- 1NYU Grossman School of Medicine, New York, NY, United States
- 2NYC Health + Hospitals, New York, NY, United States
- 3Veterans Affairs, Orlando, FL, United States
- 4Department of Behavioral and Community Health, School of Public Health, University of Maryland, College Park, MD, United States
- 5Program in Oncology, University of Maryland Marlene and Stewart Greenebaum Comprehensive Cancer Center, Baltimore, MD, United States
Introduction: Interventions emphasizing healthful lifestyle behaviors are proliferating in traditional health care settings, yet there is a paucity of published clinical outcomes, outside of pay-out-of-pocket or employee health programs.
Methods: We assessed weight, hemoglobin A1c (HbA1c), blood pressure, and cholesterol for 173 patients of the Plant-Based Lifestyle Medicine Program piloted in a New York City safety-net hospital. We used Wilcoxon signed-rank tests to assess changes in means, from baseline to six-months, for the full sample and within baseline diagnoses (i.e., overweight or obesity, type 2 diabetes, prediabetes, hypertension, hyperlipidemia). We calculated the percentage of patients with clinically meaningful changes in outcomes for the full sample and within diagnoses.
Findings: The full sample had statistically significant improvements in weight, HbA1c, and diastolic blood pressure. Patients with prediabetes or overweight or obesity experienced significant improvements in weight and those with type 2 diabetes had significant improvements in weight and HbA1c. Patients with hypertension had significant reductions in diastolic blood pressure and weight. Data did not show differences in non-high-density lipoprotein cholesterol (non-HDL-C), but differences in low-density lipoprotein cholesterol (LDL-C) were approaching significance for the full sample and those with hyperlipidemia. The majority of patients achieved clinically meaningful improvements on all outcomes besides systolic blood pressure.
Conclusion: Our study demonstrates that a lifestyle medicine intervention within a traditional, safety-net clinical setting improved biomarkers of cardiometabolic disease. Our findings are limited by small sample sizes. Additional large-scale, rigorous studies are needed to further establish the effectiveness of lifestyle medicine interventions in similar settings.
Introduction
Chronic diseases such as heart disease, cancer, and type 2 diabetes are among the leading causes of death and disability in the United States (1). Sixty percent of adults live with at least one chronic disease and 40% live with two or more (2). Given increased prescription costs, the need for continuous care, and more frequent health care visits, the management of chronic diseases accounts for 90% of annual health care expenditures--making chronic diseases the leading drivers of healthcare costs in the U.S. (3–5) Health behaviors such as tobacco use, poor nutrition characterized by diets high in sodium and saturated fats and low in fruits and vegetables, lack of physical activity, and insufficient sleep are modifiable risk factors that contribute to the development of chronic disease (6).
There is strong evidence supporting the use of lifestyle interventions to prevent and treat chronic disease. For example, healthful, plant-predominant diets have been associated with significant reductions in the risk of ischemic heart disease, cancer, type 2 diabetes, obesity, and hypertension (7–12). This dietary pattern has also been shown to improve existing conditions such as coronary artery disease, type 2 diabetes, and obesity (12–17). In addition, other lifestyle behaviors including regular physical activity, restorative sleep, and stress management are associated with positive health outcomes (18–20). Leading medical organizations including the American Diabetes Association (21), American Association of Clinical Endocrinology (22), American College of Cardiology (23), American Heart Association (24), American Society for Preventive Cardiology (25), National Lipid Association (26), and the American Cancer Society (27) all recommend lifestyle change, including plant-predominant eating patterns, as first-line therapy.
Lifestyle medicine is a medical specialty that uses therapeutic lifestyle interventions as a primary modality to treat chronic lifestyle-related conditions (28). Trained clinicians deliver evidence-based care focused on encouraging a healthful, plant-predominant eating pattern, regular physical activity, restorative sleep, stress management, avoidance of risky substances, and positive healthy relationships. Lifestyle medicine has proliferated rapidly in recent years. More than 80 major health systems across the United States currently participate in the Health Systems Council for the American College of Lifestyle Medicine, indicating their commitment to integrating lifestyle medicine into their services (29). Lifestyle medicine interventions have been shown to improve cardiometabolic risk factors by lowering weight, blood pressure, fasting lipids, and blood sugar, while reducing angina and cardiac events (13, 30). However, there is a paucity of published data on the clinical impact of lifestyle medicine programs in traditional health care settings, outside of employee health, pay-out-of-pocket, or residential programs. Furthermore, even fewer studies have examined the impact of a lifestyle medicine program within a safety-net clinical setting that serves high-needs populations experiencing disproportionate rates of chronic diseases.
The Plant-Based Lifestyle Medicine (PBLM) Program is a pilot clinical program established in an urban public healthcare system. The program’s goal is to help patients adopt a healthful plant-based eating pattern and other positive behaviors. The evaluation of the pilot program focused primarily on feasibility of implementation and demand for the program (31). We found demand for the program was high, far exceeding capacity, and patients reported joining the program in order to gain control over their lives, reduce their medication burden, and lose weight. The program’s team, overall approach, and resources all were popular among patients despite reported barriers to accessing the program as well as administrative and insurance challenges. In this paper, we build on the implementation findings of the PBLM Program by assessing preliminary data on clinical outcomes including blood sugar, weight, blood pressure, and cholesterol among program patients. Our study is novel because, to our knowledge, the pilot PBLM Program is the first lifestyle medicine program operating within a traditional safety-net healthcare system to publish clinical outcomes data.
Methods
The primary goal of the pilot PBLM Program evaluation was to assess the feasibility of implementing the program and the demand for its services. A secondary goal was to assess preliminary data related to clinical outcomes. The study uses a pre/post single sample design. Approval for this study was obtained from both the New York University (NYU) Grossman School of Medicine Institutional Review Board as well as the Office of Research and Administration for Implementation at NYC Health + Hospitals/Bellevue.
Intervention
A detailed description of the 1-year pilot of the PBLM Program has been published previously (31). Briefly, the PBLM Program at NYC Health + Hospitals/Bellevue, a large public hospital in New York City, was designed to provide structured, interdisciplinary support for lifestyle changes to improve patients’ cardiometabolic health. Adults with excess weight (i.e., BMI ≥ 25), type 2 diabetes, prediabetes, hypertension, atherosclerotic heart disease, and/or hyperlipidemia were eligible to participate. Individuals learned of the program through earned media (e.g., TV news, newspapers), social media, announcements from the office of the Brooklyn Borough President, and limited health care provider referrals from within Bellevue. Thus, most patients self-selected into the program and joined by adding their name to a waitlist. Enrollment in the program was on a first-come, first-serve basis. The program encouraged patients to transition to a healthful plant-based diet, improve sleep health, increase physical activity, improve stress management, foster positive social connections, and avoid substance use. Patients met individually with clinicians who have expertise in plant-based nutrition and lifestyle medicine, including a physician, a registered dietitian, and a health coach, to set goals and monitor progress. Group classes supplemented one-on-one visits, emphasizing education, skills-building, action planning, and peer-to-peer support. In addition, an exercise trainer offered classes focused on aerobic and strength training. Resources available to all patients included a plant-based diet starter guide, cookbook(s), HealthBucks (coupons that can be used to purchase fresh fruits and vegetables at all New York City farmer’s markets), a Healthy Savings Card for grocery store discounts on fresh produce, and access to a private Facebook group for social support, advice, and recipe sharing. The frequency and duration of program engagement for each individual was determined jointly by the PBLM Program provider team and each of the patients.
Data sources
Clinical outcomes for all patients of the PBLM Program were analyzed using de-identified electronic health record (EHR) data. In total, 173 individuals had at least one visit with the program between January 16, 2019 and February 15, 2020 and had EHR data. Because data were de-identified, consent was not required. Data were pulled at one time point and contained all recorded values during the study period. Additionally, program staff maintained a separate administrative database to track the following data for all patients: (1) limited demographic data (i.e., age, gender); (2) baseline clinical diagnoses determined by the treating physician (i.e., type 2 diabetes, prediabetes, overweight or obesity, hypertension, heart disease, hyperlipidemia); and (3) medications and their dosages at baseline, three-months, and six-months. The two datasets were merged for analyses using a unique identifier.
Measures
There were six clinical outcomes of interest in this study: (1) weight (kg); (2) hemoglobin A1c (HbA1c) (%); (3) systolic blood pressure (mm Hg); (4) diastolic blood pressure (mm Hg); (5) non-high-density lipoprotein cholesterol (non-HDL-C) (mg/dl); and (6) low-density lipoprotein cholesterol (LDL-C) (mg/dl).
In order to assess the contribution of behavior change to clinical outcomes, independent of medication changes, we created a stable or reduced medication regimen variable for those with type 2 diabetes, hypertension, and hyperlipidemia diagnoses at baseline. We first calculated the total number of medications prescribed for each participant within diagnoses (e.g., total number of diabetes medications prescribed for someone with type 2 diabetes) at baseline and six-months. Then we coded changes to medications by calculating the difference in the total number of medications from baseline to six-months (+1 for new medication added, −1 for medication removed, 0 for no change in medications). For those with no change in the number of disease-specific medications, we assessed changes in dosage (+1 for increase, −1 for decrease, 0 for no change). We then combined these two factors for a medication and dosage variable where a positive number indicates a net increase (increased medication regimen), a negative number indicates a net decrease (reduced medication regimen), and 0 indicates no net change (stable medication regimen).
In addition, we created variables to represent clinically meaningful change in each of the six clinical outcomes. Clinically meaningful improvement was based on published literature and was defined as follows: ≥3% decrease in weight (32, 33), ≥0.5 percentage point reduction in HbA1c for patients with a baseline diagnosis of type 2 diabetes (34–36), no change or any decrease in HbA1c for patients with a baseline diagnosis of prediabetes (i.e., no progression to type 2 diabetes) (37), ≥3 mm Hg reduction in systolic or diastolic blood pressure (38, 39), and any decrease in non-HDL-C or LDL-C (40, 41). First, we calculated the change from baseline to six-months (positive number indicates an increase, negative number indicates a decrease, zero indicates no change). Then we created a binary variable (yes/no) to indicate whether that change was clinically meaningful for each of the clinical outcomes.
Analyses
We assessed comparability in demographic characteristics and baseline diagnoses by gender using Chi-squared tests. We conducted analyses to assess changes in patients’ clinical outcomes between baseline and six-months for the full sample and then within diagnosis (i.e., overweight or obesity, type 2 diabetes, prediabetes, hypertension, hyperlipidemia) using only patients that had data at both time points; heart disease was excluded from subgroup analysis due to the small sample size. When analyzing outcomes within the baseline diagnosis subgroups, we assessed change over time only for the most relevant outcomes (e.g., non-HDL-C and LDL-C for patients with hyperlipidemia) in addition to weight because a substantial majority (i.e., ≥ 80%) of patients had a BMI ≥ 25 within each condition. We also assessed change in weight and relevant clinical outcomes for three disease-specific subgroups of patients (i.e., type 2 diabetes, hypertension, hyperlipidemia) with stable or reduced disease-specific medication regimens over the study period. Our goal in reviewing outcomes by stable or reduced medication status was to isolate, to the best possible extent, the effects of lifestyle change alone. Change in medication status was not reviewed for the prediabetes, overweight or obesity, or heart disease subgroups, given that disease-specific medications were not used for prediabetes or overweight or obesity, and the sample size of the heart disease subgroup was too small for analysis. For most patients, baseline values were established at the first appointment. In selected cases where an individual did not have clinical data from their first visit, we used lab results that were no more than 90 days before their first appointment or no more than 30 days after the first appointment. For six-month values, we used the first value 182 days or more after the baseline visit. In those instances where there were no available values at six-months or more, we used their last value as long as it was 45 days or more after the baseline value. The only exception was for HbA1c, where we increased the inclusion criteria to at least 60 days after the first appointment.
Lastly, we calculated the percentage of patients with clinically meaningful improvement in clinical outcomes between baseline and six-months for the full sample and then within diagnoses for clinically relevant outcomes. These data are shown for descriptive purposes only. We did not assess for clinically meaningful changes in HbA1c for the full sample because two-thirds of patients did not have type 2 diabetes; thus, their HbA1c levels were more likely to have been in “control” at baseline and any clinically meaningful improvement detected would not be representative of the full sample.
The sample size in analyses fluctuates due to missing outcome data. We used Wilcoxon signed-rank tests to assess changes in means of the clinical outcomes. This test is the non-parametric test used when data are not normally distributed. When sample sizes were less than 20, we did not perform statistical tests in order to avoid comparing estimates with limited statistical stability. In those cases, we present data descriptively. All analyses were conducted using SPSS version 26 (42). Results were considered statistically significant if they had a value of p less than 0.05.
Results
Demographic characteristics, baseline diagnoses, and days between measures
Table 1 shows the demographic characteristics and baseline diagnoses of all PBLM Program patients, stratified by gender. The majority of patients were female (69.4%) and were, on average, about 55 years old. The majority of patients had a baseline diagnosis of overweight or obesity (87.3%), roughly half of the sample was hypertensive or had a diagnosis of hyperlipidemia (53.2 and 49.7%, respectively), about one-third had a baseline diagnosis of type 2 diabetes (33.5%), a little over one-fifth had prediabetes (21.4%), and a small percentage of the sample had a baseline diagnosis of heart disease (12.1%). There was a statistically significant difference in heart disease among males and females. Specifically, a larger percentage of male patients had a baseline diagnosis of heart disease as compared to female patients (22.6% vs. 7.5%). The average number of days between patients’ baseline and six-month measures varied: HbA1c 172 days (SD = 70), weight, systolic blood pressure, diastolic blood pressure 169 days (SD = 66); non-HDL-C 167 days (SD = 81); LDL-C 167 days (SD = 80) (not shown).
Average change in clinical outcomes over time
Table 2 displays findings for all six clinical outcomes for the full sample. We then stratified by baseline condition and show weight in addition to the measure most relevant to that condition. Among the full sample of patients, including those without overweight/obesity, type 2 diabetes, prediabetes, hypertension, or hyperlipidemia, there were statistically significant reductions in patients’ weight (92.5 kg vs. 88.8 kg), HbA1c levels (6.7% vs. 6.3%), and diastolic blood pressure (76.6 mm Hg vs. 73.9 mm Hg) over time. Change in LDL-C was approaching significance (101.7 mg/dl vs. 93.0 mg/dl, p < 0.10). For the subgroup of patients that had a baseline diagnosis of overweight or obesity (n = 151), we found significant improvements in weight (95.6 kg vs. 91.7 kg). For the subgroup of patients with type 2 diabetes (n = 58), there were improvements in HbA1c (7.9% vs. 7.2%) and weight (98.2 kg vs. 93.3 kg). For the subgroup of patients with prediabetes (n = 37), only weight was evaluated for statistical significance, as there were too few patients with HbA1c measurements at both timepoints to perform statistical tests. Weight, on average, decreased from 88.1 kg to 85.0 kg (p < 0.05) for those with prediabetes. Statistically significant improvements were found in diastolic blood pressure among patients with a diagnosis of hypertension (n = 92; 79.1 mm Hg vs. 75.0 mm Hg) and weight (92.0 kg vs. 88.5 kg) between baseline and six-months. No statistically significant changes were detected in systolic blood pressure. Lastly, among individuals with hyperlipidemia (n = 86), the change in LDL-C was approaching significance (112.1 mg/dL vs. 98.0 mg/dl, p < 0.10) and weight was significant (93.2 kg vs. 90.0 kg).
Average change in clinical outcomes for those with a stable medication regimen
We assessed changes in clinical outcomes for patients with a baseline diagnosis of type 2 diabetes, hypertension, or hyperlipidemia who also had a stable or reduced disease-specific medication regimen (Table 3). Among those with type 2 diabetes, there were statistically significant improvements in HbA1c (7.8% vs. 7.1%) and weight (97.2 kg vs. 91.9 kg). For those with hypertension, we detected a significant reduction in diastolic blood pressure from 79.8 mm Hg to 75.4 mm Hg over time in addition to weight (92.0 kg vs. 88.6 kg). Changes in both non-HDL-C and LDL-C approached statistical significance (140.4 to 124.7 and 112.8 to 98.6 mg/dl, respectively) between baseline and the six-month visit for those with hyperlipidemia while there was a statistically significant improvement in weight (92.9 kg vs. 89.7 kg).
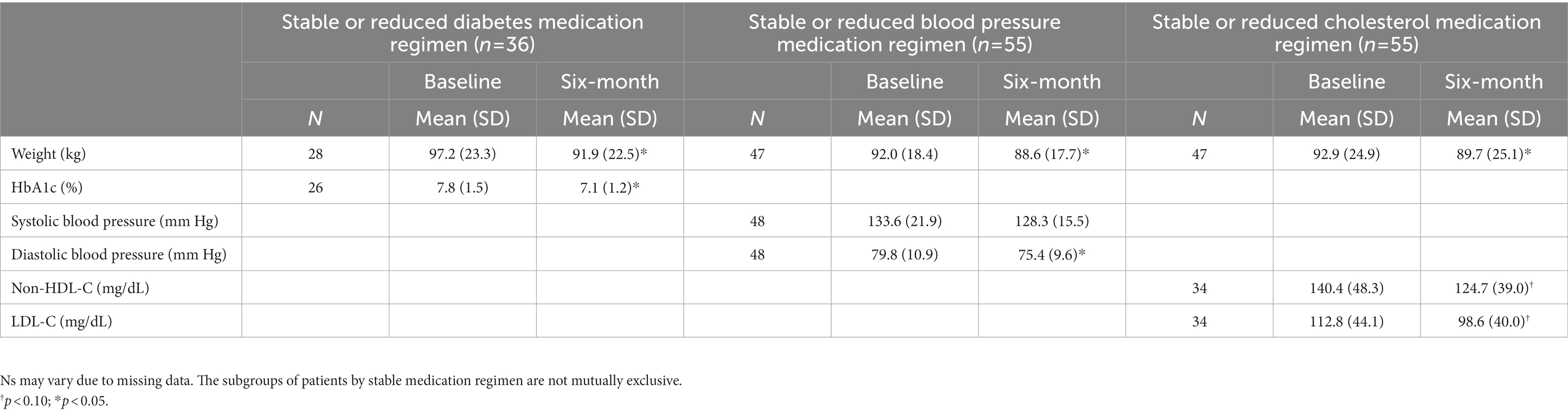
Table 3. Clinical outcomes by stable or reduced medication regimen for patients with a baseline diagnosis of type 2 diabetes, hypertension, or hyperlipidemia.
Clinically meaningful change in outcomes over time
Table 4 shows the proportion of patients who had clinically meaningful change over time for the full sample and then by baseline diagnosis. In the full sample, more than half of patients had clinically meaningful changes in four of the five outcomes assessed (HbA1c was not assessed for the full sample). Specifically, a substantial proportion of patients had improvements in their weight (50.4%), diastolic blood pressure (50.4%), non-HDL-C (54.3%), and LDL-C (55.7%); this includes patients who did not have a baseline diagnosis of hypertension or hyperlipidemia. One half of those with overweight or obesity were found to have a clinically meaningful reduction in weight. For those with type 2 diabetes, we determined that at least half of patients had clinically meaningful improvements in HbA1c (50.0%) and weight (51.4%). Among those with prediabetes at baseline, a sizable proportion had clinically meaningful outcomes in both HbA1c (76.5%) and weight (50.0%). Forty percent of those with hypertension at baseline had a clinically meaningful reduction in systolic blood pressure, 56.7% had improvement in diastolic blood pressure, and 56.1% had a clinically meaningful reduction in weight. Additionally, almost two thirds of the hyperlipidemia subgroup (60.5%) had clinically meaningful reductions in both non-HDL-C and LDL-C over time, while 51.9% had a meaningful improvement in weight.
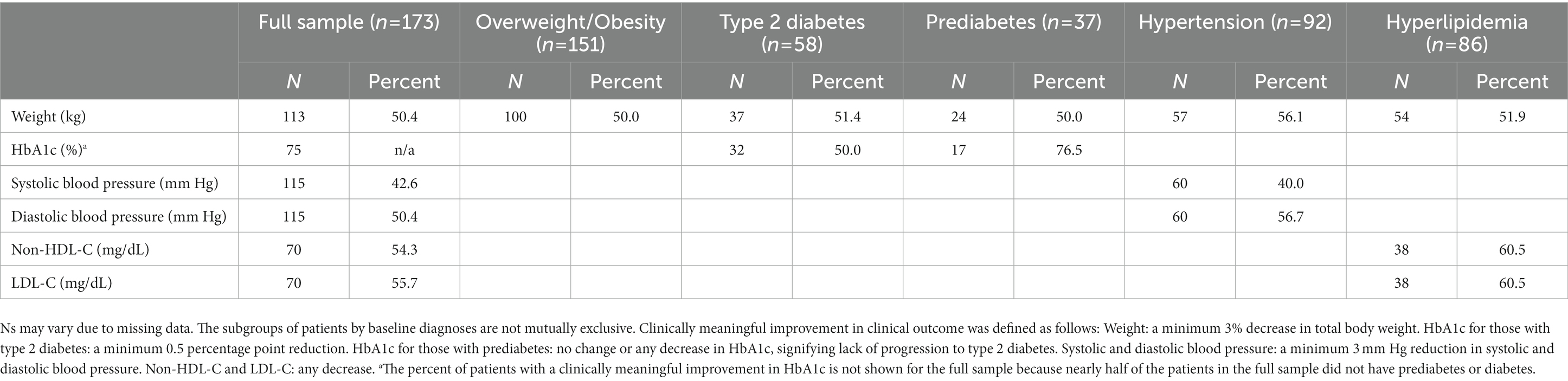
Table 4. Percent of patients with a clinically meaningful improvement in clinical outcomes for full sample and by baseline diagnosis.
Discussion
We have previously published findings that the pilot PBLM Program is feasible with high levels of patient satisfaction and interest (31). In this study, we now demonstrate a significant reduction in key biomarkers of cardiometabolic disease, including HbA1c, body weight, lipids, and diastolic blood pressure. We report findings that are both statistically significant and represent clinically meaningful improvements related to cardiometabolic risk. While some residential, community-based, employee health, and/or pay-out-of-pocket lifestyle programs have also shown improvement in biomarkers (43–45), this study demonstrates that a lifestyle medicine program based in the primary care clinic of a resource-limited safety-net hospital, working within traditional administrative and insurance structures, can be effectively delivered to patients while also achieving clinically meaningful improvements.
The goal of identifying patients with prediabetes is to help implement healthy lifestyle behaviors to prevent the progression to type 2 diabetes and its complications. Of those identified with prediabetes in our program, 76.5% successfully reduced their HbA1c or avoided an increase. Long-term follow-up of combined diet and exercise programs has demonstrated success in preventing diabetes while also achieving cost savings (46, 47). Among the patients with type 2 diabetes, the average HbA1c reduction was 0.7 percentage points, on par with many glucose-lowering medications (48). Moreover, 50% were able to attain at least a 0.5% reduction in their HbA1c, an amount that has historically been considered clinically meaningful, especially since a positive dose–response association has been reported between HbA1c and cardiovascular outcomes and mortality (34, 35). A meta-analysis by Giugliano et al. evaluated cardiovascular outcome trials of diabetes medications and demonstrated that a 0.5% reduction in HbA1c was associated with a 20% reduction in major cardiovascular events (MACE) (36). Clinically meaningful HbA1c lowering was reported only for patients with diabetes or prediabetes, as further lowering of blood sugar among patients with normal HbA1c is less likely to occur and is of uncertain clinical benefit.
Half of the patients lost at least 3% of their body weight, an amount that is clinically meaningful as it has been shown to translate into meaningful improvement in obesity-related comorbidities (33). Notably, the weight loss occurred without prescribed calorie restriction. The program emphasizes the consumption of unprocessed or minimally processed plant-foods – which are high in fiber and nutrient density, and low in energy density – while encouraging patients to limit or avoid animal products, saturated fats, sodium, added sugars, and ultra-processed foods. Several factors may contribute to the weight-management benefits of a healthful plant-based diet: higher fiber, lower energy density, gut microbiome effects, and increased thermogenesis (49).
We used a 3-mm Hg drop in either systolic or diastolic blood pressure as clinically meaningful as this aligns with the FDA’s criteria for approval of blood pressure lowering medications (38, 39). Moreover, evidence suggests that even modest reductions in blood pressure have the potential to result in meaningful decreases in cardiovascular risk (50, 51). In the entire study population, including those without a diagnosis of hypertension, 43% of patients achieved at least a 3-mm drop in systolic blood pressure and 50% achieved at least a 3-mm drop in diastolic blood pressure. Among those with a diagnosis of hypertension, 40% of patients attained this clinically meaningful drop in systolic blood pressure and 57% attained this clinically meaningful drop in diastolic blood pressure, signifying an important reduction in cardiovascular risk (52, 53).
The baseline LDL-C of patients with hyperlipidemia in our pilot program was 112 mg/dl, with the majority already on statin therapy to lower cholesterol. Long-term drug treatment studies have shown that there is a continuous relationship between LDL-C and MACE, even at low levels of LDL-C (41, 54). For this reason, any LDL-C lowering appears to be clinically meaningful. In this pilot program, 60.5% of patients with hyperlipidemia experienced a decrease in their LDL-C and non-HDL-C. Among those on a stable or reduced cholesterol medication regimen, the average LDL-C was lowered by 14.2 mg/dl to 98.6 mg/dl.
A key finding in this study is that clinical improvements occurred among patients with stable or reduced medication regimens for diabetes, blood pressure, or cholesterol. This strongly suggests that the noted improvements in glycemic control, diastolic blood pressure, lipids, and body weight were due to lifestyle changes themselves, rather than to increases in medications. Typically, healthy lifestyle behaviors are synergistic with medications, resulting in clinical improvements and lower cardiometabolic risk without higher medication burden (21–25). In some cases lifestyle changes may allow for the reduction or cessation of medication. These benefits extend not only to patients’ short- and long-term health, but to lower cost of prescription medications and lower risk of medication side effects.
There are limitations to this study worth noting. First, the majority of patients of the PBLM Program were highly motivated individuals who self-referred into the program. Consequently, the findings may be biased upwards if these individuals were more earnest in their attempts to adhere to program recommendations than a typical patient. Second, some patients reported they were already following a healthful plant-based diet at baseline. Therefore, it is also possible that the study did not fully capture the clinical benefits of transitioning from a less-healthful dietary pattern; this would bias the findings toward the null. Third, findings for the full sample includes patients with a variety of baseline conditions potentially obscuring changes for individual outcomes when only a subset of patients would be expected to improve. Fourth, without a comparison group, we cannot be certain that improvements in clinical outcomes were a result of participation in the PBLM Program. Fifth, certain clinical measurements used in this study may be unreliable. For example, in-office blood pressure measurements are known to be unreliable due to “white-coat” effects and improper measurement technique (55, 56). Additionally, lipid measurements may have been impacted by inconsistency in whether patients fasted prior to measurement. However, this limitation does not apply to non-HDL-C measurements which are not significantly affected fasting status. Sixth, it is also important to note that at times sample sizes were rather small, particularly when evaluating subgroups. Small sample sizes result in reduced power to detect statistically significant differences, as well as increased margin of error. However, because the primary goal of the evaluation was to assess implementation and demand, this study was not designed or powered to assess the PBLM Program’s impact on clinical outcomes and can only provide a preliminary signal as to the effectiveness of the program. Despite these limitations, this study is novel and to our knowledge, the first of its kind to include an under-resourced and vulnerable population in a public healthcare setting.
Given the burden of chronic disease and the high cost of health care, it is essential that the care delivery system move beyond merely managing lifestyle-related illnesses, but rather, addressing their key root causes. Lifestyle medicine, where providers help build a foundation for healthful eating, active living, and emotional resilience, is a promising approach for reaching this goal. The findings from this pilot study are encouraging and may have implications for healthcare practitioners and health systems. Further study is needed to demonstrate additional health benefits over time and the potential for cost-savings to society.
Data availability statement
The raw data supporting the conclusions of this article will be made available by the authors, without undue reservation.
Ethics statement
Approval for this study was obtained by the NYU Grossman School of Medicine Institutional Review Board and the Office of Research and Administration for Implementation at NYC Health + Hospitals/Bellevue. Written informed consent was not required for this study in accordance with the national legislation and the institutional requirements.
Author contributions
SLA and MM co-designed the study. SLA led the study and drafted the manuscript. REM led data management and performed data analysis with LK. LC and MM led data acquisition. HEA advised data analyses. All authors contributed to the interpretation of the results, writing the manuscript, and approved the final draft.
Funding
This work was supported by NYC Health + Hospitals.
Acknowledgments
We thank Krisann Polito-Moller for her professional services in the Plant-Based Lifestyle Medicine Program.
Conflict of interest
The authors declare that the research was conducted in the absence of any commercial or financial relationships that could be construed as a potential conflict of interest.
Publisher’s note
All claims expressed in this article are solely those of the authors and do not necessarily represent those of their affiliated organizations, or those of the publisher, the editors and the reviewers. Any product that may be evaluated in this article, or claim that may be made by its manufacturer, is not guaranteed or endorsed by the publisher.
References
1. Murphy, SL, Kochanek, KD, Xu, J, and Arias, E. Mortality in the United States, 2020. NCHS Data Brief. (2021) 427:1–8.
2. Promotion NCfCDPaH. (2022). About chronic diseases: Centers for Disease Control and Prevention. Available at: https://www.cdc.gov/chronicdisease/about/index.htm (Accessed January 8, 2023).
3. Promotion NCfCDPaH. (2022). Health and economic costs of chronic diseases: Centers for Disease Control and Prevention. Available at: https://www.cdc.gov/chronicdisease/about/costs/index.htm (Accessed January 8, 2023).
4. Buttorff, C, Ruder, T, and Bauman, M. Multilple Chronic Conditions in the United States. Santa Monica, CA: RAND Corporation (2017).
6. Collaborators, GBDD. Health effects of dietary risks in 195 countries, 1990-2017: a systematic analysis for the global burden of disease study 2017. Lancet. (2019) 393:1958–72. doi: 10.1016/S0140-6736(19)30041-8
7. Satija, A, Bhupathiraju, SN, Spiegelman, D, Chiuve, SE, Manson, JE, Willett, W, et al. Healthful and unhealthful plant-based diets and the risk of coronary heart disease in U.S. Adults J Am Coll Cardiol. (2017) 70:411–22. doi: 10.1016/j.jacc.2017.05.047
8. Dinu, M, Abbate, R, Gensini, GF, Casini, A, and Sofi, F. Vegetarian, vegan diets and multiple health outcomes: a systematic review with meta-analysis of observational studies. Crit Rev Food Sci Nutr. (2017) 57:3640–9. doi: 10.1080/10408398.2016.1138447
9. Melina, V, Craig, W, and Levin, S. Position of the academy of nutrition and dietetics: vegetarian diets. J Acad Nutr Diet. (2016) 116:1970–80. doi: 10.1016/j.jand.2016.09.025
10. Qian, FLG, Hu, FB, Bhupathiraju, SN, and Sun, Q. Association between plant-based dietary patterns and risk of type 2 diabetes: a systematic review and meta-analysis. JAMA Intern Med. (2019) 179:1335–44. doi: 10.1001/jamainternmed.2019.2195
11. Kim, H, Caulfield, LE, Garcia-Larsen, V, Steffen, LM, Coresh, J, and Rebholz, CM. Plant-based diets are associated with a lower risk of incident cardiovascular disease, cardiovascular disease mortality, and all-cause mortality in a general population of middle-aged adults. J Am Heart Assoc. (2019) 8:e012865. doi: 10.1161/JAHA.119.012865
12. Turner-McGrievy, G, Mandes, T, and Crimarco, A. A plant-based diet for overweight and obesity prevention and treatment. J Geriatr Cardiol. (2017) 14:369–74. doi: 10.11909/j.issn.1671-5411.2017.05.002
13. Ornish, D, Scherwitz, LW, Billings, JH, Brown, SE, Gould, KL, Merritt, TA, et al. Intensive lifestyle changes for reversal of coronary heart disease. JAMA. (1998) 280:2001–7. doi: 10.1001/jama.280.23.2001
14. Barnard, ND, Cohen, J, Jenkins, DJ, Turner-McGrievy, G, Gloede, L, Green, A, et al. A low-fat vegan diet and a conventional diabetes diet in the treatment of type 2 diabetes: a randomized, controlled, 74-wk clinical trial. Am J Clin Nutr. (2009) 89:1588S–96S. doi: 10.3945/ajcn.2009.26736H
15. Bodai, BI, Nakata, TE, Wong, WT, Clark, DR, Lawenda, S, Tsou, C, et al. Lifestyle medicine: a brief review of its dramatic impact on health and survival. Perm J. (2018) 22:17–025. doi: 10.7812/TPP/17-025
16. Barnard, RJ, Jung, T, and Inkeles, SB. Diet and exercise in the treatment of NIDDM. The need for early emphasis. Diabetes Care. (1994) 17:1469–72. doi: 10.2337/diacare.17.12.1469
17. Termannsen, AD, Clemmensen, KKB, Thomsen, JM, Nørgaard, O, Díaz, LJ, Torekov, SS, et al. Effects of vegan diets on cardiometabolic health: a systematic review and meta-analysis of randomized controlled trials. Obes Rev. (2022) 23:e13462. doi: 10.1111/obr.13462
18. Young, JBJ, and Sokolof, J. Lifestyle medicine: physical activity. J Fam Pract. (2022) 71:S17–23. doi: 10.12788/jfp.0253
19. Baban, KAMD. Lifestyle medicine and stress management. J Fam Pract. (2022) 71:S24–9. doi: 10.12788/jfp.0285
20. Dedhia, PMR. Sleep and health—a lifestyle medicine approach. J Fam Pract. (2022) 71:S30–4. doi: 10.12788/jfp.0295
21. ElSayed, NA, Aleppo, G, Aroda, VR, Bannuru, RR, Brown, FM, Bruemmer, D, et al. 5. Facilitating positive health behaviors and well-being to improve health outcomes: standards of Care in Diabetes—2023. Diabetes Care. (2023) 46:S68–96. doi: 10.2337/dc23-S005
22. Blonde, L, Umpierrez, GE, Reddy, SS, McGill, JB, Berga, SL, Bush, M, et al. American Association of Clinical Endocrinology Clinical Practice Guideline: developing a diabetes mellitus comprehensive care Plan-2022 update. Endocr Pract. (2022) 28:923–1049. doi: 10.1016/j.eprac.2022.08.002
23. Arnett, DK, Blumenthal, RS, Albert, MA, Buroker, AB, Goldberger, ZD, Hahn, EJ, et al. 2019 ACC/AHA guideline on the primary prevention of cardiovascular disease: a report of the American College of Cardiology/American Heart Association task force on clinical practice guidelines. Circulation. (2019) 140:e596–646. doi: 10.1161/CIR.0000000000000678
24. Lichtenstein, AH, Appel, LJ, Vadiveloo, M, Hu, FB, Kris-Etherton, PM, Rebholz, CM, et al. 2021 dietary guidance to improve cardiovascular health: a scientific statement from the American Heart Association. Circulation. (2021) 144:e472–87. doi: 10.1161/CIR.0000000000001031
25. Belardo, D, Michos, ED, Blankstein, R, Blumenthal, RS, Ferdinand, KC, Hall, K, et al. Practical, evidence-based approaches to nutritional modifications to reduce atherosclerotic cardiovascular disease: an American society for preventive cardiology clinical practice statement. Am J Prev Cardiol. (2022) 10:100323. doi: 10.1016/j.ajpc.2022.100323
26. Jacobson, TA, Maki, KC, Orringer, CE, Jones, PH, Kris-Etherton, P, Sikand, G, et al. National Lipid Association Recommendations for patient-centered Management of Dyslipidemia: part 2. J Clin Lipidol. (2015) 9:129–69. doi: 10.1016/j.jacl.2015.02.003
27. Rock, CL, Thomson, C, Gansler, T, Gapstur, SM, McCullough, ML, Patel, AV, et al. American Cancer Society guideline for diet and physical activity for cancer prevention. CA Cancer J Clin. (2020) 70:245–71. doi: 10.3322/caac.21591
28. Benigas, S, Shurney, D, and Stout, R. Making the case for lifestyle medicine. J Fam Pract. (2022) 71:296. doi: 10.12788/jfp.0296
29. American College of Lifestyle Medicine. (2021). Health systems council. Available at: https://lifestylemedicine.org/ACLM/Partners/Health-Systems-Council/ACLM/Partners/Health-Systems/Health-Systems-Council.aspx?hkey=ca721dcc-8bfa-457c-b1cc-ee10e7866172 (Accessed January 8, 2023).
30. Aldana, SG, Greenlaw, RL, Diehl, HA, Salberg, A, Merrill, RM, Ohmine, S, et al. The behavioral and clinical effects of therapeutic lifestyle change on middle-aged adults. Prev Chronic Dis. (2006) 3:A05.
31. Albert, SL, Massar, RE, Kwok, L, Correa, L, Polito-Moller, K, Joshi, S, et al. Pilot plant-based lifestyle medicine program in an urban public healthcare system: evaluating demand and implementation. Am J Lifestyle Med. (2022). doi: 10.1177/15598276221113507. [Epub ahead of print].
32. Jensen, MD, Ryan, DH, Apovian, CM, Ard, JD, Comuzzie, AG, Donato, KA, et al. AHA/ACC/TOS guideline for the management of overweight and obesity in adults: a report of the American College of Cardiology/American Heart Association task force on practice guidelines and the Obesity Society. J Am Coll Cardiol. (2014) 63:2985–3023. doi: 10.1016/j.jacc.2013.11.004
33. Wharton, S, Lau, DCW, Vallis, M, Sharma, AM, Biertho, L, Campbell-Scherer, D, et al. Obesity in adults: a clinical practice guideline. Can Med Assoc J. (2020) 192:E875–91. doi: 10.1503/cmaj.191707
34. Little, RR, and Rohlfing, CL. The long and winding road to optimal HbA1c measurement. Clin Chim Acta. (2013) 418:63–71. doi: 10.1016/j.cca.2012.12.026
35. Zhang, Y, Hu, G, Yuan, Z, and Chen, L. Glycosylated hemoglobin in relationship to cardiovascular outcomes and death in patients with type 2 diabetes: a systematic review and meta-analysis. PLoS One. (2012) 7:e42551. doi: 10.1371/journal.pone.0042551
36. Giugliano, D, Chiodini, P, Maiorino, MI, Bellastella, G, and Esposito, K. Cardiovascular outcome trials and major cardiovascular events: does glucose matter? A systematic review with meta-analysis. J Endocrinol Investig. (2019) 42:1165–9. doi: 10.1007/s40618-019-01047-0
37. Reduction in the incidence of type 2 diabetes with lifestyle intervention or metformin. N Engl J Med. (2002) 346:393–403. doi: 10.1056/NEJMoa012512
38. Juraschek, SP, Miller, ER 3rd, Weaver, CM, and Appel, LJ. Effects of sodium reduction and the DASH diet in relation to baseline blood pressure. J Am Coll Cardiol. (2017) 70:2841–8. doi: 10.1016/j.jacc.2017.10.011
39. United States. Department of Health and Human Services Food and Drug Administration. Statistical Review and Evaluation Clinical Studies SPP100/Aliskiren (Tekturna/Rasilez). Department of Health and Human Services Food and Drug Administration (2017).
40. Collins, R, Reith, C, Emberson, J, Armitage, J, Baigent, C, Blackwell, L, et al. Interpretation of the evidence for the efficacy and safety of statin therapy. Lancet. (2016) 388:2532–61. doi: 10.1016/S0140-6736(16)31357-5
41. Ray, KK, Ginsberg, HN, Davidson, MH, Pordy, R, Bessac, L, Minini, P, et al. Reductions in Atherogenic lipids and major cardiovascular events: a pooled analysis of 10 ODYSSEY trials comparing Alirocumab with control. Circulation. (2016) 134:1931–43. doi: 10.1161/CIRCULATIONAHA.116.024604
43. Friedman, SM, Barnett, CH, Franki, R, Pollock, B, Garver, B, and Barnett, TD. Jumpstarting health with a 15-day whole-food plant-based program. Am J Lifestyle Med. (2022) 16:374–81. doi: 10.1177/15598276211006349
44. Drozek, D, DeFabio, A, Amstadt, R, and Dogbey, GY. Body mass index change as a predictor of biometric changes following an intensive lifestyle modification program. Adv Prev Med. (2019) 2019:1–5. doi: 10.1155/2019/8580632
45. Campbell, EK, Fidahusain, M, and Campbell Ii, TM. Evaluation of an eight-week whole-food plant-based lifestyle modification program. Nutrients. (2019) 11:2068. doi: 10.3390/nu11092068
46. Li, R, Qu, S, Zhang, P, Chattopadhyay, S, Gregg, EW, Albright, A, et al. Economic evaluation of combined diet and physical activity promotion programs to prevent type 2 diabetes among persons at increased risk: a systematic review for the community preventive services task force. Ann Intern Med. (2015) 163:452–60. doi: 10.7326/M15-0469
47. Gilmer, T, O'Connor, PJ, Schiff, JS, Taylor, G, Vazquez-Benitez, G, Garrett, JE, et al. Cost-effectiveness of a community-based diabetes prevention program with participation incentives for Medicaid beneficiaries. Health Serv Res. (2018) 53:4704–24. doi: 10.1111/1475-6773.12973
48. Taylor, SI, Yazdi, ZS, and Beitelshees, AL. Pharmacological treatment of hyperglycemia in type 2 diabetes. J Clin Invest. (2021) 131:42243. doi: 10.1172/JCI142243
49. Najjar, RS, and Feresin, RG. Plant-based diets in the reduction of body fat: physiological effects and biochemical insights. Nutrients. (2019) 11:2712. doi: 10.3390/nu11112712
50. National High Blood Pressure Education Program Working Group report on primary prevention of hypertension. Arch Intern Med. (1993) 153:186–208. doi: 10.1001/archinte.1993.00410020042003
51. Stamler, R. Implications of the INTERSALT study. Hypertension. (1991) 17:I16–20. doi: 10.1161/01.HYP.17.1_Suppl.I16
52. Hardy, ST, Loehr, LR, Butler, KR, Chakladar, S, Chang, PP, Folsom, AR, et al. Reducing the blood pressure-related burden of cardiovascular disease: impact of achievable improvements in blood pressure prevention and control. J Am Heart Assoc. (2015) 4:e002276. doi: 10.1161/JAHA.115.002276
53. Ettehad, D, Emdin, CA, Kiran, A, Anderson, SG, Callender, T, Emberson, J, et al. Blood pressure lowering for prevention of cardiovascular disease and death: a systematic review and meta-analysis. Lancet. (2016) 387:957–67. doi: 10.1016/S0140-6736(15)01225-8
54. Wadhera, RK, Steen, DL, Khan, I, Giugliano, RP, and Foody, JM. A review of low-density lipoprotein cholesterol, treatment strategies, and its impact on cardiovascular disease morbidity and mortality. J Clin Lipidol. (2016) 10:472–89. doi: 10.1016/j.jacl.2015.11.010
55. Burkard, T, Mayr, M, Winterhalder, C, Leonardi, L, Eckstein, J, and Vischer, AS. Reliability of single office blood pressure measurements. Heart. (2018) 104:1173–9. doi: 10.1136/heartjnl-2017-312523
Keywords: plant-based diet, lifestyle medicine, chronic disease, lifestyle modification, cardiovascular risk, outcome evaluation, lifestyle intervention, cardiometabolic risk
Citation: Albert SL, Massar RE, Correa L, Kwok L, Joshi S, Shah S, Boas R, Alcalá HE and McMacken M (2023) Change in cardiometabolic risk factors in a pilot safety-net plant-based lifestyle medicine program. Front. Nutr. 10:1155817. doi: 10.3389/fnut.2023.1155817
Edited by:
Andrea K. Boggild, University of Toronto, CanadaReviewed by:
Jay Sutliffe, Northern Arizona University, United StatesGeeta Sikand, University of California, Irvine, United States
Copyright © 2023 Albert, Massar, Correa, Kwok, Joshi, Shah, Boas, Alcalá and McMacken. This is an open-access article distributed under the terms of the Creative Commons Attribution License (CC BY). The use, distribution or reproduction in other forums is permitted, provided the original author(s) and the copyright owner(s) are credited and that the original publication in this journal is cited, in accordance with accepted academic practice. No use, distribution or reproduction is permitted which does not comply with these terms.
*Correspondence: Stephanie L. Albert, c3RlcGhhbmllLmFsYmVydEBueXVsYW5nb25lLm9yZw==