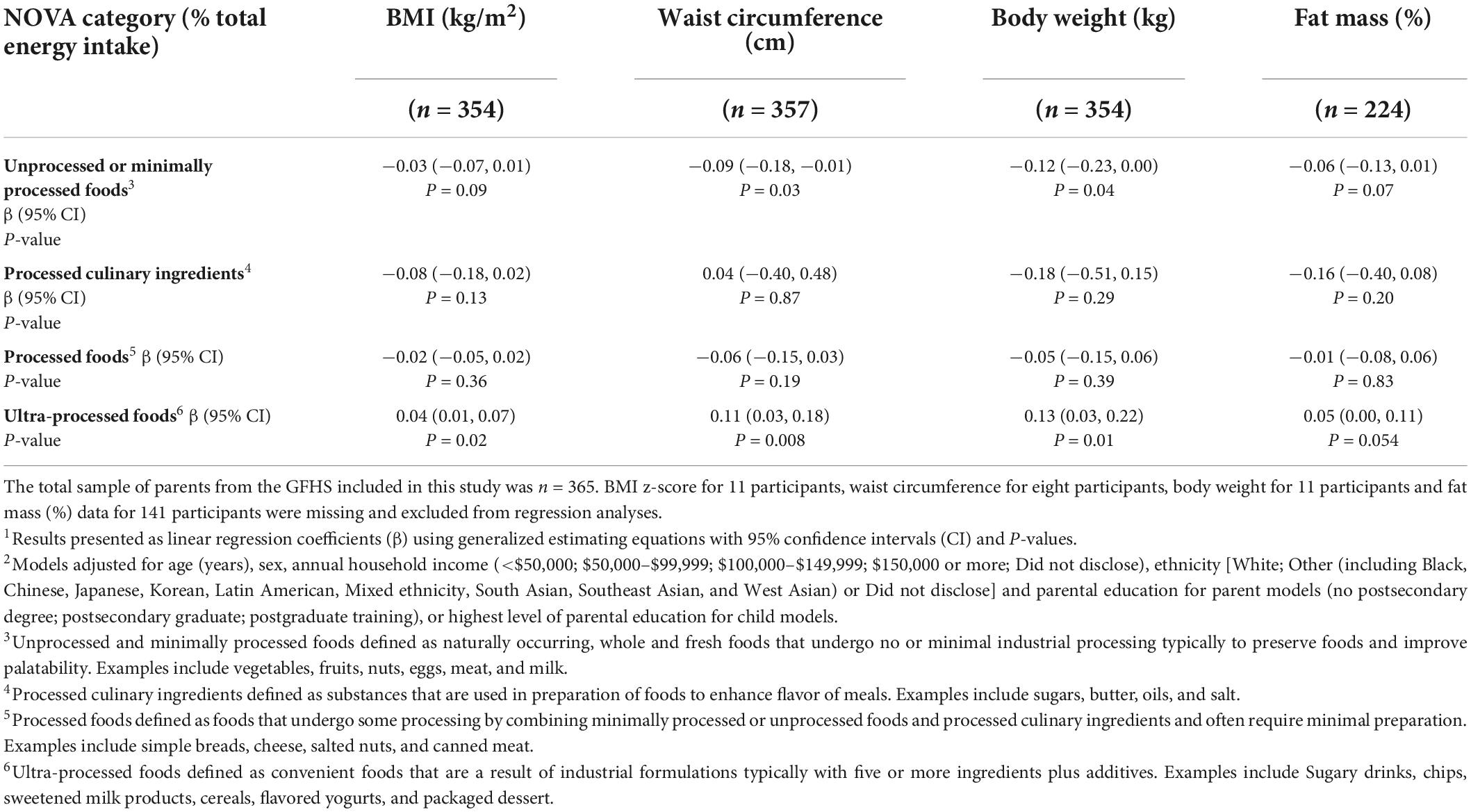
Table 3. Association between the intakes of the NOVA food classification categories and anthropometric indicators of obesity1,2 among parents (n = 365 participants from 242 families).
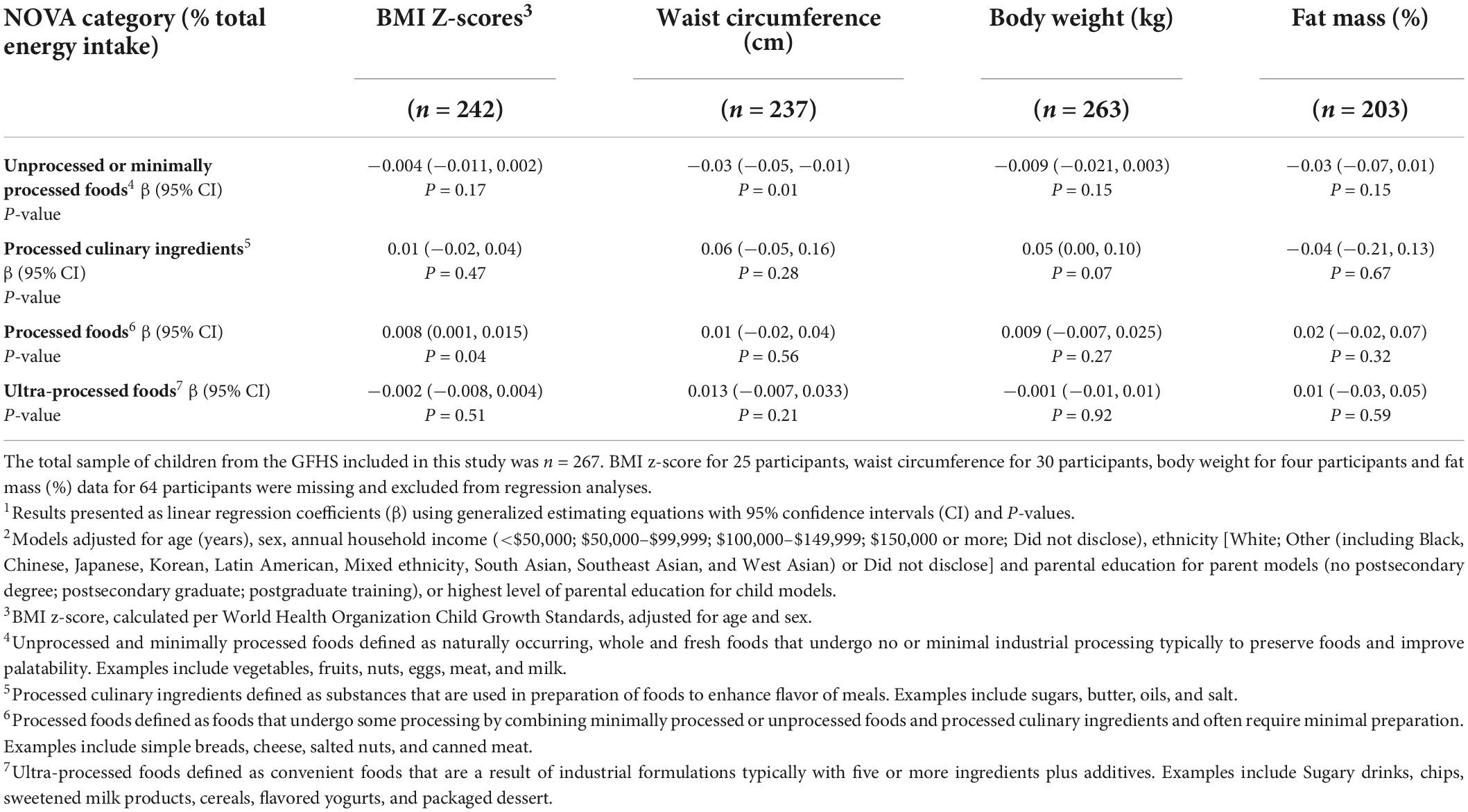
Table 4. Association between the intakes of the NOVA food classification categories and anthropometric indicators of obesity1,2 among preschool-aged children (n = 267 participants from 242 families).
Discussion
This cross-sectional study examined associations between the degree of food processing and anthropometric measures of obesity among a sample of Canadian families with young children. The results of this study showed that ultra-processed foods accounted for a greater proportion of energy intake relative to the other less processed food categories among both parents (44%) and preschoolers (41%). Ultra-processed food intake was positively associated with a small but significant increase in BMI, waist circumference, and body weight, as well as a marginally significant increase in percent body fat in parents, but not children. Unprocessed or minimally processed foods were inversely associated with waist circumference in both parents and children, and body weight in parents only. Processed foods were positively associated with BMI z-scores in children, but no further associations with anthropometric measures were noted in parents or children.
The findings of this current study are consistent with existing research highlighting a associations between excess ultra-processed food consumption and weight gain, and conversely, unprocessed food consumption and weight loss, in adults (7). In a recent randomized controlled trial, Hall et al. (7) found that inpatients who consumed an ultra-processed diet for 2 weeks gained 0.9 ± 0.3 kg and had higher ad libitum energy intake relative to an unprocessed diet, whereas patients lost, on average 0.9 ± 0.3 kg while consuming an unprocessed diet. In support of our results, cross-sectional associations between ultra-processed food intake and obesity have been reported among adults from the USA (41), Canada (42), Australia (10), and Brazil (43). Findings from recent prospective studies also revealed that greater ultra-processed food intake was associated with increased incidence of obesity or weight gain among Brazilian (14), UK (13), Spanish (15), and French adults (16), as well as increased visceral fat deposition in overweight or obese older adults (aged 55–75 years old) with metabolic syndrome (17). Along with a greater risk of obesity, greater ultra-processed food intake has been associated with an increased risk of all-cause mortality and other diet-related non-communicable diseases including type 2 diabetes, hypertension and cancer (7, 44, 45). Conversely, a diet high in unprocessed or minimally processed foods (e.g., fruits, vegetables, nuts, seeds, whole grains, and fish) has been observed to have a protective effect on cardiometabolic health (46). Therefore, decreasing ultra-processed food consumption and increasing unprocessed foods in the diet may be effective health promotion strategies.
In contrast to adult studies, the association between the degree of food processing and obesity measures among children have been inconsistent (9, 47). Prospective studies found that greater ultra-processed food intake was associated with greater waist circumference in young children (aged 4–8 years old) (19), and increased adiposity trajectories tracing into early adulthood (from ages 7 to 24 years) (20). In contrast, cross-sectional studies in school-aged children and adolescents found no associations between ultra-processed or unprocessed food consumption and BMI (48) or additional indicators of obesity, including waist circumference and waist-to-height ratio (49), whereas another cross-sectional study identified a significant association between the consumption of unprocessed or minimally processed foods and excess weight among adolescents, but not ultra-processed foods (50). Our study found that energy intake from ultra-processed foods was not significantly associated with measures of obesity including BMI, waist circumference, body weight, or percent body fat in children. However, processed foods were significantly associated with BMI z-scores in children, but this small positive association may not be biologically relevant. In particular, un-disaggregated culinary preparations, which represent an important part of energy consumed by children, may be influencing the association between the consumption of processed foods and BMI z-scores. Significant inverse associations between unprocessed or minimally processed food intake and waist circumference were also identified in children. One possible explanation for the absence of this relationship in children may be due to their young age, as preschool-aged children are also rapidly growing. As the effect of ultra-processed foods was small, it may be difficult to disentangle this from normal growth (51).
While the underlying mechanisms driving the associations between the degree of food processing and risk of obesity are yet to be fully elucidated, there are several plausible mechanisms. According to the protein leverage hypothesis, the overconsumption of ultra-processed foods is driven by the need to minimize variations in absolute protein intake as a result of the reduction in dietary protein density of these foods (52). Thus, the resulting energy overconsumption from increased intake of low-protein ultra-processed foods may drive weight gain. Another possible explanation for the influence of ultra-processed foods on obesity risk is the tendency of these foods to displace nutrient-dense, unprocessed or minimally processed foods in the diet and thus, promote poor dietary patterns (53, 54). The high palatability, convenience, affordability and lower satiety of these foods may also facilitate over-eating and weight gain (53–56). A recent study proposed that compared to unprocessed foods, ultra-processed foods have a greater “energy intake rate” (a measure of energy density combined with eating rate that quantifies the rate at which energy from foods is consumed), which may further promote excess energy intakes (57). However, the proposed mechanisms by which ultra-processed foods may be related to anthropometric measures warrant further study. Further, along with dietary intake, other factors which were not examined in the current study, including physical activity, sedentary behavior, smoking status, alcohol consumption status, lipid profiles, genetics, and psychological factors, also contribute to the development of obesity and related diseases (49, 58–61).
The present study found that ultra-processed foods contributed over 40% of total energy intake in the diet of both parents and children. The relatively high intake of ultra-processed foods found in this study is also supported by previous reports (62–64). Data on household food acquisition of ultra-processed foods from countries including Canada, Brazil, Mexico, Taiwan, and Sweden showed marked increases in the contribution of ultra-processed foods, and consequent decreases in the contribution of unprocessed foods in the diet (65–69). Nationally representative dietary surveys also confirmed that ultra-processed foods represent half of the total energy in the diet of high-income countries including the USA (56%) (70), Canada (48%) (25), and UK (57%) (45). The findings of our study corroborate this data, as ultra-processed foods comprised a greater proportion of energy intake in the diet of Canadian households of middle to high-income parents (44%) and their preschool-aged children (41%), relative to the other less processed food categories. The high intakes of ultra-processed foods among preschool-aged children in our study is concerning since dietary patterns during early years of life may shape food preferences in adulthood, which could translate into the development of chronic diseases associated with poor diet (27). Further, the overall dietary intake of children in our study closely resembled the energy intake values of parents, highlighting the importance of assessing dietary patterns within the family unit. In support of our findings, studies have shown that parental dietary patterns and the food environment influence children’s feeding behaviors (28, 71). Thus, the family environment may facilitate the consumption of ultra-processed foods and should be taken into account when designing nutrition intervention strategies to elicit behavior changes (30).
This study contributes to our understanding of the associations between the degree of food processing and anthropometric indicators of obesity among a unique family based cohort of preschool-aged children. Our study explored cross-sectional associations in both parents and children, providing additional evidence for the importance of the family environment in shaping the early life dietary preferences of children. In addition, the use of individual-level dietary data in this study, as opposed to household surveys of food purchases, provides data that is more reflective of the current diet. Although this study employs a novel approach examining the association between the degree of food processing and obesity risk in a family based cohort, some limitations should be considered. Our sample included predominantly White participants from middle to high-income households and so, the generalizability of these findings to ethnically diverse and low-income households may be limited. Also, dietary reporting using the self-administered ASA24 may be subject to recall bias and underreporting of foods deemed less healthful by participants due to social desirability bias. However, these concerns are mitigated as the ASA24 is a validated dietary assessment tool for use in both adults and children. Further, culinary preparations were not disaggregated as participants were not required to report detailed recipes. Instead, culinary preparations were classified as unprocessed or minimally processed foods or processed foods, thereby potentially overestimating the dietary contribution of these categories and underestimating the contribution of processed culinary ingredients and ultra-processed foods. Due to the cross-sectional nature of our analyses, the potential causal mechanisms underlying the results of this study and the longitudinal effects of the associations between the degree of food processing and anthropometric measures in families require further study.
Conclusion
Our study found relatively high intakes of ultra-processed foods in the diets of Canadian parents and their preschool-aged children. Ultra-processed foods were found to be positively associated with anthropometric indicators of obesity in parents, but not children. Unprocessed foods were inversely associated with abdominal obesity in both parents and children, and body weight in parents only. The overall findings from this study support the current recommendation by health professionals to reduce the consumption of ultra-processed foods and promote the consumption of unprocessed or minimally processed foods as an effort to prevent obesity. Additionally, further studies exploring prospective associations between the degree of food processing and obesity markers in a diverse family based cohort are warranted.
Data availability statement
The datasets presented in this article are not readily available because due to Research Ethics Board restrictions and participant confidentiality, we do not make participant data publicly available. The GFHS welcomes external collaborators. Interested investigators can contact GFHS investigators to explore this option, which preserves participant confidentiality and meets the requirements of our Research Ethics Board, to protect human subjects. Requests to access the datasets should be directed to DM, davidma@uoguelph.ca.
Ethics statement
The studies involving human participants were reviewed and approved by University of Guelph Research Ethics Board approved the study (REB #17-07-003). Written informed consent to participate in this study was provided by the participants’ legal guardian/next of kin.
Author contributions
RA and DM designed the research, and analyzed and interpreted the data. RA conducted the research, wrote the first draft of the manuscript, and had primary responsibility for final content. All authors contributed to the review and revision of the manuscript, read, and approved the final manuscript.
Funding
Funding for this project was provided by the Heart and Stroke Foundation of Canada and the Canadian Institutes of Health Research. The funding organizations had no role in the project design, data collection, analyses, interpretation of data or writing of the manuscript.
Acknowledgments
We gratefully acknowledge and thank the research team of the Guelph Family Health Study (GFHS) including Angela Annis, Madeline Nixon, and Adam Sadowski for their assistance in facilitating this study, as well as all the participating GFHS families without whom this study would not have been possible. We also acknowledge the Guelph Family Health Team and Wellington-Dufferin-Guelph Public Health for their support of participant recruitment and knowledge dissemination.
Conflict of interest
The authors declare that the research was conducted in the absence of any commercial or financial relationships that could be construed as a potential conflict of interest.
Publisher’s note
All claims expressed in this article are solely those of the authors and do not necessarily represent those of their affiliated organizations, or those of the publisher, the editors and the reviewers. Any product that may be evaluated in this article, or claim that may be made by its manufacturer, is not guaranteed or endorsed by the publisher.
References
1. Hall KD. Did the food environment cause the obesity epidemic? Obesity. (2018) 26:11–3. doi: 10.1002/oby.22073
2. Nardocci M, Polsky JY, Moubarac J-C. Consumption of ultra-processed foods is associated with obesity, diabetes and hypertension in Canadian adults. Can J Public Health. (2021) 112:421–9. doi: 10.17269/s41997-020-00429-9
3. Poti JM, Braga B, Qin B. Ultra-processed food intake and obesity: what really matters for health-processing or nutrient content? Curr Obes Rep. (2017) 6:420–31. doi: 10.1007/s13679-017-0285-4
4. Monteiro CA, Cannon G, Levy R, Moubarac J-C. NOVA. The star shines bright. World Nutr. (2016) 7:28–38.
5. Vandevijvere S, Jaacks LM, Monteiro CA, Moubarac JC, Girling-Butcher M, Lee AC, et al. Global trends in ultraprocessed food and drink product sales and their association with adult body mass index trajectories. Obes Rev. (2019) 20:10–9. doi: 10.1111/obr.12860
6. Monteiro CA, Moubarac J-C, Levy RB, Canella DS, da Louzada ML, Cannon G. Household availability of ultra-processed foods and obesity in nineteen European countries. Public Health Nutr. (2018) 21:18–26. doi: 10.1017/S1368980017001379
7. Hall KD, Ayuketah A, Brychta R, Walter PJ, Yang S, Zhou M, et al. Ultra-processed diets cause excess calorie intake and weight gain: an inpatient randomized controlled trial of ad libitum food intake. Cell Metab. (2019) 30:67–77. doi: 10.1016/j.cmet.2019.05.008
8. Cordova R, Kliemann N, Huybrechts I, Rauber F, Vamos EP, Levy RB, et al. Consumption of ultra-processed foods associated with weight gain and obesity in adults: a multi-national cohort study. Clin Nutr. (2021) 40:5079–88. doi: 10.1016/j.clnu.2021.08.009
9. Neri D, Steele EM, Khandpur N, Cediel G, Zapata ME, Rauber F, et al. Ultraprocessed food consumption and dietary nutrient profiles associated with obesity: a multicountry study of children and adolescents. Obes Rev. (2022) 23:1–13. doi: 10.1111/obr.13387
10. Machado PP, Steele EM, Levy RB, da Costa Louzada ML, Rangan A, Woods J, et al. Ultra-processed food consumption and obesity in the Australian adult population. Nutr Diabetes. (2020) 10:39. doi: 10.1038/s41387-020-00141-0
11. Rauber F, Steele EM, da Louzada ML, Millett C, Monteiro CA, Levy RB. Ultra-processed food consumption and indicators of obesity in the United Kingdom population (2008-2016). PLoS One. (2020) 15:e0232676. doi: 10.1371/journal.pone.0232676
12. Chen X, Zhang Z, Yang H, Qiu P, Wang H, Wang F, et al. Consumption of ultra-processed foods and health outcomes: a systematic review of epidemiological studies. Nutr J. (2020) 19:1–10. doi: 10.1186/s12937-020-00604-1
13. Rauber F, Chang K, Vamos EP, da Costa Louzada ML, Monteiro CA, Millett C, et al. Ultra-processed food consumption and risk of obesity: a prospective cohort study of UK Biobank. Eur J Nutr. (2021) 60:2169–80. doi: 10.1007/s00394-020-02367-1
14. Canhada SL, Luft VC, Giatti L, Duncan BB, Chor D, Fonseca M, et al. Ultra-processed foods, incident overweight and obesity, and longitudinal changes in weight and waist circumference: the Brazilian Longitudinal Study of Adult Health (ELSA-Brasil). Public Health Nutr. (2020) 23:1076–86. doi: 10.1017/S1368980019002854
15. Mendonça RD, Pimenta AM, Gea A, de la Fuente-Arrillaga C, Martinez-Gonzalez MA, Lopes ACS, et al. Ultraprocessed food consumption and risk of overweight and obesity: the University of Navarra Follow-Up (SUN) cohort study. Am J Clin Nutr. (2016) 104:1433–40. doi: 10.3945/ajcn.116.135004
16. Beslay M, Srour B, Méjean C, Allès B, Fiolet T, Debras C, et al. Ultra-processed food intake in association with BMI change and risk of overweight and obesity: a prospective analysis of the French NutriNet-Santé cohort. PLoS Med. (2020) 17:e1003256. doi: 10.1371/journal.pmed.1003256
17. Konieczna J, Morey M, Abete I, Bes-Rastrollo M, Ruiz-Canela M, Vioque J, et al. Contribution of ultra-processed foods in visceral fat deposition and other adiposity indicators: prospective analysis nested in the PREDIMED-Plus trial. Clin Nutr. (2021) 40:4290–300. doi: 10.1016/j.clnu.2021.01.019
18. Leffa PS, Hoffman DJ, Rauber F, Sangalli CN, Valmórbida JL, Vitolo MR. Longitudinal associations between ultra-processed foods and blood lipids in childhood. Br J Nutr. (2020) 124:341–8. doi: 10.1017/S0007114520001233
19. Costa CS, Rauber F, Leffa PS, Sangalli CN, Campagnolo PDB, Vitolo MR. Ultra-processed food consumption and its effects on anthropometric and glucose profile: a longitudinal study during childhood. Nutr Metab Cardiovasc Dis. (2019) 29:177–84. doi: 10.1016/j.numecd.2018.11.003
20. Chang K, Khandpur N, Neri D, Touvier M, Huybrechts I, Millett C, et al. Association between childhood consumption of ultraprocessed food and adiposity trajectories in the avon longitudinal study of parents and children birth cohort. JAMA Pediatr. (2021) 175:e211573. doi: 10.1001/jamapediatrics.2021.1573
21. Askari M, Heshmati J, Shahinfar H, Tripathi N, Daneshzad E. Ultra-processed food and the risk of overweight and obesity: a systematic review and meta-analysis of observational studies. Int J Obes. (2020) 44:2080–91. doi: 10.1038/s41366-020-00650-z
22. Moradi S, Entezari MH, Mohammadi H, Jayedi A, Lazaridi AV, Kermani M, et al. Ultra-processed food consumption and adult obesity risk: a systematic review and dose-response meta-analysis. Crit Rev Food Sci Nutr. (2021) [Online ahead of print]. doi: 10.1080/10408398.2021.1946005
23. Silva Meneguelli T, Viana Hinkelmann J, Hermsdorff HHM, Zulet MÁ, Martínez JA, Bressan J. Food consumption by degree of processing and cardiometabolic risk: a systematic review. Int J Food Sci Nutr. (2020) 71:678–92. doi: 10.1080/09637486.2020.1725961
24. Neri D, Martinez-Steele E, Monteiro CA, Levy RB. Consumption of ultra-processed foods and its association with added sugar content in the diets of US children, NHANES 2009-2014. Pediatr Obes. (2019) 14:1–11. doi: 10.1111/ijpo.12563
25. Moubarac J-C, Batal M, Louzada ML, Martinez Steele E, Monteiro CA. Consumption of ultra-processed foods predicts diet quality in Canada. Appetite. (2017) 108:512–20. doi: 10.1016/j.appet.2016.11.006
26. Baraldi LG, Martinez Steele E, Canella DS, Monteiro CA. Consumption of ultra-processed foods and associated sociodemographic factors in the USA between 2007 and 2012: evidence from a nationally representative cross-sectional study. BMJ Open. (2018) 8:e020574. doi: 10.1136/bmjopen-2017-020574
27. Birch L, Savage JS, Ventura A. Influences on the development of children’s eating behaviours: from infancy to adolescence. Can J Diet Pract Res. (2007) 68:s1–56.
28. Scaglioni S, De Cosmi V, Ciappolino V, Parazzini F, Brambilla P, Agostoni C. Factors influencing children’s eating behaviours. Nutrients. (2018) 10:706. doi: 10.3390/nu10060706
29. Montaño Z, Smith JD, Dishion TJ, Shaw DS, Wilson MN. Longitudinal relations between observed parenting behaviors and dietary quality of meals from ages 2 to 5. Appetite. (2015) 87:324–9. doi: 10.1016/j.appet.2014.12.219
30. Haines J, Douglas S, Mirotta JA, O’Kane C, Breau R, Walton K, et al. Guelph family health study: pilot study of a home-based obesity prevention intervention. Can J Public Health. (2018) 109:549–60. doi: 10.17269/s41997-018-0072-3
31. Kirkpatrick SI, Subar AF, Douglass D, Zimmerman TP, Thompson FE, Kahle LL, et al. Performance of the automated self-administered 24-hour recall relative to a measure of true intakes and to an interviewer-administered 24-h recall. Am J Clin Nutr. (2014) 100:233–40. doi: 10.3945/ajcn.114.083238
32. Wallace A, Kirkpatrick SI, Darlington G, Haines J. Accuracy of parental reporting of preschoolers’ dietary intake using an online self-administered 24-h recall. Nutrients. (2018) 10:1–10. doi: 10.3390/nu10080987
33. Monteiro CA, Levy RB, Claro RM, de Castro IRR, Cannon G. A new classification of foods based on the extent and purpose of their processing. Cad Saude Publica. (2010) 26:2039–49. doi: 10.1590/s0102-311x2010001100005
34. Monteiro CA, Cannon G, Lawrence M, Louzada CML, Pereira Machado P. Ultra-Processed Foods, Diet Quality, and Health using the NOVA Classification System. (2019). Available online at: www.fao.org/3/ca5644en/ca5644en.pdf (accessed May 13, 2020).
35. Bleiweiss-Sande R, Bailey CP, Sacheck J, Goldberg JP. Addressing challenges with the categorization of foods processed at home: a pilot methodology to inform consumer-facing guidance. Nutrients. (2020) 12:1–11. doi: 10.3390/nu12082373
36. Myatt M, Guevarra E. R Package Version 0.3.1. (2019). Available online at: https://cran.r-project.org/package=zscorer (accessed August 15, 2021).
37. Kushner R, Schoeller DA, Fjeld CR, Danford L. Is the Impedance index (ht2/R) significant in predicting total body water? Am J Clin Nutr. (1992) 56:835–9. doi: 10.1093/ajcn/56.5.835
38. Fomon SJ, Haschke F, Ziegler EE, Nelson SE. Body composition of reference children from birth to age 10 years. Am J Clin Nutr. (1982) 35:1169–75. doi: 10.1093/ajcn/35.5.1169
40. Liang K-Y, Zeger SL. Longitudinal data analysis using generalized linear models. Biometrika. (1986) 73:13–22. doi: 10.1093/biomet/73.1.13
41. Juul F, Martinez-Steele E, Parekh N, Monteiro CA, Chang VW. Ultra-processed food consumption and excess weight among US adults. Br J Nutr. (2018) 120:90–100. doi: 10.1017/S0007114518001046
42. Nardocci M, Leclerc B-S, Louzada M-L, Monteiro CA, Batal M, Moubarac J-C. Consumption of ultra-processed foods and obesity in Canada. Can J Public Health. (2019) 110:4–14. doi: 10.17269/s41997-018-0130-x
43. Da Louzada ML, Baraldi LG, Steele EM, Martins APB, Canella DS, Moubarac J-C, et al. Consumption of ultra-processed foods and obesity in Brazilian adolescents and adults. Prev Med. (2015) 81:9–15. doi: 10.1016/j.ypmed.2015.07.018
44. Rico-Campà A, Martínez-González MA, Alvarez-Alvarez I, De Deus Mendonça R, De La Fuente-Arrillaga C, Gómez-Donoso C, et al. Association between consumption of ultra-processed foods and all cause mortality: sun prospective cohort study. BMJ. (2019) 365:1949. doi: 10.1136/bmj.l1949
45. Rauber F, da Louzada ML, Steele EM, Millett C, Monteiro CA, Levy RB. Ultra-processed food consumption and chronic non-communicable diseases-related dietary nutrient profile in the UK (2008–2014). Nutrients. (2018) 10:587. doi: 10.3390/nu10050587
46. Miller V, Micha R, Choi E, Karageorgou D, Webb P, Mozaffarian D. Evaluation of the quality of evidence of the association of foods and nutrients with cardiovascular disease and diabetes: a systematic review. JAMA Netw Open. (2022) 5:e2146705. doi: 10.1001/jamanetworkopen.2021.46705
47. De Amicis R, Mambrini SP, Pellizzari M, Foppiani A, Bertoli S, Battezzati A, et al. Ultra-processed foods and obesity and adiposity parameters among children and adolescents: a systematic review. Eur J Nutr. (2022) 61:2297–311. doi: 10.1007/s00394-022-02873-4
48. Bleiweiss-Sande R, Sacheck JM, Chui K, Goldberg JP, Bailey C, Evans EW. Processed food consumption is associated with diet quality, but not weight status, in a sample of low-income and ethnically diverse elementary school children. Appetite. (2020) 151:104696. doi: 10.1016/j.appet.2020.104696
49. Oliveira T, Ribeiro I, Jurema-Santos G, Nobre I, Santos R, Rodrigues C, et al. Can the consumption of ultra-processed food be associated with anthropometric indicators of obesity and blood pressure in children 7 to 10 years old? Foods. (2020) 9:1567. doi: 10.3390/foods9111567
50. De Melo ISV, Costa CACB, Dos Santos JVL, Dos Santos AF, Florêncio TM, Bueno NB. Consumption of minimally processed food is inversely associated with excess weight in adolescents living in an underdeveloped city. PLoS One. (2017) 12:e0188401. doi: 10.1371/journal.pone.0188401
51. Sahoo K, Sahoo B, Choudhury AK, Sofi NY, Kumar R, Bhadoria AS. Childhood obesity: causes and consequences. J Fam Med Prim Care. (2015) 4:187–92. doi: 10.4103/2249-4863.154628
52. Martínez Steele E, Raubenheimer D, Simpson SJ, Baraldi LG, Monteiro CA. Ultra-processed foods, protein leverage and energy intake in the USA. Public Health Nutr. (2018) 21:114–24. doi: 10.1017/S1368980017001574
53. Moubarac J-C, Parra DC, Cannon G, Monteiro CA. Food classification systems based on food processing: significance and implications for policies and actions: a systematic literature review and assessment. Curr Obes Rep. (2014) 3:256–72. doi: 10.1007/s13679-014-0092-0
54. Monteiro CA, Moubarac JC, Cannon G, Ng SW, Popkin B. Ultra-processed products are becoming dominant in the global food system. Obes Rev. (2013) 14:21–8. doi: 10.1111/obr.12107
55. Fardet A. Minimally processed foods are more satiating and less hyperglycemic than ultra-processed foods: a preliminary study with 98 ready-to-eat foods. Food Funct. (2016) 7:2338–46. doi: 10.1039/c6fo00107f
56. Lerner A, Matthias T. Changes in intestinal tight junction permeability associated with industrial food additives explain the rising incidence of autoimmune disease. Autoimmun Rev. (2015) 14:479–89. doi: 10.1016/j.autrev.2015.01.009
57. Forde CG, Mars M, de Graaf K. Ultra-processing or oral processing? A role for energy density and eating rate in moderating energy intake from processed foods. Curr Dev Nutr. (2020) 4:nzaa019. doi: 10.1093/cdn/nzaa019
58. Traversy G, Chaput J-P. Alcohol consumption and obesity: an update. Curr Obes Rep. (2015) 4:122–30. doi: 10.1007/s13679-014-0129-4
59. Bush T, Lovejoy JC, Deprey M, Carpenter KM. The effect of tobacco cessation on weight gain, obesity, and diabetes risk. Obesity. (2016) 24:1834–41. doi: 10.1002/oby.21582
60. Jackson SE, Llewellyn CH, Smith L. The obesity epidemic – nature via nurture: a narrative review of high-income countries. SAGE Open Med. (2020) 8:2050312120918265. doi: 10.1177/2050312120918265
61. Klop B, Elte JWF, Cabezas MC. Dyslipidemia in obesity: mechanisms and potential targets. Nutrients. (2013) 5:1218–40. doi: 10.3390/nu5041218
62. Griffin J, Albaloul A, Kopytek A, Elliott P, Frost G. Effect of ultraprocessed food intake on cardiometabolic risk is mediated by diet quality: a cross-sectional study. BMJ Nutr Prev Health. (2021) 4:174–80. doi: 10.1136/bmjnph-2020-000225
63. Machado PP, Steele EM, da Louzada ML, Levy RB, Rangan A, Woods J, et al. Ultra-processed food consumption drives excessive free sugar intake among all age groups in Australia. Eur J Nutr. (2020) 59:2783–92. doi: 10.1007/s00394-019-02125-y
64. Koiwai K, Takemi Y, Hayashi F, Ogata H, Matsumoto S, Ozawa K, et al. Consumption of ultra-processed foods decreases the quality of the overall diet of middle-aged Japanese adults. Public Health Nutr. (2019) 22:2999–3008. doi: 10.1017/S1368980019001514
65. Juul F, Hemmingsson E. Trends in consumption of ultra-processed foods and obesity in Sweden between 1960 and 2010. Public Health Nutr. (2015) 18:3096–107. doi: 10.1017/S1368980015000506
66. Chen Y, Huang Y, Lo YC, Wu H, Mark L, Lee M. Secular trend towards ultra-processed food consumption and expenditure compromises dietary quality among Taiwanese adolescents. Food Nutr Res. (2018) 62:1565. doi: 10.29219/fnr.v62.1565
67. Marrón-Ponce JA, Tolentino-Mayo L, Hernández-F M, Batis C. Trends in ultra-processed food purchases from 1984 to 2016 in Mexican households. Nutrients. (2019) 11:1–15. doi: 10.3390/nu11010045
68. Martins APB, Levy RB, Claro RM, Moubarac JC, Monteiro CA. Increased contribution of ultra-processed food products in the Brazilian diet (1987-2009). Rev Saude Publica. (2013) 47:656–65. doi: 10.1590/S0034-8910.2013047004968
69. Moubarac J-C, Batal M, Martins APB, Claro R, Levy RB, Cannon G, et al. Processed and ultra-processed food products: consumption trends in Canada from 1938 to 2011. Can J Diet Pract Res. (2014) 75:15–21. doi: 10.3148/75.1.2014.15
70. Martínez Steele E, Popkin BM, Swinburn B, Monteiro CA. The share of ultra-processed foods and the overall nutritional quality of diets in the US: evidence from a nationally representative cross-sectional study. Popul Health Metr. (2017) 15:6. doi: 10.1186/s12963-017-0119-3
Keywords: ultra-processed foods, NOVA food classification system, obesity, BMI, ASA24, children, health, disease prevention
Citation: Ashraf R, Duncan AM, Darlington G, Buchholz AC, Haines J and Ma DWL (2022) The degree of food processing is associated with anthropometric measures of obesity in Canadian families with preschool-aged children. Front. Nutr. 9:1005227. doi: 10.3389/fnut.2022.1005227
Received: 29 July 2022; Accepted: 06 September 2022;
Published: 23 September 2022.
Edited by:
Fernanda Rauber, University of São Paulo, BrazilReviewed by:
Ariene Carmo, Minas Gerais State University, BrazilManon Cairat, International Agency for Research on Cancer (IARC), France
Copyright © 2022 Ashraf, Duncan, Darlington, Buchholz, Haines and Ma. This is an open-access article distributed under the terms of the Creative Commons Attribution License (CC BY). The use, distribution or reproduction in other forums is permitted, provided the original author(s) and the copyright owner(s) are credited and that the original publication in this journal is cited, in accordance with accepted academic practice. No use, distribution or reproduction is permitted which does not comply with these terms.
*Correspondence: David W. L. Ma, davidma@uoguelph.ca