Red, white, and processed meat consumption related to inflammatory and metabolic biomarkers among overweight and obese women
- 1Department of Community Nutrition, School of Nutritional Sciences and Dietetics, Tehran University of Medical Sciences (TUMS), Tehran, Iran
- 2Department of Nutrition, Science and Research Branch, Islamic Azad University, Tehran, Iran
- 3Department of Exercise Physiology, University of Isfahan, Isfahan, Iran
- 4Department of Health and Human Performance, Marymount University, Arlington, VA, United States
- 5Faculty of Sports Sciences, Waseda University, Tokorozawa, Japan
Background: Considering that a high meat intake is directly associated with obesity, it is critical to address the relationship between consuming different types of meat with inflammation and metabolism in overweight and obese cohorts. Thus, we evaluated the association between red, white, and processed meat consumption with inflammatory and metabolic biomarkers in overweight and obese women.
Methods: The current cross-sectional study was conducted on 391 overweight and obese Iranian women. Dietary intake was obtained from a food frequency questionnaire (FFQ) with 147 items. The anthropometric measurements, serum lipid profile, and inflammatory markers were measured by standard protocols. All associations were assessed utilizing one-way analysis of variance (ANOVA), analysis of covariance (ANCOVA), and linear regression models.
Results: In the adjusted model, it was established that higher intake of processed meat had a significant positive association with leptin levels (β: 0.900, 95% CI: 0.031;1.233, p = 0.015). Moreover, after considering the confounders, a significant positive association between processed meat and macrophage inflammatory protein (MCP-1) levels was observed (β: 0.304, 95% CI:0.100;1.596, p = 0.025). Positive significant associations between high-sensitivity C-reactive protein (hs-CRP) (β:0.020, 95% CI:0.000;0.050, P = 0.014) and plasminogen activator inhibitor 1 (PAI-1) (β:0.263, 95% CI:0.112;0.345, p = 0.053) and MCP-1 (β:0.490, 95% CI: 0.175;1.464, p = 0.071) levels with red meat were also shown; while there was a significant negative association between red meat and the homeostasis model assessment of insulin resistance (HOMA-IR) (β: −0.016, 95% CI: −0.022, −0.001, p = 0.033). Furthermore, a significant negative association were established following confounding adjustment between Galectin-3 (Gal-3) (β: −0.110, 95% CI: −0.271;0.000, p = 0.044), MCP-1 (β: −1.933, 95% CI: −3.721;0.192, p = 0.022) and Homeostatic Model Assessment for Insulin Resistance (HOMA-IR) (β: −0.011, 95% CI: −0.020,0.000, p = 0.070) levels with high adherence of white meat intake. In contrast, a significant marginally positive association between PAI-1 levels and high adherence to white meat intake (β: −0.340, 95% CI: −0.751;0.050, p = 0.070) has been shown.
Conclusions: Higher red and processed meat consumption were positively associated with inflammatory and metabolic markers in overweight and obese women. In contrast, negative relationships between high adherence to white meat and various inflammatory and metabolic parameters were established. Further studies are needed to confirm the causality of these associations and potential mediating pathways.
Introduction
Global obesity rates are rising (1). Currently, more than 26% of Iranian adults are obese, however, Iranian women are more affected than males (57 vs. 22%) (2). Obesity is defined as a genetically based chronic multifactorial condition that is brought on by the buildup of extra fat tissue (3). Numerous serious comorbidities are caused by it, including insulin resistance, hypertension, diabetes mellitus, and low-grade inflammation (1, 4–6). Excess adipose tissue produces and secretes an increasing number of inflammatory mediators into the systemic circulation, enhancing the inflammatory profile (7, 8). Among these are acute-phase proteins, including plasminogen activator inhibitor 1 (PAI-1), and classic peptide mediators of inflammation such as interleukin 1 (IL-1) (9, 10), macrophage inflammatory protein (MCP-1) (11), transforming growth factor (TGF-b) (12), and high-sensitivity C-reactive protein (hs-CRP) (13). Moreover, increasing abdominal fat mass is associated with insulin resistance as one inflammation indicator (14) and ablation of Galectin-3 (Gal-3), which hastens lipid-induced atherogenesis and plays an essential role in cell-cell adhesion, cell-matrix interactions, macrophage activation, metastasis, and apoptosis (15, 16).
Various factors are directly related to causing obesity and as a result, causing inflammation or changes in inflammatory levels, but undoubtedly one of the most important factors is food intake, which is, directly and indirectly, effective in changing inflammatory levels (17, 18). The food sources received from the main food groups, especially protein intake from different sources, are effective in causing obesity and consequently inflammation, or directly in the occurrence of inflammation (19–21). One of the food items that have conflicting and challenging results in studies on inflammation and metabolic diseases is getting protein from different food sources, especially red and processed meat (22–24). Research to date indicates that a high meat intake is directly associated with obesity. Indeed, inflammation and insulin resistance related to excess adipose tissue have been proposed to explain the documented link between red meat consumption and metabolic disorders in obese cohorts (22–24). This relationship may be explained by the negative effects of saturated fat, animal protein, and red meat's high iron content, mainly heme iron, saturated fatty acid (SFA) that has been linked to increased adiposity, inflammation, and insulin resistance (IR) (25–27). On the other hand, several studies have shown that fish and its components favor inflammatory markers (28–30). For instance, eating white meat improves interleukin 6 (IL-6) synthesis, affecting hs-CRP development in the liver (21). Despite these findings, investigations evaluating the relationship between consuming different types of meat and inflammatory and metabolic markers in overweight and obese cohorts are scarce. Addressing these relationships in overweight and obese women is particularly important, as they are more likely to develop inflammatory and metabolic abnormalities than their male counterparts (31, 32).
According to an explanation and despite the existing controversies regarding the relationship between the consumption of red and processed meat with inflammatory factors needed. In addition, this issue has not been investigated sufficiently in Iran, especially in obese and overweight women, so with a view more comprehensively, the intake of red, processed, and white meat was examined in the present study. Therefore, we evaluated the relationship between red, white, and processed meat consumption with inflammatory and metabolic biomarkers in overweight and obese women.
Materials and methods
Participants
In this cross-sectional study recruited, a total of 391 healthy overweight (BMI = 25–29.9), and obese (BMI ≥ 30) women, aged 18–56 years old that had been referred to health centers in Tehran. The exclusion criteria were as follows: smoking; chronic disease histories such as the history of hypertension, cardiovascular diseases, diabetes mellitus, impaired renal and liver function inflammatory diseases, cancer, thyroid disease, regular use of medicine (including oral contraceptive pill), alcohol use, supplement consumption (vitamins and minerals and both) pregnancy, lactation period, and menopause, people who had been on an arbitrary special diet plan and anyone whose body weight had changed noticeably during the previous year, participated in sports, if their total calorie consumption did not fall between 800 and 4,200 (17,556–3,344 kJ) (33), and individuals with weight fluctuations in the past year were also excluded from the study. Before participating in the study, each participant signed a written informed consent form. The study was approved by the Ethical Committee of the Tehran University of Medical Sciences (TUMS) and performed according to the ethical standards of the Declaration of Helsinki (IR.TUMS.VCR.REC.1395.1597).
Study design
In this descriptive cross-sectional study, sampling and data collection were completed in 2018. Multi-stage random cluster sampling was performed among health centers affiliated with the TUMS to select certain regions from among all the regions of the city; 20 clusters were selected. Two visits were conducted: in the first visit, a demographic questionnaire, food frequency questionnaire (FFQ), blood pressure as well as anthropometric and body composition measurements were performed. In the second visit, blood samples were taken from individuals. All measurements were performed in the Nutrition and Biochemistry Laboratory of the School of Nutritional and Dietetics at TUMS.
Dietary assessments
Dietitians assessed dietary intake using a validated 147-item semi-quantitative food frequency questionnaire (FFQ) by face-to-face interview (34, 35). Individuals reported the frequency of each food item consumed in the past year, which was consequently converted to grams per day using household measures (36). Energy and dietary nutrients were calculated using the Iranian Food Composition Table and NUTRITIONIST IV (version 7.0; N-Squared Computing, Salem, OR). The current study is based on the consumption of three different types of meat extracted from the FFQ as gram/day: (1) the red meat category was defined as the sum of red (beef, lamb, sheep) and organ meats (beef liver, kidney, tongue, and heart); (2) white meat consisted of fish and poultry, such as chicken and turkey; and (3) processed meats included sausages, hamburger, Kalbas, Mortadella, and canned fish. All kinds of meat intake were adjusted by energy intake by residual method.
Anthropometric and body composition assessment
The anthropometrics and body composition examination were conducted between 8 and 9 a.m., following a 12-h overnight fast. Participants also abstained from unusual physical activity for 72 h prior to the assessments. Weight was measured using a digital scale (Seca, Hamburg, Germany) in light clothing and without shoes with a precision near 0.1 kg, and stature was measured via a stadiometer with an accuracy close to 0.1 cm. Hip circumference (HC) and waist circumference (WC) were measured separately in the smallest and largest girth, respectively, with accuracy nearest to 0.1 cm. A multi-frequency bioelectrical impedance analyzer (BIA) called the InBody 770 Scanner was used to measure body composition (Inbody Co., Seoul, Korea). Using electrical impulses from the hands and feet, this device measures the resistance of bodily tissues. Participants were instructed to thoroughly urinate (void) and refrain from drinking water 30 min before the test. Participants were instructed to remove any metal objects, including tools, jewelry, coats, jackets, and shoes, following the manufacturer's instructions (25). They were also requested to remove their socks before the device was placed on them, to boost accuracy. Our BIA in our lab has a test-retest reliability of r = 0.98.
BMI, skeletal muscle mass (SMM), fat-free mass (FFM), body fat mass (BFM), bone mass content (BMC), soft lean mass (SLM), visceral fat level (cm), trunk fat, waist to hip ratio (WHR), fat mass index (FMI), fat-free mass index (FFMI) as body composition components were established with a BIA.
Biochemical assessment
Blood samples were taken after a 10–12 h fast, and serum was stored at −80 °C. All tests were analyzed according to the manufacturer's guidelines. The glucose oxidase method was utilized for fasting blood sugar (FBS) appraisal, and the affront level was measured by an enzyme-linked immunosorbent measure (ELISA) unit (Human affront ELISA unit, DRG Pharmaceuticals, GmbH, Germany). Triglyceride (TG), total cholesterol (Chole), Low-density lipoprotein cholesterol (LDL-c), and High-density lipoprotein cholesterol (HDL-c) were measured by related packs (Pars Azemun, Iran). Serum glutamic pyruvic transaminase (SGPT) and serum glutamic-oxaloacetic transaminase (SGOT) were evaluated utilizing the Universal League of Clinical Chemistry and Research facility Medication standardization. The HOMA-IR was assessed as the item of fasting glucose and affront level isolated by 22.5 with a molar unit (mmol/L) (37). The Enzyme-Linked Immunosorbent Assays (ELISA) technique was used to evaluate the levels of inflammatory biomarkers (such as hs-CRP and IL-1b), Gal-3. (R&D Systems, Minneapolis, MN), MCP-1 (Zell Bio GmbH, ULM, Germany), TGF-β (HUMAN TGF-BETA 1 Quantikine ELIZA kit R&D System-USA), PA-I (Human PAI-1*96 T ELIZA kit Crystal Company) and serum leptin concentrations (Mediagnost, Reutlingen, Germany). The inter-assay and intra-assay variability for all tests were <12 and 10%, respectively.
Blood pressure
Systolic and diastolic blood pressure (SBP, DBP) were assessed after 15 min of rest using an automatic sphygmomanometer (OMRON, Germany). Three measurements at 1-min intervals were taken and averaged.
Demographic variables and physical activity
A demographic questionnaire was utilized to assess quality characteristics such as education, employment, marital status, economic levels, and family history of obesity. The validated International Physical Activity Questionnaire (IPAQ) was converted to Minutes per week using Metabolic Equivalents (MET-min/week) to determine physical activity criteria (38).
Statistical analysis
The sample size was computed according to the following formula: n = [(Z 1-α + Z1-β) × (√1- r2)/r)2] which r = 0.21, β = 0.90 and α = 0.05. The evaluation of the histogram curve and the Kolmogorov-Smirnov test was used to ensure that the data were distributed normally (p > 0.05). In addition according to the central limit theorem, all dependent variables are taken into account using the normal distribution (39, 40). The mean and standard deviation were used to describe quantitative variables, whereas number and percent (%) were used to represent categorical variables. One-way analysis of variance (ANOVA) was validated for assessing the mean difference of quantitative variables across red, processed, and white meat' medians. Relationships between inflammatory indicators were investigated, and analysis of covariance (ANCOVA) was employed to control the effect of confounders in 2 models. The Chi-square (χ2) test was used to examine categorical variables. Three models used linear regression to control for confounders and covariates points and validated associations between red, processed, and white meat consumption and inflammatory markers. This analysis was present by beta (β) and 95% Confidence Interval (CI) and goodness of fit (GOF) of r squared (R2). All linear regression analysis's assumptions and concerns, including normality, the normality of residual error, linearity, homoscedasticity, and collinearity, were evaluated. IBM SPSS version 26.0 was used to conduct statistical analyses (SPSS, Chicago, IL, USA). The significance level was set at 2-sided P < 0.05 and P = 0.05–0.07, considered marginally significant.
Results
Study population characteristics
The present study was conducted on 391 obese and overweight Iranian women, of which 27% were single, 46.8% had a college education, and 27.3% had good economic status. The means and standard deviation (SD) of age, weight, BMI and WHR, FFM, and BFM of individuals were 36.681 (9.150) years, 80.320 (11.065) kg, 31.011 (3.920) kg/m2, 1.162 (4.575), 46.523 (5.690) kg and 34.804 (8.800) kg, respectively. Moreover, the mean intake of red meat was 40.161 (19.660) gr/day, while white and processed meat had mean intakes of 59.300 (40.421) gr/day and17.896 (14.325) gr, respectively.
Population characteristics among medians of red, white, and processed meat consumption
General characteristics of participants, such as body composition, biochemical assessment, and others among lower vs. higher than the median of processed meat, red meat, and white meat intake, are presented in Table 1. P-values for all variables were reported before the adjustment in the crude model by ANOVA, and after adjustment with potential confounders, including age, BMI, physical activity, and energy intake (Table 1).
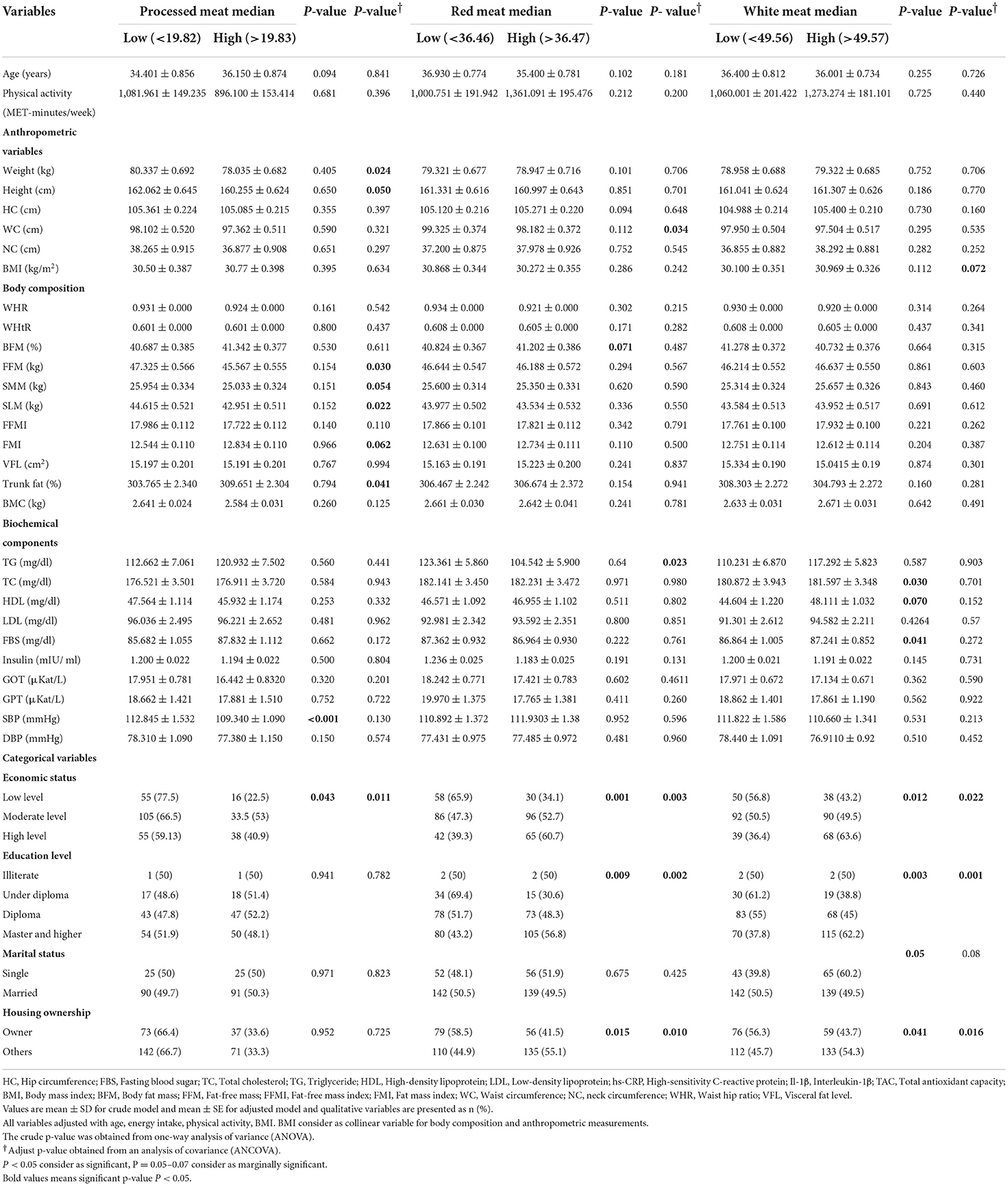
Table 1. Study participant characteristics between medians of processed meat, red meat, and white meat (g/d) in 391 obese and overweight women.
General characteristics of participants among processed meat intake categories
Among processed meat categories, there was a significant mean difference for SBP (p < 0.001). But, significance was lost after adjusting for potential confounders (p = 0.130). In the adjusted model, in body composition variables, there were significant mean differences for SMM (p = 0.054), FFM (p = 0.030), SLM (p = 0.022), and trunk fat (p = 0.041), FMI (p = 0.062) and in categorical variables; economic status (p = 0.011) (Table 1).
General characteristics of participants among red meat intake categories
There was a significant difference in economic status (p = 0.0003), education level (p = 0.002), and housing ownership (p = 0.010) before and after adjustment Table 1. Moreover, the crude model showed a marginally significant difference between groups for BFM (p = 0.071). High adherence to red meat was associated with lower levels of TG (p = 0.023) and WC (p = 0.034). No significant differences were noted in other variables (p > 0.05) (Table 1).
General characteristics of participants among white meat intake categories
After adjusting for potential confounders, there was a significant marginal difference in BMI (p = 0.072). There were also significant differences in economic status (p = 0.002), education level (p = 0.001), and housing ownership (p = 0.016). Women with a higher intake of white meat had marginally significantly higher mean HDL (p = 0.070), total cholesterol (p = 0.030), and lowered FBS (p = 0.041), but these associations were not present after controlling confounders (Table 1).
Dietary intake of the study population across intakes of red, white, and processed meat
The dietary intake of the participants across two groups to intakes of red meat, white meat, and processed meat is shown in Table 2. The mean energy intake in low and high-intake processed meat was 2,763.591 vs. 2,447.06 kcal/day, respectively, and was statistically significant (p = 0.002). Additionally, the intake of energy was high in low intake white meat, 2,695 vs. 2,495.27 gr with (p = 0.053). After adjustment for confounders (including age, BMI, physical activity, and total energy intake) mean of red meat, protein, vegetables, saturated fatty acid (SFA), eicosapentaenoic acid (EPA), Docosahexaenoic acid (DHA), zinc, copper, potassium, vitamins of C, A, B3, B6, and B12 consumption was higher in the upper median group of red meat (p ≤ 0.05). In contrast, intake of total fiber, linoleic acid, polyunsaturated fatty acids (PUFA), and vitamin E were low in participants with high red meat intake (p ≤ 0.05). Our results also showed that sodium and vitamin B12 were significantly higher (p ≤ 0.05) in subjects with increased consumption of processed meat compared to those with low intake. Protein, vegetables, EPA, DHA, zinc, calcium, potassium, and vitamins of A, B3, B6, B2, and B12 consumption was greater in those with high white meat intake (p < 0.05). On the other hand, fat, monounsaturated fatty acids (MUFA), linoleic acid, PUFA, Manganese, and vitamin E consumption was low in participants with high white meat intake (p ≤ 0.05).
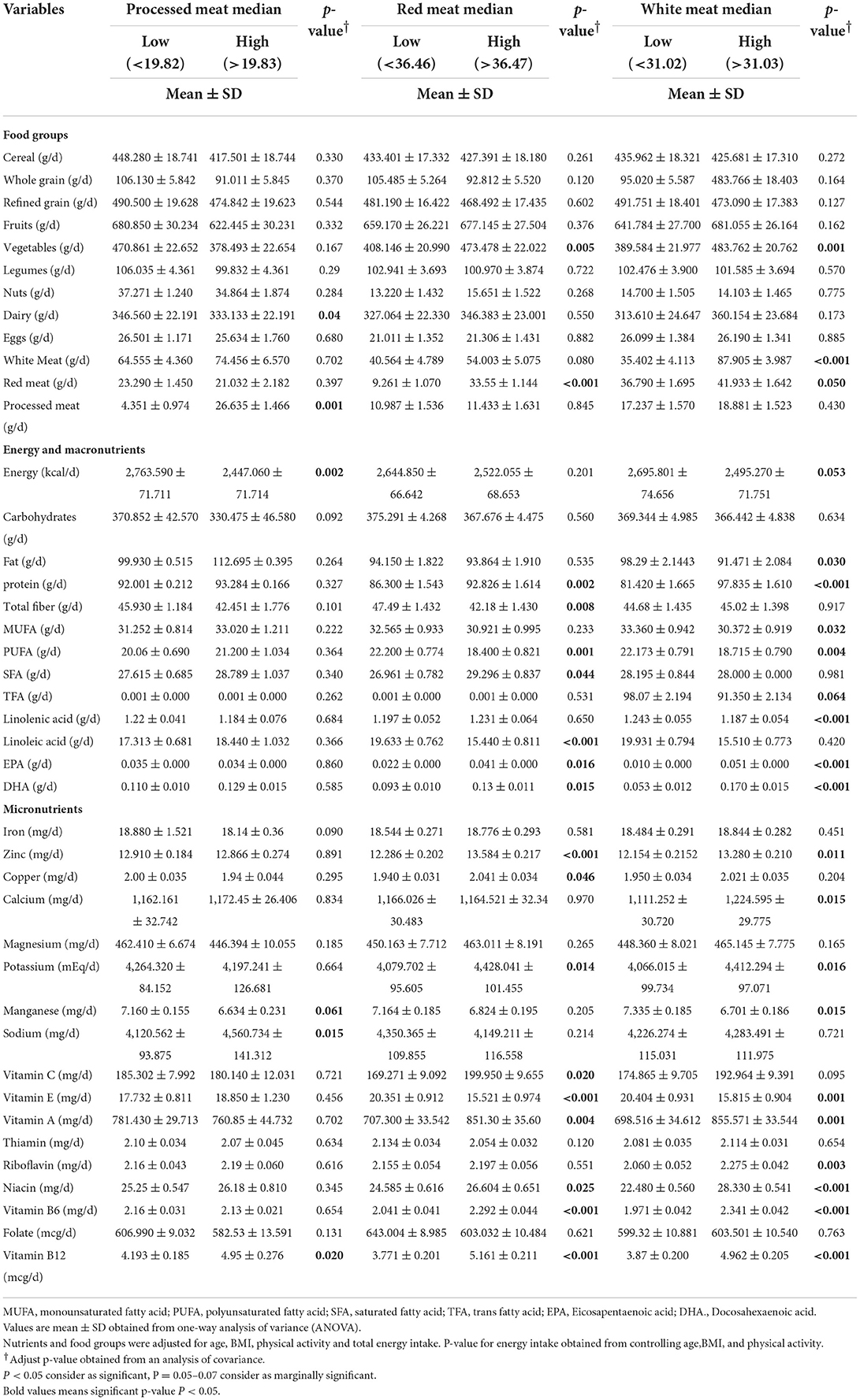
Table 2. Dietary intakes of study population between medians of processed meat, red meat, and white meat in 391 obese and overweight women.
Inflammatory biomarkers among medians of red, white, and processed meat
There was no significant difference in PAI-1, Gal-3, hs-CRP, or MCP-1 between low and high processed meat intake categories before adjustment (p > 0.05), but in model 2 that adjusted for potential confounder, mean PAI-1 (p = 0.052), Gal-3 (p = 0.054), hs-CRP (p = 0.050), MCP-1 (p = 0.046) was higher in a participant with high adherence of proceeded meat, Table 3. The mean of HOMA-IR (p = 0.045) and IL1b (p = 0.071) was low in women with a high intake of red meat in models 1 and 2. However, in the crude model mean of HOMA-IR was high in subjects with a high white meat intake (p = 0.024), but in model 2 mean of its index was low in subjects with a high intake of white meat with no significance (p = 0.610). The mean of leptin was higher in those with a low intake of white meat in the crude model (p = 0.882); after adjustment, this association remained significant (p = 0.024), (Table 3).
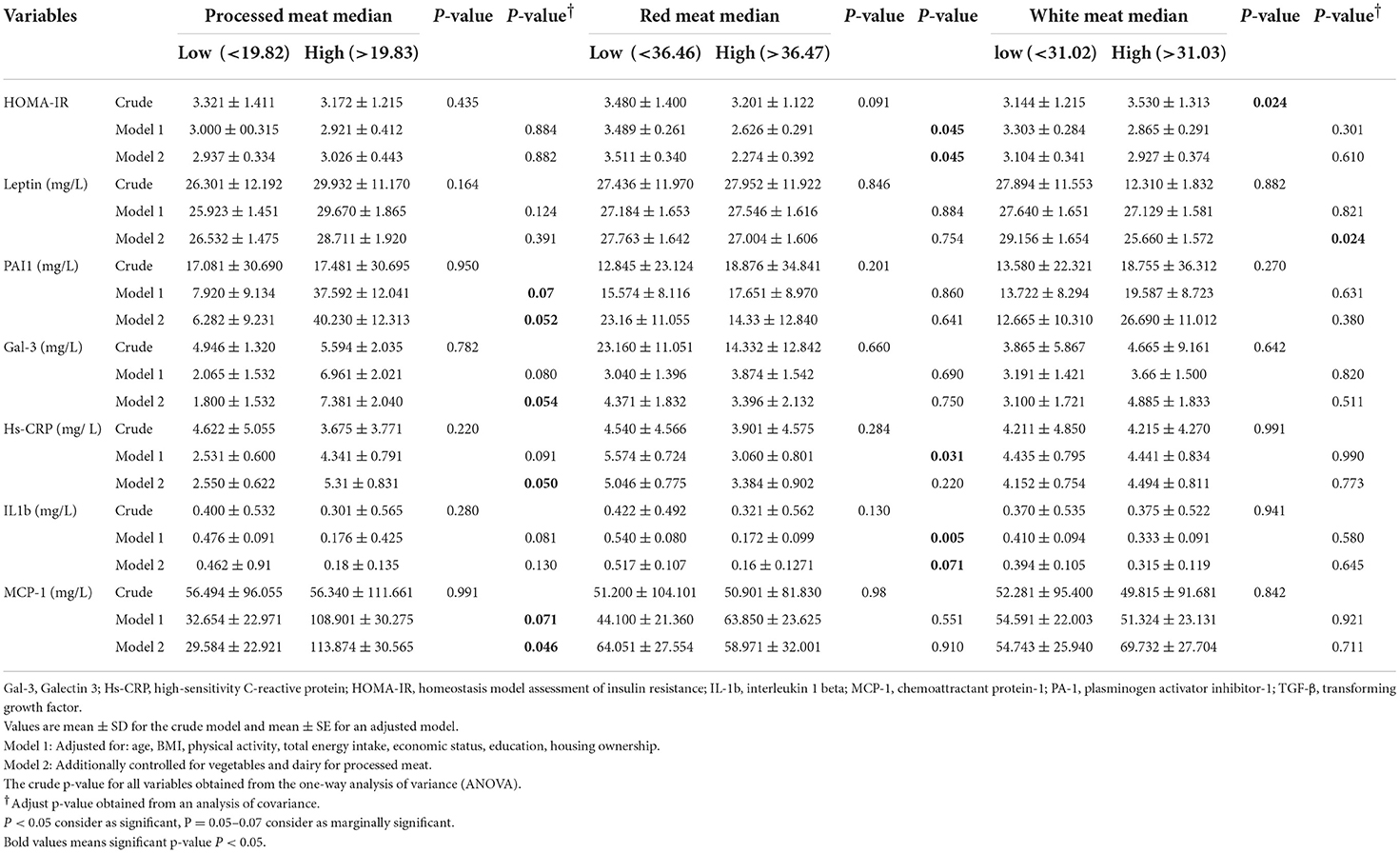
Table 3. Inflammatory biomarkers between low and high category of processed meat, red meat and white meat (g/d) in 391 obese and overweight women.
Association between red, white as well as processed meat and inflammatory biomarkers
In the crude model, there was a significant association between HOMA-IR and processed meat (β: 0.410, 95% CI: 0.070;0.750, p = 0.011), but after adjusting for potential confounders, in model 3 this association disappears (β: −0.007, 95% CI: −0.021;0.007, p = 0.384). Result in the crude model showed that increased intake of processed meat had a significant positive association with the level of leptin (β: 0.172, 95% CI:0.020;0.321, p = 0.021) and Gal-3 (β: 0.052, 95% CI: 0.001,0.205, p = 0.031) that after adjustment in model 3 it remained significant (β: 0.900, 95% CI: 0.031;1.233, p = 0.015). A positive association with the level of MCP-1 (β:17.287, 95% CI: −12.692;47.267, p = 0.250) was not statistically significant, but after considering the confounding factors, a significant relationship was observed (β:0.304, 95% CI:0.100;1.596, p = 0.025) Table 4. Moreover, a positive significant association between the level of hs-CRP, PAI-1 and MCP1 and high adherence to red meat (β:0.020, 95% CI:0.001;0.050, p = 0.014), (β:0.263, 95% CI:0.112;0.345, p = 0.053), (β:0.490, 95% CI: 0.175;1.464, p = 0.071) and inverse association with HOMA-IR (β: −0.016, 95% CI: −0.022, −0.001, p = 0.033) in model 3 was established. Our analysis demonstrated a significantly negative association between levels of Gal-3 and MCP-1 and high adherence to white meat (β: −0.110, 95% CI: −0.271;0.000, p = 0.044) and (β: −1.933, 95% CI: −3.721;0.192, p = 0.022) after adjustment for confounder. In contrast, a significant positive association between the level of PAI-1 and high adherence to white meat (β: 0.154, 95% CI: 0.021;0.283, p = 0.028) was observed in the crude model, but after adjustment, this association reached marginal significance (β: −0.340, 95% CI: −0.751;0.050, p = 0.070). After controlling potential confounders, there was a marginally significant inverse association between higher intake of white meat with HOMA-IR (β: −0.011, 95% CI: −0.020,0.000, p = 0.070) (Table 4).
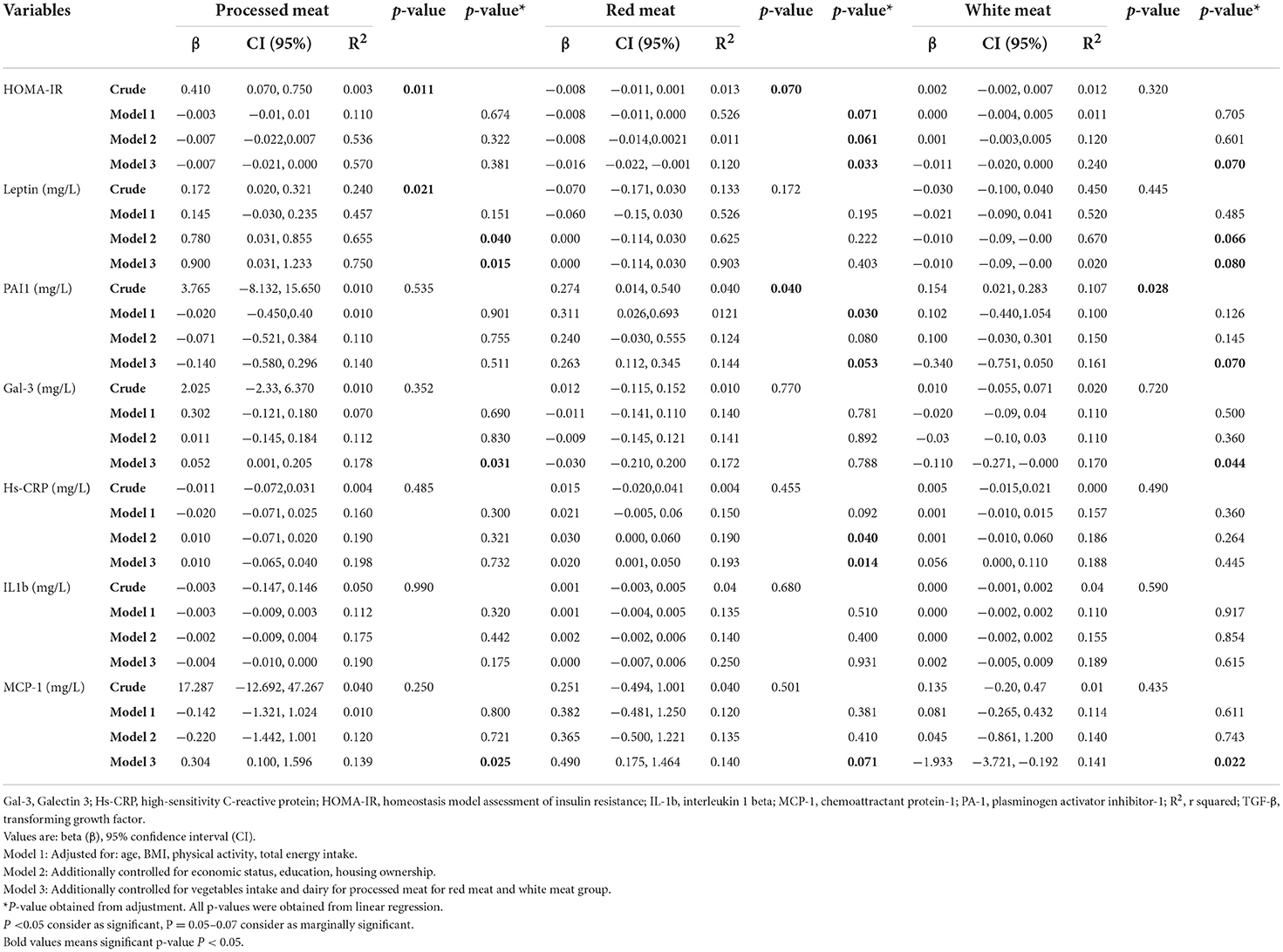
Table 4. Association between inflammatory biomarkers with processed meat, red meat, and white meat (g/d) in 391 obese and overweight women.
Discussion
In this study, we evaluated the relationship between the consumption of red, white, and processed meats with inflammatory and metabolic biomarkers in overweight and obese women. We found that red meat intake was positively associated with PAL-1, hs-CRP, and MCP-1 levels. Moreover, processed meat intake was positively associated with different biomarkers such as leptin, Gal-3, and MCP-1. On the contrary, negative relationships between high adherence to white meat intake and Gal-3, MCP-1, PA1, and HOMA-IR were established.
In the current cross-sectional study, greater processed meat intakes were initially positively associated with higher HOMA-IR and leptin levels. However, HOMA-IR was no longer associated with processed meat intake after adjustment for all confounders. After controlling for all confounders in the last model of adjustment, there was a positive association between processed meat intake and levels of leptin, Gal-3, and MCP-1. In line with our study, a cohort study displayed that processed meat consumption was positively associated with leptin and CRP in both men and women after 9 years of follow-up (41). Another study expressed that higher processed meat consumption is positively associated with inflammatory markers in 403,886 British adults (42). That seems the binding capacity of iron in our body might be exceeded by consuming processed meat containing high amounts of heme iron. Oxidative stress can be increased by free iron and, as a result, act as a proinflammatory agent (43).
In our study, consumption of white meat was negatively associated with HOMA-IR, Gal-3, MCP-1, and PAL-1. In line with these findings, several studies have reported that higher consumption of white meat such as poultry has been related to lower inflammatory markers such as CRP (44–46). The consumption of fish and seafood, considered white meat, was shown to have anti-inflammatory properties due to the high contents of omega-3 fatty acids and lesser amount of heme iron and lower cholesterol (47–49).
Our results also showed a significant positive association between red meat intake and levels of PAL-1, hs-CRP, and MCP-1; however, we observed a negative association between red meat consumption and HOMA-IR. Similar to our results, in two separate studies, including 482 Iranian women and 2,500 German individuals (both studies included healthy adults), greater red meat intake was associated with a higher plasma CRP concentration (50, 51). However, in a Dutch study, only processed meat intake was associated with higher CRP, while red meat intake was not (52). In a clinical trial, replacing energy from carbohydrates with protein from unprocessed lean red meat for 8 weeks did not augment inflammatory marker concentrations (19). In a randomized cross-over controlled trial in 36 individuals aged 39–97 years (53), 5 weeks of dietary treatment with 28, 113, or 153 g of lean red meat per day had similar advantageous effects on LDL-c and similar disadvantages effects on HDL-c. The authors include lean red meat in a diet to reduce cardiovascular disease (CVD) risk (53). This discrepancy with our study can be attributed to methodological or ethnic differences. In Iran, per capita, meat consumption is around 35.5 kg/year, comprising 30 gr of red meat and 60 gr of white meat per day (54). In our study, the average consumption of red meat is generally low compared to other countries such as 136 gr/d in 2021 in the united states (55). Indeed, that seems obese/overweight women in this study population even with a higher than the median red meat intake have just reached the ordinary intake of red meat intake like other populations (54, 55). Additionally, participants with higher red meat intake in the current study also had higher BFM levels and higher vegetable intakes; hence, all this information should be considered when interpreting our results. However, it seems that the mechanism is not completely clear and there is a need to design studies for further investigations. Monton et al. evaluated the association between the consumption of red meat and circulating hs-CRP levels in 2,198 men and women and expressed a positive association in these values (50). A diet high in red and processed meat can contribute to weight gain and body fat accumulation, which induces the obesity-related inflammatory process (41). However, decreasing body fat might be more relevant than lowering the intake of dietary red and processed meat to improve circulating levels of adipokines and inflammatory markers (31).
Iron may be another component of red meat contributing to the progression of metabolic abnormalities (56–58). Indeed, iron is a prooxidant that has been related to increased oxidative stress (59). High iron contents of red meat, especially heme iron, and meat processing particularly through high-temperature cooking, results in the formation of carcinogenic chemicals, including N-nitroso-compounds (NOC) and polycyclic aromatic hydrocarbons (PAH) (60, 61). Since inflammation is a key risk factor for metabolic diseases, our results may suggest that high red and processed meat consumption can affect metabolic disease development through the inflammatory pathway. Dietary intake of heme iron, abundant in red meat, has also been related to increased CVD risk especially inflammation (62), likely through inflammation and lipid peroxidation mechanisms.
A recent study reported that increased red meat consumption is associated with higher mortality risk in both women and men (63). Mercapturic acid of dihydroxynonane (DHN-MA), a substance that indicates the production of hydroxynonenal (HNE) in food or during digestion, is excreted in large amounts by diets that include heme iron and −6 fatty acids, such as hemoglobin or red meat and safflower oil (64, 65). Foods containing heme iron and PUFAs also contain HNE (66). The HNE, an end product of the oxidative breakdown of −6 fatty acids and a well-known bioactive marker of lipid peroxidation involved in cell growth control and signaling, is the main urinary metabolite of HNE, and it is also known as DHN-MA. As a result, DHN-MA has also been employed as an oxidative stress marker (67, 68).
It has been expressed that the red meat dietary intervention resulted in significant increments in plasma concentrations of trimethylamine-N-oxide (TMAO) (69) which has been linked to inflammation and CVD (70, 71). TMAO may cause inflammation. The inflammatory cytokines IL-1 and IL-18 were generated by the TXNIP-NLRP3 inflammasome after TMAO dramatically increased oxidative stress and activated it, but it also reduced the generation of nitric oxide (NO) and endothelial nitric oxide synthase (eNOS) (72). In addition preservatives such as sodium, nitrates, advanced glycation end products (AGEs), and their by-products may contribute to the relationship between red meat intake and increment of inflammation (73–75). Exposure to high temperatures can generate high levels of AGEs in meat, which have been shown to augment oxidative and inflammatory processes (73). Christ et al. (76) identified a functional role for NLRP3/IL-1β in the induction of innate immune memory in monocytes as triggered by a high intake of red meat and represented how this promotes inflammatory diseases (76). Apart from the meat cooking method of meat, enhancing flavor or improving preservation through methods such as smoking, salting, curing, or even adding chemical preservatives may affect oxidative stress and inflammation status (60, 77). The iron content in red meat has been related to upregulating inflammatory mediators, such as IL-6, IL-1β, and tumor necrosis factor-alpha (TNF-α) (78). Moreover, another proposed mechanism in the relationship between meat consumption and inflammation is probably related to the gut microbiome. It has also been displayed that red meat's gut microbiota-dependent breakdown metabolic processes can trigger inflammatory disease (70, 79). The most mechanistic relation of all kinds of meat on inflammation is present in Figure 1.
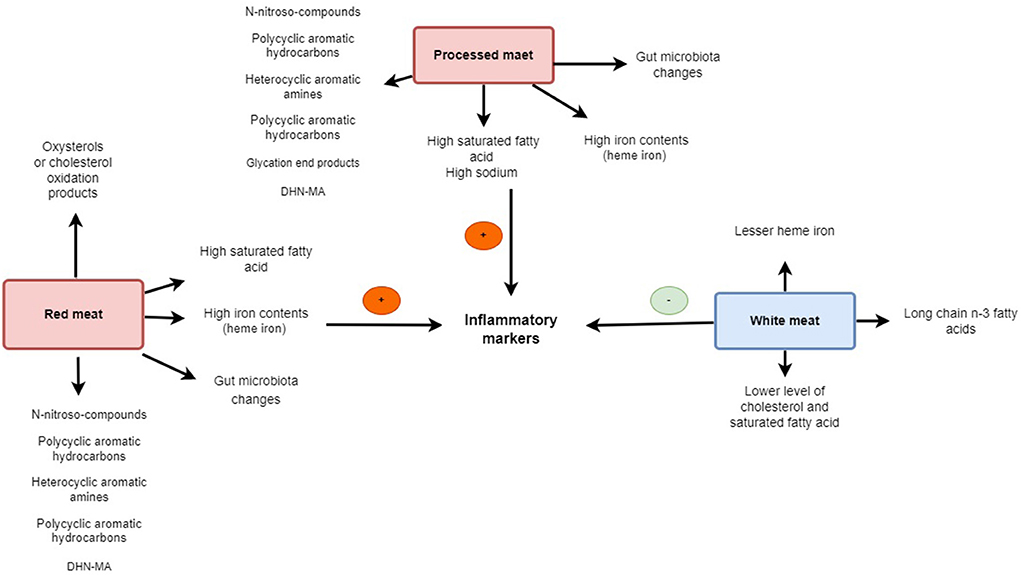
Figure 1. The scheme illustrates the different mechanisms of red, processed, and white meat on inflammation.
The present study has several strengths and limitations. First, this is the first study that evaluated the association between red, white, and processed meat on inflammatory and metabolic biomarkers in overweight and obese women. Second, dietary intake was assessed using a validated FFQ, which an experienced dietitian completed to decrease measurement errors. Anthropometric indices and body composition outcomes were assessed by the same person each time to improve the accuracy of the measurements (80). Nonetheless, a few limitations should be considered. First, the cross-sectional nature of the current study limited the ability to suggest a causal association between meat consumption and inflammation. Second, small errors might exist in the dietary assessment, mainly because of misremembering, overestimation, or underestimation of dietary intake and misclassification errors. Third, since our study included women only, the results are not generalizable to men and even to women with normal weight and other countries. fourth, cooking methods as important confounders didn't assess in the present study. Fifth, meat quality from the conditions of animal husbandry, especially environmental toxins, the type of food and water consumed, environmental conditions, to the stage of the cooking method and process, even genetic variation in dietary response, the culture and food habits of each society for eating of all types of meat can influence the relation of all kinds of meat with inflammation on that people. Sixth, further research must take into account other variables, such as menopause and the participant's hormonal state, which may have an impact on the accuracy of the findings. Seventh, the consumption ratio of all kinds of meats white to red meat or proceed meat, probably affects the inflammation that it is not possible to control the effect by only adjusting it in the analysis stage, and there is a need to design clinical trial studies in this field by proper diet intervention. Finally, although all the analyses were adjusted for potential confounders, residual confounding may still exist.
Conclusion
In conclusion, according to the results, it seems that the greater consumption of white meat with the mentioned mechanisms is probably negatively related to the inflammatory and metabolic factors. In contrast, the consumption of red and processed meat is positively related to the level of inflammatory factors in overweight and obese women. Of course, a higher intake of red meat had shown an association with a lower level of Gal-3 and insulin resistance, which seems that it is due to the amount of consumption that is lower than the average intake of the other population, and probably with more consumption of red meat, we might have faced different results. Perhaps the situation is clear regarding the recommendation to reduce the consumption of processed meats. However, there is a risk regarding the reduction of red meat consumption despite the reduction of inflammation, and there is concern regarding the consumption of white meat especially sea foods in Iran where some are habitual and some global concerns. Although in this suggestion, we must consider that Iran's estimated anemia prevalence ranges from 10 to 30%, with greater rates among children and teenagers, women with the prominent role of iron deficiency (81), and on the other hand, apart from poultry, seafood consumption in Iran is low due to eating habits and its consumption rate per capita is much lower (82). That change these conditions requires awareness of advantages and the provision of conditions. Other global concerns and risks are heavy metals pollution and the poisoning in sea foods (83, 84). Overall, it seems that it is very difficult to conclude the relationship of meat with inflammatory markers because limitations mentioned. It seems that to make a better judgment and suggestion for public health policy there is still a need for comprehensive studies to investigate the amount of meat consumed compared to the daily dietary protein requirement of individuals, the total energy received from that, along with taking into account controlling cooking methods, eating habits, etc. In addition, long-term controlled feeding studies are needed to confirm the causality of these associations and potential mediating pathways to determine optimal preventative dietary strategies for the progression of inflammation and inflammation-related diseases.
Data availability statement
The raw data supporting the conclusions of this article will be made available by the authors, without undue reservation.
Ethics statement
The studies involving human participants were reviewed and approved by Tehran University of Medical Sciences (Ethics Number: IR.TUMS.VCR.REC.1395.1597). The patients/participants provided their written informed consent to participate in this study.
Author contributions
FSH, DH, and AM wrote the paper. FSH performed the statistical analyses and revised the paper. KHM had full access to all of the data in the study and took responsibility for the integrity and accuracy of the data. AW, RB, and KS revised the paper. All authors read and approved the final manuscript.
Funding
This study was funded by grants from the Tehran University of Medical Sciences (TUMS) (Grant ID: 95-03-161-33142 and 96-01-161-34479).
Acknowledgments
The authors thank the study participants for their cooperation and assistance in physical examinations. They also thank those involved in nutritional evaluation and database management, as well as the Tehran University of Medical Sciences.
Conflict of interest
The authors declare that the research was conducted in the absence of any commercial or financial relationships that could be construed as a potential conflict of interest.
Publisher's note
All claims expressed in this article are solely those of the authors and do not necessarily represent those of their affiliated organizations, or those of the publisher, the editors and the reviewers. Any product that may be evaluated in this article, or claim that may be made by its manufacturer, is not guaranteed or endorsed by the publisher.
Abbreviations
FFQ, food frequency questionnaire; ANOVA, one-way analysis of variance; ANCOVA, analysis of covariance; MCP-1, macrophage inflammatory protein; hs-CRP, high-sensitivity C-reactive protein; PAI-1, plasminogen activator inhibitor 1; HOMA-IR, homeostasis model assessment of insulin resistance; Gal-3, Galectin-3; TGF-b, transforming growth factor; IL-1, interleukin 1; SFA, saturated fatty acid; IL-6, interleukin 6; BMI, body mass index; TUMS, Tehran University of Medical Sciences; FFQ, food frequency questionnaire; WC, waist circumference; TG, triglyceride; HC, hip circumference; NC, neck circumference; WHR, waist-to-hip ratio; WHtR, weight-to-height ratio; SMM, skeletal muscle mass; FFM, fat-free mass; BFM, body fat mass; BMC, bone mass content; SLM, soft lean mass; FMI, fat mass index; FFMI, fat-free mass index; FBS, fasting blood sugar; Chole, total cholesterol; LDL-c, Low-density lipoprotein cholesterol; HDL-c, High-density lipoprotein cholesterol; SGPT, Serum glutamic pyruvic transaminase; SGOT, serum glutamic-oxaloacetic transaminase; SBP, Systolic blood pressure; DBP, diastolic blood pressure; IPAQ, International Physical Activity Questionnaire; MET, Metabolic Equivalents; β, beta; CI, Confidence Interval; CVD, cardiovascular disease; PAH, polycyclic aromatic hydrocarbons; TNF-a, necrosis factor-alpha; GOF, goodness of fit; R2, R squared; AGEs, advanced glycation end products; NO, nitric oxide; eNOS, endothelial nitric oxide synthase; DHN-MA, Mercapturic acid of dihydroxynonane; HNE, hydroxynonenal; MUFA, monounsaturated fatty acids; SFA, saturated fatty acid; EPA, eicosapentaenoic acid; DHA, Docosahexaenoic acid.
References
1. Conklin AI, Ponce NA, Crespi CM, Frank J, Nandi A, Heymann J. Economic policy and the double burden of malnutrition: cross-national longitudinal analysis of minimum wage and women's underweight and obesity. Public Health Nutr. (2018) 21:940–7. doi: 10.1017/S1368980017003433
2. Arabzadegan N, Daneshzad E, Fatahi S, Moosavian SP, Surkan PJ, Azadbakht L. Effects of dietary whole grain, fruit, and vegetables on weight and inflammatory biomarkers in overweight and obese women. Eat Weight Disord. (2020) 25:1243–51. doi: 10.1007/s40519-019-00757-x
3. Lin X, Lim IY, Wu Y, Teh AL, Chen L, Aris IM, et al. Developmental pathways to adiposity begin before birth and are influenced by genotype, prenatal environment and epigenome. BMC Med. (2017) 15:1–18. doi: 10.1186/s12916-017-0800-1
4. Blum CA, Muller B, Huber P, Kraenzlin M, Schindler C, De Geyter C, et al. Low-grade inflammation and estimates of insulin resistance during the menstrual cycle in lean and overweight women. J Clin Endocrinol Metab. (2005) 90:3230–5. doi: 10.1210/jc.2005-0231
5. Pedrinelli R. Dell'Omo G, Di Bello V, Pellegrini G, Pucci L, Del Prato S, et al. Low-grade inflammation and microalbuminuria in hypertension. Arterioscler Thromb Vasc Biol. (2004) 24:2414–9. doi: 10.1161/01.ATV.0000147415.40692.7f
6. Garcia C, Feve B, Ferre P, Halimi S, Baizri H, Bordier L, et al. Diabetes and inflammation: fundamental aspects and clinical implications. Diabetes Metab. (2010) 36:327–38. doi: 10.1016/j.diabet.2010.07.001
7. Shoelson SE. Herrero L, Naaz A. Obesity, inflammation, and insulin resistance. Gastroenterology. (2007) 132:2169–80. doi: 10.1053/j.gastro.2007.03.059
8. Bagheri R, Rashidlamir A, Ashtary-Larky D, Wong A, Alipour M, Motevalli MS, et al. Does green tea extract enhance the anti-inflammatory effects of exercise on fat loss? Br J Clin Pharmacol. (2020) 86:753–62. doi: 10.1111/bcp.14176
9. Straczkowski M, Dzienis-Straczkowska S, Stêpieñ A, Kowalska I, Szelachowska M, Kinalska I. Plasma interleukin-8 concentrations are increased in obese subjects and related to fat mass and tumor necrosis factor-α system. J Clin Endocrinol Metab. (2002) 87:4602–6. doi: 10.1210/jc.2002-020135
10. Straczkowski M, Kowalska I, Nikolajuk A, Dzienis-Straczkowska S, Szelachowska M, Kinalska I. Plasma interleukin 8 concentrations in obese subjects with impaired glucose tolerance. Cardiovasc Diabetol. (2003) 2:1–5. doi: 10.1186/1475-2840-2-5
11. Eskandari M, Hooshmand Moghadam B, Bagheri R, Ashtary-Larky D, Eskandari E, Nordvall M, et al. Effects of interval jump rope exercise combined with dark chocolate supplementation on inflammatory adipokine, cytokine concentrations, and body composition in obese adolescent boys. Nutrients. (2020) 12:3011. doi: 10.3390/nu12103011
12. Visser M, Bouter LM, McQuillan GM, Wener MH, Harris TB. Elevated C-reactive protein levels in overweight and obese adults. JAMA. (1999) 282:2131–5. doi: 10.1001/jama.282.22.2131
13. Bagheri R, Rashidlamir A, Ashtary-Larky D, Wong A, Grubbs B, Motevalli MS et al. Effects of green tea extract supplementation and endurance training on irisin, pro-inflammatory cytokines, and adiponectin concentrations in overweight middle-aged men. Eur J Appl Physiol. (2020) 120:915–23. doi: 10.1007/s00421-020-04332-6
14. DeBoer MD. Obesity, systemic inflammation, and increased risk for cardiovascular disease and diabetes among adolescents: a need for screening tools to target interventions. Nutrition. (2013) 29:379–86. doi: 10.1016/j.nut.2012.07.003
15. Iacobini C, Menini S, Ricci C, Scipioni A, Sansoni V, Cordone S et al. Accelerated lipid-induced atherogenesis in galectin-3-deficient mice: role of lipoxidation via receptor-mediated mechanisms. Arterioscler Thromb Vasc Biol. (2009) 29:831–6. doi: 10.1161/ATVBAHA.109.186791
16. Henderson NC. SethiT. The regulation of inflammation by galectin-3. Immunol Rev. (2009) 230:160–71. doi: 10.1111/j.1600-065X.2009.00794.x
17. González-Gil EM, Santabárbara J, Russo P, Ahrens W, Claessens M, Lissner L, et al. Food intake and inflammation in European children: the IDEFICS study. Eur J Nutr. (2016) 55:2459–68. doi: 10.1007/s00394-015-1054-3
18. Galland L. Diet and inflammation. Nutr Clin Pract. (2010) 25:634–40. doi: 10.1177/0884533610385703
19. Hodgson JM, Ward NC, Burke V, Beilin LJ, Puddey IB, et al. Increased lean red meat intake does not elevate markers of oxidative stress and inflammation in humans. J Nutr. (2007) 137:363–7. doi: 10.1093/jn/137.2.363
20. Schwedhelm C, Pischon T, Rohrmann S, Himmerich H, Linseisen J, Nimptsch K. Plasma inflammation markers of the tumor necrosis factor pathway but not C-reactive protein are associated with processed meat and unprocessed red meat consumption in Bavarian adults. J Nutr. (2017) 147:78–85. doi: 10.3945/jn.116.237180
21. O'Connor LE, Kim JE, Clark CM, Zhu W, Campbell WW. Effects of total red meat intake on glycemic control and inflammatory biomarkers: a meta-analysis of randomized controlled trials. Adv Nutr. (2021) 12:115–27. doi: 10.1093/advances/nmaa096
22. Pan A, Sun Q, Bernstein AM, Schulze MB, Manson JE, Willett WC et al. Red meat consumption and risk of type 2 diabetes: 3 cohorts of US adults and an updated meta-analysis. Am J Clin Nutr. (2011) 94:1088–96. doi: 10.3945/ajcn.111.018978
23. Consortium I. Association between dietary meat consumption and incident type 2 diabetes: the EPIC-InterAct study. Diabetologia. (2013) 56:47–59. doi: 10.1007/s00125-012-2718-7
24. Feskens EJ, Sluik D, van Woudenbergh GJ. Meat consumption, diabetes, and its complications. Curr Diab Rep. (2013) 13:298–306. doi: 10.1007/s11892-013-0365-0
25. Fung TT, Schulze M, Manson JE, Willett WC, Hu FB. Dietary patterns, meat intake, and the risk of type 2 diabetes in women. Arch Intern Med. (2004) 164:2235–40. doi: 10.1001/archinte.164.20.2235
26. Van Dam RM, Willett WC, Rimm EB, Stampfer MJ, Hu FB. Dietary fat and meat intake in relation to risk of type 2 diabetes in men. Diabetes Care. (2002) 25:417–24. doi: 10.2337/diacare.25.3.417
27. Tappel A. Heme of consumed red meat can act as a catalyst of oxidative damage and could initiate colon, breast and prostate cancers, heart disease and other diseases. Med Hypotheses. (2007) 68:562–4. doi: 10.1016/j.mehy.2006.08.025
28. Ciubotaru I, Lee YS, Wander RC. Dietary fish oil decreases C-reactive protein, interleukin-6, and triacylglycerol to HDL-cholesterol ratio in postmenopausal women on HRT. J Nutr Biochem. (2003) 14:513–21. doi: 10.1016/S0955-2863(03)00101-3
29. Trebble T, Arden NK, Stroud MA, Wootton SA, Burdge GC, Miles EA, et al. Inhibition of tumour necrosis factor-α and interleukin 6 production by mononuclear cells following dietary fish-oil supplementation in healthy men and response to antioxidant co-supplementation. Br J Nutr. (2003) 90:405–12. doi: 10.1079/BJN2003892
30. Pischon T, Hankinson SE, Hotamisligil GS, Rifai N, Willett WC, Rimm EB. Habitual dietary intake of n-3 and n-6 fatty acids in relation to inflammatory markers among US men and women. Circulation. (2003) 108:155–60. doi: 10.1161/01.CIR.0000079224.46084.C2
31. Koster A, Stenholm S, Alley DE, Kim LJ, Simonsick EM, Kanaya AM, et al. Body fat distribution and inflammation among obese older adults with and without metabolic syndrome. Obesity. (2010) 18:2354–61. doi: 10.1038/oby.2010.86
32. Cohen E, Margalit I, Shochat T, Goldberg E, Krause I. Markers of chronic inflammation in overweight and obese individuals and the role of gender: a cross-sectional study of a large cohort. J Inflamm Res. (2021) 14:567. doi: 10.2147/JIR.S294368
33. Willett W. Issues in analysis and presentation of dietary data. Nutr Epidemiol. (1998). doi: 10.1093/acprof:oso/9780195122978.003.13
34. Graf S, Karsegard VL, Viatte V, Heidegger CP, Fleury Y, Pichard C et al. Evaluation of three indirect calorimetry devices in mechanically ventilated patients: which device compares best with the Deltatrac II®? A prospective observational study. Clin Nutr. (2015) 34:60–5. doi: 10.1016/j.clnu.2014.01.008
35. Neelakantan N, Whitton C, Seah S, Koh H, Rebello SA, Lim JY et al. Development of a semi-quantitative food frequency questionnaire to assess the dietary intake of a multi-ethnic urban Asian population. Nutrients. (2016) 8:528. doi: 10.3390/nu8090528
36. Ghaffarpour M, Houshiar-Rad A, Kianfar H. The Manual for Household Measures, Cooking Yields Factors and Edible Portion of Foods. Tehran: Nashre Olume Keshavarzy (1999).
37. Gutch M, Kumar S, Razi SM, Gupta KK, Gupta A. Assessment of insulin sensitivity/resistance. Indian J Endocrinol Metab. (2015) 19:160. doi: 10.4103/2230-8210.146874
38. Klishadi R, Khosravi A, Famouri F, Sadeghi M, Shirani S, et al. Assessment of physical activity of adolescents in Isfahan. J Shahrekord Univ Med Sci. (2001) 3. Available online at: http://eprints.skums.ac.ir/id/eprint/5577
39. Altman DG, Bland JM. Statistics notes: the normal distribution. BMJ. (1995) 310:298. doi: 10.1136/bmj.310.6975.298
40. Pallant J, Manual SS. A step by step guide to data analysis using SPSS for windows. SPSS Surv Manual. (2007) 14:20–30.
41. Chai W, Morimoto Y, Cooney RV, Franke AA, Shvetsov YB, Le Marchand L, et al. Dietary red and processed meat intake and markers of adiposity and inflammation: the multiethnic cohort study. J Am Coll Nutr. (2017) 36:378–85. doi: 10.1080/07315724.2017.1318317
42. Papier K, Hartman L, Tong TY, Key TJ, Knuppel A. Higher meat intake is associated with higher inflammatory markers, mostly due to adiposity: results from UK biobank. J Nutr. (2022) 152:183–9. doi: 10.1093/jn/nxab314
43. Fernández-Real JM, López-Bermejo A, Ricart W. Cross-talk between iron metabolism and diabetes. Diabetes. (2002) 51:2348–54. doi: 10.2337/diabetes.51.8.2348
44. Esmaillzadeh A, Kimiagar M, Mehrabi Y, Azadbakht L, Hu FB, Willett WC. Dietary patterns and markers of systemic inflammation among Iranian women. J Nutr. (2007) 137:992–8. doi: 10.1093/jn/137.4.992
45. Lopez-Garcia E, Schulze MB, Fung TT, Meigs JB, Rifai N, Manson JE et al. Major dietary patterns are related to plasma concentrations of markers of inflammation and endothelial dysfunction. Am J Clin Nutr. (2004) 80:1029–35. doi: 10.1093/ajcn/80.4.1029
46. Fung TT, Rimm EB, Spiegelman D, Rifai N, Tofler GH, Willett WC, et al. Association between dietary patterns and plasma biomarkers of obesity and cardiovascular disease risk. Am J Clin Nutr. (2001) 73:61–7. doi: 10.1093/ajcn/73.1.61
47. Grieger JA, MillerMD, Cobiac L. Investigation of the effects of a high fish diet on inflammatory cytokines, blood pressure, and lipids in healthy older Australians. Food Nutr Res. (2014) 58:20369. doi: 10.3402/fnr.v58.20369
48. Kemp DC, Kwon JY. Fish and shellfish-derived anti-inflammatory protein products: properties and mechanisms. Molecules. (2021) 26:3225. doi: 10.3390/molecules26113225
49. Bingham SA, Hughes R, Cross AJ. Effect of white versus red meat on endogenous N-nitrosation in the human colon and further evidence of a dose response. J Nutr. (2002) 132:3522S−5S. doi: 10.1093/jn/132.11.3522S
50. Montonen J, Boeing H, Fritsche A, Schleicher E, Joost HG, Schulze MB et al. Consumption of red meat and whole-grain bread in relation to biomarkers of obesity, inflammation, glucose metabolism and oxidative stress. Eur J Nutr. (2013) 52:337–45. doi: 10.1007/s00394-012-0340-6
51. Azadbakht L, Esmaillzadeh A. Red meat intake is associated with metabolic syndrome and the plasma C-reactive protein concentration in women. J Nutr. (2009) 139:335–9. doi: 10.3945/jn.108.096297
52. Van Woudenbergh GJ, Kuijsten A, Tigcheler B, Sijbrands EJ, Van Rooij FJ, Hofman A, et al. Meat consumption and its association with C-reactive protein and incident type 2 diabetes: the rotterdam study. Diabetes Care. (2012) 35:1499–505. doi: 10.2337/dc11-1899
53. Roussell MA, Hill AM, Gaugler TL, West SG, Vanden Heuvel JP, Alaupovic P, et al. Beef in an optimal lean diet study: effects on lipids, lipoproteins, and apolipoproteins. Am J Clin Nutr. (2012) 95:9–16. doi: 10.3945/ajcn.111.016261
54. Ranaei V, Pilevar Z, Esfandiari C, Khaneghah AM, Dhakal R, Vargas-Bello-Pérez E et al. Meat value chain losses in Iran. Food Sci Anim Resour. (2021) 41:16–33. doi: 10.5851/kosfa.2020.e52
55. Shahbandeh M. Per capita consumption of red meat in the United States from 2017 to 2031(in pounds). (2022). Available online at: https://www.statista.com/statistics/183627/per-capita-consumption-of-red-meat-in-the-us-since-2000/
56. Bernstein AM, Willett WC. Conclusions of the optimal lean diet study go beyond the data. Am J Clin Nutr. (2012) 95:1294–5. doi: 10.3945/ajcn.112.034363
57. Micha R, Michas G, Mozaffarian D. Unprocessed red and processed meats and risk of coronary artery disease and type 2 diabetes–an updated review of the evidence. Curr Atheroscler Rep. (2012) 14:515–24. doi: 10.1007/s11883-012-0282-8
58. Micha R, Michas G, Lajous M, Mozaffarian D. Processing of meats and cardiovascular risk: time to focus on preservatives. BMC Med. (2013) 11:136. doi: 10.1186/1741-7015-11-136
59. Rajpathak SN, Crandall JP, Wylie-Rosett J, Kabat GC, Rohan TE, Hu FB. The role of iron in type 2 diabetes in humans. Biochim Biophys Acta. (2009) 1790:671–81. doi: 10.1016/j.bbagen.2008.04.005
60. Bouvard V, Loomis D, Guyton KZ, Grosse Y, El Ghissassi F, Benbrahim-Tallaa L, et al. Carcinogenicity of consumption of red and processed meat. Lancet Oncol. (2015) 16:1599–600. doi: 10.1016/S1470-2045(15)00444-1
61. Turner N, Lloyd SK. Association between red meat consumption and colon cancer: a systematic review of experimental results. Exp Biol Med. (2017) 242:813–39. doi: 10.1177/1535370217693117
62. Fang X, An P, Wang H, Wang X, Shen X, Li X, et al. Dietary intake of heme iron and risk of cardiovascular disease: a dose-response meta-analysis of prospective cohort studies. Nutr Metab Cardiovasc Dis. (2015) 25:24–35. doi: 10.1016/j.numecd.2014.09.002
63. Zheng Y, Li Y, Satija A, Pan A, Sotos-Prieto M, Rimm E, et al. Association of changes in red meat consumption with total and cause specific mortality among US women and men: two prospective cohort studies. BMJ. (2019) 365:l2110. doi: 10.1136/bmj.l2110
64. Pierre F, Santarelli R, Taché S, Guéraud F, Corpet DE. Beef meat promotion of dimethylhydrazine-induced colorectal carcinogenesis biomarkers is suppressed by dietary calcium. Br J Nutr. (2008) 99:1000–6. doi: 10.1017/S0007114507843558
65. Pierre F, Peiro G, Taché S, Cross AJ, Bingham SA, Gasc N, et al. New marker of colon cancer risk associated with heme intake: 1, 4-dihydroxynonane mercapturic acid. Cancer Epidemiol Biomarkers Prev. (2006) 15:2274–9. doi: 10.1158/1055-9965.EPI-06-0085
66. Gasc N, Taché S, Rathahao E, Bertrand-Michel J, Roques V, Guéraud F. 4-hydroxynonenal in foodstuffs: heme concentration, fatty acid composition and freeze-drying are determining factors. Redox Report. (2007) 12:40–4. doi: 10.1179/135100007X162257
67. Zarkovic N. 4-Hydroxynonenal as a bioactive marker of pathophysiological processes. Mol Aspects Med. (2003) 24:281–91. doi: 10.1016/S0098-2997(03)00023-2
68. Peiro G, Alary J, Cravedi JP, Rathahao E, Steghens JP, Guéraud F. Dihydroxynonene mercapturic acid, a urinary metabolite of 4-hydroxynonenal, as a biomarker of lipid peroxidation. Biofactors. (2005) 24:89–96. doi: 10.1002/biof.5520240110
69. Wang Z, Bergeron N, Levison BS Li XS, Chiu S, Jia X, et al. Impact of chronic dietary red meat, white meat, or non-meat protein on trimethylamine N-oxide metabolism and renal excretion in healthy men and women. Eur Heart J. (2019) 40:583–94. doi: 10.1093/eurheartj/ehy799
70. Koeth RA, Wang Z, Levison BS, Buffa JA, Org E, Sheehy BT, et al. Intestinal microbiota metabolism of L-carnitine, a nutrient in red meat, promotes atherosclerosis. Nat Med. (2013) 19:576–85. doi: 10.1038/nm.3145
71. Schiattarella GG, Sannino A, Toscano E, Giugliano G, Gargiulo G, Franzone A, et al. Gut microbe-generated metabolite trimethylamine-N-oxide as cardiovascular risk biomarker: a systematic review and dose-response meta-analysis. Eur Heart J. (2017) 38:2948–56. doi: 10.1093/eurheartj/ehx342
72. Sun X, Jiao X, Ma Y, Liu Y, Zhang L, He Y et al. Trimethylamine N-oxide induces inflammation and endothelial dysfunction in human umbilical vein endothelial cells via activating ROS-TXNIP-NLRP3 inflammasome. Biochem Biophys Res Commun. (2016) 481:63–70. doi: 10.1016/j.bbrc.2016.11.017
73. Clarke RE, Dordevic AL, Tan SM, Ryan L, Coughlan MT. Dietary advanced glycation end products and risk factors for chronic disease: a systematic review of randomised controlled trials. Nutrients. (2016) 8:125. doi: 10.3390/nu8030125
74. Raubenheimer K, Bondonno C, Blekkenhorst L, Wagner KH, Peake JM, Neubauer O. Effects of dietary nitrate on inflammation and immune function, and implications for cardiovascular health. Nutr Rev. (2019) 77:584–99. doi: 10.1093/nutrit/nuz025
75. Zhu H, Pollock NK, Kotak I, Gutin B, Wang X, Bhagatwala J, et al. Dietary sodium, adiposity, and inflammation in healthy adolescents. Pediatrics. (2014) 133:e635–42. doi: 10.1542/peds.2013-1794
76. Christ A, Günther P, Lauterbach MA, Duewell P, Biswas D, Pelka K, et al. Western diet triggers NLRP3-dependent innate immune reprogramming. Cell. (2018) 172:162–75.e14. doi: 10.1016/j.cell.2017.12.013
77. Wolk A. Potential health hazards of eating red meat. J Intern Med. (2017) 281:106–22. doi: 10.1111/joim.12543
78. Klaunig JE, Kamendulis LM. The role of oxidative stress in carcinogenesis. Annu Rev Pharmacol Toxicol. (2004) 44:239–67. doi: 10.1146/annurev.pharmtox.44.101802.121851
79. O'Neill LA, Zaslona Z. Macrophages remember cheeseburgers and promote inflammation via NLRP3. Trends Mol Med. (2018) 24:335–7. doi: 10.1016/j.molmed.2018.02.005
80. Mirmiran P, Esfahani FH, Mehrabi Y, Hedayati M, Azizi F. Reliability and relative validity of an FFQ for nutrients in the Tehran lipid and glucose study. Public Health Nutr. (2010) 13:654–62. doi: 10.1017/S1368980009991698
81. WHO. Iran (Islamic Rrepublic of). The database on Anaemia Includes Data by Country on Prevalence of Anaemia and Mean Haemoglobin Concentration. Vitamin and Mineral Nutrition Information System. Geneva, World Health Organization (2007).
83. Mansfield B. Is fish health food or poison? Farmed fish and the material production of un/healthy nature. Antipode. (2011) 43:413–34. doi: 10.1111/j.1467-8330.2010.00743.x
Keywords: red meat, white meat, inflammatory markers, processed meat, obesity, women
Citation: Shiraseb F, Hosseininasab D, Mirzababaei A, Bagheri R, Wong A, Suzuki K and Mirzaei K (2022) Red, white, and processed meat consumption related to inflammatory and metabolic biomarkers among overweight and obese women. Front. Nutr. 9:1015566. doi: 10.3389/fnut.2022.1015566
Received: 09 August 2022; Accepted: 27 October 2022;
Published: 10 November 2022.
Edited by:
Mainul Haque, National Defence University of Malaysia, MalaysiaReviewed by:
Fatemeh Haidari, Ahvaz Jundishapur University of Medical Sciences, IranRahnuma Ahmad, Medical College for Women and Hospital, Bangladesh
Kona Chowdhury, Gonoshathaya Samaj Vittik Medical College, Bangladesh
Copyright © 2022 Shiraseb, Hosseininasab, Mirzababaei, Bagheri, Wong, Suzuki and Mirzaei. This is an open-access article distributed under the terms of the Creative Commons Attribution License (CC BY). The use, distribution or reproduction in other forums is permitted, provided the original author(s) and the copyright owner(s) are credited and that the original publication in this journal is cited, in accordance with accepted academic practice. No use, distribution or reproduction is permitted which does not comply with these terms.
*Correspondence: Khadijeh Mirzaei, mirzaei_kh@tums.ac.ir