- 1School of Science and the Environment/Boreal Ecosystems Research Initiative, Memorial University of Newfoundland, Corner Brook, NL, Canada
- 2Neonatal Intensive Care Unit, Orlando Health Winne Palmer Hospital for Women and Babies, Orlando, FL, United States
- 3Department of Pediatric Newborn Medicine, Brigham and Women’s Hospital, Boston, MA, United States
- 4Harvard Medical School, Boston, MA, United States
- 5Department of Biochemistry, Memorial University of Newfoundland, St. John’s, NL, Canada
- 6Department of Mathematics, University of Bari Aldo Moro, Bari, Italy
Breast milk is the ideal source of nutrients for infants in early life. Lipids represent 2–5% of the total breast milk composition and are a major energy source providing 50% of an infant’s energy intake. Functional lipids are an emerging class of lipids in breast milk mediating several different biological functions, health, and developmental outcome. Lipidomics is an emerging field that studies the structure and function of lipidome. It provides the ability to identify new signaling molecules, mechanisms underlying physiological activities, and possible biomarkers for early diagnosis and prognosis of diseases, thus laying the foundation for individualized, targeted, and precise nutritional management strategies. This emerging technique can be useful to study the major role of functional lipids in breast milk in several dimensions. Functional lipids are consumed with daily food intake; however, they have physiological benefits reported to reduce the risk of disease. Functional lipids are a new area of interest in lipidomics, but very little is known of the functional lipidome in human breast milk. In this review, we focus on the role of lipidomics in assessing functional lipid composition in breast milk and how lipid bioinformatics, a newly emerging branch in this field, can help to determine the mechanisms by which breast milk affects newborn health.
Introduction
Breast milk is a complex biofluid of carbohydrates, lipids, proteins, enzymes, vitamins, and hormones. Lipids play several roles in an infant’s development and health (1). Functional lipids refer to lipids conferring known health benefits beyond basic nutritional requirements. Functional lipids include medium-chain triglycerides (MCTs), diacylglycerides (DAGs), fatty acid hydroxy fatty acids (FAHFA), conjugated linoleic, or linolenic acids (CLAs or CLNs), omega-3, omega-6, and omega-9 fatty acids, phospholipids, plasmalogens, and sphingolipids. MCTs are quickly hydrolyzed by gastrointestinal lipases and bile salts are not required for their hydrolyzation. The hydrolyzed products are absorbed and passed directly to the liver. As such, MCTs are not normally stored as body fat (2). Phospholipids play a crucial role in cell membrane integrity, and neural and brain development, as well as modulate the inflammatory responses in infants (3–6). Ceramide (Cer) and sphingomyelin are involved in various cellular functions such as proliferation, differentiation, apoptosis, and inflammation (7). In the cell membrane, they act as a platform for hormonal and growth factor receptors and cell signaling (8), as well as aid in the development of the immune system in infants (9). Plasmalogens play a role in the prevention of oxidative stress and act as anti-inflammatory agents in infants during the response to infection (10, 11). FAHFAs have been shown to possess anti-inflammatory and anti-diabetic properties (12). The word lipidome describes the whole set of lipid species in a cell or organ (13). Lipidomics is the study of the entire cellular lipidome at the molecular level (14). Multiple recent advances in mass spectrometric technology and workflows provide accurate analytical approaches for the identification and quantification of lipidome in biological samples, including functional lipid composition. The information generated can help to better understand how lipid metabolic pathways, metabolic flux, and network integration culminate from a systems biology perspective to impact human nutrition and health outcomes (15).
Although the beneficial effects of lipids in health promotion are widely studied, there is limited data on the structure, function, composition, and potential health benefits of functional lipids present in breast milk lipidome and their role in improving pediatric health outcomes. Applying lipidomics to the analysis of breast milk lipidome can aid in the understanding of the composition of functional lipids in breast milk and how they vary with maternal physiology. This can also assist in identifying possible contributions to infant development, lactational programming of transgenerational obesity, and pediatric health outcomes. To fill this gap, this review will discuss breast milk lipidome and the role of lipidomics in assessing breast milk functional lipid composition.
Lipidomics
Lipidomics is a subset of metabolomics aimed at studying the metabolism, structure, and function of lipids and their roles in modulating health and disease outcome. This field has the potential to identify biomarkers for diagnosis, prognosis, prevention, and therapeutic response for various diseases (16). Innovations in mass spectrometry technology have facilitated the advancement of lipidomics as a subdiscipline in omics over recent years. The typical workflow of lipidomics consists of three major processes: sample preparation and extraction; mass spectrometry data acquisition; and data processing and interpretation (16). A summary of these processes in the context of breast milk is illustrated in Figure 1.
Sample preparation
Sampling
Human breast milk studies have used several sampling protocols such as full expression, expressed alternate breast, mid-feed sampling, and fore and hind sampling. Full expression is a collection of whole milk from the breast. Expressed alternate breast refers to the collection of milk from the opposite breast of infant feeding. Mid-feed sampling is the collection of milk after 3–5 min of feeding. Foremilk sampling is the collection of milk prior to the infant feeding and hind milk is the sample right before the infant completes feeding. Furthermore, breast milk can be collected either manually or via electric pump (17, 18) and the fat content in the collected milk can vary during a feed. Foremilk sample contains lower fat compared to the mid and hindmilk samples (19). Fat content in milk varies from morning to evening as well. Fat content is high in evening milk compared to samples collected in the morning (20). Fat content also increases from colostrum to mature milk (21). The fat percentage of milk varies with time, feed, lactation stage, and sampling methods. The ideal method of sampling is milk collection from full expression of both breasts over 24 h with a sub-sample used for analysis. However, this is not often feasible (17, 22). Variability in milk fat with different sampling techniques necessitates those studies of human milk lipids standardize collection techniques within a study, balancing scientific rigor with practical limitations in milk collection for a given population.
Storage
Proper storage is critical for preventing lipolysis activity by breast milk lipase. Immediate analysis can prevent compositional changes caused by lipase activity; however, this is not always possible. To prevent lipase activity, milk samples should be stored in the freezer at –20°C for 8 days or less or –80°C for longer storage (23, 24).
Lipid extraction methods
Modified Bligh and Dyer, Folch, methyl tert-butyl ether (MTBE), and butanol/methanol (BUME, 3:1, v/v) are the most popular extraction techniques generally used in breast milk lipidomics (25–27). Each of these techniques has pros and cons for lipid extraction and are described briefly below.
Modified Bligh and Dyer method
Chloroform/methanol/water (1:1:0.9, V/V/V), followed by a water wash is used to extract lipids from the whole breast milk sample. After phase separation the chloroform phase contains the total lipids. This is a widely practiced method and is very effective in extracting large amounts of lipids from the sample. The drawback of this method is the use of a hazardous solvent (chloroform) and chloroform may carry water-soluble contents during the extraction of breast milk (25, 26).
Modified Folch method
A total of 20 mL chloroform and methanol (chloroform: methanol 2:1) were used to extract lipids from 1 mL of breast milk (28). Then, 0.2 equivalents (of the total volume of the chloroform/methanol mixture) of water are added to the suspension to induce phase separation. It has similar advantages and disadvantages as the Modified Bligh and Dyer method (25, 27). Furthermore, This approach was unable to extract low-concentration breast milk lipids, particularly glycerol phospholipids and sphingolipids (29).
Methyl tert-butyl ether method
Methyl tert-butyl ether (MTBE)/methanol/water can be used to efficiently extract lipids from breast milk sample. This method does not contain a hazardous solvent such as chloroform. After phase separation, the MTBE is in the upper layer. It is used in high productivity automated lipidomics applications. The disadvantages of this method are the MTBE phase carry a significant amount of water-soluble components during extraction and MTBE is an expensive solvent (25, 30).
Butanol/methanol (BUME, 3:1, v/v) method
In the butanol/methanol (3:1, V/V) method, an equal volume of heptane/ethyl acetate (3:1, V/V) and 1% acetic acid is used to extract lipids from the sample. The 1% acetic acid is used to induce phase separation. This method does not carry any water-soluble components with it. The limitation of this method is that the evaporation of butanol is very difficult and limit high throughput applications (31).
Recommended lipid extraction methods for breast milk
The Bligh and Dyer and the Folch method are similar extraction techniques. However, the Bligh and Dyer method is a less time-consuming method and it give great lipid recovery. The MTBE method reduces cross-contamination compared to the other two methods. We recommend the Modified Bligh and Dyer method for breast milk extraction because of its simplicity, superior lipid yield, and capacity for high throughput sample extraction.
Mass-spectrometry-based lipidomic approaches
Following lipid extraction, shotgun lipidomics, separation-based lipidomics, and imaging or spatial lipidomics are three mass spectrometry-based approaches commonly used to assess the lipidome in biological samples and can be applied to breast milk.
Shotgun lipidomics
Shotgun lipidomics is also known as direct infusion-based lipidomics since there is no chromatographic separation performed before the sample is injected in the mass spectrometer. The lipids are ionized by a source, typically an electrospray source, to produce negative or positive ions representative of the lipids in the sample. The lipid extraction is completed by several extraction strategies based on stability, hydrophobicity, and reactivity of the lipid classes and subclasses (32). Pre-step approaches, such as derivatization, pre-separation methods, or ionization sources [such as electro spray ionization (ESI), atmospheric pressure chemical ionization (APCI), or atmospheric pressure photoionization (APPI)] are used to ionize the different lipid molecular species depending on the difficulty associated with ionization (33). The ionization of lipids is based on selective ionization. Typically, different lipid classes have different charges due to their chemical structure or composition. This is referred to as intra-source separation. For example, polar lipids are separated based on their polar head group (34). There are three approaches used in shotgun lipidomics and include tandem mass spectrometry-based shotgun lipidomics, high mass accuracy-based shotgun lipidomics, and multi-dimensional-based shotgun lipidomics (32). Shotgun lipidomics is commonly used in the lipid characterization of breast milk, especially phospholipids and Regio isomeric TAG (35, 36).
Tandem mass spectrometry-based shotgun lipidomics
Tandem mass spectrometry is known as MS/MS and is performed complimentary with full scan. In this approach, lipid molecules are separated according to distinct structure and class characteristics using precursor ions and specific neutral loss scans giving fragments diagnostic for example to identify the head groups in different classes of polar or membrane lipids (16).
This approach includes several advantages such as simplicity, high sensitivity, easy operational handling, and low-cost instrumentation (32). However, the selection of internal standards is difficult. Differential fragmentation kinetics of individual molecular species can influence the accuracy of the quantification and structural integrities (37, 38). Tandem mass based approaches are very effective in identifying compositional and structural features of milk lipids, especially glycerophospholipids and TAG molecular species or regioisomers (36).
High mass accuracy-based shotgun lipidomics
High mass accuracy can be used in quantitative and qualitative lipid analysis. This technique measures the ratio between mass and charge of fragment ions. This approach has several benefits, such as high efficiency, multifaceted, and precise measurements of lipid molecular species (32).
Multi-dimensional mass spectrometry-based shotgun lipidomics
Multi-dimensional mass spectrometry-based shotgun lipidomics (MDMS-SL) combines a full mass scan and all tandem mass spectrometric scans. A two-step quantification procedure is used in MDMS-SL to identify the lipid molecular species (39). Identification of individual lipid species is done by using characteristic structural units or fragments that is diagnostic of each lipid molecules (40). A two-dimensional mass map can be obtained in MDMS-SL (41). By using this approach, the lipidome from a small number of samples can be analyzed (33, 39). This method assists with identifying the individual lipid molecular species and their isomers. MDMS-SL needs less width factor compared to liquid chromatography-mass spectrometry-based lipidomics (39). Precise identification and quantification of a whole set of lipids can be obtained by this method.
Separation based lipidomics
Liquid chromatography-mass spectrometry-based lipidomics
Liquid chromatography-mass spectrometry (LC-MS) is a highly effective lipidomics analysis platform that simplifies the analytical protocol and increases chromatographic segregation power and precision of detection. It can detect several lipids in a very short time (42). It is typically used in breast milk lipidomics for the identification of triglycerides and phospholipids (43). Selected and multiple reaction monitoring (SRM/MRM) are two strong quantitative methods in LCMS-based targeted analysis. These methods play a crucial role in clinical lipidomic analysis and identification of untargeted lipids (44). Data-based analysis is one of the unique procedures in LC-MS used for the identification and classification of individual lipid species by selecting most of the ions in a peak width after pre-separation. Higher peaks for untargeted analysis can be obtained in two-dimensional liquid chromatography (2D-LC) technology (45). There are two types of 2D-LC methods, namely: Reversed-phase LC (RPLC) and Normal-phase LC (NPLC). The separation of lipids using RPLC method is based on their hydrophobicity, chain length, and degree of unsaturation of fatty acyl chains. Lipid species are eluted in the following order: lipids containing longer acyl chains followed by shorter chain lipids, saturated, and polyunsaturated species. In NPLC, lipid species are identified according to their hydrophilic functionalities and their characteristic head-groups into distinct classes (15). The combination of RPLC and NPLC is the most superior separation technique in 2D-LC methods (32). RPLC classically used nonpolar C18 columns with polar solvents. Nowadays, C30 stationary phase is also used to conduct untargeted lipidomics in biological samples (46, 47).
C30 reverse phase chromatography is superior to C18 reverse phase chromatography for identifying geometric lipid isomers (48, 49). The optimized C30 reverse phase chromatography allows for excellent intra-class separation of lipid isomers with different fatty acid compositions or head group modifications. Chromatography coupled with high- resolution tandem mass spectrometry can efficiently identify lipid isomers. As a result, it can differentiate di/triglycerides, plasmalogens, and ether iso-forms of lipids in a biological sample (50).
Hydrophilic interaction liquid chromatography (HILIC) is another lipid separation technique in LC -based mass spectrometry analysis. The combination of columns with a polar stationary phase and reverse phase solvents (acetonitrile and water) is used in HILIC. Lipid separation occurs at the water/silica interface. HILIC separation is based on polar head groups, therefore they separate lipids into distinct classes based on head group composition (51, 52). Phospholipids are also identified via LC-MS. However, for the quantification of phospholipid molecular species, a pure standard is necessary (53). The characterization and quantification of phospholipids and sphingomyelins in breast milk is generally accomplished by using a high-performance liquid chromatography (HPLC) coupled with mass spectrometer detector. UHPLC system coupled with a high-resolution mass spectrometer are used in the identification of triglycerides and fatty acids in breast milk (54).
Other separation methods
Other separation-based approaches for lipid identification include gas chromatography (GC) and thin layer chromatography (TLC). GC offers a high separation, resolution, and quantification accuracy. It is suitable for lipids with high volatility and good thermal stability (32). In breast milk lipidomics research, GC with flame ionization detection (GC-FID) and GC coupled to MS (GC–MS) are commonly employed to identify total fat and fatty acid content (55–57). Due to the low volatility of phospholipids in breast milk, GC-MS cannot be used to identify it (58). Non-volatile compounds are analyzed using TLC. It is simple to use, but it lacks the separation resolution of other mass-based approaches (32). It is commonly used to identify short and long chain fatty acids, however, it is rarely used in breast milk lipidomics studies (59).
Imaging mass spectrometry
Imaging mass spectrometry (IMS) is a new lipid imaging method which is utilized to identify the spatial distribution of lipids in a sample using two-dimensional MS technique. Several mass spectrometric-based imaging techniques are available and are differentiated based on whether they operate under vacuum or at atmospheric conditions with or without the use of a matrix. IMS has different types, such as matrix-assisted laser desorption/ionization-mass spectrometry (MALDI-MS), desorption electrospray ionization mass spectrometry (DESI-MS), secondary ion mass spectrometry (SIMS), and laser ablation secondary ion mass spectrometry (LASI-MS) (60, 61). Though imaging mass spectrometry is seldomly used in breast milk lipidomics; it has the capacity to analyze the lipid droplets or milk fat globule in breast milk especially when using applications with high spatial resolution.
Matrix-assisted laser desorption/ionization-mass spectrometry imaging
MALDI-IMS is used to identify lipid molecules with high-resolution imaging (62, 63). The mass to charge ratios of ionized forms of molecules are used in the identification. This process involves three major steps. First, the ionizing source changes the form of molecules into ions. Next, the mass analyzer separates the ions based on mass to charge ratio. Then, the ion detector detects and separates the ions based on their relative abundance. This is a suitable technique to visualize lipid distribution and concentration in a sample (64). This technique can assist with identifying the localization of known and unknown molecules without labeling and helps to identify lipid biomarkers based on spatial location (65).
Desorption electrospray ionization imaging
DESI imaging is a commonly used atmospheric pressure-based MS imaging technique (66). In DESI, charged primary organic molecules in a solution touch the top of the sample surface with definite kinetic energy. This causes the secondary molecules to move along the surface due to the action of the primary droplets. The samples are split into nanoparticles and become charged. The charged ion then enters the mass spectrometer after the charge transfer process which moves through an electric field (67). DESI assesses the inner lipid structure of biomolecules and is the best spectrometry for the surface analysis of samples. This technique does not require a matrix and is performed under atmospheric conditions (68, 69).
Secondary ion mass spectrometry imaging
SIMS imaging is carried out via the passage of a high-energy ion beam along the surface of a sample. The high-energy beam hits on the outer part of the sample producing a secondary ion. The resolution level of SIMS imaging is equal to the resolution level of optical microscopes. This technique has been applied in single-cell lipid imaging. The challenge is that it is not a soft ionization technique and lipids suffer from fragmentation using this method. The advantage of SIMS is that its ultrahigh resolution, produces three-dimensional images by separating the sample into layers at the nanometer scale (70).
Recommended chromatographic and mass spectrometry method for breast milk lipidomics
We recommend a combination of HILIC in combination with C30 reverse phase chromatography for the routine analysis of breast milk as a complex lipid mixture. HILIC distinguishes lipids into classes including subclasses with modified head groups according to their polarity and electrostatic interactions to improve ionization and reproducibility by combining the best properties of normal phase liquid chromatography (NPLC) and reverse phase liquid chromatography (RPLC). RPLC mobile phase has high ionization efficiency compared to NPLC. HILIC/RPLC separates lipids based on the interaction between the hydrophobic stationary phase and the hydrophobicity of the fatty acyl chains. It allows for differentiation of lipid molecules based on chain length and degree of unsaturation, allowing for intra-class separation of lipids (50–52). C18 reverse phase (RP) is a widely used non-polar stationary phase used in lipidomics (49). Recently, C30 RP has been gaining popularity in lipidomic analysis due to higher peak shape selectivity permitting superior resolution of geometric lipid isomers. C30 RP is efficient in identifying lipid isomers with different fatty acid compositions, degrees of unsaturation, or head group modifications. Furthermore, C30 chromatography was shown to be very good at distinguishing and resolving triglyceride isomers which only differed based on the alternation of the fatty acids at their sn1, sn2, and sn3 positions. Considering triglycerides account for the majority of the breast milk lipidome, C30 RP chromatography would be indispensable in determining novel triglyceride regioisomers during routine analysis. Formate buffer is used in the C30 RP chromatography, while acetate buffer is used in the HILIC solvent systems. This differentiates lipids separated using HILIC as acetate adducts and those by C30 RP as formate adducts when combined with mass spectrometry. HILIC can resolve breast milk lipids into different classes including subclasses with modified head groups. On the other hand, C30 RP chromatography allows the intra-class separation of breast milk lipid isomers based on chain length and degree of unsaturation. We recommend combining HILIC and C30 RP coupled with high resolution accurate mass tandem mass spectrometry as the best approach to identify neutral lipids, modified lipids, di/triglycerides, plasmalogens, and ether iso-forms of lipids as well as isobars and regioisomers (sn-positional isomers) during routine lipid analysis of breast milk (50). The breast milk lipidome is complex and comprised of different classes of lipids such as phospholipids, sphingolipids, plasmalogens, triglycerides, glycolipids, and modified lipids. High resolution accurate mass tandem mass spectrometry will allow the resolution of lipids based on their molecular weight down to 3–5 ppm. Combining the resolution provided by HILIC and C30 RP chromatography with the high resolving mass power of high-resolution accurate mass tandem mass spectrometry provide superior capabilities in resolving breast milk lipids according to classes, modification in head group or fatty acids, isobars, linkages between monomers, and isomeric composition during routine lipidomics.
HILIC complements with C30 RP coupled with high resolution accurate mass tandem mass spectrometry can be very effective in resolving breast milk lipidome during targeted and untargeted analysis and is recommended as one of the best approaches to consider during routine analysis of breast milk.
Bioinformatics for lipidomics and assessing functional lipids in breast milk lipidome
Bioinformatics is a new emerging discipline combining computer science, mathematics, physics, and biology to help with the management of data in modern biology (71). Large amounts of lipidomics data generated from breast milk lipidome can be derived from mass spectrometric analysis and the statistical analysis. Identifying methods to best evaluate the data can be challenging. Bioinformatics includes advanced databases, computational, and statistical techniques supported by mathematical theory to solve formal and practical problems in the analysis of the data. Bioinformatics in lipidomics is the combination of pre-processing and analyzing data sets using both univariate and multivariate approaches such as lipid modeling, correlation tests, dimensionality reduction, clustering or ordination techniques, classification, pathway, and network analysis (72).
Data pre-processing for functional lipid identification and quantification in breast milk
Data processing helps to convert the mass spectrometry data from biological samples (breast milk) into a final lipidomics (breast milk lipidomics) dataset. The final lipidomics data can be used for both quantitative and qualitative analysis as well as data interpretation (73–75).
Lipid databases and identification tools
LIPID MAPS is a database used to assist in identifying possible structures from mass spectrometry data. It has several tools such as structural classification and nomenclature, statistical tools, computer-aided spectral comparisons, and MS/MS fragment information (32). Lipid finder, Lipid QA, Lipid miner, and Lipid search are widely used tools in lipid identification. They use the fragment information generated by mass spectrometry for the identification and quantification of lipid species (32).
Metabolomics data processing approaches can also be applied in lipidomics mass spectrometry-based applications. MZmine is one of the open-source software widely used (73). This is a Java-based software package comprising multiple data processing steps, such as spectral filtering, peak detection, alignment, normalization, Exploratory Data Analysis (EDA), and visualization. MZmine is flexible to integrate new algorithms and applications, and thus encourages active community participation in new algorithm and application development within its framework. It can also be parallelized, which makes it suitable for large-scale profiling applications such as in large clinical studies (76).
Statistical data analysis
Statistical analysis is a very important aspect of the analytical workflow due to the high dimensionality typical of breast milk lipidomics dataset (77). This workflow ranges from univariate to multivariate approaches depending on the structure of the data and is aimed primarily at reducing the dimensionality of the data, as well as identifying features in the data that will facilitate meaningful interpretation of trends in the data of biological relevance or significance.
Univariate analysis
These are a collection of statistical approaches used to analyze the data based on the research hypothesis. Univariate analysis, the basic form of statistical analysis, is a recognized hypothesis testing approach in data analytics and it is often used to compare the mean of groups. For the comparison of two or more groups, one may resort to univariate methods such as the t-test, the Wilcoxon and Mann-Whitney test, or the analysis of variance (ANOVA) depending on the distribution of data (78). This kind of analysis is used to inspect one variable at a time in lipidomics and other fields (79). Univariate tests can be applied to all types of variables and outcomes and their results are easy to interpret. A disadvantage of univariate tests is that they disregard relations between features that may be important for biological interpretation, or which may improve statistical inference through their inclusion in the statistical test (80). Furthermore, this approach can be challenging to interpret in large dimensionality data sets.
Multivariate analysis
Multivariate analysis plays an important role in the extraction of valuable information from large dimensionality data such as the mass spectral data obtained following breast milk lipidomics. The advances in mass spectrometry increase the quantity and dimensionality of raw lipid data (81). For analysis, the high dimension data needs to be changed to more meaningful lower-dimensional data without losing important information. The selection of the most appropriate techniques to reduce the dimensionality of the data is one of the challenging factors in multivariate analysis. Principal Component Analysis (PCA), Canonical Correlation Analysis (CCA), Independent Component Analysis (ICA), and Partial Least-Squares Discriminant Analysis (PLS-DA), factor analysis are some dimensionality reduction techniques widely used (82). Most of these approaches are unsupervised method that aim to reduce the size of the original data projecting it in a new low-dimensional space by some assumptions. PCA is one of the most widely used method and it reduces the original data space by constructing new orthogonal dimensions in which the original data can be projected (83). These orthogonal variables are called principal components, and are linear combination of original variables that explain the maximum variance of the data (84). CCA is another multivariate approach exploiting the correlation between variables. Differently from PCA, it finds components maximizing the amount of correlation in the data (85). PLS-DA is a supervised method widely used in the analysis of high-dimensional lipidomic data. It increases the covariance rather than the correlation between components following some prior information already available from data (85). Multivariate techniques help to identify unique features in lipidomic data sets. These can facilitate useful interpretation of the data (such as biomarkers discovery or lipid metabolism associated with disease phenotypes) and may simplify the interpretation of complex biological models or data.
Lipid pathway and network analysis
Pathway and network analysis require a similar approach with some differences. Networks are large-scale systems which are generated from omics data whereas lipid pathways are small scale-systems that are constructed from literature (86). Lipid pathway analysis is currently a major analytical tool in lipidomics. This is based on biological databases with statistical testing, mathematical analyses, and computational algorithms (87). The interactions between compounds in lipid pathway analysis help to identify biomarkers of diseases, molecular mechanisms, and biological activity that may reflect known biological pathways (86). Organizing biological processes into useful, interactive pathways and networks is the biggest challenge in bioinformatics. SphinGOMAP and LIPID MAPS Biopathways Workbench (LMBP) are some of the lipid pathway software available (88, 89). The lipid network is another emerging tool in lipidomic data analysis. Biological (Lipid) networks are graphical and mathematical tools used to describe complex biological systems (90). Relationships/interactions and variables are noted by edges and nodes, respectively. Networks show the magnitude (node size), the directionality of changes (node fill or border colors), and lipid class (node shape). Significant changes in lipids are denoted by node colors. Nodes for species failing to reach statistical significance are denoted with colored borders, and non-measured or undetected species are displayed in a different color (91). Meta Map R is a free software that helps to merge molecular biochemical and structural information with mass spectral similarity based on correlations (92, 93). This allows for a clear display of the relationship between lipidome and clinical outcomes of infants.
All these methods from multivariate and univariate analysis, such as PLS-DA, PCA, and ANOVA, are commonly used in breast milk lipidomic studies (55, 94). However, other approaches in this fields commonly used in lipidomic research can also be applied in human breast milk lipidomic studies. This will assist in the identification of novel lipid biomarkers and the construction of biological models or metabolic pathways to better understand breast milk lipid metabolism and relationships to health outcomes from a systems biology perspective.
Lipids in breast milk
Human milk is a complex biofluid of macronutrients consisting of proteins, lipids, carbohydrates, vitamins, minerals, and trace elements. The composition of breast milk varies with several factors, such as stage of lactation, environmental factors, maternal age, body composition, and diet of a lactating mother. Breast milk has several long-term and short-term health benefits for breastfed infants (95, 96), such as adequate growth, cognitive development, immune development, regulation of inflammation and infection, and reduction of metabolic and cardiovascular disease in adult life (55, 97).
Among the macronutrients present in breast milk, lipids are a crucial source of energy for neonates up to 6 months of age (55, 98). Infants gain approximately 50–60% of energy from lipids. The fat content of breast milk varies significantly among colostrum, transitional milk, and mature milk, increasing with lactation stage (99, 100). Colostrum fat content is approximately 2.2 g/100 ml, increasing to 3 g/100 ml in transition milk, and 3.4 g/100 ml in mature milk (Figure 2A) (21). Lipids in breast milk exist structurally as fat globules (Figure 3) consisting mainly of triglycerides encircled by a double layer milk fat globule membrane (MFGM), which is composed of phospholipids, sphingolipids, protein, and cholesterol (98). The inner membrane consists of phosphatidylethanolamine as a single layer and the outer membrane is made of phosphatidylcholine, sphingomyelin, cholesterol, glycoproteins, bioactive peptides, cerebrosides, and gangliosides (55, 98).
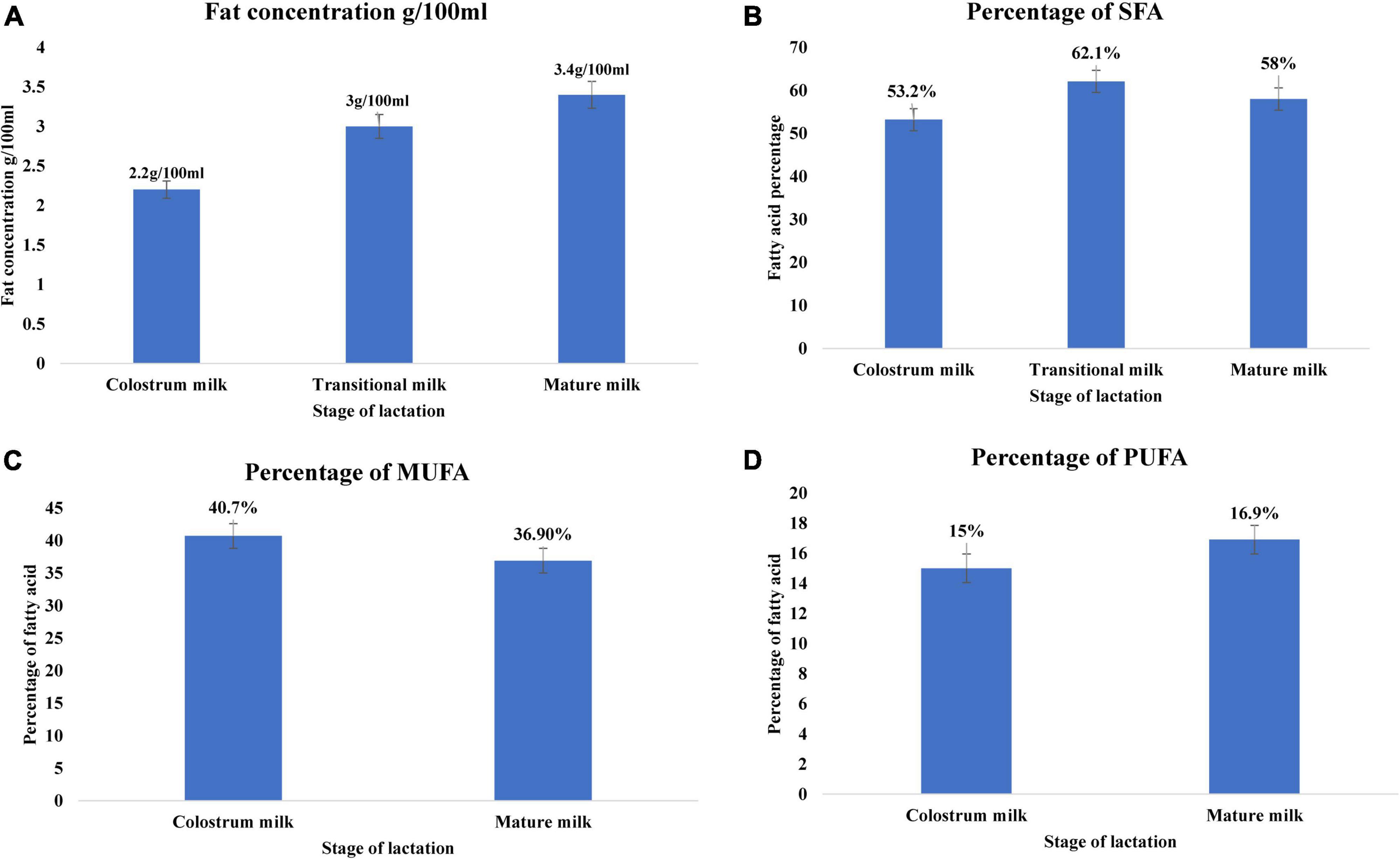
Figure 2. Lipid profile of breast milk across lactation stage (21). (A) Fat concentration (g/100 mL) of breast milk across the lactation stage. (B) Percentage of saturated fatty acid of breast milk across lactation stage. (C) Percentage of monounsaturated fatty acid of breast milk across lactation stage. (D) Percentage of polyunsaturated fatty acid of breast milk across lactation stage. Lactation stage—colostrum milk 0–5 days of postnatal life, transitional milk—6–15 days of postnatal life, and mature milk—16–60 days of postnatal life.
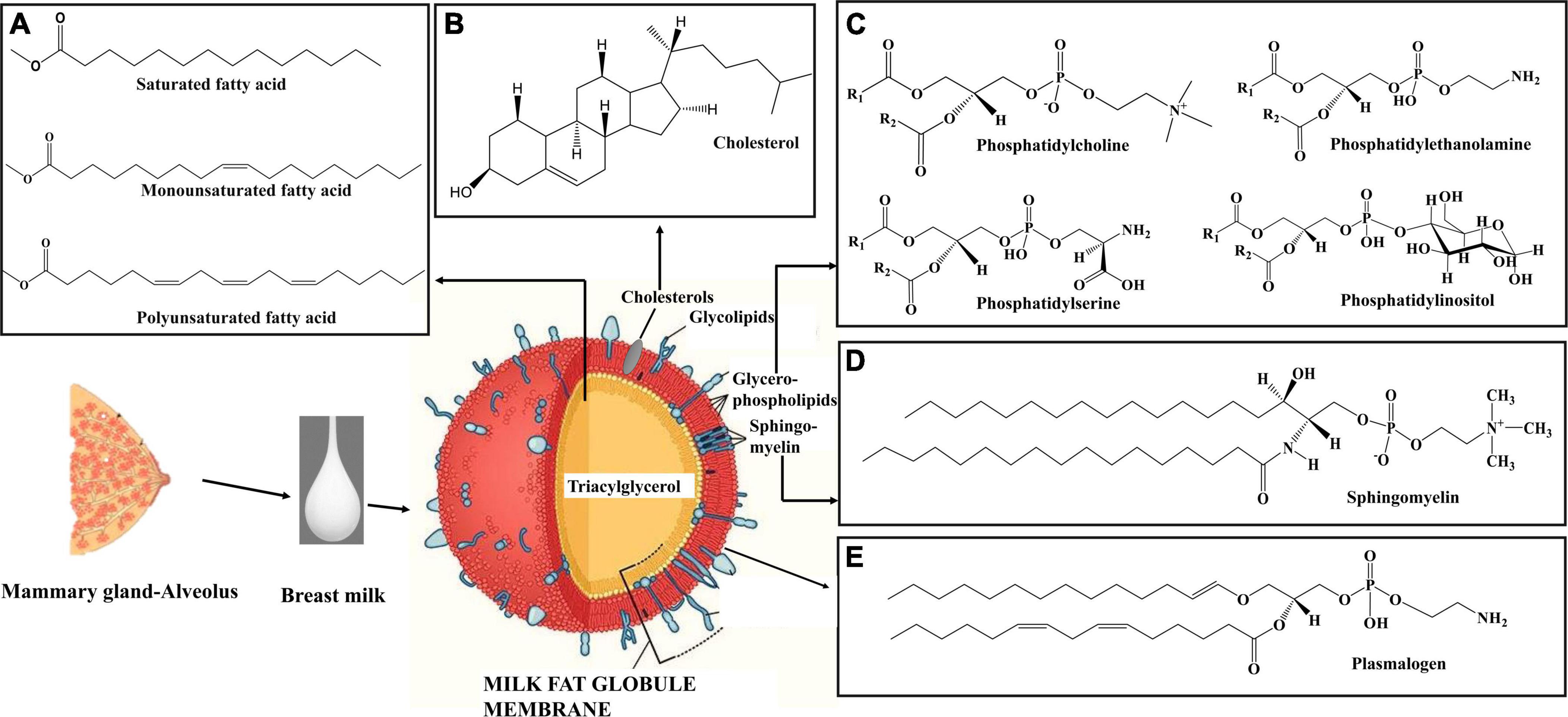
Figure 3. Schematic diagram of milk fat globule (108). (A) Structure of different types of fatty acids in breast milk triacylglycerol. (B) Structure of cholesterol in breast milk. (C) Structure of phospholipid classes in breast milk. (D) Structure of sphingomyelin in breast milk. (E) Structure of plasmalogen in breast milk. The milk fat globule membrane structure was modified from Mead Johnson Nutrition (108). Structures were created using Chem Draw 16.
Lipid composition of breast milk
Milk fat globules are secreted by mammary alveolar cells (Figure 3). Triglycerides, comprising 98–99% of milk lipids, and a small number of monoglycerides and diglycerides are in the center of the milk fat globule forming a lipid rich core (Figure 3A). The glycolipids in the core are encircled by a milk fat membrane comprised of esterified cholesterol (Figure 3B), phospholipids (Figure 3C), sphingolipids (Figure 3D), glycosylated polypeptides, filaments, mucin, and other components (53, 101). Triglycerides contain three fatty acids (99), which can be divided into saturated fatty acids (SFA), monounsaturated fatty acids (MUFA), and polyunsaturated fatty acids (PUFA). SFAs are abundant in human milk (102), followed by MUFAs, and PUFAs. SFAs in human milk ranges from 53.2 to 58% of total fatty acid, MUFAs from 23 to 55%, and PUFAs from 6 to 36% (98). SFAs in breast milk are caprylic acid (C8:0), capric acid (C10:0), lauric acid (C12:0), myristic acid (C14:0), palmitic acid (C16:0), stearic acid (C18:0), and arachidic acid (C20:0) (103). Total SFA content increases from colostrum to transitional milk and decreases in mature milk (Figure 2B) (98). Palmitic acid is the most abundant SFA in breast milk and decreases significantly from colostrum (23.2%) to mature milk (19.8%) (Figure 4A) (21). MUFAs in breast milk are myristoleic acid (C14:1 n-5), palmitoleic acid (C16:1 n-7), oleic acid (C18:1 n-9), vaccenic acid (C18:1 n-7) and erucic acid (C22:1 n-9). MUFAs decrease from 40.7% in colostrum to 36.9% in mature milk (Figure 2C) (55). Oleic acid is the most abundant MUFA in breast milk and ranges from 35.3% in colostrum to 32.9% in mature milk (Figure 4B) (21). Total PUFAs increase from 15% in colostrum to 16.9% in mature milk (Figure 2D) (55). PUFAs, such as linoleic acid (LA, C18:2 n-6) and α-linolenic acid (ALA, C18:3 n-3), are precursors of long chain PUFAs, such as arachidonic acid (AA, C20:4 n-6), eicosapentaenoic acid (EPA, C20:5 n-3) and docosahexaenoic acid (DHA, C22:6 n-3). LA and ALA are the abundant PUFAs in breast milk and increase with lactation stage (Figures 4C,D) (21).
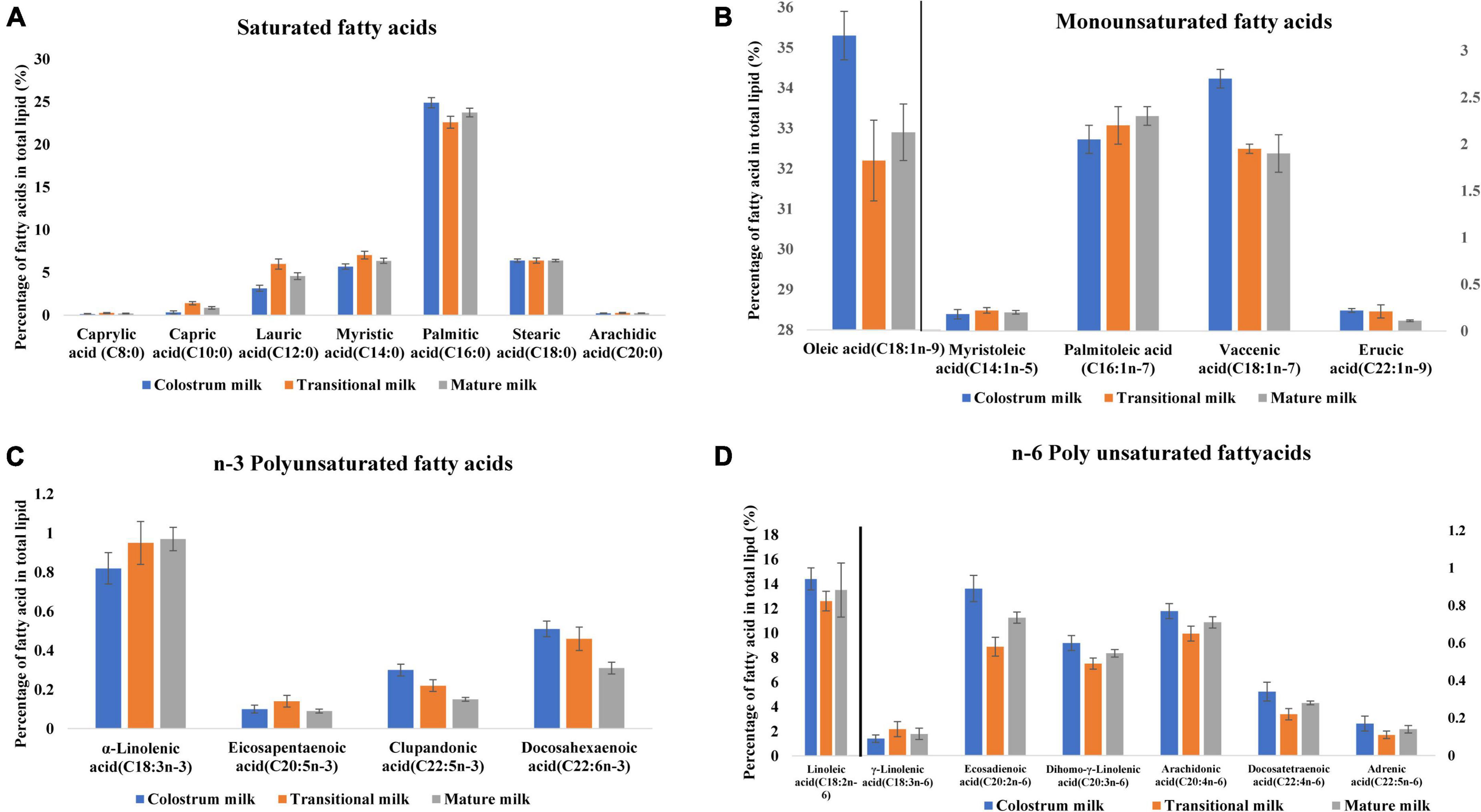
Figure 4. Fatty acid profile of breast milk across lactation stage (21). (A) Saturated fatty acids profile of breast milk across lactation stage. (B) Monounsaturated fatty acids profile of breast milk across lactation stage. (C) n-3 Polyunsaturated fatty acids profile of breast milk across lactation stage. (D) n-6 Polyunsaturated fatty acids profile of breast milk across lactation stage. Lactation stage—colostrum milk 0–5 days of postnatal life, transitional milk—6–15 days of postnatal life, and mature milk—16–60 days of postnatal life.
Complex lipids in breast milk
In breast milk, 0.2–2% of total lipids (approximately 10–40 mg/100 mL) are complex lipids (104, 105). Complex lipids are rich in MFGMs and extracellular vesicles in breast milk (21). Complex lipids impact the physiology of the brain, gut, and skin through signal transmission and cell recognition (104). Glycerophospholipids are phosphorous containing polar lipids with different head groups in the sn-3 position. Phosphatidylinositol (PtdIns), phosphatidylethanolamine (PtdEtn), phosphatidylserine (PtdSer), and phosphatidylcholine (PtdCho) are the classes of phospholipids in human milk which have inositol, ethanolamine, serine, and choline head groups, respectively (55). The sphingolipid metabolic pathways are complex and are metabolically interrelated with each other by an enzymatic breakdown. Through this complex reaction they form ceramide, sphingomyelin, and glycosphingolipids in human breast milk (106). Sphingolipids are formed by a sphingoid base which is connected with fatty acids via amide bonds and different head groups. The head groups are comprised of hydrogen (ceramide), phosphocholine (sphingomyelin), and oligosaccharides (glycosphingolipids) (107). Plasmalogens are a species of phospholipids distinguished by having a vinyl ether bond linking a fatty aldehyde to the stereospecific numbered carbon 1 (sn1) position and a fatty acyl esterified at the sn2 position of the glycerol moiety (10, 11, 109). Sphingomyelin (36%) is the most abundant complex lipid in breast milk. It is followed by glycerophospholipids, which are comprised of primarily of phosphatidylethanolamine (29%) and phosphatidylcholine (25%) (53, 55, 110).
Cholesterol
Free and esterified cholesterol is present in the milk fat globule. The total cholesterol content in human milk is 9–15 mg/ml. Cholesterol plays several functions in infants. It is the building block of myelin in the nervous system, and also acts as a substrate for the synthesis of bile acids, lipoproteins, vitamin D, and hormones (55).
Maternal factors affecting fatty acid composition in breast milk
The percentage of fatty acids in breast milk is affected by several factors, including maternal diet (53, 111, 112). Studies have found that maternal dietary intake affects SFAs such as capric acid, lauric acid, myristic acid, MUFAs such as oleic acid, and PUFAs such as EPA, DHA, LA, and ALA (112–118). Collectively, these studies show that the diet of lactating mothers is a very potent mediator of breast milk lipid composition during lactation. In addition, maternal age, body mass index, stress, and genetics have all been shown to influence breast milk fatty acid composition (119, 120). Maternal body mass index has been shown to have a positive correlation with concentrations of SFAs and a negative correlation with Omega-3 fatty acids in breast milk (120, 121).
Functional lipids in breast milk and their role in improving infant health
Functional food can be defined as foods or ingredients which assist in decreasing the risk of disease and/or improving health beyond nutritive purposes (122). Functional lipids are found in a wide variety of food. They are consumed with daily food intake, however, they have physiological benefits reported to reduce the risk of disease (123). Functional lipids in breast milk include MCTs, DAGs, CLAs or CLNs, omega 3, 6, and 9 fatty acids, phospholipids, plasmalogens, sphingolipids, and FAHFAs (11, 53, 124).
Physiologic role of breast milk functional lipids
Here we review the functional breast milk lipids that have been reported to impact physiologic processes in infants based on human observational studies, clinical studies, and randomized controlled trials. Butyrate (4:0) is a common SFA present in breast milk and acts as an anti-inflammatory and gene expression regulator in the intestine of infants (125). Other SFAs such as caproic (C6:0), caprylic (C8:0), capric (C10:0), and lauric (C12:0) acids inhibit the biological activities of microorganisms (27). C10:0, C12:0, and C14:0 are synthesized in the mammary gland and their presence at the sn1, sn2, and sn3 positions results in the formation of MCTs (126). MCTs are digested without bile salts and are passed along with short chain fatty acids directly to the liver for metabolism without lipoprotein (127). They are beneficial for infants who have abnormalities with fat digestion, absorption, transport, and metabolism (128, 129). Palmitic acid (C16:0) is the major SFA in human milk. It is typically located at the sn2 position of the glycerol moiety of triglycerides. Palmitic acid is converted into sn-2 monoacylglycerol (MAG) by pancreatic lipase at the sn-1 and sn-3 positions (130). Palmitic acid improves fat and calcium absorption, decreases intestinal injury and inflammation by upregulation of antioxidant enzymes, and also plays an important role in mucosal and gut microbiome homeostasis (21). The long-chain PUFAs are the most abundant PUFAs in breast milk. PUFAs play important roles in visual, immune, cognitive, and motor development in infants (131). CLA acts as a growth promoter in infants (132). Phospholipids containing PUFAs act as antioxidants in the gut mucosa (133). Phospholipids are important components of the central nervous system. They play a major role in cell signaling, cell proliferation, maturation of the brain, and reduction of inflammatory diseases in infants (3). They also play a crucial role in the maturation of infant lungs (3–6), which helps to reduce respiratory diseases in the newborn infant (131). PC and sphingomyelin in breast milk release large amounts of choline which plays an important role in neuronal development (134). Choline is a precursor for the neurotransmitter acetylcholine which aids in brain development (55). Choline also helps protect the gastrointestinal tract from infection (3). Plasmalogens maintain the cell membrane physical bilayer properties which is essential for membrane fusion, signal transmission, preventing oxidative stress, and mediate the inflammatory response in infants (11, 109). Sphingomyelin has a critical role in brain and immune development (106). Glycosphingolipids assist in the cognitive development of infants during the first 6 months (55). Table 1 contains a summary of functional breast milk lipids and their physiologic role in infants. However, the role of functional lipids remains largely unexplored in infants and additional studies are needed to further characterize these relationships. Emerging bioinformatics pathways will aid in identifying the relationships between lipid biomarker and clinical outcomes.
Conclusion
Human breast milk is the recommended source of nutrition for infants in the first 6 months of life and beyond due to the long-term positive impacts of its functional components, including lipids. Lipids not only provide energy, but they also play several additional roles such as aiding in the development of the immune and neurologic systems, as well as regulating metabolism. Functional lipids in breast milk may play a major role in infant outcomes. Characterizing the breast milk lipidome and linking its functional lipid components to infant outcomes will allow for targeted and precise lacto-engineering to optimize the health of children. Therefore, the development of biochemical and bioinformatic pathways to advance breast milk lipidomics may be essential in improving the health of future generations. More attention should be given to breast milk functional lipidome, maternal physiology and transgenerational influences mediating children’s health. Doing this from a systems biology perspective would provide indispensable information to further advance our understanding in this area in the scientific community.
Author contributions
FE, SS, SC, and RT: conceptualization. MG and SE: writing-original draft. MG, FE, SS, SC, RT, and SE: writing-review and editing. All authors read and approved the final draft of the manuscript.
Conflict of interest
The authors declare that the research was conducted in the absence of any commercial or financial relationships that could be construed as a potential conflict of interest.
Publisher’s note
All claims expressed in this article are solely those of the authors and do not necessarily represent those of their affiliated organizations, or those of the publisher, the editors and the reviewers. Any product that may be evaluated in this article, or claim that may be made by its manufacturer, is not guaranteed or endorsed by the publisher.
References
1. Michaelsen KF, Skafte L, Badsberg JH, Jørgensen M. Variation in macronutrients in human bank milk: Influencing factors and implications for human milk banking. J Pediatr Gastroenterol Nutr. (1990) 11:229–39. doi: 10.1097/00005176-199008000-00013
2. St-Onge M-P, Jones PJ. Physiological effects of medium-chain triglycerides: Potential agents in the prevention of obesity. J Nutr. (2002) 132:329–32. doi: 10.1093/jn/132.3.329
3. Contarini G, Povolo M. Phospholipids in milk fat: Composition, biological and technological significance, and analytical strategies. Int J Mol Sci. (2013) 14:2808–31. doi: 10.3390/ijms14022808
4. Hirabayashi Y, Furuya S. Roles of L-serine and sphingolipid synthesis in brain development and neuronal survival. Prog Lipid Res. (2008) 47:188–203. doi: 10.1016/j.plipres.2008.01.003
5. Küllenberg D, Taylor LA, Schneider M, Massing U. Health effects of dietary phospholipids. Lipids Health Dis. (2012) 11:1–16. doi: 10.1186/1476-511X-11-3
6. Mozzi R, Buratta S. Brain phosphatidylserine: Metabolism and functions. In: Lajtha A, Tettamanti G, Goracci G editors. Handbook of neurochemistry and molecular neurobiology. (New York, NY: Springer) (2010). doi: 10.1007/978-0-387-30378-9_3
7. Quinville BM, Deschenes NM, Ryckman AE, Walia JSA. Comprehensive review: Sphingolipid metabolism and implications of disruption in sphingolipid homeostasis. Int J Mol Sci. (2021) 22:5793. doi: 10.3390/ijms22115793
8. Makdissy N, Haddad K, Mouawad C, Popa I, Younsi M, Valet P, et al. Regulation of srebps by sphingomyelin in adipocytes via a caveolin and Ras-Erk-Mapk-Creb signaling pathway. PLoS One. (2015) 10:e0133181. doi: 10.1371/journal.pone.0133181
9. Ratajczak MZ, Lee H, Wysoczynski M, Wan W, Marlicz W, Laughlin MJ, et al. Novel insight into stem cell mobilization-plasma sphingosine-1-phosphate is a major chemoattractant that directs the egress of hematopoietic stem progenitor cells from the bone marrow and its level in peripheral blood increases during mobilization due to activation of complement cascade/membrane attack complex. Leukemia. (2010) 24:976–85. doi: 10.1038/leu.2010.53
10. Guan Z, Wang Y, Cairns NJ, Lantos PL, Dallner G, Sindelar PJ. Decrease and structural modifications of phosphatidylethanolamine plasmalogen in the brain with Alzheimer disease. J Neuropathol Exp Neurol. (1999) 58:740–7. doi: 10.1097/00005072-199907000-00008
11. Messias MCF, Mecatti GC, Priolli DG, de Oliveira Carvalho P. Plasmalogen lipids: Functional mechanism and their involvement in gastrointestinal cancer. Lipids Health Dis. (2018) 17:1–12. doi: 10.1186/s12944-018-0685-9
12. Yore MM, Syed I, Moraes-Vieira PM, Zhang T, Herman MA, Homan EA, et al. Discovery of a class of endogenous mammalian lipids with anti-diabetic and anti-inflammatory effects. Cell. (2014) 159:318–32. doi: 10.1016/j.cell.2014.09.035
13. Kishimoto K, Urade R, Ogawa T, Moriyama T. Nondestructive quantification of neutral lipids by thin-layer chromatography and laser-fluorescent scanning: Suitable methods for “lipidome” analysis. Biochem Biophys Res Commun. (2001) 281:657–62. doi: 10.1006/bbrc.2001.4404
14. Han X, Gross RW. Global analyses of cellular lipidomes directly from crude extracts of biological samples by ESI mass spectrometry: A bridge to lipidomics. J Lipid Res. (2003) 44:1071–9. doi: 10.1194/jlr.R300004-JLR200
15. Blanksby SJ, Mitchell TW. Advances in mass spectrometry for lipidomics. Annu Rev Anal Chem. (2010) 3:433–65. doi: 10.1146/annurev.anchem.111808.073705
16. Yang K, Han X. Lipidomics: Techniques, applications, and outcomes related to biomedical sciences. Trends Biochem Sci. (2016) 41:954–69. doi: 10.1016/j.tibs.2016.08.010
17. Miller EM, Aiello MO, Fujita M, Hinde K, Milligan L, Quinn E. Field and laboratory methods in human milk research. Am J Hum Biolol. (2013) 25:1–11. doi: 10.1002/ajhb.22334
18. George AD, Gay MC, Murray K, Muhlhausler BS, Wlodek ME, Geddes DT. Human milk sampling protocols affect estimation of infant lipid intake. J Nutr. (2020) 150:2924–30. doi: 10.1093/jn/nxaa246
19. Mitoulas LR, Kent JC, Cox DB, Owens RA, Sherriff JL, Hartmann PE. Variation in fat, lactose and protein in human milk over 24h and throughout the first year of lactation. Br J Nutr. (2002) 88:29–37. doi: 10.1079/BJN2002579
20. Lubetzky R, Littner Y, Mimouni FB, Dollberg S, Mandel D. Circadian variations in fat content of expressed breast milk from mothers of preterm infants. J Am Coll Nutr. (2006) 25:151–4. doi: 10.1080/07315724.2006.10719526
21. Ramiro-Cortijo D, Singh P, Liu Y, Medina-Morales E, Yakah W, Freedman SD, et al. Breast milk lipids and fatty acids in regulating neonatal intestinal development and protecting against intestinal injury. Nutrients. (2020) 12:534. doi: 10.3390/nu12020534
22. Kent JC, Mitoulas LR, Cregan MD, Ramsay DT, Doherty DA, Hartmann PE. Volume and frequency of breastfeedings and fat content of breast milk throughout the day. Pediatrics. (2006) 117:e387–95. doi: 10.1542/peds.2005-1417
23. Wu J, Domellöf M, Zivkovic AM, Larsson G, Öhman A, Nording ML. NMR-based metabolite profiling of human milk: A pilot study of methods for investigating compositional changes during lactation. Biochem Biophys Res Commun. (2016) 469:626–32. doi: 10.1016/j.bbrc.2015.11.114
24. Lev H, Ovental A, Mandel D, Mimouni F, Marom R, Lubetzky R. Major losses of fat, carbohydrates and energy content of preterm human milk frozen at- 80 C. J Perinatol. (2014) 34:396–8. doi: 10.1038/jp.2014.8
25. Reis A, Rudnitskaya A, Blackburn GJ, Fauzi NM, Pitt AR, Spickett CMA. Comparison of five lipid extraction solvent systems for lipidomic studies of human Ldl [S]. J Lipid Res. (2013) 54:1812–24. doi: 10.1194/jlr.M034330
26. Alexandre-Gouabau M-C, Moyon T, Cariou V, Antignac J-P, Qannari EM, Croyal M, et al. Breast milk lipidome is associated with early growth trajectory in preterm infants. Nutrients. (2018) 10:164. doi: 10.3390/nu10020164
27. Gardner AS, Rahman IA, Lai CT, Hepworth A, Trengove N, Hartmann PE, et al. Changes in fatty acid composition of human milk in response to cold-like symptoms in the lactating mother and infant. Nutrients. (2017) 9:1034. doi: 10.3390/nu9091034
28. Nasser R, Stephen AM, Goh YK, Clandinin MT. The effect of a controlled manipulation of maternal dietary fat intake on medium and long chain fatty acids in human breast milk in Saskatoon, Canada. Int Breastfeed J. (2010) 5:1–6. doi: 10.1186/1746-4358-5-3
29. Garwolińska D, Namieśnik J, Kot-Wasik A, Hewelt-Belka W. State of the art in sample preparation for human breast milk metabolomics–Merits and limitations. TrAC Trends Anal Chem. (2019) 114:1–10. doi: 10.1016/j.trac.2019.02.014
30. Villaseñor A, Garcia-Perez I, Garcia A, Posma JM, Fernández-López M, Nicholas AJ, et al. Breast milk metabolome characterization in a single-phase extraction, multiplatform analytical approach. Anal Chem. (2014) 86:8245–52. doi: 10.1021/ac501853d
31. Löfgren L, Forsberg G-B, Ståhlman M. The Bume method: A new rapid and simple chloroform-free method for total lipid extraction of animal tissue. Sci Rep. (2016) 6:1–11. doi: 10.1038/srep27688
32. Wang J, Wang C, Han X. Tutorial on lipidomics. Anal Chim Acta. (2019) 1061:28–41. doi: 10.1016/j.aca.2019.01.043
33. Wang M, Wang C, Han RH, Han X. Novel advances in shotgun lipidomics for biology and medicine. Prog Lipid Res. (2016) 61:83–108. doi: 10.1016/j.plipres.2015.12.002
34. Brügger B, Erben G, Sandhoff R, Wieland FT, Lehmann WD. Quantitative analysis of biological membrane lipids at the low picomole level by nano-electrospray ionization tandem mass spectrometry. Proc Natl Acad Sci USA. (1997) 94:2339–44. doi: 10.1073/pnas.94.6.2339
35. Kallio H, Nylund M, Boström P, Yang B. Triacylglycerol regioisomers in human milk resolved with an algorithmic novel electrospray ionization tandem mass spectrometry method. Food Chem. (2017) 233:351–60. doi: 10.1016/j.foodchem.2017.04.122
36. Sokol E, Ulven T, Færgeman NJ, Ejsing CS. Comprehensive and quantitative profiling of lipid species in human milk, cow milk and a phospholipid−enriched milk formula by Gc and Ms/Msall. Eur J Lipid Sci Technol. (2015) 117:751–9. doi: 10.1002/ejlt.201400575
37. Keereetaweep J, Chapman KD. Lipidomic analysis of endocannabinoid signaling: Targeted metabolite identification and quantification. Neural Plastic. (2016) 2016:2426398. doi: 10.1155/2016/2426398
38. Wang J, Wang C, Han X. Enhanced coverage of lipid analysis and imaging by matrix-assisted laser desorption/ionization mass spectrometry via a strategy with an optimized mixture of matrices. Anal Chim Acta. (2018) 1000:155–62. doi: 10.1016/j.aca.2017.09.046
39. Yang K, Cheng H, Gross RW, Han X. Automated lipid identification and quantification by multidimensional mass spectrometry-based shotgun lipidomics. Anal Chem. (2009) 81:4356–68. doi: 10.1021/ac900241u
40. Han X. Neurolipidomics: Challenges and developments. Front Biosci. (2007) 12:2601–15. doi: 10.2741/2258
41. Han X, Yang K, Gross RW. Multi−Dimensional Mass Spectrometry−Based Shotgun Lipidomics and Novel Strategies for Lipidomic Analyses. Mass Spectrom Rev. (2012) 31:134–78. doi: 10.1002/mas.20342
42. Cajka T, Fiehn O. Comprehensive analysis of lipids in biological systems by liquid chromatography-mass spectrometry. TrAC Trends Anal Chem. (2014) 61:192–206. doi: 10.1016/j.trac.2014.04.017
43. Garwolinìska D, Hewelt-Belka W, Namiesìnik J, Kot-Wasik A. Rapid characterization of the human breast milk lipidome using a solid-phase microextraction and liquid chromatography–mass spectrometry-based approach. J Proteome Res. (2017) 16:3200–8. doi: 10.1021/acs.jproteome.7b00195
44. Huynh K, Barlow CK, Jayawardana KS, Weir JM, Mellett NA, Cinel M, et al. High-throughput plasma lipidomics: Detailed mapping of the associations with cardiometabolic risk factors. Cell Chem Biol. (2019) 26:71.–84. doi: 10.1016/j.chembiol.2018.10.008
45. Lísa M, Cífková E, Holčapek M. Lipidomic profiling of biological tissues using off-line two-dimensional high-performance liquid chromatography–mass spectrometry. J Chromatogr A. (2011) 1218:5146–56. doi: 10.1016/j.chroma.2011.05.081
46. Narváez-Rivas M, Zhang Q. Comprehensive untargeted lipidomic analysis using core–shell c30 particle column and high field orbitrap mass spectrometer. J Chromatogr A. (2016) 1440:123–34. doi: 10.1016/j.chroma.2016.02.054
47. Narváez-Rivas M, Vu N, Chen G-Y, Zhang Q. Off-line mixed-mode liquid chromatography coupled with reversed phase high performance liquid chromatography-high resolution mass spectrometry to improve coverage in lipidomics analysis. Anal Chim Acta. (2017) 954:140–50. doi: 10.1016/j.aca.2016.12.003
48. Kiyonami R, Peake DA, Liu X, Huang Y. Large-scale lipid profiling of a human serum lipidome using a high-resolution, accurate-mass Lc/Ms/Ms approach. Waltham, MA: Thermo Fischer Scientific (2016).
49. Bird SS, Marur VR, Stavrovskaya IG, Kristal BS. Separation of Cis–trans phospholipid isomers using reversed phase lc with high resolution Ms detection. Anal Chem. (2012) 84:5509–17. doi: 10.1021/ac300953j
50. Pham TH, Zaeem M, Fillier TA, Nadeem M, Vidal NP, Manful C, et al. Targeting modified lipids during routine lipidomics analysis using hilic and c30 reverse phase liquid chromatography coupled to mass spectrometry. Sci Rep. (2019) 9:1–15. doi: 10.1038/s41598-019-41556-9
51. Cífková E, Holčapek M, Lísa M, Ovčačíkovaì MN, Lyčka AN, Lynen FDR, et al. Nontargeted quantitation of lipid classes using hydrophilic interaction liquid chromatography–electrospray ionization mass spectrometry with single internal standard and response factor approach. Anal Chem. (2012) 84:10064–70. doi: 10.1021/ac3024476
52. Baker PR, Armando AM, Campbell JL, Quehenberger O, Dennis EA. Three-dimensional enhanced lipidomics analysis combining UPLC, differential ion mobility spectrometry, and mass spectrometric separation strategies1 [S]. J Lipid Res. (2014) 55:2432–42. doi: 10.1194/jlr.D051581
53. Giuffrida F, Cruz−Hernandez C, Flück B, Tavazzi I, Thakkar SK, Destaillats F, et al. Quantification of phospholipids classes in human milk. Lipids. (2013) 48:1051–8. doi: 10.1007/s11745-013-3825-z
54. Zhang X, Liu L, Wang L, Pan Y, Hao X, Zhang G, et al. Comparative lipidomics analysis of human milk and infant formulas using Uhplc-Q-Tof-Ms. J Agric Food Chem. (2021) 69:1146–55. doi: 10.1021/acs.jafc.0c06940
55. Giuffrida F, Cruz-Hernandez C, Bertschy E, Fontannaz P, Masserey Elmelegy I, Tavazzi I, et al. temporal changes of human breast milk lipids of Chinese mothers. Nutrients. (2016) 8:715. doi: 10.3390/nu8110715
56. Liu G, Ding Z, Li X, Chen X, Wu Y, Xie L. Relationship between polyunsaturated fatty acid levels in maternal diets and human milk in the first month post−partum. J Hum Nutr Diet. (2016) 29:405–10. doi: 10.1111/jhn.12337
57. Chuang C-K, Yeung C-Y, Jim W-T, Lin S-P, Wang T-J, Huang S-F, et al. Comparison of free fatty acid content of human milk from Taiwanese mothers and infant formula. Taiwanese J Obstetr Gynecol. (2013) 52:527–33. doi: 10.1016/j.tjog.2013.10.013
58. Guo M. Human milk biochemistry and infant formula manufacturing technology. Sawston: Woodhead Publishing (2020). doi: 10.1016/B978-0-08-102898-8.00005-2
59. Chappell JE, Clandinin MT, Mcvey MA, Chance GW. Free fatty acid content of human milk: Physiologic significance and artifactual determinants. Lipids. (1985) 20:216–21. doi: 10.1007/BF02534191
60. Zemski Berry KA, Hankin JA, Barkley RM, Spraggins JM, Caprioli RM, Murphy RC. Maldi imaging of lipid biochemistry in tissues by mass spectrometry. Chem Rev. (2011) 111:6491–512. doi: 10.1021/cr200280p
61. Norris JL, Caprioli RM. Analysis of tissue specimens by matrix-assisted laser desorption/ionization imaging mass spectrometry in biological and clinical research. Chem Rev. (2013) 113:2309–42. doi: 10.1021/cr3004295
62. Baker TC, Han J, Borchers CH. Recent advancements in matrix-assisted laser desorption/ionization mass spectrometry imaging. Curr Opin Biotechnol. (2017) 43:62–9. doi: 10.1016/j.copbio.2016.09.003
63. Buchberger AR, DeLaney K, Johnson J, Li L. Mass spectrometry imaging: A review of emerging advancements and future insights. Anal Chem. (2018) 90:240. doi: 10.1021/acs.analchem.7b04733
64. Girolamo FD, Lante I, Muraca M, Putignani L. The role of mass spectrometry in the “omics” era. Curr Organ Chem. (2013) 17:2891–905. doi: 10.2174/1385272817888131118162725
65. Goto-Inoue N, Hayasaka T, Zaima N, Setou M. Imaging mass spectrometry for lipidomics. Biochim Biophys Acta. (2011) 1811:961–9. doi: 10.1016/j.bbalip.2011.03.004
66. Pirro V, Guffey S, Sepulveda M, Mahapatra C, Ferreira C, Jarmusch A, et al. Lipid dynamics in zebrafish embryonic development observed by Desi-Ms imaging and nanoelectrospray-Ms. Mol BioSyst. (2016) 12:2069–79. doi: 10.1039/C6MB00168H
67. Henderson F, Jones E, Denbeigh J, Christie L, Batey MA, Claude E, et al. Automated, high-throughput 3d desorption electrospray ionization (Desi) mass spectrometry imaging of a xenograft model of glioblastoma. Cancer Res. (2018) 78:33–33. doi: 10.1158/1538-7445.MOUSEMODELS17-A06
68. Pirro V, Alfaro CM, Jarmusch AK, Hattab EM, Cohen-Gadol AA, Cooks RG. Intraoperative assessment of tumor margins during glioma resection by desorption electrospray ionization-mass spectrometry. Proc Natl Acad Sci USA. (2017) 114:6700–5. doi: 10.1073/pnas.1706459114
69. Zhou Z, Zare RN. Personal information from latent fingerprints using desorption electrospray ionization mass spectrometry and machine learning. Anal Chem. (2017) 89:1369–72. doi: 10.1021/acs.analchem.6b04498
70. Robinson MA, Graham DJ, Castner DG. Tof-sims depth profiling of cells: Z-correction, 3d imaging, and sputter rate of individual Nih/3t3 fibroblasts. Anal Chem. (2012) 84:4880–5. doi: 10.1021/ac300480g
71. Bayat A. Science, medicine, and the future: Bioinformatics. BMJ. (2002) 324:1018. doi: 10.1136/bmj.324.7344.1018
72. Niemelä PS, Castillo S, Sysi-Aho M, Orešič M. Bioinformatics and computational methods for lipidomics. J Chromatogr B. (2009) 877:2855–62. doi: 10.1016/j.jchromb.2009.01.025
73. Katajamaa M, Orešič M. Processing methods for differential analysis of Lc/Ms profile data. BMC Bioinformatics. (2005) 6:179. doi: 10.1186/1471-2105-6-179
74. Katajamaa M, Miettinen J, Orešič M. Mzmine: Toolbox for processing and visualization of mass spectrometry based molecular profile data. Bioinformatics. (2006) 22:634–6. doi: 10.1093/bioinformatics/btk039
75. Schwudke D, Oegema J, Burton L, Entchev E, Hannich JT, Ejsing CS, et al. Lipid profiling by multiple precursor and neutral loss scanning driven by the data-dependent acquisition. Anal Chem. (2006) 78:585–95. doi: 10.1021/ac051605m
76. Orešič M, Hänninen VA, Vidal-Puig A. Lipidomics: A new window to biomedical Frontiers. Trends Biotechnol. (2008) 26:647–52. doi: 10.1016/j.tibtech.2008.09.001
77. Yetukuri L, Ekroos K, Vidal-Puig A, Orešič M. Informatics and computational strategies for the study of lipids. Mol BioSyst. (2008) 4:121–7. doi: 10.1039/B715468B
78. Want E, Masson P. Processing and analysis of Gc/Lc-Ms-based metabolomics data. Methods Mol Biol. (2011) 708:277–98. doi: 10.1007/978-1-61737-985-7_17
80. Chadeau−Hyam M, Campanella G, Jombart T, Bottolo L, Portengen L, Vineis P, et al. Deciphering the complex: Methodological overview of statistical models to derive omics−based biomarkers. Environ Mol Mutagen. (2013) 54:542–57. doi: 10.1002/em.21797
81. Checa A, Bedia C, Jaumot J. Lipidomic data analysis: Tutorial, practical guidelines and applications. Anal Chim Acta. (2015) 885:1–16. doi: 10.1016/j.aca.2015.02.068
82. Colgana RE, Gutierrezb DE, Sundramc J, Tenalid GB. Analysis of medical data using dimensionality reduction techniques. Proceedings of the Conference: AMALTHEA –2013. Melbourne, FL: Florida Institute of Technology (2013). doi: 10.13140/2.1.2270.1762
83. Joliffe IT, Morgan B. Principal component analysis and exploratory factor analysis. Stat Methods Med Res. (1992) 1:69–95. doi: 10.1177/096228029200100105
84. Abdi H, Williams LJ. Principal component analysis: Wiley interdisciplinary reviews. Comput Stat. (2010) 2:433–59. doi: 10.1002/wics.101
85. Meng C, Zeleznik OA, Thallinger GG, Kuster B, Gholami AM, Culhane AC. Dimension reduction techniques for the integrative analysis of multi-omics data. Brief Bioinform. (2016) 17:628–41. doi: 10.1093/bib/bbv108
86. Creixell P, Reimand J, Haider S, Wu G, Shibata T, Vazquez M, et al. Pathway and network analysis of cancer genomes. Nat Methods. (2015) 12:615. doi: 10.1038/nmeth.3440
87. Folger O, Jerby L, Frezza C, Gottlieb E, Ruppin E, Shlomi T. Predicting selective drug targets in cancer through metabolic networks. Mol Syst Biol. (2011) 7:501. doi: 10.1038/msb.2011.35
88. Fahy E, Cotter D, Sud M, Subramaniam S. Lipid classification, structures and tools. Biochim Biophys Acta Mol Cell Biol Lipids. (2011) 1811:637–47. doi: 10.1016/j.bbalip.2011.06.009
89. Merril AH Jr. Sphingomap–a web-based biosynthetic pathway map of sphingolipids and glycosphingolipids. Glycobiology. (2005) 15:15G–G. doi: 10.1093/glycob/cwi070
90. Guzzi PH, Roy S. Biological network analysis: Trends, approaches, graph theory, and algorithms. Amsterdam: Elsevier (2020).
91. Grapov D, Fiehn O, Campbell C, Chandler CJ, Burnett DJ, Souza EC, et al. Exercise plasma metabolomics and xenometabolomics in obese, sedentary, insulin-resistant women: Impact of a fitness and weight loss intervention. Am J Physiol Endocrinol Metab. (2019) 317:E999–1014. doi: 10.1152/ajpendo.00091.2019
92. Grapov D, Wanichthanarak K, Fiehn O. Metamapr: Pathway independent metabolomic network analysis incorporating unknowns. Bioinformatics. (2015) 31:2757–60. doi: 10.1093/bioinformatics/btv194
93. Wang R, Li B, Lam SM, Shui G. Integration of lipidomics and metabolomics for in-depth understanding of cellular mechanism and disease progression. J Genet Genom. (2020) 47:69–83. doi: 10.1016/j.jgg.2019.11.009
94. Prentice P, Koulman A, Matthews L, Acerini CL, Ong KK, Dunger DB. Lipidomic analyses, breast-and formula-feeding, and growth in infants. J Pediatr. (2015) 166:276.–281. doi: 10.1016/j.jpeds.2014.10.021
95. Victora CG, Adair L, Fall C, Hallal PC, Martorell R, Richter L, et al. Maternal and child undernutrition: Consequences for adult health and human capital. Lancet. (2008) 371:340–57. doi: 10.1016/S0140-6736(07)61692-4
96. Roseboom T, de Rooij S, Painter R. The Dutch famine and its long-term consequences for adult health. Early Hum Dev. (2006) 82:485–91. doi: 10.1016/j.earlhumdev.2006.07.001
97. Binns C, Lee M, Low WY. The long-term public health benefits of breastfeeding. Asia Pac J Public Health. (2016) 28:7–14. doi: 10.1177/1010539515624964
98. Koletzko B. Human milk lipids. Ann Nutr Metab. (2016) 69(Suppl. 2):27–40. doi: 10.1159/000452819
100. Uchiyama S-I, Sekiguchi K, Akaishi M, Anan A, Maeda T, Izumi T. Characterization and chronological changes of preterm human milk gangliosides. Nutrition. (2011) 27:998–1001. doi: 10.1016/j.nut.2010.10.016
101. Michalski M-C. Lipids and milk fat globule properties in human milk. In: Zibadi S, Watson RR, Preedy VR editors. Handbook of dietary and nutritional aspects of human breast milk. (Wageningen: Wageningen Academic Publishers) (2013). p. 213–44. doi: 10.3920/978-90-8686-764-6_16
102. German JB, Dillard CJ. Saturated fats: A perspective from lactation and milk composition. Lipids. (2010) 45:915–23. doi: 10.1007/s11745-010-3445-9
103. Floris L, Stahl B, Abrahamse-Berkeveld M, Teller I. Human milk fatty acid profile across lactational stages after term and preterm delivery: A pooled data analysis. Prostaglandins Leukot Essent Fatty Acids. (2020) 156:102023. doi: 10.1016/j.plefa.2019.102023
104. Tanaka K, Hosozawa M, Kudo N, Yoshikawa N, Hisata K, Shoji H, et al. The pilot study: Sphingomyelin-fortified milk has a positive association with the neurobehavioural development of very low birth weight infants during infancy, randomized control trial. Brain Dev. (2013) 35:45–52. doi: 10.1016/j.braindev.2012.03.004
105. Delplanque B, Gibson R, Koletzko B, Lapillonne A, Strandvik B. Lipid quality in infant nutrition: Current knowledge and future opportunities. J Pediatr Gastroenterol Nutr. (2015) 61:8. doi: 10.1097/MPG.0000000000000818
106. Dei Cas M, Paroni R, Signorelli P, Mirarchi A, Cerquiglini L, Troiani S, et al. Human breast milk as source of sphingolipids for newborns: Comparison with infant formulas and commercial cow’s milk. J Transl Med. (2020) 18:1–13. doi: 10.1186/s12967-020-02641-0
107. Ridgway N, McLeod R. Biochemistry of lipids, lipoproteins and membranes. Amsterdam: Elsevier (2008).
108. Wu SS, Richards J, Khoury DE, Walsh KR. New Insights Building A Foundation Into Pediatric Nutrition: Milk Fat Globule Membrane. USA; Mead Johnson Pediatric Nutrition Institute. (2016). Available online at: http://hcpnutramigenhusky-dev.eu-west-1.elasticbeanstalk.com/media/1410/mfgm-monograph.pdf (accessed November 18, 2021).
109. Gaposchkin DP, Zoeller RA. Plasmalogen status influences docosahexaenoic acid levels in a macrophage cell line: Insights using ether lipid-deficient variants. J Lipid Res. (1999) 40:495–503. doi: 10.1016/S0022-2275(20)32454-8
110. Visentainer JV, Santos OO, Maldaner L, Zappielo C, Neia V, Visentainer L, et al. Lipids and fatty acids in human milk: Benefits and analysis. Biochem Health Benefits Fat Acids. (2018) 1:1–22.
111. Aumeistere L, Ciproviča I, Zavadska D, Andersons J, Volkovs V, Ceļmalniece K. Impact of maternal diet on human milk composition among lactating women in latvia. Medicina. (2019) 55:173. doi: 10.3390/medicina55050173
112. Siziba LP, Lorenz L, Brenner H, Carr P, Stahl B, Mank M, et al. Changes in human milk fatty acid composition and maternal lifestyle-related factors over a decade: A comparison between the two ulm birth cohort studies. Br J Nutr. (2021) 126:228–35. doi: 10.1017/S0007114520004006
113. Innis SM. Dietary triacylglycerol structure and its role in infant nutrition. Adv Nutr. (2011) 2:275–83. doi: 10.3945/an.111.000448
114. Koletzko B, Boey CC, Campoy C, Carlson SE, Chang N, Guillermo-Tuazon MA, et al. Current information and asian perspectives on long-chain polyunsaturated fatty acids in pregnancy, lactation, and infancy: Systematic review and practice recommendations from an early nutrition academy workshop. Ann Nutr Metab. (2014) 65:49–80. doi: 10.1159/000365767
115. Koletzko B, Thiel I, Abiodun PO. The fatty acid composition of human milk in Europe and Africa. J Pediatr. (1992) 120:S62–70. doi: 10.1016/S0022-3476(05)81238-7
116. Peng Y, Zhou T, Wang Q, Liu P, Zhang T, Zetterström R, et al. Fatty acid composition of diet, cord blood and breast milk in Chinese mothers with different dietary habits. Prostaglandins Leukot Essent Fatty Acids. (2009) 81:325–30. doi: 10.1016/j.plefa.2009.07.004
117. Marangoni F, Agostoni C, Lammard AM, Giovannini M, Galli C, Riva E. Polyunsaturated fatty acid concentrations in human hindmilk are stable throughout 12-months of lactation and provide a sustained intake to the infant during exclusive breastfeeding: An Italian study. Br J Nutr. (2000) 84:103–9. doi: 10.1017/S0007114500001288
118. Francois CA, Connor SL, Wander RC, Connor WE. Acute effects of dietary fatty acids on the fatty acids of human milk. Am J Clin Nutr. (1998) 67:301–8. doi: 10.1093/ajcn/67.2.301
119. Sinanoglou VJ, Cavouras D, Boutsikou T, Briana DD, Lantzouraki DZ, Paliatsiou S, et al. Factors affecting human colostrum fatty acid profile: A case study. PLoS One. (2017) 12:e0175817. doi: 10.1371/journal.pone.0175817
120. Leghi GE, Netting MJ, Middleton PF, Wlodek ME, Geddes DT, Muhlhausler BS. The impact of maternal obesity on human milk macronutrient composition: A systematic review and meta-analysis. Nutrients. (2020) 12:934. doi: 10.3390/nu12040934
121. de la Garza Puentes A, Martí Alemany A, Chisaguano AM, Montes Goyanes R, Castellote AI, Torres-Espínola FJ, et al. The effect of maternal obesity on breast milk fatty acids and its association with infant growth and cognition–the preobe follow-up. Nutrients. (2019) 11:2154. doi: 10.3390/nu11092154
122. Roberfroid MB. Prebiotics and probiotics: Are they functional foods? Am J Clin Nutr. (2000) 71:1682S–7S. doi: 10.1093/ajcn/71.6.1682S
123. Moreau R. An overview of functional lipids. In: Proceedings of the 102nd AOCS Annual Meeting and Exposition. Cincinnati, OH: (2011).
124. Enstad S, Cheema S, Thomas R, Fichorova RN, Martin CR, O’Tierney-Ginn P, et al. The impact of maternal obesity and breast milk inflammation on developmental programming of infant growth. Eur J Clin Nutr. (2021) 75:180–8. doi: 10.1038/s41430-020-00720-5
125. Gao Y, Davis B, Zhu W, Zheng N, Meng D, Walker WA. Short-chain fatty acid butyrate, a breast milk metabolite, enhances immature intestinal barrier function genes in response to inflammation in vitro and in vivo. Am J Physiol Gastrointest Liver Physiol. (2021) 320:G521–30. doi: 10.1152/ajpgi.00279.2020
126. Hachey DL, Silber GH, Wong WW, Garza C. Human lactation Ii: Endogenous fatty acid synthesis by the mammary gland. Pediatr Res. (1989) 25:63–8. doi: 10.1203/00006450-198901000-00015
127. Stein J. Chemically defined structured lipids: Current status and future directions in gastrointestinal diseases. Int J Colorectal Dis. (1999) 14:79–85. doi: 10.1007/s003840050190
128. Smits B, Wilding P, Cooke W. Ready adsorption of medium chain triglyceride in the steatorrhoea syndrome. Gut. (1968) 9:28. doi: 10.1136/gut.9.1.28
129. Marten B, Pfeuffer M, Schrezenmeir J. Medium-chain triglycerides. Int Dairy J. (2006) 16:1374–82. doi: 10.1016/j.idairyj.2006.06.015
130. de Queiroz Leite ME, Lasekan J, Baggs G, Ribeiro T, Menezes-Filho J, Pontes M, et al. Calcium and fat metabolic balance, and gastrointestinal tolerance in term infants fed milk-based formulas with and without palm olein and palm kernel oils: A randomized blinded crossover study. BMC Pediatr. (2013) 13:215. doi: 10.1186/1471-2431-13-215
131. Farquharson J, Jamieson E, Logan R, Cockburn F, Patrick WA. Infant cerebral cortex phospholipid fatty-acid composition and diet. Lancet. (1992) 340:810–3. doi: 10.1016/0140-6736(92)92684-8
132. McGuire MK, Park Y, Behre RA, Harrison LY, Shultz TD, McGuire MA. Conjugated linoleic acid concentrations of human milk and infant formula. Nutr Res. (1997) 17:1277–83. doi: 10.1016/S0271-5317(97)00111-5
133. Grażyna C, Hanna C, Adam A, Magdalena BM. Natural antioxidants in milk and dairy products. Int J Dairy Technol. (2017) 70:165–78. doi: 10.1111/1471-0307.12359
Keywords: breast milk, bioinformatics, lipids, functional lipids, lipidomics
Citation: Ganeshalingam M, Enstad S, Sen S, Cheema S, Esposito F and Thomas R (2022) Role of lipidomics in assessing the functional lipid composition in breast milk. Front. Nutr. 9:899401. doi: 10.3389/fnut.2022.899401
Received: 18 March 2022; Accepted: 02 August 2022;
Published: 02 September 2022.
Edited by:
Adenilda Cristina Honorio-França, Federal University of Mato Grosso, BrazilReviewed by:
Sonia Baig, National University of Singapore, SingaporeTuulia Hyötyläinen, Örebro University, Sweden
Copyright © 2022 Ganeshalingam, Enstad, Sen, Cheema, Esposito and Thomas. This is an open-access article distributed under the terms of the Creative Commons Attribution License (CC BY). The use, distribution or reproduction in other forums is permitted, provided the original author(s) and the copyright owner(s) are credited and that the original publication in this journal is cited, in accordance with accepted academic practice. No use, distribution or reproduction is permitted which does not comply with these terms.
*Correspondence: Moganatharsa Ganeshalingam, bWdhbmVzaGFsaW5nQG11bi5jYQ==; Raymond Thomas, cnRob21hc0BncmVuZmVsbC5tdW4uY2E=