- 1Pain and Rehabilitation Centre, Department of Health, Medicine and Caring Sciences, Linköping University, Linköping, Sweden
- 2Department of Neurosurgery and Pain Rehabilitation, Skåne University Hospital, Lund, Sweden
- 3Department of Health Sciences, Faculty of Medicine, Lund University, Lund, Sweden
Background: Both chronic pain and depressive and/or anxiety symptoms are associated with negative impacts on daily living, including interference and lack of life control. However, little is known about how pain and psychological distress affect these impacts.
Aim: The first aim was to assess how pain intensity, psychological distress, and social support interact with interference and lack of life control. A second aim was to investigate whether the strength of these relationships is moderated by the presence or absence of depression and/or anxiety.
Subjects and methods: Patient-Reported Outcome Measures (PROMs), which are available in the Swedish Quality Registry for Pain Rehabilitation (SQRP), were retrieved for patients with chronic pain (N = 40,184). A theoretical model with the constructs/latent variables pain intensity, psychological distress, interference, lack of life control, and social support was proposed and analyzed using Partial Least Squares Structural Equation Modelling (PLS-SEM). Indicators for these constructs were identified from the PROMs of the SQRP. Two models of the total cohort, which differed with respect to the causal relationship between pain intensity and psychological distress, were investigated. The moderating effects of anxiety and/or depression were also analyzed.
Results: Relatively low correlation and explanatory power (R2 = 0.16) were found for the pain intensity-psychological distress relationship. Pain intensity had a stronger effect on interference than on lack of life control. The reverse was found for psychological distress – i.e., psychological distress seemed to have a higher negative influence on function than on interference. The underlying assumption of the causal relationship between pain intensity and psychological distress determined how strong pain intensity and psychological distress influenced interference and lack of life control. Social support showed very similar absolute significant correlations with interference and lack of life control. Interference and lack of life control showed relatively weak associations. The psychological distress level was a moderating factor for several of the paths investigated.
Discussion and conclusion: A clinical treatment consequence of the low correlation between pain intensity and psychological distress may be that clinically treating one may not reduce the effect of the other. The relative importance of pain intensity and psychological distress on interference and lack of life control depends on the underlying assumption concerning the pain intensity-psychological distress relationship. Interference and lack of life control showed relatively weak associations, underscoring the need to clinically assess them separately. Social support influenced both impact constructs investigated. The cohort display heterogeneity and thus presence of definite signs of anxiety and/or depression or not was a moderating factor for several of the associations (paths) investigated. The results are important both for the assessments and the design of treatments for patients with chronic pain.
1. Introduction
Chronic pain conditions are associated with increased risks for intense and disturbing pain, psychological distress, disability, poor health, and low quality of life (1–6). Pain is a complex interaction of biological, psychological, social, and contextual factors (7–9). Hence, modern clinical practice uses a biopsychosocial framework when assessing patients and designing and performing interventions (10, 11).
Depression and anxiety are common co-morbidities in chronic pain. Data from the Swedish Quality Registry for Pain Rehabilitation (SQRP) covering specialist care for chronic pain patients indicated that 39.5% of the cohort fulfilled the criteria for a highly probable anxiety case according to Hospital Anxiety and Depression Scale (HADS); the corresponding figure for depressive symptoms was 35.2% (12). Some studies report higher prevalence for depression, which can be due to methods applied and societal factors (13, 14). Complex bidirectional relationships between pain and mood exist according to cross-sectional and longitudinal studies, but these and other studies posit different theories to explain these relationships (13, 15–23). According to a large SQRP study (N > 35,000 chronic pain patients), the levels of pain intensity and mood aspects were significantly correlated but with relatively low explained variances (R2) (pain intensity variables vs. depressive symptoms of HAD: R2 = 0.07–0.11; pain intensity vs. anxiety symptoms: R2 = 0.07–0.09) (12). A network analysis of another SQRP cohort (N = 2,241) confirmed weak correlations between pain intensity and the two mood variables (24).
Both chronic pain and depressive and anxiety symptoms are associated with different negative impacts on daily living and functioning, treatment responses, and health costs (15, 25–34). Thus, pain and psychological distress are associated with interference in daily life and perceived lack of life control (11, 35). For example, a mix of variables (pain intensity, emotion variables, fear, etc.) influence life impact variables such as life control and interference as previously reported from SQRP (35–38). Pain intensity was somewhat more important for interference than depressive symptoms, whereas life control was more strongly associated with symptoms of anxiety and depression (38). However, only 43%–53% of the variations in these two life impact variables were accounted for. Thus, there is an incomplete understanding of what variables determine these aspects. Also, in the network study, pain intensity, depression, and anxiety showed strong associations with pain interference (24). However, for life control aspects, anxiety and depression had stronger relationships than pain intensity (24). Differentiated associations for pain intensity and mood variables in relation to impact aspects have also been reported in tension-type headache (39).
In addition to traditional outcomes such as pain, psychological distress, interference and function, also social outcomes have been advocated as important (40). Social support is defined as the resources received and perceived as being available from others in one's social network (41). Social support can help patients cope with and distract from negative life events and manage pain and its impacts (41–43).
The holistic biopsychosocial model considers complex relationships between the different facets of living with chronic pain. For example, pain intensity may act both via direct and indirect (mediating) effects on aspects of life impact. There is a knowledge gap of the relative importance of pain intensity and psychological distress for aspects of life impact such as interference and life control in real life practise settings. Presence of moderating variables indicate cohort heterogeneity. The combination of both pain and depression is associated with poorer treatment outcomes than either condition alone (44, 45), which may indicate that psychological distress is a moderating factor.
Partial Least Squares Structural Equation Modelling (PLS-SEM) is a non-parametric method that can be applied to model and estimate complex relationships (paths) among multiple dependent and independent variables (46). Rather than using only one variable for indicating a certain aspect, PLS-SEM is based on latent variables/constructs, which generally are covered by several variables (indicators). Therefore, the construct (e.g., pain intensity) will be more accurately measured (i.e., reduced measurement error) (46). PLS-SEM is always based on a theoretical model – i.e., a complex hypothesis. The relationships between the constructs1 in the proposed theoretical model are shown in Figure 1. In agreement with the referred literature, we expected that Pain intensity and Psychological distress were intercorrelated and that both components in turn affect Interference and Lack of life control (47, 48). Interference is the disruption of daily life activities directly due to chronic pain, which, in the literature has been related to a low level of physical function (11). To the best of our knowledge, there is no well-established broader definition of Lack of control. Hence, in this study we define Lack of life control as the impaired ability to control daily life situations, including social life activities. Moreover, we assume that Interference has a positive association with Lack of life control (i.e., prominent interference impairs life control, including social life activities). Furthermore, as a robust social network has a “pain-buffering” effect (49), we hypothesised that Social support affects both the constructs of Interference and Lack of life control.
Earlier studies from this cohort (not imputed data) found that age, sex, education level, country of birth, pain duration, and spatial extent of pain on the body had low correlations with variables indicating our proposed constructs. Therefore, we primarily did not involve them in the theoretical model.
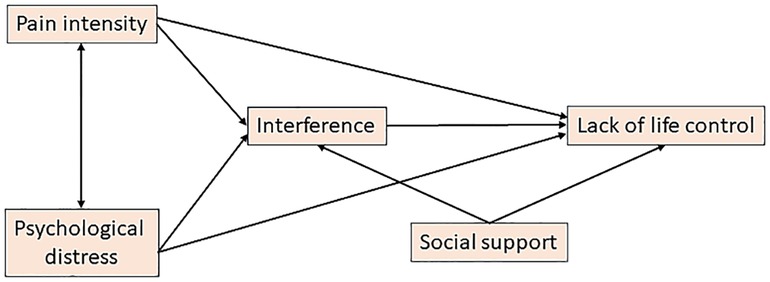
Figure 1. The theoretical model. Constructs/latent variables are shown together with the paths including directions. For the pain intensity-psychological distress relationship, two options (models) are explored (here indicated by a line with an arrowhead at either end).
This large clinical registry-based cohort study has two aims. First, using advanced path analysis, we assess how Pain intensity, Psychological distress, and Social support interact directly and indirectly with Interference and Lack of life control. Second, we investigate whether the strength of these relationships is moderated by the presence of definite signs of depression and/or anxiety.
2. Materials and methods
2.1. Subjects and the Swedish quality registry for pain rehabilitation (SQRP)
The SQRP registers Patient-Reported Outcome Measures (PROMs) data from a majority of the Swedish specialist chronic pain departments (35). This study investigates adult patients (i.e., ≥18 years) with chronic (≥3 months) non-malignant pain registered in the SQRP between 2008 and 2016. Most of the patients were referred by primary care, but some were referred by rheumatology and orthopaedic specialist departments. Patients enrolled in SQRP have complex pain conditions that often include comorbidities (e.g., depression and anxiety), insufficient coping strategies, long sick leave, low social participation, and/or unresponsiveness to monomodal treatments (e.g., pharmacological, and physiotherapeutic interventions). As SQRP is a clinical registry of patients with chronic pain conditions, the SQRP does not have strict inclusion and exclusion criteria. General inclusion criteria include disabling chronic pain (i.e., chronic pain that results in sick leave or major interference in daily life), 18 years and older, medically fully investigated, and written consent to participate. General exclusion criteria include severe psychiatric morbidity, abuse of alcohol and/or drugs, diseases that do not allow physical exercise, and specific pain conditions with other treatment options available (i.e., conditions associated with “red flags”).
2.2. Ethics
The study was conducted in accordance with the Helsinki Declaration. It was approved by the Ethical Review Board in Linköping (Dnr: 2015/108-31). All the participants received written information about the study and gave their written consent.
2.3. Patient-Reported outcome measures (PROMs)
The PROMs capture a patient's background including demographic aspects, pain intensity, pain-related cognitions, and psychological distress symptoms as well as activity/participation aspects and health-related quality of life variables. PROMs are completed by patients on up to three occasions: before the first visit (baseline assessment), immediately after treatment for those who participated in interdisciplinary pain rehabilitation programs (IPRP; approximately 50%–55% of the patients assessed), and at a 12-month follow-up. In the present study, only baseline data are analysed. In the present baseline study, 19 variables were initially considered for inclusion in the PLS-SEM analyses – i.e., six background variables and 13 of 22 mandatory outcome variables (i.e., variables that all participating clinics/units collect from patients. In addition to this, there are other – non-mandatory – variables that the clinic/unit can include if desired) (Table 1).
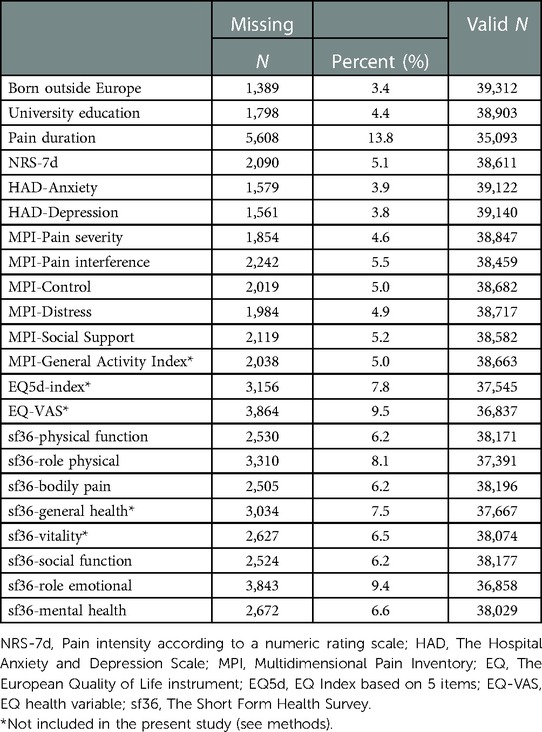
Table 1. Variables with missing data before imputation – baseline data. Gender, age, and PRI had no missing data. Note that some of these variables were not included in the subsequent analyses (see methods).
2.3.1. Background variables
The following background variables have been described in detail elsewhere (50–53):
• age (years)
• sex
• education level (university vs. no university)
• country of birth (born in vs. outside of Europe)
• pain duration (days)
• spatial extent of pain [Pain Region Index (PRI) – i.e., painful areas marked on 36 predefined anatomical regions] (12, 53)
2.3.2. Mandatory outcome variables in SQRP
The SQRP includes 22 mandatory outcome variables registered on up to three occasions. These variables are consistent with the outcome domains of the Initiative on Methods, Measurement, and Pain Assessment in Clinical Trials (IMMPACT) (11, 54) and the Validation and Application of a patient-relevant core set of outcome domains to assess multimodal PAIN therapy (VAPAIN) (55) initiatives. The mandatory variables, including psychometric properties, have been detailed elsewhere (50–53):
• Mean pain intensity over the past seven days is measured using an 11-point numerical rating scale (0 = no pain to 10 = worst possible pain; NRS-7d).
• The Hospital Anxiety and Depression Scale (HAD) captures signs of depression (HAD-D) and anxiety (HAD-A) (56, 57). Levels ≥11 (possible range: 0–21 for each subscale) indicates a definite case for anxiety or depression. In the multivariate analyses, the two scales (HAD-tot) were summed according to a previous large psychometric study (58).
• The Swedish version of the Multidimensional Pain Inventory (MPI) describes the multidimensional nature of chronic pain. Part one has five subscales: pain severity (MPI-Pain severity), interference (MPI-Pain interference), life control (MPI-Control), psychological distress (MPI-Distress), and perceived social support (MPI-Social Support). MPI-Pain Severity concerns current pain intensity and average pain intensity over the previous seven days. MPI-Pain Interference is based on 11 items covering disturbances due to pain in daily activities, work capacity, leisure activities, general reductions in activities, and the ability to socialize and have relations with family and friends. MPI-Control is based on four items concerning the ability to manage daily life problems, pain, and stressful situations. MPI-Distress is based on three items – mood, irritation, and anxiety in the previous seven days. MPI-Social Support is based on two items – support and help from family or relatives and consideration from family or relatives when having pain. In part two, patients report how they perceive significant others' responses to pain or suffering expressed by the patient in three subscales. The second part was not used in the present study due to high proportions of missing data. Part three measures participation in various activities (i.e., household chores, outdoor work, activities away from home, and being together with family and friends), which are combined into a General Activity Index in the Swedish version (59). The index was not included in the present study due to lack of construct validity (60).
• The European Quality of Life instrument (EQ-5D) measures generic Health-Related Quality of Life (61–63). EQ-5D was not included in the multivariate analyses of the present study.
• The Short Form Health Survey (sf36) assesses eight aspects/dimensions (scored from 0 to 100, with higher scores indicating a better perception) of generic Health-Related Quality of Life (64): (1) physical functioning is a 10-item measure of physical limitation/ability in a range of activities from self-care to exercise (sf36-physical function); (2) role limitations are related to physical functioning including work and daily activities based on four items (sf36-role physical); (3) bodily pain is based on two items measuring pain intensity and the extent pain interferes with daily activities (sf36-bodily pain); (4) general health is a five-item scale concerning self-related health (sf36-general health); (5) the vitality scale is based on four items concerning vitality, energy level, and fatigue with the intention to measure subjective well-being (sf36-vitality); (6) social functioning is based on two items covering the impact of physical and mental health on social functioning (sf36-social function); (7) role limitations due to emotional problems is based on three items (sf36-role emotional); and (8) mental health scale has five items measuring anxiety, depression, loss of behavioural/emotional control, and psychological well-being (sf36-mental health) (65). In the present study, sf36-general health and sf36-vitality were not included in the multivariate analyses as they measure global aspects of health and wellbeing, which are not the focus of this study.
2.3.3. Identification of indicators for the constructs/latent variables
The constructs according to our theoretical model/hypotheses are shown in Figure 1. Both MPI and sf36 are comprehensive instruments and composed by several subscales. Clinical reasoning as well as descriptions in the literature of the different subscales were applied when determining the usefulness of the above selected SQRP variables as indicators in relation to the latent variables (Table 2) (59, 65–67). Since all indicators of a certain construct must be positively intercorrelated some of the potential indicators were reversed. We identified three variables that mainly measure pain intensity – NRS-7d, MPI-Pain severity, and sf-36-bodily pain (was reversed). We identified four indicators of psychological distress – HAD-tot, MPI-Distress, sf36-mental health (reversed), and sf36-role emotional (reversed). For Interference, we identified three indicators – MPI-Pain interference, sf36-physical function (reversed), and sf36-role physical (reversed). Although these aspects are interchangeably labelled as Interference and physical functioning (68), we chose the former expression to reflect interference and associated physical function (11). Our construct Interference agrees with other studies that bring these together into one domain, at least in cross-sectional studies (47, 68). Thus, our concept Interference covers pain interference and physical function (11). The Lack of life control construct indicates the inability to control life aspects, including social life activities. MPI-Control (reversed) and sf36-social function (reversed) were identified as indicators. MPI-Social support was used as a single indicator of social support, which mainly reflects support from a spouse and significant others.
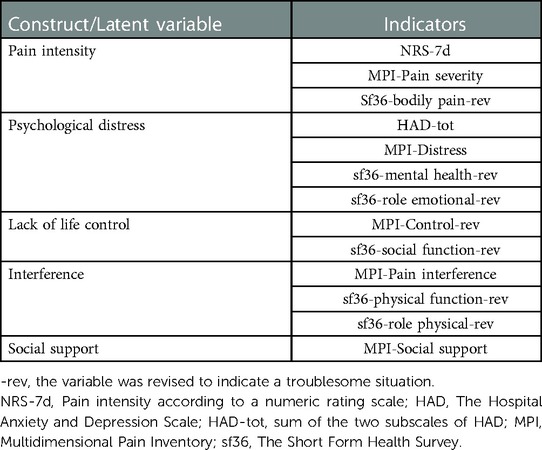
Table 2. Summary of constructs/latent variables and indicators included in the initial PLS-SEM analysis of the total cohort.
As mentioned above, earlier analyses have found that age, sex, education level, country of birth, pain duration, and spatial extent of pain (i.e., the PRI) have low correlations with variables indicating our constructs. At first, we did not use them in the theoretical model. However, we eventually decided to include them in the theoretical model if our previous results were not confirmed using imputed data for the cohort.
2.4. Statistics
We used three statistical packages: IBM SPSS Statistics (version 28.0; IBM Corporation, Route 100 Somers, New York, United States); SIMCA-P + (version 17.0; Sartorius Stedim Biotech, Umeå, Sweden); and Smart-PLS version 3 (Ringle, C. M., Wende, S., and Becker, J.-M. 2015. “SmartPLS 3” Boenningstedt: SmartPLS GmbH, http://www.smartpls.com). In the text and tables, the mean value ± one standard deviation (±1 SD) of continuous variables and percentages (%) for categorical variables are reported. To compare groups, Student's t-test for independent samples and Chi squared tests were applied. In large samples, small differences may be significant and effects size can be used to evaluate the clinical importance of the significant differences. Effect sizes (ES; Cohen's d) for within group analyses were computed and Hedges' g – a measure of effect size weighted by the relative size of each sample – was used for between group ESs. The absolute effect size was considered clinically insignificant for <0.20, small for 0.20–0.49, moderate for 0.50–0.79, and large for ≥0.80 (69).
The retrieved SQRP data included missing data (Table 1). Our analysis of missingness mechanisms indicated that data were not missing completely at random (MCAR) (i.e., Little's Test of Missing Completely at Random was not significant) and therefore data were missing either at random (MAR) or not missing at random (NMAR) (70). One choice may have been to use listwise deletion, but, multiple imputation gives less biased results than listwise deletion (70). The multiple imputation option in SPSS was used for the imputation of our variables (Random generator: Mersenne Twister with a fixed starting point for the active generator initialization; all variables were included in five imputations, which were pooled). The imputed data are shown in Table 3.
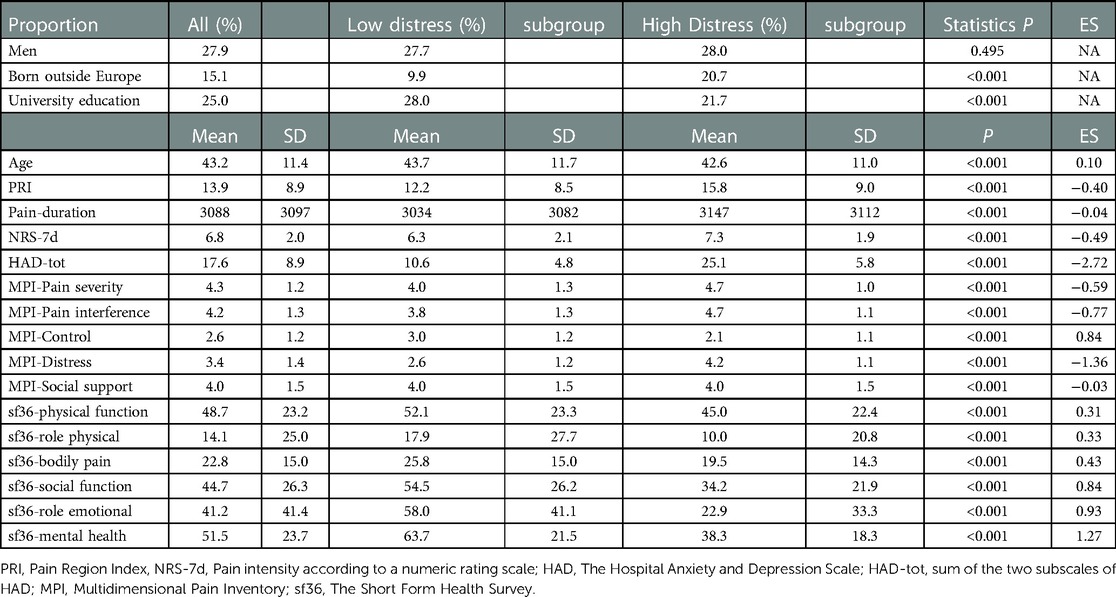
Table 3. Mean and SD for the imputed data for the total cohort (N = 40,186 for all variables) (left). On the right is shown the two subgroups (i.e., Low distress (N = 20,986) and High distress (N = 19,288). Furthest to the right are shown the results of the comparisons between the two subgroups (P-value and effect size ES (Hedges' g) for the quantitative variables.
Previous studies have discussed the necessity of using advanced multivariate analyses (MVDA) when accounting for system-wide aspects and multicollinearity problems (53, 71). Advanced Principal Component Analysis (PCA), which is available in SIMCA-P+, can be used to determine multivariate outliers and multivariate correlation patterns. Outliers were identified using score plots in combination with Hotelling's T2 and distance to model in X-space. PCA can conceptually be viewed as a kind of multivariate correlation analysis that extracts and displays systematic variation in the data matrix. To identify nontrivial components (p), we used a cross validation technique. Variables loading on the same component p are correlated; variables with high loadings but opposing signs are negatively correlated. Variables with high absolute loadings were considered significant. The obtained components are not correlated but are arranged in decreasing order with respect to explained variation. R2 describes the goodness of fit – the fraction of sum of squares of all the variables explained by a principal component (72). Q2 describes the goodness of prediction – the fraction of the total variation of the variables that can be predicted using principal component cross validation methods (72).
Orthogonal Partial Least Square Regressions (OPLS) was used for the multivariate regression analyses of pain intensity and psychological distress variables. The variable influence on projection (VIP) indicates the relevance of each X-variable pooled over all dimensions and Y-variables – the group of variables that best explains Y (72). A variable was considered significant when VIP > 1.0 (or VIPpred if > one component) and had a 95% jack-knife uncertainty confidence interval non-equal to zero. P(corr) was used to note the direction of the relationship (positive or negative) – i.e., the loading of each variable was scaled as a correlation coefficient and therefore standardised the range from −1 to +1 (71). Thus, a variable/regressor was considered statistically significant when VIP or VIPpred > 1.0. A regression model was obtained, including one or several components (the first is always the predictive component), if certain predefined criteria were fulfilled. The validity of the model was estimated using cross validation. Hence, for each regression, we report R2, Q2, and the P-value of a cross-validated analysis of variance (CV-ANOVA).
SMART-PLS version 3 (Ringle, C. M., Wende, S., and Becker, J.-M. 2015. “SmartPLS 3.” Boenningstedt: SmartPLS GmbH, http://www.smartpls.com) was used for the Partial Least Squares Structural Equation Modelling (PLS-SEM). This non-parametric method enables researchers to simultaneously model and estimate complex relationships among multiple dependent and independent variables. The method has a causal-predictive approach to SEM, which intends to explain the variance of the dependent variables; for details of this method, see (46). The recommendations by Hair Jr et al. were followed when performing the PLS-SEM analyses (46). This method is based on constructs (latent variables) that are generally associated with several indicators. The indicators of a certain component must have the same direction – i.e., they cannot be negatively intercorrelated. The measurement model (outer model) displays the relationships between the constructs and its indicator variables. In the present study, a reflective relationship was assumed for all indicator-construct relationships (not relevant for single indicator constructs). Exogenous latent variables are constructs that explain other constructs – i.e., endogenous latent variables (46). The structural model displays the relationships (paths) between the constructs. Indicator reliability was determined using the outer loadings (possible range: −1–1), and it is generally required that the absolute loadings are >0.708. It is recommended that indicators with absolute loadings ≤0.40 be omitted, and indicators with absolute loadings between 0.40 and 0.708 be omitted only if this result increased internal consistency or convergent validity. Internal consistency reliability was determined using exact (consistent) reliability coefficient (ρA; possible range: 0–1), and >0.50 was required. Convergent validity was determined using Average Variance Extracted (AVE; possible range: 0–1), and >0.50 was required. Heterotrait-monotrait ratio (HTMT) was used as an indicator of discriminant validity, and we required values <0.90, preferably <0.85.
To evaluate the structural/inner model, presence of collinearity was checked using variance inflation factor (VIF), and values < 5 were acceptable, preferably <3 (46). Path coefficients (i.e., standardized regression coefficients displaying direct effects) were determined, including mean ± SD, t-values, p-values, and 95% confidence intervals (95% CI). To determine path coefficients and total effects, indirect effects and specific indirect effects, we performed bootstrapping (options: 10,000 samples, complete bootstrapping, percentile boot strap, two-tailed, p = 0.05). Explanatory power was determined from coefficient of determination (R2, possible range: 0–1) as well as f2 effect size. The latter expresses the change in R2 when a specific predecessor construct is omitted from the model (<0.02 = no measurable effect; 0.02–0.14 = small effect; 0.15–0.34 = medium effect; and ≥0.35 large effect). Bootstrapping was performed to obtain t-values, p-values, and 95% CI of these coefficients. In this explorative study, predictive power was not in focus. However, using the PLSpredictive option (10 folds and 10 repetitions), we determined Q2predictive values. Values > 0 indicated that the model had predictive relevance. The greater value of Q2, the greater predictability of the model: < 0.02 = no predictive relevance, 0.02–0.14 = small relevance, 0.15–0.34 = relevance, and ≥0.35 = large predictive relevance (73).
3. Results
3.1. Descriptive data for the total cohort
The imputed data are shown in Table 3. Most patients were women, a large majority were born in Europe, and one-fourth had a university education. Patients had on average a relatively high pain intensity and reported high levels of psychological distress and negative effects upon interference, physical and social function, and life control. The prevalence of definite cases for anxiety and depression according to HAD were 38.0% and 33.9%. Hence, this clinical pattern is consistent with non-imputed data for this cohort (12, 53).
3.2. PCA including check for multivariate outliers
Using a PCA in SIMCA-P+, we found two strong multivariate outliers (MCEID: 12543 and 16266), so we excluded them from the subsequent analysis. Thereafter, a PCA of the included variables (R2cummulative = 0.38, Q2cummulative = 0.25) was conducted (some MPI variables and all sf36 variables were reversed so that high values indicated a troublesome/negative situation) (Figure 2), which identified two significant components. Important variables for the first component (R2 = 0.27, Q2 = 0.19) were psychological variables (sf36-mental health, HAD-tot, MPI-Distress, and sf36-role emotional) as well as pain intensity variables (sf36-bodily pain, NRS-7d, and MPI-Pain severity). Also, interference (MPI-Pain interference), physical function aspects (sf36-physical function and sf36-role physical), social aspects (sf36-social function), and control (MPI-Control) had importance for this component. The second component (R2 = 0.12, Q2 = 0.05) mainly reflected social support (MPI-Social support) but also mental health (sf36-mental health) and control aspects (MPI-Control). Several of the sociodemographic variables showed low importance (loaded near zero) – i.e., pain duration, age, gender, university, outside-Europe, and PRI.
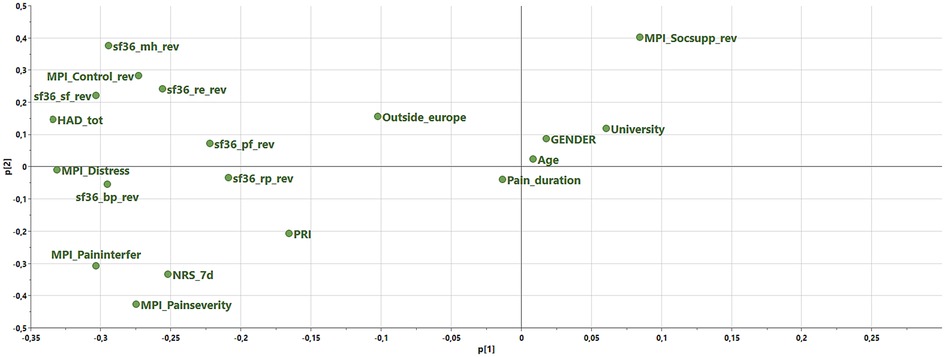
Figure 2. Loadings of a principal component analysis (PCA) of the included variables for the total cohort (N = 40,184). Two components (p1 and p2) are shown. Note that some of the MPI variables and all sf36 variables were revised (indicated with _rev in the variable name) so that high values indicated a troublesome/negative situation. NRS_7d, Pain intensity according to a numeric rating scale; University, University education (binary variable); Outside_Europe, born outside Europe (binary variable), PRI, Pain region Index; HAD, The Hospital Anxiety and Depression Scale; HAD-tot, sum of the two subscales of HAD; MPI, Multidimensional Pain Inventory; MPI_Paininterfer, MPI Pain interference; MPI_Painseverity, MPI Pain severity; MPI_Socsupp, MPI Social support; sf36, The Short Form Health Survey; sf36_pf, sf36-physical function; sf36_rp, sf36-role physical; sf36_bp, sf36-bodily pain; sf36_sf, sf36-social function; sf36_re, sf36-role emotional; sf36_mh, sf36-mental health.
3.3. OPLS regressions of psychological distress and pain intensity variables
In the next step, the four psychological variables and the three pain intensity variables were regressed (Supplementary Tables S1, S2). As expected, the psychological distress variables and the three pain intensity variables were generally intercorrelated. Pain intensity and psychological distress exhibited different strengths in their relationships to the impacts. Hence, psychological distress aspects were associated with control (MPI-Control), and social function (sf36-social function) in the four regressions and with interference in three of the regressions (MPI-Pain interference). Generally, MPI-Control was a stronger regressor than MPI-Pain interference. In the regressions of the pain intensity variables, pain interference was an important regressor in three regressions, and physical function (sf36-physical function) and social support (MPI-Social support) were important regressors (X-variables) in two regressions.
3.4. PLS SEM of the total cohort
3.4.1. Selection of variables for PLS-SEM analyses
To achieve a parsimonious model in the PLS-SEM analysis, only variables with absolute loadings >0.20 in the PCA were included (Figure 2). In addition, the OPLS regressions indicated that these excluded variables had low importance (Supplementary Tables S1, S2). The included indicators in the subsequent PLS-SEM analyses as well as their constructs/latent variables are listed in Table 2. We assumed that psychological distress directly affected pain intensity (model 1) and then assumed that pain intensity directly affected psychological distress (model 2) (Figure 3).
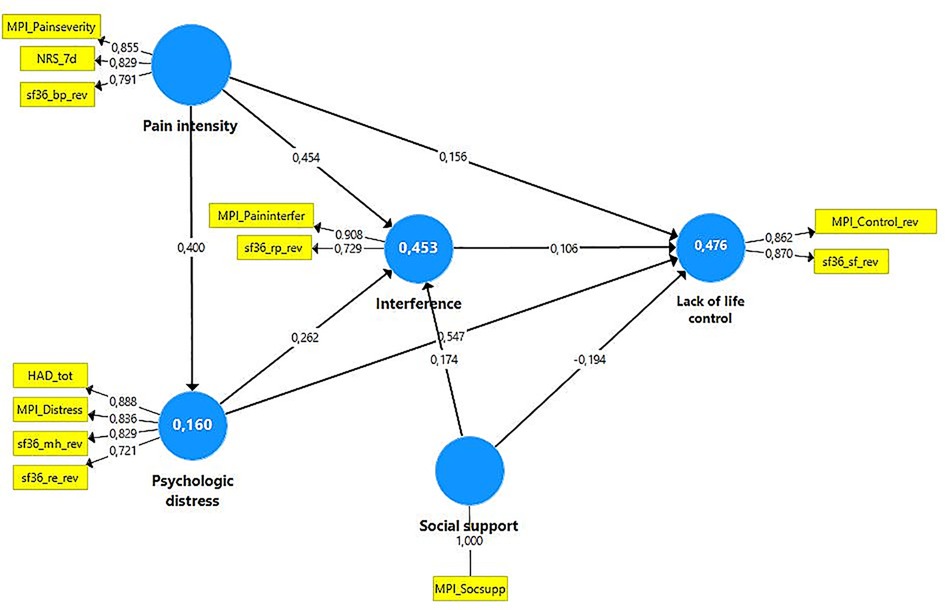
Figure 3. Model 2 for all subjects (N = 40,184). That is, if pain intensity affects psychological distress, model 1 is identical including loadings and path coefficients except for the arrow between pain intensity and psychological distress and R2 for pain intensity then is 0.160. The blue circles show the constructs/latent variables. The yellow boxes show the indicators (reflective) for each construct/latent variable; the figure attached to the thin arrows show the loading. The thicker arrows show the suggested relations between the constructs, and the figure attached shows the path coefficient (direct effect). The explained variance (R2) is reported within the relevant constructs. Note that some of the MPI variables and all sf36 variables were revised (indicated with _rev in the variable name) so that high values indicated a troublesome/negative situation. NRS_7d, Pain intensity according to a numeric rating scale; HAD, The Hospital Anxiety and Depression Scale; HAD-tot, sum of the two subscales of HAD; MPI, Multidimensional Pain Inventory; MPI_Paininterfer, MPI Pain interference; MPI_Painseverity, MPI Pain severity; MPI_Socsupp, MPI Social support; sf36, The Short Form Health Survey; sf36_rp, sf36-role physical; sf36_bp, sf36-bodily pain; sf36_sf, sf36-social function; sf36_re, sf36-role emotional; sf36_mh, sf36-mental health.
3.4.2. Evaluation of the reflective measurement (outer) models
Indicator reliability was above the threshold of 0.708 for all indicators except sf36-physical function and sf36-role physical, but these had absolute values well above the level used for elimination (i.e., 0.40). Internal consistency reliability according to the exact (consistent) reliability coefficient was satisfactory for all latent variables as well as convergent validity according to average variance extracted (AVE) – i.e., both >0.50. The discriminant validity was satisfactory for all relevant latent variable combinations except for pain vs. interference according to HTMT. When sf36-physical function was excluded as an indicator of interference, all HTMT values were below 0.90 (i.e., indicating discriminant validity). In addition, the indicator reliability for all indicators was above the threshold of 0.708. Loadings are shown in Figure 3 and the other final characteristics are shown in Supplementary Table S3. The two models were identical with respect to indicator reliability, internal consistency reliability, convergent validity, and discriminant validity (Figure 3 and Supplementary Table S3). To conclude, the final outer model for the total cohort was associated with good indicator reliability, internal consistency reliability, convergent validity, and discriminant validity in both models.
3.4.3. Evaluation of the structural (inner) models
VIF values were below 2 (1.0–1.83) – i.e., model collinearity was not an issue in either of the two models. Thereafter, the path coefficients (direct effects) as well as total and indirect effects were determined (Tables 4A,B). All path coefficients (direct effects), total effects, and indirect effects were significant in both models (<0.001) (Table 4A). Effect sizes (f2) are shown in Table 5.
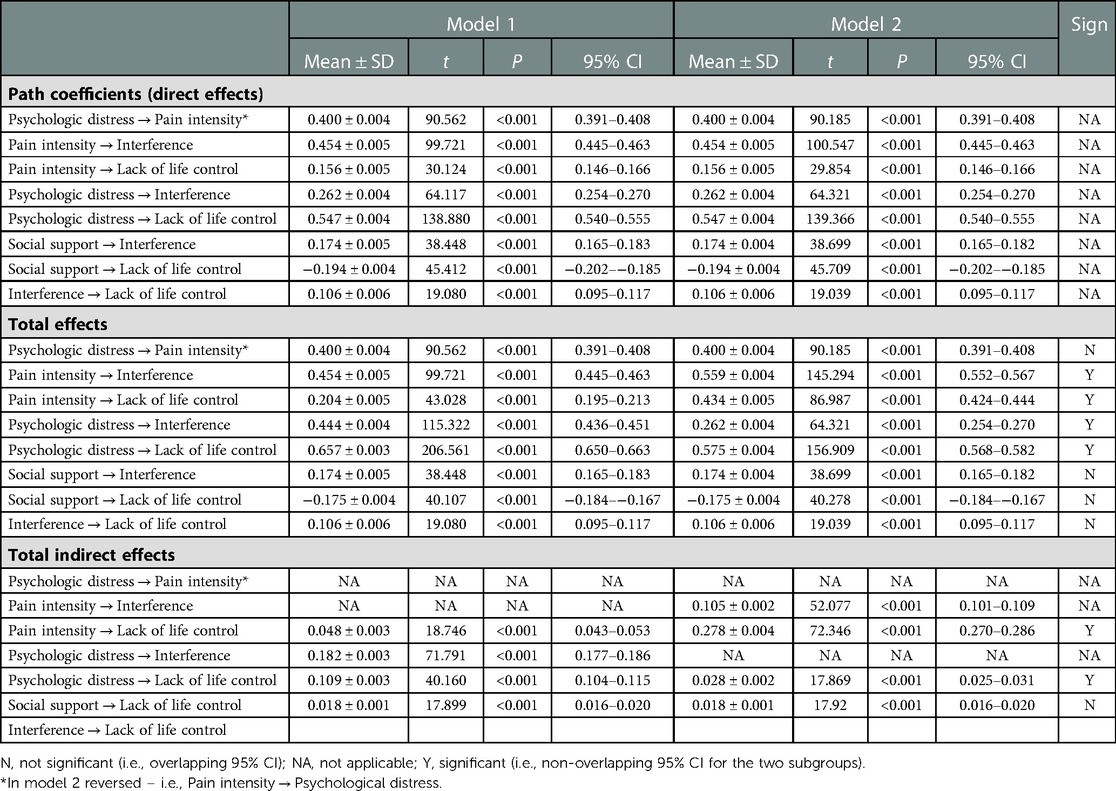
Table 4A. Path coefficients (direct effects), total and total indirect effects for the two models of the total cohort (N = 40,184). In model 1, psychological distress → Pain intensity and in model 2 the opposite (i.e., Pain intensity → Psychological distress). Mean ± SD, t-values, P-values, and 95% confidence intervals (95% CI) are reported.
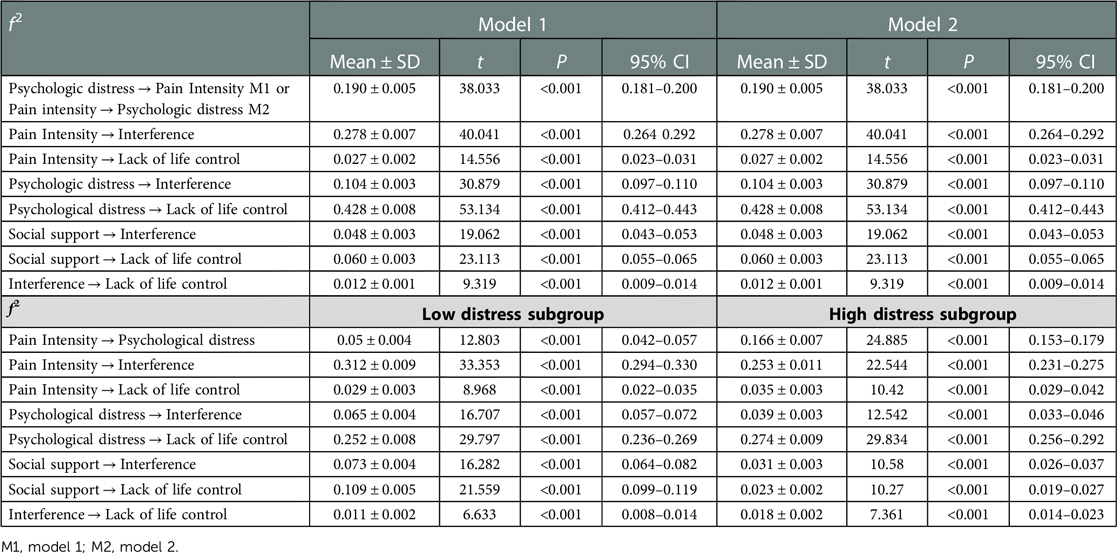
Table 4B. Specific indirect effects for the two models of the total cohort (N = 40,184). In model 1 psychological distress → Pain intensity and in model 2 the opposite (i.e., Pain intensity → Psychological distress). Mean ± SD, t-values, P-values, and 95% confidence intervals (95% CI) are reported.
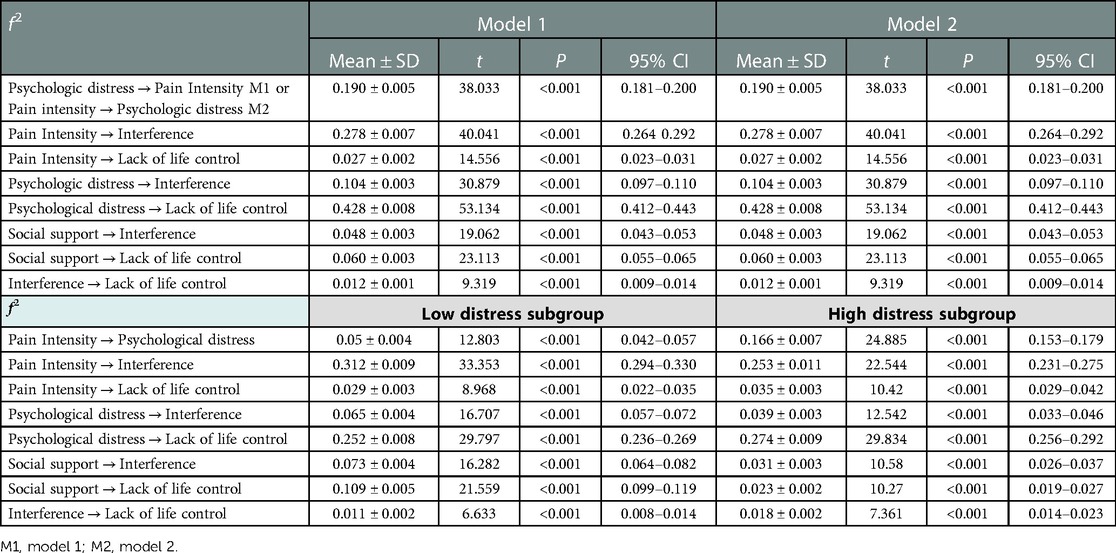
Table 5. F-square values (f2) for the total cohort (model 1 and 2) (N = 40,184) and for the two subgroups Low distress (N = 20,986) and high distress (N = 19,288). Mean ± SD, t-values, P-values, and 95% confidence intervals (95% CI) are reported.
3.4.3.1. Direct effects (path coefficients)
The path coefficients were identical in the two models (Table 4A and Figure 3). Psychological distress vs. Pain intensity (model 1) showed a relatively prominent direct path coefficient (and the reverse in model 2) (0.40; medium f2). Pain intensity had a relatively strong association with Interference (0.45; medium f2) and a weaker direct association (0.16; small f2) with Lack of life control. Psychological distress had a stronger direct association with Lack of life control (0.55; large f2) than with Interference (0.26; small f2). Interference vs. Lack of life control had a small path coefficient (0.11; no measurable effect). Social support had a positive path coefficient with Interference (0.17; small f2) and a negative (−0.19; small f2) with Lack of life control.
3.4.3.2. Total effects
To some extent, total effects differed between the two models (Tables 4A,B). In both models, the total effects for Pain intensity were stronger on Interference than on Lack of life control (Table 4A). These total effects for Pain intensity were stronger in model 2 than in model 1. In both models, Psychological distress showed stronger total effects on Lack of life control than for Interference (Table 4A). These total effects for Psychological distress on Lack of life control and Interference were stronger in model 1 than in model 2.
The total effects of Pain intensity and Psychological distress on Interference were similar in model 1 (0.45 vs. 0.44) (Table 4A). However, prominent differences existed in model 2; corresponding effects were 0.56 and 0.26 (Table 4A). The total effects of Pain intensity and Psychological distress on Lack of life control showed prominent differences (0.20 vs. 0.66) in model 1. A similar pattern but less pronounced was noted for model 2 (0.43 vs. 0.58).
3.4.3.3. Indirect effects – mediations
In both models, all possible indirect effects/mediating effects were significant (complementary mediations) but dependent on the model (Table 4A). In model 1, Pain intensity is a mediating construct between Psychological distress and Interference (indirect specific effect = 0.18) and between Psychological distress and Lack of life control (indirect specific effect = 0.06). The other indirect/mediating effects in model 1 were significant but small (i.e., indirect specific effects <0.05). In model 2, Psychological distress was a mediating construct between Pain intensity and Interference (indirect specific effect = 0.11) and between Pain intensity and Lack of life control (indirect specific effect = 0.22). The other indirect/mediating effects in model 2 were significant but small (i.e., indirect specific effects <0.05).
3.4.3.4. Explanatory and predictive powers
In both models, the coefficient of determination (R2) was higher for Lack of life control (R2 = 0.48) and Interference (R2 = 0.45) than for Pain intensity (model 1)/Psychological distress (model 2) (R2 = 0.16). The Predictive power analyses indicated that the two models had predictive relevance (Supplementary Table S5).
3.5. PLS-SEM in two subgroups with low and high psychological distress
3.5.1. Descriptive data for two subgroups
To investigate whether psychological distress was a moderating factor, we divided the total cohort into two subgroups. One subgroup, labelled High distress subgroup, had signs of definite depression and/or anxiety according to subscales of HAD (i.e., ≥11). The other subgroup, labelled Low distress subgroup, had lower signs of depression and anxiety according to HAD (i.e., <11). Descriptive data for all variables of both subgroups are shown in Table 3. All variables except sex/gender proportions differed significantly between the two subgroups. The High distress subgroup was born outside Europe to a larger extent and had university education to a lesser degree (Table 3). The Low distress subgroup reported a better situation on all clinical variables. The largest ESs were noted for the psychological distress variables (>0.90). The other variables had lower ESs. For example, pain intensity variables were associated with small to medium ESs.
3.5.2. Evaluation of the reflective measurement (outer) models
Separate PLS-SEM analysis were conducted for the two subgroups using the same latent variables, the same indicators, and the same causal patterns as for model 2 of the total cohort (Figures 4, 5). The outer model of both subgroups was associated with good indicator reliability, internal consistency reliability, convergent validity, and discriminant validity. The outer model characteristics are shown in Supplementary Table S4. Indicator loadings for each construct/latent variable are shown in Figures 4, 5. Indicators with loadings >0.40 and <0.708 were not omitted since it did not result in increased internal consistency or convergent validity.
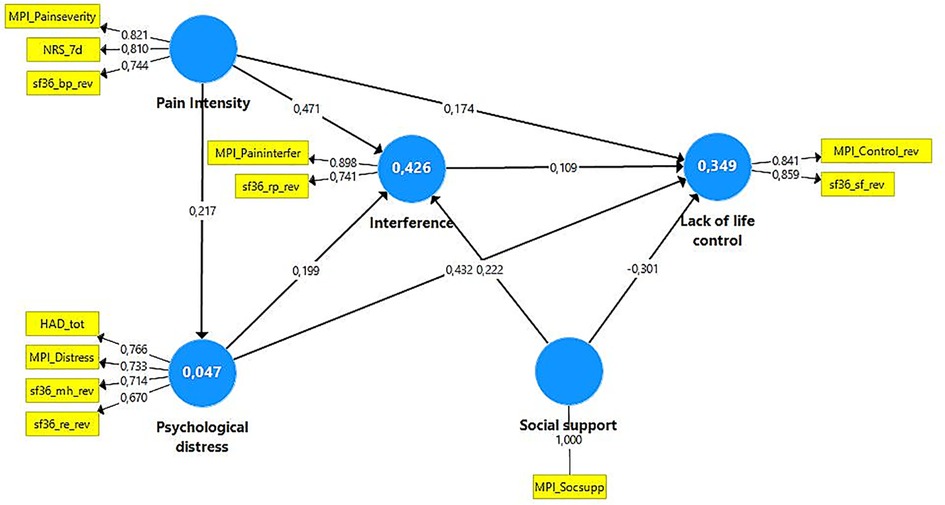
Figure 4. Model 2 (pain intensity affects psychological distress) for the subgroup with low distress (N = 20,986). The blue circles show the constructs. The yellow boxes show the indicators (reflective) for each latent variable; the figure attached to the thin arrows show the loading. The thicker arrows show the suggested relations between the constructs. The figure attached shows the path coefficient (direct effect). The explained variance (R2) is reported within the relevant constructs. Note that some of the MPI variables and all sf36 variables were revised (indicated with _rev in the variable name) so that high values indicated a troublesome/negative situation. NRS_7d, Pain intensity according to a numeric rating scale; HAD, The Hospital Anxiety and Depression Scale; HAD-tot, sum of the two subscales of HAD; MPI, Multidimensional Pain Inventory; MPI_Paininterfer, MPI Pain interference; MPI_Painseverity, MPI Pain severity; MPI_Socsupp, MPI Social support; sf36, The Short Form Health Survey; sf36_rp, sf36-role physical; sf36_bp, sf36-bodily pain; sf36_sf, sf36-social function; sf36_re, sf36-role emotional; sf36_mh, sf36-mental health.
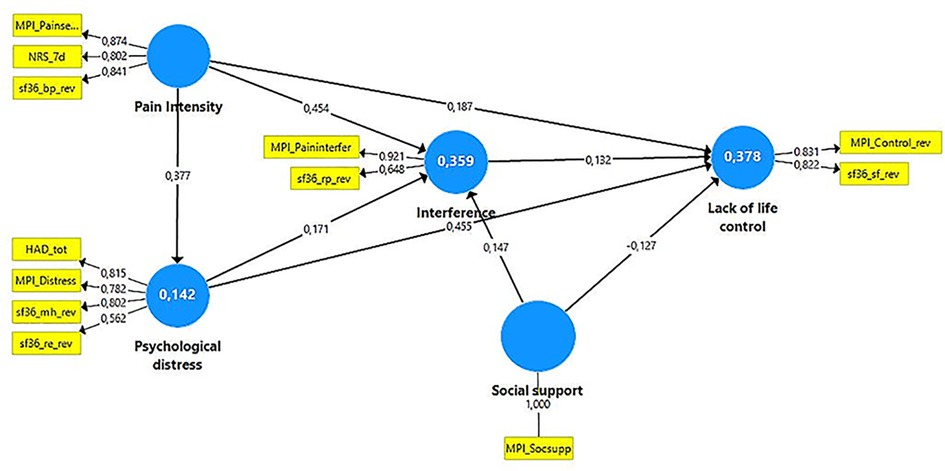
Figure 5. Model 2 (pain intensity affects psychological distress) for the subgroup with high distress (N = 19,288). The blue circles show the constructs. The yellow boxes show the indicators (reflective) for each latent variable; the figure attached to the thin arrows show the loading. The thicker arrows show the suggested relations between the constructs. The figure attached shows the path coefficient (direct effect). The explained variance (R2) is reported within the relevant constructs. Note that some of the MPI variables and all sf36 variables were revised (indicated with _rev in the variable name) so that high values indicated a troublesome/negative situation. NRS_7d, Pain intensity according to a numeric rating scale; HAD, The Hospital Anxiety and Depression Scale; HAD-tot, sum of the two subscales of HAD; MPI, Multidimensional Pain Inventory; MPI_Paininterfer, MPI Pain interference; MPI_Painseverity, MPI Pain severity; MPI_Socsupp, MPI Social support; sf36, The Short Form Health Survey; sf36_rp, sf36-role physical; sf36_bp, sf36-bodily pain; sf36_sf, sf36-social function; sf36_re, sf36-role emotional; sf36_mh, sf36-mental health.
3.5.3. Evaluation of the structural (inner) models
Collinearity was not an issue in the two subgroups. VIF values were below 2 in both subgroups (Low distress subgroup: 1.00–1.74; High distress subgroup: 1.00–1.60).
The direct effects (path coefficients) (Figures 4, 5), total effects, and indirect effects were all significant (all P < 0.001) in the two subgroups (Table 6).
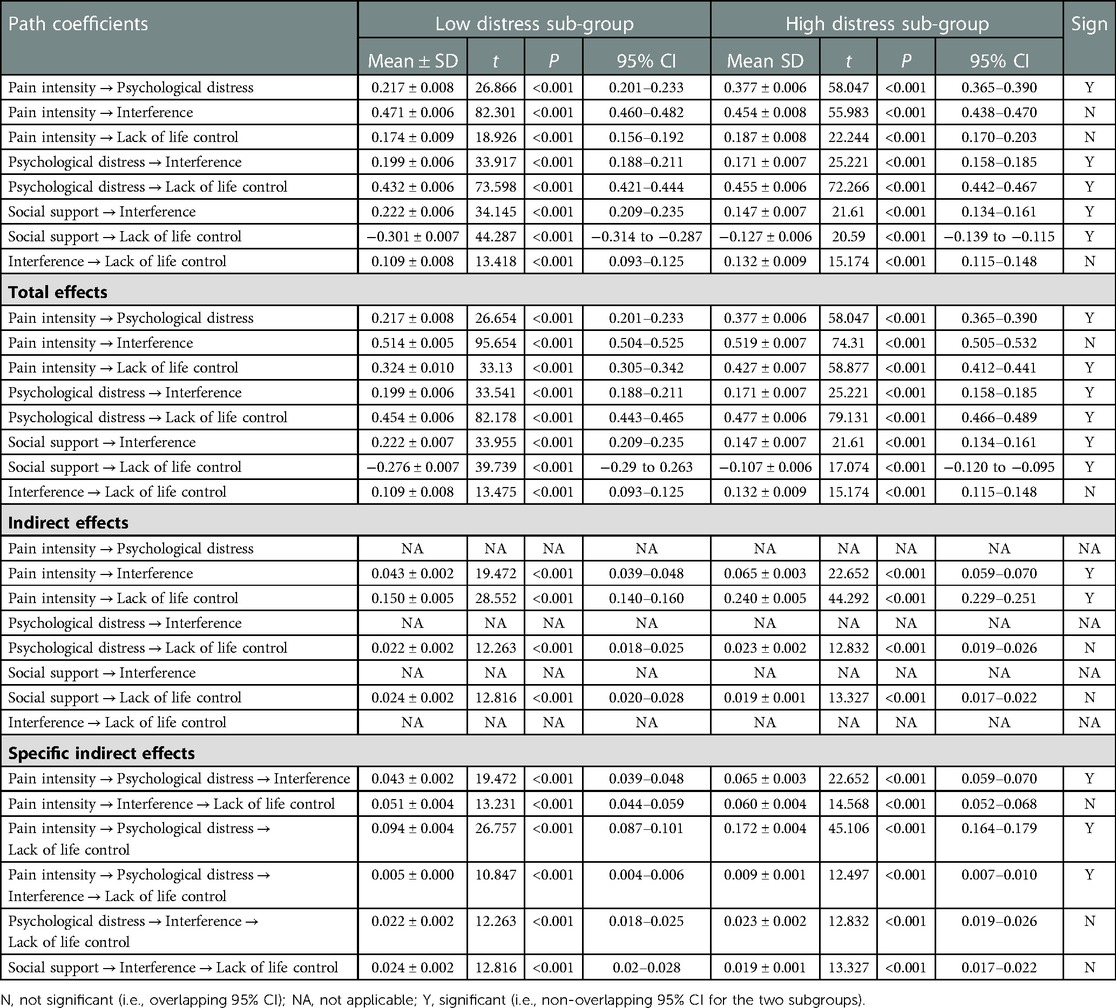
Table 6. Path coefficients, total effects, indirect, and specific indirect effects for the Low distress (N = 20,986) and high distress (N = 19,288) subgroups. Mean ± SD, t-values, P-values, and 95% confidence intervals (95% CI) are reported.
3.5.3.1. Direct effects (path coefficients)
Scrutinizing the 95% CIs for the coefficients pairwise between the two subgroups, we found some obvious significant differences in directs effects (path coefficients) between the two subgroups (Table 6, Figures 4, 5). The direct effect (path coefficient) between Pain intensity and Psychological distress was significantly stronger in the High distress group than in the Low distress group; 0.38 (medium f2) vs. 0.22 (small f2). Pain intensity vs. Interference had relatively high direct effects in both subgroups but did not differ significantly between the two subgroups (0.47 vs. 0.45; both medium f2). No significant differences were noted for the direct effects of Pain intensity vs. Lack of life control between the High distress subgroup and Low distress subgroup (0.19 vs. 0.17; both small f2). Psychological distress vs. Interference had a somewhat stronger direct effect in the Low distress group than in the High distress group (0.20 vs. 0.17; both small f2). Relatively high direct effects were noted for the Psychological distress - Lack of life control relationship in the Low distress and High distress subgroups (0.43 vs. 0.46; both medium f2). Stronger direct effects in the Low distress subgroup than in the High distress subgroup were noted for the relations between Social support and Interference and Lack of life control, respectively (all groups with small f2). No significant subgroup difference existed in the direct effects for Interference vs. Lack of life control (Low distress: 0.11 vs. High distress: 0.13; both with no measurable effect).
3.5.3.2. Total effects
The total effect of Pain intensity vs. Interference did not differ significantly between the two subgroups (Table 6). The total effect of Pain intensity on Lack of life control differed significantly between the two subgroups and was strongest in the High distress subgroup (0.43 vs. 0.32). The total effect for Psychological distress vs. Interference was significantly weaker in the High distress group than the Low distress group (0.17 vs. 0.20). In the High distress subgroup, the total effect for the Psychological distress-Lack of life control relationship was somewhat (significantly) higher than in the Low distress subgroup (0.48 vs. 0.45) (Table 6). Social support had weaker absolute total effects in the High distress subgroup than in the Low distress group (Social support-Interference relation: 0.15 vs. 0.22; Social support-Lack of life control relation: −0.11 vs. −0.28).
3.5.3.3. Indirect effects – mediations
In the Low distress group, Psychological distress was a mediating construct between Pain intensity and Lack of life control (indirect specific effect = 0.09). Furthermore, Interference was a mediating construct between pain Intensity and Lack of life control (indirect specific effect = 0.05). In the High distress subgroup, Psychological distress was a mediating construct between Pain intensity and Lack of life control (indirect specific effect = 0.17) and between Pain intensity and Interference (indirect specific effect = 0.07). In addition, Interference was a mediating construct between Pain intensity and Lack of life control (indirect specific effect = 0.06). Hence, the mediating effects were stronger for these three relationships in the High distress subgroup.
3.5.3.4. Explanatory powers and predictive powers
The coefficients of determination R2 for Psychological distress and Interference differed significantly between the two subgroups (Figures 4, 5). Hence, R2 of psychological distress was lower in the Low distress group (R2 = 0.047 ± 0.004, P < 0.001, 95% CI: 0.041–0.054) than in the High distress group (R2 = 0.142 ± 0.005 (P < 0.001, 95% CI: 0.133–0.152). A significantly higher R2 for Interference was found in the Low distress subgroup (R2 = 0.426 ± 0.006, P < 0.001, 95% CI: 0.415–0.437) compared to the High distress group (R2 = 0.359 ± 0.007, P < 0.001, 95% CI: 0.345–0.373). The explained variances for Lack of life control did not differ significantly between the low distress group (R2 = 0.349 ± 0.006, P < 0.001, 95% CI: 0.337–0.360) and the high distress group (R2 = 0.378 ± 0.006, P < 0.001, 95%CI: 0336–0.391). Q2predictive values indicated that the models of the two subgroups had predictive relevance except for sf36-mh-rev in the Low distress subgroup (Supplementary Table S5).
4. Discussion
4.1. Major results
Modern clinical pain management relies on the biopsychosocial model of pain. The model is usually presented graphically in a relatively simple way (74–76). However, associated explanations of the model suggest significant complexity between the constituents of the three subcomponents. But these relationships are only partially known. PLS-SEM can contribute to the understanding of these relationships. Hence, this real-life study of more than 40,000 patients investigated to what extent and how Pain intensity, Psychological distress, and Social support interact with Interference and Lack of life control aspects.
Although high prevalence of psychological distress was found in the cohort, relatively low correlation and explanatory power existed for the Pain intensity - Psychological distress relationship. Pain intensity had stronger effect on Interference than on Lack of life control, whereas Psychological distress had stronger effect on Lack of life control than on Interference. Depending on the underlying assumption concerning the Pain intensity - Psychological distress relationship, Pain intensity and Psychological distress could complementarily mediate each other in relation to the two impact constructs (Interference and Lack of life control). Social support showed very similar absolute correlations with Interference and Lack of life control. Interference and Lack of life control showed relatively weak associations. Psychological distress level was a moderating factor for several of the significant and important associations/paths.
4.2. Pain intensity-psychological distress relationships
Chronic pain conditions are associated with high prevalence of anxiety and depressive symptoms (77–80). This does not necessarily mean that high correlations exist between pain intensity and psychological distress levels. The present and other studies have shown that the intercorrelations between pain intensity and psychological distress levels are relatively low (24, 81, 82) – 16% of the variance explained (R2) in this study. As noted above, pain intensity and psychological distress are intercorrelated both in cross sectional and longitudinal studies. Also, day-to-day pain variability studies generally indicate reciprocal associations with negative affect factors (83, 84). Closely related to day-to-day fluctuations are the concepts of trait and state, which are relevant both for pain intensity and psychological distress (85–87). Several models including pain and psychological distress relationships (e.g., the misdirected problem-solving model and the fear avoidance model) suggest mediating factors between these two constructs (7, 88, 89). Cohort heterogeneity for the relationships between pain and mood aspects further complicates the understanding of pain intensity and psychological distress interactions both in short- and long-term perspectives (91, 94). Future studies of mediating (transdiagnostic) factors (e.g., attention, cognition, coping strategies, and emotion regulation) for pain intensity and psychological distress may shed further light on their intricate relationship even though understanding causal directions may be problematic.
4.3. Pain intensity and psychological distress vs. Interference and Lack of life control
As expected in the literature, we observed that both Pain intensity and Psychological distress were positively and significantly associated with the Interference and Lack of life control constructs (24, 81) (Figure 3). Relatively similar explanatory power (R2) was found for Interference and Lack of life control (0.45 vs. 0.48) in the total cohort (Figure 3). Thus, also other factors than Pain intensity, Psychological distress, and Social support contribute to the explanatory power of these constructs.
For both models of the total cohort, Pain intensity had stronger total effects on Interference than on Lack of life control, whereas the reverse was found for Psychological distress vs. Interference and Lack of life control (Table 4A). These results are consistent with results from another study of this cohort using other methods. The study found that pain intensity variables were more important for interference than for depressive symptoms, whereas life control was more strongly associated with symptoms of anxiety and depression (38). In our network study, anxiety and depression levels had stronger relationships than pain intensity with life control aspects (24).
The relative importance of Pain intensity and Psychological distress for the two impact constructs depend on the underlying assumption concerning their interrelationship. Hence, model differences were also obvious. For instance, the total effects of Pain intensity vs. both Interference and Lack of life control were markedly larger in model 2 than in model 1 (Table 4A). Psychological distress had relatively weaker total effects on Interference in model 2 than in model 1 (Table 4A). Differences also existed across the two models when comparing the relative contributions of Pain intensity and Psychological distress for the explanation of Interference and Lack of life control. For example, Pain intensity and Psychological distress in model 1 had very similar total effects on Interference, whereas Pain intensity in model 2 showed a significantly stronger total effect than psychological distress on Interference (0.56 vs. 0.26). In addition, the indirect (mediating) effects were significant and model dependent (Tables 4A,B). Psychological stress and Pain intensity can complementarily mediate each other in relation to Interference and Lack of life control (Tables 4A,B).
The reports of heterogeneity for the causal directions between pain intensity and psychological distress must reasonably have clinical implications (91–94). Thus, the clinical management must take this heterogeneity into account when designing individual treatment interventions.
4.4. Interference and Lack of life control relationship
Interference and Lack of life control showed relatively weak positive correlations, which suggests that they are different constructs of the umbrella construct of life impact. Clinically, they should be assessed separately. Although several large studies from the SQRP, including this one, have focused on factors that intercorrelate with these two separate constructs of life impact, more studies from large real-life cohorts that can identify variables with significant associations with interference and lack of life control are desirable (12, 24, 38). One possible limitation is that we chose indicators from different instruments – e.g., MPI and sf36 – to cover the two constructs. However, the instruments and their subscales are well established in pain research.
4.5. Social support showed very similar correlations with Interference and Lack of life control
Chronic pain increases the risk for social isolation, whereas social support can improve coping, management of treatments, and faster recovery of the pain condition (40, 95). Ferreira-Valente et al. reported negative associations between social support aspects and pain interference (48). A cross-sectional analysis of social isolation, which can be viewed as a negative aspect of social support, showed significant correlations both with pain interference (positive association) and physical function (negative association) (49). As in these studies, we noted that Social support was associated with decreased levels of Lack of life control (i.e., a negative association). Paradoxically, Social support correlated positively with Interference. We have no definite explanation for this counterintuitive finding. It could be due to lack of a mediating factor in our theoretical model. Hence, a recent study investigated social isolation in relation to physical function and identified depression as a mediating factor (49).
4.6. Psychological distress level as a moderator
The presence or non-presence of severe psychological distress according to HAD was a moderating factor. Thus, several intercorrelations between the constructs/latent variables depend on whether definite signs of anxiety and/or depression are present. Specifically, Pain intensity, Psychological distress, and Social support affect Interference and Lack of life control with different strengths in the two subgroups. For example, Pain intensity and Psychological distress were more strongly intercorrelated in the High distress subgroup than in the Low distress subgroup (Figures 4, 5). Moreover, Psychological distress showed significantly stronger direct effects on Lack of life control but weaker direct effects on Interference in the High distress subgroup compared to the Low distress subgroup. In addition, Interference was associated with lower coefficients of determination (R2) in the High distress subgroup.
Such heterogeneity was also noted for the effects of Social support on Interference and Lack of life control (Figures 4, 5). Hence, the buffering effect of Social support is different and, in some aspects, diminished in the most severe chronic pain conditions, which might be interpreted as social support being of greater value for the ability to be physically active and lead meaningful social lives in chronic patients with a lesser degree of psychological co-morbidity. We also found that the three most important complementary mediating effects were stronger in the High distress subgroup.
Our results taken together indicate cohort heterogeneity and emphasise the need to subgroup patients with complex chronic pain conditions. In the literature there are several studies identifying subgroups [for references see (12)]. There is no agreement concerning the important input variables for such subgroupings; most studies have been hypothesis driven. This study as well as other studies indicate that psychological variables are important (96–101). There is a need for data driven methods in large cohorts of chronic pain patients for identifying the relevant input variables (12, 38). Studies using such methods also identify pain intensity aspects as important for the heterogeneity. Furthermore, studies including from SQRP have found that subgroups show different outcomes of IPRP (12, 51, 53). The different treatment outcomes could partially be due to the presence of moderating effects from definite anxiety and/or depression as well as from other currently unknown variables. Future research needs to focus more on identifying moderating factors. Covering such a knowledge gap may be important for improving outcomes of rehabilitation interventions.
4.7. Strengths and limitations
A strength is the large number of chronic pain patients with nation-wide representation. Our results are relevant for patients referred to specialist care. Women are overrepresented in specialist clinics in Sweden compared to the community prevalence (12, 96). In an earlier large SQRP study we could not confirm reports that women assessed at specialist clinics have a more severe clinical situation than men or are judged to be more prone to behavioral change (50, 102). The reasons for this skewed assortment/selection are unclear and need to be addressed. All constructs except Social support had several indicators, which were scales of well-established instruments. This is an advantage from a measurement error point of view compared to path analyses, which only use single items or scales representing one aspect/construct. A limitation is that our theoretical model and subsequent analyses is based on cross-sectional data. For example, it was not possible to determine the most common causal relationship between pain intensity and psychological distress, which is a disadvantage of our models. Our construct Interference covers pain interference and physical function. These aspects are often used interchangeably, but recent reports suggest that it may be necessary to differentiate these measures in, for example, longitudinal perspectives (47, 103).
5. Conclusions
The results are important both for the assessments and the design of treatments for patients with chronic pain. In this large real-life study of more than 40,000 patients, a relatively low correlation for the Pain intensity - Psychological distress relationship was found. A clinical treatment consequence may be that lowering one of them may not result in lowering the other. Similarly, Interference and Lack of life control showed relatively weak associations, which emphasises the need to clinically assess them separately. Pain intensity had a stronger effect on Interference than on Lack of life control, whereas Psychological distress had a stronger effect on Lack of life control than on Interference. Thus, it seems that anxiety and depression impair function and the ability to lead socially meaningful lives to a greater extent, whereas higher pain levels have a more detrimental effect on physical functioning in chronic pain states. The relative strengths of Pain intensity and Psychological distress on the two impact constructs depend on the underlying assumption concerning the Pain intensity-Psychological distress relationship. Social support influenced both impact constructs investigated although to a greater extent in individuals with chronic pain who had lower clinical severity – i.e., a definite sign (or lack of sign) of anxiety and/or depression was a moderating factor.
Data availability statement
The raw data supporting the conclusions of this article will be made available by the authors, without undue reservation.
Ethics statement
The studies involving human participants were reviewed and approved by Ethical Review Board in Linköping (Dnr: 2015/108-31). The patients/participants provided their written informed consent to participate in this study.
Author contributions
Conceptualization, ÅR, ED, MRF and BG; Data curation, BG; Formal analysis, BG and ED; Methodology, ÅR, ED, MRF and BG; Writing – original draft, BG; Writing – review & editing, ÅR, ED, MRF and BG. All authors contributed to the article and approved the submitted version.
Conflict of interest
The authors declare that the research was conducted in the absence of any commercial or financial relationships that could be construed as a potential conflict of interest.
Publisher's note
All claims expressed in this article are solely those of the authors and do not necessarily represent those of their affiliated organizations, or those of the publisher, the editors and the reviewers. Any product that may be evaluated in this article, or claim that may be made by its manufacturer, is not guaranteed or endorsed by the publisher.
Supplementary material
The Supplementary Material for this article can be found online at: https://www.frontiersin.org/articles/10.3389/fpain.2023.1093002/full#supplementary-material.
Footnote
1Throughout the text, we capitalize the initial letter of constructs/latent variables – e.g., Interference and Lack of life control.
References
1. Turk DC, Dworkin RH, Revicki D, Harding G, Burke LB, Cella D, et al. Identifying important outcome domains for chronic pain clinical trials: an IMMPACT survey of people with pain. Pain. (2008) 137(2):276–85. doi: 10.1016/j.pain.2007.09.002
2. Casarett D, Karlawish J, Sankar P, Hirschman K, Asch DA. Designing pain research from the patient's perspective: what trial end points are important to patients with chronic pain? Pain Med. (2001) 2(4):309–16. doi: 10.1046/j.1526-4637.2001.01041.x
3. Robinson ME, Brown JL, George SZ, Edwards PS, Atchison JW, Hirsh AT, et al. Multidimensional success criteria and expectations for treatment of chronic pain: the patient perspective. Pain Med. (2005) 6(5):336–45. doi: 10.1111/j.1526-4637.2005.00059.x
4. Brown JL, Edwards PS, Atchison JW, Lafayette-Lucey A, Wittmer VT, Robinson ME. Defining patient-centered, multidimensional success criteria for treatment of chronic spine pain. Pain Med. (2008) 9(7):851–62. doi: 10.1111/j.1526-4637.2007.00357.x
5. Merrick D, Sundelin G, Stålnacke BM. One-Year follow-up of two different rehabilitation strategies for patients with chronic pain. J Rehabil Med. (2012) 44(9):764–73. doi: 10.2340/16501977-1022
6. Vos T, Flaxman AD, Naghavi M, Lozano R, Michaud C, Ezzati M, et al. Years lived with disability (YLDs) for 1160 sequelae of 289 diseases and injuries 1990-2010: a systematic analysis for the global burden of disease study 2010. Lancet. (2012) 380(9859):2163–96. doi: 10.1016/S0140-6736(12)61729-2
7. Linton S, Bergbom S. Understanding the link between depression and pain. Scand J Pain. (2011) 2:47–54. doi: 10.1016/j.sjpain.2011.01.005
8. Ossipov MH, Dussor GO, Porreca F. Central modulation of pain. J Clin Invest. (2010) 120(11):3779–87. doi: 10.1172/JCI43766
9. Gatchel R, Peng Y, Peters M, Fuchs P, Turk D. The biopsychosocial approach to chronic pain: scientific advances and future directions. Psychol Bull. (2007) 133:581–624. doi: 10.1037/0033-2909.133.4.581
11. Dworkin RH, Turk DC, Farrar JT, Haythornthwaite JA, Jensen MP, Katz NP, et al. Core outcome measures for chronic pain clinical trials: iMMPACT recommendations. Pain. (2005) 113(1–2):9–19. doi: 10.1016/j.pain.2004.09.012
12. Gerdle B, Akerblom S, Brodda Jansen G, Enthoven P, Ernberg M, Dong HJ, et al. Who benefits from multimodal rehabilitation - an exploration of pain, psychological distress, and life impacts in over 35,000 chronic pain patients identified in the Swedish quality registry for pain rehabilitation. J Pain Res. (2019) 12:891–908. doi: 10.2147/JPR.S190003
13. Hooten W. Chronic pain and mental health disorders: shared neural mechanisms, epidemiology, and treatment. Mayo Clin Proc. (2016) 91:955–70. doi: 10.1016/j.mayocp.2016.04.029
14. Armbrecht E, Shah A, Schepman P, Shah R, Pappadopulos E, Chambers R, et al. Economic and humanistic burden associated with noncommunicable diseases among adults with depression and anxiety in the United States. J Med Econ. (2020) 23(9):1032–42. doi: 10.1080/13696998.2020.1776297
15. Bair MJ, Robinson RL, Katon W, Kroenke K. Depression and pain comorbidity: a literature review. Arch Intern Med. (2003) 163(20):2433–45. doi: 10.1001/archinte.163.20.2433
16. Fishbain DA, Cutler R, Rosomoff HL, Rosomoff RS. Chronic pain-associated depression: antecedent or consequence of chronic pain? A review. Clin J Pain. (1997) 13(2):116–37. doi: 10.1097/00002508-199706000-00006
17. May A. Chronic pain may change the structure of the brain. Pain. (2008) 137(1):7–15. doi: 10.1016/j.pain.2008.02.034
18. Romano JM, Turner JA. Chronic pain and depression: does the evidence support a relationship? Psychol Bull. (1985) 97(1):18–34. doi: 10.1037/0033-2909.97.1.18
19. Vlaeyen JW, Linton SJ. Fear-avoidance model of chronic musculoskeletal pain: 12 years on. Pain. (2012) 153(6):1144–7. doi: 10.1016/j.pain.2011.12.009
20. Stubbs B, Koyanagi A, Thompson T, Veronese N, Carvalho AF, Solomi M, et al. The epidemiology of back pain and its relationship with depression, psychosis, anxiety, sleep disturbances, and stress sensitivity: data from 43 low- and middle-income countries. Gen Hosp Psychiatry. (2016) 43:63–70. doi: 10.1016/j.genhosppsych.2016.09.008
21. Bisgaard TH, Allin KH, Keefer L, Ananthakrishnan AN, Jess T. Depression and anxiety in inflammatory bowel disease: epidemiology, mechanisms and treatment. Nat Rev Gastroenterol Hepatol. (2022) 19:717–26. doi: 10.1038/s41575-022-00634-6
22. Angst F, Benz T, Lehmann S, Wagner S, Simmen BR, Sandor PS, et al. Extended overview of the longitudinal pain-depression association: a comparison of six cohorts treated for specific chronic pain conditions. J Affect Disord. (2020) 273:508–16. doi: 10.1016/j.jad.2020.05.044
23. Qiu Y, Ma Y, Huang X. Bidirectional relationship between body pain and depressive symptoms: a pooled analysis of two national aging cohort studies. Front Psychiatry. (2022) 13:881779. doi: 10.3389/fpsyt.2022.881779
24. Akerblom S, Cervin M, Perrin S, Rivano Fischer M, Gerdle B, McCracken LM. A network analysis of clinical variables in chronic pain: a study from the Swedish quality registry for pain rehabilitation (SQRP). Pain Med. (2021) 22(7):1591–602. doi: 10.1093/pm/pnaa473
25. Ruiz-Párraga GT, López-Martínez AE. The contribution of posttraumatic stress symptoms to chronic pain adjustment. Health Psychol. (2014) 33(9):958–67. doi: 10.1037/hea0000040
26. Linton SJ, Nicholas MK, Macdonald S, Boersma K, Bergbom S, Maher C, et al. The role of depression and catastrophizing in musculoskeletal pain. Eur J Pain. (2011) 15(4):416–22. doi: 10.1016/j.ejpain.2010.08.009
27. Reme S, Shaw W, Steenstra I, Woiszwillo M, Pransky G, Distressed LS. Immobilized, or lacking employer support? A sub-classification of acute work-related low back pain. J Occup Rehabil. (2012) 22(4):541. doi: 10.1007/s10926-012-9370-4
28. Salazar A, Dueñas M, Mico JA, Ojeda B, Agüera-Ortiz L, Cervilla JA, et al. Musculoskeletal section: undiagnosed mood disorders and sleep disturbances in primary care patients with chronic musculoskeletal pain. Pain Med. (2013) 14:1416–25. doi: 10.1111/pme.12165
29. Outcalt S, Kroenke K, Krebs E, Chumbler N, Wu J, Yu Z, et al. Chronic pain and comorbid mental health conditions: independent associations of posttraumatic stress disorder and depression with pain, disability, and quality of life. J Behav Med. (2015) 38(3):535–43. doi: 10.1007/s10865-015-9628-3
30. Rayner L, Hotopf M, Petkova H, Matcham F, Simpson A, McCracken LM. Depression in patients with chronic pain attending a specialised pain treatment centre: prevalence and impact on health care costs. Pain. (2016) 157(7):1472–9. doi: 10.1097/j.pain.0000000000000542
31. Sullivan MJ, Adams H, Tripp D, Stanish WD. Stage of chronicity and treatment response in patients with musculoskeletal injuries and concurrent symptoms of depression. Pain. (2008) 135(1–2):151–9. doi: 10.1016/j.pain.2007.05.021
32. Tunks ER, Crook J, Weir R. Epidemiology of chronic pain with psychological comorbidity: prevalence, risk, course, and prognosis. Can J Psychiatry. (2008) 53(4):224–34. doi: 10.1177/070674370805300403
33. Trompetter H, Bohlmeijer E, Lamers S, Schreurs K. Positive psychological wellbeing is required for online self-help acceptance and commitment therapy for chronic pain to be effective. Front Psychol. (2016) 7:353. doi: 10.3389/fpsyg.2016.00353
34. Turner J, Holtzman S, Mancl L. Mediators, moderators, and predictors of therapeutic change in cognitive-behavioral therapy for chronic pain. Pain. (2007) 127(3):276–86. doi: 10.1016/j.pain.2006.09.005
35. Bromley Milton M, Borsbo B, Rovner G, Lundgren-Nilsson A, Stibrant-Sunnerhagen K, Gerdle B. Is pain intensity really that important to assess in chronic pain patients? A study based on the Swedish quality registry for pain rehabilitation (SQRP). PloS One. (2013) 8(6):e65483. doi: 10.1371/journal.pone.0065483
36. Payne-Murphy JC, Beacham AO. Revisiting chronic pain patient profiling: an acceptance-based approach in an online sample. Clin Psychol Psychother. (2015) 22(3):240–8. doi: 10.1002/cpp.1886
37. Borsbo B, Peolsson M, Gerdle B. The complex interplay between pain intensity, depression, anxiety and catastrophising with respect to quality of life and disability. Disabil Rehabil. (2009) 31(19):1605–13. doi: 10.1080/09638280903110079
38. Gerdle B, Åkerblom S, Stålnacke B-M, Brodda Jansen G, Enthoven P, Ernberg M, et al. The importance of emotional distress, cognitive behavioural factors and pain for life impact at baseline and for outcomes after rehabilitation – a SQRP study of more than 20 000 chronic pain patients. Scand J Pain. (2019) 19(4):693–711. doi: 10.1515/sjpain-2019-0016
39. Fernandez-de-Las-Penas C, Palacios-Cena M, Valera-Calero JA, Cuadrado ML, Guerrero-Peral A, Pareja JA, et al. Understanding the interaction between clinical, emotional and psychophysical outcomes underlying tension-type headache: a network analysis approach. J Neurol. (2022) 269(8):4525–34. doi: 10.1007/s00415-022-11039-5
40. Ashton-James CE, Anderson SR, Mackey SC, Darnall BD. Beyond pain, distress, and disability: the importance of social outcomes in pain management research and practice. Pain. (2022) 163(3):e426–31. doi: 10.1097/j.pain.0000000000002404
41. Che X, Cash R, Ng SK, Fitzgerald P, Fitzgibbon BM. A systematic review of the processes underlying the main and the buffering effect of social support on the experience of pain. Clin J Pain. (2018) 34(11):1061–76. doi: 10.1097/AJP.0000000000000624
42. Jensen MP, Ehde DM, Hoffman AJ, Patterson DR, Czerniecki JM, Robinson LR. Cognitions, coping and social environment predict adjustment to phantom limb pain. Pain. (2002) 95(1–2):133–42. doi: 10.1016/S0304-3959(01)00390-6
43. Clauw DJ, Essex MN, Pitman V, Jones KD. Reframing chronic pain as a disease, not a symptom: rationale and implications for pain management. Postgrad Med. (2019) 131(3):185–98. doi: 10.1080/00325481.2019.1574403
44. Dhanju S, Kennedy SH, Abbey S, Katz J, Weinrib A, Clarke H, et al. The impact of comorbid pain and depression in the United States: results from a nationally representative survey. Scand J Pain. (2019) 19(2):319–25. doi: 10.1515/sjpain-2018-0323
45. Roughan WH, Campos AI, Garcia-Marin LM, Cuellar-Partida G, Lupton MK, Hickie IB, et al. Comorbid chronic pain and depression: shared risk factors and differential antidepressant effectiveness. Front Psychiatry. (2021) 12:643609. doi: 10.3389/fpsyt.2021.643609
46. Hair J Jr, Hult G, Ringle C, Sarstedt M. A primer on partial least squares structural equation modelling (PLS-SEM). 3rd ed. Los Angeles: Sage (2022).
47. Karayannis NV, Sturgeon JA, Chih-Kao M, Cooley C, Mackey SC. Pain interference and physical function demonstrate poor longitudinal association in people living with pain: a PROMIS investigation. Pain. (2017) 158(6):1063–8. doi: 10.1097/j.pain.0000000000000881
48. Ferreira-Valente MA, Pais-Ribeiro JL, Jensen MP. Associations between psychosocial factors and pain intensity, physical functioning, and psychological functioning in patients with chronic pain: a cross-cultural comparison. Clin J Pain. (2014) 30(8):713–23. doi: 10.1097/AJP.0000000000000027
49. Karayannis NV, Baumann I, Sturgeon JA, Melloh M, Mackey SC. The impact of social isolation on pain interference: a longitudinal study. Ann Behav Med. (2019) 53(1):65–74. doi: 10.1093/abm/kay017
50. Gerdle B, Boersma K, Asenlof P, Stalnacke BM, Larsson B, Ringqvist A. Influences of sex, education, and country of birth on clinical presentations and overall outcomes of interdisciplinary pain rehabilitation in chronic pain patients: a cohort study from the Swedish quality registry for pain rehabilitation (SQRP). J Clin Med. (2020) 9(8):2374. doi: 10.3390/jcm9082374
51. Gerdle B, Cervin M, Rivano Fischer M, Ringqvist A. Outcomes of interdisciplinary pain rehabilitation across subgroups of the multidimensional pain inventory - A study from the Swedish quality registry for pain rehabilitation. Pain Pract. (2021) 21(6):662–79. doi: 10.1111/papr.13007
52. Gerdle B, Rivano Fischer M, Cervin M, Ringqvist Å. Spreading of pain in patients with chronic pain is related to pain duration and clinical presentation and weakly associated with outcomes of interdisciplinary pain rehabilitation: a cohort study from the Swedish quality registry for pain rehabilitation (SQRP). J Pain Res. (2021) 14:173–87. doi: 10.2147/JPR.S288638
53. Ringqvist Å, Dragioti E, Björk M, Larsson B, Gerdle B. Moderate and stable pain reductions as a result of interdisciplinary pain rehabilitation – a cohort study from the Swedish quality registry for pain rehabilitation (SQRP). J Clin Med. (2019) 8:905. doi: 10.3390/jcm8060905
54. Turk DC, Dworkin RH, Allen RR, Bellamy N, Brandenburg N, Carr DB, et al. Core outcome domains for chronic pain clinical trials: iMMPACT recommendations. Pain. (2003) 106(3):337–45. doi: 10.1016/j.pain.2003.08.001
55. Kaiser U, Kopkow C, Deckert S, Neustadt K, Jacobi L, Cameron P, et al. Developing a core outcome-domain set to assessing effectiveness of interdisciplinary multimodal pain therapy: the VAPAIN consensus statement on core outcome-domains. Pain. (2018) 159(4):673–83. doi: 10.1097/j.pain.0000000000001129
56. Zigmond AS, Snaith RP. The hospital anxiety and depression scale. Acta Psychiatr Scand. (1983) 67(6):361–70. doi: 10.1111/j.1600-0447.1983.tb09716.x
57. Bjelland I, Dahl AA, Haug TT, Neckelmann D. The validity of the hospital anxiety and depression scale. An updated literature review. J Psychosom Res. (2002) 52(2):69–77. doi: 10.1016/S0022-3999(01)00296-3
58. LoMartire R, Ang BO, Gerdle B, Vixner L. Psychometric properties of short form-36 health survey, EuroQol 5-dimensions, and hospital anxiety and depression scale in patients with chronic pain. Pain. (2020) 161(1):83–95. doi: 10.1097/j.pain.0000000000001700
59. Bergstrom G, Jensen IB, Bodin L, Linton SJ, Nygren AL, Carlsson SG. Reliability and factor structure of the multidimensional pain inventory–Swedish language version (MPI-S). Pain. (1998) 75(1):101–10. doi: 10.1016/S0304-3959(97)00210-8
60. Bergstrom KG, Jensen IB, Linton SJ, Nygren AL. A psychometric evaluation of the Swedish version of the multidimensional pain inventory (MPI-S): a gender differentiated evaluation. Eur J Pain. (1999) 3(3):261–73. doi: 10.1016/S1090-3801(99)90053-8
61. EuroQol. Euroqol: a new facility for the measurement of health-related quality of life. Health Policy. (1990) 16:199–208. doi: 10.1016/0168-8510(90)90421-9
62. Brooks R. Euroqol: the current state of play. Health Policy. (1996) 37(1):53–72. doi: 10.1016/0168-8510(96)00822-6
63. Dolan P, Sutton M. Mapping visual analogue scale health state valuations onto standard gamble and time trade-off values. Soc Sci Med. (1997) 44(10):1519–30. doi: 10.1016/S0277-9536(96)00271-7
64. Sullivan M, Karlsson J, Ware J. The Swedish 36 health survey. Evaluation of data quality, scaling assumption, reliability and construct validity across general populations in Sweden. Soc Sci Med. (1995) 41:1349–58. doi: 10.1016/0277-9536(95)00125-Q
65. Hooker S. SF-36. In: Gellman M, Turner J, editors. Encyclopedia of behavioral medicine. New York, NY: Springer (2013). p. 1784–6.
66. Kerns RD, Turk DC, Rudy TE. The west haven-Yale multidimensional pain inventory (WHYMPI). Pain. (1985) 23(4):345–56. doi: 10.1016/0304-3959(85)90004-1
67. Ware J. SF36 Health survey - manual and intrepretation guide. Boston: the health insitute. New England Medical Center. (1993). P. 1–316
68. Tseli E, Stalnacke BM, Boersma K, Enthoven P, Gerdle B, Ang BO, et al. Prognostic factors for physical functioning after multidisciplinary rehabilitation in patients with chronic musculoskeletal pain: a systematic review and meta-analysis. Clin J Pain. (2019) 35(2):148–73. doi: 10.1097/AJP.0000000000000669
69. Bäckryd E, Persson EB, Larsson AI, Fischer MR, Gerdle B. Chronic pain patients can be classified into four groups: clustering-based discriminant analysis of psychometric data from 4665 patients referred to a multidisciplinary pain centre (a SQRP study). PLoS One. (2018) 13(2):e0192623. doi: 10.1371/journal.pone.0192623
70. van Ginkel JR, Linting M, Rippe RCA, van der Voort A. Rebutting existing misconceptions about multiple imputation as a method for handling missing data. J Pers Assess. (2020) 102(3):297–308. doi: 10.1080/00223891.2018.1530680
71. Wheelock AM, Wheelock CE. Trials and tribulations of ‘omics data analysis: assessing quality of SIMCA-based multivariate models using examples from pulmonary medicine. Mol Biosyst. (2013) 9(11):2589–96. doi: 10.1039/c3mb70194h
72. Eriksson L, Byrne T, Johansson E, Trygg J, Vikström C. Multi- and megavariate data analysis: Basic principles and applications. 3rd revised ed. Malmö: MKS Umetrics AB (2013).
73. Sheko A, Braimllari A. Information technology inhibitors and information quality in supply chain management: a PLS-SEM analysis. Acad J Interdiscip Stud. (2018) 7(3):125–38. doi: 10.2478/ajis-2018-0064
74. Fillingim RB. Individual differences in pain: understanding the mosaic that makes pain personal. Pain. (2017) 158(Suppl 1):S11–S8. doi: 10.1097/j.pain.0000000000000775
75. Cohen SP, Vase L, Hooten WM. Chronic pain: an update on burden, best practices, and new advances. Lancet. (2021) 397(10289):2082–97. doi: 10.1016/S0140-6736(21)00393-7
76. Rankin L. Chronic pain: from the study of student attitudes and preferences to the in vitro investigation of a novel treatment strategy. Umeå: Umeå University (2020).
77. Demyttenaere K, Bruffaerts R, Lee S, Posada-Villa J, Kovess V, Angermeyer MC, et al. Mental disorders among persons with chronic back or neck pain: results from the world mental health surveys. Pain. (2007) 129(3):332–42. doi: 10.1016/j.pain.2007.01.022
78. Pope CJ, Sharma V, Sharma S, Mazmanian D. A systematic review of the association between psychiatric disturbances and endometriosis. J Obstet Gynaecol Can. (2015) 37(11):1006–15. doi: 10.1016/s1701-2163(16)30050-0
79. Vincent A, Whipple MO, McAllister SJ, Aleman KM, St Sauver JL. A cross-sectional assessment of the prevalence of multiple chronic conditions and medication use in a sample of community-dwelling adults with fibromyalgia in olmsted county, Minnesota. BMJ Open. (2015) 5(3):e006681. doi: 10.1136/bmjopen-2014-006681
80. Gerrits MM, Vogelzangs N, van Oppen P, van Marwijk HW, van der Horst H, Penninx BW. Impact of pain on the course of depressive and anxiety disorders. Pain. (2012) 153(2):429–36. doi: 10.1016/j.pain.2011.11.001
81. Adams MH, Dobscha SK, Smith NX, Yarborough BJ, Deyo RA, Morasco BJ. Prevalence and correlates of low pain interference among patients with high pain intensity who are prescribed long-term opioid therapy. J Pain. (2018) 19(9):1074–81. doi: 10.1016/j.jpain.2018.04.005
82. Hadlandsmyth K, Sabic E, Zimmerman MB, Sluka KA, Herr KA, Clark CR, et al. Relationships among pain intensity, pain-related distress, and psychological distress in pre-surgical total knee arthroplasty patients: a secondary analysis. Psychol Health Med. (2017) 22(5):552–63. doi: 10.1080/13548506.2016.1189581
83. Wesolowicz DM, Bishop MD, Robinson ME. An examination of day-to-day and intraindividual pain variability in low back pain. Pain Med. (2021) 22(10):2263–75. doi: 10.1093/pm/pnab119
84. Okifuji A, Bradshaw DH, Donaldson GW, Turk DC. Sequential analyses of daily symptoms in women with fibromyalgia syndrome. J Pain. (2011) 12(1):84–93. doi: 10.1016/j.jpain.2010.05.003
85. Davis KD, Cheng JC. Differentiating trait pain from state pain: a window into brain mechanisms underlying how we experience and cope with pain. Pain Rep. (2019) 4(4):e735. doi: 10.1097/PR9.0000000000000735
86. Shukla R, Newton DF, Sumitomo A, Zare H, McCullumsmith R, Lewis DA, et al. Molecular characterization of depression trait and state. Mol Psychiatry. (2022) 27(2):1083–94. doi: 10.1038/s41380-021-01347-z
87. Endler NS, Kocovski NL. State and trait anxiety revisited. J Anxiety Disord. (2001) 15(3):231–45. doi: 10.1016/S0887-6185(01)00060-3
88. Linton SJ, Shaw WS. Impact of psychological factors in the experience of pain. Phys Ther. (2011) 91(5):700–11. doi: 10.2522/ptj.20100330
89. De Ridder D, Adhia D, Vanneste S. The anatomy of pain and suffering in the brain and its clinical implications. Neurosci Biobehav Rev. (2021) 130:125–46. doi: 10.1016/j.neubiorev.2021.08.013
90. Eccleston C, Crombez G. Worry and chronic pain: a misdirected problem solving model. Pain. (2007) 132(3):233–6. doi: 10.1016/j.pain.2007.09.014
91. Zhu Z, Galatzer-Levy IR, Bonanno GA. Heterogeneous depression responses to chronic pain onset among middle-aged adults: a prospective study. Psychiatry Res. (2014) 217(1–2):60–6. doi: 10.1016/j.psychres.2014.03.004
92. Leino-Arjas P, Rajaleid K, Mekuria G, Nummi T, Virtanen P, Hammarstrom A. Trajectories of musculoskeletal pain from adolescence to middle age: the role of early depressive symptoms, a 27-year follow-up of the northern Swedish cohort. Pain. (2018) 159(1):67–74. doi: 10.1097/j.pain.0000000000001065
93. Schneider S, Junghaenel DU, Keefe FJ, Schwartz JE, Stone AA, Broderick JE. Individual differences in the day-to-day variability of pain, fatigue, and well-being in patients with rheumatic disease: associations with psychological variables. Pain. (2012) 153(4):813–22. doi: 10.1016/j.pain.2012.01.001
94. Page MG, Gauvin L, Sylvestre MP, Nitulescu R, Dyachenko A, Choiniere M. An ecological momentary assessment study of pain intensity variability: ascertaining extent, predictors, and associations with quality of life, interference and health care utilization among individuals living with chronic low back pain. J Pain. (2022) 23(7):1151–66. doi: 10.1016/j.jpain.2022.01.001
95. Bannon S, Greenberg J, Mace RA, Locascio JJ, Vranceanu AM. The role of social isolation in physical and emotional outcomes among patients with chronic pain. Gen Hosp Psychiatry. (2021) 69:50–4. doi: 10.1016/j.genhosppsych.2021.01.009
96. Svanberg M, Stalnacke BM, Enthoven P, Brodda-Jansen G, Gerdle B, Boersma K. Impact of emotional distress and pain-related fear on patients with chronic pain: subgroup analysis of patients referred to multimodal rehabilitation. J Rehabil Med. (2017) 49(4):354–61. doi: 10.2340/16501977-2212
97. Wurm M, Edlund S, Tillfors M, Boersma K. Characteristics and consequences of the co-occurrence between social anxiety and pain-related fear in chronic pain patients receiving multimodal pain rehabilitation treatment. Scand J Pain. (2016) 12:45–52. doi: 10.1016/j.sjpain.2016.03.006
98. Westman AE, Boersma K, Leppert J, Linton SJ. Fear-avoidance beliefs, catastrophizing, and distress: a longitudinal subgroup analysis on patients with musculoskeletal pain. Clin J Pain. (2011) 27(7):567–77. doi: 10.1097/AJP.0b013e318219ab6c
99. Huijnen IP, Rusu AC, Scholich S, Meloto CB, Diatchenko L. Subgrouping of low back pain patients for targeting treatments: evidence from genetic, psychological, and activity-related behavioral approaches. Clin J Pain. (2015) 31(2):123–32. doi: 10.1097/AJP.0000000000000100
100. Flink IK, Boersma K, Linton SJ. Changes in catastrophizing and depressed mood during and after early cognitive behaviorally oriented interventions for pain. Cogn Behav Ther. (2014) 43(4):332–41. doi: 10.1080/16506073.2014.940605
101. de Rooij A, van der Leeden M, Roorda LD, Steultjens MP, Dekker J. Predictors of outcome of multidisciplinary treatment in chronic widespread pain: an observational study. BMC Musculoskelet Disord. (2013) 14(1):133. doi: 10.1186/1471-2474-14-133
102. Rovner GS, Sunnerhagen KS, Bjorkdahl A, Gerdle B, Borsbo B, Johansson F, et al. Chronic pain and sex-differences; women accept and move, while men feel blue. PLoS One. (2017) 12(4):e0175737. doi: 10.1371/journal.pone.0175737
Keywords: anxiety, chronic pain, depression, distress, interference, life control, pain intensity, social support
Citation: Gerdle B, Dragioti E, Rivano Fischer M and Ringqvist Å (2023) Pain intensity and psychological distress show different associations with interference and lack of life control: A clinical registry-based cohort study of >40,000 chronic pain patients from SQRP. Front. Pain Res. 4:1093002. doi: 10.3389/fpain.2023.1093002
Received: 8 November 2022; Accepted: 14 February 2023;
Published: 2 March 2023.
Edited by:
Ling Cao, University of New England, United StatesReviewed by:
Carolina Beraldo Meloto, McGill University, CanadaEleni Hapidou, McMaster University, Canada
© 2023 Gerdle, Dragioti, Rivano Fischer and Ringqvist. This is an open-access article distributed under the terms of the Creative Commons Attribution License (CC BY). The use, distribution or reproduction in other forums is permitted, provided the original author(s) and the copyright owner(s) are credited and that the original publication in this journal is cited, in accordance with accepted academic practice. No use, distribution or reproduction is permitted which does not comply with these terms.
*Correspondence: Björn Gerdle Ympvcm4uZ2VyZGxlQGxpdS5zZQ==
†These authors share last authorship
Specialty Section: This article was submitted to Clinical Trials, Methods, and Evidence Synthesis, a section of the journal Frontiers in Pain Research